DOI:
10.1039/D4LC00563E
(Critical Review)
Lab Chip, 2025, Advance Article
Challenges in blood fractionation for cancer liquid biopsy: how can microfluidics assist?†
Received
3rd July 2024
, Accepted 14th November 2024
First published on 8th January 2025
Abstract
Liquid biopsy provides a minimally invasive approach to characterise the molecular and phenotypic characteristics of a patient's individual tumour by detecting evidence of cancerous change in readily available body fluids, usually the blood. When applied at multiple points during the disease journey, it can be used to monitor a patient's response to treatment and to personalise clinical management based on changes in disease burden and molecular findings. Traditional liquid biopsy approaches such as quantitative PCR, have tended to look at only a few biomarkers, and are aimed at early detection of disease or disease relapse using predefined markers. With advances in the next generation sequencing (NGS) and single-cell genomics, simultaneous analysis of both circulating tumour DNA (ctDNA) and circulating tumour cells (CTCs) is now a real possibility. To realise this, however, we need to overcome issues with current blood collection and fractionation processes. These include overcoming the need to add a preservative to the collection tube or the need to rapidly send blood tubes to a centralised processing lab with the infrastructure required to fractionate and process the blood samples. This review focuses on outlining the current state of liquid biopsy and how microfluidic blood fractionation tools can be used in cancer liquid biopsy. We describe microfluidic devices that can separate plasma for ctDNA analysis, and devices that are important in isolating the cellular component(s) in liquid biopsy, i.e., individual CTCs and CTC clusters. To facilitate a better understanding of these devices, we propose a new categorisation system based on how these devices operate. The three categories being 1) solid Interaction devices, 2) fluid Interaction devices and 3) external force/active devices. Finally, we conclude that whilst some assays and some cancers are well suited to current microfluidic techniques, new tools are necessary to support broader, clinically relevant multiomic workflows in cancer liquid biopsy.
1. Importance of liquid biopsy
Liquid biopsy provides a unique opportunity to characterise individual cancers and to understand a patient's unique disease and response to treatment. By identifying cells and/or cell products that are associated with cancer in the blood of patients, it is possible to detect the presence of even low disease burden without the need to biopsy the tumour or to anatomically locate the metastatic lesions. Although originally designed to allow detection of cancer, the role of liquid biopsy has expanded and now has application in altering treatment plans based on the patient's own molecular profile. For this reason, it is set to become an important test in the application of precision genomic medicine.
Liquid biopsy has traditionally targeted 3 main biological targets. These include: 1) protein biomarkers 2) circulating tumour DNA (ctDNA) and 3) circulating tumour cells (CTCs). In addition, small extracellular vesicles, CTC clusters and lipids are becoming important targets for liquid biopsy. More details on the common liquid biopsy analytes can be found in Box 1. Whilst these analytes can be found in various body fluids (including cerebrospinal fluid (CSF), ascites, saliva, urine, etc.) liquid biopsy traditionally relies on detecting these targets in peripheral blood. This reliance likely stems from the fact that the peripheral blood system is readily available, can be assessed with minimal risk to the patient, and blood draws are a common procedure routinely done without the need for surgical intervention (unlike sampling of CSF, bone marrow, and ascites which are more invasive and less common routes for liquid biopsy).
Box 1 Common analytes in cancer related liquid biopsy
Protein biomarkers such as PSA1 and a host of serum antigens2 formed the base of some of the earliest liquid biopsy approaches in cancer. Many of these proteins are not solely expressed by cancer, and thus additional confirmation tests are required to confirm a cancer diagnosis.
Circulating tumour DNA (ctDNA) is a subset of cell free DNA that contains cancer specific mutational changes. Since at least 1996, ctDNA profiles have been identified in the blood of patients with cancer.3,4 The identification of mutational change in ctDNA is commonly used to detect disease, identify the molecular subtype of disease and studies have indicated that changes in ctDNA levels may be prognostic of outcome.5,6 As ctDNA potentially originates from any tumour cell, it can be thought of as a measure of total tumour burden.
Circulating tumour cells (CTCs) are cancer cells that can be found in the circulation. For solid tumours they are a subset of disseminated tumour cells (DTCs) that have found their way out of the confines of either the primary or metastatic lesion but have not yet completed the process of metastatic spread. As CTCs are the primary route by which cancer spreads, they can be thought of as measure of potential metastasis.
Small extracellular vesicles are small lipid particles 30–150 nm7 which are thought to carry key cell signalling messages. Whilst beyond the scope of this review, more details relating to microfluidic approaches to small extracellular vesicle capture can be readily found.8–10
CTC clusters consist of one or more CTCs and may form clusters with either other CTCs or “normal” cells and are increasingly being recognised as important players in the metastatic cascade.11–14
Lipids form a diverse range of biomolecules including fats, phospholipids and steroids. They have been implicated in early diagnosis and monitoring of cancer in a number of studies.15,16
|
While early liquid biopsy tended to focus on detection of a single marker or basic CTC enumeration, the field of liquid biopsy is now able to shed light on many important clinical questions. Modern liquid biopsy has important application in the early detection of disease,17–19 assessing cancer heterogeneity,20,21 and determining molecular drivers of disease.22 When applied longitudinally, it can help determine relapse, monitor resistance to therapy,23,24 and assess clonal evolution.24–26 Moreover, as liquid biopsy can readily be repeated throughout the patient's disease journey, it can be used to dynamically inform treatment options.27–29
2. Next generation liquid biopsy
With the advent of affordable low input next-generation sequencing (NGS), it became possible to not only detect disease, but to sequence the underlying genetic abnormalities seen in a cancer patient by interogating at cell free DNA (cfDNA) in the plasma. This meant the ctDNA could either be interrogated agnostically by analysing the entire genomic profile using whole genome sequencing (WGS)30 or specifically through the use of targeted NGS panels. These panels can either be designed to include genetic variants common across a number of patients or constructed against the unique molecular profile of the individual patient to deeply sequence a smaller number of high value gene targets.31
WGS approaches were initially applied at relatively low sequencing depth. Sequencing depths as low as ∼0.4× or 0.6× genome coverage have been shown to be able to detect disease.32,33 However, by sequencing further (6× to 8× genome coverage), it is possible to look at the tissue of origin for the cancer in a process involving fragmentomics (see reviews34,35). These approaches are unlikely to capture every individual mutation. Therefore, low coverage WGS approaches are more suitable for tumours which tend to have a high tumour mutation burden (TMB) (as more potential targets exist) or in diseases characterised by copy number aberrations (CNAs). For tumours not characterised by CNAs or high TMBs, low pass WGS has limited application, and it can be useful to apply targeted panels. Targeted panels come in two forms: pan-cancer panels and personalised panels. Pan-cancer panels, such as the Trusight Oncology 500 ctDNA from Illumina or the Xgen Pan-Cancer hybridisation panel from IDT, are useful as they cover many of the commonly mutated cancer genes. In cancer types with well conserved genetic changes across many patients, it is possible to design cohort specific panels, such as the UltraSEEK lung Panel. These panels focus on reducing the number of probes required without the additional cost of sequencing regions unlikely to be mutated. When a disease cohort is highly heterogeneous and where genetic mutations are not shared amongst multiple patients, two approaches can be enacted to enable sufficient detection sensitivity. The first approach requires a large pool of targets, such that can be achieved using whole exome sequencing or with very large capture/amplicon panels, whilst the second approach relies on knowing which targets are present in the patient's own tumour. This approach is truly personalised and whilst it reduces liquid biopsy sequencing costs, it requires the patient's tumour to be sequenced in advance.
Larger, multiple target panels, such as those identified using the INVAR approach,31 work as they increase the chance of detecting mutations by including more detection options, while personalised panels work by heavily preselecting targets that have an increased likelihood of being found in the patient's own tumour. The issue with large panels is primarily around cost while the problem with personalised panels is the need to have a priori knowledge of the individual's cancer genome. Studies such as the TRACER X,36 PROPHET,37 and others38–40 are excellent examples of where personalised ctDNA panels have been effectively applied.
Just as we have seen advances in NGS that have improved cancer liquid biopsy, advancements in cytometry and single-cell genomics analysis have exponentially increased the number of targets that can be simultaneously analysed at the single cell level.41–43 This is important because, although detection of CTCs above a clinically relevant level (e.g. >5 CTCs ml−1 in breast cancer) generally indicates poorer outcomes,44 it does not provide certainty of disease outcome.45 This finding suggests that metrics other than simple enumeration could provide more accurate prognosis. By taking advantage of the improvements in sensitivity, reduction in cost, and the capability to perform simultaneous multiomic analysis, the field of single cell sequencing and genomic cytometry is now making it possible to characterise cells in terms of DNA i.e. (single nucleotide variants (SNV),27,46 copy number aberrations (CNA)23,47), RNA expression levels,20 and epigenetic changes48–50 of individual cells, including CTCs.51,52 The ability to genomically profile CTCs is important, as by knowing both CTC burden and the molecular profile of the cells that may set up distant metastatic lesions, we will be better equipped to not only escalate or de-escalate treatment but also alter treatment in response to the detection of therapeutic resistance.
Whilst independently assessing ctDNA and CTC profiles in cancer has been powerful, multimodal profiling is promising to allow the simultaneous profiling of both the ctDNA and the CTCs from a single blood draw. If this can be effectively implemented, it will allow a more detailed picture of a patient's individual disease in the most minimally invasive manner and provide both a total tumour burden measure (from ctDNA) and the ability to look for active evidence of metastatic spread (CTCs). As cancer is a complex disease, the rationale is that the more information that can be garnered from a single blood draw, the better we can assess and treat the patient.
3. Challenges in blood fractionation and cancer liquid biopsy
The first step in any liquid biopsy assay is to separate assay targets (such as ctDNA and CTCs) from the complex mixture in which they are found. In the case of ctDNA, the first step is to obtain pure cell-free plasma, while for CTCs, the first step is to debulk the cellular fraction without losing rare CTCs.
Blood is a complex mixture and contains two main components used in cancer liquid biopsy. These are the plasma and the cellular layer (buffy coat). Cell Free DNA (cfDNA), which contains ctDNA is found within the plasma fraction and can be subjected to assays such as next generation sequencing and quantitative PCR. The buffy coat contains the nucleated cells, including the CTCs, CTC cluster and immune cells. This layer can be subjected to analysis via bulk NGS, flow cytometry and fluorescent activated cell sorting (FACS), single cell genomics and imaging. A schematic of technologies related to the analysis of these blood fractions is shown in Fig. 1A. Fig. 1B shows the types of information that can be gathered through further analysis of these fractions. Analysis of the cfDNA in the plasma allows the detection of genomic alterations such as single nucleotide polymorphisms, copy number aberrations, structural variants, and can even be analysed using fragmentomics to identify the tissue of origin for the tumour. Using cytometric approaches on the cell layer, it is possible to enumerate, visualise, and genomically characterise CTCs and other cell types. Importantly, it is possible to quantify the cellular co-expression of RNA, protein, DNA mutations at the level of the single cell, and to perform cell type composition analysis and to track clonal expansion. By analysing the blood of cancer patients, it is possible to 1) quantify levels of disease, 2) identify tissue of origin (see review of cell-free DNA fragmentomics53) and 3) isolate the molecular mechanism responsible for disease and particularly therapeutic resistance. This can be used to aid in identifying treatment options that are most likely to address the molecular alterations identified in the patient's individual cancer.
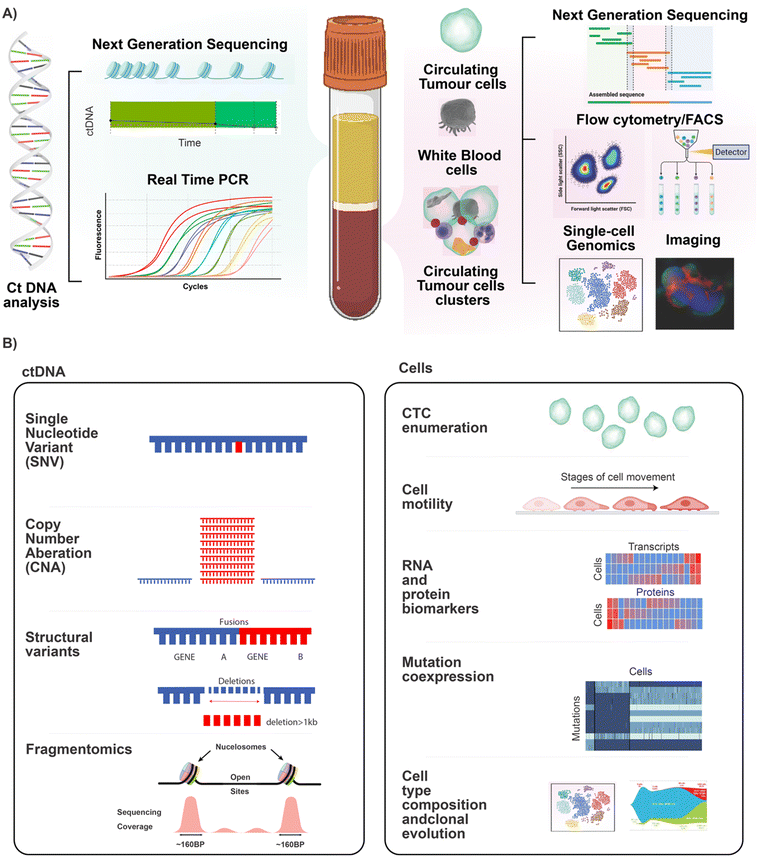 |
| Fig. 1 Key components of the peripheral blood and how they are leveraged into cancer liquid biopsy applications. A) Shows the components found within the blood that can be used in cancer liquid biopsy. On the left-hand side is the ctDNA which is found in the plasma and can be analysed using molecular approaches such as next generation sequencing and single target quantitative PCR. The right panel shows the cellular evidence for cancer, this includes CTCs, CTC clusters, and immune cells. These can be analysed using bulk genomic approaches, flow cytometry for analysis or FACS for associated cell sorting, and genomic cytometry/single cell genomics and imaging. B) Highlights the biologically relevant information that can be retrieved via characterisation of ctDNA and CTCs. Left panel: depending on the methods employed to analyse ctDNA it is possible to look at SNV and CNA profiles as well as structural variants. In addition, emerging approaches such as fragmentomics are allowing us to deconvolute the ctDNA to look at tissue of origin and other key metrics. Right panel: By using traditional cytometric approaches alongside single-cell genomics it is now possible to perform CTC enumeration, analyse CTC motility, perform deep phenotypic profiling (using RNA and oligo antibody approaches in scRNASeq), and look for mutational profiles in the single cells (via single-cell DNA sequencing). By using these molecular profiling techniques, it is also possible to perform detailed cell type profiling and to track clonal evolution. | |
3.1. ctDNA
Early work showing that cell-free DNA levels tend to be elevated in cancer patients was published in 1977.54 Isolated ctDNA has been used to detect known mutations,55 fusions,56 copy number variations57 and epigenetic profiles.58,59 It has also been used to measure tumour burden in cancers including lung,24,37,57 melanoma,17 breast,26,60 neuroblastoma,61 pancreas,18 prostate,62,63 colon,64 and colorectal.5,38
ctDNA fragments are found in the plasma fraction of the blood and are small DNA nucleic acid molecules around 160 base pairs in length. They are found alongside other cell-free DNA molecules that do not originate from the tumour and are often very rare. Therefore, there is a significant challenge in obtaining sufficient volume of plasma and cell-free DNA to reliably detect cancer at low levels.65 As such it is critical to develop systems that provide high purity plasma with excellent recovery and can do this without allowing cellular breakdown and compromising the quality of the resulting plasma.
The challenges when dealing with ctDNA include:
1. Cell-free DNA (cfDNA) is found in both healthy individuals and those with cancer and whilst there is some evidence to suggest that patients with cancer may have elevated levels of cfDNA,66 quantification of cfDNA alone is generally considered as having low diagnostic value.54 Therefore, simple measurements of cfDNA are not sufficient, even for detection of cancer.
2. Clinically relevant ctDNA levels can be low (<1% of all cfDNA molecules in plasma). It is therefore important that all potential ctDNA molecules are captured.
3. ctDNA can be readily contaminated by cellular DNA via the introduction of high molecular weight genomic DNA contamination from leukocyte lysis.67,68 Therefore, samples must be processed quickly, or preservatives must be introduced to prevent WBC lysis.
4. To confirm if an individual has cancer, the cfDNA must be profiled and cfDNA containing mutations associated with cancer must be identified.69 Therefore, highly efficient molecular pipelines are required.
3.2. CTCs
The existence of CTCs has been known since 1869 when Thomas Ashworth first described the existence of tumour-like cells in the blood of a patient.70 Since then, many studies, mainly concerning epithelial cancers such as breast,44 colorectal,64 prostate,46 kidney,71 and lung72 have highlighted the importance of CTCs. With the advent of new cell surface biomarkers and label free methods, the link between CTC levels and prognosis is becoming clearer for non-epithelial cancers. This includes brain cancer,73 neuroblastoma,74 and sarcoma.75
CTCs are important as they are the route through which cancer spreads and can provide a valuable window into a patient's individual disease, may help to assess tumour heterogeneity,76,77 and overcome sampling issues associated with solid biopsies that may result in missing mutations associated with metastasis.46 Clinically, CTC detection can allow earlier detection of disease when compared to imaging techniques,78 and levels of CTCs can be prognostic of disease outcome.79,80 In addition, there is strong evidence that CTCs can be used to track clonal evolution and dynamic heterogeneity, especially from haematological malignancy where CTC numbers can be high.
CTCs reside in the cellular component of the blood and are vastly outnumbered by red blood cells (RBCs). RBCs form the majority of the blood's cellular compartment81 and are biconcave disks of 7.5 to 8.7 μm in diameter and are 1.7 to 2.2 μm thick.82 Removal of the RBCs can be achieved with very small filters.83 The requirement for pore sizes significantly smaller than the size of the RBC is due to the high deformability of RBCs which allows them to squeeze through passages smaller than their actual physical size.82 In addition, devices designed to be used with whole blood must be capable of dealing with the very high concentrations of cells found in blood. This is a significant challenge as blood is normally composed of around 40–45% haematocrit, which translates to a cellular concentration comprising 4.8 to 5.31 billion (109) red blood cells (RBCs), 5.8 to 7.44 million (106) white blood cells (WBCs) and 230 to 285 million (106) platelets per ml.81
The challenges when dealing with CTCs include:
1. CTCs are exceedingly rare cells with as little as 5 CTCs per ml of blood being clinically significant.44 Therefore, every cell counts.
2. In addition to being rare, CTCs are generally overwhelmed (>1 billion to one), by normal blood cells. Therefore, systems must be capable of sorting large numbers of cells.
3. There is significant variation in CTC numbers based on disease type and stage. Estimates of CTC numbers in patients vary from zero to ∼25
000 in 7.5 ml of blood.84 Therefore, systems of capture must be able to handle anywhere from one CTC to several thousands of cells.
4. CTCs have a short half-life.85,86 Therefore, CTC analysis should be performed as soon as possible after collection.
5. CTCs may be found as either individual cancer cells or as cell clusters, in which case they are known as CTC clusters. As CTC clusters can be composed of 2 or many cells, the system must be able to handle clusters of highly variable sizes.
6. In addition to forming clusters with other CTCs, CTCs may cluster with other cell types, including WBCs (heterotypic). Therefore, capture systems that rely on the depletion of WBCs (i.e., through immunoaffinity methods) may deplete some CTC clusters.
7. The emerging acceptance of cancer-associated cells in the blood is also driving changes in the way blood needs to be processed for liquid biopsy. Immune profiling as part of liquid biopsy means that WBCs are no longer a waste product in liquid biopsy. Therefore, systems of capture must not create biases in the WBC populations.
8. CTC based separation overwhelmingly relies on the assumption that these cells either uniquely express a single cell surface marker or they are larger in size when compared to other cells. EMT transformation, the increasing recognition of CTC heterogeneity, and evidence pointing to the existence of small CTCs mean that existing methods may not capture all CTCs. Therefore, systems that use multiple CTC attributes may be more efficient at capturing all CTCs.
3.3. Conventional approaches to blood fractionation
Traditional blood fractionation approaches have generally relied on separation through either density or filtration-based discrimination. By centrifuging blood containing an anticoagulant at high speeds, a density gradient forms, and the blood separates into three distinct compartments. These fractions are: 1) the lighter plasma fraction, 2) the buffy coat cell fraction, and 3) the bottom RBC fraction. The drawback with the centrifugal-based approaches is that the interface between the layers can be difficult to accurately define and the choice of where to apply the cut off between the layers is left entirely to the operator.
With regards to plasma fractionation, inaccurate determination of the plasma and the cell layers can have the effect of both losing plasma (when not enough plasma is taken) or risk contamination of the cfDNA fraction (when part of the cellular layer is accidentally transferred with the plasma fraction and dying cells release high molecular weight DNA87). Although these issues can be minimised with operator training or with automation, this requires the development of expertise or the acquisition of expensive and bulky liquid handling robotics and is generally not achievable outside of specialist labs.
