Analysis of interactions between amino acids and monolayers of charged side chains†
Received
3rd September 2024
, Accepted 15th November 2024
First published on 18th November 2024
Abstract
Protein–protein interactions (PPIs) are regulated by multiple interactions among amino acids. However, the contribution of individual amino acid–amino acid interactions (AAIs) in PPIs is currently unclear because it is difficult to analyze the weak and nonspecific interactions among amino acids. Therefore, we constructed a quantitative analytical model to evaluate AAIs using a device with self-assembled monolayers (SAMs). We could evaluate the μM-order dissociation constant between amino acids and the side chain of amino acids based on the electrical response. In the cationic amino acid group, concentration-dependent responses were observed on a negatively charged SAM (3-mercaptopropionic acid). These responses were modulated by the concentration and valence of the competing ions, which indicated that the strength of electrostatic interactions among amino acids is different. In contrast, nonspecific responses to all amino acids used in this study were obtained on a positively charged SAM (2-mercaptoethylamine). These results indicate that the selectivity of interaction depends on the type of side chain in the assembled state. We believe that the analytical platform constructed in this study can be adapted to evaluate various AAIs that govern PPIs.
Introduction
Protein–protein interactions (PPIs), known as macromolecular interactions between homologous or heterologous proteins, drive the formation of supra-organizations of proteins, such as nanometer-sized complexes and micrometer-sized assemblies.1,2 Protein assemblies formed by phase separation regulate complex biological reactions (e.g., signalling, metabolism, etc.).3 PPI analysis is also critical for industries dependent on proteins, such as those involved in the development of enzymatic biomass and drug discovery.4,5 PPIs include specific interactions through structural subunits and nonspecific interactions, such as electrostatic interactions, hydrogen bonding, and hydrophobic interactions, resulting from multiple interactions among amino acids.6,7 Therefore, for desired control of PPIs, it is critical to understand amino acid–amino acid interactions (AAIs) at the molecular level.
PPIs mediated by structural domains or small molecules have been evaluated using various analytical methods.8 For example, changes in PPIs induced by thermal and electromagnetic radiation are analyzed using in vitro methods.9,10 In in vivo methods, PPIs are examined based on changes in fluorescence intensity of labeling probes and transcriptional activity.11,12 In addition, computational approaches are employed to predict PPIs using in silico modeling and simulations based on sequence and structure information.13 Consequently, a tremendous amount of knowledge on macro- and mesoscopic interactions of proteins has accumulated, and several databases for such interactions have been created.14,15 However, the microscopic contributions of each AAI in governing PPIs remain unclear.7 Although computational approaches have been devised to unravel individual interactions between single amino acid molecules,16 experimental analysis of such interactions is still in its infancy. In conventional methods for analyzing molecular interactions, the determination of individual AAIs in a protein assembly is challenging,17 meaning that the analysis of weak and nonspecific interactions between individual amino acids is difficult using the above-mentioned approaches for evaluating PPIs. Hence, the development of analytical methods for weak molecular interactions of amino acids is desirable.
The use of self-assembled monolayers (SAMs) is one of the key approaches to evaluate intermolecular interactions because they provide the reaction field in which the molecules assemble.18 SAM systems can be applied to model biological responses modulated by specific host–guest interactions, such as catalytic reactions and immunoassays.19,20 Moreover, by introducing artificial receptors into SAMs, small molecules such as sugars, ions and biogenic amines can also be detected.21–23 Therefore, SAMs can detect not only specific reactions triggered by macromolecules, but also weak molecular interactions between small molecules. In particular, SAM systems combined with field-effect transistors (FETs) are on-site sensing platforms that enable high throughput and sensitivity of analyte detection due to their integration capability and electrochemical detection mechanism.23,24 In light of these findings, we propose that FETs modified by SAMs with amino acid functional groups could be considered as substructural models of proteins and used to detect weak molecular interactions between amino acids.
Mutations in charged amino acids in proteins can dramatically alter PPIs, affecting the function, solubility, and assembly state of proteins.25,26 Hence, understanding the contribution of individual AAIs between charged amino acids expands the potential applications of proteins in various industries. In this study, we constructed a quantitative analysis system using a FET-based sensor modified with different surface charges composed of two types of amino acid side-chain mimicking monolayers. We then evaluated the AAIs between charged amino acids in solution and the side chain on SAMs by measuring the electrical response. Finally, we investigated the influence of ionic strength in solution on AAIs to understand the mode of electrostatic AAIs in detail. Using SAM systems, we have successfully analyzed the differences in the strength of AAIs as a function of solution conditions and amino acid species.
