DOI:
10.1039/D4LC00823E
(Critical Review)
Lab Chip, 2025,
25, 996-1014
Retina-on-chip: engineering functional in vitro models of the human retina using organ-on-chip technology
Received
30th September 2024
, Accepted 14th January 2025
First published on 15th January 2025
Abstract
The retina is a complex and highly metabolic tissue in the back of the eye essential for human vision. Retinal diseases can lead to loss of vision in early and late stages of life, significantly affecting patients' quality of life. Due to its accessibility for surgical interventions and its isolated nature, the retina is an attractive target for novel genetic therapies and stem cell-based regenerative medicine. Understanding disease mechanisms and evaluating new treatments require relevant and robust experimental models. Retina-on-chip models are microfluidic organ-on-chip systems based on human tissue that capture multi-cellular interactions and tissue-level functions in vitro. Various retina-on-chip models have been described in literature. Some of them capture basic retinal barrier functions while others replicate key events underlying vision. In addition, some of these cellular systems have also been used in studies to explore their added value in retinal disease modeling. Most existing retina-on-chip models capture limited aspects of the phenotypic complexity of human diseases. This limitation arises primarily from the challenges related to controlled recapitulation of retinal function, including the relevant multi-cellular interactions and functional read-outs. In this review, we provide an update on recent advancements in the field of retina-on-chip, and we discuss the biotechnical strategies to further enhance the physiological relevance of the models. We emphasize that developers and researchers should prioritize the incorporation of the full spectrum of retinal complexity to effectuate a direct impact of retina-on-chip models in disease modeling and development of therapeutic strategies.
1 Introduction
The retina is a complex multi-layered tissue located at the back of the eye that converts light into electrical signals. This neuronal tissue is crucial for vision, and diseases affecting the different cells of the retina are major causes of progressive vision loss leading to severe visual impairment or blindness. Laboratory tissue culture (in vitro) models of the back of the eye will be instrumental in understanding retinal physiology and retinal disease as well as in developing therapeutic strategies. Recent advances in microfluidic organ-on-chip technology as well as human stem cell technology offer unique opportunities to develop ‘retina-on-chip’ models that will aid in painting the full picture of retinal diseases and support development of novel therapies.
In this review, we propose that, to realize this potential, current retina-on-chip models must become more advanced. We briefly describe the anatomy and physiology of the back of the eye and use it to illustrate key mechanisms in retinal function and disease progression. An overview of existing retina-on-chip models is provided, including their typical design, cellular composition and read-outs. We then highlight how further controlled integration of tissues, local biophysical and biochemical parameters and relevant read-outs will bring retina-on-chip models to the next level. These developments will pave the way for retina-on-chip models to contribute to understanding pathophysiology and developing therapies for people suffering from retinal diseases.
1.1 Anatomy of and function of the human retina
The process of vision starts when light enters the eye and reaches the retina, a neural tissue located in the back of the eye. This tissue is responsible for converting light into an electrical signal that is sent to the brain and is processed to form an image of the world around us. The mammalian neuroretina is a highly complex and organized tissue and consists of three distinct nuclear layers (outer nuclear layer (ONL), inner nuclear layer (INL), retinal ganglion cell (RGC) layer), where different retinal cell types reside (Fig. 1). The conversion of the light starts when the photons reach the photosensitive cells: the photoreceptor cells. These cells consist of a cell soma located in the ONL, photoreceptor inner segments (IS), and photoreceptor outer segments (OS). In particular, the OS is a membranous structure with stacked disks containing the photopigments (so called opsins), which start the phototransduction and visual cycle. There are two distinct types of photoreceptors, namely rod and cone photoreceptors.1 In humans, rod photoreceptors are important for low-light vision and express the photopigment rhodopsin, while cone photoreceptors are responsible for color vision and details. Cone photoreceptors express specific opsins, which are sensitive to short, medium or long-wavelength light. Rod and cone photoreceptors are not distributed equally in the human retina. While rods are in the periphery, cones are concentrated in the central, avascular area called the macula. The photoreceptor cells are in contact with cells of the INL. Here, bipolar cells, horizontal cells and amacrine cells are responsible for the amplification, conduction, and feedback of the signals received from the photoreceptors. This signal is then transferred to the RGCs which via the optic nerve ends in the brain, where it is further processed. Lastly, Müller glia cells located in the INL provide metabolic and structural support to the retina.
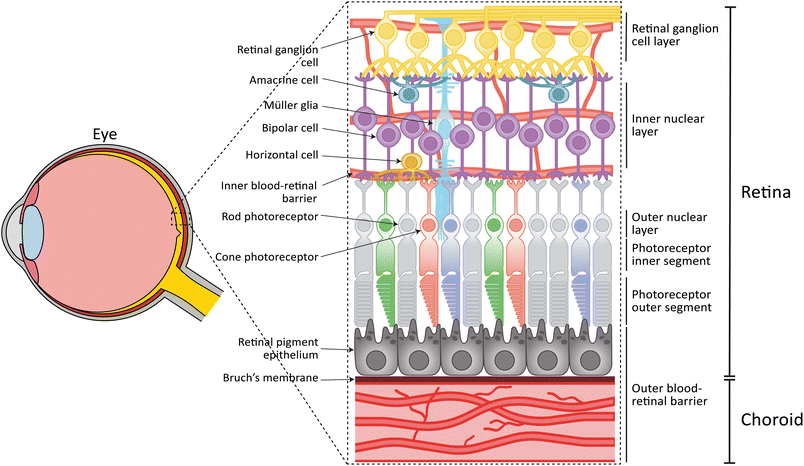 |
| Fig. 1 Anatomy of the human retina. The human retina is located in the back of the eye and consists of several distinct cell types and cell layers. The neuroretina, containing rod and cone photoreceptor cells in the outer nuclear layer, bipolar cells, horizontal cells, amacrine cells and Müller glia in the inner nuclear layer, and retinal ganglion cells in the retinal ganglion cell layer. The retinal pigment epithelium, Bruch's membrane, and the choroid form the oBRB. A vasculature surrounding the inner retinal layers form the iBRB. | |
Except for the avascular macular area, the neuroretina is vascularized by capillaries that provide nutrients and oxygen. The retinal capillaries are similar to the microvasculature found in the central nervous system in that they exhibit a strong barrier function, known as the inner blood-retinal barrier (iBRB). The vascular endothelial cells (ECs) of the retinal capillaries express tight junctions and membrane transporters, thereby limiting passive diffusion over their collective surface.
The survival and function of the rod and cone photoreceptors is highly dependent on retinal pigment epithelial (RPE) cells. RPE cells are in contact with the photoreceptor OS on their apical side, and the choroid on the basal side (Fig. 1). The choroid is the vasculature of the eye which together with the RPE form the outer blood-retinal barrier (oBRB).2 RPE cells are responsible for recycling photoreceptor OS through phagocytosis3,4 and their dark pigmentation functions to absorb excess light. Furthermore, RPE cells play a key role in the visual cycle, recycling all-trans retinol to 11-cis retinol, which are, together with metabolites, then provided back to the photoreceptors where they are used in phototransduction. Apart from their function in the visual cycle, RPE cells clear waste products and provide the photoreceptor cells with oxygen and nutrients, through the underlying Bruch's membrane and choroidal vasculature.
Bruch's membrane separates the choroid from the RPE and has a thickness of a few microns.5 Its structure can roughly be divided into five layers, namely: the basal lamina of RPE, inner collagenous layer, elastin layer, outer collagenous layer, and the basal lamina of the choroid. Both basal laminae are collagen type IV- and laminin-rich structures secreted by the RPE and choroid. The collagenous layers are collagen type I-, III-, and V-rich, while the elastin layer is composed of linear elastin fibers.6 Not only does Bruch's membrane provide an adhesive surface and support for the RPE, but it also limits migration of ECs from the choroid to the RPE. Furthermore, the membrane controls the exchange of oxygen, water, lipids, nutrients, ions, and metabolic waste.6
The underlying choroid is one of the most complex vascular layers with the highest blood flow rate per unit weight of any tissue in the human body and plays an integral role in catering to the high metabolic demand of the neuroretina.7 The choroidal endothelium is characterized by fenestrations – tiny holes in the vessel walls – which increase endothelial permeability and maximize metabolite exchange with the neuroretina. Approximately 80% of the metabolites and oxygen required by the neuroretina reach it by passive diffusion from the choriocapillaris towards the RPE through Bruch's membrane. At the same time, the choroid depends on the growth factors secreted by RPE. The cellular composition of the choroid includes not only choroidal ECs but also melanocytes, fibroblasts, pericytes, vascular smooth muscle cells, intrinsic choroidal neurons, and immune cells. Together, these cells support vascular integrity and regulate the immune response of the choroid.8
1.2 Retinal disease and disease modelling
The interplay between different cell layers in the human retina highlights how aberrant functioning of one or multiple cell types can lead to a plethora of retinal degenerative diseases, ultimately resulting in blindness. The leading causes for blindness in adults are caused by age-related macular degeneration (AMD) which affects over 200 million people worldwide, and diabetic retinopathy (DR), a common consequence of diabetes mellitus that affects approximately 34% of diabetic patients worldwide.9–14 In the case of DR, the disruption of the BRB causes BRB leakage, neovascularization, hemorrhage and increased inflammatory responses. Early-stage AMD is characterized by the appearance of larger drusen lipid deposits in Bruch's membrane and loss of choroidal EC,15 while late-stage AMD can be subdivided into dry AMD, characterized by geographic atrophy, and wet AMD, which involves neovascularization of the choroid into the neuroretina.14,16,17 Next to these multifactorial diseases, inherited retinal diseases (IRDs) are the main cause of blindness in the first two decades of life. There are over 50 subtypes of IRDs and over 270 IRD associated genes identified, with an overall combined prevalence of 1 in 2000. In IRDs either rod photoreceptors, cone photoreceptors, or both are mainly affected, although RPE and bipolar cells can also play a crucial role. The most prevalent IRD is retinitis pigmentosa (RP), a progressive degenerative disease initially affecting rod photoreceptors, resulting in loss of vision over time.18–20 Similar to DR and AMD, multiple cell types can be affected in IRDs including RP, Stargardt disease (STGD1), and Leber congenital amaurosis (LCA), where both RPE and photoreceptor functioning can be impaired.20–22
DR, AMD, and IRDs all show how the disruption of interactions between different cell types and cell layers of the retina can ultimately lead to vision loss. Understanding the molecular and cellular mechanisms behind these and other retinal degenerative diseases is therefore an important factor for therapy development. However, the complexity of the human eye poses challenges in disease modeling. Current research makes use of different types of models, each with their advantages and limitations. For instance, immortalized cell lines are relatively quick and easy to be used to study molecular and cellular mechanism of disease. However, these cells lack physiological characteristics of the retinal cell types they are representing in vivo and do not capture tissue-level interactions.23,24 Alternatively, rodent in vivo models do include the morphological retinal context and have been used for retinal disease modeling for decades. Researchers have developed multiple models for both IRDs and multifactorial diseases like AMD and DR.25–27 Although in vivo models contain the full tissue-level interactions, it has been shown that often these animal models do not fully recapitulate the human disease phenotype. STGD1 mouse models show a rather late phenotype in which there is indeed accumulation of lipids and lipofuscin in the RPE, however, in the mouse model, this does not lead to photoreceptor degradation as seen in humans.28 These differences could be explained by differences in human and rodent eye morphology, molecular function and behavior. For example, rodents do not have a cone-rich macula, have a higher photoreceptor density, and their Bruch's membrane is thinner as compared to humans.29 These differences can lead to changes in RPE functioning between rodent models and humans, as, due to a relatively high photoreceptor to RPE cell ratio, the phagocytic load in the mouse could be much higher compared to human RPE.29 Furthermore, additional changes on both cellular and genetic levels further hinder the relevance of rodent disease models as the human retina expresses three different cone subtypes, corresponding with short, medium and long-wavelength opsins, while mice only express two: short and medium-opsin.30 Furthermore, although it is believed that the DNA sequences between rodents and humans are highly conserved, there are major differences in the expression of the genes, with some genes not even found in the murine genome.31 Lastly, daily habits are also different, for example mice are nocturnal while humans are diurnal. These differences limit the use of these retinal disease models in understanding human disease mechanisms as well as their application in testing potential, especially genetic, therapies. Therefore, designing tissue-level models based on human cells would be of strong benefit for retinal disease modeling. Importantly, the eye is at the forefront of genetic therapeutic development. These novel personalized treatment strategies (e.g., antisense technology or genome editing) rely on specific human gene sequences and mutations. Therefore, human models are needed in order to evaluate efficacy and safety of new genetic therapies.