To assist with separating the buffy coat cells from the RBC layer, density gradient media, such as Lymphoprep, Ficoll, Percoll®, and Histopaque®, have been developed. The use of density media can be further enhanced through specially designed tubes, such as SepMate™, PluriMate, leucosep, which are designed to help prevent the layers from mixing. Products, such as RosetteSep™ and HetaSep™, also incorporate the capacity to deplete unwanted cells. However, in cancer applications, all these methods are dependent on the target CTC's having a known density profile and only separate the major blood fractions. As such CTCs are still heavily contaminated with either RBCs, WBCs, or a combination of both and require further processing. Such methods usually include:
• RBC lysis is usually achieved using ammonium chloride. However, buoyancy (Akadeum human RBC depletion microbubbles) and microfluidic methods have also been developed.88
• Magnetic activated cell sorting (MACS).89
• Fluorescent activated cell sorting (FACS).90,91
Analysis of both ctDNA and CTCs to produce clinically relevant information is a non-trivial process. It requires close attention, not only to the specifics of the chosen analysis method but also to a host of pre-analytical variables including the way the sample is prepared.87,92–94 Time is one of the major pre-analytical factors that can affect the results of liquid biopsy assays. This is related to the short half-lives of both ctDNA and CTCs and the fact that when non-cancer cells break down in the tube they will release normal DNA that can dilute the ctDNA signal. To minimise this, several blood collection tubes (BCTs), containing mostly proprietary preservatives have been developed and commercialised by companies such as Streck, Roche, ZYMO and Becton Dickinson. These tubes can be used to store blood at room temperature and allow up to 5 days between collection and processing without compromising cfDNA integrity.68,95 However, the biggest challenge in using these BCTs in deep characterisation workflows lies in the fact that they significantly impact the quality of RNA for downstream applications and should only be used when ctDNA is the target.96 Our own findings also show that these tubes significantly compromise the cytometric profiles. As such, it is currently recommended that multimodal analysis of ctDNA and CTC still relies on blood being collected into different BCTs.95,97
Processing blood samples for liquid biopsy using conventional approaches is currently far from routine and samples are often shipped to specialised laboratories for processing where automation and specialist wet lab staff are trained to process samples. An alternative approach to the use of BCTs containing preservatives and centralised processing is to use microfluidic blood fractionation at the point-of-draw. Due to the rarity of CTCs and the low abundance of ctDNA, an array of microfluidic approaches have been developed. This review focuses on microfluidics devices that facilitate liquid biopsy for ctDNA and CTCs, how they work, where they fall short, and what would be required to advance genomic profiling of disease through minimally invasive blood tests.
4. Overcoming challenges, a role for microfluidics
Microfluidics is well suited as an approach to improve blood fractionation for cancer liquid biopsy. This is because it: 1) allows accurate manipulation of small sample volumes (especially important in paediatric liquid biopsy), 2) comes with low infrastructure costs (important for uptake in an already stretched healthcare system), 3) imparts minimal stress on the cells (minimal stress results in higher viability), 4) can be used without the need for complex labelling/handling protocols, and 5) are generally closed systems (making them low loss and safe to operate as no aerosols are created). Over the years, several different microfluidic approaches for fractionating blood into its various components have been developed and have previously been reviewed, particularly for CTCs.98–100 However, there is still no consensus about which approach is best applied for which biological context, especially in the context of multiomic liquid biopsy.
Microfluidic plasma separation is commonly achieved via several methods, including 1) physical filtration, 2) plasma skimming via the Fareheus effect, 3) bifurcation or the Zweifach–Fung effect, 4) deterministic lateral displacement (DLD) (requires significant dilution of blood), 5) inertial focusing (requires significant dilution of blood), and 6) acoustophoresis. A description of the microfluidic devices available, their strengths, weakness and their working mechanism is provided in Table 1 (plasma separation).
Table 1 Overview of microfluidic devices for plasma separation
Device name |
Target fraction |
Year |
Category |
Mechanism and separation characteristic |
Efficiency and purity |
Speed or max volume |
Chip complexity (1 is simple, 5 is complex) |
Pre-processing and running buffers required |
Reference |
Planar microfilters |
Plasma |
2005 |
SI |
Filtration |
N/A |
|
4 |
|
101 |
Size |
N/A |
DLD–PD |
Plasma |
2006 |
SI |
DLD |
100% |
0.4 μl min−1 |
3 |
No |
102 |
Size |
|
Yes |
Zweifach–Fung |
Plasma |
2006 |
FI |
Zweifach–Fung |
15–25% |
10 μl min−1 |
4 |
N/A |
103 |
Size |
|
No |
|
Plasma |
2016 |
FI |
Zweifach–Fung |
1–6% yield |
0.5 ml min−1 |
2 |
No |
104 |
Size |
Residual contamination is 20 000 RBCs μL−1 and ∼80 WBCs μL−1 |
No |
|
Plasma |
2009 |
EF |
Acoustophoresis |
RBC contamination of 3.65 × 10 ^9 per litre |
80 μl min−1 |
4 |
No |
105 |
Bead packed microchannels |
Plasma |
2010 |
SI |
Filtration |
|
20 μl of whole blood |
1 |
|
106 |
Size |
Contraction expansion arrays |
Plasma |
2011 |
FI |
Inertial |
62% yield |
1.2 ml h−1 |
1 |
10× dilution |
107 |
Size |
60% RBC rejection ratio |
Yes, running buffer required |
Capillary driven, dielectrophoresis enabled microfluidic system |
Plasma |
2015 |
SI + EF |
Filtration + dielectrophoresis |
|
165 nl of plasma extracted in 15 min |
2 |
N/A |
108 |
Size |
No |
|
Plasma |
2016 |
EF |
Electrothermal flow |
|
50 μl h−1 |
5 |
10× dilution |
109 |
All-glass bifurcation microfluidic chip |
Plasma |
2017 |
FI |
Bifurcation |
74% (@25% haematocrit) |
700 μl min−1 |
2 |
2× dilution |
110 |
Size |
No |
Fully automated, on-site isolation of cfDNA |
Plasma |
2018 |
EF (LOD) |
Centrifugation |
Not disclosed |
3 ml in ∼30 min to produce cfDNA |
5 but simple to run |
As this device is designed for automated cfDNA extraction many reagents are required |
111 |
Buoyancy/density |
|
Plasma |
2020 |
SI |
Filtration |
71.7% yield @45% haematocrit |
60 μl in 6 min |
3 |
No |
112 |
Size |
RBC deletion of 99.8% and WBC depletion of 91.8% |
No |
|
Plasma |
2022 |
SI |
Filtration |
50.3% to 88.5% of max plasma volume |
50 μl blood in 2 min |
3 |
No |
113 |
Size |
Not disclosed |
No |
|
Plasma |
2010 |
FI |
Size |
Up to 17.8% yields |
100 μl min−1 |
3 |
1 : 20 dilution |
114 |
No |
|
Plasma + WBC capture |
2018 |
SI |
Filtration + affinity capture |
|
6 μl whole blood at 0.3 μl min−1 |
4 |
Yes, collected plasma is diluted at 0.76× |
115 |
Size + epitope expression |
CTC separation by microfluidics can be achieved with either passive or active methods, these commonly include 1) physical capture, 2) affinity surface capture, 3) deterministic lateral displacement (DLD), 4) acoustophoresis, 5) microfluidic magnetic associated cell separation (mMACS), 6) intertial separation and 7) microfluidic fluorescent activated cell sorting (mFACS). A description of the microfluidic devices available, their strengths, weaknesses, and their working mechanism is provided in Table 2 (CTC separation).
Table 2 Overview of microfluidic devices for CTC separation
Device name |
Target fraction |
Year |
Category |
Mechanism and separation characteristic |
Efficiency and purity |
Sample speed or max volume |
Chip complexity (1 is simple, 5 is complex) |
Pre-processing and running buffers required |
Reference |
Integrated inertial ferrohydrodynamic cell separation (i2FCS) |
CTC |
2021 |
FI + EF |
Inertial + macs |
99.7% |
60 ml h−1 (2 × 105 cells per s−1) |
4 |
Cell staining and RBC lysis |
116 |
Size and epitope expression (CD4/16/66b/3 depletion) |
4.07-log depletion, removing 99.992% of leukocytes |
Sample resuspended in ferrofluid and pluronic F-68 surfactant |
Cluster-wells |
CTC clusters |
2022 |
SI |
Filtration |
LNCAP: 100% 6 or more cells, ∼98.7% of 5-cell clusters, dropping to ∼75.8% of LNCAP doublets |
25 ml h−1 |
4 but simple to run |
No |
117 |
Cell clusters |
<0.06% WBC <0.025% platelet contamination |
No |
Continuous centrifugal microfluidics (CCM) |
ctDNA and CTC |
2022 |
EF (LOD) |
Centrifugal + MACS |
∼80% depending on cell line |
5.4 ml whole blood |
5 but simple to run |
Pre-filling of density gradient media and antiCD45 MACS beads required |
118 |
CD45 depletion |
WBCs depletion > 99% |
Spiral-contraction-expansion channel |
CTC and CTC clusters |
2024 |
FI |
Inertial |
97% MDA-MB-231 and 90% CTC clusters |
3.5 ml min−1 |
4 |
Tested on pleural and abdominal effusions |
119 |
Size |
WBC contamination is 31% in CTCs and 87% CTC clusters |
No |
Single-looped spiral |
Large particle |
2023 |
FI |
Inertial |
Numerical simulation only |
N/A |
2 |
|
120 |
Size |
Yes |
U-turn spiral |
Large particle |
2023 |
FI |
Inertial |
>90% of larger particles |
1.7 ml min−1 |
2 |
|
121 |
Size |
|
Yes |
Cascaded spiral |
CTC |
2023 |
FI + SI |
Inertial + filtration |
>85% for SGC-7901 cells, A549 cells and LoVo cells |
300 μl min−1 |
2 |
No |
122 |
Size |
>90% in rabbit blood |
No |
N/A |
CTC |
2014 |
EF |
mMACS |
Depends on antigen chosen and expression levels on cells of interest |
2 to 40 ml h−1 |
3 |
Cell staining |
123 |
EPCAM + others |
<∼200 non target cells captured |
Washing buffers |
Isoflux |
CTC |
|
EF |
MACS |
|
|
|
Cell staining |
Fluxion bioscience |
Parsortix |
CTC + CTC clusters |
2015 and 2018 |
SI |
Filtration |
54.4% PC, 57.3% DU145 and 54.7 to 78% MCF7 (study dependant) |
∼5 ml h−1 |
2 |
Yes 1 : 1 dilution |
124, 125 |
Size + compressibility |
Purity of PC3 in blood is 4.6% |
Slanted spiral |
CTC |
2014 |
FI |
Inertial |
80% T24, 85% MCF7, 87% MDA-MB-231 |
1.7 ml min−1 |
2 |
RBC lysis required. |
126 |
Size |
4 log WBC depletion |
No |
CROSS chip |
CTC |
2019 |
SI |
Filtration |
70% with SW480 cell lines |
80 μl min−1 and <4 ml whole blood per chip (4 sections) |
2 |
No |
127 |
Size |
99.99% depletion of WBC |
No |
Celsee (now BioRad genesis) |
CTC |
2014 and 2016 |
SI |
Filtration |
81% MCF7 and 83% for MDA-MB-231 |
∼1 ml min−1 |
4 |
Fixation prior followed by 2× dilution |
128, 129 |
Size |
|
Washing buffers |
Vortex |
CTC |
2014 |
FI |
Inertial |
27% to 42% at 40× blood dilution |
4 ml min−1 |
2 |
20× dilution |
130 |
Size |
Purity of 57–94% |
Washing buffers |
Oncobean |
CTC |
2014 |
SI |
Affinity capture |
|
1 to 10 ml h−1 |
2 |
No |
131 |
EpCAM |
No |
Cluster-chip |
CTC clusters |
2015 |
SI |
Filtration |
99% of MDA-MB-231 cluster >4 cells |
2.5 ml h−1 |
3 |
No |
132 |
Shape |
— |
NanoVelcro CTC chip |
CTC |
2013 |
SI |
Affinity capture |
80–95% with cell lines |
0.5 ml h−1 |
3 |
Cell staining |
133 |
EpCAM |
— |
Washing buffers |
ieSCI-chip |
CTC |
2022 |
FI + SI |
Inertial + filtration |
87 to 98.5% with cell lines |
1.4 ml min−1 |
5-single cell immunoblotting included |
RBC lysis and dilution |
134 |
Size |
89% with MCF7 in isolated WBCs |
No |
MyCTC |
CTC and CTC clusters |
2022 |
SI |
Filtration |
>95% capture in cell lines BR16, Brx50 and Brx07, increased to >97% for clusters |
50 μl min−1 |
5 as it allows drug testing on the same chip |
No |
135 |
Size |
Not disclosed |
No |
DLD arrays for large and small cluster separation |
CTC clusters |
2017 |
SI |
DLD |
Large clusters ∼98%, smaller clusters ∼65% in whole blood. |
Up to 1 ml hr−1 |
4 |
Yes |
136 |
Size and asymmetry |
>5 log depletion of RBCs |
No |
N/A |
CTC |
2011 |
FI |
Inertial |
10–43% of HeLA cells |
7.5 × 106 cells per s−1 |
2 |
Yes |
137 |
Size |
Not defined |
Dilution to 1% haematocrit required |
Herringbone-chip (HB-chip) |
CTC |
2010 |
SI |
Affinity capture |
91.8% of PC3 cells at 1.2 ml h−1 (large chip) |
1.5 to 2.5 ml h−1 up to ∼4 ml |
2 |
No |
138 |
EpCAM |
>40%14% purity in cell lines spiked into WBC |
No (buffer used to flush unbound cells post capture) |
i-DLD sorter |
CTC |
2019 |
FI + SI |
Inertial + DLD |
91.34% recovery in blood |
400 μl min−1 |
4 |
No |
139 |
Size |
17.68% purity in blood |
No |
CTC-chip |
CTC |
2007 |
SI |
Affinity capture |
>60% in cell lines expressing variable levels of EpCAM |
1 to 2 ml h−1 (efficiency drops when >2.5 ml h−1) |
2 |
No |
140 |
EpCAM |
Average purity was 49% in metastatic prostate cancer to 67% in colorectal cancer |
No |
Geometrically enhanced differential immunocapture (GEDI) |
CTC |
2010 |
SI |
Affinity capture |
85% of LNCAP cells in blood |
1 ml h−1 up to 1 ml |
2 |
No |
141 |
PSMA |
62% purity |
No (buffer used to flush unbound cells post capture) |
Graphene oxide (GO) chip |
CTC |
2013 |
SI |
Affinity capture |
>87% of MCF7 in whole blood when >10 cells spiked in |
3 ml h−1 |
3 |
No |
142 |
EpCAM |
Not defined |
No |
Hydroseq |
Cells |
2019 |
SI |
Filtration |
∼70% of targets cells we successfully paired with a bead |
N/A |
>5 |
Is used for scRNASeq and requires lots of prep |
143 |
Size |
Not d |
Acoustophoresis |
Cells |
2019 |
EF |
Acoustophoresis |
60–97% recovery |
100 μl min−1 and 1 × 106 cells per ml−1 |
5 |
No |
144 |
Size |
Purity is inversely related to purity in blood |
Sorting buffer required |
N/A |
Cells |
2021 |
EF |
Affinity capture |
∼75% when tested for cMYC and EpCAM capture of PC3 cells in blood |
2 ml h−1 |
4 |
Fixation, permeabilization and cell staining |
145 |
Epitope expression |
Not defined |
No |
3d printed monolithic device |
CTC |
2019 |
SI |
Affinity capture + filtration |
∼90% recovery with HeyA8, MDA-MB-231 and LNCaP |
500 μl h−1 per layer, thus a 32-layer device can run at 16 ml h−1 |
4 |
Cell staining |
146 |
Epitope expression and size |
83% depletion of WBC in CTC fraction |
Wash buffer required |
Monolithic CTC-iChip |
CTC |
2017 |
EF + FI + SI |
DLD + inertial + MACS |
Median recovery of 99.5% across multiple cell lines |
|
5 |
Cell staining |
147 |
Epitope and size |
445 WBCs per mL of input blood |
Yes |
Cascaded filter deterministic lateral displacement (CFD) |
CTC |
2021 |
SI |
DLD |
>96% for k562 and A549 cell lines |
1 ml min−1 |
4 |
5 : 4 dilution (blood : PBS) |
148 |
Size |
WBC removal of 99.995%, RBC depletion is 100% |
Running buffer required |
Non-equilibrium inertial separation Array-XL (NISA-XL) |
CTC clusters |
2020 |
SI + FI |
Inertial + filtration |
80% final recovery |
Depends on application but >30 ml h−1 |
4 |
1 : 1 dilution |
149 |
Size |
4.2 log10 reduction in WBC |
No |
Vortex HT |
CTC |
2016 |
FI |
Inertial |
Assume similar to original vortex |
7 ml min−1 |
3 |
10× dilution |
150 |
Size |
Running and wash buffer required |
N/A |
CTC |
2009 |
SI |
Filtration |
∼80% for MCF-7, MDA-MB231 and HT-29 cell lines |
0.7 ml h−1 |
2 |
1 : 2 dilution |
151 |
Size |
Purity >85% at 5 kPa |
No |
Fluid-assisted separation technology (FAST) |
CTC |
2017 |
EF + SI (LOD) |
Filtration |
>96% for MDA-MB-231, MDA-MB-436, AGS, MCF7 and HCC78 |
>3 ml min−1 |
5 but simple to run |
None |
152 |
Size |
>2.5 log depletion of WBC |
PBS to wash |
All-in-one centrifugal microfluidic device for CTCs |
CTC |
2014 |
EF + SI (LOD) |
Centrifugation + filtration |
∼55% MCF7 in 1 ml blood |
3 ml in 20 s for filtration |
5 but simple to run |
None |
153 |
Size |
∼500 WBC per ml of blood |
Fully automated CTC isolation platform |
CTC |
2014 |
EF (LOD) |
Centrifugation |
>90% for MCF7 and HCC827 in blood |
5 ml |
5 but simple to run |
Microbeads added to blood |
154 |
Buoyancy/density/EpCAM |
<12 WBC per ml and <4.2 × 102 RBCs per ml |
No |
Multi-flow straight channel |
CTC |
2019 |
FI |
Inertial |
>93% recovery for H640 and ∼80% for HCC827 |
100 μl min−1 |
2 |
RBC lysis + dilution in PBS |
155 |
Size |
>87% purity |
Running buffer |
DFF cascaded spiral system |
CTC |
2013 |
FI |
Inertial |
85% with cell lines |
3 ml h−1 |
3 |
2× dilution |
156 |
Size |
Purity of ∼10% in clinical samples |
Running buffer |
Despite the apparent complexity of microfluidic devices available, blood fractionation is generally achieved through separations based on either size/deformability or cell surface expression of protein. The mechanism of action can be used to separate these devices into three main categories. These groupings help to understand when and where an approach can be utilised, is based on the forces used to affect separation, and whether the cells are required to come into physical contact with the device. We propose that microfluidic devices for blood fractionation can be divided into three categories based on the working mechanism. These are:
Solid interactions devices
This category relies on physical interactions to facilitate fractionation based on particle size. Whilst devices in this family can be quite distinct from one another, they all use physical interactions of the particles in blood to facilitate fractionation. As blood components can vary in size from very small, such as exosomes 30–150 nm,7 to very large CTC clusters consisting of many cells that can be up to 340 μm in head and neck cancer,157 this category of devices can be used to separate all components in the blood.
Fluid interactions devices
Microfluidic devices that separate blood into its constituent elements using fluid interactions do so through a complex mix of physical forces that can be largely explained through fluid mechanics. Commonly, these devices rely on observed effects such as the Zweifach–Fung effect, the Fareheus effect forces, and forces such as the Dean drag force as well as inertial lift forces that are leveraged in inertial devices. This category includes devices that can separate plasma or CTCs. Plasma collection is predominantly achieved via use of the Zweifach–Fung effect and the Fareheus effect forces, while inertial devices are common in CTC enrichment and have been effectively used in cancer that produce CTCs that are larger than the majority of blood cells.
External force/active microfluidics
Devices that use external forces are the most varied category and whilst forces such as electrophoresis, dielectrophoresis, acoustophoresis, and magnetophoresis are often employed, most devices can further be subdivided into one of two categories: 1) those reliant on labelling the targeted fraction with either a specific tag (often facilitated by protein-antibody interactions, or complementary DNA binding), and 2) those that operate in a label free manner. Except for acoustophoresis and lab-on-a-disk devices, devices in this category are generally focused solely on the isolation of CTCs and CTC clusters.
