DOI:
10.1039/D4LC00869C
(Perspective)
Lab Chip, 2025, Advance Article
Microfluidics for morpholomics and spatial omics applications
Received
14th October 2024
, Accepted 10th January 2025
First published on 10th January 2025
Abstract
Creative designs, precise fluidic manipulation, and automation have supported the development of microfluidics for single-cell applications. Together with the advancements in detection technologies and artificial intelligence (AI), microfluidic-assisted platforms have been increasingly used for new modalities of single-cell investigations and in spatial omics applications. This review explores the use of microfluidic technologies for morpholomics and spatial omics with a focus on single-cell and tissue characterization. We emphasize how various fluid dynamic principles and unique design integrations enable highly precise fluid manipulation, enhancing sample handling in morpholomics. Additionally, we examine the use of microfluidics-assisted spatial barcoding with micrometer resolutions for the spatial profiling of tissue specimens. Finally, we discuss how microfluidics can serve as a bridge for integrating multiple unique fields in omics research and outline key challenges that these technologies may face in practical translation.
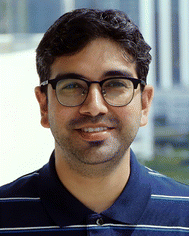 Nishanth Venugopal Menon | Nishanth Venugopal Menon is a Senior Postdoctotal Fellow at the National University of Singapore (NUS). He earned his Ph.D. in Biomedical Engineering from the Nanyang Technological University (NTU), Singapore and has worked as a Postdoctoral Fellow at NTU and NUS. He works on cancer research and is focused on developing microfluidics-based platforms for cancer screening and diagnosis. Another research focus is developing organ-on-chips to study disease pathology and progression for pharmaceutical applications. |
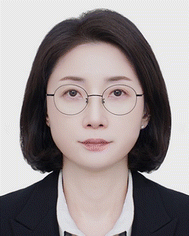 Jeeyeon Lee | Jeeyeon Lee is a Senior Research Fellow at the Institute of Health Innovation & Technology (iHealthtech) at the National University of Singapore (NUS). She earned her B. A. and M. A. degrees from Korea University, South Korea and completed her Ph.D. in Chemical Biology at Purdue University, USA. After her doctoral studies, she conducted postdoctoral research at The Scripps Research Institute before joining NUS. Her research focuses on host–microbe interactions and developing microfluidic drug testing platforms. |
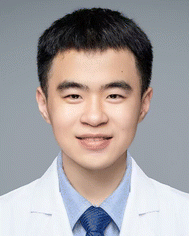 Tao Tang | Tao Tang is a Principal Investigator in the Department of Neurosurgery at Chongqing General Hospital, Chongqing University, China. He has held this position since 2024. Dr. Tang earned his Ph.D. in Materials Science from the Nara Institute of Science and Technology (Japan) in 2022 and subsequently worked as a Research Fellow in Biomedical Engineering at the National University of Singapore. His research focuses on glioblastoma and its microenvironment, employing techniques such as organ-on-a-chip models, microfluidics, and artificial intelligence. Currently, his work centers on understanding how tumor and immune cells reshape both the glioblastoma microenvironment and themselves. |
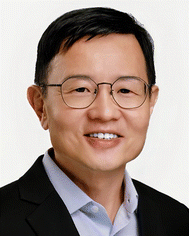 Chwee Teck Lim | Professor Chwee Teck Lim is the NUSS Chair Professor and Director of the Institute for Health Innovation and Technology at the National University of Singapore. His research interests are in human disease mechanobiology and wearable microfluidic biomedical technologies. He has coauthored over 500 journal publications and cofounded six startups. Prof Lim and his team have garnered over 120 research awards and honors including being elected fellow in nine academies including the UK Royal Society, Nature Lifetime Achievement Award for Mentoring in Science, ASEAN Outstanding Engineering Achievement Award, Asian Scientists 100, Wall Street Journal Asian Innovation Award and the President's Technology Award. |
Introduction
With over 40 years of development, microfluidics systems have been extensively studied and used owing to the size effect coupled with precise spatio-temporal fluid manipulation, ensuring its integration for miniaturization of conventional biotechnological assays. Coupled with its integration with electronics,1 photonics,2 nanomaterials,3 plasmonics4 and advanced microscopy,5 microfluidics improves sensitivity for biological sample analysis with lowered sample requirements and opportunities for automation. Microfluidic systems have been used across multiple applications such as disease monitoring6,7 and modeling,8 single cell analysis,9 food quality,10 environmental applications,11 biomanufacturing,11,12 drug development,13 and plant biology.14 Notable applications of microfluidics include flow cells in next-generation sequencing (NGS) for parallelized sequencing of millions of DNA fragments,15,16 microfluidic chip electrophoresis (MCE) for isolation of proteins,17,18 and digital PCR (dPCR) for nucleic acid quantification.19
Recent advancements in machine learning and AI have enhanced the data analytics capabilities20 opening new horizons for microfluidic-assisted applications. Complimentary developments in material sciences,21–24 robotics,25 and biology16 further expand the potential of microfluidics-based systems. This versatility of microfluidic platforms stems from the inherent advantages of miniaturization and the mature microfabrication techniques and design tools used to create such platforms. These traits make them invaluable for sample manipulation, especially in biomedical sciences. Also, their miniature size, in the order of single cells enables its use in applications requiring sub-cellular resolutions. As such, multiple strategies have been extensively used in single-cell molecular biology studies. Microvalves,26,27 microwells,28 hydrodynamic trapping,29 and droplet microfluidic systems30,31 are the common classification of microfluidic-based techniques for sample handling in single-cell omics. By integrating these platforms with suitable chemistry or detection methodologies, genomic, transcriptomic, epigenomic, and proteomic information can be gathered from a single cell. Numerous reviews are available discussing the microfluidics-based platforms used for single-cell omics32 (Fig. 1).
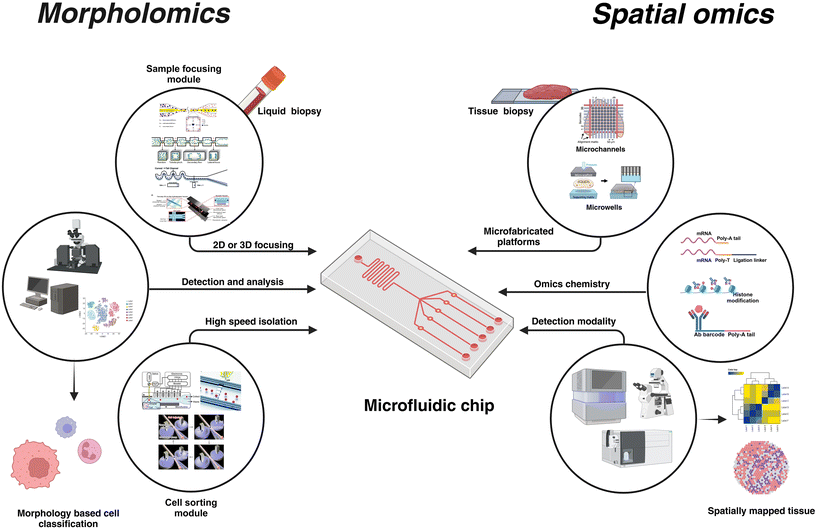 |
| Fig. 1 Microfluidics is a versatile and customizable technology that can be tailored to the application. For morpholomics, microfluidic platforms incorporate multiple modules such as the sample focusing module and cell sorting module, apart from its integration into sophisticated optical systems for high-speed single-cell imaging. Microfluidics for spatial omics offers the flexibility of using simple microchannels that can house different detection chemistry for multi-omics investigation. Unique molecular identifiers (UMI) are used to tag the spatial location in the tissue and respective detection modalities are used to complete the omics analysis. Recreated with permissions from ref. 33–40. Created in BioRender. Menon, N. (2024) https://www.BioRender.com/k90w460. | |
This review highlights two emerging yet distinct fields – morpholomics and spatial omics – where advancements in microfabrication techniques and sample handling have shown enormous promise. Morpholomics utilizes cell morphology as a modality for pathogenesis.41,42 Features such as cell geometry, nucleus shape, nucleus-to-cytoplasm ratio, and granularity are effective indicators of the cellular function and state.43,44 While pathologists rely on observable cues to differentiate cells, improvements in AI, computational biology tools, and optics can allow the extraction of high dimensional data from images that are not discernable to the human eye.45–47 This deeper understanding of the functional states of the cells can aid in diagnosis, prognosis, and therapeutics development. While single cell studies have largely focused on basic morphological analysis alongside in-depth genomic, transcriptomic, or proteomic characterization of single cells, morpholomics aims to revolutionize single-cell morphological analysis through high throughput imaging, and computational biology for diagnostic and therapeutic applications.