The discovery of induced pluripotent stem cells (iPSCs) facilitated the development of human-derived in vitro cell models. iPSCs have been used widely to develop cellular models for the back of the eye, including the choroid, RPE, photoreceptor precursor cells, ganglion cells and 3D retinal organoids (ROs) which will be thoroughly discussed later in this review.23,32–35 iPSC-derived models hold great promise, as they have been used to model several retinal dystrophies and could serve as a model to test efficacy of new personalized therapeutics. However, iPSC-derived retinal cell models often resemble an immature, embryonic tissue rather than a mature tissue.36,37 Therefore, the disease phenotypes in these models are often quite subtle, and severe degeneration, as seen in retinal diseases, is not detected. Furthermore, these separate iPSC-derived models still lack the interactions that are important for retinal functioning. Some efforts have been made by combining different tissue layers in vitro either combining ROs and an RPE monolayer, combining RPE with a vasculature modeling the BRB, or all three layers (neuroretina, epithelial and endothelial layers). However, an established high complexity in vitro model of the human eye has not been described. This is where controlled microfluidic co-culture models known as organs-on-a-chip can play a crucial and decisive role. By combining iPSC-derived cells with organ-on-a-chip technology, human retina-on-chip models can be developed that offer controlled tissue-level interactions, potentially increasing cell maturity, organ-level function and relevant disease phenotypes.
2 Retina-on-chip models
In recent years, researchers have tried to model the retina using organ-on-chip technology. Although we are aware that others used microfluidic chips to improve maturation in ROs and to facilitate culture procedures,38,39 we will here focus on current literature that have used human cells to create retina-on-chip models that are used to understand retinal function and retinal diseases. Specifically, we will include models of the iBRB, oBRB, and those co-culturing RPE with the neuroretina using a microfluidic chip. We will first discuss the cell types used in these models and then highlight the technical aspects in terms of chip design, materials, flow and read-outs. Finally, we discuss how these models have been applied in modelling diseases. A full list of retinal organ-on-chip models described in this section including fabrication methods, chip materials, membranes, induction of flow, cellular components, readouts and applications per model is listed in Table 1. Highlighted on-chip models are depicted in Fig. 2.
Table 1 Summary of current retina-on-chip models
Model |
Fabrication method |
Chip material |
Membranes |
Induction of flow |
Cellular components |
Readouts |
Application |
Ref. |
Commercially available chip from MIMETAS, The Netherlands.
Commercially available chip from AIM Biotech Pte. Ltd., Singapore.
|
oBRB-on-chip |
Photolithography |
PDMS |
|
Daily medium change |
HUVECs |
Fluorescent staining of proteins |
Modeling wet AMD-like choroidal neovascularization |
40
|
Soft lithography |
HLFs |
Vessel integrity/perfusability |
ARPE-19 |
Quantitative network analysis |
Barrier integrity dextran diffusion assay |
Photolithography |
PDMS |
|
Daily medium change |
HUVECs |
Fluorescent staining of proteins |
Modeling diabetes mellitus |
41
|
Soft lithography |
HLFs |
Vessel integrity/perfusability |
ARPE-19 |
Barrier integrity dextran diffusion assay |
Quantitative network analysis |
Photolithography |
PDMS |
PET (10–20 μm thickness, 3 μm pore size) |
Syringe pump |
HMVECs |
Fluorescent staining of proteins |
Model characteristics related to choroidal drug reactions and considering immunology |
42
|
Soft lithography |
PBMCs |
Vessel integrity/perfusability |
Melanocytes |
Barrier integrity dextran diffusion assay |
iRPE |
Cell viability assay |
Cytokine release |
CNC milling |
PDMS |
PET (10 μm thickness, 8 μm pore size) |
Gravity driven, bidirectional flow |
HUVECs |
Fluorescent staining of proteins |
Create a model with physiologically relevant biochemical readouts |
43
|
Soft lithography |
ARPE-19 |
Fluorescent angiography |
OCT |
SLA 3D printing |
PDMS |
|
Daily medium change |
HUVECs |
Fluorescent staining of proteins |
Modeling wet AMD-like choroidal neovascularization |
44
|
Soft lithography |
ARPE-19 |
Barrier integrity dextran diffusion assay |
Quantitative RPE & network analysis |
SLA 3D printing |
PDMS |
|
Syringe pump |
HRMVECs |
Fluorescent staining of proteins |
Propose a model for the oBRB |
45
|
Soft lithography |
HOCFs |
Quantitative RPE analysis |
iRPE |
Injection molding |
PS |
|
Daily medium change |
HUVECs |
Fluorescent staining of proteins |
Propose a model for the oBRB in a high-throughput 96-well format |
46
|
ARPE-19 |
Barrier integrity dextran diffusion assay |
Quantitative RPE & network analysis |
qPCR |
TEER |
iBRB-on-chip |
|
Virgin PSa |
|
Gravity driven, bidirectional flow |
HUVECs |
Fluorescent staining of proteins |
Modeling changes in vascular permeability in response to vascular leakage mediators |
47
|
hTERT HRMVECs |
Quantifying vessel permeability |
HRMVECs |
|
COPb |
|
Daily medium change |
HRMVECs |
Fluorescent staining of proteins |
DR |
48
|
HRPs |
Vessel integrity/perfusability |
HRAs |
Quantitative network analysis |
RNA-sequencing |
Injection molding |
PS |
|
Daily medium change |
HRMVECs |
Fluorescent staining of proteins |
|
46
|
HRPs |
RPE-neuroretina co-culture on-chip |
Photolithograph |
PDMS |
PET (10–20 μm thickness, 3 μm pore size) |
Syringe pump |
iRPE |
Fluorescent staining of proteins |
Drug-induced retinopathy |
49, 50 |
Soft lithography |
RO |
qPCR |
Translational platform for AAV retinal gene therapy vectors |
TEM |
ELISA |
Phagocytosis assay |
SLA 3D printing |
PDMS |
|
Peristaltic pump |
iRPE |
Fluorescent staining of proteins |
RP |
51
|
Soft lithography |
RO |
Western blot |
qPCR |
Retina-on-chip |
Photolithography |
Glass |
Glass microgrooves (2 μm width, 4 μm depth, 10 μm spaced) |
Syringe pump |
HRMVECs |
Fluorescent staining of proteins |
Create a multi-compartment model with integrated electrodes |
52
|
Soft lithography |
PDMS |
ARPE-19 |
Permeability assay |
SH-SY5Y |
TEER |
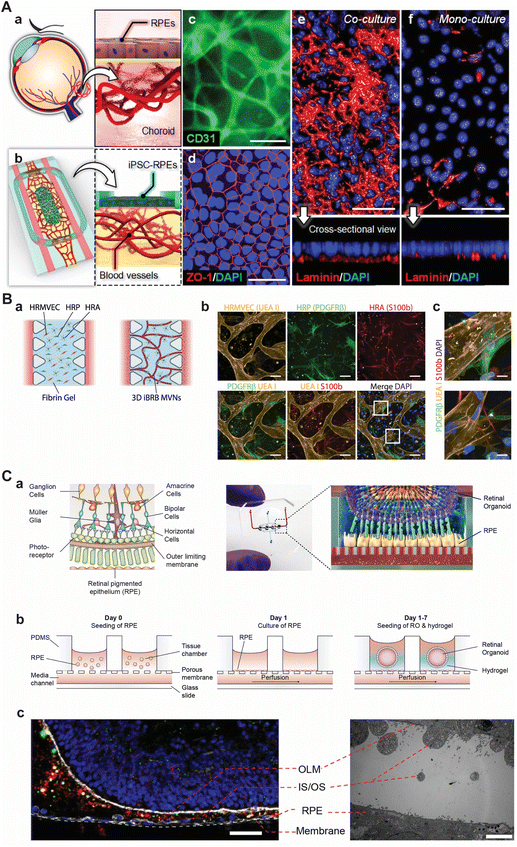 |
| Fig. 2 Selection of current approaches to model various parts of the retina using microfluidic chips. (A) Model of the oBRB as presented by Paek et al. (a) Schematic depiction of the oBRB. (b) Schematic of the microfluidic chip that comprises of two flanking medium channels with an inner hydrogel compartment and an open top on which iRPEs were cultured. (c) Immunolabeling of CD31 in primary HRMVECs revealed a 3D vascular network in the hydrogel compartment after 14 days of culture. (d) Immunolabeling of ZO-1 in RPE. (e and f) Immunolabeling of basement membrane protein laminin showing the difference in maturation of having a monoculture RPE and co-culture vessels and RPE. In c–f, scale bars are 50 μm. Reprinted (adapted) with permission from Jungwook Paek, Sunghee E. Park, Qiaozhi Lu, et al. copyright 2019 American Chemical Society.45 (B) Model of the iBRB as presented by Maurissen et al. (a) Schematic of the microfluidic chip in which HRMVECs, pericytes, and astrocytes were seeded in a fibrin hydrogel with flanking medium channels. (b and c) Immunolabeling of HRMVECs (agglutinin I (UEA I)), pericytes (PDGFRβ), and astrocytes (S100b) showing co-localization of the supporting cells with the network of HRMVECs. In b and c, scale bars are 100 μm and 10 μm, respectively. Adapted from Maurissen et al., Nat. Comm., 2024, CC BY 4.0.48 (C) The retina-on-chip model as proposed by Achberger et al. (a) Schematic of the retina with its complexity (left) and how the retina was modelled in a microfluidic chip by combining RPE cells with ROs by Achberger et al. (right). (b) Schematic section view of the open-top microfluidic chip and the procedure to incorporate vascular-like perfusion, RPE cells, and ROs. (c) Immunolabeling of day 260 ROs co-cultured in proximity with RPE after 7 days (left). The inner and outer segments (IS/OS) of photoreceptors are shown in green (rod outer segment membrane protein 1 (ROM1)), the cytoskeleton in white (F-actin), and rod photoreceptors in red (rhodopsin), scale bar is 40 μm. Transmission electron microscopy image highlighting the proximity of RPE and RO (right), scale bar is 5 μm. Adapted from Achberger et al., eLife, 2019, CC BY 4.0.49 | |
2.1 Cell types
Cells are an integral component of any organ-on-chip model. Depending on their source, culture methods, and genetics, cells have inherent functionalities (their ‘phenotype’) that are essential for developing models that capture tissue-level or even organ-level physiology. For all human in vitro models, there are three main sources of cells: immortalized cell lines, primary cells and stem cell-derived cells. As we will see in the sections below, many early retina-on-chip models incorporate cell lines or primary cells, with a progressive shift towards stem cell-derived cells. Typically, cell lines or primary cells are easier and cheaper to handle and are more accessible to those who are not experts in stem cell technology, while stem cell-derived cells have a more representative phenotype of the retinal tissue and can be obtained even from patients.