To help understand how microfluidic blood fractionation in cancer liquid biopsy has developed, a timeline of device development is shown in Fig. 2. For simplicity, the methods are separated into those that allow plasma fractionation and those that are focused on CTC and CTC cluster separation. Microfluidic devices for plasma fractionation are still relatively rare but those that allow CTC, and CTC cluster isolation have exploded since around 2014. The methods have been further colour coded according to whether they fall into the categories of solid interactions devices (red), fluid interactions devices (green) or external force/active devices (blue), those that utilise a combination of mechanisms are illustrated as black dots.
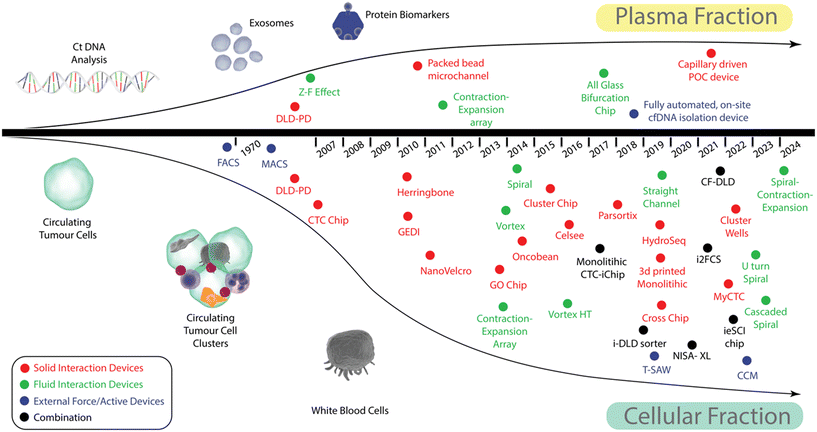 |
| Fig. 2 Timeline of tools for blood fractionation. To provide clarity, the timeline has been split to show those devices that can provide plasma or those predominantly concerned with cellular enrichment. Devices have further been colour coded according to whether they fall into the categories of solid interactions devices (red), fluid interactions devices (green) or external force/active devices (blue), those that utilise a combination of mechanisms are illustrated as black dots. Top: Shows the devices that have been used to fractionate plasma containing cfDNA from blood. Bottom: shows the devices that have been developed to allow cellular evidence of cancer to be detected in the peripheral blood draws. Devices in this category overwhelmingly focus on CTC enrichment. | |
4.1. Solid interactions devices
Microfluidic methods relying on physical interactions include: 1) physical filtration, 2) deterministic lateral displacement (DLD) and 3) affinity surface capture. The simplified representation of the working principle of devices in this category is shown in Fig. 3 while specific examples of devices are shown in Fig. S1.†
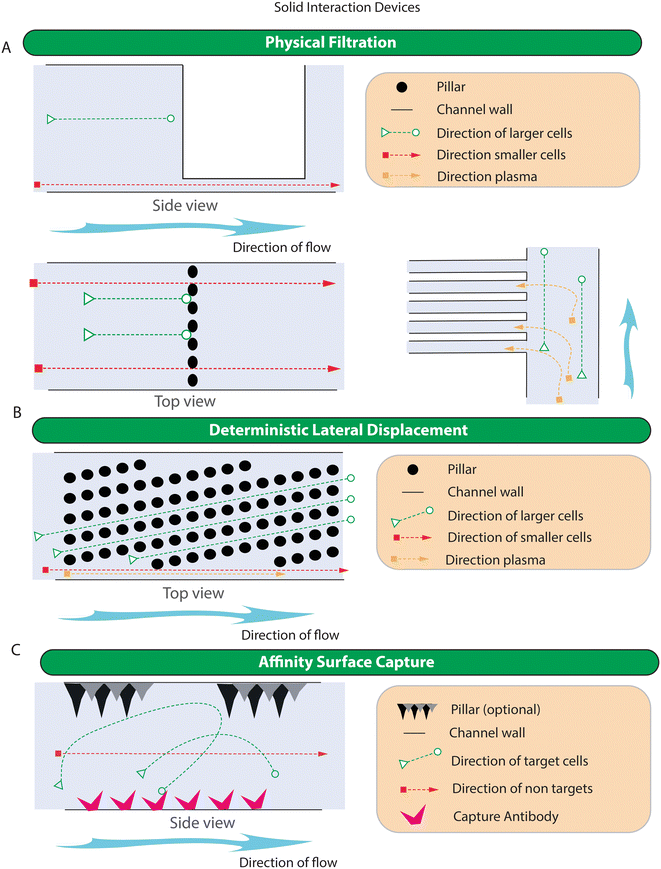 |
| Fig. 3 Schematic representation of the working principles for solid interaction microfluidic device in blood fractionation. A) Physical filtration devices use physical barriers to block the progress of cells; this can be used to block all cells entering plasma collection channels, to exclusively allow small cells such as RBCs to progress through into different parts of the chip (usually used for RBC depletion) or to capture larger CTCs in a particular region of the chip. B) Deterministic lateral displacement devices use offset pillar arrays to move cells above a defined critical diameter across the direction of flow and thus enable size-based separation. C) Affinity surface capture devices rely on the expression of cell surface proteins to enable exclusive capture of cells by tethering antibodies against specific proteins to the chip and bringing cells into contact with them. Once bound to these antibodies, the cells can no longer move in the direction of flow and are thus captured. | |
4.1.1. Physical filtration. This category includes devices that either rely on small filter pore sizes to prevent the passage of cells into the cell-free collection channels or specifically designed cell capture geometries that can capture CTCs/CTC clusters based on their larger size and other physical properties such as deformability. As a result of the extremely small pore sizes required and the overwhelming number of RBCs that need to be filtered out, designs that aim to minimise clogging have been developed. This has been achieved with 1) carefully designed filter geometries, 2) larger channels that can be packed with beads (10 μm to 20 μm diameter) to create a filter bed with a greater filter surface area than achievable with individual channels created during the process of standard lithography106,115,158 (Fig. S1A†), 3) devices that incorporate the use of commercially available filter membranes into devices with large surface areas,146,159 4) the use of cross flow filtration108,115 (Fig. S1B†), 5) antibody mediated debulking of the sample prior to filtrations or separation,146,160 and 6) incorporating a mechanism to provide and active clearing of the filter bed.108,161 These approaches work as they either reduce the number of cells that the filters must prevent from passing (debulking), increase the available surface area (thus increasing the throughput possible before the filter becomes blocked) or actively clear the filter area (so that the device can run for a longer period before the filter is overwhelmed with particles blocking the passage of the plasma).Devices that use physical filtration to extract plasma may produce plasma with high purities, but they tend to operate at very low flow rates and have a very low total blood processing volume. When low extraction volumes are coupled to the low concentrations of cfDNA found in the plasma (estimated at around 1000 genomes in 1 ml of blood plasma65), the sensitivity of the downstream assay can be severely compromised. The maximum sensitivity can be calculated based on the average yields of cfDNA in plasma and the weight of the human genome to calculate the equivalent genomes present in the extracted cfDNA. To put this in perspective, as the diploid human cell contains approximately 6.5 pg. of DNA,162 and the human (haploid) genome would weigh half of this (approximately 3 pg), in systems such as those proposed by Shim et al., that can only process 20 μl of whole blood,106 the extractable amount of cfDNA would be around 180 pg or 60 genomes (based on a haematocrit of 45% and an cfDNA yield of 20 ng ml−1 of plasma). Even assuming 100% cfDNA recovery and conversion of cfDNA into measurable product, assays relying on such low inputs are unlikely to contain sufficient starting ctDNA and would not be suitable for sensitive detection of cancer. This fact is further highlighted by systems such as those proposed by Szydzik et al. which only yield 180 nl of plasma.108
Filtration and solid capture approaches for CTCs and CTC cluster capture have also been created.132,146,151 Due to the overwhelming numbers of RBCs, these are often implemented after RBC depletion. This depletion can be via osmotic RBC lysis, RBC depletion with MACS or via on-chip RBC depletion.115 In some cases, depletion of white blood cells (usually via CD45 mediated immunogenic capture) is also performed.146 This, however, would not be suitable for heterogenic CTC clusters containing CD45 positive immune cells, as the clusters would likely be removed from further analysis. Filtration for CTC enrichment generally functions by retaining CTCs and letting the smaller cells through. In some cases, these use commercially available filters (i.e. 3D printed Monolithic device146), in other cases, the filters are created as part of the device design. Examples of this include the Cross chip (Fig. S1E†) which creates a basic single row filter to prevent large cells from passing,127 the Parsortix system (Fig. S1D†) which uses a graduated step filter and tangential flow to prevent larger cells from passing into the waste stream,124,125 and a device in which perforated cups, oriented so that the cup opening faces the direction of fluid flow, are designed in order to capture large CTCs while letting smaller cells to pass through.151 CTC clusters can also be captured using physical filtration approaches. An example of such a device includes the Cluster-Chip which uses carefully arranged triangular pillars to capture multilobular CTC clusters. Clusters are held in place against the vertices of the triangular pillar using the pressure of the fluid flow to prevent the CTC cluster from being carried along the flow streams.132
4.1.2. Deterministic lateral displacement. Deterministic lateral displacement (DLD) devices take advantage of the fact that pillar/post arrays can be created to force cells above a certain size to move laterally in relation to the direction of fluid flow. DLD devices have extraordinary resolving capacity and are capable of separating particles with a resolution of as little as 10 nanometres.163 The particle size at which this movement occurs is known as the critical diameter (dc) and can be calculated by the equation dc = 20% × 2w (where w is the separation gap between the pillars).83 These devices are generally created using an array laid out in one of two ways. The first being the row shifted parallelogram array and the second being the rotated square. Work from Vernekar et al. has shown that the rotated-square arrays are less prone to anisotropic permeability and therefore provide more consistent performance.164 Work to use DLD approaches to separate plasma from the blood has successfully resulted in the simultaneous separation of plasma and cells from undiluted blood102 (Fig. S1C†). Although this multistage device did result in plasma separation with a claimed theoretical achievable efficiency of 100% with no contaminants above 1 um in diameter, the flow rate was only 0.4 μl per minute. Systems such as this would therefore process only 24 μl of blood each hour. Considering a cfDNA yield of 20 ng ml−1 of plasma, a haematocrit of 45% and perfect extraction of cfDNA this is equivalent to approximately one genome every 8 min.Although not common, DLD devices can also be used to separate cells based on size. For example, the DLD-FD device designed by Davis et al. could separate cells based on a diameter above 9 μm.102 In this way, RBCs could be depleted from whole blood. Whilst these devices can provide extremely accurate size selection, they are almost always prohibitively slow for use in all but the lowest volume clinical assays. Traditional DLD devices are also prone to clogging149 and thus may not yet be robust enough to be put into routine clinical workflows. Recently however, a modified pillar-post structure based around the concept of filtered deterministic lateral displacement has allowed rapid fractionation of CTCs through a two-step process involving the initial debulking of small cells, followed by a secondary fraction process aimed at purifying CTCs based on size.148 The device uses a modified DLD pillar design in which a filter channel is incorporated into the pillars themselves. This decreases the critical diameter when compared to pillar designs without the filter (i.e., the filter DLD pillar design has an effective critical diameter of 10 μm while a non-filtered equivalent DLD array would have a critical diameter of 15 μm). The first stage of the device allows parallel processing of the sample to remove RBCs. Following RBC depletion, the sample is combined with a flow buffer to enrich CTCs. This approach depletes 100% of RBCs and >99.95% of WBCs with a high capture rate for large CTCs and allows blood to be processed at fluid flow rates of 1 ml min−1 and low fluid velocities (∼1.5 cm per s−1 with only a 1
:
1 dilution of whole blood148) (Fig. S1K†).
CTC clusters can also be enriched using DLD. The NISA-XL device proposed by Edd et al. is reported to have on-chip CTC cluster yields of 100% at flow rates of more than 30 ml hour−1 and can run with Haematocrit level of up to 20% (ref. 149) (Fig. S1J†). Additionally, Au et al. used an integrated 2 stage DLD array to enrich large and asymmetrical clusters from whole blood165 (Fig. S1I†). They showed that, in whole blood, large clusters could be collected with an efficiency of around 98% while the smaller, asymmetrical clusters performed worse with an efficiency of just around 65%. Like many DLD devices, the device was relatively slow and could only run 500 μl of blood per hour.
4.1.3. Affinity surface capture. Affinity surface capture devices directly tether the capture antibody to the substrate of the microfluidic chip. We have included affinity surface capture devices in the category of solid interaction devices as they rely on a cell/particle to come in contact with an antibody that is directly tethered to the device. Affinity-based microfluidics capture methods are perhaps the most common of all microfluidic-based CTC capture devices and include devices such as the Oncobean131 (Fig. S1F†), Nanovelcro,133 CTC chip,140 GO Chip142 (Fig. S1G†), GEDI,141 and Herringbone-chip138 (Fig. S1H†). Nagrath and colleagues proposed a microchannel, called “CTC-chip” for separation of CTCs from patient blood. In this channel, micro posts were functionalized with an anti-EpCAM antibody, providing specificity for capturing CTCs expressing this epithelial cell marker.140 To increase the efficiency of capturing rare cells, the interaction between cells and the coated surface needs to be high. To achieve this, the “Herringbone-chip” was developed. It works by generating a vortex in the coated microchannel in order to increase the number of times a cell comes into contact with the chip surface. Despite the increased surface interactions, the recovery rate for prostate cancer cells in this channel was still only reported to be more than 21% using anti-EpCAM antibody.138The effectiveness of affinity-based systems is dependent on a number of factors, including 1) the specificity of the antibody chosen, 2) the ability of the devices to bring the cell into contact with the capture antibody,133,138 3) the balance between the forces generated in the devices itself,140 4) the avidity of the antibody used (i.e. the total binding strength of the antibody to the cell), and 5) the antibody dissociation constant.166 Affinity-based methods are suitable when a particular epitope is exclusively expressed on the surface of CTCs (positive selection) or when it is known that the CTCs do not express a particular marker (depletion). Commonly CTCs have been targeted using EpCAM,44,140 GD2,167,168 EGFR,166,169 PSMA,141 HER2,170 and HER3.169
Whilst diseases such as breast, colon, lung, and prostate cancer have utilised EpCAM mediated binding, it has been shown that by accounting for EpCAM negative CTCs, the number of patients in which CTCs can be found increases.21,171 In other diseases that lack EpCAM expression, independent methods are required.172 For example, markers such as GD2 in neuroblastoma and CD99 in Ewing's sarcoma, although expressed by cancer cells, are not restricted to CTCs alone167,173 and therefore require technologies, such as flow or imaging cytometry, that can perform hierarchical classifications such that the CD99 positive population can be enriched for CTC by selecting only CD45 negative cells.173 Additionally, many carcinomas will undergo an epithelial-to-mesenchymal transition (EMT) as part of the metastatic cascade. During EMT, the epithelial cell surface marker EpCAM is downregulated or lost,174 and as a result, affinity-based methods relying on CTCs being separated using EpCAM will not be suitable.147,174–177 Examples of the loss of EpCAM during cancer progression to metastatic disease have been shown in renal cell carcinoma,47 breast cancer,178,179 and particularly in CTC clusters rather than individual CTCs.12 To bypass the requirement for CTCs to express a specific target molecule, affinity-based methods have been developed to deplete non-CTCs, these often target the immune cell marker CD45.146 Whilst effective at removing WBC contamination, these approaches will also deplete CTCs that are travelling as clusters composed of immune cells.
While affinity based devices almost exclusively rely on cell surface expression of proteins, novel systems that allow separation based on intracellular proteins have also been developed;145 however, this is far from a standard approach and requires cell fixation and permeabilization. Nonetheless, it provides an interesting and potentially beneficial approach in some cases. The system works by first fixing and permeabilizing the cell before staining with an antibody raised against an intracellular protein target. Following antibody binding, magnetic nanoparticles conjugated to an oligo that is complementary to a sequence on the antibody itself are introduced. The cells are then flown through a microfluidic chip with a progressively decreasing magnetic field to allow separation based on protein expression levels. This approach removes the need to restrict potential CTC markers to those expressed only on the cell surface and can be used to directly targets therapeutic protein targets such as c-Myc.145
4.2. Fluid interaction devices
Microfluidic methods relying on fluid interactions utilise the working mechanism of 1) plasma skimming and the Fareheus effect, 2) bifurcation effect, and 3) inertial focusing. Plasma skimming and the Fareheus effect as well as the bifurcation effect are exclusively used for the collection of the plasma while inertial focusing, in all its forms, is predominantly used to purify cells based on size. The simplified representation of the working principle of devices in this category is shown in Fig. 4 while specific examples of devices are shown in Fig. S2.†
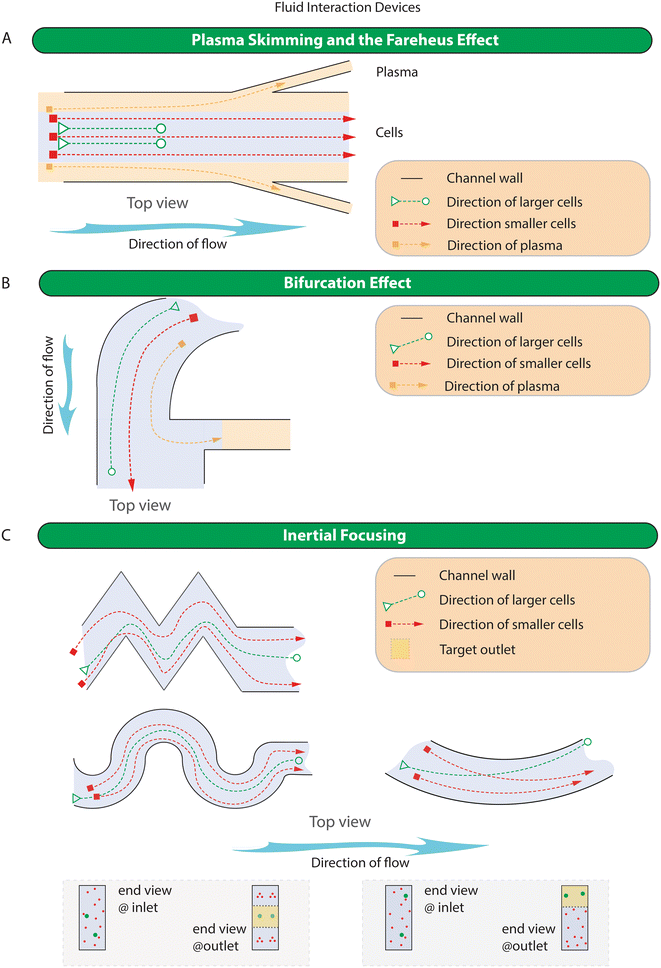 |
| Fig. 4 Schematic representation of the working principles for fluid interaction microfluidic devices in blood fractionation. A) Plasma skimming and the Fareheus effect devices. The Fareheus effect occurs as cells tend to orient themselves toward the centre of the channel and can be used to collect plasma from the cell free region that exist close to the walls of small vessels B) bifurcation effect devices. The bifurcation effect occurs as cells tend to migrate towards faster flowing fluid streams after they encounter a bifurcation or split, C) inertial focusing devices come in many different configurations including spiral, serpentine, straight, and contraction expansion devices, whilst the physics in these devices are different, they all work by allowing targets cells to be trapped in equilibrium locations within the fluid flow. These devices are often used for CTC enrichment but have also been applied to plasma separation. | |
4.2.1. Plasma skimming and the Fareheus effect. The Fareheus effect describes the propensity of RBCs to position themselves in the centre of tubes with a diameter of less than 0.3 μm.180 The cell-free layer (CFL) is dependent on the diameter of the channel, the haematocrit, and flow rate.181,182 Considering these factors, it is possible to design a device that essentially skims a small portion of the CFL while leaving the majority of cells untouched in the main channel.103,183 As the relative CFL thickness becomes smaller as the tube diameter increases many of the devices relying on the Fareheus effect utilise small channels. Additionally, as the CFL thickness also decreases with increasing haematocrit dilution of whole blood is often performed. Modelling by Fedosov et al. showed that the thickness of the CFL in a 40 μm diameter tube decreased from around 5 μm when the haematocrit was 15% (diluted blood) to under 5 μm when the haematocrit was 45% (normal blood).181
4.2.2. Bifurcation effect. The bifurcation or Zweifach–Fung effect can be observed as the tendency for cells flowing through a bifurcation to preferentially travel in the post bifurcation channel with the highest flow rate. Experimentally, devices relying on the Zweifach–Fung effect work best when the flow ratios between the channels exceed 1 to 6184 and rising haematocrit levels result in smaller CFLs and poorer plasma purities.185 Nonetheless when designed and implemented appropriately, plasma purities of >99.5% can be achieved, but yields are often poor 1–6%.104 A number of device designs exist in this space; importantly, these devices have larger feature sizes than either physical filtration or plasma skimming devices and thus operate at significantly elevated flow rates.104,110,186,187Collection of the plasma using plasma skimming or the bifurcation approach can result in high purity, but throughput tends to be very low, and yields can be poor. In devices such as those described by Kuan et al. and Yang et al. (Fig. S2A†) that operate at 0.3 ml min−1, 10 ml h−1 respectively,115,184 the volume of plasma processed would be insufficient as it would only yield between 90 and 162 pg of cfDNA per hour. An exception to this may be the all glass bifurcation chip110 that can operate at 700 μl min−1 and return 74% of the plasma with only a two (2) fold dilution of the blood (Fig. S2B†) and a PDMS chip by Tripathi et al. that can operate at 500 μl min−1.104
4.2.3. Inertial focusing. Inertial microfluidics is used to focus a particle into a particular region of a microfluidic channel. In a straight channel, particles and cells are affected by inertial lift forces that include shear-induced and wall-induced lift forces. The balance of these two forces creates equilibrium positions where particles will tend to sit.188 The location and the number of equilibrium positions are governed by the channel shape and its hydrodynamic diameter.189 In the case of non-straight channels such as spiral, serpentine, or contraction-expansion microchannels, Dean drag forces also contributed to the focusing particles within the channel cross-section. For a given channel design, the value and strength of inertial lift and Dean drag forces are related to the particle size.190 Within the inertial regime, particles above a certain threshold in PDMS-made channels (ap/h > 0.07, where ap is particle diameter and h is channel height) are heavily influenced by inertial forces; hence, one can accurately focus cells into lateral position within the channel.191 This criteria has been refined for rigid channels to be ap/h > 0.04.192In 2007 Seo et al. used a double spiral design to enrich 10 μm partciles by 660 fold193 (Fig. S2D†). Inertial focusing channels have proven to be an extremely popular method to fractionate cells and particles from fluids. As such, in addition to single spiral devices with rectangular channel cross-sections194 (Fig. S2E†), a number of spiral device variants have been created; these include cascaded spiral microchannels,156,195,196 labyrinth-shaped channel197,198 (Fig. S2F†), multiplexed spiral channels,199 double spiral microchannel,200 spiral with slanted walls,126 triple parallelizing spiral device,201 ordered micro-obstacles spiral device,202 sequentially connected spiral channels,203 single loop spirals,120 and spirals that include u-turns.121 Recently Zhu et al. developed a spiral device that incorporates contraction–expansion arrays in the last loop resulting in improved separation of both CTCs and CTC clusters at high flow rates (3.5 ml min−1)119 (Fig. S2G†). Recognising the usefulness of the spiral design but acknowledging the need to further purify and isolate CTCs, Lu et al. designed a cascaded microfluidic chip consisting of an initial five loop spiral microchannel followed by an array of perforated cups to catch CTCs and to allow residual smaller cells to pass through to waste.122 Furthermore, when Xiang et al. coupled a DLD array downstream of a spiral device, they showed improvements to capture purities and separation efficiencies.139 Additionally non-spiral geometries for CTC enrichment include straight channels155 (Fig. S2H†) and the contraction–expansion array (CEA) microchannel devices developed by Hur et al.137 Sollier et al.130 (Fig. S2I†), and Lee et al.204 have been developed. These concentration–expansion arrays trap cells based on size in laminar vortices created in the areas of expansion.137
For CTC enrichment, inertial focusing has been applied to several cancers including breast,205 prostate,206 lung,207 head and neck,157 and glioblastoma.73 These studies rely on the assumption that CTCs are larger than the majority of cells in the blood (>∼15 μm); as such, the devices may have limited use in the capture of small CTCs. The inability to isolate small CTCs may be problematic as CTC heterogeneity suggests CTC sizes may vary, not only between cancers but within patients208 and by the compartment in which they are found (i.e., CSF CTCs VS blood CTC's).209 A study by Mendear et al. which assessed the size of CTCs (as captured by the cell search system – EpCAM capture and verified by Cytokeratin positive staining), showed that CTC size can vary significantly between tumour types and the compartment in which CTCs were found. For example, CTCs from colorectal and bladder cancer (7.5 and 8.6 μm median diameters respectively) were much smaller than the cut-offs commonly used in the design of size-based CTC capture devices and importantly from lymphocytes (9.4 μm median diameter), while CTCs isolated from breast cancer and prostate cancer, although bigger (12.4 and 10.3 μm median diameters respectively) were not as big as generally assumed, and the breast cancer CTCs from the blood were significantly smaller than those found in the CSF (120.4 μm2 vs. 141.3 μm2 respectively). Furthermore, they showed that the cell lines often used to validate devices do not necessarily reflect the size of the CTCs found in the patient e.g. the average size for breast cancer CTCs in the blood (12.4 μm median diameter) was significantly smaller than that of common breast cancer cell lines such as MCF-7 (18.4 μm median diameter), T47D (18.35 μm median diameter, and SKBR3 (22.01 μm median diameter).209 This finding is also supported in prostate cancer.208 To overcome the issue of size-based heterogeneity, improve throughputs, and reduce contamination, Liu et al. have combined inertial focusing (serpentine channels) with MACs.116 The device still requires RBC lysis and cell staining but achieves a flow rate of 60 ml h−1 and reduces WBC contamination down to 507 WBCs per ml.