Experimental details
Materials
To evaluate AAIs, we used L-lysine (Lys), L-arginine (Arg), L-histidine (His), L-glutamic acid (Glu), L-aspartic acid (Asp), and glycine (Gly) as analytes (Fig. 1a). Lys, Arg, His, Glu, Asp, calcium chloride (CaCl2), and dimethyl sulfoxide (DMSO) were purchased from Wako Pure Chemical Industries, Ltd. (Osaka, Japan). Gly, isopropyl alcohol (IPA), and sodium chloride (NaCl) were purchased from Kanto Chemical Co., Inc. (Tokyo, Japan). 3-Mercaptopropionic acid (3-MPA) and 2-mercaptoethylamine (2-MEA), used as self-assembled monolayer (SAM) monomers (Fig. 1b), were purchased from Tokyo Chemical Industry Co., Ltd. (Tokyo, Japan). 3-Morpholinopropanesulfonic acid (MOPS) was purchased from Sigma-Aldrich (St. Louis, MO, USA). The buffer solutions were prepared in Milli-Q water (18 MΩ cm resistivity at 25 °C). All the reagents utilized in this study were used as supplied.
 |
| Fig. 1 Chemical structures of (a) amino acid analytes and (b) monomers used for preparing self-assembled monolayers (SAMs) mimicking the electrically charged side chains examined in this study. The described pKa values (a1, α-carboxyl group; a2, α-amino group; a3, side chain) were referred from previous studies.27–29 (c) Schematic illustration of a field-effect transistor (FET) sensor functionalized with a SAM, mimicking the amino acid side chains. | |
Functionalization of FET-based sensors
We utilized an on-chip sensor array chip consisting of 64 × 64 extended-gate type FETs. All FET devices were equipped with a gold (Au) extended-gate electrode (BC2, BioCMOS Co., Ltd.). Because the sensing part (the extended-gate) and transducing unit (the FET channel) in the FET sensor are separated, repeatable and stable measurements of analytes in aqueous solutions are ensured by the extended-gate configuration of the FETs (Fig. 1c). Before functionalization of the FET devices, the surface of the Au extended-gate electrodes was first rinsed with IPA and Milli-Q water. The electrodes were then cleaned by vacuum plasma treatment for 1 min (introduced gas: atmospheric air). The Au electrodes were immersed in a DMSO solution containing 1 mM SAM monomers for 1 h at room temperature. We used two types of SAM monomers that mimicked the electrically charged side chains of amino acids (Fig. 1b). 3-MPA and 2-MEA were used as mimics of the negatively charged and positively charged side chains, respectively. Finally, the SAM-functionalized electrodes were rinsed with IPA and Milli-Q water.
Surface characterization of the SAM-modified electrodes
Elemental analyses of the Au surfaces modified with SAM-functionalized Au electrodes were performed using X-ray photoelectron spectroscopy (XPS). The changes in the work function of the Au electrodes with and without each SAM were evaluated using photoemission yield spectroscopy in air (PYSA). Quartz crystal microbalance (QCM) measurements were performed to evaluate the molecular density of the SAMs on the Au surfaces. The adsorption of SAMs was evaluated based on the change in frequency when solutions of 0 or 1 mM amino acid derivatives in DMSO were passed. The base frequency for the QCM measurements was defined to be 8.9 MHz.
Electrical detection of AAIs using the SAM-modified electrodes
After functionalization of the extended-gate sensing electrode, the FET sensor chip was connected to the measuring equipment (BCT-II, BioCMOS Co., Ltd.). An Ag/AgCl reference electrode with an inner solution of 3 M NaCl (RE-3VT, BAS Inc.) was also coupled with the measurement equipment as a control-gate electrode (Fig. 1c). First, a MOPS buffer solution (10 mM, pH 7.0) containing 1 or 10 mM electrolyte (NaCl or CaCl2) was applied to the FET sensor chip. The change in the output voltage with time because of the fluctuation in electrode potential in the buffer solution was measured.30 The drift of the output voltage was almost stabilized after 2 h. Hence, the device was incubated with the buffer solution for 2 h. The drift fraction was formulated to analyze the output voltage as a sensing signal (Fig. S1a†). Then, the change in output voltage (VOUT) was measured while titrating the amino acid solution containing 0–10 μM amino acid and 1 or 10 mM electrolyte in 10 mM MOPS (pH 7.0) (Fig. S1b†). Calibrated VOUT was determined by subtracting the drift fraction from the measured VOUT (Fig. S1c†). All the electrical measurements were performed at room temperature. The VOUT was determined from 42 independent detection points on SAMs, and the mean and standard error of 42 data were calculated.