Spatial omics, another investigative tool that is gaining increasing interest, merges spatial information about cellular organization in the tissue with molecular profiling (genomics, proteomics, and transcriptomics) to generate spatial atlases of the tissue. While scRNA-seq is suitable for liquid biopsy or hematological disorders, traditional sequencing modalities require tissue dissociation into single cells. These methods compromise the spatial information, which is a major contributor to cellular heterogeneity in tissues.48 For example, in cancer research, the correlation of omics information to spatial mapping will help unravel the functional state of the tumor cells and the composition of the tumor microenvironment (TME), identify biomarkers, and guide the development of therapeutics.49 Similar advantages of tissue spatial information have been highlighted in aging,50 neuroscience,51 immunology,52 and microbiome studies.53
Despite their unique propositions, morpholomics and spatial omics share a common goal as investigative tools for understanding human biology. They differ in several key aspects: sample types (single cell vs. tissue), modalities (cell morphology vs. RNA, protein, DNA), and methodologies (sample processing, instrumentation, and data collection). Nevertheless, the foundational studies of microfluidics have enabled its usage in such diverse applications. It holds the promise of integrating morpholomics and spatial omics into a unified platform, potentially leading to the development of a new generation of assays for biology and biomedical applications.
Microfluidics for morpholomics
Morpholomics combines highly resolved cell morphological information with deep learning to achieve unprecedented insights into cell phenotypes and functions. Traditional technologies for cell morphology investigations such as microscopy and fluorescence-activated cell sorting (FACS), have served as gold standards for morphological analysis. Yet, they suffer from limitations such as low throughput high dimensional data from tissue samples for microscopes54 and high throughput low dimensional morphological data (granularity and size) from single cells for FACS.55 Imaging flow cytometers (IFC) are alternatives that combine the high content capabilities of microscopy with the high throughput feature of FACS.56,57 However, existing IFCs are suitable only for large sample operations and are not well-suited for sterile operations. Moreover, these systems employ a trade-off between cell sorting and the extent of cell morphology data collected. Microfluidics offers significant advantages owing to its miniature size and low-cost production, making it available as disposable cartridges. Also, the gentle operational requirements can ensure very high viability of the cells processed through such platforms. Integration of multiple functional components into a single platform ensures that the eventual lab-on-chip system incorporates debris isolation, sample-focusing features, high-resolution imaging zones, and cell sorting capabilities. Additionally, advancements in imaging technologies and high-speed data processing have facilitated the extraction of complex morphological signatures for their accurate classification, which is otherwise challenging. In this section, we discuss several microfluidics-based imaging flow cytometers that showcase simple microfluidic technologies being integrated with sophisticated optical detection systems and in most cases complemented by an intelligent AI based data processing units. Based on the components a prerequisite for morpholomics is a system integrating multiple sub-systems; a fluidic system for sample handling, a robust detection unit to collect direct and indirect signals of cell morphology, a strong computational component to analyze and process high-content data at high speeds, and a cell sorting system that works in tandem with the detection and data processing unit for cell isolation or sorting. Microfluidic approaches such as droplet microfluidics, hydrodynamic trapping, inertial microfluidics, and digital microfluidics have previously been used for single-cell investigation.58,59 These platforms isolate cells based on specific characteristics such as cell size or antibody markers. The isolated cells are explored further through downstream processing including subsequent culture and expansion for drug testing applications or sequencing for disease diagnosis. While some of these approaches offer integrability with real-time detection, they do not favor precise spatial and temporal alignment of cells which is key for sequential probing as is the case in cell morphology assessment (Fig. 2).
Sorting enabled microfluidic platforms
To this end, deep cell introduced REM-I by combining high-speed imaging, artificial intelligence (AI), and microfluidics for real-time and label-free single-cell sorting (Fig. 2a). The platform assesses high dimensional cell morphology to understand cell heterogeneity60 from brightfield images acquired through a high-speed CMOS camera. The integrated microfluidic chip combines multiple features to manipulate cell alignment and placement within the channel. In brief, the REM-I chip includes a multi-orifice channel to generate inertial lift forces that ensure cell alignment along the channel walls, a series of asymmetric sinusoidal channel expansions and contractions for 3D cell focusing necessary to ensure that the cells are aligned along the center of the microchannel (focal plane) for optical imaging.61 Besides, the chip incorporates an ordering region created using a staged channel design comprising curved and straight pinched sections that generate inertial lift forces and dean drag forces to space the particles for efficient imaging and subsequent sorting. The platform also allows for 6-way sorting using valve action controlled by pneumatic actuation. Alternate systems make use of active particle-focusing approaches with a piezoelectric transducer to generate an acoustic standing wave to precisely position the particles or cells in 2D, at a desired plane (along the longitudinal axis) for probing. Nitta et al. combined high-speed-multispectral imaging using a CMOS detector and a 3-stage flow focusing approach that included two stages of sheath flow to position the cells in the center of the microfluidic channel for imaging (Fig. 2b). To minimize the drift of the cells downstream, a PZT transducer was used to re-center the cells prior to sorting with an on-chip dual membrane push–pull sorter.40 Suzuki et al. combined the use of stimulated Raman scattering (SRS) as the detection modality and active focusing mechanism on microfluidics for sample handling to achieve label-free cell classification cells based on molecular signatures.62 By adding deep-learning the authors demonstrated the ability to conduct label-free cancer detection in blood and metabolic activity of microalgal cells. Using a 3-stage particle focusing approach and an on-chip sorter, this study was further extended to enable label-free cell detection and isolation.63 Another method of indirect cell morphology measurement was demonstrated by Ota et al.64 This method of ghost cytometry (Fig. 2c), commercialized by Thinkcyte Inc., uses a single-pixel detector for image-free morphology-based cytometry. The microfluidic chip used here employs a multi-stage system comprising a 3D hydrodynamic focusing section followed by voltage driven PZT actuator to perform high-speed sorting. In ghost cytometry, cells are passed across a static randomly structured light illumination to record the temporal information and the intensity distribution. Coupled with machine learning, the data recorded from the detector is used for cell classification at throughputs of about 3000 events per s.