Inertial focusing has also been used for plasma separation;107,199 however, this requires significant sample dilution, making the plasma unusable for many biological assays. By way of illustration, a standard blood collection tube used in adults has a maximum volume of 10 ml and commonly ∼8 ml is collected. If blood was to be fractionated in its undiluted state, the resulting fractions would be roughly 4 ml plasma and 4 ml cells (including RBCs). This volume is compatible with current extraction methods for cfDNA. However, if the blood was diluted even 1
:
10 such as described by Lee et al.,107 then the resulting “plasma” fraction would have a volume of 40 ml and new cfDNA extraction methods would need to be devised. Furthermore, as described, the device only has a 60% RBC rejection rate and a 62% plasma yield (Fig. S2C†). If diluted to 1
:
100, so as to achieve a blood haematocrit of ∼0.5%,199 then the volume of plasma from which cfDNA is to be extracted would be 400 ml, thus extraction of cfDNA from diluted whole blood would be impractical and costly.
4.3. External force/active microfluidics
Although more complicated than the passive approaches, active devices are more amenable to end-user modification. This is because, once created, devices that rely on fluid or solid interactions tend to have their parameters set during the device fabrication, while those that provide external forces can be tweaked according to the end user's preference. For example, to select a new target cell in mMACS and mFACS, one simply needs to label cells with a new antibody, or if separation is achieved using acoustophoresis, it is possible to tune the applied field to separate particles with different characteristics including size and cell viability.210 Devices in this category rely on the working mechanisms of 1) lab-on-a-disk, 2) acoustophoresis, 3) microfluidic magnetic associated cell separation (mMACS), 4) microfluidic fluorescent cell sorting (mFACS), 5) piezoelectric-membrane actuated mFACS, and 6) electrokinetic actuated mFACS. The simplified representation of the working principle of devices in this category is shown in Fig. 5 while specific examples of devices are shown in Fig. S3.†
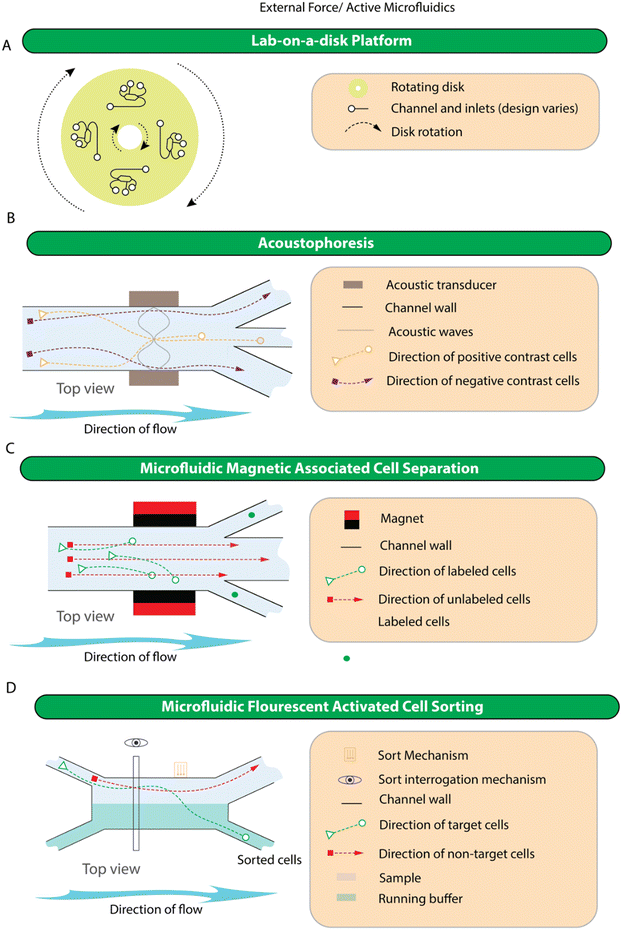 |
| Fig. 5 Schematic representation of the working principles for external force/active microfluidic device in blood fractionation. A) Lab-on-a-disk devices. Devices in this category can be designed for both plasma and CTC enrichment and whilst highly varied, all use rotational forces to help process the blood. B) Acoustophoresis can be used to separate blood into plasma and enrich for specific cell types. The example device shown uses a standing wave to focus cells at the node and the antinode depending on a cell's acoustic properties and the characteristics of the acoustic wave. Other devices can tune the wave to focus all cells so that plasma can be obtained. Acoustic waves can also be used as the sort mechanism in microfluidic cell sorting. C) Microfluidic magnetic associated cell separation. These devices require cells to be labelled with an antibody attached to a ferrous particle so that when the labelled cells enter a magnetic field they are deflected according to the polarity of the magnetic field. D) Microfluidic fluorescent activated cell sorting devices allow the interrogation of fluorescently labelled cells using a light source and detector(s) to enable active selection of cells based on expression or absence of certain markers. Fluorescent labels are often attached to antibodies, but this does not need to be the case and theoretically any fluorescent tag(s) can be used. Sorting the cell of interest can be achieved in a number of ways, these include piezoelectric-membrane actuated mFACS, acoustic wave actuated mFACS and electrokinesis. | |
4.3.1. Lab-on-a-disk. Centrifugal microfluidic platforms, often referred to as lab-on-a-disk systems due to their disc-like design, have emerged as promising tools for liquid biopsy and blood fractionation. These platforms leverage the principles of centrifugal force to manipulate fluid samples within microscale channels built onto a spinning disc. The rotation of the disc enables precise control over sample handling, mixing, separation, and analysis, all within a compact and portable format.211Centrifugal microfluidics has been used for plasma separation. In 2006, Haeberle et al. presented a centrifugal microfluidic platform to isolate plasma from whole blood using a decanting structure. The device has been successfully utilized to extract 2 μl of plasma from 5 μl of whole blood, with a residual cell concentration of below 0.11%.212 As another example, through the use of a curving channel with one-fourth of a turn, plasma of a blood sample with 6% haematocrit concentration can be isolated with 96% efficiency and achieved within 5–6 seconds.213 The practicality of the devices was illustrated by performing prothrombin test213 and creatinine test.214 However, these volumes are a long way from being useable in modern liquid biopsy for genomic analysis and the need to dilute blood to known haematocrit concentrations is counterproductive for high-sensitivity assays where the number of targets into the system matters.
In 2022, Shing and colleagues presented a portable centrifugal microfluidic device for plasma separation. This device comprises multiple chambers and microchannels, wherein a plasma reservoir and a cell reservoir are interconnected and aligned with the centre of the disc. This configuration achieves a plasma purity of 99.9%, separation efficiency of 99.9% (with a blood haematocrit of 48%), and a plasma recovery rate of 32.5% within 50 seconds.215 In another study, Lenz et al. introduced a microfluidic device designed for centrifugal serum separation from blood, suitable for on-site use. This system accommodates both hydrophilic and hydrophobic biomarkers. The cross-flow filtration mechanism efficiently separates serum from blood, comparable to conventional techniques, while preserving amphiphilic biomarkers within the serum for subsequent detection.216 Hatami developed a fully automatic centrifugal microfluidic platform to isolate plasma for the isolation of cell-free foetal DNAs (cffDNAs). Besides plasma separation, all the required steps for cfDNA extraction, including adding proteinase K, lysis buffer, binding buffer, washing buffer, and elution buffer, have been considered. To accomplish this goal, magnetic particles were utilized first to attach the cfDNAs, and then transfer them between the chambers.217
Centrifugal microfluidics has also been developed to advance CTC isolation from blood. For instance, Park et al. developed a centrifugal microfluidic platform aiming for fully automated, high-purity isolation of CTCs. Their approach utilizes a disc device with a triangular obstacle structure in the blood chamber to manipulate blood cells. CTCs are selectively bound to microbeads coated with anti-EpCAM, allowing for density-based isolation under a density gradient medium layer. The system can process 5 mL of blood, yielding isolated cancer cells within 78 minutes with minimal contamination of approximately 12 leukocytes per millilitre.154 In 2021, two centrifugal microfluidic devices were developed for isolating rare cancer cells: a passive plan and a hybrid plan. The passive plan employs a contraction–expansion array (CEA) microchannel connected to a bifurcation region, while the hybrid plan uses a CEA microchannel reinforced with magnets to capture magnetically labelled target cells. Both designs were optimized and tested for isolating MCF-7 breast cancer cells from mouse fibroblast cells. The hybrid design, utilizing magnetite nanoparticles and permanent magnets, demonstrated superior performance (85% recovery rate at 1200 rpm) compared to the passive design (76% recovery rate at 2100 rpm). However, the passive design offers advantages such as simplified processing, compact size, and cost-effectiveness.218 In a most recent study, a simple label-free centrifugal microfluidic device was proposed to separate cancer cells from whole blood samples using synergetic hydrodynamic effects, including Y-shaped microchannel, a contraction–expansion array (CEA) microchannel, and a bifurcation region. Experimental results demonstrated the successful separation of K562 cancer cells from diluted blood samples (ratio 1
:
1.2 × 105), achieving a high efficiency of 90% at an angular velocity of 2000 rpm219 (Fig. S3E†).
Although centrifugal microfluidics removes the need for setting up complex pumps and collection systems, some challenges still require careful attention. Clogging is one of the major challenges within these platforms, although implementing filtration techniques can alleviate this challenge. Additionally, scalability and throughput are areas of concern due to the limited capacity for sample processing within these platforms.
4.3.2. Acoustophoresis. Acoustophoresis is a gentle, non-contact method whereby cells in suspension exposed to an acoustic standing wave field are affected by an acoustic radiation force.220–223 A cell experiencing this force will move toward nodes or antinodes, and its movement depends upon physical properties such as density, compressibility, and size.224–227 The implementation of acoustophoresis in microfluidic devices has gained significant appeal because it is a miniaturised, label-free active separation approach that addresses the challenge of isolating rare cells within a heterogeneous population.221,228 Microfluidic acoustophoresis systems are typically designed to separate particles or cells of different sizes or mechanical properties.221,225,226,229 These systems have been applied to blood samples to separate the blood components in a continuous and biocompatible manner. Petersson et al. demonstrated the ability to perform plasma exchange of blood using a type of acoustophoresis known as bulk acoustic wave (BAW)-based separation.230 Acoustophoresis has also been shown to isolate plasma from blood105 (Fig. S3B†) and has the ability to isolate exosomes or other types of extracellular vesicles directly from whole-blood samples.231,232In addition to plasma separation, acoustophoresis has also been demonstrated to remove platelets from peripheral blood progenitor cell products (PBPC and whole blood samples)233–235 and to isolate circulating tumour cells (CTCs) from lysed or pre-treated whole blood.144,228,231,236–238 Furthermore, acoustophoresis is also biocompatible, showing that cell viability was maintained during the separation process.228 Olm et al. used electrophoresis to separate neuroblastoma cells (SH-SY5Y) from blood cells using a two-stage approach incorporated onto a single chip. The first stage was used to align all cells into 2 parallel bands so that they are appropriately oriented for the second stage with involved acoustically focusing the larger SH-SY5Y cell to the centre of the channel where they could be collected (Fig. S3G†). Using this device, they showed that they could recover between 60 and 90% of spiked in neuroblastoma cells.144
With time, acoustophoresis-based separation offers a pathway to enhance liquid biopsy applications and impact the field of translational medicine. However, it still suffers from poor yields and is yet to be convincingly applied to high volume plasma separation or to CTC isolation across a range of cancers.
4.3.3. Microfluidic magnetic associated cell separation (μMACS). Affinity-based capture methods rely on the cells of interest expressing an epitope for which an antibody can be raised. For CTC capture, these systems have tended to rely on cancer cells expressing the epithelial cell marker EpCAM. The only FDA approved system for CTC capture and enumeration, CellSearch, captures CTCs using a magnetic core nanoparticle conjugated to an anti-EpCAM antibody. There are many microfluidic-based MACS systems available for the detection of CTC. The cell search and the isoflux systems are perhaps the best known of these but there are others. However, the increasing recognition that EMT plays a significant role in many solid tumours means that these systems are likely to miss many of the CTCs that have undergone transition to a more malignant phenotype. Furthermore, whilst these and other mMACS systems can be modified to capture cells with alternate markers, they still overwhelmingly rely on a single cell surface target molecule. Thus, they are likely to be missing the expected heterogeneity in the CTC population.One way around the reliance on a single target for enrichment is to utilise a depletion approach. Microfluidic devices such as LPCTC-iChip platform239 or the the monolithic CTC incorporate a combination of solid interactions (DLD array), fluid interactions (serpentine inertial focusing), and external forces (MACS) to achieve CTC enrichment147 (Fig. S3D†). In the monolithic device, blood is firstly depleted of red blood cells and platelets via a DLD array before the nucleated cells (CTCs and WBCs) are focused using an inertial approach to allow labelled (CD45 positive cells) to be removed by two passes through a magnetic field. The first pass depletes WBCs with >6 MACS beads (targeting CD45, CD16, or CD66b) attached while the second pass cleans up those cells with more than one magnetic bead. Once WBC depletion is complete, CTCs are collected and concentrated for downstream validation.
4.3.4. Microfluidic cell sorting (μFACS). Identifying and isolating cells of interest from complex, heterogeneous mixtures in a sequential and rapid manner represents an essential process for many areas in clinical medicine. Microfluidic-based fluorescent activated cell sorting (μFACS) relies on fluorescent probes or stains to identify cells by type. The fluorescently labelled cells are flowed through a microchannel and encounter a focused laser that scatters into a photodetector. The fluorescent signal is then analyzed, and depending on the detection criteria, an external force is generated to displace the cell into a separate channel. This technique eliminates the production of aerosols and can support small sample volumes that are orders of magnitude smaller than conventional jet-in-air cell sorters. More importantly, μFACS can provide a means toward the isolation of rare target cell populations, such as the enrichment of circulating tumour cells (CTCs).Several cell-sorting mechanisms have been used in microfluidic devices, including 1) piezoelectric actuation, 2) surface acoustic waves (SAW), and 3) pulsed laser-activated cell sorting (PLACS).240–242 Piezoelectric-membrane actuation is a mechanism used in microfluidics whereby a piezoelectric element causes the deformation of a membrane adjacent to a microchannel to displace a particle of interest into a separate channel, usually in response to fluorescent detection.240,243–245 This technique can sort individual cells at rates of up to several kHz per channel and can be easily parallelised for increased throughput.240 The use of a piezoelectric actuator has been demonstrated to work in glass microfluidic chips, which is a more commercial and clinical friendly material when compared to the commonly used PDMS.240,244 Acoustic waves have gained significant use in μFACS due to their ability to gently organize and/or displace cells or droplets into separate channels at fast rates.228,242,246–248 Traditionally, this mechanism was used for applications where cells were not labelled. However, when combined with a fluorescent detection system, this mechanism can achieve switching times as short as 25 μs (ref. 242) (Fig. S3F†).
Electrokinetic mechanisms for fluorescent label-based microfluidic cell sorting are divided into three categories: 1) electrophoresis, 2) dielectrophoresis (DEP), and 3) electroosmotic flow.249 Electrophoresis refers to the movement of particles in a uniform electric field due to their surface charge.249,250 This mechanism takes advantage of the slightly negative charge most cells possess.251 In contrast to electrophoresis, where cells move in a uniform electric field, DEP refers to the movement of cells in a non-uniform electric field due to their polarizability.249 For movement in response to a dielectrophoretic force, cells do not need to possess a surface charge because, unlike a DC field, an alternating current (AC) can induce a dipole moment across the cell.249,250 DEP is commonly used in droplet-based microfluidic applications, whereby cells are encapsulated into picolitre volumes for single-cell assays.252,253 The use of DEP in a fluorescence-activated droplet sorter has been demonstrated to sort up to several or more thousand cells per second.254,255 This technique also enables the compartmentalisation of cells into emulsions with antibody-coated beads to analyse the secretion of antibodies from cells for downstream sorting.256 Electroosmotic flow, on the other hand, moves the bulk fluid surrounding a cell due to the electrically induced migration of solvated ions.257,258 This method, however, can generate bubbles and harmful compounds such as hydrogen peroxide, which can adversely affect cells.259
In addition to using electrokinetic forces in microfluidic FACS, it is also possible to harness this force for separation of plasma. As described in the filtration section, DEP is also used to clear filter beds during plasma fractionation108,161 (Fig. S3A†). Xing et al. has also applied electrokinesis in the separation of plasma109 (Fig. S3C†). However, whilst the system provided good plasma protein yields, the need to dilute blood to 4% haematocrit, the use of a high conductivity buffer, and the low processing volumes make the system not suitable for use in ctDNA based liquid biopsy.