Results
Chemical composition of the amino acid-mimicking SAMs
To confirm the immobilization of the amino acid-mimicking SAMs on the Au surface, we first performed an elemental analysis of the SAMs using XPS (Fig. 2). Compositional changes in the SAM systems on the Au surfaces were observed as shifts in each elemental peak of the amino acid derivatives in the C 1s, O 1s, N 1s, and S 2p regions. The C 1s peak at approximately 285 eV for each SAM could be imputed to the carbon attached to the sulfur atom. In addition, the C 1s peak at approximately 288 eV and the O 1s peak at approximately 533 eV in 3-MPA modified SAM could be imputed to the carbon and oxygen in the carboxyl group (Fig. 2a). The N 1s peak at approximately 400 eV in the 2-MEA modified SAM could be imputed to the nitrogen in the amino group (Fig. 2b). The peaks derived from the orbital doublets of S 2p3/2 (∼162 eV) and S 2p1/2 (∼163 eV) could be imputed to the sulfur in the thiol group. Notably, the changes in the peak ratios between S 2p3/2 and S 2p1/2 of each SAM were obtained (Fig. S2a and b†), which could be assigned to the presence of small amounts of disulfide-like compounds on the Au film. Based on the peak separation analysis in the S 2p region, the presence of disulfide-like compounds was more for 3-MPA than for 2-MEA, and that of thiol-derived compounds was more for 2-MEA than for 3-MPA. These results suggest that each amino acid derivative was adsorbed onto the Au surface, and the intermolecular distance of thiol groups on SAMs was shorter for 3-MPA than for 2-MEA.
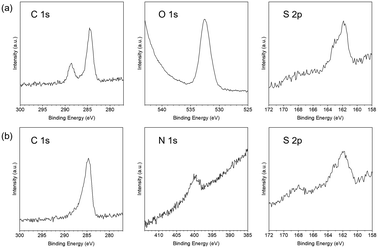 |
| Fig. 2 X-ray photoelectron spectroscopy of C 1s, O 1s, N 1s, and S 2p regions of the Au film modified with (a) 3-mercaptopropionic acid or (b) 2-mercaptoethylamine monolayers. | |
We then performed PYSA measurements to confirm the donor or acceptor properties of the Au surfaces (Fig. 3). These properties of the Au surfaces were determined from a shift in the work function. The work function of the untreated SAM was 4.83 ± 0.02 eV. After 3-MPA modification, the work function shifted to 4.92 ± 0.01 eV, suggesting the presence of electron-withdrawing substituents (–COOH). In contrast, the work function of the Au film shifted to 4.71 ± 0.01 eV after modification of the film with 2-MEA, indicating the presence of electron-donating substituents (–NH2) on the Au film. Notably, 3-MPA modification reduced the photoelectron yield from the Au surface. The dense adsorption of 3-MPA on the Au surface could inhibit the emission of photoelectrons.
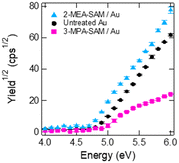 |
| Fig. 3 Result of photoemission yield spectroscopy in air of the Au electrodes. Untreated Au (black), 3-mercaptopropionic acid (3-MPA)-treated Au (pink), and 2-mercaptoethylamine (2-MEA)-treated Au (blue). Error bars represent the standard errors of three measurements. | |
Finally, we evaluated the density of the amino acid derivatives on the Au surfaces using QCM measurements (Table 1). Frequency changes (Δf) and molecular densities (ρ) were similar to those reported previously.31,32 The ρ of 3-MPA on the Au surface was approximately 2.4-times higher than that of 2-MEA. 3-MPA forms hydrogen bonds between the carboxyl groups,33 probably resulting in a denser adsorption than does 2-MEA. Based on the density and the interaction of ligand molecules, 3-MPA could stand on the gold surface due to unlikely interaction between the carboxyl group and the gold. On the other hand, 2-MEA could lie on the gold surface due to the interaction between the amino group and the gold. Collectively, the XPS, PYSA, and QCM results showed that 3-MPA and 2-MEA were certainly adsorbed on the Au surface and that the alignment patterns varied depending on the molecule (Fig. S2c and d†). Moreover, these results suggested the formation of a monolayer on the Au surface, hence the thickness of the SAMs would be about the length of a 3-MPA or 2-MEA molecule.