2.1.1 Vascular cells.
ECs support the retina and are essential to control transport between blood and the neuroretina through the BRB. In fact, most of the retinal organ-on-chip models explored so far, relate to modelling of the BRB. Early oBRB-on-chip models contained ECs, most derived from primary human umbilical vein endothelial cells (HUVECs)40,41,43,44,46,47 and other primary ECs, such as human microvascular endothelial cells (HMVECs) and (immortalized) human retinal microvascular endothelial cells (HRMVECs).42,45–48,52 HUVECs are primary cells isolated from human umbilical cords and are among the most widely used and well-characterized ECs in in vitro models. Although HUVECs can be used for in vitro modeling in tissue engineering and organ-on-chip technology, they do not show any choroidal EC phenotype,53 and come with certain other disadvantages which have been thoroughly discussed elsewhere,54,55 posing a challenge to model the oBRB. HMVECs are commercially available from various tissues, including the retina. These cells express more adult EC markers and have distinct morphology compared to HUVECs.56 However, primary cells tend to lose their phenotype upon passaging in vitro limiting their use and making them an expensive source of ECs in general.57
In addition to ECs, several works have employed other cells which reside in the choroid, such as fibroblasts,40,41,45 pericytes/vascular smooth muscle cells,46,48 and even primary melanocytes which regulate choroidal homeostasis and inflammation.42,58 To support EC network formation in oBRB-on-chip models, two types of fibroblast cells have been used: primary human lung fibroblasts (HLFs)40,41 primary human ocular choroid fibroblasts (HOCFs) which seem to have a more retinal profile.45 A combination of choroidal fibroblasts and pericytes was investigated in a 3D-bioprinted oBRB model by Song et al.59 Fibroblasts and pericytes are found to differ in function as fibroblasts are known to support formation and survival of vascular networks while pericytes give more structural support.41,60 For iBRB-on-chip models, HRMVECs, were co-cultured with retinal microvascular pericytes (HRPs) and human retinal astrocytes (HRAs), which both increased stability of formed vessels.48
Currently, thanks to the revolution in iPSC technology, several groups are exploring the development of iPSC-derived ECs. However, further improvements are required to be able to implement these cells in BRB-on-chip models as iPSC-derived ECs have limited culture times compared to RPE and neuroretinal cells, as it has been shown that after 30 days in vitro iPSC-derived ECs go into senescence and seem to deteriorate.61 Furthermore, iPSC-derived ECs still have limited tissue specificity, however, iPSC-derived choroidal EC differentiation protocols are currently being developed.32,62
2.1.2 Retinal pigment epithelial cells.
In early studies of the oBRB in retina-on-chip models, two main sources of RPE cells were used: the spontaneously immortalized ARPE-19 cell line40,41,43,44,46,52 and the hTERT-RPE1 cell line of immortalized RPE cells.23 Both immortalized cell lines do show phenotypic characteristics of RPE polarization and consequently polarized VEGF secretion. However, there are considerable differences in its barrier resistance, pigmentation, and gene expression compared to human RPE cells in vivo making them a less representative cell model.63,64 Moreover, ARPE-19 cell lines are known to have abnormal karyotypes and have reduced differentiation capacity due to prolonged culture and passaging.64 It has been reported that, by adjusting culture conditions, differentiation capacity of ARPE-19 can be improved, but these cell lines overall show an under differentiated phenotype,23,65 making them physiologically less relevant.
The most relevant cell type would be human primary RPE cells, as they are able to mature, have high barrier resistance, and pigmentation.23 However, these are not readily available and only have a limited lifespan. Even easier to obtain mouse primary RPE cells have limited ex vivo culturing capabilities and are not suitable to create a human-based retina-on-chip.
With the development of several optimized differentiation protocols to obtain iPSC-derived RPE cells (iRPE), researchers have been moving towards the use of these cells to model disease and develop retina-on-chip models which will be discussed thoroughly in section 3.1.42,45,49–51
2.1.3 Neuroretinal cells.
Initial studies have appeared in which neural cells are incorporated in retina-on-chip models. For example, SH-SY5Y cells were used to model the neuroretina.52 The SH-SY5Y cell line is a human neuroblastoma cell line derived from a metastatic bone tumor and can generally be used to study neurological diseases and their underlying mechanisms.66 However, using this cell type is an oversimplified model for the neuroretina as it simply lacks phenotypic characteristics of the cells found in this tissue.
Alternative cellular systems for use in retina-on-chip models could be murine cell lines, such as 661W67 and RGC-5, which were believed to be mouse photoreceptor progenitor cells and rat retinal ganglion cells, respectively. However, the controversy raised by several publications in which RGC-5 lines showed a mouse background and similar features as 661W has led to questioning the use of RGC-5 as cellular system to model retinal conditions in vitro.68,69 Furthermore, these cell lines also lack other, relevant, retinal cell types, and do not have a three-dimensional structure needed to model a retina-on-chip.
Given the limitations of these neural and neuroretinal cell lines, most of the current in vitro models of the neuroretina have used iPSC-derived cells, either in 2D or 3D cultures, using iPSCs from various sources. These cells have also been used in retina-on-chip models including iPSC-derived ROs from keratinocytes, urine cells, and peripheral blood mononuclear cells.49–51 Currently, there are a variety of protocols used to obtain neuroretinal cells derived from iPSCs as highlighted elsewhere.34,70 Most promising though, are iPSC-derived ROs, which are three-dimensional, express different cell types of the retina, and have a similar structure to the human retina, making it a suitable model to include on the retina-on-chip. We will elaborate more on the use ROs for retina-on-chip systems in section 3.1.
2.2 Organ-on-chip technology
Currently, no full retina-on-chip model, containing all layers and cell types found in the human retina, has been described in literature. However, several publications have demonstrated the use of organ-on-a-chip technology to model specific parts of the back of the eye, such as iBRB46–48 oBRB40–46 or neuroretina.49,50,52 Certain examples are depicted in Fig. 2 and key characteristics of current retina-on-chip models can be found in Table 1.
2.2.1 Designs, materials, and perfusion.
Most of the current retina-on-chip models utilize a multicompartment chip design to enable controlled co-culture of the various cell types to model different sections of the retina. These compartments can be arranged side-by-side, separated by partially open barrier structures like pillars or ridges,40,41,44–48 as exemplified in Fig. 2A and B. Alternatively, these compartments can be arranged vertically, separated by semi-permeable membranes or a membrane-like structure,42,43,49,52 as shown in Fig. 2C. RPE cells can be cultured as monolayers, either on hydrogel surfaces (Fig. 2A) or on semi-permeable membranes (Fig. 2C). In addition, the formation of 3D vessels is typically enabled by introducing hydrogels like collagen43,47 or fibrin40,45,46,48 in the vascular microcompartments, as depicted in Fig. 2B. Moreover, some retina-on-chip models contain open compartments filled with medium or inert hydrogels like hyaluronic acid to enable controlled integration of 3D ROs allowing for close contact between RPE and retinal organoids to represent the neuroretina, as shown in Fig. 2Cb and c.49–51
In most cases, the material of choice for fabricating these microfluidic chips is polydimethylsiloxane (PDMS).40–45,49,52 Choosing PDMS has several benefits, such as its optical transparency, low cost, and its biocompatibility. Besides, PDMS-to-PDMS and glass-to-PDMS bonding can easily be realized by plasma treatment.71 PDMS is often cast on molds made by photolithography using a photosensitive negative epoxy, like SU-8.40–42,49,52 Others have used 3D printing techniques, such as stereolithography (SLA), to create the molds44,45,51 while Arik and colleagues chose computer numerical control (CNC) milling to create molds made from polymethylmethacrylate (PMMA).43 However, PDMS does come with certain limitations in cell culture, such as the absorption of small hydrophobic molecules and its gas permeability when attempting to control oxygen levels.72 Therefore, commercially available microfluidic chips made from virgin polystyrene (PS, MIMETAS, The Netherlands),47 cyclin olefin polymer (COP, AIM Biotech, Singapore),48 and home-made PS microfluidic chips46 were utilized as well in current retina-on-chip models. These materials are known to have great mechanical properties, good biocompatibility, and low permeability to small molecules and gas. Nonetheless, these materials do come with high machinery and tooling costs and have less flexibility when it comes to design changes.73
Active microfluidic perfusion is typically necessary to maintain viability and functionality of the cultured cells in retina-on-chip models. Therefore, flow was generated by hydrostatic pressure or by connecting syringe pumps.49–51 Some studies also demonstrated perfusion of circulating immune cells.42
2.2.2 Read-outs.
Researchers who have developed a microfluidic chip in which a vascular component was added, used roughly the same readouts. Visual characterization of the vascular network was accomplished by fluorescent staining of cluster of differentiation 31 (CD31) or platelet endothelial cell adhesion molecule (PECAM)-1 (Fig. 2Ac), zonula occludens (ZO)-1, vascular endothelial (VE)-cadherin, F-actin, and Ulex Europaeus agglutinin (UAE) 1 as in Fig. 2Bb and c.40–42,44–48,74 Besides, pericytes and astrocytes, if included, were visualized using antibodies against platelet-derived growth factor receptor (PDGFR)β and S100b (Fig. 2Bb).48
In some cases, vessel integrity and/or perfusability was assessed by fluorescent dextran perfusion.40,42,43,45,47,48,52,75 Others included extensive quantitative network analysis by, for example, characterizing EC/pericyte area, EC–pericyte distance, vascular volume, vessel diameter, and number of branch points.40,41,44,48 Interestingly, Arik and coworkers focused on clinically relevant readouts and showed the compatibility of optical coherence tomography (OCT) for on-chip quantification of vessels and detection of vessel sprouts.43
Readouts for RPE cells also seem similar across current literature. Maturation of RPE is visually characterized by pigmentation under a regular bright field microscope or by fluorescent staining of ZO-1, to mark the tight junctions unveiling the classical polygonal monolayer of RPE cells (Fig. 2Ad).40–46,49,52 Other markers like ezrin and RPE65 were used to evaluate polarization and visualize RPE-specific features, respectively,41,45 while paired box (PAX)6 and microphthalmia-associated transcription factor (MITF) were used to identify expression of proteins involved in pigmentation.49 Besides, visualizing the apical microvilli on polarized RPE was demonstrated using transmission electron microscopy (TEM) and functionality of polarized RPE was shown by quantifying basal vascular endothelial growth factor (VEGF) secretion using an enzyme-linked immunosorbent assay (ELISA).49 Quantifying expression of key proteins within the RPE, such as bestrophin-1 and premelanosome protein (PMEL), was performed using Western blot by Su and colleagues.51 On the gene expression level, few showed compatibility of executing a quantitative polymerase chain reaction (qPCR) for RPE-related genes on-chip.33,46,49,51 On the basal side of the RPE, some have visualized structural proteins, like collagen type IV and laminin as an indication for Bruch's membrane development,40,41,44,45 as shown in Fig. 2Ae and f. Furthermore, as barrier integrity of the RPE cells is an important property, some have quantified this by dextran diffusion assays40,42,44,46 while others demonstrated compatibility and integration of transepithelial/transendothelial electrical resistance (TEER) measurements on-chip.46,52
ROs on-chips were analyzed mainly by end-point analyses, including immunofluorescent imaging and western blotting for photoreceptor markers (incluidng rhodopsin, arrestin 3) and cell death markers (TUNEL), as well as TEM, and -omics technologies.49,51 The interaction of ROs with the RPE monolayer was analyzed by visualizing photoreceptor outer segment shedding and phagocytosis by the RPE using both immunofluorescent imaging for and TEM, as shown in Fig. 2Cc.49
2.3 Disease modeling
When it comes to disease modeling in current organ-on-chip models for the retina, most have focused on investigating wet AMD or DR using oBRB-on-chip40–46 or iBRB-on-chip models,46–48 and few retina-on-chip models49–52 have been reported to investigate other retinal diseases, which combined RPE cells, retinal organoids, and microfluidics (Table 1).
Most BRB-on-chip models for wet AMD or DR have simulated events by either inducing hypoxic stress, oxidative stress, inflammatory stress, or increasing VEGF concentration in the vascular part.40,41,43,44,46,48 As predicted, all stimuli led to major changes, such as an increase in vessel thickness, damaged vessels, and damaged RPE monolayers. For example, Maurissen et al. found that stimulating iBRB-on-chip models with tumor necrosis factor (TNF)-α, IL-6 and a high glucose concentration mirrored DR features including the production of proinflammatory factors, ghost vessels and vascular regression.48 Furthermore, the addition of these factors induced decreased tight junction integrity and led to RPE barrier disruption in an oBRB-on-chip model.41 In some cases, the damaging effects caused by stress-inducing factors were counteracted by the addition of different drugs. Although these models show their potential for therapy development, they rely on the artificial addition of proinflammatory factors in order to cause the defects observed. Instead, Cipriano and coworkers established an immunocompetent choroid-on-a-chip by the perfusion of peripheral immune cells and detected in vivo-like cytokine release, which is more representative for the human retina.42
Few retina-on-chip disease models have been reported previously by either using IRD patient-derived cells, or by chemically inducing retinopathy in ROs and RPE.49–51 Here, known retinopathic adverse effects of chloroquine and gentamycin were assessed in RO and retina-on-chip models.49 Interestingly, the effects of gentamicin-induced retinopathy were diminished in the retina-on-chip models compared to retinal organoids without RPE, demonstrating protective function of the RPE. To further validate the protective role of RPE in retina-on-chip models, retinal organoids with USH2A mutations exhibited enhanced survival and upregulated extracellular matrix component expression within the microfluidic retina-on-chip system compared to standard retinal organoid culture.51 Lastly, the retina-on-chip model was used as a platform to test the transduction efficiency of adeno-associated viral vectors, that in the future can be used for therapy delivery.50 Therefore, the added effect of the retina-on-chip models compared to static RO cultures for drug development, toxicology, and personalized medicine studies highlights the need for more complex retina-on-chip.
3 Future advances in retina-on-chip
Current retina-on-chip models have demonstrated the technical feasibility of the controlled integration of multiple cell types in an engineered microenvironment. They also provide the first proof that retina-on-chip models can capture tissue-level physiology and dysfunction, as they exhibit elements of the visual cycle, controlled transport, as well as disease-related processes like decreased vascular density, neovascularization and loss of controlled barrier function.