5. Strengths and limitations of current microfluidic approaches
Traditional bulk separation methods of centrifugation and filtration have served as the foundation upon which liquid biopsy has begun to offer crucial insights into cancer, while current microfluidic devices have, to some extent, filled the need for rapid processing of samples and the ability to effectively process low sample volumes. Current microfluidic tools have allowed numerous studies across a number of different cancers and have been instrumental in proving that liquid biopsy can provide a powerful mechanism to quantitatively assess tumour burden, measure metastatic potential through CTC enumeration and to track genomic changes as part of disease progression or in response to treatment induced change. Importantly, by enabling this on peripheral blood samples, liquid biopsy reduces the need for invasive procedures and can help improve the quality of life for patients during their cancer journey. To understand how microfluidics is contributing to enabling liquid biopsy, an overview of the general strengths of microfluidic approaches to blood fractionation is given in Table 3.
Table 3 Strengths of current microfluidic tools for plasma (ctDNA) and CTC analysis. It should be noted that not all the strengths are applicable to all devices
Plasma devices |
Strengths |
Impact |
Ability to handle low sample volumes |
It can be very difficult to handle low sample volumes using conventional methods such as centrifugation. Microfluidic devices often have extremely low dead volumes and are therefore ideally suited for handling low input samples. Due to biological considerations, low input volumes often make ctDNA and CTCs analysis difficult; however, emerging techniques in high throughput proteomics and improved efficiencies in genomic preparations are reducing the volume of sample required |
CTC devices |
Strengths |
Impact |
Many devices can process cell in parallel |
Unlike techniques such as fluorescent activated cell sorting or flow cytometry, many microfluidic approaches for CTC enrichment allow parallel processing of individual cells. This can significantly increase the throughput and reduce the operational time for CTC enrichment. Shorter processing times may minimise pre-analytical variables and thus allow a truer reflection of biology |
Many devices are label free |
Techniques, particularly those that use fluid interaction and to a lesser extent external forces to separate CTCs, do not require prior knowledge of cell surface proteins on the CTC. This is useful when no markers are known or when biological process may be down regulating known cell surface markers |
General |
Semi-automated nature of microfluidics |
As many devices are semi-automated, the ability of an operator to affect outcomes can be minimised. This of course depends heavily on the device design so care should be taken here |
Some devices have been specially designed to reduce the stress on cells during the fractionation process |
Microfluidics can be designed to have low pressures and to minimise shear forces acting on cells. This means that the samples may be less affected by preanalytical factors that may otherwise lead to compromised results. This is device dependant and should be a consideration when assessing any device |
Microfluidic devices generally have a small footprint and a low initial cost |
Microfluidic devices are often relatively simple channels made from cheap material (active devices can be an exception to this rule). This makes the startup cost relatively low. The also tend to have small footprints meaning that they do not require a lot of laboratory space |
Systems are generally closed and do not produce aerosols |
Handling of primary samples can produce a biohazard for operators. The closed nature of many microfluidic devices increase operator safety of sample are not aerosolized and therefore more readily contained |
Despite the important role of bulk fractionation and current microfluidic methods in the development of liquid biopsy for cancer patients, there remains a critical need to further improve the processing of blood samples. This process would ideally occur close to the point-of-draw and enable rapid sample processing and produce fractions that are suitable for multiomic liquid biopsy. There are, however, no solutions that allow a full multiomic workflow leveraging both ctDNA and CTC analysis at the same time. Furthermore, the current generation of microfluidic fractionation devices all have limitations that mean they are still not optimally suited to use in emerging next generation analysis pipelines. These limitations are shown in Table 4.
Table 4 Limitations of current generation of microfluidic tools for plasma (ctDNA) and CTC analysis. It should be noted that not all the limitations listed are applicable to all devices
Plasma devices |
Limitations |
Impact |
Speed of processing |
To extract sufficient material (and hence equivalent genomes) for high sensitivity detection of cancer a significant amount of blood needs to be processed >2 ml. Some devices run at less than 1 μl per minute, therefore retrieving enough cfDNA to perform the assays is impossible |
Prone to clogging |
Clogging of chips can prematurely terminate the collection of plasma and therefore reduce the overall yield of plasma from a run. The inability to collect all the sample prior to mechanical failure due to clogging has the effect of reducing yield and may compromise the purity of the sample |
Often require dilution |
Dilution of blood (sometimes by up to 100-fold), means that vast quantities of plasma will be collected. Even if this plasma was free form impurities and the yield was high, the volumes would prevent any meaningful downstream analysis and purification of cfDNA would be extremely difficult and likely cost prohibitive |
CTC devices |
Limitations |
Impact |
Many cannot process whole blood and require dilution of RBC lysis |
Blood dilution for CTC increases the processing time and whilst the affect is not as pronounced as with cfDNA (CTCs can be more readily concentrated) it nonetheless still provides potential delay to analysis. Where RBC lysis is utilised, CTCs are subject to unfavourable conditions and therefore subjected to unnecessary stress (which may result in experimentally induced changes) |
Immunoaffinity methods require prior knowledge of protein targets |
While some cancers such as prostate, breast, lung, and neuroblastoma shed CTCs that express well-known cell surface markers, others do not have well-defined cell surface markers. In addition, most immunoaffinity methods rely on a single marker and given the well-known heterogeneity in CTCs (as a result of EMT) many CTCs may not be captured and the population is biased towards a single CTC subtype |
There is no way to perform intracellular immunoaffinity collection in live cells |
Whilst cell surface targets allow CTC enrichment in some cancers, many cancer specific epitopes are intracellular. Therefore, by relying on cell surface protein markers for live CTC enrichment, we are reducing the number of targets available. Furthermore, restricting to protein epitopes may be limiting, as cancer is primarily a disease of genetic alteration |
Immunoaffinity depletion methods remove all cells that express the target |
Methods that rely on depletion using an antibody/antibodies risk losing CTCs that either co-express the marker or are traveling as clusters that contain cells expression the marker. Therefore, these methods will bias CTC selection, reduce capture numbers and may be blinded to the contribution of heterotypic CTC clusters |
Size based methods assume all CTCs are above a certain size |
Heterogeneity in CTCs size has been shown in several cancers. Therefore, the assumption that CTCs are always bigger than other cells in the blood means that smaller CTCs are lost |
Despite the strengths of many microfluidic devices for blood fractionation in cancer liquid biopsy, the limitations shown in Table 4 suggests that there is now a need for new devices and a significant opportunity to improve on current microfluidics approaches for blood fraction in relation to cancer liquid biopsy. For example, a rapid, low-cost, point-of-draw fractionation method that allows the separation of all biologically relevant blood fractions into their constituent elements in a format compatible with multiple emerging downstream assays would be a game-changer. Such a device would allow each blood component to be safely stored under the correct conditions for downstream analysis without compromising the other biologically relevant fractions found within the blood and could simplify pre-analytical workflows.
6. Conclusion and perspectives
Liquid biopsy holds enormous promise in the clinical management of patients with cancer. Through longitudinal, high-sensitivity liquid biopsy, it is possible to detect disease early, track disease burden, monitor response to treatment, and understand disease heterogeneity. However, the ability to perform multiple assays from the one blood draw is still limited. As such, there remains an opportunity for a device that can rapidly isolate plasma and cells from low volume blood draws at the point-of-draw and to use microfluidics to change patient management and provide an important tool for the delivery of better patient outcomes.
By developing new devices that take the most effective parts of existing devices and combining these to simultaneously produce plasma and cellular fractions, it would be possible to provide plasma and cellular fractions compatible with emerging next generation approaches. For example, using a high efficiency system capable of separating cells and plasma at the point-of-draw would allow plasma, cleaned from containing any residual cells, to be safely snap-frozen whilst CTCs and CTC clusters could be collected without the need to use troublesome preservatives that interfere with downstream RNA and cytometric profiling. This would also allow analysis of both ctDNA and CTC from a single blood draw, thereby reducing the total volume of blood required when analysing both CTCs and ctDNA simultaneously. This is illustrated in Fig. 6.
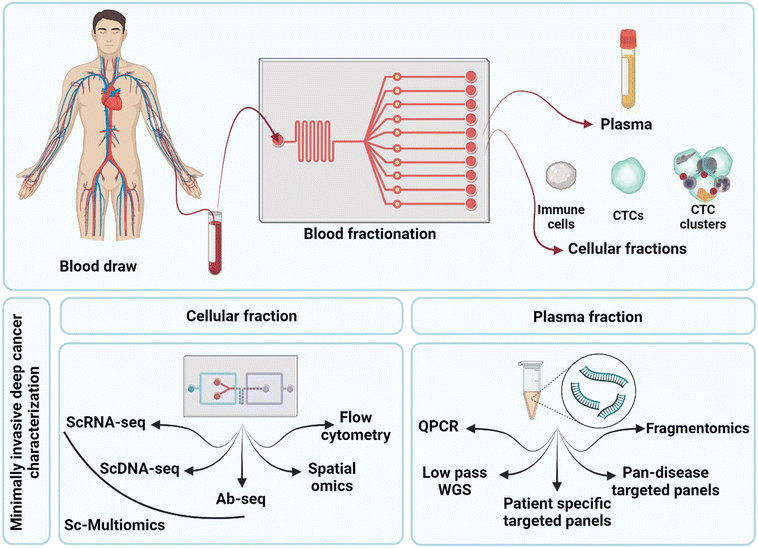 |
| Fig. 6 Workflows for using microfluidics to overcome challenges in liquid biopsy. By enabling the simultaneous separation of plasma and cells from a single blood draw at the point-of-draw, the use of preservatives can be removed, and the cells and ctDNA can be provided in a form compatible with next-generation liquid biopsy approaches. | |
For these devices to be effective they would need to be:
• Robust and produce highly reproducible results.
• Rapid and not induce any biological change to the sample.
• Operable at the point-of-draw with minimal training required.
• Simple enough to ensure that human error is minimised.
• Free from contaminants.
• Ideally disposable to reduce the chance of sample cross contamination.
Finally, to be clinically implementable, they must provide the best cost-to-benefit ratio to patients and the healthcare system. As blood fractionation and sample preparation are significant parts of the process, devices that support this must also be cost effective. The need for cost-effective methods in liquid biopsy is accentuated by the need to perform these assays at multiple time points during the patient's disease journey.
Here, we have outlined the biological relevance of liquid biopsy approaches in the management of cancer and have proposed a new categorisation approach that we believe helps biologists engage with the complex field of the microfluidic devices that are reshaping our ability to fractionate blood and are set to give rise to new capabilities in liquid biopsy. Finally, through a thorough review of the current state of microfluidics in cancer liquid biopsy, we have identified that many of the current devices are not compatible with multimodal, multiomic analysis. As such, we have proposed several requirements for future devices. We believe that devices allowing rapid and robust point-of-draw fractionation of plasma, CTCs, CTC clusters, and immune cells would provide enormous value to the clinical management of cancer and would therefore have significant impact on the lives of cancer patients.
Abbreviations
CTC | Circulating tumour cell |
SNV | Single nucleotide variant |
ctDNA | Circulating tumour DNA |
CNA | Copy number aberration |
cfDNA | Cell free DNA |
NGS | Next generation sequencing |
WGS | Whole genome sequencing |
DTC | Disseminated tumour cell |
Data availability
No data is available for this review article.
Author contributions
RS: conceptualisation, formal analysis, investigation, writing – original draft and editing, project administration, visualisation SRB: conceptualisation, writing – original draft, visualisation, investigation, KM: writing – original draft DGO: writing – review & editing, MW: writing – review & editing, supervision DW: writing – review & editing, supervision DJ: writing – review & editing, funding acquisition, supervision.
Conflicts of interest
The authors declare no conflicts of interest.
Acknowledgements
DGO is supported by the Elaine Henry Fellowship from the National Breast Cancer Foundation, Australia, IIRS-21-096. RS was supported by funding from Medical Research Futures Fund, Australia (EPCD000015). SRB was supported through generous donations from the Charles Warman foundation, Australia and Cancer Institute of New South Wales, Australia. The work at Harvard University was supported in part by the National Science Foundation through the Harvard MRSEC (DMR-2011754). We acknowledge the support of the ACRF Child Cancer Liquid Biopsy Program and the Australian Cancer Research Foundation.
References
- H. Lilja, D. Ulmert and A. J. Vickers, Nat. Rev. Cancer, 2008, 8, 268–278 CrossRef CAS PubMed.
- G. L. Perkins, E. D. Slater, G. K. Sanders and J. G. Prichard, Am. Fam. Physician, 2003, 68, 1075–1082 Search PubMed.
- X. Q. Chen, M. Stroun, J. L. Magnenat, L. P. Nicod, A. M. Kurt, J. Lyautey, C. Lederrey and P. Anker, Nat. Med., 1996, 2, 1033–1035 CrossRef CAS PubMed.
- H. Nawroz, W. Koch, P. Anker, M. Stroun and D. Sidransky, Nat. Med., 1996, 2, 1035–1037 CrossRef CAS.
- H. Osumi, E. Shinozaki, K. Yamaguchi and H. Zembutsu, Sci. Rep., 2019, 9, 17358 CrossRef.
- Y. J. Kim, Y. Kang, J. S. Kim, H. H. Sung, H. G. Jeon, B. C. Jeong, S. I. Seo, S. S. Jeon, H. M. Lee, D. Park, W.-Y. Park and M. Kang, Sci. Rep., 2021, 11, 5600 CrossRef CAS.
- B. Zhou, K. Xu, X. Zheng, T. Chen, J. Wang, Y. Song, Y. Shao and S. Zheng, Signal Transduction Targeted Ther., 2020, 5, 144 CrossRef CAS.
- S. Lin, Z. Yu, D. Chen, Z. Wang, J. Miao, Q. Li, D. Zhang, J. Song and D. Cui, Small, 2020, 16, 1903916 CrossRef CAS.
- F. Tian, C. Liu, J. Deng and J. Sun, Acc. Mater. Res., 2022, 3, 498–510, DOI:10.1021/accountsmr.1c00276.
- B. Lin, Y. Lei, J. Wang, L. Zhu, Y. Wu, H. Zhang, L. Wu, P. Zhang and C. Yang, Small Methods, 2021, 5, 2001131 CrossRef CAS.
- B. M. Szczerba, F. Castro-Giner, M. Vetter, I. Krol, S. Gkountela, J. Landin, M. C. Scheidmann, C. Donato, R. Scherrer, J. Singer, C. Beisel, C. Kurzeder, V. Heinzelmann-Schwarz, C. Rochlitz, W. P. Weber, N. Beerenwinkel and N. Aceto, Nature, 2019, 566, 553–557 CrossRef CAS.
- M. Yu, A. Bardia, B. S. Wittner, S. L. Stott, M. E. Smas, D. T. Ting, S. J. Isakoff, J. C. Ciciliano, M. N. Wells, A. M. Shah, K. F. Concannon, M. C. Donaldson, L. V. Sequist, E. Brachtel, D. Sgroi, J. Baselga, S. Ramaswamy, M. Toner, D. A. Haber and S. Maheswaran, Science, 2013, 339, 580–584 CrossRef CAS.
- N. Aceto, A. Bardia, D. T. Miyamoto, M. C. Donaldson, B. S. Wittner, J. A. Spencer, M. Yu, A. Pely, A. Engstrom, H. Zhu, B. W. Brannigan, R. Kapur, S. L. Stott, T. Shioda, S. Ramaswamy, D. T. Ting, C. P. Lin, M. Toner, D. A. Haber and S. Maheswaran, Cell, 2014, 158, 1110–1122 CrossRef CAS PubMed.
- K. J. Cheung, V. Padmanaban, V. Silvestri, K. Schipper, J. D. Cohen, A. N. Fairchild, M. A. Gorin, J. E. Verdone, K. J. Pienta, J. S. Bader and A. J. Ewald, Proc. Natl. Acad. Sci. U. S. A., 2016, 113, E854–E863 CAS.
- Z. Yu, H. Chen, J. Ai, Y. Zhu, Y. Li, J. A. Borgia, J.-S. Yang, J. Zhang, B. Jiang, W. Gu and Y. Deng, Onco Targets Ther, 2017, 8, 107899–107906 Search PubMed.
- Y. Takanashi, T. Kahyo, K. Sekihara, A. Kawase, M. Setou and K. Funai, Lipids Health Dis., 2024, 23, 154 CrossRef CAS.
- E. S. Gray, H. Rizos, A. L. Reid, S. C. Boyd, M. R. Pereira, J. Lo, V. Tembe, J. Freeman, J. H. Lee, R. A. Scolyer, K. Siew, C. Lomma, A. Cooper, M. A. Khattak, T. M. Meniawy, G. V. Long, M. S. Carlino, M. Millward and M. Ziman, Onco Targets Ther, 2015, 6, 42008–42018 Search PubMed.
- H. Cheng, C. Liu, J. Jiang, G. Luo, Y. Lu, K. Jin, M. Guo, Z. Zhang, J. Xu, L. Liu, Q. Ni and X. Yu, Int. J. Cancer, 2017, 140, 2344–2350 CrossRef CAS PubMed.
- F. Diehl, K. Schmidt, M. A. Choti, K. Romans, S. Goodman, M. Li, K. Thornton, N. Agrawal, L. Sokoll, S. A. Szabo, K. W. Kinzler, B. Vogelstein and L. A. Diaz, Jr., Nat. Med., 2008, 14, 985–990 CrossRef CAS.
- G. Ledergor, A. Weiner, M. Zada, S. Y. Wang, Y. C. Cohen, M. E. Gatt, N. Snir, H. Magen, M. Koren-Michowitz, K. Herzog-Tzarfati, H. Keren-Shaul, C. Bornstein, R. Rotkopf, I. Yofe, E. David, V. Yellapantula, S. Kay, M. Salai, D. Ben Yehuda, A. Nagler, L. Shvidel, A. Orr-Urtreger, K. B. Halpern, S. Itzkovitz, O. Landgren, J. San-Miguel, B. Paiva, J. J. Keats, E. Papaemmanuil, I. Avivi, G. I. Barbash, A. Tanay and I. Amit, Nat Med, 2018, 24, 1867–1876 CrossRef CAS.
- S. D. Wit, G. V. Dalum, A. T. M. Lenferink, A. G. J. Tibbe, T. J. N. Hiltermann, H. J. M. Groen, C. J. M. van Rijn and L. W. M. M. Terstappen, Sci. Rep., 2015, 5, 12270 CrossRef.
- J. M. Riedl, S. O. Hasenleithner, G. Pregartner, L. Scheipner, F. Posch, K. Groller, K. Kashofer, S. W. Jahn, T. Bauernhofer, M. Pichler, H. Stöger, A. Berghold, G. Hoefler, M. R. Speicher, E. Heitzer and A. Gerger, Ther. Adv. Med. Oncol., 2021, 13, 1758835920987658 CrossRef CAS PubMed.
- C. L. Hodgkinson, C. J. Morrow, Y. Li, R. L. Metcalf, D. G. Rothwell, F. Trapani, R. Polanski, D. J. Burt, K. L. Simpson, K. Morris, S. D. Pepper, D. Nonaka, A. Greystoke, P. Kelly, B. Bola, M. G. Krebs, J. Antonello, M. Ayub, S. Faulkner, L. Priest, L. Carter, C. Tate, C. J. Miller, F. Blackhall, G. Brady and C. Dive, Nat. Med., 2014, 20, 897–903 CrossRef CAS PubMed.