Table 1 Amounts of amino acid derivatives on the Au surface determined using the quartz crystal microbalance
SAM monomer |
Δf (Hz) |
Δm (ng) |
ρ (nmol cm−2) |
The weights of the SAM monomers on the Au surface were calculated from the frequency change.34 The sensing area of the Au surface was 0.196 cm2. Values represent the mean and standard error of three measurements. SAM, self-assembled monolayer; 2-MEA, 2-mercaptoethylamine; 3-MPA, 3-mercaptopropionic acid. |
3-MPA |
40.38 ± 1.21 |
44.22 ± 1.33 |
2.13 ± 0.06 |
2-MEA |
12.44 ± 0.77 |
13.62 ± 0.85 |
0.90 ± 0.06 |
Electrical detection of AAIs using amino acid-mimicking SAMs
First, we investigated the interactions between free amino acids and the negatively charged amino acid assembled on the Au electrode. Fig. 4 shows the relationship between the concentration of free amino acids and the changes in the output voltage (VOUT) of the 3-MPA-modified FET device. In titration experiments with cationic amino acids (Lys, Arg, and His), concentration-dependent responses were observed (Fig. 4a–c). Based on the titration isotherms for these analytes, the apparent dissociation constant (Kd) for each titration experiment was estimated using the Hill–Waud model (Table 2). Considering the pH of the analyte solution (pH 7.0) and the pKa values of 3-MPA, Lys, Arg, and His (Fig. 1), the electrical responses obtained in these titrations are attributed to electrostatic interactions between 3-MPA and the cationic amino acids. In contrast, the electrical responses to the anionic amino acids (Glu and Asp) were weaker than those to the cationic amino acids (Lys, Arg, and His) (Fig. 4d and e and Table 2). This is presumably due to the electrostatic repulsion between the carboxy moieties of 3-MPA and the carboxy–oxygens of Glu and Asp (cf. pKa in Fig. 1). In particular, the lower response of the device to Gly (Fig. 4f and Table 2) indicated that the positively charged amino group in the main chain of the amino acids was insufficient to interact with the 3-MPA assembled on the Au surface.
 |
| Fig. 4 Electrical detection of amino acids using the field-effect transistor sensor modified with the anionic monolayer (3-mercaptopropionic acid-self-assembled monolayer). Changes in the output voltage upon addition of Lys (a), Arg (b), His (c), Glu (d), Asp (e), or Gly (f) to the 3-morpholinopropanesulfonic acid buffer solution (10 mM) with 1 or 10 mM electrolyte (NaCl or CaCl2) at pH 7.0. [Analyte] = 0–10 μM. | |
Table 2 Apparent dissociation constants (Kd/μM) obtained using the 3-mercaptopropionic acid-modified self-assembled monolayer
Amino acid |
Electrolyte in the analyte solution |
1 mM NaCl |
10 mM NaCl |
1 mM CaCl2 |
10 mM CaCl2 |
The apparent Kd values were calculated using the fitting calculation based on the Hill–Waud model35 upon addition of each amino acid to 10 mM 3-morpholinopropanesulfonic acid buffer solution with 1 or 10 mM electrolyte (NaCl or CaCl2) at pH 7.0. ND indicates a low correlation between the concentration and voltage (R2 < 0.7). ND indicates that the error region is higher than the value. |
Lys |
0.45 ± 0.03 |
0.39 ± 0.02 |
0.49 ± 0.02 |
NDb |
Arg |
0.70 ± 0.05 |
0.33 ± 0.02 |
0.44 ± 0.05 |
NDa |
His |
0.66 ± 0.16 |
0.44 ± 0.05 |
0.34 ± 0.02 |
NDa |
Glu |
NDa |
NDb |
0.25 ± 0.05 |
NDb |
Asp |
NDa |
0.77 ± 0.16 |
NDa |
NDa |
Gly |
NDa |
0.70 ± 0.08 |
NDa |
NDb |
Next, we examined the interactions between amino acids and positively charged amino acid-mimicking molecules. Fig. 5 illustrates the relationship between the concentration of amino acids and the changes in the VOUT of the 2-MEA-modified FET device. Concentration-dependent responses were observed in the group of cationic amino acids as well as anionic amino acids (Lys, Arg, Glu, and Asp), and Kd was calculated (Fig. 5 and Table 3). The lower response of the device to Gly (Fig. 5f and Table 3) indicated that the negatively charged carboxyl groups in the main chain of the amino acids were probably insufficient to interact with the 2-MEA assembled on the Au surface. Considering the pH of the analyte solution (pH 7.0) and the pKa values of Glu, Asp, and 2-MEA (Fig. 1), the interaction between 2-MEA and the anionic amino acids is probably driven by electrostatic interactions. In contrast, based on the pKa values of Lys, Arg, and 2-MEA (Fig. 1), the interaction between 2-MEA and the cationic amino acids could be unique reactions via amino groups.36