For retina-on-chip models to have a real impact on biomedical science and therapy development for retinal diseases, it will be essential to improve their physiological relevance. For this, the cells need to be stimulated towards improved cellular maturation in terms of retinal-specific or even patient-specific phenotypes. Moreover, the dynamic microenvironment needs to be improved by using relevant (bio)materials, fluid flow and light stimulation. A schematic of an advanced and high-throughput retina-on-chip system is depicted in Fig. 3. While achieving these technical improvements, novel retina-on-chip models will need to be qualified for modeling specific disease processes, for example by including relevant read-outs and comparing with patient samples. Finally, their throughput and usability by future end-users will need to be taken into account.
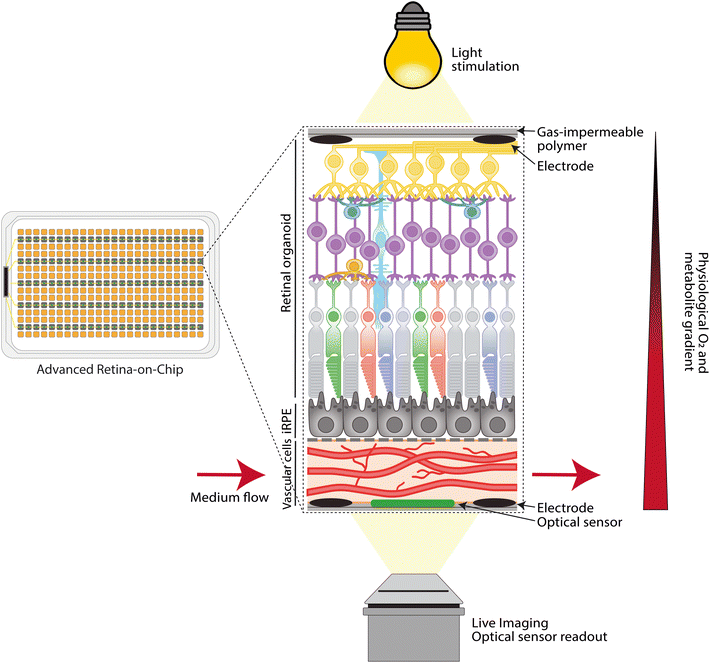 |
| Fig. 3 Advanced retina-on-chip platform, a high-throughput system designed to mimic retinal physiology. The platform comprises a choroid layer consisting of phenotypical more relevant choroidal cells forming a functional vascular layer separated from iRPE by a novel scaffold membrane, with the neural retina integrated on top. A physiological oxygen and metabolite gradient is established by exclusively perfusing medium through the choroid layer. The use of gas-impermeable materials further enhances the native microenvironment. Integrated optical sensors allow real-time monitoring of O2, pH, and temperature, while embedded electrodes enable live TEER and electrophysiological measurements. Additionally, live imaging facilitates precise monitoring of RoC throughout cultivation. | |
3.1 Improving retinal phenotypes of cells
Current iPSC-derived cells all suffer from the issue that they are not fully representative of adult human cells. For example, iRPE are phenotypically similar to RPE cells in the developing fetus,63 and ECs derived from iPSC lack a distinct retinal or choroidal phenotype and therefore limit the relevance of modeling the BRB. Similarly, iPSC-derived ROs have immature, fetal phenotypes and suffer from a shifting cellular content due to selective differentiation, degradation and proliferation of cell types.36,76,77 The main strategies for achieving more realistic phenotypes in stem cell-derived cells focus on optimizing differentiation protocols62 and on co-culture with relevant cell types in, for instance, the retina-on-chip as proposed in Fig. 3.
3.1.1 Vascular cells.
The most recent approach to differentiate choroidal ECs is based on supplementing connective tissue growth factor (CTGF) in the differentiation medium, which has previously been demonstrated to drive the differentiation towards a choroidal ECs.78 In this approach, the differentiated cells expressed the endothelial marker CD31, the fenestration marker plasmalemma vesicle-associated protein (PLVAP), and the more choroidal ECs-specific marker forkhead box protein A2 (FOXA2) and carbonic anhydrase 4 (CA4). Besides, it was shown that these ECs can form tubes in vitro.32 This marks an important step to generate choroidal-like ECs which can potentially be further matured when seeded in retina-on-chip models.
It is well established that the co-culture of ECs with stromal or mural cells have a synergistic positive effect and enable further maturation.60 Recently, the co-culture of iRPE, pericytes, fibroblasts and ECs led to an enhanced choroidal fate in the ECs after six weeks. It was shown that choroidal and capillary marker expression was significantly enhanced. Additionally, the co-culture showed a beneficial effect not only on the ECs but also on the fibroblasts and pericytes with enhanced maturity, high extracellular matrix (ECM) marker expression, and an overall gene expression profile more similar to the native oBRB.59 This confirms previous findings where co-culture with RPE enhanced choroidal fate in ECs and showed a positive effect on the RPE as well.45
Since retinal capillary ECs share the same origin and have similar characteristics as brain ECs, it may be possible that iPSC differentiation protocols for human brain microvascular ECs can be repurposed for generating retinal ECs.79 Additionally, co-culture with pericytes and astrocytes, which play key roles in retinal vascular development,80,81 may induce further phenotypic maturation. Similarly, co-culturing ECs with ROs could potentially enhance the retinal phenotype.82
3.1.2 Retinal pigment epithelial cells.
The generation of functionally mature iRPE has been relatively well-established as iRPE cultured on transwell inserts are pigmented, polarized, and form a barrier by expressing tight junction proteins. Additionally, polarized secretion of growth factors including VEGF can be detected in these RPE cells, and after stress induction, there is polarized secretion of additional growth factors, as well as stress factors and other metabolites.83 The RPEs retain phagocytic capabilities, being able to display photoreceptor OS phagocytosis.
3.1.3 Neuroretinal cells.
The differentiation of ROs from iPSCs closely mimics the development of the human retina. Here, different cell types arise in the ROs in a specific temporal order.36 Starting with the development of retinal progenitor cells, early cell types, such as RGCs, horizontal cells and amacrine cells start to differentiate. Late-born cell types in ROs include rod photoreceptors, bipolar cells, and Müller glia. Due to the close resemblance of development of the retina in vivo and RO development, researchers have found that RO transcriptome closely resembles that of the fetal retina.36,76 Similar to the neuroretina, ROs develop a laminated structure, with photoreceptor cells located on the outside of the organoid and a brush-like border representing photoreceptor OS. These photoreceptors display IS which include mitochondria and have a connecting cilium.84 However, there are often few, and disorganized, disk segments in the photoreceptor OS.85 West et al. showed that supplementation of RO media with antioxidants and docosahexaenoic acid (DHA), an abundant retinal lipid, improved the structure of photoreceptor outer segments.86 Researchers not only detected increased number of disk segments per photoreceptor, but the disks also had improved organization compared to ROs cultured without antioxidants and DHA.
RO development might also be affected by factors excreted from RPE cells.87 Supplementation of RO culture media with decellularized ECM derived from bovine RPE indeed improved the development of rod photoreceptors and showed functional improvement by enhanced synaptic marker expression and light responses of ROs. Similarly, iRPE conditioned media improved rod photoreceptor development in ROs.88 These findings are further supported by a study performing co-culture experiment with human iPSC-derived ROs and mouse primary RPE cells.89 After two weeks of co-culture, ROs had increased expression of both rod and cone photoreceptor markers compared to ROs cultures without RPE. In static culturing conditions, the limited nutrient and oxygen supply influences RO morphology. Integration of media perfusion in RO culturing could therefore further improve RO development and maturation. Several groups have studied RO culturing in combination with media perfusion.38 When culturing ROs in a rotating-wall vessel bioreactor, mouse ROs enhanced proliferation and differentiation of retinal cells compared to static cultures.90 Similarly, improved retinal ganglion cell development was found in human RO cultures in microfluidic devices.38 Therefore, an advanced retina-on-chip system, as depicted in Fig. 3, should allow for improved nutrient and oxygen supply by incorporating flow using the microfluidic system, and therefore enhance RO maturation.
Lastly, although no research on vascularized ROs has been published, we believe that vascularized ROs, mimicking the iBRB, could further improve phenotypic maturation, as similar improvements were measured in other models, such as in brain organoids.91,92
3.1.4 Patient cells.
Models based on iPSC-derived cells come with the advantage that they can contain cells from specific individuals, including patients. This means that models can be fully ‘personalized’ with all distinct cell types derived from the same iPSC-line, and therefore same person. This allows studies on personalized treatments or targeted gene therapy.93,94
For example, mutations of complement factor H (CFH) are correlated with AMD. The mutations have implications on the RPE but are also associated with choroidal degradation in early AMD.95 Therefore, deriving all cells from patients carrying the CFH mutation would enable mechanistic studies, including the associated phenotypic effects on all layers of the oBRB.96,97 Similarly, RPE cells derived from iPSCs of a STGD1 patient showed measurable aberrant lipid metabolism and photoreceptor OS phagocytic capabilities.98 Similarly, using ROs, researchers investigated protein expression and localization, especially in IRDs, and have tested whether therapeutic intervention can restore aberrant protein functioning.99–101 For example, by using iPSC-derived models, the role of a deep-intronic variant in CEP290 was identified. This gene typically causes severe syndromic diseases in an autosomal recessive manner, however, this particular variant was found to cause only blindness. While studies in blood and fibroblasts revealed 50% correct CEP290 mRNA, it was assumed that the retina required high levels of the CEP290 protein for proper function. Parfitt and collaborators demonstrated that, in a retinal background (using ROs), the correct CEP290 mRNA in the retina was less than 10%, highlighting the importance of the retinal molecular background in understanding and modeling diseases.102,103 Following on these findings, a therapeutic molecule based on an antisense oligonucleotide104,105 for this variant was evaluated in these ROs, and the data gathered from this system led to the initiation of a clinical trial, which is now in phase 3.106,107 Similar examples where iPSC-derived models have been crucial for understanding disease or assessing therapeutics have been documented in the literature for various retinal diseases, like STGD1 disease or Usher syndrome.100,108–113
3.2 Enhanced microenvironment engineering
Organ-on-a-chip models rely on recapitulating organ-level function by culturing relevant cell types in an engineered microenvironment, which includes relevant cell–cell interactions, tissue geometries, dynamic biophysical stimulation and local biochemical control.114–116 Therefore, to replicate the human retina as physiologically relevant as possible, only the integration of the retinal cells together in one microfluidic chip model will not be sufficient. A suitable near native microenvironment to cultivate and mature the model needs to be engineered. An advanced retina-on-chip should provide integration of membranes, establishment of metabolite gradients, continuous medium perfusion, integration of sensing technology to monitor and assess cultivation parameters, and tissue-specific responses (Fig. 3). In this section, we will elaborate on these parameters for an advanced retina-on-chip.
3.2.1 Scaffolding membranes.
Over the last two decades, researchers have enabled (bio)engineering strategies to model Bruch's membrane. Although we are aware that Bruch's membrane explants could potentially be used for retina-on-chip models, its availability is a major issue and will therefore not be included in this section.117 Focusing on engineered membranes, certain natural and synthetic strategies were recently reviewed by Molins et al.118 In essence, one should be able to fabricate a membrane that has the same physical properties as Bruch's membrane. In ideal case, the fabricated membrane should have the same thickness (few μms), porosity, and mechanical properties.118 Membranes that, for example, are thicker than those of the Bruch's membrane could lack the close interactions between the choroid and the RPE found in vivo. Furthermore, one should conserve the molecular composition of the Bruch's membrane as this is imperative to recapitulate sufficient RPE behaviour.118 For that, the appropriate microenvironment provides the cues to steer and maintain cellular behavior as discussed before.119 In addition to the above, the fabricated membrane should allow for long-term cultivation of RPE since it was found that this is vitally important for high expression of maturation markers and recovery time after passaging.120 Synthetic membranes are reproducible, can be fully characterized, and allow for long-term culture. The membranes can be coated with biological molecules such as basement membrane proteins to allow cells to attach.118,121
Current retina-on-chip models have used either polyester or PET that could be functionalized to facilitate RPE attachment.43,49 Yet, most commercially available membranes have a thickness between 10 and 20 micron which is much greater than Bruch's membrane. Although many thinner synthetic alternatives for Bruch's membrane based on, for instance, film casting and electrospinning exists,118,122 incorporating such membranes in microfluidic chips could be challenging as one should be able to include the membrane without any leaking. To overcome these issues, a solution for PDMS-based chips was recently published by Zakharova et al.123 Here, PDMS-based membranes of two micron-thickness and defined pore sizes were fabricated on silicon wafers with a sacrificial layer that could be dissolved with acetone. More recently, Song et al. integrated a two to ten micron-thick poly-(lactic-co-glycolic acid) (PLGA) electrospun porous scaffold in their 3D-bioprinted model of the oBRB.59 PLGA is a biodegradable material and after six weeks, the scaffold was replaced by RPE- and EC-secreted ECM, consisting of proteins found in the native Bruch's membrane, as discussed in section 1.1.