- C. Abbosh, N. J. Birkbak, G. A. Wilson, M. Jamal-Hanjani, T. Constantin, R. Salari, J. Le Quesne, D. A. Moore, S. Veeriah, R. Rosenthal, T. Marafioti, E. Kirkizlar, T. B. K. Watkins, N. McGranahan, S. Ward, L. Martinson, J. Riley, F. Fraioli, M. Al Bakir, E. Gronroos, F. Zambrana, R. Endozo, W. L. Bi, F. M. Fennessy, N. Sponer, D. Johnson, J. Laycock, S. Shafi, J. Czyzewska-Khan, A. Rowan, T. Chambers, N. Matthews, S. Turajlic, C. Hiley, S. M. Lee, M. D. Forster, T. Ahmad, M. Falzon, E. Borg, D. Lawrence, M. Hayward, S. Kolvekar, N. Panagiotopoulos, S. M. Janes, R. Thakrar, A. Ahmed, F. Blackhall, Y. Summers, D. Hafez, A. Naik, A. Ganguly, S. Kareht, R. Shah, L. Joseph, A. Marie Quinn, P. A. Crosbie, B. Naidu, G. Middleton, G. Langman, S. Trotter, M. Nicolson, H. Remmen, K. Kerr, M. Chetty, L. Gomersall, D. A. Fennell, A. Nakas, S. Rathinam, G. Anand, S. Khan, P. Russell, V. Ezhil, B. Ismail, M. Irvin-Sellers, V. Prakash, J. F. Lester, M. Kornaszewska, R. Attanoos, H. Adams, H. Davies, D. Oukrif, A. U. Akarca, J. A. Hartley, H. L. Lowe, S. Lock, N. Iles, H. Bell, Y. Ngai, G. Elgar, Z. Szallasi, R. F. Schwarz, J. Herrero, A. Stewart, S. A. Quezada, K. S. Peggs, P. Van Loo, C. Dive, C. J. Lin, M. Rabinowitz, H. Aerts, A. Hackshaw, J. A. Shaw, B. G. Zimmermann, T. R. Consortium, P. Consortium and C. Swanton, Nature, 2017, 545, 446–451 CrossRef CAS PubMed.
- M. Murtaza, S. J. Dawson, D. W. Tsui, D. Gale, T. Forshew, A. M. Piskorz, C. Parkinson, S. F. Chin, Z. Kingsbury, A. S. Wong, F. Marass, S. Humphray, J. Hadfield, D. Bentley, T. M. Chin, J. D. Brenton, C. Caldas and N. Rosenfeld, Nature, 2013, 497, 108–112 CrossRef CAS.
- S. L. Kong, X. Liu, S. J. Tan, J. A. Tai, L. Y. Phua, H. M. Poh, T. Yeo, Y. W. Chua, Y. X. Haw, W. H. Ling, R. C. H. Ng, T. J. Tan, K. W. J. Loh, D. S.-W. Tan, Q. S. Ng, M. K. Ang, C. K. Toh, Y. F. Lee, C. T. Lim, T. K. H. Lim, A. M. Hillmer, Y. S. Yap and W.-T. Lim, Front. Oncol., 2021, 11, 698551, DOI:10.3389/fonc.2021.698551.
- Y. Wang, L. Guo, L. Feng, W. Zhang, T. Xiao, X. Di, G. Chen and K. Zhang, Oncol. Rep., 2018, 39, 2147–2159 CAS.
- E. S. Antonarakis, C. Lu, H. Wang, B. Luber, M. Nakazawa, J. C. Roeser, Y. Chen, T. A. Mohammad, Y. Chen, H. L. Fedor, T. L. Lotan, Q. Zheng, A. M. De Marzo, J. T. Isaacs, W. B. Isaacs, R. Nadal, C. J. Paller, S. R. Denmeade, M. A. Carducci, M. A. Eisenberger and J. Luo, N. Engl. J. Med., 2014, 371, 1028–1038 CrossRef.
- T. T. Kwan, A. Bardia, L. M. Spring, A. Giobbie-Hurder, M. Kalinich, T. Dubash, T. Sundaresan, X. Hong, J. A. LiCausi, U. Ho, E. J. Silva, B. S. Wittner, L. V. Sequist, R. Kapur, D. T. Miyamoto, M. Toner, D. A. Haber and S. Maheswaran, Cancer Discovery, 2018, 8, 1286–1299 CrossRef CAS.
- A. Zviran, R. C. Schulman, M. Shah, S. T. K. Hill, S. Deochand, C. C. Khamnei, D. Maloney, K. Patel, W. Liao, A. J. Widman, P. Wong, M. K. Callahan, G. Ha, S. Reed, D. Rotem, D. Frederick, T. Sharova, B. Miao, T. Kim, G. Gydush, J. Rhoades, K. Y. Huang, N. D. Omans, P. O. Bolan, A. H. Lipsky, C. Ang, M. Malbari, C. F. Spinelli, S. Kazancioglu, A. M. Runnels, S. Fennessey, C. Stolte, F. Gaiti, G. G. Inghirami, V. Adalsteinsson, B. Houck-Loomis, J. Ishii, J. D. Wolchok, G. Boland, N. Robine, N. K. Altorki and D. A. Landau, Nat. Med., 2020, 26, 1114–1124, DOI:10.1038/s41591-020-0915-3.
- J. C. M. Wan, K. Heider, D. Gale, S. Murphy, E. Fisher, F. Mouliere, A. Ruiz-Valdepenas, A. Santonja, J. Morris, D. Chandrananda, A. Marshall, A. B. Gill, P. Y. Chan, E. Barker, G. Young, W. N. Cooper, I. Hudecova, F. Marass, R. Mair, K. M. Brindle, G. D. Stewart, J. E. Abraham, C. Caldas, D. M. Rassl, R. C. Rintoul, C. Alifrangis, M. R. Middleton, F. A. Gallagher, C. Parkinson, A. Durrani, U. McDermott, C. G. Smith, C. Massie, P. G. Corrie and N. Rosenfeld, Sci. Transl. Med., 2020, 12, eaaz8084, DOI:10.1126/scitranslmed.aaz8084.
- J. J. Szymanski, R. T. Sundby, P. A. Jones, D. Srihari, N. Earland, P. K. Harris, W. Feng, F. Qaium, H. Lei, D. Roberts, M. Landeau, J. Bell, Y. Huang, L. Hoffman, M. Spencer, M. B. Spraker, L. Ding, B. C. Widemann, J. F. Shern, A. C. Hirbe and A. A. Chaudhuri, PLoS Med., 2021, 18, e1003734 CrossRef CAS PubMed.
- F. Mouliere, D. Chandrananda, A. M. Piskorz, E. K. Moore, J. Morris, L. B. Ahlborn, R. Mair, T. Goranova, F. Marass, K. Heider, J. C. M. Wan, A. Supernat, I. Hudecova, I. Gounaris, S. Ros, M. Jimenez-Linan, J. Garcia-Corbacho, K. Patel, O. Østrup, S. Murphy, M. D. Eldridge, D. Gale, G. D. Stewart, J. Burge, W. N. Cooper, M. S. van der Heijden, C. E. Massie, C. Watts, P. Corrie, S. Pacey, K. M. Brindle, R. D. Baird, M. Mau-Sørensen, C. A. Parkinson, C. G. Smith, J. D. Brenton and N. Rosenfeld, Sci. Transl. Med., 2018, 10, eaat4921 CrossRef PubMed.
- A. R. Thierry, Cell Genomics, 2023, 3, 100242 CrossRef CAS PubMed.
- S. C. Ding and Y. M. D. Lo, Diagnostics, 2022, 12, 978, DOI:10.3390/diagnostics12040978.
- C. Abbosh, A. M. Frankell, T. Harrison, J. Kisistok, A. Garnett, L. Johnson, S. Veeriah, M. Moreau, A. Chesh, T. L. Chaunzwa, J. Weiss, M. R. Schroeder, S. Ward, K. Grigoriadis, A. Shahpurwalla, K. Litchfield, C. Puttick, D. Biswas, T. Karasaki, J. R. M. Black, C. Martínez-Ruiz, M. A. Bakir, O. Pich, T. B. K. Watkins, E. L. Lim, A. Huebner, D. A. Moore, N. Godin-Heymann, A. L'Hernault, H. Bye, A. Odell, P. Roberts, F. Gomes, A. J. Patel, E. Manzano, C. T. Hiley, N. Carey, J. Riley, D. E. Cook, D. Hodgson, D. Stetson, J. C. Barrett, R. M. Kortlever, G. I. Evan, A. Hackshaw, R. D. Daber, J. A. Shaw, H. Aerts, A. Licon, J. Stahl, M. Jamal-Hanjani, N. J. Birkbak, N. McGranahan and C. Swanton, Nature, 2023, 616, 553–562 CrossRef CAS PubMed.
- K. Chen, F. Yang, H. Shen, C. Wang, X. Li, O. Chervova, S. Wu, F. Qiu, D. Peng, X. Zhu, S. Chuai, S. Beck, N. Kanu, D. Carbone, Z. Zhang and J. Wang, Cancer Cell, 2023, 41, 1749–1762 CrossRef CAS PubMed , e1746.
- S.-B. Ryoo, S. Heo, Y. Lim, W. Lee, S. H. Cho, J. Ahn, J.-K. Kang, S. Y. Kim, H.-P. Kim, D. Bang, S.-B. Kang, C. S. Yu, S. T. Oh, J. W. Park, S.-Y. Jeong, Y.-J. Kim, K. J. Park, S.-W. Han and T.-Y. Kim, Br. J. Cancer, 2023, 129, 374–381 CrossRef CAS PubMed.
- S. B. Ng, C. Chua, M. Ng, A. Gan, P. S. Y. Poon, M. Teo, C. Fu, W. Q. Leow, K. H. Lim, A. Chung, S.-L. Koo, S. P. Choo, D. Ho, S. Rozen, P. Tan, M. Wong, W. F. Burkholder and I. B. Tan, Sci. Rep., 2017, 7, 40737 CrossRef CAS PubMed.
- A. Santonja, W. N. Cooper, M. D. Eldridge, P. A. W. Edwards, J. A. Morris, A. R. Edwards, H. Zhao, K. Heider, D. L. Couturier, A. Vijayaraghavan, P. Mennea, E. J. Ditter, C. G. Smith, C. Boursnell, R. Manzano García, O. M. Rueda, E. Beddowes, H. Biggs, S. J. Sammut, N. Rosenfeld, C. Caldas, J. E. Abraham and D. Gale, EMBO Mol. Med., 2023, 15, e16505 CrossRef CAS PubMed.
- M. Stoeckius, C. Hafemeister, W. Stephenson, B. Houck-Loomis, P. K. Chattopadhyay, H. Swerdlow, R. Satija and P. Smibert, Nat. Methods, 2017, 14, 865–868 CrossRef CAS PubMed.
- E. Z. Macosko, A. Basu, R. Satija, J. Nemesh, K. Shekhar, M. Goldman, I. Tirosh, A. R. Bialas, N. Kamitaki, E. M. Martersteck, J. J. Trombetta, D. A. Weitz, J. R. Sanes, A. K. Shalek, A. Regev and S. A. McCarroll, Cell, 2015, 161, 1202–1214 CrossRef CAS PubMed.
- R. Zilionis, J. Nainys, A. Veres, V. Savova, D. Zemmour, A. M. Klein and L. Mazutis, Nat. Protoc., 2017, 12, 44–73 CrossRef CAS PubMed.
- M. Cristofanilli, G. T. Budd, M. J. Ellis, A. Stopeck, J. Matera, M. C. Miller, J. M. Reuben, G. V. Doyle, W. J. Allard, L. W. Terstappen and D. F. Hayes, N. Engl. J. Med., 2004, 351, 781–791 CrossRef CAS PubMed.
- N. Aceto, Onco Targets Ther, 2019, 10, 2658–2659 Search PubMed.
- J. G. Lohr, V. A. Adalsteinsson, K. Cibulskis, A. D. Choudhury, M. Rosenberg, P. Cruz-Gordillo, J. M. Francis, C. Z. Zhang, A. K. Shalek, R. Satija, J. J. Trombetta, D. Lu, N. Tallapragada, N. Tahirova, S. Kim, B. Blumenstiel, C. Sougnez, A. Lowe, B. Wong, D. Auclair, E. M. Van Allen, M. Nakabayashi, R. T. Lis, G. S. Lee, T. Li, M. S. Chabot, A. Ly, M. E. Taplin, T. E. Clancy, M. Loda, A. Regev, M. Meyerson, W. C. Hahn, P. W. Kantoff, T. R. Golub, G. Getz, J. S. Boehm and J. C. Love, Nat. Biotechnol., 2014, 32, 479–484 CrossRef CAS.
- V. Cappelletti, E. Verzoni, R. Ratta, M. Vismara, M. Silvestri, R. Montone, P. Miodini, C. Reduzzi, M. Claps, P. Sepe, M. G. Daidone and G. Procopio, Int. J. Mol. Sci., 2020, 21, 1475, DOI:10.3390/ijms21041475.
- M. Chimonidou, A. Strati, A. Tzitzira, G. Sotiropoulou, N. Malamos, V. Georgoulias and E. S. Lianidou, Clin. Chem., 2011, 57, 1169–1177 CrossRef CAS PubMed.
- L. Zhao, X. Wu, J. Zheng and D. Dong, Oncogene, 2021, 40, 1884–1895 CrossRef CAS PubMed.
- S. Mastoraki, A. Strati, E. Tzanikou, M. Chimonidou, E. Politaki, A. Voutsina, A. Psyrri, V. Georgoulias and E. Lianidou, Clin. Cancer Res., 2018, 24, 1500–1510 CrossRef CAS PubMed.
- S. Gkountela, F. Castro-Giner, B. M. Szczerba, M. Vetter, J. Landin, R. Scherrer, I. Krol, M. C. Scheidmann, C. Beisel, C. U. Stirnimann, C. Kurzeder, V. Heinzelmann-Schwarz, C. Rochlitz, W. P. Weber and N. Aceto, Cell, 2019, 176(98–112), e114 Search PubMed.
- I. Krol, F. Castro-Giner, M. Maurer, S. Gkountela, B. M. Szczerba, R. Scherrer, N. Coleman, S. Carreira, F. Bachmann, S. Anderson, M. Engelhardt, H. Lane, T. R. J. Evans, R. Plummer, R. Kristeleit, J. Lopez and N. Aceto, Br. J. Cancer, 2018, 119, 487–491 CrossRef CAS PubMed.
- A. R. Thierry, Cell Genomics, 2023, 3(1), 100242 CrossRef CAS PubMed.
- S. A. Leon, B. Shapiro, D. M. Sklaroff and M. J. Yaros, Cancer Res., 1977, 37, 646–650 CAS.
- F. Diehl, M. Li, Y. He, K. W. Kinzler, B. Vogelstein and D. Dressman, Nat. Methods, 2006, 3, 551–559 CrossRef CAS PubMed.
- R. Jiang, Y. T. Lu, H. Ho, B. Li, J. F. Chen, M. Lin, F. Li, K. Wu, H. Wu, J. Lichterman, H. Wan, C. L. Lu, W. OuYang, M. Ni, L. Wang, G. Li, T. Lee, X. Zhang, J. Yang, M. Rettig, L. W. Chung, H. Yang, K. C. Li, Y. Hou, H. R. Tseng, S. Hou, X. Xu, J. Wang and E. M. Posadas, Onco Targets Ther, 2015, 6, 44781–44793 Search PubMed.
- H. Peng, L. Lu, Z. Zhou, J. Liu, D. Zhang, K. Nan, X. Zhao, F. Li, L. Tian, H. Dong and Y. Yao, Genes, 2019, 10, 926 CrossRef CAS PubMed.
- T. E. Liggett, A. A. Melnikov, J. R. Marks and V. V. Levenson, Int. J. Cancer, 2011, 128, 492–499 CrossRef CAS PubMed.
- F. Nassiri, A. Chakravarthy, S. Feng, S. Y. Shen, R. Nejad, J. A. Zuccato, M. R. Voisin, V. Patil, C. Horbinski, K. Aldape, G. Zadeh and D. D. De Carvalho, Nat. Med., 2020, 26, 1044–1047 CrossRef CAS PubMed.
- N. C. Turner, B. Kingston, L. S. Kilburn, S. Kernaghan, A. M. Wardley, I. R. Macpherson, R. D. Baird, R. Roylance, P. Stephens, O. Oikonomidou, J. P. Braybrooke, M. Tuthill, J. Abraham, M. C. Winter, H. Bye, M. Hubank, H. Gevensleben, R. Cutts, C. Snowdon, D. Rea, D. Cameron, A. Shaaban, K. Randle, S. Martin, K. Wilkinson, L. Moretti, J. M. Bliss and A. Ring, Lancet Oncol., 2020, 21, 1296–1308 CrossRef CAS PubMed.
- F. Cimmino, V. A. Lasorsa, S. Vetrella, A. Iolascon and M. Capasso, Front. Oncol., 2020, 10, 596191, DOI:10.3389/fonc.2020.596191.
- S. Bang, D. Won, S. Shin, K. S. Cho, J. W. Park, J. Lee, Y. D. Choi, S. Kang, S. T. Lee, J. R. Choi and H. Han, Cancers, 2023, 15, 3998, DOI:10.3390/cancers15153998.
- M. Kohli, W. Tan, T. Zheng, A. Wang, C. Montesinos, C. Wong, P. Du, S. Jia, S. Yadav, L. G. Horvath, K. L. Mahon, E. M. Kwan, H. Fettke, J. Yu and A. A. Azad, EBioMedicine, 2020, 54, 102728, DOI:10.1016/j.ebiom.2020.102728.
- J. Tie, Y. Wang, C. Tomasetti, L. Li, S. Springer, I. Kinde, N. Silliman, M. Tacey, H.-L. Wong, M. Christie, S. Kosmider, I. Skinner, R. Wong, M. Steel, B. Tran, J. Desai, I. Jones, A. Haydon, T. Hayes, T. J. Price, R. L. Strausberg, L. A. Diaz, N. Papadopoulos, K. W. Kinzler, B. Vogelstein and P. Gibbs, Sci. Transl. Med., 2016, 8, 346ra392-346ra392 Search PubMed.
- R. C. Fitzgerald, A. C. Antoniou, L. Fruk and N. Rosenfeld, Nat. Med., 2022, 28, 666–677 CrossRef CAS PubMed.
- Y.-M. Yeh, P.-C. Lin, C.-T. Lee, S.-H. Chen, B.-W. Lin, S.-C. Lin, P.-C. Chen, R.-H. Chan and M.-R. Shen, Mol. Cancer, 2020, 19, 150 CrossRef CAS PubMed.
- S. Nikolaev, L. Lemmens, T. Koessler, J.-L. Blouin and T. Nouspikel, Anal. Biochem., 2018, 542, 34–39 CrossRef CAS PubMed.
- Y. Zhao, Y. Li, P. Chen, S. Li, J. Luo and H. Xia, J. Clin. Lab. Anal., 2019, 33, e22670–e22670 CrossRef PubMed.
- F. Mouliere and N. Rosenfeld, Proc. Natl. Acad. Sci. U. S. A., 2015, 112, 3178–3179 CrossRef CAS PubMed.
- T. R. Ashworth, Aust. J. Med., 1869, 14, 146–147 Search PubMed.
- T. P. Leitão, P. Corredeira, S. Kucharczak, M. Rodrigues, P. Piairo, C. Rodrigues, P. Alves, A. M. Cavaco, M. Miranda, M. Antunes, J. Ferreira, J. Palma Reis, T. Lopes, L. Diéguez and L. Costa, Int. J. Mol. Sci., 2023, 24, 8404 CrossRef PubMed.
- F. Jin, L. Zhu, J. Shao, M. Yakoub, L. Schmitt, C. Reißfelder, S. Loges, A. Benner and S. Schölch, Eur. Respir. J., 2022, 31, 220151 CrossRef PubMed.
- J. Müller Bark, A. Kulasinghe, G. Hartel, P. Leo, M. E. Warkiani, R. L. Jeffree, B. Chua, B. W. Day and C. Punyadeera, Front. Oncol., 2021, 11, 681130, DOI:10.3389/fonc.2021.681130.
- M. Kojima, T. Harada, T. Fukazawa, S. Kurihara, R. Touge, I. Saeki, S. Takahashi and E. Hiyama, Cancer Sci., 2023, 114, 1616–1624 CrossRef CAS PubMed.
- M. Hayashi, P. Zhu, G. McCarty, C. F. Meyer, C. A. Pratilas, A. Levin, C. D. Morris, C. M. Albert, K. W. Jackson, C.-M. Tang and D. M. Loeb, Onco Targets Ther, 2017, 8, 78965–78977, DOI:10.18632/oncotarget.20697.
- C. Paoletti, A. K. Cani, J. M. Larios, D. H. Hovelson, K. Aung, E. P. Darga, E. M. Cannell, P. J. Baratta, C.-J. Liu, D. Chu, M. Yazdani, A. R. Blevins, V. Sero, N. Tokudome, D. G. Thomas, C. Gersch, A. F. Schott, Y.-M. Wu, R. Lonigro, D. R. Robinson, A. M. Chinnaiyan, F. Z. Bischoff, M. D. Johnson, B. H. Park, D. F. Hayes, J. M. Rae and S. A. Tomlins, Cancer Res., 2018, 78, 1110–1122 CrossRef CAS PubMed.
- D. Ramsköld, S. Luo, Y.-C. Wang, R. Li, Q. Deng, O. R. Faridani, G. A. Daniels, I. Khrebtukova, J. F. Loring, L. C. Laurent, G. P. Schroth and R. Sandberg, Nat. Biotechnol., 2012, 30, 777–782 CrossRef PubMed.
- G. T. Budd, M. Cristofanilli, M. J. Ellis, A. Stopeck, E. Borden, M. C. Miller, J. Matera, M. Repollet, G. V. Doyle, L. W. Terstappen and D. F. Hayes, Clin. Cancer Res., 2006, 12, 6403–6409 CrossRef CAS PubMed.