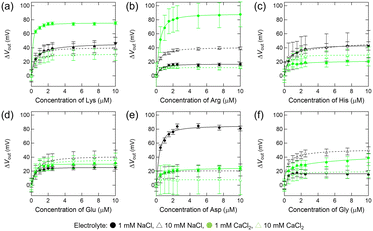 |
| Fig. 5 Electrical detection of amino acids using the field-effect transistor sensor modified with the cationic monolayer (2-mercaptoethylamine-self-assembled monolayer). Changes in the output voltage upon addition of Lys (a), Arg (b), His (c), Glu (d), Asp (e), or Gly (f) to the 3-morpholinopropanesulfonic acid buffer solution (10 mM) with 1 or 10 mM electrolyte (NaCl or CaCl2) at pH 7.0. [Analyte] = 0–10 μM. | |
Table 3 Apparent dissociation constants (Kd/μM) obtained using the 2-mercaptoethylamine-modified self-assembled monolayer
Amino acid |
Electrolyte in the analyte solution |
1 mM NaCl |
10 mM NaCl |
1 mM CaCl2 |
10 mM CaCl2 |
The apparent Kd values were calculated using the fitting calculation based on the Hill–Waud model35 upon addition of each amino acid to 10 mM 3-morpholinopropanesulfonic acid buffer solution with 1 or 10 mM electrolyte (NaCl or CaCl2) at pH 7.0. ND indicates a low correlation between the concentration and voltage (R2 < 0.7). ND indicates that the error region is higher than the value. |
Lys |
0.49 ± 0.07 |
0.49 ± 0.03 |
0.15 ± 0.04 |
0.37 ± 0.04 |
Arg |
0.44 ± 0.08 |
0.33 ± 0.03 |
0.39 ± 0.01 |
NDa |
His |
0.56 ± 0.02 |
NDb |
NDb |
0.48 ± 0.08 |
Glu |
0.26 ± 0.02 |
0.63 ± 0.03 |
0.40 ± 0.06 |
0.32 ± 0.06 |
Asp |
0.35 ± 0.06 |
0.41 ± 0.05 |
0.34 ± 0.06 |
NDa |
Gly |
NDa |
0.31 ± 0.03 |
NDb |
0.44 ± 0.05 |
Electrolyte effects on amino acid interactions
Finally, we investigated the effect of the added electrolyte on the interaction between the amino acids and SAM-modified FETs (Fig. 6). The image of charges of SAMs depending on the electrolyte concentration is shown in Fig. S3.† When the 3-MPA-SAM was used, the Kd values of Lys, Arg and His decreased with increasing ionic strength (Table 2), indicating higher affinities of these amino acids for the assembled carboxyl groups (3-MPA-SAM). These amino acids can easily form chelating complexes with metal cations,37 indicating that the obtained affinity can be attributed to the complexation of these amino acids with each other via alkali- or alkaline earth metals (Na+ or Ca2+).38,39 However, in the case of high ionic strength, such as in the presence of 10 mM CaCl2, the response could be poor owing to strong electrostatic shielding. Interestingly, the lowest Kd value was obtained for Glu in the 1 mM CaCl2 solution (Table 2). As carboxylic acids containing long alkyl groups readily form Ca2+-mediated crosslinked structures,40 the affinity between Glu and 3-MPA could be increased by Ca2+-mediated crosslinking.
 |
| Fig. 6 Heat map of the interactions depending on the ionic strength and the type of molecule based on the dissociation constant (Tables 2 and 3). White areas represent that the interactions could not be properly evaluated. | |
In the titration experiments on the 2-MEA-SAM, the Kd of Glu increased with increasing ionic strength (Table 3). This indicated that the carboxy moiety in these amino acids preferentially interacts with Na+ or Ca2+, resulting in a lower affinity between the amino-terminated group on the 2-MEA-SAM and the carboxy moiety in the amino acids. However, increasing the ionic strength and Kd of Lys, Arg, and Asp resulted in little change, indicating that the affinity of these amino acids for 2-MEA was not ion-sensitive (Table 3). Unlike the 3-MPA-modified SAM, the 2-MEA-modified SAM showed a concentration-dependent response at high ionic strength, such as in the presence of 10 mM CaCl2 (Fig. 4 and 5). The contribution of the electrostatic interactions to the electrical response was probably less for the amine-modified SAM than for the carboxylic acid-modified SAM (Fig. 6).