Natural membranes are those that consist of materials found in nature. Many have fabricated or derived natural membranes to mimic the Bruch's membrane from various sources, such as (spider) silk fibroin, soy, cellulose, alginate, hagfish slime intermediate filament proteins and the amniotic sac.118 Those derived from spider silk fibroin and hagfish slime seem most promising as they showed similar thickness, mechanical properties, and barrier functions as compared to the native Bruch's membrane. Besides, in both materials, no additional coating of ECM proteins was required to let the RPE grow on the membrane. However, these membranes were secured to transwell inserts using silicone rings which makes it difficult to implement them in microfluidic systems.124,125
3.2.2 Controlled transport and flow.
Metabolites and oxygen diffuse from the choroidal layer, driven by passive diffusion in a steep gradient from the choroid (100 mmHg) towards the photoreceptors (20 mmHg). This gradient is believed to be crucial for retinal health, and any disruption is associated with retinal diseases and should be taken into account when setting up novel retina-on-chip models. The oxygen levels in current retina-on-chip models are only controlled through environmental oxygen (incubator settings), and oxygen level control on-chip is not implemented. Normoxia in vitro, in standard cell culture at around 140 mmHg, is far above physiological levels, and the implications of a non-physiological oxygen environment are widely unaddressed for in vitro cell culture models.126 For RPE cells, it was shown in vitro that unsuitable oxygen levels impact RPE physiology.127In vivo, hyperoxia increases oxidative stress in the human retina, and in premature infants exposed to supplemental oxygen, hyperoxia-induced retinopathy can develop.128129 In addition, retinogenesis in ROs can be improved by cultivation under changing oxygen levels, as early stages of differentiation require low oxygen levels, and improved oxygen supply is needed in later stages of differentiation to improve ganglion cell survival and match the increased oxygen demand as cell proliferation is enhanced.130
Establishing near-native oxygen and metabolite gradients could therefore be an important next step to improve retina-on-chip models. Using PDMS as the main chip material hinders the control of oxygen levels and the establishment of oxygen gradients, as the material is highly permeable to oxygen. By using low oxygen-permeable chip materials, e.g., glass, PMMA and polystyrene, the oxygen intake in the chip can be limited exclusively by the oxygenated medium, enabling simple control of the oxygen intake in the system. By enabling continuous oxygenated medium perfusion exclusively through a vascular layer, as seen in the work by Paek et al.45 or Cipriano et al.,42 but in a gas-impermeable chip, intravascular supply of oxygen and metabolites can be established. Restricting the intake of oxygen and metabolites in the system makes continuous perfusion with fresh medium and the monitoring of the cultivation conditions on-chip essential to avoid unfavorable culture conditions. The oxygen level in the medium could be conditioned beforehand to physiological levels before pumping it through the vascular layer. Continuous medium perfusion stimulates ECs and the maturation of the vascular layer,131 which may have a favorable impact on retina-on-chip functionality.
To control and monitor oxygen levels, as well as other cultivation parameters such as pH and temperature, and to assess metabolites like glucose or lactate, the integration of suitable sensors within the retina-on-chip models will be the next fundamental step. Different sensor principles and sensor integration in microphysiological systems are extensively reviewed elsewhere.132,133 Optical sensors, based on dynamic quenching of a luminescent dye in the presence of an analyte that influences lifetime, are promising in terms of cost, robustness, ease of integration, ease of use, and high sensitivity, and allow for high-throughput and parallel readout of several cultivation parameters.134,135
3.2.3 Stimulation with light.
The principal function of the retina is the conversion of light into an electrical signal. It is therefore crucial that in vitro models can recapitulate these features. In the mammalian retina, the conversion of light occurs in the photoreceptor OS where photons convert 11-cis-retinol to all-trans-retinol.136 This conversion leads to the activation of photopigments in the photoreceptor OS and the recruitment of transducin and a phosphodiesterase (PDE). PDE activation results in reduced cyclic guanosinemonophosphate (cGMP), and therefore the closure of cyclic nucleotide gated channels (CNGCs), stopping the influx of sodium ions leading to hyperpolarization of the cell.137,138
It is believed that the phototransduction pathway is conserved in ROs. Previous research revealed that photoreceptors in ROs are able to respond to light similarly to the non-human primate eye.85,139 Using patch-clamp recording, researchers were able to detect distinct electrophysiological properties during RO development. Cone photoreceptor cells in ROs were able to respond to different intensities and between different wavelengths of light. In ROs, this light responsiveness is then transferred to retinal ganglion cells. Activity of retinal ganglion cells can be measured by plating ROs on microelectrode arrays (MEA). Here, responses to light being switched on and off can be detected from RGCs by cutting the RO and plating the RGC side on electrodes of the MEA.140,141 Stimulating ROs with white light pulses revealed distinct responses that could be modulated by the addition of 8-br-cGMP or Gamma-aminobutyric acid (GABA).140 Current MEA experiments only investigate the short-term activity of RGCs, while the effect of long-term follow-up of RO light responses has not been reported.
In an open top retina-on-chip design, which allows stacking of cellular and ECM components as exemplified in Fig. 2A and C,45,49 combined with a plated cut RO, light would travel through the same retinal cell layers as in the human eye, first passing through RGCs and cells of the INL and ONL, before reaching the photoreceptor OS that are in contact with RPE cells (Fig. 3). The connection between the RPE and the photoreceptors could possibly further enhance RO light responsiveness, as the combination of cell types restores the complete visual cycle thanks the RPEs conversion of all-trans-retinol to 11-cis-retinol which could then be taken up by the photoreceptors again. Therefore, light responses of photoreceptors in a retina-on-chip could resemble more closely the light responses in the human retina. These light responses could then be passed on to the different cell layers back to the RGCs, where activity could be measured by integrated electrodes.
3.3 Implementation and impact
The future impact of retina-on-chip models will be strongly dependent on their successful implementation in relevant settings, particularly in studying disease mechanisms and developing safe and efficacious treatment strategies.
3.3.1 Qualification of retina-on-chip models.
To facilitate qualification of a retina-on-chip model as a relevant model for human disease, two key aspects need to be addressed. Firstly, the (patho)physiological relevance of the models must be demonstrated. This could be achieved by analyzing the models in ways that enable direct comparison with clinical specimens or patient data.142 For example, models could be analyzed with clinical imaging techniques like fluorescence angiography or OCT, or with histological sectioning and immunostaining.43 Additionally, the physiological relevance of the models could be demonstrated by leveraging-omics techniques that enable side-by-side comparison with human data, such as single cell gene expression patterns and comparison with the human retina cell atlas.143 A significant challenge in the current drug development pipeline is the lack of translatability between preclinical in vitro and in vivo studies, and clinical testing. One of the major issues proposed is the lack of cell diversity in the current in vitro gold standard (immortalized cell lines) and genetic diversity in both in vitro and in vivo gold standard (immortalized cell lines and inbred animal models).144 Inbred strains display over 98% of shared genetics between animals, making these closer to technical replicates rather than biological replicates.145 To tackle these issues, the FDA launched the FDA Modernization Act 2.0, which allows for the use of alternatives to animal testing, including organoids, advanced artificial intelligence, but also iPSC-based organ-on-chip models such a retina-on-chip.144 The ability to mimic the complexity of the human retina through the co-culture of ECs, RPE, and ROs, and the use of iPSC-derived cells tackles both of the issues mentioned above. For uptake in preclinical drug development and regulatory science, it will be crucial to clearly define the ‘context of use’ (COU) of retina-on-chip models as they are being developed. The COU describes the specific role and scope of the model in addressing a well-defined question of interest. This strong need for defining a COU is also highlighted more broadly in the field of organ-on-chip by industry and regulatory scientists.146,147 To demonstrate that a retina-on-chip model is qualified for a particular COU, it will be important to define read-outs that are relevant for specific (patho)physiological mechanisms, to analyze variability from technical, biological, and inter-lab sources, and to test predictive value (sensitivity, specificity) with appropriate positive and negative controls and, where possible, relevant reference compounds. Identifying these measurable readouts will not only help to advance the diseases modeling but will also provide valuable biomarkers to test efficacy and safety for therapeutic interventions. These aspects of model characterization and qualification will rely on multi-stakeholder efforts, not only from future users from industry and regulatory bodies, but also from the early developers of retina-on-chip models, including engineers and biologists from academia.
3.3.2 Throughput and usability.
Live monitoring of culture parameters inside the retina-on-chip models not only enables feedback loops for parameter control but also adds a non-destructive readout for tissue integrity and -specific responses, offering a valuable tool alongside endpoint analysis techniques like immunofluorescence microscopy or qPCR.148 It has been shown that metabolic activity and drug-induced metabolic shifts can be directly measured by assessing the oxygen and pH changes with integrated optical sensors.149 In combination with optical sensors, the integration of electrodes in a retina-on-chip model would further provide non-invasive readouts. Continuous assessment of the barrier integrity of the RPE and vascular layer using TEER measurements provides valuable information about the maturation of the retina-on-chip model and is a well-established readout that can also be used to monitor electrical activity. Methods to integrate electrodes on chips are reviewed elsewhere.150 Live monitoring of various parameters allows for the continuous generation of datasets throughout the entire cultivation period of a single sample, including real-time imaging data. Combined with endpoint analysis, this approach provides a more detailed and accurate interpretation of results, offering a deeper understanding of the experimental outcomes.
To enable the use of retina-on-chip models for drug development or drug screening, efforts should focus on developing platforms with increased throughput that combine all the points discussed above within each individual chip unit, allowing for higher sample numbers and increasing the significance of the experiments conducted.135 Ideally, the retina-on-chip platforms should be designed in accordance with ISO standards to facilitate integration into highly parallelized and fully automated platforms, to further enhance robustness, reproducibility, and throughput.151,152 At the same time, the focus should not only be on increasing throughput, but also on generating high-content models that generate a diverse dataset for each sample, encompassing live monitoring of various cultivation parameters, impedance measurements, electrophysiology, high-throughput imaging, and additional transcriptomics and genomics data. The vast amount of high-content data produced will make the use of machine learning (ML) for effective analysis and interpretation crucial. ML algorithms can identify patterns, predict outcomes, and optimize experimental conditions, thereby making the research process faster, more efficient, and more precise.153–155
4 Conclusion
Current retina-on-chip technology mimics aspects of human retinal structure and function, aiding in the study of retinal diseases like macular degeneration and diabetic retinopathy. Current work focuses on replicating complex cellular interactions, providing insights into disease mechanisms. Next-generation retina-on-chip models could focus on capturing patient-specific aspects of disease, thereby enhancing personalized disease modeling and therapeutic strategies. Additionally, the next generation of retina-on-chip technology should facilitate medium-throughput or even high-throughput drug screening, accelerating the development of new treatments and reducing reliance on animal models. Overall, this evolution promises significant advancements in ophthalmology, offering more personalized and effective treatments for eye disease.
Data availability
Data will be available upon request from the corresponding author.
Author contributions
TG, DV, EvO contributed equally to this work. TG, DV, EvO, AG, AvdM designed, wrote, edited, the critical review. TG, DV, EvO generated/created the figures included in this critical review. LS, AG, AvdM were responsible for supervising, reviewing and editing the critical review. All co-authors have checked and approved the critical review.
Conflicts of interest
There are no conflicts to declare.
Acknowledgements
DV and EvO are supported by the Human Measurement 2.0 (grant nr. 18958) with additional funding from Proefdiervrij; and it is supported by the Association of Collaborating Health Foundations (SGF), NWO Domain AES and the Netherlands Organisation for Health Research and Development (ZonMw), as part of their joint strategic research programme: Human Measurement Models. The collaboration project is co-funded by the PPP Allowance made available by Health∼Holland, Top Sector Life Sciences & Health, to the Association of Collaborating Health Foundations (SGF) to stimulate public-private partnerships granted to AG and AvdM. Additional funding has been obtained through contributions from health foundations and supported in part by interested industrial research partners. In addition, funding was received from the European Union's Horizon 2020 research and innovation program under the Marie Skłodowska-Curie grant agreement (grant nr. 812954) and by the Netherlands Organ-on-Chip initiative, an NWO Gravitation project (024.003.001) funded by Ministry of Education, Culture and Science of the government of the Netherlands.