- M. G. Krebs, J.-M. Hou, T. H. Ward, F. H. Blackhall and C. Dive, Ther. Adv. Med. Oncol., 2010, 2, 351–365 CrossRef PubMed.
- S. Pang, H. Li, S. Xu, L. Feng, X. Ma, Y. Chu, B. Zou, S. Wang and G. Zhou, Sci. Rep., 2021, 11, 13441 CrossRef CAS PubMed.
- D. W. Ross, L. H. Ayscue, J. Watson and S. A. Bentley, Am. J. Clin. Pathol., 1988, 90, 262–267 CrossRef CAS PubMed.
- M. Diez-Silva, M. Dao, J. Han, C. T. Lim and S. Suresh, MRS Bull., 2010, 35, 382–388 CrossRef CAS PubMed.
- H. W. Hou, A. A. S. Bhagat, W. C. Lee, S. Huang, J. Han and C. T. Lim, Micromachines, 2011, 2, 319–343 CrossRef.
- W. J. Allard, J. Matera, M. C. Miller, M. Repollet, M. C. Connelly, C. Rao, A. G. J. Tibbe, J. W. Uhr and L. W. M. M. Terstappen, Clin. Cancer Res., 2004, 10, 6897–6904 CrossRef PubMed.
- S. L. Stott, R. J. Lee, S. Nagrath, M. Yu, D. T. Miyamoto, L. Ulkus, E. J. Inserra, M. Ulman, S. Springer, Z. Nakamura, A. L. Moore, D. I. Tsukrov, M. E. Kempner, D. M. Dahl, C.-L. Wu, A. J. Iafrate, M. R. Smith, R. G. Tompkins, L. V. Sequist, M. Toner, D. A. Haber and S. Maheswaran, Sci. Transl. Med., 2010, 2, 25ra23 Search PubMed.
- S. Meng, D. Tripathy, E. P. Frenkel, S. Shete, E. Z. Naftalis, J. F. Huth, P. D. Beitsch, M. Leitch, S. Hoover, D. Euhus, B. Haley, L. Morrison, T. P. Fleming, D. Herlyn, L. W. M. M. Terstappen, T. Fehm, T. F. Tucker, N. Lane, J. Wang and J. W. Uhr, Clin. Cancer Res., 2004, 10, 8152–8162 CrossRef PubMed.
- R. M. Trigg, L. J. Martinson, S. Parpart-Li and J. A. Shaw, Heliyon, 2018, 4, e00699, DOI:10.1016/j.heliyon.2018.e00699.
- P. Sethu, M. Anahtar, L. L. Moldawer, R. G. Tompkins and M. Toner, Anal. Chem., 2004, 76, 6247–6253 CrossRef CAS PubMed.
- S. Miltenyi, W. Muller, W. Weichel and A. Radbruch, Cytometry, 1990, 11, 231–238 CrossRef CAS PubMed.
- H. R. Hulett, W. A. Bonner, J. Barrett and L. A. Herzenberg, Science, 1969, 166, 747–749 CrossRef CAS PubMed.
- L. A. Herzenberg, R. G. Sweet and L. A. Herzenberg, Sci. Am., 1976, 234, 108–118 CrossRef CAS PubMed.
- J. L. Sherwood, C. Corcoran, H. Brown, A. D. Sharpe, M. Musilova and A. Kohlmann, PLoS One, 2016, 11, e0150197 CrossRef PubMed.
- M. Fleischhacker and B. Schmidt, J. Lab. Med., 2020, 44, 117–142 CrossRef.
- M. A. Kerachian, M. Azghandi, S. Mozaffari-Jovin and A. R. Thierry, Clin. Epigenet., 2021, 13, 193 CrossRef CAS PubMed.
- A. Ward Gahlawat, J. Lenhardt, T. Witte, D. Keitel, A. Kaufhold, K. K. Maass, K. W. Pajtler, C. Sohn and S. Schott, Int. J. Mol. Sci., 2019, 20, 704 CrossRef PubMed.
- L. Sorber, K. Zwaenepoel, J. Jacobs, K. De Winne, S. Goethals, P. Reclusa, K. Van Casteren, E. Augustus, F. Lardon, G. Roeyen, M. Peeters, J. Van Meerbeeck, C. Rolfo and P. Pauwels, Cancers, 2019, 11, 458 CrossRef CAS PubMed.
- D. Grolz, S. Hauch, M. Schlumpberger, K. Guenther, T. Voss, M. Sprenger-Haussels and U. Oelmuller, Curr. Pathobiol. Rep., 2018, 6, 275–286 CrossRef PubMed.
- H. Cho, J. Kim, H. Song, K. Y. Sohn, M. Jeon and K.-H. Han, Analyst, 2018, 143, 2936–2970 RSC.
- L. Descamps, D. Le Roy and A. L. Deman, Int. J. Mol. Sci., 2022, 23, 1981, DOI:10.3390/ijms23041981.
- S.-J. Hao, Y. Wan, Y.-Q. Xia, X. Zou and S.-Y. Zheng, Adv. Drug Delivery Rev., 2018, 125, 3–20 CrossRef CAS PubMed.
- T. A. Crowley and V. Pizziconi, Lab Chip, 2005, 5, 922–929 RSC.
- J. A. Davis, D. W. Inglis, K. J. Morton, D. A. Lawrence, L. R. Huang, S. Y. Chou, J. C. Sturm and R. H. Austin, Proc. Natl. Acad. Sci. U. S. A., 2006, 103, 14779–14784 CrossRef CAS PubMed.
- S. Yang, A. Undar and J. D. Zahn, Lab Chip, 2006, 6, 871–880 RSC.
- S. Tripathi, Y. V. Kumar, A. Agrawal, A. Prabhakar and S. S. Joshi, Sci. Rep., 2016, 6, 26749 CrossRef CAS PubMed.
- A. Lenshof, A. Ahmad-Tajudin, K. Järås, A.-M. Swärd-Nilsson, L. Åberg, G. Marko-Varga, J. Malm, H. Lilja and T. Laurell, Anal. Chem., 2009, 81, 6030–6037 CrossRef CAS PubMed.
- J. S. Shim, A. W. Browne and C. H. Ahn, Biomed. Microdevices, 2010, 12, 949–957 CrossRef PubMed.
- M. G. Lee, S. Choi, H.-J. Kim, H. K. Lim, J.-H. Kim, N. Huh and J.-K. Park, Appl. Phys. Lett., 2011, 98, 253702 CrossRef.
- C. Szydzik, K. Khoshmanesh, A. Mitchell and C. Karnutsch, Biomicrofluidics, 2015, 9, 064120 CrossRef PubMed.
- X. Xing, M. He, H. Qiu and L. Yobas, Anal. Chem., 2016, 88, 5197–5204 CrossRef CAS PubMed.
- H. Jang, M. R. Haq, J. Ju, Y. Kim, S.-m. Kim and J. Lim, Micromachines, 2017, 8, 67 CrossRef.
- C.-J. Kim, J. Park, V. Sunkara, T.-H. Kim, Y. Lee, K. Lee, M.-H. Kim and Y.-K. Cho, Lab Chip, 2018, 18, 1320–1329 RSC.
- Q. Gao, Y. Chang, Q. Deng and H. You, Anal. Methods, 2020, 12, 2560–2570 RSC.
- W. Brakewood, K. Lee, L. Schneider, N. Lawandy and A. Tripathi, Front. Lab. Chip. Technol., 2022, 1, 1051552, DOI:10.3389/frlct.2022.1051552.
- E. Sollier, M. Cubizolles, Y. Fouillet and J.-L. Achard, Biomed. Microdevices, 2010, 12, 485–497 CrossRef PubMed.
- D. H. Kuan, C. C. Wu, W. Y. Su and N. T. Huang, Sci. Rep., 2018, 8, 15345 CrossRef PubMed.
- Y. Liu, W. Zhao, R. Cheng, J. Hodgson, M. Egan, C. N. C. Pope, P. G. Nikolinakos and L. Mao, Lab Chip, 2021, 21, 3583–3597 RSC.
- M. Boya, T. Ozkaya-Ahmadov, B. E. Swain, C.-H. Chu, N. Asmare, O. Civelekoglu, R. Liu, D. Lee, S. Tobia, S. Biliya, L. D. McDonald, B. Nazha, O. Kucuk, M. G. Sanda, B. B. Benigno, C. S. Moreno, M. A. Bilen, J. F. McDonald and A. F. Sarioglu, Nat. Commun., 2022, 13, 3385 CrossRef CAS PubMed.
- H. J. Woo, S. H. Kim, H. J. Kang, S. H. Lee, S. J. Lee, J. M. Kim, O. Gurel, S. Y. Kim, H. R. Roh, J. Lee, Y. Park, H. Y. Shin, Y. I. Shin, S. M. Lee, S. Y. Oh, Y. Z. Kim, J. I. Chae, S. Lee, M. H. Hong, B. C. Cho, E. S. Lee, K. Pantel, H. R. Kim and M. S. Kim, Theranostics, 2022, 12, 3676–3689 CrossRef CAS PubMed.
- Z. Zhu, H. Ren, D. Wu, Z. Ni and N. Xiang, Microsyst. Nanoeng., 2024, 10, 36 CrossRef CAS PubMed.
- W. Pakhira, R. Kumar and K. M. Ibrahimi, Chem. Eng. Sci., 2023, 275, 118724 CrossRef CAS.
- V. Omrani, M. Z. Targhi, F. Rahbarizadeh and R. Nosrati, Sci. Rep., 2023, 13, 3213 CrossRef CAS PubMed.
- Y. Lu, S. Yue, M. Liang, T. Wang, R. Wang, Z. Chen and J. Fang, Sens. Actuators, B, 2023, 393, 134174 CrossRef CAS.
- R. M. Mohamadi, J. D. Besant, A. Mepham, B. Green, L. Mahmoudian, T. Gibbs, I. Ivanov, A. Malvea, J. Stojcic, A. L. Allan, L. E. Lowes, E. H. Sargent, R. K. Nam and S. O. Kelley, Angew. Chem., Int. Ed., 2015, 54, 139–143 CrossRef CAS PubMed.
- M. C. Miller, P. S. Robinson, C. Wagner and D. J. O'Shannessy, Cytometry, Part A, 2018, 93, 1234–1239 CrossRef PubMed.
- L. Xu, X. Mao, A. Imrali, F. Syed, K. Mutsvangwa, D. Berney, P. Cathcart, J. Hines, J. Shamash and Y. J. Lu, PLoS One, 2015, 10, e0138032 CrossRef PubMed.
- M. E. Warkiani, G. Guan, K. B. Luan, W. C. Lee, A. A. Bhagat, P. K. Chaudhuri, D. S. Tan, W. T. Lim, S. C. Lee, P. C. Chen, C. T. Lim and J. Han, Lab Chip, 2014, 14, 128–137 RSC.
- S. Ribeiro-Samy, M. I. Oliveira, T. Pereira-Veiga, L. Muinelo-Romay, S. Carvalho, J. Gaspar, P. P. Freitas, R. López-López, C. Costa and L. Diéguez, Sci. Rep., 2019, 9, 8032 CrossRef PubMed.
- R. Riahi, P. Gogoi, S. Sepehri, Y. Zhou, K. Handique, J. Godsey and Y. Wang, Int. J. Oncol., 2014, 44, 1870–1878 CrossRef PubMed.
- P. Gogoi, S. Sepehri, Y. Zhou, M. A. Gorin, C. Paolillo, E. Capoluongo, K. Gleason, A. Payne, B. Boniface, M. Cristofanilli, T. M. Morgan, P. Fortina, K. J. Pienta, K. Handique and Y. Wang, PLoS One, 2016, 11, e0147400 CrossRef PubMed.
- E. Sollier, D. E. Go, J. Che, D. R. Gossett, S. O'Byrne, W. M. Weaver, N. Kummer, M. Rettig, J. Goldman, N. Nickols, S. McCloskey, R. P. Kulkarni and D. Di Carlo, Lab Chip, 2014, 14, 63–77 RSC.
- V. Murlidhar, M. Zeinali, S. Grabauskiene, M. Ghannad-Rezaie, M. S. Wicha, D. M. Simeone, N. Ramnath, R. M. Reddy and S. Nagrath, Small, 2014, 10, 4895–4904 CrossRef CAS PubMed.
- A. F. Sarioglu, N. Aceto, N. Kojic, M. C. Donaldson, M. Zeinali, B. Hamza, A. Engstrom, H. Zhu, T. K. Sundaresan, D. T. Miyamoto, X. Luo, A. Bardia, B. S. Wittner, S. Ramaswamy, T. Shioda, D. T. Ting, S. L. Stott, R. Kapur, S. Maheswaran, D. A. Haber and M. Toner, Nat. Methods, 2015, 12, 685–691 CrossRef CAS PubMed.
- Y. T. Lu, L. Zhao, Q. Shen, M. A. Garcia, D. Wu, S. Hou, M. Song, X. Xu, W. H. Ouyang, W. W. Ouyang, J. Lichterman, Z. Luo, X. Xuan, J. Huang, L. W. Chung, M. Rettig, H. R. Tseng, C. Shao and E. M. Posadas, Methods, 2013, 64, 144–152 CrossRef CAS PubMed.
- A. Abdulla, T. Zhang, S. Li, W. Guo, A. R. Warden, Y. Xin, N. Maboyi, J. Lou, H. Xie and X. Ding, Microsyst. Nanoeng., 2022, 8, 13 CrossRef CAS PubMed.
- F. D. Schwab, M. C. Scheidmann, L. L. Ozimski, A. Kling, L. Armbrecht, T. Ryser, I. Krol, K. Strittmatter, B. D. Nguyen-Sträuli, F. Jacob, A. Fedier, V. Heinzelmann-Schwarz, A. Wicki, P. S. Dittrich and N. Aceto, Microsyst. Nanoeng., 2022, 8, 130 CrossRef CAS PubMed.
- S. H. Au, J. Edd, A. E. Stoddard, K. H. K. Wong, F. Fachin, S. Maheswaran, D. A. Haber, S. L. Stott, R. Kapur and M. Toner, Sci. Rep., 2017, 7, 2433 CrossRef PubMed.
- S. C. Hur, A. J. Mach and D. Di Carlo, Biomicrofluidics, 2011, 5, 022206 CrossRef PubMed.
- S. L. Stott, C.-H. Hsu, D. I. Tsukrov, M. Yu, D. T. Miyamoto, B. A. Waltman, S. M. Rothenberg, A. M. Shah, M. E. Smas, G. K. Korir, F. P. Floyd, A. J. Gilman, J. B. Lord, D. Winokur, S. Springer, D. Irimia, S. Nagrath, L. V. Sequist, R. J. Lee, K. J. Isselbacher, S. Maheswaran, D. A. Haber and M. Toner, Proc. Natl. Acad. Sci. U. S. A., 2010, 107, 18392–18397 CrossRef CAS PubMed.
- N. Xiang, J. Wang, Q. Li, Y. Han, D. Huang and Z. Ni, Anal. Chem., 2019, 91, 10328–10334 CrossRef CAS PubMed.
- S. Nagrath, L. V. Sequist, S. Maheswaran, D. W. Bell, D. Irimia, L. Ulkus, M. R. Smith, E. L. Kwak, S. Digumarthy, A. Muzikansky, P. Ryan, U. J. Balis, R. G. Tompkins, D. A. Haber and M. Toner, Nature, 2007, 450, 1235–1239 CrossRef CAS PubMed.
- J. P. Gleghorn, E. D. Pratt, D. Denning, H. Liu, N. H. Bander, S. T. Tagawa, D. M. Nanus, P. A. Giannakakou and B. J. Kirby, Lab Chip, 2010, 10, 27–29 RSC.
- H. J. Yoon, T. H. Kim, Z. Zhang, E. Azizi, T. M. Pham, C. Paoletti, J. Lin, N. Ramnath, M. S. Wicha, D. F. Hayes, D. M. Simeone and S. Nagrath, Nat. Nanotechnol., 2013, 8, 735–741 CrossRef CAS PubMed.
- Y. H. Cheng, Y. C. Chen, E. Lin, R. Brien, S. Jung, Y. T. Chen, W. Lee, Z. Hao, S. Sahoo, H. Min Kang, J. Cong, M. Burness, S. Nagrath, M. S. Wicha and E. Yoon, Nat. Commun., 2019, 10, 2163 CrossRef PubMed.
- F. Olm, A. Urbansky, J. H. Dykes, T. Laurell and S. Scheding, Sci. Rep., 2019, 9, 8777 CrossRef PubMed.
- M. Labib, Z. Wang, S. U. Ahmed, R. M. Mohamadi, B. Duong, B. Green, E. H. Sargent and S. O. Kelley, Nat. Biomed. Eng., 2021, 5, 41–52 CrossRef CAS PubMed.
- C. H. Chu, R. Liu, T. Ozkaya-Ahmadov, M. Boya, B. E. Swain, J. M. Owens, E. Burentugs, M. A. Bilen, J. F. McDonald and A. F. Sarioglu, Lab Chip, 2019, 19, 3427–3437 RSC.
- F. Fachin, P. Spuhler, J. M. Martel-Foley, J. F. Edd, T. A. Barber, J. Walsh, M. Karabacak, V. Pai, M. Yu, K. Smith, H. Hwang, J. Yang, S. Shah, R. Yarmush, L. V. Sequist, S. L. Stott, S. Maheswaran, D. A. Haber, R. Kapur and M. Toner, Sci. Rep., 2017, 7, 10936 CrossRef PubMed.
- Z. Liu, Y. Huang, W. Liang, J. Bai, H. Feng, Z. Fang, G. Tian, Y. Zhu, H. Zhang, Y. Wang, A. Liu and Y. Chen, Lab Chip, 2021, 21, 2281–2891, 10.1039/D1LC00360G.
- J. F. Edd, A. Mishra, T. D. Dubash, S. Herrera, R. Mohammad, E. K. Williams, X. Hong, B. R. Mutlu, J. R. Walsh, F. Machado de Carvalho, B. Aldikacti, L. T. Nieman, S. L. Stott, R. Kapur, S. Maheswaran, D. A. Haber and M. Toner, Lab Chip, 2020, 20, 558–567 RSC.
- M. Dhar, E. Pao, C. Renier, D. E. Go, J. Che, R. Montoya, R. Conrad, M. Matsumoto, K. Heirich, M. Triboulet, J. Rao, S. S. Jeffrey, E. B. Garon, J. Goldman, N. P. Rao, R. Kulkarni, E. Sollier-Christen and D. Di Carlo, Sci. Rep., 2016, 6, 35474 CrossRef CAS PubMed.
- S. J. Tan, L. Yobas, G. Y. H. Lee, C. N. Ong and C. T. Lim, Biomed. Microdevices, 2009, 11, 883–892 CrossRef PubMed.
- T.-H. Kim, M. Lim, J. Park, J. M. Oh, H. Kim, H. Jeong, S. J. Lee, H. C. Park, S. Jung, B. C. Kim, K. Lee, M.-H. Kim, D. Y. Park, G. H. Kim and Y.-K. Cho, Anal. Chem., 2017, 89, 1155–1162 CrossRef CAS PubMed.
- A. Lee, J. Park, M. Lim, V. Sunkara, S. Y. Kim, G. H. Kim, M.-H. Kim and Y.-K. Cho, Anal. Chem., 2014, 86, 11349–11356 CrossRef CAS PubMed.
- J.-M. Park, M. S. Kim, H.-S. Moon, C. E. Yoo, D. Park, Y. J. Kim, K.-Y. Han, J.-Y. Lee, J. H. Oh, S. S. Kim, W.-Y. Park, W.-Y. Lee and N. Huh, Anal. Chem., 2014, 86, 3735–3742 CrossRef CAS PubMed.
- J. Zhou, A. Kulasinghe, A. Bogseth, K. O'Byrne, C. Punyadeera and I. Papautsky, Microsyst. Nanoeng., 2019, 5, 8 CrossRef PubMed.
- H. W. Hou, M. E. Warkiani, B. L. Khoo, Z. R. Li, R. A. Soo, D. S.-W. Tan, W.-T. Lim, J. Han, A. A. S. Bhagat and C. T. Lim, Sci. Rep., 2013, 3, 1259 CrossRef PubMed.
- A. Kulasinghe, J. Zhou, L. Kenny, I. Papautsky and C. Punyadeera, Cancers, 2019, 11, 89, DOI:10.3390/cancers11010089.
- M.-D. Chen, Y.-T. Yang, Z.-Y. Deng, H.-Y. Xu, J.-N. Deng, Z. Yang, N. Hu and J. Yang, Chin. J. Anal. Chem., 2019, 47, 661–668 CAS.
- P. C. Chen, C. C. Chen and K. C. Young, Biomicrofluidics, 2016, 10, 054112 CrossRef PubMed.