We finally consider not only the direct intervention of electrolytes in the AAIs, but also the indirect intervention of electrolytes in relation to the Hofmeister series.41 In general, the salting-out effect is said to be stronger for Na+ than for Ca2+, meaning that van der Waals attraction and hydrophobic interactions between AAIs are stronger in the presence of Na+ compared to Ca2+. However, the Hofmeister series can be reversed depending on the charge and hydrophobicity of the interaction surface; for example, that of cations is reversed at positively charged interfaces.42 This implies that van der Waals attraction and hydrophobic interactions are more effective in the presence of Ca2+ on the 2-MEA-SAM. Thus, Lys with a long alkyl chain would likely exhibit a lower Kd value under such conditions due to hydrophobic interactions (Table 3).
Discussion
We presented an analytical model for AAIs using electrical detection on the Au surface. Since the Kd depends on the ionic strength and the type of molecule with different pKa values (Tables 2 and 3), the electrostatic AAIs could mainly be evaluated. Because approximately 0.1 mL of 0–10 μM amino acid solution was flowed over the Au surface on which amino acid derivatives in nmol order were adsorbed, we could observe interaction among nmol-order molecules. Solubility experiments are used for evaluating AAIs, but they can only detect millimolar to molar order interactions, and the AAIs may depend on solvent properties, such as viscosity and pH.43,44 Computational simulations have been used to evaluate the AAIs in the protein structure and in solution,16,45 but the spatial and temporal scale restrictions were severe, and many approximations had to be introduced. One feature of our system is the on-site evaluation of AAIs at low molecular concentrations, where AAIs are barely affected by solvent properties, without any approximation or constraints.
In this study, two types of SAMs with different surface charges were prepared. Based on XPS, PYSA, and QCM analyses, the negatively charged 3-MPA-SAM showed a higher molecular density on the Au surface than did the positively charged 2-MEA-SAM on the same surface. This indicated that the intermolecular distance of the carboxy-terminated group (3-MPA) in the SAM was shorter than that of the amine-terminated group (2-MEA) in the SAM (Fig. S2 and Table 1). In other words, the amount of Au exposed on the SAM was probably smaller for 3-MPA than for 2-MEA. In our SAM system, 3-MPA selectively responded to cationic amino acids, whereas 2-MEA did not (Fig. 4 and 5). Considering previous reports on the nonspecific adsorption of amino acids onto bare Au,46 in the case of the 2-MEA-SAM, the affinity between Au and amino acids as well as the interaction between 2-MEA and amino acids could be represented as responses. Thiol molecules containing long-chain alkyl groups easily accumulate on the Au surface.47,48 In the future, SAMs should be modified with such molecules to evaluate the interaction between amino acids and assembled amines without affinity between amino acids and Au.
Considering PPIs, our findings regarding the differences in the selectivity of interactions and the degree of molecular accumulation have interesting implications. 3-MPA, a mimic of the Glu side chain, and 2-MEA, a mimic of the Lys side chain, were modified on the Au surface. Cationic amino acids interacted selectively in the Glu-assembled region, whereas all amino acids interacted non-selectively in the Lys-assembled region (Fig. 6). Proteins abundant in charged amino acids interact with each other and form protein assemblies, such as droplets and aggregates.26,49 Notably, Glu-rich proteins tend to drive protein assembly formation, whereas Lys-rich proteins tend to be incorporated into protein assemblies.50 In view of our results, Glu-rich regions would lead to selective interactions with certain proteins, resulting in the formation of protein assemblies with different compositions. In contrast, Lys-rich regions might be easily incorporated into various protein assemblies through nonselective interactions. Based on the protein data bank, in the distribution of amino acids in protein conformations, the abundance of Glu is greater than that of Lys in the folded structure, whereas it is the opposite in the disordered structure.51,52 Because the Glu side chain assembles more easily than the Lys side chain (Table 1), Glu is probably more involved in the compact protein structure than Lys. Our observations on the assembly propensity of amino acid side chains are significant for reconsidering protein folding. Moreover, amine compounds inhibit the inactivation and aggregation of proteins, but the underlying mechanisms for these effects remain unelucidated.53,54 We observed nonselective interactions of amino groups (Fig. 6), possibly describing the potential for inhibition of PPIs by amine compounds. Our system can be used to explore new compounds that inhibit PPIs.