References
- K. A. Hussey, S. E. Hadyniak and R. J. Johnston Jr, Front. Cell Dev. Biol., 2022, 10, 878350 CrossRef PubMed.
-
V. M. Clark, in The Retina a Model for Cell Biology Studies, Elsevier, 1986, pp. 129–168 Search PubMed.
- W. Kwon and S. A. Freeman, Front. Immunol., 2020, 11, 604205 CrossRef CAS PubMed.
- A. L. Moran, J. D. Fehilly, D. Floss Jones, R. Collery and B. N. Kennedy, FASEB J., 2022, 36, e22556 CAS.
- K. R. Chirco, E. H. Sohn, E. M. Stone, B. A. Tucker and R. F. Mullins, Eye, 2017, 31, 10–25 CrossRef CAS PubMed.
- M. A. Fields, L. V. Del Priore, R. A. Adelman and L. J. Rizzolo, Prog. Retinal Eye Res., 2020, 76, 100803 CrossRef CAS PubMed.
- R. Urs, J. A. Ketterling, A. C. H. Yu, H. O. Lloyd, B. Y. S. Yiu and R. H. Silverman, Transl. Vis. Sci. Technol., 2018, 7, 5 CrossRef PubMed.
- M. K. Farazdaghi and K. B. Ebrahimi, J. Ophthalmic Vision Res., 2019, 14, 78 CrossRef PubMed.
- J. W. Yau, S. L. Rogers, R. Kawasaki, E. L. Lamoureux, J. W. Kowalski, T. Bek, S.-J. Chen, J. M. Dekker, A. Fletcher and J. Grauslund, Diabetes Care, 2012, 35, 556–564 CrossRef PubMed.
- D. Tonade and T. S. Kern, Prog. Retinal Eye Res., 2021, 83, 100919 CrossRef PubMed.
- D. A. Antonetti, P. S. Silva and A. W. Stitt, Nat. Rev. Endocrinol., 2021, 17, 195–206 CrossRef PubMed.
- J. Augustine, E. P. Troendle, P. Barabas, C. A. McAleese, T. Friedel, A. W. Stitt and T. M. Curtis, Front. Endocrinol., 2020, 11, 621938 CrossRef PubMed.
- C. Altmann and M. H. H. Schmidt, Int. J. Mol. Sci., 2018, 19, 110 CrossRef PubMed.
- R. H. Guymer and T. G. Campbell, Lancet, 2023, 401, 1459–1472 CrossRef CAS PubMed.
- K. Mulfaul, J. F. Russell, A. P. Voigt, E. M. Stone, B. A. Tucker and R. F. Mullins, Annu. Rev. Vis. Sci., 2022, 8, 33–52 CrossRef PubMed.
- F. G. Holz, E. C. Strauss, S. Schmitz-Valckenberg and M. van Lookeren Campagne, Ophthalmology, 2014, 121, 1079–1091 CrossRef PubMed.
- P. S. Mettu, M. J. Allingham and S. W. Cousins, Prog. Retinal Eye Res., 2021, 82, 100906 CrossRef CAS PubMed.
- N. Schneider, Y. Sundaresan, P. Gopalakrishnan, A. Beryozkin, M. Hanany, E. Y. Levanon, E. Banin, S. Ben-Aroya and D. Sharon, Prog. Retinal Eye Res., 2022, 89, 101029 CrossRef CAS PubMed.
- N. Cross, C. van Steen, Y. Zegaoui, A. Satherley and L. Angelillo, Clin. Ophthalmol., 2022, 1993–2010 CrossRef PubMed.
- S. Ferrari, E. Di Iorio, V. Barbaro, D. Ponzin, F. S. Sorrentino and F. Parmeggiani, Curr. Genomics, 2011, 12, 238–249 CrossRef CAS PubMed.
- A. I. Den Hollander, R. Roepman, R. K. Koenekoop and F. P. Cremers, Prog. Retinal Eye Res., 2008, 27, 391–419 CrossRef CAS PubMed.
- F. P. Cremers, W. Lee, R. W. Collin and R. Allikmets, Prog. Retinal Eye Res., 2020, 79, 100861 CrossRef CAS PubMed.
- K. Bharti, A. I. den Hollander, A. Lakkaraju, D. Sinha, D. S. Williams, S. C. Finnemann, C. Bowes-Rickman, G. Malek and P. A. D'Amore, Exp. Eye Res., 2022, 222, 109170 CrossRef CAS PubMed.
- Y. Zhu, B. Cao, A. Tolone, J. Yan, G. Christensen, B. Arango-Gonzalez, M. Ueffing and F. Paquet-Durand, Front. Neurosci., 2022, 16, 938089 CrossRef PubMed.
- A. Moshiri, Int. Ophthalmol. Clin., 2021, 61, 113–130 CrossRef PubMed.
- M. E. Pennesi, M. Neuringer and R. J. Courtney, Mol. Aspects Med., 2012, 33, 487–509 CrossRef CAS PubMed.
- M. Z. Sadikan, N. A. Abdul Nasir, L. Lambuk, R. Mohamud, N. H. Reshidan, E. Low, S. A. Singar, A. S. Mohmad Sabere, I. Iezhitsa and R. Agarwal, BMC Ophthalmol., 2023, 23, 421 CrossRef PubMed.
- P. C. Issa, A. R. Barnard, M. S. Singh, E. Carter, Z. Jiang, R. A. Radu, U. Schraermeyer and R. E. MacLaren, Invest. Ophthalmol. Visual Sci., 2013, 54, 5602–5612 CrossRef CAS PubMed.
- S. Volland, J. Esteve-Rudd, J. Hoo, C. Yee and D. S. Williams, PLoS One, 2015, 10, e0125631 CrossRef PubMed.
- F. M. Nadal-Nicolás, V. P. Kunze, J. M. Ball, B. T. Peng, A. Krishnan, G. Zhou, L. Dong and W. Li, eLife, 2020, 9, e56840 CrossRef PubMed.
- A. Bennis, T. G. Gorgels, J. B. Ten Brink, P. J. van der Spek, K. Bossers, V. M. Heine and A. A. Bergen, PLoS One, 2015, 10, e0141597 CrossRef PubMed.
- K. Mulfaul, J. C. Giacalone, A. P. Voigt, M. J. Riker, D. Ochoa, I. C. Han, E. M. Stone, R. F. Mullins and B. A. Tucker, Stem Cell Res. Ther., 2020, 11, 409 CrossRef CAS PubMed.
- K. Achberger, J. C. Haderspeck, A. Kleger and S. Liebau, Adv. Drug Delivery Rev., 2019, 140, 33–50 CrossRef CAS PubMed.
- T. A. V. Afanasyeva, J. C. Corral-Serrano, A. Garanto, R. Roepman, M. E. Cheetham and R. W. J. Collin, Cell. Mol. Life Sci., 2021, 78, 6505–6532 CrossRef CAS PubMed.
- C. M. Bell, D. J. Zack and C. A. Berlinicke, Annu. Rev. Vis. Sci., 2020, 6, 91–114 CrossRef PubMed.
- A. Sridhar, A. Hoshino, C. R. Finkbeiner, A. Chitsazan, L. Dai, A. K. Haugan, K. M. Eschenbacher, D. L. Jackson, C. Trapnell and O. Bermingham-McDonogh, Cell Rep., 2020, 30, 1644–1659.e1644 CrossRef CAS PubMed.
- C. S. Cowan, M. Renner, M. De Gennaro, B. Gross-Scherf, D. Goldblum, Y. Hou, M. Munz, T. M. Rodrigues, J. Krol and T. Szikra, Cell, 2020, 182, 1623–1640.e1634 CrossRef CAS PubMed.
- J. Gong, Y. Gong, T. Zou, Y. Zeng, C. Yang, L. Mo, J. Kang, X. Fan, H. Xu and J. Yang, Lab Chip, 2023, 23, 3820–3836 RSC.
- Y. Xue, M. J. Seiler, W. C. Tang, J. Y. Wang, J. Delgado, B. T. McLelland, G. Nistor, H. S. Keirstead and A. W. Browne, Lab Chip, 2021, 21, 3361–3377 RSC.
- M. Chung, S. Lee, B. J. Lee, K. Son, N. L. Jeon and J. H. Kim, Adv. Healthcare Mater., 2018, 7, 1700028 CrossRef PubMed.
- U. Nam, S. Lee and J. S. Jeon, ACS Biomater. Sci. Eng., 2023, 9, 4929–4939 CrossRef CAS PubMed.
- M. Cipriano, K. Schlünder, C. Probst, K. Linke, M. Weiss, M. J. Fischer, L. Mesch, K. Achberger, S. Liebau, M. Mesquida, V. Nicolini, A. Schneider, A. M. Giusti, S. Kustermann and P. Loskill, Commun. Biol., 2022, 5, 52 CrossRef PubMed.
- Y. B. Arik, W. Buijsman, J. Loessberg-Zahl, C. Cuartas-Velez, C. Veenstra, S. Logtenberg, A. M. Grobbink, P. Bergveld, G. Gagliardi, A. I. den Hollander, N. Bosschaart, A. van den Berg, R. Passier and A. D. van der Meer, Lab Chip, 2021, 21, 272–283 RSC.
- S. Lee, S. Kim and J. S. Jeon, Lab Chip, 2022, 22, 4359–4368 RSC.
- J. Paek, S. E. Park, Q. Lu, K.-T. Park, M. Cho, J. M. Oh, K. W. Kwon, Y.-S. Yi, J. W. Song, H. I. Edelstein, J. Ishibashi, W. Yang, J. W. Myerson, R. Y. Kiseleva, P. Aprelev, E. D. Hood, D. Stambolian, P. Seale, V. R. Muzykantov and D. Huh, ACS Nano, 2019, 13, 7627–7643 CrossRef CAS PubMed.
- J. Kim, Y. Song, A. L. Jolly, T. Hwang, S. Kim, B. Lee, J. Jang, D. H. Jo, K. Baek, T. L. Liu, S. Yoo and N. L. Jeon, Adv. Mater. Technol., 2024, 9, 569537 Search PubMed.
- H. Ragelle, K. Dernick, S. Khemais, C. Keppler, L. Cousin, Y. Farouz, C. Louche, S. Fauser, S. Kustermann, M. W. Tibbitt and P. D. Westenskow, Adv. Healthcare Mater., 2020, 9, 2001531 CrossRef CAS PubMed.
- T. L. Maurissen, A. J. Spielmann, G. Schellenberg, M. Bickle, J. R. Vieira, S. Y. Lai, G. Pavlou, S. Fauser, P. D. Westenskow and R. D. Kamm, Nat. Commun., 2024, 15, 1372 CrossRef CAS PubMed.
- K. Achberger, C. Probst, J. Haderspeck, S. Bolz, J. Rogal, J. Chuchuy, M. Nikolova, V. Cora, L. Antkowiak, W. Haq, N. Shen, K. Schenke-Layland, M. Ueffing, S. Liebau and P. Loskill, eLife, 2019, 8, e46188 CrossRef PubMed.
- K. Achberger, M. Cipriano, M. J. Duchs, C. Schon, S. Michelfelder, B. Stierstorfer, T. Lamla, S. G. Kauschke, J. Chuchuy, J. Roosz, L. Mesch, V. Cora, S. Pars, N. Pashkovskaia, S. Corti, S. M. Hartmann, A. Kleger, S. Kreuz, U. Maier, S. Liebau and P. Loskill, Stem Cell Rep., 2021, 16, 2242–2256 CrossRef CAS PubMed.
- T. Su, L. Liang, L. Zhang, J. Wang, L. Chen, C. Su, J. Cao, Q. Yu, S. Deng, H. F. Chan, S. Tang, Y. Guo and J. Chen, Front. Bioeng. Biotechnol., 2022, 10 DOI:10.3389/fbioe.2022.939774.
- J. Yeste, M. García-Ramírez, X. Illa, A. Guimerà, C. Hernández, R. Simó and R. Villa, Lab Chip, 2018, 18, 95–105 RSC.
- S. J. Kim, J. S. Lim, J. H. Park and J. Lee, Invest. Ophthalmol. Visual Sci., 2023, 64, 35–35 CAS.