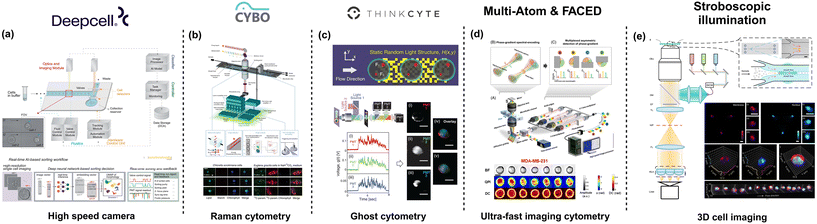 |
| Fig. 2 Microfluidic-based technologies for cell morphology profiling. (a) Deep cell offers high throughput image classification and sorting based on AI-processed images. Figure recreated from ref. 60. (b) CYBO provides a Raman-based single-cell detection and sorting system, enabling label-free measurement of intracellular molecules. Figure recreated from ref. 63; (c) THINKCYTE designed Ghost cytometry, demonstrating that clear cell images are not essential for cell identification figure recreated from ref. 64. (d) Multi-ATOM & FACED developed ultra-fast imaging cytometry for label-free cell detection and sorting. Figure recreated from ref. 65 and 66; and (e) stroboscopic illumination was used to achieve high speed 3D cell imaging. Figure recreated from ref. 67. Created in https://BioRender.com. | |
Microfluidic platforms without sorting
While cell sorting is integral to morpholomic applications, multiple microfluidics-based imaging flow cytometer platforms have been developed that allow for high throughput cell imaging but without the presence of a sorting subsystem. Wu et al., employed a simple straight microfluidic channel to focus particles based on inertial focusing. Despite the simple microfluidics, the authors introduce a novel approach of using variations of quantitative phase imaging (QPI); free-space angular-chirp-enhanced-delay (FACED)65 and multiplexed asymmetric-detection time-stretch optical microscopy (multi-ATOM), to analyze the single-cell biophysical characteristics66,68 (Fig. 2d). This approach has been demonstrated to operate at throughputs of over 10
000 events per s, which is on par with the best FACS systems. Holzner et al. described a multi-parametric imaging flow cytometer that allowed brightfield and fluorescence imaging of single cells at a throughput of higher than 10
000 events per s and subcellular resolution.69 The authors used a high aspect ratio microfluidic device about 90 mm in length, 665 μm wide, and 59 μm deep. Particle focusing on a single dimension (focal plane) was achieved using elasto-inertial flow using a viscoelastic buffer such as polyethylene oxide (PEO). A key component of the system is the stroboscopic illumination, to minimize motion blur, coupled with a CMOS camera for image acquisition. By parallelizing the microfluidic channels, Rane et al. achieved a throughput of over 60
000 events per s with stroboscopic illumination.70 Noteworthy that in this study, the authors used a winding channel to bring forth 3D inertial focusing of the cells. In a recent publication, Hua et al.,67 developed a light-field cytometer that improved on the advantages of stroboscopic illumination for multiparametric 3D cell imaging at a throughput of over 5000 cells per s (Fig. 2e). Using a simple microfluidic channel with sheath flow to focus the cells in 2D, the authors achieved volumetric visualization of single cells with a subcellular resolution closer to the diffraction limit (400 nm to 600 nm). Hence, by leveraging the extensive body of work in microfluidics and with significant improvements to the optical setup and computational capabilities, a wide variety of high throughput-high dimensional data systems have been demonstrated for assessing cell morphology at high resolutions that can be useful for the growing field of morpholomics.
Microfluidics for spatial OMICS
The biological process in eukaryotic cells is driven by genomic regulations and the subcellular localization of proteins within different cellular compartments.71 Understanding the spatial localization, distribution, and organization of mRNA and proteins is crucial for deciphering cellular heterogeneity within tissues. This knowledge is essential for unravelling cellular dysfunctions and various pathological states, which can lead to significant advancements in disease diagnosis and treatment.72,73 Spatial omics represents a transformative approach in this context, integrating spatial information with molecular data to provide a comprehensive view of cellular behaviour.74 Various microfluidic strategies including droplet microfluidics, valve-based chips, and hydrodynamic traps-based platforms, have been effective for single omics or multi-omics analysis. For spatial studies, the generation of spatial barcodes, a platform for in situ tagging of the target molecules, and the analysis modality – imaging-based, sequencing-based, or mass-spectrometry-based – play a vital role. Microfluidic platforms offer multiple advantages including design flexibility for channel multiplexing with single-cell resolution, precise fluid manipulations, and automation. Customization and adaptability for multi-omics applications are significant features of microfluidic-based technology. The ability to tailor different chemical reactions for different targets enables easy substrate preparation by altering the reagent to achieve multi-omics applications.
The chemical reactions used in spatial omics depend on the target analytes and the detection modality. To capture the spatial position of the analytes specific barcodes or unique molecular identifiers (UMI) are utilized. For instance, in proteomics, fluorescent antibodies are used for imaging-based detection,75 while metal-ion conjugated antibodies are used for imaging-based mass spectrometry (IMC).76 Alternatively for multi-omics applications using a specific modality such as sequencing, modifications to the chemistry are required. Although sequencing is a common approach for spatial transcriptomics, it is not applicable for direct assessment of proteins. However, by tagging proteins with antibodies conjugated to spatial DNA barcode or antibody-derived DNA tags (ADT), the spatial information of the proteins can be converted into DNA sequences. These sequences can be reverse transcribed and amplified for an indirect measure of protein abundance. This approach offers several advantages, including the amplification of signals from low-abundance proteins, quantitative results through molecular indices, limitless multiplexing, and compatibility with immunofluorescence imaging and other multi-omics approaches. Alternatively, a multi-modal approach can be used to detect different analytes.77 For example, sequential sample preparation can be performed to ensure spatial transcriptomics via sequencing followed by mass spectrometry for spatial proteomics analysis.78 Such integration of techniques enables a more comprehensive understanding of cellular dynamics and enhances the overall depth of spatial omics investigations (Fig. 3).
Microchannel-based platforms
Another key aspect of spatial omics is the sample preparation that can be achieved using microfabricated technologies. Broadly such technologies can be classified as microchannel-based, microarray-based and micro-scaffold-based platforms. Microchannel-based platforms, such as DBIT-seq77 and spatial Cite-seq79 utilize microchannel to patterns antibodies conjugated with spatial DNA barcodes (Fig. 3a). The microfluidic platform includes 50 equally spaced microchannels with dimensions from 50 μm to 10 μm. The chip is clamped onto the tissue slice and reagents are introduced through the channels. For a spatial transcriptomic pipeline, the reagent includes a barcode with oligo-dT sequence to bind to mRNA, spatial barcode (Ai), and a ligation linker. Subsequently, in situ reverse transcription is performed to synthesize cDNA which readily binds to the spatial barcode. This configuration provides 1-D information on the chip. To generate pixelated spatial data, the microfluidic chip is removed to make way for another chip with similar microchannel design and dimension to facilitate a crossflow scheme by clamping the chip perpendicular to the orientation of the first chip. A reagent composed of a ligation linker, unique spatial barcode (Bj), UMI, a PCR handle, T4 ligase and complimentary ligation linker. In situ ligation is performed at the intersected portions of the channels resulting in a pixelated mosaic pattern with a combined barcode (AiBj). For multi-omics investigations, the DBIT-seq workflow can be modified to integrate different chemistry such as spatial CITE-seq for the simultaneous assessment of transcriptomes and proteins. In brief, an additional step of antibody-derived DNA tags (ADT) is included prior to the in situ ligation of the barcodes. The DNA tags contain a unique barcode for the target proteins and a poly(A) tail that can be annealed to the oligo-dT sequence in the reverse transcription primer. Finally, optical or fluorescent imaging can be performed to generate the map of the transcriptome or proteins. DBIT-seq initially maps a panel of 22 proteins, while spatial Cite-seq demonstrated spatial profiling of 189 proteins in mouse tissue, and 273 proteins in human tissue using ∼200–300 ADT tags, highlighting its capability for relatively high-throughput (−100 protein) spatial proteomic profiling. Along with transcriptome and proteins, epigenomes can also be spatially resolved using the DBIT-seq platform.80,81 Spatial-ATAC-seq is a platform based on the DBIT-seq chip for spatial accessible chromatin while Spatial CUT & Tag-seq has been demonstrated as a multi-omics methodology used for spatial ATAC (chromatin accessibility) and trancriptomics based on sequencing. Wirth et al., used a multiplexed DBIT-seq (xDBIT-seq) to investigate 9 tissue slices in parallel with pixelated transcriptomic spots of 50 μm × 50 μm.39 Matrix-seq is another approach making use of a similar configuration as that used in DBIT-seq.82 However, instead of relying on in situ hybridization, the spatial barcodes are patterned on the pre-treated glass slide followed by in situ capture and sequencing. Pre-patterning the glass slide improves user-friendliness and simplifies the overall protocol followed. Recently, Zhu et al., showcased another multiplexed crossflow microfluidic setup (MAGIC-seq) making use of a combination of serpentine and straight channels to create a grid of transcriptomic spots83 (Fig. 3b). Depending on the combination of designs up to 9 capture areas can be developed on a slide with each area measuring 7 mm × 7 mm with 4900 spots of 50 μm × 50 μm. The chip was used to prefabricate a barcoded DNA array to improve robustness and reliability. Tissue sections are mounted on the patterned spot followed by in situ mRNA capture, reverse transcription and cDNA amplification. Sequencing was eventually used to achieve the spatial transcriptomic signatures of the tissue. Taken together, the versatility and inertness of microfluidics enables the integration of multiple spatial omics modalities to map different targets, thereby providing a detailed view of the mechanisms underlying the spatial organization of the cells in a tissue. It is noteworthy that all the microfluidic setup discussed employ a similar approach of using crossflow to pattern the spots. Importantly, similar chip configurations have been adopted irrespective of the type of omics modality signifying the adaptability and the potential to serve as a standard platform to synchronize multi-omics research.