Conclusions
We established an experimental model and analytical platform for the evaluation of AAIs using a FET-based sensor functionalized with amino acid-mimicking monolayers. Using this system, the interactions between charged amino acids and accumulated side chains were detected in nmol order based on electrical responses. Interestingly, cationic amino acids responded selectively to carboxylic acid-accumulated monolayers, whereas all amino acids responded non-selectively to amine-accumulated monolayers. The slight difference in the molecular structure of the SAM probably contributed to the change in the interaction. In addition, we could also evaluate the differences in AAIs as a function of ionic strength. We believe that the applicability of this model could be expanded to the analysis of not only other AAIs governing PPIs but also non-specific interactions between proteins and small molecules.
Data availability
The data supporting this article have been included as part of the ESI.†
Author contributions
A. N.: conceptualization, investigation, writing – original draft. K. S.: conceptualization, supervision. T. M.: investigation, methodology, supervision. All authors: writing – review and editing.
Conflicts of interest
There are no conflicts to declare.
Acknowledgements
A part of this study was conducted at the AIST Nano-Processing Facility, supported by the “Nanotechnology Platform Program” of the Ministry of Education, Culture, Sports, Science and Technology (MEXT), Japan. We acknowledge BioCMOS Co., Ltd. and Mitorika Co., Ltd. for preparing and supplying the FET sensor array chip. We would also like to thank Dr. H. Sugai for useful discussions on the selection of SAMs that mimic the electrically charged side chains of amino acids. We gratefully acknowledge financial support provided by the Japan Science and Technology Agency, Precursory Research for Embryonic Science and Technology (JST PRESTO) program (Grant No. JPMJPR21RB), the Japan Society for the Promotion of Science (JSPS) KAKENHI (Grant No. 22K19284, 23KJ0259), the Tanaka Kikinzoku Memorial Foundation, and the Fuji Seal Foundation, and a special strategic grant from AIST.
Notes and references
- J. Zhu, N. Avakyan, A. Kakkis, A. M. Hoffnagle, K. Han, Y. Li, Z. Zhang, T. S. Choi, Y. Na, C.-J. Yu and F. A. Tezcan, Chem. Rev., 2021, 121, 13701–13796 CrossRef CAS PubMed.
- V. N. Uversky, Curr. Opin. Struct. Biol., 2017, 44, 18–30 CrossRef CAS PubMed.
- S. F. Banani, H. O. Lee, A. A. Hyman and M. K. Rosen, Nat. Rev. Mol. Cell Biol., 2017, 18, 285–298 CrossRef CAS PubMed.
- R. Kumar, S. Singh and O. V. Singh, J. Ind. Microbiol. Biotechnol., 2008, 35, 377–391 CrossRef CAS PubMed.
- J. A. Wells and C. L. McClendon, Nature, 2007, 450, 1001–1009 CrossRef CAS.
- I. M. A. Nooren and J. M. Thornton, EMBO J., 2003, 22, 3486–3492 CrossRef CAS PubMed.
- G. L. Dignon, R. B. Best and J. Mittal, Annu. Rev. Phys. Chem., 2020, 71, 53–75 CrossRef CAS PubMed.
- V. S. Rao, K. Srinivas, G. N. Sujini and G. N. S. Kumar, Int. J. Proteomics, 2014, 2014, 147648 Search PubMed.
- M. M. Pierce, C. S. Raman and B. T. Nall, Methods, 1999, 19, 213–221 CrossRef CAS PubMed.
- J. Vaynberg and J. Qin, Trends Biotechnol., 2006, 24, 22–27 CrossRef CAS.
- B. Causier and B. Davies, Plant Mol. Biol., 2002, 50, 855–870 CrossRef CAS PubMed.
- D. W. Piston and G.-J. Kremers, Trends Biochem. Sci., 2007, 32, 407–414 CrossRef CAS PubMed.
- G. T. Valente, M. L. Acencio, C. Martins and N. Lemke, PLoS One, 2013, 8, e65587 CrossRef CAS PubMed.