- X. Su, J. Zhang, D. Zhang, Y. Wang, M. Chen, Z. Weng, J. Wang, J. Zeng, Y. Zhang, S. Zhang, S. Ge, J. Zhang and N. Xia, Micromachines, 2020, 11, 352, DOI:10.3390/mi11040352.
- Y. Nakashima, S. Hata and T. Yasuda, Sens. Actuators, B, 2010, 145, 561–569 CrossRef CAS.
- A. Piovesan, M. C. Pelleri, F. Antonaros, P. Strippoli, M. Caracausi and L. Vitale, BMC Res. Notes, 2019, 12, 106 CrossRef PubMed.
- L. R. Huang, E. C. Cox, R. H. Austin and J. C. Sturm, Science, 2004, 304, 987–990 CrossRef CAS PubMed.
- R. Vernekar, T. Kruger, K. Loutherback, K. Morton and D. W. Inglis, Lab Chip, 2017, 17, 3318–3330 RSC.
- S. H. Au, J. Edd, A. E. Stoddard, K. H. K. Wong, F. Fachin, S. Maheswaran, D. A. Haber, S. L. Stott, R. Kapur and M. Toner, Sci. Rep., 2017, 7, 2433 CrossRef PubMed.
- T. Ohnaga, Y. Takei, T. Nagata and Y. Shimada, Sci. Rep., 2018, 8, 12005 CrossRef PubMed.
- S. Merugu, L. Chen, E. Gavens, H. Gabra, M. Brougham, G. Makin, A. Ng, D. Murphy, A. S. Gabriel, M. L. Robinson, J. H. Wright, S. A. Burchill, A. Humphreys, N. Bown, D. Jamieson and D. A. Tweddle, Clin. Cancer Res., 2020, 26, 122 CrossRef CAS PubMed.
- F. Rifatbegovic, C. Frech, M. R. Abbasi, S. Taschner-Mandl, T. Weiss, W. M. Schmidt, I. Schmidt, R. Ladenstein, I. M. Ambros and P. F. Ambros, Int. J. Cancer, 2018, 142, 297–307 CrossRef CAS PubMed.
- H. Scharpenseel, A. Hanssen, S. Loges, M. Mohme, C. Bernreuther, S. Peine, K. Lamszus, Y. Goy, C. Petersen, M. Westphal, M. Glatzel, S. Riethdorf, K. Pantel and H. Wikman, Sci. Rep., 2019, 9, 7406–7406 CrossRef PubMed.
- G. Galletti, M. S. Sung, L. T. Vahdat, M. A. Shah, S. M. Santana, G. Altavilla, B. J. Kirby and P. Giannakakou, Lab Chip, 2014, 14, 147–156 RSC.
- R. Königsberg, E. Obermayr, G. Bises, G. Pfeiler, M. Gneist, F. Wrba, M. de Santis, R. Zeillinger, M. Hudec and C. Dittrich, Acta Oncol., 2011, 50, 700–710 CrossRef PubMed.
- X. Liu, Z. Zhang, B. Zhang, Y. Zheng, C. Zheng, B. Liu, S. Zheng, K. Dong and R. Dong, EBioMedicine, 2018, 35, 244–250 CrossRef PubMed.
- S. G. Dubois, C. L. Epling, J. Teague, K. K. Matthay and E. Sinclair, Pediatr. Blood Cancer, 2010, 54, 13–18 CrossRef PubMed.
- K. A. Hyun, G. B. Koo, H. Han, J. Sohn, W. Choi, S. I. Kim, H. I. Jung and Y. S. Kim, Onco Targets Ther, 2016, 7, 24677–24687 Search PubMed.
- F. Le Du, T. Fujii, K. Kida, D. W. Davis, M. Park, D. D. Liu, W. Wu, M. Chavez-MacGregor, C. H. Barcenas, V. Valero, D. Tripathy, J. M. Reuben and N. T. Ueno, PLoS One, 2020, 15, e0229903 CrossRef CAS PubMed.
- J. Kitz, D. Goodale, C. Postenka, L. E. Lowes and A. L. Allan, Clin. Exp. Metastasis, 2021, 38, 97–108 CrossRef CAS PubMed.
- T. M. Gorges, I. Tinhofer, M. Drosch, L. Rose, T. M. Zollner, T. Krahn and O. von Ahsen, BMC Cancer, 2012, 12, 178 CrossRef CAS PubMed.
- C. G. Rao, D. Chianese, G. V. Doyle, M. C. Miller, T. Russell, R. A. Sanders, Jr. and L. W. Terstappen, Int. J. Oncol., 2005, 27, 49–57 CAS.
- A. Markiewicz, J. Topa, A. Nagel, J. Skokowski, B. Seroczynska, T. Stokowy, M. Welnicka-Jaskiewicz and A. J. Zaczek, Cancers, 2019, 11, 59, DOI:10.3390/cancers11010059.
- S. Mukherjee, T. G. Kang, Y. Chen and S. Kim, Crit. Rev. Biomed. Eng., 2009, 37, 517–529 CrossRef PubMed.
- D. A. Fedosov, B. Caswell, A. S. Popel and G. E. Karniadakis, Microcirculation, 2010, 17, 615–628 CrossRef PubMed.
- S. Tripathi, Y. V. B. Varun Kumar, A. Prabhakar, S. S. Joshi and A. Agrawal, J. Micromech. Microeng., 2015, 25, 083001 CrossRef.
- X. Xue, M. K. Patel, M. Kersaudy-Kerhoas, M. P. Y. Desmulliez, C. Bailey and D. Topham, Appl. Math. Model., 2012, 36, 743–755 CrossRef.
- S. Yang, A. Ündar and J. D. Zahn, Lab Chip, 2006, 6, 871–880 RSC.
- X. Li, A. S. Popel and G. E. Karniadakis, Phys. Biol., 2012, 9, 026010–026010 CrossRef PubMed.
- R. D. Jäggi, R. Sandoz and C. S. Effenhauser, Microfluid. Nanofluid., 2007, 3, 47–53 CrossRef.
- M. Kersaudy-Kerhoas, R. Dhariwal, M. P. Y. Desmulliez and L. Jouvet, Microfluid. Nanofluid., 2009, 8, 105 CrossRef.
- D. Di Carlo, D. Irimia, R. G. Tompkins and M. Toner, Proc. Natl. Acad. Sci. U. S. A., 2007, 104, 18892–18897 CrossRef CAS PubMed.
- D. Di Carlo, Lab Chip, 2009, 9, 3038–3046 RSC.
- A. A. S. Bhagat, S. S. Kuntaegowdanahalli and I. Papautsky, Microfluid. Nanofluid., 2009, 7, 217–226 CrossRef CAS.
- H. Amini, W. Lee and D. Di Carlo, Lab Chip, 2014, 14, 2739–2761 RSC.
- S. Razavi Bazaz, A. Mihandust, R. Salomon, H. A. N. Joushani, W. Li, H. A. Amiri, F. Mirakhorli, S. Zhand, J. Shrestha, M. Miansari, B. Thierry, D. Jin and M. Ebrahimi Warkiani, Lab Chip, 2022, 22, 4093–4109 RSC.
- J. Seo, M. H. Lean and A. Kole, J. Chromatogr. A, 2007, 1162, 126–131 CrossRef CAS PubMed.
- A. A. S. Bhagat, S. S. Kuntaegowdanahalli and I. Papautsky, Lab Chip, 2008, 8, 1906–1914 RSC.
- T. H. Kim, H. J. Yoon, P. Stella and S. Nagrath, Biomicrofluidics, 2014, 8, 064117 CrossRef PubMed.
- M. Robinson, H. Marks, T. Hinsdale, K. Maitland and G. Cote, Biomicrofluidics, 2017, 11, 024109 CrossRef CAS PubMed.
- E. Lin, L. Rivera-Báez, S. Fouladdel, H. J. Yoon, S. Guthrie, J. Wieger, Y. Deol, E. Keller, V. Sahai, D. M. Simeone, M. L. Burness, E. Azizi, M. S. Wicha and S. Nagrath, Cell Syst., 2017, 5, 295–304.e294 CrossRef CAS PubMed.
- S. Wan, T. H. Kim, K. J. Smith, R. Delaney, G. S. Park, H. Guo, E. Lin, T. Plegue, N. Kuo, J. Steffes, C. Leu, D. M. Simeone, N. Razimulava, N. D. Parikh, S. Nagrath and T. H. Welling, Sci. Rep., 2019, 9, 18575 CrossRef CAS PubMed.
- M. Rafeie, J. Zhang, M. Asadnia, W. Li and M. E. Warkiani, Lab Chip, 2016, 16, 2791–2802 RSC.
- J. Sun, M. Li, C. Liu, Y. Zhang, D. Liu, W. Liu, G. Hu and X. Jiang, Lab Chip, 2012, 12, 3952–3960 RSC.
- H. Chen, Sci. Rep., 2018, 8, 4042 CrossRef PubMed.
- S. Shen, C. Tian, T. Li, J. Xu, S.-W. Chen, Q. Tu, M.-S. Yuan, W. Liu and J. Wang, Lab Chip, 2017, 17, 3578–3591 RSC.
- B. Miller, M. Jimenez and H. Bridle, Sci. Rep., 2016, 6, 36386 CrossRef CAS PubMed.
- M. G. Lee, J. H. Shin, C. Y. Bae, S. Choi and J.-K. Park, Anal. Chem., 2013, 85, 6213–6218 CrossRef CAS PubMed.
- M. Zuvin, N. Mansur, S. Z. Birol, L. Trabzon and A. Sayı Yazgan, Microsyst. Technol., 2016, 22, 645–652 CrossRef CAS.
- A. S. Rzhevskiy, S. Razavi Bazaz, L. Ding, A. Kapitannikova, N. Sayyadi, D. Campbell, B. Walsh, D. Gillatt, M. Ebrahimi Warkiani and A. V. Zvyagin, Cancers, 2019, 12, 81 CrossRef PubMed.
- B. L. Khoo, M. E. Warkiani, D. S.-W. Tan, A. A. S. Bhagat, D. Irwin, D. P. Lau, A. S. Lim, K. H. Lim, S. S. Krisna and W.-T. Lim, PLoS One, 2014, 9, e99409 CrossRef PubMed.
- S. Park, R. R. Ang, S. P. Duffy, J. Bazov, K. N. Chi, P. C. Black and H. Ma, PLoS One, 2014, 9, e85264–e85264 CrossRef PubMed.
- P. A. J. Mendelaar, J. Kraan, M. Van, L. L. Zeune, L. Terstappen, E. Oomen-de Hoop, J. W. M. Martens and S. Sleijfer, Mol. Oncol., 2021, 15, 116–125 CrossRef CAS PubMed.
- M. C. Zalis, J. F. Reyes, P. Augustsson, S. Holmqvist, L. Roybon, T. Laurell and T. Deierborg, Integr. Biol., 2016, 8, 332–340 CrossRef CAS PubMed.
- L. Clime, J. Daoud, D. Brassard, L. Malic, M. Geissler and T. Veres, Microfluid. Nanofluid., 2019, 23, 29 CrossRef.
- S. Haeberle, T. Brenner, R. Zengerle and J. Ducrée, Lab Chip, 2006, 6, 776–781 RSC.
- J.-N. Kuo and B.-S. Li, Biomed. Microdevices, 2014, 16, 549–558 CrossRef CAS PubMed.
- J.-N. Kuo and X.-F. Chen, Microsyst. Technol., 2015, 21, 2485–2494 CrossRef CAS.
- Y. Shi, P. Ye, K. Yang, B. Qiaoge, J. Xie, J. Guo, C. Wang, Z. Pan, S. Liu and J. Guo, Electrophoresis, 2022, 43, 2250–2259 CrossRef CAS PubMed.
- K. D. Lenz, S. Jakhar, J. W. Chen, A. S. Anderson, D. C. Purcell, M. O. Ishak, J. F. Harris, L. E. Akhadov, J. Z. Kubicek-Sutherland, P. Nath and H. Mukundan, Sci. Rep., 2021, 11, 5287 CrossRef CAS PubMed.
- A. Hatami, M. Saadatmand and M. Garshasbi, Talanta, 2024, 267, 125245 CrossRef CAS PubMed.
- A. Shamloo, A. Naghdloo and M. Besanjideh, Sci. Rep., 2021, 11, 1939 CrossRef CAS PubMed.
- C.-C. Lin, J.-C. Tsai, Y.-Z. Liu and J.-N. Kuo, Microfluid. Nanofluid., 2024, 28, 10 CrossRef CAS.
- S. Gupta and A. Bit, in Bioelectronics and Medical Devices, ed. K. Pal, H.-B. Kraatz, A. Khasnobish, S. Bag, I. Banerjee and U. Kuruganti, Woodhead Publishing, 2019, pp. 123–144, DOI:10.1016/B978-0-08-102420-1.00008-X.
- M. Wu, A. Ozcelik, J. Rufo, Z. Wang, R. Fang and T. Jun Huang, Microsyst. Nanoeng., 2019, 5, 32 CrossRef PubMed.
- W. L. Ung, K. Mutafopulos, P. Spink, R. W. Rambach, T. Franke and D. A. Weitz, Lab Chip, 2017, 17, 4059–4069 RSC.
- T. Franke, A. R. Abate, D. A. Weitz and A. Wixforth, Lab Chip, 2009, 9, 2625–2627 RSC.
- G. Destgeer, B. H. Ha, J. Park, J. H. Jung, A. Alazzam and H. J. Sung, Phys. Procedia, 2015, 70, 34–37 CrossRef CAS.
- A. Lenshof, C. Magnusson and T. Laurell, Lab Chip, 2012, 12, 1210–1223 RSC.
- T. Laurell, F. Petersson and A. Nilsson, Chem. Soc. Rev., 2007, 36, 492–506 RSC.
- F. Petersson, L. Åberg, A.-M. Swärd-Nilsson and T. Laurell, Anal. Chem., 2007, 79, 5117–5123 CrossRef CAS PubMed.
- P. Li, Z. Mao, Z. Peng, L. Zhou, Y. Chen, P.-H. Huang, C. I. Truica, J. J. Drabick, W. S. El-Deiry, M. Dao, S. Suresh and T. J. Huang, Proc. Natl. Acad. Sci. U. S. A., 2015, 112, 4970 CrossRef CAS PubMed.
- J. Shi, H. Huang, Z. Stratton, Y. Huang and T. J. Huang, Lab Chip, 2009, 9, 3354–3359 RSC.
- F. Petersson, A. Nilsson, H. Jönsson and T. Laurell, Anal. Chem., 2005, 77, 1216–1221 CrossRef CAS PubMed.
- P. Augustsson, C. Magnusson, M. Nordin, H. Lilja and T. Laurell, Anal. Chem., 2012, 84, 7954–7962 CrossRef CAS PubMed.
- M. Wu, Y. Ouyang, Z. Wang, R. Zhang, P.-H. Huang, C. Chen, H. Li, P. Li, D. Quinn, M. Dao, S. Suresh, Y. Sadovsky and T. J. Huang, Proc. Natl. Acad. Sci. U. S. A., 2017, 114, 10584 CrossRef CAS PubMed.
- J. Dykes, A. Lenshof, I.-B. Åstrand-Grundström, T. Laurell and S. Scheding, PLoS One, 2011, 6, e23074 CrossRef CAS PubMed.
- J. Nam, H. Lim, D. Kim and S. Shin, Lab Chip, 2011, 11, 3361–3364 RSC.
- Y. Chen, M. Wu, L. Ren, J. Liu, P. H. Whitley, L. Wang and T. J. Huang, Lab Chip, 2016, 16, 3466–3472 RSC.
- C. Magnusson, P. Augustsson, A. Lenshof, Y. Ceder, T. Laurell and H. Lilja, Anal. Chem., 2017, 89, 11954–11961 CrossRef CAS PubMed.
- M. Antfolk, C. Antfolk, H. Lilja, T. Laurell and P. Augustsson, Lab Chip, 2015, 15, 2102–2109 RSC.
- M. Wu, P. H. Huang, R. Zhang, Z. Mao, C. Chen, G. Kemeny, P. Li, A. V. Lee, R. Gyanchandani, A. J. Armstrong, M. Dao, S. Suresh and T. J. Huang, Small, 2018, 14, e1801131 CrossRef PubMed.
- A. Mishra, T. D. Dubash, J. F. Edd, M. K. Jewett, S. G. Garre, N. M. Karabacak, D. C. Rabe, B. R. Mutlu, J. R. Walsh, R. Kapur, S. L. Stott, S. Maheswaran, D. A. Haber and M. Toner, Proc. Natl. Acad. Sci. U. S. A., 2020, 117, 16839–16847 CrossRef CAS PubMed.
- R. Hulspas, L. Villa-Komaroff, E. Koksal, K. Etienne, P. Rogers, M. Tuttle, O. Korsgren, J. C. Sharpe and D. Berglund, Cytotherapy, 2014, 16, 1384–1389 CrossRef CAS PubMed.
- T.-H. Wu, Y. Chen, S.-Y. Park, J. Hong, T. Teslaa, J. F. Zhong, D. Di Carlo, M. A. Teitell and P.-Y. Chiou, Lab Chip, 2012, 12, 1378–1383 RSC.
- K. Mutafopulos, P. Spink, C. D. Lofstrom, P. J. Lu, H. Lu, J. C. Sharpe, T. Franke and D. A. Weitz, Lab Chip, 2019, 19, 2435–2443 RSC.
- C. H. Chen, S. H. Cho, F. Tsai, A. Erten and Y.-H. Lo, Biomed. Microdevices, 2009, 11, 1223 CrossRef CAS PubMed.
- S. Sakuma, Y. Kasai, T. Hayakawa and F. Arai, Lab Chip, 2017, 17, 2760–2767 RSC.
- A. Isozaki, H. Mikami, H. Tezuka, H. Matsumura, K. Huang, M. Akamine, K. Hiramatsu, T. Iino, T. Ito, H. Karakawa, Y. Kasai, Y. Li, Y. Nakagawa, S. Ohnuki, T. Ota, Y. Qian, S. Sakuma, T. Sekiya, Y. Shirasaki, N. Suzuki, E. Tayyabi, T. Wakamiya, M. Xu, M. Yamagishi, H. Yan, Q. Yu, S. Yan, D. Yuan, W. Zhang, Y. Zhao, F. Arai, R. E. Campbell, C. Danelon, D. Di Carlo, K. Hiraki, Y. Hoshino, Y. Hosokawa, M. Inaba, A. Nakagawa, Y. Ohya, M. Oikawa, S. Uemura, Y. Ozeki, T. Sugimura, N. Nitta and K. Goda, Lab Chip, 2020, 20, 2263–2273 RSC.
- T. Franke, S. Braunmuller, L. Schmid, A. Wixforth and D. A. Weitz, Lab Chip, 2010, 10, 789–794 RSC.
- L. Schmid, D. A. Weitz and T. Franke, Lab Chip, 2014, 14, 3710–3718 RSC.
- D. J. Collins, A. Neild and Y. Ai, Lab Chip, 2016, 16, 471–479 RSC.
- C. W. T. Shields, C. D. Reyes and G. P. Lopez, Lab Chip, 2015, 15, 1230–1249 RSC.
- J. Voldman, Annu. Rev. Biomed. Eng., 2006, 8, 425–454 CrossRef CAS PubMed.
- K. Takahashi, A. Hattori, I. Suzuki, T. Ichiki and K. Yasuda, J. Nanobiotechnol., 2004, 2, 5 CrossRef PubMed.
- P. S. Dittrich, M. Jahnz and P. Schwille, ChemBioChem, 2005, 6, 811–814 CrossRef CAS PubMed.
- L. Shang, Y. Cheng and Y. Zhao, Chem. Rev., 2017, 117, 7964–8040 CrossRef CAS PubMed.
- J. C. Baret, O. J. Miller, V. Taly, M. Ryckelynck, A. El-Harrak, L. Frenz, C. Rick, M. L. Samuels, J. B. Hutchison, J. J. Agresti, D. R. Link, D. A. Weitz and A. D. Griffiths, Lab Chip, 2009, 9, 1850–1858 RSC.
- A. Sciambi and A. R. Abate, Lab Chip, 2015, 15, 47–51 RSC.
- L. Mazutis, J. Gilbert, W. L. Ung, D. A. Weitz, A. D. Griffiths and J. A. Heyman, Nat. Protoc., 2013, 8, 870–891 CrossRef CAS PubMed.
- O. D. Velev and K. H. Bhatt, Soft Matter, 2006, 2, 738–750 RSC.
- A. Y. Fu, C. Spence, A. Scherer, F. H. Arnold and S. R. Quake, Nat. Biotechnol., 1999, 17, 1109–1111 CrossRef CAS PubMed.
- P. G. Erlandsson and N. D. Robinson, Electrophoresis, 2011, 32, 784–790 CrossRef CAS PubMed.
|
This journal is © The Royal Society of Chemistry 2025 |
Click here to see how this site uses Cookies. View our privacy policy here.