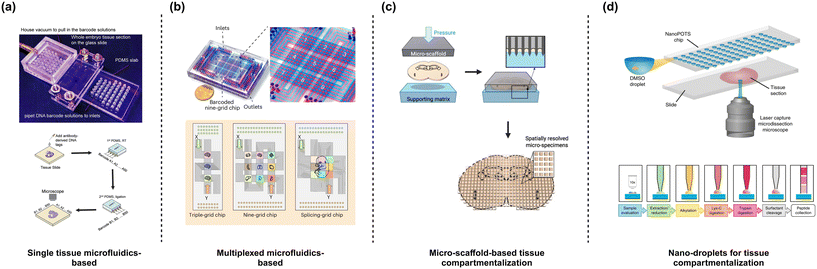 |
| Fig. 3 Designs of a microfluidic device for spatial omics. (a) DBIT-seq. Microfluidic channels for generating barcoded spots make use of a crossflow. Figure recreated from ref. 77. (b) MAGIC-seq follows a similar approach to DBIT-seq except for using serpentine microfluidic channels that enable multiplexing. Figure recreated from ref. 83, (c) MASP uses microwells sectioned using a sharp-edged micro-scaffold with a spatial resolution of ∼100 μm. Figure recreated from ref. 38. (d) NanoPOTs use microwells where small tissue sections (smallest section of ∼20 μm) are generated using laser capture microdissection and captured within customized microwells. Figure recreated from ref. 84 and 85. Created in BioRender. Menon, N. (2025) https://BioRender.com/i18q543. | |
Microwell-based platforms
3D printed micro-scaffolds have also been employed for spatial proteomics applications where ∼900 microwells with dimensions 400 × 400 μm are used for tissue compartmentalization (Fig. 3c). Herein, the tissue to be probed is immobilized and micro-dissected using the scaffold into individual microwells.38 The section of the tissue in the individual wells are lysed and analysed by liquid chromatography-mass spectrometry (LC-MS), enabling the spatial mapping of over 5000 cerebral proteins across whole tissue slice with excellent quantitative quality. Another approach of splitting tissue into microwells is nanodroplet processing in one pot for trace sample (NanoPOTs) (Fig. 3d). The microwells have a volume of 200 nl and is fabricated on a glass substrate with an array of 27 nanowells, each with a 1.2 mm diameter. The nanowell are used for one-pot sample processing platform by filling 200 nl of DMSO droplets and using laser capture microdissection (LCM) to dissect the tissue and immobilizing within the DMSO nanodroplets. Digested proteins are then analysed84,85 by LC-MS/MS, mapping over 2000 proteins with 100 μm spatial resolution. Hence, compartmentalization of tissues into distinct microwells can be used to perform high content spatial proteomics that is otherwise challenging for the microfluidic transcriptomic spots-based approach discussed earlier.
Discussion
Traditionally, cell morphology has been a crucial read-out in clinical pathology for the manual annotation of histological tissue samples. With the advent of spatial omics, detailed molecular information can now be extracted from individual cells while preserving their spatial context, providing a comprehensive view of tissue architecture and cellular heterogeneity. Cell morphology analysis from high-resolution imaging is semi-qualitative, measuring various cellular phenotypic features such as cell or nucleus size, shape, circularity, granularity, and the nucleus-to-cytoplasm ratio. In contrast, spatial omics yields semi-quantitative information on the presence of specific molecular targets as pixels or spots. Despite their differences, both approaches generate high-dimensional data that can be leveraged through machine learning (ML) and artificial intelligence (AI) frameworks to gain comprehensive insights into the tissue under investigation. These frameworks can translate H&E (hematoxylin and eosin) or DAPI-stained tissue morphology images into features that correlate with spatial omics data. Additionally, they facilitate the integration of spatial omics and morphology information, enriching the overall sample description.86–90
Although spatial omics offers several advantages, such as preserving spatial information and avoiding tissue dissociation during sample preparation, existing approaches lack single-cell resolution and suffer from poor transcript capture. Multiple studies have demonstrated the benefits of combining spatial omics analysis with single-cell RNA sequencing (scRNA-seq) to gain deeper insights into the transcriptomic profiles of individual cells while elucidating spatial relationships within tissues.91–93 Recent reports also highlight the impact of cellular genetic variation on morphology, underscoring the correlation between morphological data and transcriptomic analysis and its role in advancing functional genomics.94 This suggests the potential to progress from basic morphological analysis to high-content single-cell morpholomics, combined with spatial multi-omics, for deeper insights into tissue organization and cellular heterogeneity. Pairing morpholomics with spatially resolved omics can provide detailed tissue architecture at single-cell resolution. This symbiotic relationship allows morpholomic data to identify features indicating genetic variations and predict spatial gene expression, while spatial omics provides context to the morpholomic data, broadening its scope for processing tissue samples.
Notably, significant advancements in machine learning (ML) and artificial intelligence (AI) contribute to both morpholomics and spatial omics. These technologies enable the integration of different data sources and the evaluation of high-dimensional data, including morphological feature extraction and pattern recognition from spatial omics data. They also offer cost reduction, faster turnaround times, and robustness for pivoting applications through task-specific feature learning.95
Another technology that offers such robustness is microfluidics, where mature microfabrication methodologies and cost-effectiveness have enabled its extensive use in biomedical applications. An undervalued potential of microfluidics is its integrability with automation, which can bridge the skills gap in operating sophisticated instruments. Additionally, microfluidics platforms are characterized by reduced sample requirements, sterile operations, and established know-how to manipulate sample flow. In morpholomics, these advantages translate into creation of suitable cartridges for precise cell positioning for high-resolution images and integrated accessories for sorting cells of interest. For spatial omics, microfluidics enables customization, accessibility, and the versatility to integrate multi-omics modalities with minor modifications to workflow. However, significant challenges remain that hinder its widespread adoption compared to traditional or contemporary technologies.
Single-cell morphology investigations using FACS achieve throughputs exceeding 10
000 events per s, contrasting sharply with commercial microfluidic-based platforms that can process fewer than 3000 events per s. This prolongs sample processing time, especially critical in cancer liquid biopsies where the principle of ‘no cell left behind’ is essential to ensure comprehensive investigation, and probing millions of cells extends the time for sample processing, and adversely impacts cell viability. Limited throughput can be attributed to the operational speed of microfluidic components such as the sample handling units and the cell sorters. Furthermore, existing microfluidic technologies for single-cell analysis do not support multiplexing; they can process one sample at a time, unlike FACS systems that support a 96-well plate operation. To effectively translate microfluidic platforms into practical applications, significant upgrades are necessary to improve throughput and enable multiplexed operations. There have been dedicated efforts to improve the optical set-up to facilitate high-speed single-cell investigation.65,66 Operational improvements to the cell sorter speed from tens of milliseconds to less than 1 ms will significantly increase the throughput. Additionally, advancements in photoelectronic chips have been shown to reduce image acquisition times to nanoseconds – three orders faster than the state-of-the-art high-speed camera.96
Conversely, existing microfluidic technologies for tissue analysis lack resolution due to the microchannel dimensions exceeding 10 μm as opposed to alternative technologies that can achieve resolutions down to 300 nm. Recent work by You et al. showcases a systematic comparison of different spatial transcriptomics methods and highlights the poor resolution of the DBIT-seq method as compared to the other technologies.97 Noteworthy, a significant limitation in the observation made by You et al. is the comparison of commercial platforms against a ‘recreated’ DBIT-seq assay. The data collected from the DBIT-seq assay in this study has a pixel size of 20 μm as compared to the best-case scenario of 10 μm spot size as reported previously by Liu et al.77 Hence an under-optimized setup is used, which does not accurately represent the DBIT-seq assay. However, to improve shortcomings associated with the pattern dimensions, newer approaches such as nanofluidics need to be explored to create sub-micrometer patterns while retaining the advantages offered by the microfluidic-based spatial omics platforms. Microfluidics offers a unique value proposition through its capacity to integrate diverse techniques, resulting in platforms that provide a comprehensive overview of tissue and its cellular constituents. By combining single-cell analysis with spatial omics in an integrated microfluidic setup, this approach generates sub-cellular information that effectively addresses the resolution challenges associated with DBIT-seq assays. Furthermore, it enhances throughput with tissue size, thereby overcoming a significant limitation found in current morpholomic analyses. An overview of the different microfluidics based platforms for morpholomic and spatial omic research can be found in Table 1.