- I. Kocherova, A. Bryja, P. Mozdziak, A. Angelova Volponi, M. Dyszkiewicz-Konwińska, H. Piotrowska-Kempisty, P. Antosik, D. Bukowska, M. Bruska and D. Iżycki, J. Clin. Med., 2019, 8, 1602 CrossRef CAS PubMed.
- D. J. Medina-Leyte, M. Domínguez-Pérez, I. Mercado, M. T. Villarreal-Molina and L. Jacobo-Albavera, Appl. Sci., 2020, 10, 938 CrossRef CAS.
- H. Uwamori, Y. Ono, T. Yamashita, K. Arai and R. Sudo, Microvasc. Res., 2019, 122, 60–70 CrossRef CAS PubMed.
- P. Hughes, D. Marshall, Y. Reid, H. Parkes and C. Gelber, BioTechniques, 2007, 43, 575–586 CrossRef CAS PubMed.
- A. V. Cioanca, C.-L. Wu, R. Natoli, R. M. Conway, P. J. McCluskey, M. J. Jager, E. I. Sitiwin, S. S. Eamegdool and M. C. Madigan, Pigm. Cell Melanoma Res., 2021, 34, 928–945 CrossRef CAS PubMed.
- M. J. Song, R. Quinn, E. Nguyen, C. Hampton, R. Sharma, T. S. Park, C. Koster, T. Voss, C. Tristan, C. Weber, A. Singh, R. Dejene, D. Bose, Y. C. Chen, P. Derr, K. Derr, S. Michael, F. Barone, G. Chen, M. Boehm, A. Maminishkis, I. Singec, M. Ferrer and K. Bharti, Nat. Methods, 2023, 20, 149–161 CrossRef CAS PubMed.
- N. Kosyakova, D. D. Kao, M. Figetakis, F. López-Giráldez, S. Spindler, M. Graham, K. J. James, J. Won Shin, X. Liu, G. T. Tietjen, J. S. Pober and W. G. Chang, npj Regener. Med., 2020, 5, 1 CrossRef CAS PubMed.
- S. de Boer, S. Laan, R. Dirven and J. Eikenboom, PLoS One, 2024, 19, e0297465 CrossRef CAS PubMed.
- J. Nguyen, Y.-Y. Lin and S. Gerecht, Cell Stem Cell, 2021, 28, 1188–1204 CrossRef CAS PubMed.
- E. K. Markert, H. Klein, C. Viollet, W. Rust, B. Strobel, S. G. Kauschke, B. Makovoz, H. Neubauer, R. A. Bakker and T. A. Blenkinsop, Front. Cell Dev. Biol., 2022, 10 DOI:10.3389/fcell.2022.910040.
- R. A. Hazim, S. Volland, A. Yen, B. L. Burgess and D. S. Williams, Exp. Eye Res., 2019, 179, 18–24 CrossRef CAS PubMed.
- N. V. Strunnikova, A. Maminishkis, J. J. Barb, F. Wang, C. Zhi, Y. Sergeev, W. Chen, A. O. Edwards, D. Stambolian, G. Abecasis, A. Swaroop, P. J. Munson and S. S. Miller, Hum. Mol. Genet., 2010, 19, 2468–2486 CrossRef CAS PubMed.
- Z. B. Kaya, V. Santiago-Padilla, M. Lim, S. L. Boschen, P. Atilla and P. J. McLean, Sci. Rep., 2024, 14, 4775 CrossRef CAS PubMed.
- E. Tan, X.-Q. Ding, A. Saadi, N. Agarwal, M. I. Naash and M. R. Al-Ubaidi, Invest. Ophthalmol. Visual Sci., 2004, 45, 764–768 CrossRef PubMed.
- J. Hurst, G. Attrodt, K. U. Bartz-Schmidt, U. A. Mau-Holzmann, M. S. Spitzer and S. Schnichels, Int. J. Mol. Sci., 2023, 24, 13801 CrossRef CAS PubMed.
- N. J. Van Bergen, J. P. Wood, G. Chidlow, I. A. Trounce, R. J. Casson, W. K. Ju, R. N. Weinreb and J. G. Crowston, Invest. Ophthalmol. Visual Sci., 2009, 50, 4267–4272 CrossRef PubMed.
- E. L. Wagstaff, A. Heredero Berzal, C. J. F. Boon, P. M. J. Quinn, A. L. M. A. ten Asbroek and A. A. Bergen, Int. J. Mol. Sci., 2021, 22, 7081 CrossRef PubMed.
- A. Tony, I. Badea, C. Yang, Y. Liu, K. Wang, S. M. Yang and W. Zhang, Polymers, 2023, 15, 1006 CrossRef CAS PubMed.
- K. J. Regehr, M. Domenech, J. T. Koepsel, K. C. Carver, S. J. Ellison-Zelski, W. L. Murphy, L. A. Schuler, E. T. Alarid and D. J. Beebe, Lab Chip, 2009, 9, 2132–2139 RSC.
- U. M. N. Cao, Y. Zhang, J. Chen, D. Sayson, S. Pillai and S. D. Tran, Int. J. Mol. Sci., 2023, 24, 3232 CrossRef CAS PubMed.
- A. D. van der Meer, V. V. Orlova, P. ten Dijke, A. van den Berg and C. L. Mummery, Lab Chip, 2013, 13, 3562–3568 RSC.
- F. Regent, L. Morizur, L. Lesueur, W. Habeler, A. Plancheron, K. Ben M'Barek and C. Monville, Sci. Rep., 2019, 9, 10646 CrossRef PubMed.
- P. Wahle, G. Brancati, C. Harmel, Z. He, G. Gut, J. S. del Castillo, A. X. d. S. dos Santos, Q. Yu, P. Noser, J. S. Fleck, B. Gjeta, D. Pavlinić, S. Picelli, M. Hess, G. W. Schmidt, T. T. A. Lummen, Y. Hou, P. Galliker, D. Goldblum, M. Balogh, C. S. Cowan, H. P. N. Scholl, B. Roska, M. Renner, L. Pelkmans, B. Treutlein and J. G. Camp, Nat. Biotechnol., 2023, 41, 1765–1775 CrossRef CAS PubMed.
- A. Tresenrider, A. Sridhar, K. C. Eldred, S. Cuschieri, D. Hoffer, C. Trapnell and T. A. Reh, Cells Rep. Methods, 2023, 3, 100548 CrossRef CAS PubMed.
- A. E. Songstad, K. S. Worthington, K. R. Chirco, J. C. Giacalone, S. S. Whitmore, K. R. Anfinson, D. Ochoa, C. M. Cranston, M. J. Riker, M. Neiman, E. M. Stone, R. F. Mullins and B. A. Tucker, Stem Cells Transl. Med., 2017, 6, 1533–1546 CrossRef CAS PubMed.
- T. Qian, S. E. Maguire, S. G. Canfield, X. Bao, W. R. Olson, E. V. Shusta and S. P. Palecek, Sci. Adv., 2017, 3, e1701679 CrossRef PubMed.
- D. Y. Park, J. Lee, J. Kim, K. Kim, S. Hong, S. Han, Y. Kubota, H. G. Augustin, L. Ding, J. W. Kim, H. Kim, Y. He, R. H. Adams and G. Y. Koh, Nat. Commun., 2017, 8, 15296 CrossRef CAS PubMed.
- C. E. Paisley and J. N. Kay, Dev. Biol., 2021, 478, 144–154 CrossRef CAS PubMed.
- L. Chen, N. D. Perera, A. J. Karoukis, K. L. Feathers, R. R. Ali, D. A. Thompson and A. T. Fahim, Sci. Rep., 2022, 12, 12694 CrossRef CAS PubMed.
- M. O'Hara-Wright and A. Gonzalez-Cordero, Development, 2020, 147, dev189746 CrossRef PubMed.
- K. J. Wahlin, J. A. Maruotti, S. R. Sripathi, J. Ball, J. M. Angueyra, C. Kim, R. Grebe, W. Li, B. W. Jones and D. J. Zack, Sci. Rep., 2017, 7, 766 CrossRef PubMed.
- A. Saha, E. Capowski, M. A. F. Zepeda, E. C. Nelson, D. M. Gamm and R. Sinha, Cell Stem Cell, 2022, 29, 460–471.e463 CrossRef CAS PubMed.
- E. L. West, P. Majumder, A. Naeem, M. Fernando, M. O'Hara-Wright, E. Lanning, M. Kloc, J. Ribeiro, P. Ovando-Roche and I. O. Shum, Stem Cell Rep., 2022, 17, 775–788 CrossRef CAS PubMed.
- A. E. Ghareeb, M. Lako and D. H. Steel, Stem Cells Transl. Med., 2020, 9, 1531–1548 CrossRef PubMed.
- B. Dorgau, M. Felemban, G. Hilgen, M. Kiening, D. Zerti, N. C. Hunt, M. Doherty, P. Whitfield, D. Hallam and K. White, Biomaterials, 2019, 199, 63–75 CrossRef CAS PubMed.
- T. Akhtar, H. Xie, M. I. Khan, H. Zhao, J. Bao, M. Zhang and T. Xue, Stem Cell Res., 2019, 39, 101491 CrossRef CAS PubMed.
- T. DiStefano, H. Y. Chen, C. Panebianco, K. D. Kaya, M. J. Brooks, L. Gieser, N. Y. Morgan, T. Pohida and A. Swaroop, Stem Cell Rep., 2018, 10, 300–313 CrossRef CAS PubMed.
- B. Cakir, Y. Xiang, Y. Tanaka, M. H. Kural, M. Parent, Y. J. Kang, K. Chapeton, B. Patterson, Y. Yuan, C. S. He, M. S. B. Raredon, J. Dengelegi, K. Y. Kim, P. Sun, M. Zhong, S. Lee, P. Patra, F. Hyder, L. E. Niklason, S. H. Lee, Y. S. Yoon and I. H. Park, Nat. Methods, 2019, 16, 1169–1175 CrossRef CAS PubMed.
- X. Y. Sun, X. C. Ju, Y. Li, P. M. Zeng, J. Wu, Y. Y. Zhou, L. B. Shen, J. Dong, Y. J. Chen and Z. G. Luo, eLife, 2022, 11, e76707 CrossRef CAS PubMed.
- F. Soldner and R. Jaenisch, Cell, 2018, 175, 615–632 CrossRef CAS PubMed.
- T. L. Maurissen, M. Kawatou, V. López-Dávila, K. Minatoya, J. K. Yamashita and K. Woltjen, Sci. Rep., 2024, 14, 2586 CrossRef CAS PubMed.
- K. Mulfaul, N. K. Mullin, J. C. Giacalone, A. P. Voigt, M. R. DeVore, E. M. Stone, B. A. Tucker and R. F. Mullins, J. Pathol., 2022, 257, 29–38 CrossRef CAS PubMed.
- A. B. Garcia-Delgado, S. M. Calado, L. M. Valdes-Sanchez, A. Montero-Sanchez, B. Ponte-Zuñiga, B. de la Cerda, S. S. Bhattacharya and F. J. Diaz-Corrales, Stem Cell Res., 2019, 38, 101473 CrossRef CAS PubMed.
- L. Koolen, G. Gagliardi, S. C. A. ten Brink, A. de Breuk, T. J. Heesterbeek, C. B. Hoyng, S. Albert and A. I. den Hollander, Stem Cell Res., 2022, 60, 102669 CrossRef CAS PubMed.
- M. Farnoodian, D. Bose, V. Khristov, P. J. Susaimanickam, S. Maddileti, I. Mariappan, M. Abu-Asab, M. Campos, R. Villasmil and Q. Wan, Stem Cell Rep., 2022, 17, 2438–2450 CrossRef CAS PubMed.
- P. E. Sladen, A. Naeem, T. Adefila-Ideozu, T. Vermeule, S. L. Busson, M. Michaelides, S. Naylor, A. Forbes, A. Lane and A. Georgiadis, Int. J. Mol. Sci., 2024, 25, 1839 CrossRef CAS PubMed.
- J. C. Corral-Serrano, P. E. Sladen, D. Ottaviani, O. F. Rezek, D. Athanasiou, K. Jovanovic, J. Van der Spuy, B. C. Mansfield and M. E. Cheetham, Cells, 2023, 12, 1575 CrossRef CAS PubMed.
- M. Kaltak, P. de Bruijn, D. Piccolo, S.-E. Lee, K. Dulla, T. Hoogenboezem, W. Beumer, A. R. Webster, R. W. Collin and M. E. Cheetham, Mol. Ther.–Nucleic Acids, 2023, 31, 674–688 CrossRef CAS PubMed.