Table 1 Microfluidic-based approaches for morpholomics and spatial omics applications
Technology |
Microfluidic approach |
Optical setup |
Detection method |
Metrics |
Spatial resolution |
Throughput |
Cell sorting |
Ref. |
Morpholomics |
Deepcell |
Inertial lift forces and dean drag forces |
Widefield |
CMOS |
AI based multiparametric analysis |
8 μm to 20 μm |
1000 cells per s |
Yes |
60, 61 |
CYBO |
Piezoelectric transducer |
Raman |
Si photodetector for stimulated Raman scattering detection |
Label-free detection of biomolecules: lipids, proteins |
<2 μm |
∼140 cells per s |
Yes |
62, 63 |
Multi-ATOM & FACED |
Inertial focusing |
Quantitative phase imaging |
CMOS |
Morphology: volume, roundness, dry mass |
∼1 μm |
>10 000 cells per s |
No |
65, 66, 68 |
Ghost cytometry |
Sheath focusing and piezoelectric transduction |
Laser across a static and randomly structured light illumination |
Single-pixel photodetector |
1-D signal: purity, root mean square deviation |
Single cells |
>10 000 cells per s |
Yes |
64 |
Imaging flow cytometry |
3D inertial focusing in a parallelized microchannel |
Laser based stroboscopic illumination |
CMOS |
Nucleus area, dark-field intensity, volumetric cell imaging |
400 nm to 600 nm |
5000 cells to 50 000 cells |
No |
69, 70 |
Spatial omics |
DBiT seq |
Crossflow microchannels to pattern barcodes on tissue |
Fluorescence detection |
Sequencing or immunofluorescence |
Transcriptome |
10–50 μm |
1 tissue slice |
NA |
77 |
Spatial CITE-seq |
Transcriptome and proteome |
79 |
Spatial-ATAC-seq |
Epigenome |
80 |
Spatial Cut&Tag-seq |
Epigenome and transcriptome |
81 |
Matrix-seq |
Crossflow microchannels for pre-patterning spots on slides |
Transcriptome |
50 μm |
82 |
xDBIT-seq |
Crossflow microchannels to pattern barcodes on tissue |
Transcriptome |
9 tissue slices |
39 |
MAGIC-seq |
Transcriptome |
83 |
MASP |
Microwells with tissue sectioning scaffolds |
|
Mass-spectrometry (LC-MS) |
Proteome |
400 μm |
1 tissue slice |
38 |
NanoPOTs |
Microwells with tissue sectioning using laser |
Proteome |
100 μm |
84, 85 |
The future of microfluidics as a complementary technology in sample preparation and handling is highly promising, particularly as researchers increasingly prioritize single-cell analysis and subcellular resolution. Supported by foundational research, microfluidic platforms are becoming more commercialized and are integrating multiple modules for a variety of biomedical applications. One notable advantage of microfluidics is its cost-effective customization; however, this has led to a lack of standardization in materials and fabrication technologies. For instance, the choice between polydimethylsiloxane (PDMS) and alternatives such as polymethyl methacrylate (PMMA) or polycarbonate presents challenges. While PDMS is favored for academic and low-throughput investigations due to its favorable properties, it is unsuitable for mass production, making it a more expensive option compared to PMMA or polycarbonate.98 Additionally, research findings derived from PDMS-based microfluidic devices may not be easily reproducible using alternative materials, complicating the validation of results. As the accessibility of microfluidic platforms continues to grow, these challenges are likely to intensify. Establishing suitable standards will be essential to ensure consistency and quality across microfluidic platforms used in biomedical applications. Ultimately, the future of biomedical research is closely linked to advancements in microfluidics, which holds significant potential to redefine our understanding of cellular dynamics and tissue architecture.
Data availability
No primary research results, software, or code have been included and no new data were generated or analysed as part of this review.
Conflicts of interest
There are no conflicts to declare.
Acknowledgements
The authors would like to thank financial support by the Startup Grant (Grant no. A-8001301-00-00) and the Institute for Health Innovation and Technology Grant (Grant no. A-0001415-06-00) from the National University of Singapore (NUS).
References
- V. F. Annese and C. Hu, Micromachines, 2022, 13, 1923 CrossRef PubMed.
- I. Rodríguez-Ruiz, T. N. Ackermann, X. Muñoz-Berbel and A. Llobera, Anal. Chem., 2016, 88, 6630–6637 CrossRef PubMed.
- S. Khizar, H. B. Halima, N. M. Ahmad, N. Zine, A. Errachid and A. Elaissari, Electrophoresis, 2020, 41, 1206–1224 CrossRef CAS PubMed.
- C. Wang, Y. Cai, A. MacLachlan and P. Chen, IEEE Nanotechnol. Mag., 2020, 14, 46-C3 Search PubMed.
- Y. Xie, X. Xu, J. Wang, J. Lin, Y. Ren and A. Wu, Lab Chip, 2023, 23, 2922–2941 RSC.
- N. V. Menon, S. B. Lim and C. T. Lim, Curr. Opin. Pharmacol., 2019, 48, 155–161 CrossRef PubMed.
- T. Lehnert and M. A. M. Gijs, Lab Chip, 2024, 24, 1441–1493 RSC.
- J. Lee, N. Menon and C. T. Lim, Adv. Sci., 2024, 11, 2302113 CrossRef CAS PubMed.
- F. C. Jammes and S. J. Maerkl, Microsyst. Nanoeng., 2020, 6, 45 CrossRef CAS PubMed.
- X. An, P. Zuo and B.-C. Ye, Talanta, 2020, 209, 120571 CrossRef CAS PubMed.
- P. Aryal, C. Hefner, B. Martinez and C. S. Henry, Lab Chip, 2024, 24, 1175–1206 RSC.
- Y. Luo, Y. Huang, Y. Li, X. Duan, Y. Jiang, C. Wang, J. Fang, L. Xi, N.-T. Nguyen and C. Song, Lab Chip, 2023, 23, 2766–2777 RSC.
- Y. Liu, L. Sun, H. Zhang, L. Shang and Y. Zhao, Chem. Rev., 2021, 121, 7468–7529 CrossRef CAS PubMed.
- N. Yanagisawa, E. Kozgunova, G. Grossmann, A. Geitmann and T. Higashiyama, Plant Cell Physiol., 2021, 62, 1239–1250 CrossRef CAS PubMed.
- E. R. Mardis, Trends Genet., 2008, 24, 133–141 CrossRef CAS PubMed.
- S. Ma, T. W. Murphy and C. Lu, Biomicrofluidics, 2017, 11(2), 021501 CrossRef PubMed.
- H. Cong, X. Xu, B. Yu, H. Yuan, Q. Peng and C. Tian, J. Micromech. Microeng., 2015, 25, 053001 CrossRef.
- Y. Kuo and H. H. Lee, Electrochem. Solid-State Lett., 2001, 4, H23 CrossRef CAS.
- L. L. Tan, N. Loganathan, S. Agarwalla, C. Yang, W. Yuan, J. Zeng, R. Wu, W. Wang and S. Duraiswamy, Crit. Rev. Biotechnol., 2023, 43, 433–464 CrossRef PubMed.
- C. J. Cremin, S. Dash and X. Huang, Curr. Res. Biotechnol., 2022, 4, 138–151 CrossRef CAS.
- J. S. Kuo and D. T. Chiu, Lab Chip, 2011, 11, 2656–2665 RSC.
- J. C. McDonald, D. C. Duffy, J. R. Anderson, D. T. Chiu, H. Wu, O. J. A. Schueller and G. M. Whitesides, Electrophoresis, 2000, 21, 27–40 CrossRef CAS PubMed.
- A. W. Martinez, S. T. Phillips, M. J. Butte and G. M. Whitesides, Angew. Chem., Int. Ed., 2007, 46, 1318–1320 CrossRef CAS PubMed.
- N. W. Choi, M. Cabodi, B. Held, J. P. Gleghorn, L. J. Bonassar and A. D. Stroock, Nat. Mater., 2007, 6, 908–915 CrossRef CAS PubMed.
- J. Durrer, P. Agrawal, A. Ozgul, S. C. F. Neuhauss, N. Nama and D. Ahmed, Nat. Commun., 2022, 13, 6370 CrossRef CAS PubMed.
- T. N. Chen, A. Gupta, M. D. Zalavadia and A. Streets, Lab Chip, 2020, 20, 3899–3913 RSC.
- A. K. Shalek, R. Satija, J. Shuga, J. J. Trombetta, D. Gennert, D. Lu, P. Chen, R. S. Gertner, J. T. Gaublomme, N. Yosef, S. Schwartz, B. Fowler, S. Weaver, J. Wang, X. Wang, R. Ding, R. Raychowdhury, N. Friedman, N. Hacohen, H. Park, A. P. May and A. Regev, Nature, 2014, 510, 363–369 CrossRef CAS PubMed.
- J. C. Love, J. L. Ronan, G. M. Grotenbreg, A. G. van der Veen and H. L. Ploegh, Nat. Biotechnol., 2006, 24, 703–707 CrossRef CAS PubMed.
- V. Narayanamurthy, S. Nagarajan, A. A. Y. F. Khan, F. Samsuri and T. M. Sridhar, Anal. Methods, 2017, 9, 3751–3772 RSC.
- L. Mazutis, J. Gilbert, W. L. Ung, D. A. Weitz, A. D. Griffiths and J. A. Heyman, Nat. Protoc., 2013, 8, 870–891 CrossRef CAS PubMed.
- Z. Jiang, H. Shi, X. Tang and J. Qin, TrAC, Trends Anal. Chem., 2023, 159, 116932 CrossRef CAS.
- S. Lin, D. Feng, X. Han, L. Li, Y. Lin and H. Gao, Anal. Chim. Acta, 2024, 1294, 342217 CrossRef CAS PubMed.
- M. A. Faridi, H. Ramachandraiah, I. Banerjee, S. Ardabili, S. Zelenin and A. Russom, J. Nanobiotechnol., 2017, 15, 3 CrossRef PubMed.
- J.-S. Park, S.-H. Song and H.-I. Jung, Lab Chip, 2009, 9, 939–948 RSC.
- D. J. Collins, A. Neild and Y. Ai, Lab Chip, 2016, 16, 471–479 RSC.
- A. E. Reece, K. Kaastrup, H. D. Sikes and J. Oakey, RSC Adv., 2015, 5, 53857–53864 RSC.
- A. Isozaki, Y. Nakagawa, M. H. Loo, Y. Shibata, N. Tanaka, D. L. Setyaningrum, J.-W. Park, Y. Shirasaki, H. Mikami, D. Huang, H. Tsoi, C. T. Riche, T. Ota, H. Miwa, Y. Kanda, T. Ito, K. Yamada, O. Iwata, K. Suzuki, S. Ohnuki, Y. Ohya, Y. Kato, T. Hasunuma, S. Matsusaka, M. Yamagishi, M. Yazawa, S. Uemura, K. Nagasawa, H. Watarai, D. Di Carlo and K. Goda, Sci. Adv., 2020, 6, eaba6712 CrossRef CAS PubMed.
- M. Ma, S. Huo, M. Zhang, S. Qian, X. Zhu, J. Pu, S. Rasam, C. Xue, S. Shen, B. An, J. Wang and J. Qu, Nat. Commun., 2022, 13, 7736 CrossRef CAS PubMed.
- J. Wirth, N. Huber, K. Yin, S. Brood, S. Chang, C. P. Martinez-Jimenez and M. Meier, Nat. Commun., 2023, 14, 1523 CrossRef CAS PubMed.
- N. Nitta, T. Sugimura, A. Isozaki, H. Mikami, K. Hiraki, S. Sakuma, T. Iino, F. Arai, T. Endo, Y. Fujiwaki, H. Fukuzawa, M. Hase, T. Hayakawa, K. Hiramatsu, Y. Hoshino, M. Inaba, T. Ito, H. Karakawa, Y. Kasai, K. Koizumi, S. Lee, C. Lei, M. Li, T. Maeno, S. Matsusaka, D. Murakami, A. Nakagawa, Y. Oguchi, M. Oikawa, T. Ota, K. Shiba, H. Shintaku, Y. Shirasaki, K. Suga, Y. Suzuki, N. Suzuki, Y. Tanaka, H. Tezuka, C. Toyokawa, Y. Yalikun, M. Yamada, M. Yamagishi, T. Yamano, A. Yasumoto, Y. Yatomi, M. Yazawa, D. Di Carlo, Y. Hosokawa, S. Uemura, Y. Ozeki and K. Goda, Cell, 2018, 175, 266–276.e213 CrossRef CAS PubMed.
- A. Nguyen, M. Yoshida, H. Goodarzi and S. F. Tavazoie, Nat. Commun., 2016, 7, 11246 CrossRef CAS PubMed.
- P.-H. Wu, D. M. Gilkes, J. M. Phillip, A. Narkar, T. W.-T. Cheng, J. Marchand, M.-H. Lee, R. Li and D. Wirtz, Sci. Adv, 2020, 6, eaaw6938 CrossRef CAS PubMed.
- T. C. von Erlach, S. Bertazzo, M. A. Wozniak, C.-M. Horejs, S. A. Maynard, S. Attwood, B. K. Robinson, H. Autefage, C. Kallepitis, A. del Río Hernández, C. S. Chen, S. Goldoni and M. M. Stevens, Nat. Mater., 2018, 17, 237–242 CrossRef CAS PubMed.
- J. Lobo, E. Y.-S. See, M. Biggs and A. Pandit, J. Tissue Eng. Regener. Med., 2016, 10, 539–553 CrossRef CAS PubMed.
- M. Doron, T. Moutakanni, Z. S. Chen, N. Moshkov, M. Caron, H. Touvron, P. Bojanowski, W. M. Pernice and J. C. Caicedo, bioRxiv, 2023, preprint, DOI:10.1101/2023.06.16.545359.
- J. C. Caicedo, S. Cooper, F. Heigwer, S. Warchal, P. Qiu, C. Molnar, A. S. Vasilevich, J. D. Barry, H. S. Bansal, O. Kraus, M. Wawer, L. Paavolainen, M. D. Herrmann, M. Rohban, J. Hung, H. Hennig, J. Concannon, I. Smith, P. A. Clemons, S. Singh, P. Rees, P. Horvath, R. G. Linington and A. E. Carpenter, Nat. Methods, 2017, 14, 849–863 CrossRef CAS PubMed.
- S. N. Chandrasekaran, H. Ceulemans, J. D. Boyd and A. E. Carpenter, Nat. Rev. Drug Discovery, 2021, 20, 145–159 CrossRef CAS PubMed.
- J. Du, Y.-C. Yang, Z.-J. An, M.-H. Zhang, X.-H. Fu, Z.-F. Huang, Y. Yuan and J. Hou, J. Transl. Med., 2023, 21, 330 CrossRef PubMed.