- A. I. den Hollander, R. K. Koenekoop, S. Yzer, I. Lopez, M. L. Arends, K. E. Voesenek, M. N. Zonneveld, T. M. Strom, T. Meitinger and H. G. Brunner, Am. J. Hum. Genet., 2006, 79, 556–561 CrossRef CAS PubMed.
- D. A. Parfitt, A. Lane, C. M. Ramsden, A.-J. F. Carr, P. M. Munro, K. Jovanovic, N. Schwarz, N. Kanuga, M. N. Muthiah and S. Hull, Cell Stem Cell, 2016, 18, 769–781 CrossRef CAS PubMed.
- R. W. Collin, A. I. Den Hollander, S. D. Van Der Velde-visser, J. Bennicelli, J. Bennett and F. P. Cremers, Mol. Ther.–Nucleic Acids, 2012, 1, e14 CrossRef PubMed.
- X. Gerard, I. Perrault, S. Hanein, E. Silva, K. Bigot, S. Defoort-Delhemmes, M. Rio, A. Munnich, D. Scherman and J. Kaplan, Mol. Ther.–Nucleic Acids, 2012, 1, e29 CrossRef PubMed.
- K. Dulla, M. Aguila, A. Lane, K. Jovanovic, D. A. Parfitt, I. Schulkens, H. L. Chan, I. Schmidt, W. Beumer and L. Vorthoren, Mol.
Ther.–Nucleic Acids, 2018, 12, 730–740 CrossRef CAS PubMed.
- S. R. Russell, A. V. Drack, A. V. Cideciyan, S. G. Jacobson, B. P. Leroy, C. Van Cauwenbergh, A. C. Ho, A. V. Dumitrescu, I. C. Han and M. Martin, Nat. Med., 2022, 28, 1014–1021 CrossRef CAS PubMed.
- S. Albert, A. Garanto, R. Sangermano, M. Khan, N. M. Bax, C. B. Hoyng, J. Zernant, W. Lee, R. Allikmets, R. W. J. Collin and F. P. M. Cremers, Am. J. Hum. Genet., 2018, 102, 517–527 CrossRef CAS PubMed.
- I. Vázquez-Domínguez, C. H. Li, Z. Fadaie, L. Haer-Wigman, F. P. Cremers, A. Garanto, C. B. Hoyng and S. Roosing, Invest. Ophthalmol. Visual Sci., 2022, 63, 27–27 CrossRef PubMed.
- J. C. Corral-Serrano, I. J. Lamers, J. van Reeuwijk, L. Duijkers, A. D. Hoogendoorn, A. Yildirim, N. Argyrou, R. A. Ruigrok, S. J. Letteboer and R. Butcher, Proc. Natl. Acad. Sci. U. S. A., 2020, 117, 9922–9931 CrossRef CAS PubMed.
- M. Khan, G. Arno, A. Fakin, D. A. Parfitt, P. P. Dhooge, S. Albert, N. M. Bax, L. Duijkers, M. Niblock and K. L. Hau, Mol. Ther.–Nucleic Acids, 2020, 21, 412–427 CrossRef CAS PubMed.
- F. Chahine Karam, T. H. Loi, A. Ma, B. M. Nash, J. R. Grigg, D. Parekh, L. G. Riley, E. Farnsworth, B. Bennetts, A. Gonzalez-Cordero and R. V. Jamieson, J. Pers. Med., 2022, 12, 502 CrossRef PubMed.
- A. Westhaus, S. S. Eamegdool, M. Fernando, P. Fuller-Carter, A. A. Brunet, A. L. Miller, R. Rashwan, M. Knight, M. Daniszewski and G. E. Lidgerwood, Sci. Rep., 2023, 13, 21946 CrossRef CAS PubMed.
- S. N. Bhatia and D. E. Ingber, Nat. Biotechnol., 2014, 32, 760–772 CrossRef CAS PubMed.
- A. D. Van Der Meer and A. Van Den Berg, Integr. Biol., 2012, 4, 461–470 CrossRef CAS PubMed.
- D. Huh, Y.-S. Torisawa, G. A. Hamilton, H. J. Kim and D. E. Ingber, Lab Chip, 2012, 12, 2156–2164 RSC.
- V. K. Gullapalli, I. K. Sugino, Y. Van Patten, S. Shah and M. A. Zarbin, Exp. Eye Res., 2005, 80, 235–248 CrossRef CAS PubMed.
- B. Molins, A. Rodríguez, V. Llorenç and A. Adán, Neural Regener. Res., 2024, 19, 2626–2636 CrossRef CAS PubMed.
- J. Gong, O. Sagiv, H. Cai, S. H. Tsang and L. V. Del Priore, Exp. Eye Res., 2008, 86, 957–965 CrossRef CAS PubMed.
- A. Al-Ani, S. Sunba, B. Hafeez, D. Toms and M. Ungrin, Int. J. Mol. Sci., 2020, 21, 6066 CrossRef CAS PubMed.
- M. Paulsson, Crit. Rev. Biochem. Mol. Biol., 1992, 27, 93–127 CrossRef CAS PubMed.
- B. Lu, D. Zhu, D. Hinton, M. S. Humayun and Y.-C. Tai, Biomed. Microdevices, 2012, 14, 659–667 CrossRef CAS PubMed.
- M. Zakharova, M. P. Tibbe, L. S. Koch, H. Le-The, A. M. Leferink, A. van den Berg, A. D. van der Meer, K. Broersen and L. I. Segerink, Adv. Mater. Technol., 2021, 6, 2100138 CrossRef CAS.
- T. I. Harris, C. A. Paterson, F. Farjood, I. D. Wadsworth, L. Caldwell, R. V. Lewis, J. A. Jones and E. Vargis, ACS Biomater. Sci. Eng., 2019, 5, 4023–4036 CrossRef CAS PubMed.
- E. Rickabaugh, D. Weatherston, T. I. Harris, J. A. Jones and E. Vargis, ACS Biomater. Sci. Eng., 2023, 9, 5051–5061 CrossRef CAS PubMed.
- M. Yazdani, Curr. Eye Res., 2022, 47, 651–660 CrossRef PubMed.
- D. T. Hass, Q. Zhang, G. A. Autterson, R. A. Bryan, J. B. Hurley and J. M. Miller, Invest. Ophthalmol. Vis. Sci., 2023, 64, 4 CrossRef CAS PubMed.
- M. Lajko, H. J. Cardona, J. M. Taylor, R. S. Shah, K. N. Farrow and A. A. Fawzi, PLoS One, 2016, 11, e0166886 CrossRef PubMed.
- J. Wang, M. Li, Z. Geng, S. Khattak, X. Ji, D. Wu and Y. Dang, Oxid. Med. Cell. Longevity, 2022, 2022, 7836828 Search PubMed.
- P. M. J. Quinn and J. Wijnholds, Genes, 2019, 10, 987 CrossRef CAS PubMed.
- A. Ueda, M. Koga, M. Ikeda, S. Kudo and K. Tanishita, Am. J. Physiol., 2004, 287, H994–H1002 CAS.
- P. Tawade and M. Mastrangeli, ChemBioChem, 2024, 25, e202300560 CrossRef CAS PubMed.
- H. Chen, Z. Luo, X. Lin, Y. Zhu and Y. Zhao, Nano Res., 2023, 16, 1–28 CrossRef.
- S. H. Kann, E. M. Shaughnessey, X. Zhang, J. L. Charest and E. M. Vedula, Analyst, 2023, 148, 3204–3216 RSC.
- C. Probst, S. Schneider and P. Loskill, Curr. Opin. Biomed. Eng., 2018, 6, 33–41 CrossRef.
- C. Bavik, S. H. Henry, Y. Zhang, K. Mitts, T. McGinn, E. Budzynski, A. Pashko, K. L. Lieu, S. Zhong, B. Blumberg, V. Kuksa, M. Orme, I. Scott, A. Fawzi and R. Kubota, PLoS One, 2015, 10, e0124940 CrossRef PubMed.
- V. Y. Arshavsky, T. D. Lamb and E. N. Pugh Jr, Annu. Rev. Physiol., 2002, 64, 153–187 CrossRef CAS PubMed.
- K. A. Lucas, G. M. Pitari, S. Kazerounian, I. Ruiz-Stewart, J. Park, S. Schulz, K. P. Chepenik and S. A. Waldman, Pharmacol. Rev., 2000, 52, 375–414 CrossRef CAS PubMed.
- L. Li, H. Zhao, H. Xie, T. Akhtar, Y. Yao, Y. Cai, K. Dong, Y. Gu, J. Bao and J. Chen, Stem Cells, 2021, 39, 959–974 CrossRef CAS PubMed.
- D. Hallam, G. Hilgen, B. Dorgau, L. Zhu, M. Yu, S. Bojic, P. Hewitt, M. Schmitt, M. Uteng and S. Kustermann, Stem Cells, 2018, 36, 1535–1551 CrossRef CAS PubMed.
- C. Celiker, K. Weissova, K. A. Cerna, J. Oppelt, B. Dorgau, F. M. Gambin, J. Sebestikova, M. Lako, E. Sernagor, P. Liskova and T. Barta, iScience, 2023, 26, 107237 CrossRef CAS PubMed.
- D. M. Nahon, R. Moerkens, H. Aydogmus, B. Lendemeijer, A. Martínez-Silgado, J. M. Stein, M. Dostanić, J.-P. Frimat, C. Gontan and M. N. de Graaf, Nat. Biomed. Eng., 2024, 1–22 Search PubMed.
- J. Li, J. Wang, I. L. Ibarra, X. Cheng, M. D. Luecken, J. Lu, A. Monavarfeshani, W. Yan, Y. Zheng, Z. Zuo, S. L. Z. Colborn, B. S. Cortez, L. A. Owen, N. M. Tran, K. Shekhar, J. R. Sanes, J. T. Stout, S. Chen, Y. Li, M. M. DeAngelis, F. J. Theis and R. Chen, Res. Sq., 2023 DOI:10.21203/rs.3.rs-3471275/v1.
- P. H. Zushin, S. Mukherjee and J. C. Wu, J. Clin. Invest., 2023, 133, e175824 CrossRef CAS PubMed.
- J. A. Beck, S. Lloyd, M. Hafezparast, M. Lennon-Pierce, J. T. Eppig, M. F. Festing and E. M. Fisher, Nat. Genet., 2000, 24, 23–25 CrossRef CAS PubMed.
- D. M. Stresser, A. K. Kopec, P. Hewitt, R. N. Hardwick, T. R. Van Vleet, P. K. S. Mahalingaiah, D. O'Connell, G. J. Jenkins, R. David and J. Graham, Nat. Biomed. Eng., 2023, 1–6 Search PubMed.
- P. L. Candarlioglu, G. Dal Negro, D. Hughes, F. Balkwill, K. Harris, H. Screen, H. Morgan, R. David, S. Beken, O. Guenat, W. Rowan and A. Amour, Biochem. Soc. Trans., 2022, 50, 665–673 CrossRef CAS PubMed.
- S. R. A. Kratz, G. Höll, P. Schuller, P. Ertl and M. Rothbauer, Biosensors, 2019, 9, 110 CrossRef CAS PubMed.
- S. H. Kann, E. M. Shaughnessey, J. R. Coppeta, H. Azizgolshani, B. C. Isenberg, E. M. Vedula, X. Zhang and J. L. Charest, Microsyst. Nanoeng., 2022, 8, 109 CrossRef CAS PubMed.
- M. A. Holzreuter and L. I. Segerink, Lab Chip, 2024, 24, 1121–1134 RSC.
- A. R. Vollertsen, A. Vivas, B. van Meer, A. van den Berg, M. Odijk and A. D. van der Meer, Biomicrofluidics, 2021, 15, 051301 CrossRef CAS PubMed.
- M. Piergiovanni, S. B. Leite, R. Corvi and M. Whelan, Lab Chip, 2021, 21, 2857–2868 RSC.
- Y. Wang and H. Jeon, Trends Pharmacol. Sci., 2022, 43, 569–581 CrossRef CAS PubMed.
- J. Ko, D. Park, J. Lee, S. Jung, K. Baek, K. E. Sung, J. Lee and N. L. Jeon, Nat. Rev. Bioeng., 2024, 2, 453–469 CrossRef CAS.
- W. Anderson and M. Sarmadi, J. Stud. Res., 2024, 13, 6102 Search PubMed.
Footnote |
† Contributed equally to this work. |
|
This journal is © The Royal Society of Chemistry 2025 |
Click here to see how this site uses Cookies. View our privacy policy here.