- H. R. Ali, H. W. Jackson, V. R. T. Zanotelli, E. Danenberg, J. R. Fischer, H. Bardwell, E. Provenzano, H. R. Ali, M. Al Sa'd, S. Alon, S. Aparicio, G. Battistoni, S. Balasubramanian, R. Becker, B. Bodenmiller, E. S. Boyden, D. Bressan, A. Bruna, B. Marcel, C. Caldas, M. Callari, I. G. Cannell, H. Casbolt, N. Chornay, Y. Cui, A. Dariush, K. Dinh, A. Emenari, Y. Eyal-Lubling, J. Fan, E. Fisher, E. A. González-Solares, C. González-Fernández, D. Goodwin, W. Greenwood, F. Grimaldi, G. J. Hannon, O. Harris, S. Harris, C. Jauset, J. A. Joyce, E. D. Karagiannis, T. Kovačević, L. Kuett, R. Kunes, A. K. Yoldaş, D. Lai, E. Laks, H. Lee, M. Lee, G. Lerda, Y. Li, A. McPherson, N. Millar, C. M. Mulvey, F. Nugent, C. H. O'Flanagan, M. Paez-Ribes, I. Pearsall, F. Qosaj, A. J. Roth, O. M. Rueda, T. Ruiz, K. Sawicka, L. A. Sepúlveda, S. P. Shah, A. Shea, A. Sinha, A. Smith, S. Tavaré, S. Tietscher, I. Vázquez-García, S. L. Vogl, N. A. Walton, A. T. Wassie, S. S. Watson, S. A. Wild, E. Williams, J. Windhager, C. Xia, P. Zheng, X. Zhuang, O. M. Rueda, S.-F. Chin, S. Aparicio, C. Caldas, B. Bodenmiller and C. I. G. C. Team, Nat. Cancer, 2020, 1, 163–175 CrossRef CAS PubMed.
- Y. Chen, S. Yang, K. Yu, J. Zhang, M. Wu, Y. Zheng, Y. Zhu, J. Dai, C. Wang, X. Zhu, Y. Dai, Y. Sun, T. Wu and S. Wang, Ageing Res. Rev., 2024, 93, 102158 CrossRef CAS PubMed.
- W.-T. Chen, A. Lu, K. Craessaerts, B. Pavie, C. S. Frigerio, N. Corthout, X. Qian, J. Laláková, M. Kühnemund, I. Voytyuk, L. Wolfs, R. Mancuso, E. Salta, S. Balusu, A. Snellinx, S. Munck, A. Jurek, J. F. Navarro, T. C. Saido, I. Huitinga, J. Lundeberg, M. Fiers and B. De Strooper, Cell, 2020, 182, 976–991.e919 CrossRef CAS PubMed.
- Y. Zhang, R. Y. Lee, C. W. Tan, X. Guo, W. W. Y. Yim, J. C. T. Lim, F. Y. T. Wee, W. U. Yang, M. Kharbanda, J.-Y. J. Lee, N. T. Ngo, W. Q. Leow, L.-H. Loo, T. K. H. Lim, R. M. Sobota, M. C. Lau, M. J. Davis and J. Yeong, Curr. Opin. Biotechnol., 2024, 87, 103111 CrossRef CAS PubMed.
- S. Saarenpää, O. Shalev, H. Ashkenazy, V. Carlos, D. S. Lundberg, D. Weigel and S. Giacomello, Nat. Biotechnol., 2024, 42, 1384–1393 CrossRef PubMed.
- Y. Liu and J. Xu, Curr. Pathobiol. Rep., 2019, 7, 85–96 CrossRef CAS PubMed.
- J. W. Tung, K. Heydari, R. Tirouvanziam, B. Sahaf, D. R. Parks, L. A. Herzenberg and L. A. Herzenberg, Clin. Lab. Med., 2007, 27, 453–468 CrossRef PubMed.
- D. A. Basiji, W. E. Ortyn, L. Liang, V. Venkatachalam and P. Morrissey, Clin. Lab. Med., 2007, 27, 653–670 CrossRef PubMed , viii.
- P. Rees, H. D. Summers, A. Filby, A. E. Carpenter and M. Doan, Nat. Rev. Methods Primers, 2022, 2, 86 CrossRef CAS PubMed.
- C. W. Fung, S. N. Chan and A. R. Wu, Biomicrofluidics, 2020, 14(2), 021502 CrossRef CAS PubMed.
- T. Luo, L. Fan, R. Zhu and D. Sun, Micromachines, 2019, 10, 104 CrossRef PubMed.
- M. Salek, N. Li, H.-P. Chou, K. Saini, A. Jovic, K. B. Jacobs, C. Johnson, V. Lu, E. J. Lee, C. Chang, P. Nguyen, J. Mei, K. P. Pant, A. Y. Wong-Thai, Q. F. Smith, S. Huang, R. Chow, J. Cruz, J. Walker, B. Chan, T. J. Musci, E. A. Ashley and M. Masaeli, Commun. Biol., 2023, 6, 971 CrossRef PubMed.
- M. Masaeli, M. Salek, H.-P. Chou, S. Kahkeshani, P. Khandelwal and S. T. Shafaat, Systems and methods for particle analysis, Patent application, 2023 Search PubMed.
- Y. Suzuki, K. Kobayashi, Y. Wakisaka, D. Deng, S. Tanaka, C.-J. Huang, C. Lei, C.-W. Sun, H. Liu, Y. Fujiwaki, S. Lee, A. Isozaki, Y. Kasai, T. Hayakawa, S. Sakuma, F. Arai, K. Koizumi, H. Tezuka, M. Inaba, K. Hiraki, T. Ito, M. Hase, S. Matsusaka, K. Shiba, K. Suga, M. Nishikawa, M. Jona, Y. Yatomi, Y. Yalikun, Y. Tanaka, T. Sugimura, N. Nitta, K. Goda and Y. Ozeki, Proc. Natl. Acad. Sci. U. S. A., 2019, 116, 15842–15848 CrossRef CAS PubMed.
- N. Nitta, T. Iino, A. Isozaki, M. Yamagishi, Y. Kitahama, S. Sakuma, Y. Suzuki, H. Tezuka, M. Oikawa, F. Arai, T. Asai, D. Deng, H. Fukuzawa, M. Hase, T. Hasunuma, T. Hayakawa, K. Hiraki, K. Hiramatsu, Y. Hoshino, M. Inaba, Y. Inoue, T. Ito, M. Kajikawa, H. Karakawa, Y. Kasai, Y. Kato, H. Kobayashi, C. Lei, S. Matsusaka, H. Mikami, A. Nakagawa, K. Numata, T. Ota, T. Sekiya, K. Shiba, Y. Shirasaki, N. Suzuki, S. Tanaka, S. Ueno, H. Watarai, T. Yamano, M. Yazawa, Y. Yonamine, D. Di Carlo, Y. Hosokawa, S. Uemura, T. Sugimura, Y. Ozeki and K. Goda, Nat. Commun., 2020, 11, 3452 CrossRef CAS PubMed.
- S. Ota, R. Horisaki, Y. Kawamura, M. Ugawa, I. Sato, K. Hashimoto, R. Kamesawa, K. Setoyama, S. Yamaguchi, K. Fujiu, K. Waki and H. Noji, Science, 2018, 360, 1246–1251 CrossRef CAS PubMed.
- J.-L. Wu, Y.-Q. Xu, J.-J. Xu, X.-M. Wei, A. C. S. Chan, A. H. L. Tang, A. K. S. Lau, B. M. F. Chung, H. Cheung Shum, E. Y. Lam, K. K. Y. Wong and K. K. Tsia, Light:Sci. Appl., 2017, 6, e16196 CrossRef CAS PubMed.
- K. C. M. Lee, A. K. S. Lau, A. H. L. Tang, M. Wang, A. T. Y. Mok, B. M. F. Chung, W. Yan, H. C. Shum, K. S. E. Cheah, G. C. F. Chan, H. K. H. So, K. K. Y. Wong and K. K. Tsia, J. Biophotonics, 2019, 12, e201800479 CrossRef PubMed.
- X. Hua, K. Han, B. Mandracchia, A. Radmand, W. Liu, H. Kim, Z. Yuan, S. M. Ehrlich, K. Li, C. Zheng, J. Son, A. D. S. Trenkle, G. A. Kwong, C. Zhu, J. E. Dahlman and S. Jia, Nat. Commun., 2024, 15, 1975 CrossRef CAS PubMed.
- Z. Zhang, K. C. M. Lee, D. M. D. Siu, M. C. K. Lo, Q. T. K. Lai, E. Y. Lam and K. K. Tsia, Commun. Biol., 2023, 6, 449 CrossRef CAS PubMed.
- G. Holzner, B. Mateescu, D. van Leeuwen, G. Cereghetti, R. Dechant, S. Stavrakis and A. deMello, Cell Rep., 2021, 34(10), 1–12 Search PubMed.
- A. S. Rane, J. Rutkauskaite, A. deMello and S. Stavrakis, Chem, 2017, 3, 588–602 CAS.
- N. C. Bauer, P. W. Doetsch and A. H. Corbett, Traffic, 2015, 16, 1039–1061 CrossRef CAS PubMed.
- S. Pankow, S. Martinez-Bartolome, C. Bamberger and J. R. Yates, Curr. Opin. Chem. Biol., 2019, 48, 19–25 CrossRef CAS PubMed.
- M. C. Hung and W. Link, J. Cell Sci., 2011, 124, 3381–3392 CrossRef CAS PubMed.
- D. Bressan, G. Battistoni and G. J. Hannon, Science, 2023, 381, eabq4964 CrossRef CAS PubMed.
- E. Lundberg and G. H. H. Borner, Nat. Rev. Mol. Cell Biol., 2019, 20, 285–302 CrossRef CAS PubMed.
- C. Giesen, H. A. Wang, D. Schapiro, N. Zivanovic, A. Jacobs, B. Hattendorf, P. J. Schuffler, D. Grolimund, J. M. Buhmann, S. Brandt, Z. Varga, P. J. Wild, D. Gunther and B. Bodenmiller, Nat. Methods, 2014, 11, 417–422 CrossRef CAS PubMed.
- Y. Liu, M. Yang, Y. Deng, G. Su, A. Enninful, C. C. Guo, T. Tebaldi, D. Zhang, D. Kim, Z. Bai, E. Norris, A. Pan, J. Li, Y. Xiao, S. Halene and R. Fan, Cell, 2020, 183, 1665–1681 CrossRef CAS PubMed.
- M. Vicari, R. Mirzazadeh, A. Nilsson, R. Shariatgorji, P. Bjärterot, L. Larsson, H. Lee, M. Nilsson, J. Foyer, M. Ekvall, P. Czarnewski, X. Zhang, P. Svenningsson, L. Käll, P. E. Andrén and J. Lundeberg, Nat. Biotechnol., 2024, 42, 1046–1050 CrossRef CAS PubMed.
- Y. Liu, M. DiStasio, G. Su, H. Asashima, A. Enninful, X. Qin, Y. Deng, J. Nam, F. Gao, P. Bordignon, M. Cassano, M. Tomayko, M. Xu, S. Halene, J. E. Craft, D. Hafler and R. Fan, Nat. Biotechnol., 2023, 41, 1405–1409 CrossRef CAS PubMed.
- Y. Deng, M. Bartosovic, S. Ma, D. Zhang, P. Kukanja, Y. Xiao, G. Su, Y. Liu, X. Qin, G. B. Rosoklija, A. J. Dwork, J. J. Mann, M. L. Xu, S. Halene, J. E. Craft, K. W. Leong, M. Boldrini, G. Castelo-Branco and R. Fan, Nature, 2022, 609, 375–383 CrossRef CAS PubMed.
- Y. Deng, M. Bartosovic, P. Kukanja, D. Zhang, Y. Liu, G. Su, A. Enninful, Z. Bai, G. Castelo-Branco and R. Fan, Science, 2022, 375, 681–686 CrossRef CAS PubMed.
- H. Zhao, G. Tian and A. Hu, bioRxiv, 2022, preprint DOI:10.1101/2022.08.05.502952.
- J. Zhu, K. Pang, B. Hu, R. He, N. Wang, Z. Jiang, P. Ji and F. Zhao, Nat. Genet., 2024, 56, 2259–2270 CrossRef CAS PubMed.
- Y. Zhu, P. D. Piehowski, R. Zhao, J. Chen, Y. Shen, R. J. Moore, A. K. Shukla, V. A. Petyuk, M. Campbell-Thompson, C. E. Mathews, R. D. Smith, W.-J. Qian and R. T. Kelly, Nat. Commun., 2018, 9, 882 CrossRef PubMed.
- P. D. Piehowski, Y. Zhu, L. M. Bramer, K. G. Stratton, R. Zhao, D. J. Orton, R. J. Moore, J. Yuan, H. D. Mitchell, Y. Gao, B. M. Webb-Robertson, S. K. Dey, R. T. Kelly and K. E. Burnum-Johnson, Nat. Commun., 2020, 11, 8 CrossRef CAS PubMed.
- B. He, L. Bergenstråhle, L. Stenbeck, A. Abid, A. Andersson, Å. Borg, J. Maaskola, J. Lundeberg and J. Zou, Nat. Biomed. Eng., 2020, 4, 827–834 CrossRef CAS PubMed.
- T. Monjo, M. Koido, S. Nagasawa, Y. Suzuki and Y. Kamatani, Sci. Rep., 2022, 12, 4133 CrossRef CAS PubMed.
- D. Zhang, A. Schroeder, H. Yan, H. Yang, J. Hu, M. Y. Y. Lee, K. S. Cho, K. Susztak, G. X. Xu, M. D. Feldman, E. B. Lee, E. E. Furth, L. Wang and M. Li, Nat. Biotechnol., 2024, 42, 1372–1377 CrossRef CAS PubMed.
- J. Hu, K. Coleman, D. Zhang, E. B. Lee, H. Kadara, L. Wang and M. Li, Cell Syst., 2023, 14, 404–417.e404 CrossRef CAS PubMed.
- B. Li, F. Bao, Y. Hou, F. Li, H. Li, Y. Deng and Q. Dai, Nat. Commun., 2024, 15, 6541 CrossRef CAS PubMed.
- G. S. Gulati, J. P. D'Silva, Y. Liu, L. Wang and A. M. Newman, Nat. Rev. Mol. Cell Biol., 2025, 26, 11–31 CrossRef CAS PubMed.
- X. Wan, J. Xiao, S. S. T. Tam, M. Cai, R. Sugimura, Y. Wang, X. Wan, Z. Lin, A. R. Wu and C. Yang, Nat. Commun., 2023, 14, 7848 CrossRef CAS PubMed.
- X. Fei, J. Liu, J. Xu, H. Jing, Z. Cai, J. Yan, Z. Wu, H. Li, Z. Wang and Y. Shen, J. Transl. Med., 2024, 22, 380 CrossRef CAS PubMed.
- M. Tegtmeyer, J. Arora, S. Asgari, B. A. Cimini, A. Nadig, E. Peirent, D. Liyanage, G. P. Way, E. Weisbart, A. Nathan, T. Amariuta, K. Eggan, M. Haghighi, S. A. McCarroll, L. O'Connor, A. E. Carpenter, S. Singh, R. Nehme and S. Raychaudhuri, Nat. Commun., 2024, 15, 347 CrossRef CAS PubMed.
- E. Chelebian, C. Avenel and C. Wahlby, arXiv, 2024, preprint, arXiv:2407.20660, DOI:10.48550/arXiv.2407.20660.
- Y. Chen, M. Nazhamaiti, H. Xu, Y. Meng, T. Zhou, G. Li, J. Fan, Q. Wei, J. Wu, F. Qiao, L. Fang and Q. Dai, Nature, 2023, 623, 48–57 CrossRef CAS PubMed.
- Y. You, Y. Fu, L. Li, Z. Zhang, S. Jia, S. Lu, W. Ren, Y. Liu, Y. Xu, X. Liu, F. Jiang, G. Peng, A. Sampath Kumar, M. E. Ritchie, X. Liu and L. Tian, Nat. Methods, 2024, 21, 1743–1754 CrossRef CAS PubMed.
- L. R. Volpatti and A. K. Yetisen, Trends Biotechnol., 2014, 32, 347–350 CrossRef CAS PubMed.
Footnote |
† All authors have contributed equally. |
|
This journal is © The Royal Society of Chemistry 2025 |
Click here to see how this site uses Cookies. View our privacy policy here.