- C. Prieto and J. De Las Rivas, Nucleic Acids Res., 2006, 34, W298–W302 CrossRef CAS PubMed.
- C. Stark, B.-J. Breitkreutz, T. Reguly, L. Boucher, A. Breitkreutz and M. Tyers, Nucleic Acids Res., 2006, 34, D535–D539 CrossRef CAS PubMed.
- K. Kamagata, M. Ariefai, H. Takahashi, A. Hando, D. R. G. Subekti, K. Ikeda, A. Hirano and T. Kameda, Sci. Rep., 2022, 12, 13718 CrossRef CAS PubMed.
- K. A. Burke, A. M. Janke, C. L. Rhine and N. L. Fawzi, Mol. Cell, 2015, 60, 231–241 CrossRef CAS PubMed.
- T. Minamiki, Y. Ichikawa and R. Kurita, Sensors, 2020, 20, 1–28 CrossRef PubMed.
- T. Minami, T. Minamiki and Y. Sasaki, Electrochemistry, 2018, 86, 303–308 CrossRef CAS.
- S. M. Patrie and M. Mrksich, Anal. Chem., 2007, 79, 5878–5887 CrossRef CAS PubMed.
- P. Didier, N. Lobato-Dauzier, N. Clément, A. J. Genot, Y. Sasaki, É. Leclerc, T. Minamiki, Y. Sakai, T. Fujii and T. Minami, ChemElectroChem, 2020, 7, 1332–1336 CrossRef CAS.
- M. Wipf, R. L. Stoop, A. Tarasov, K. Bedner, W. Fu, I. A. Wright, C. J. Martin, E. C. Constable, M. Calame and C. Schönenberger, ACS Nano, 2013, 7, 5978–5983 CrossRef CAS PubMed.
- T. Minamiki, Y. Ichikawa and R. Kurita, ACS Appl. Mater. Interfaces, 2020, 12, 15903–15910 CrossRef CAS PubMed.
- N. Crivillers, E. Orgiu, F. Reinders, M. Mayor and P. Samorì, Adv. Mater., 2011, 23, 1447–1452 CrossRef CAS PubMed.
- R. M. Kramer, V. R. Shende, N. Motl, C. N. Pace and J. M. Scholtz, Biophys. J., 2012, 102, 1907–1915 CrossRef CAS PubMed.
- S. Xue, R. Gong, F. He, Y. Li, Y. Wang, T. Tan and S.-Z. Luo, Sci. Adv., 2019, 5, eaax5349 CrossRef CAS PubMed.
- M. Idrees, A. R. Mohammad, N. Karodia and A. Rahman, Antibiotics, 2020, 9, 1–23 CrossRef PubMed.
- N. Shambetova, Y. Chen, H. Xu, L. Li, J. Solandt, Y. Zhou, J. Wang, H. Su, H. Brismar and Y. Fu, J. Phys. Chem. C, 2016, 120, 3519–3529 CrossRef CAS.
- P. H. Connett and K. E. Wetterhahn, J. Am. Chem. Soc., 1986, 108, 1842–1847 CrossRef CAS.
- T. Kajisa and T. Sakata, Jpn. J. Appl. Phys., 2015, 54, 04DL06 CrossRef.
- J. J. Chance and W. C. Purdy, Thin Solid Films, 1998, 335, 237–244 CrossRef CAS.
- X. Huang, J. Xu, H.-F. Ji, G. Li and H. Chen, Anal. Methods, 2014, 6, 4530–4536 RSC.
- A. Kudelski, Surf. Sci., 2002, 502–503, 219–223 CrossRef CAS.
- H. Sota, H. Yoshimine, R. F. Whittier, M. Gotoh, Y. Shinohara, Y. Hasegawa and Y. Okahata, Anal. Chem., 2002, 74, 3592–3598 CrossRef CAS PubMed.
- J. Shi, T. Yang, S. Kataoka, Y. Zhang, A. J. Diaz and P. S. Cremer, J. Am. Chem. Soc., 2007, 129, 5954–5961 CrossRef CAS PubMed.
- Y. K. Ng and L. Konermann, J. Am. Chem. Soc., 2024, 146, 8394–8406 CrossRef CAS PubMed.
- Y. Shimazaki, M. Takani and O. Yamauchi, Dalton Trans., 2009, 7854–7869 RSC.
- H. D. M. Pham, G. C. Boles and P. B. Armentrout, J. Phys. Chem. A, 2021, 125, 6332–6347 CrossRef CAS PubMed.
- N. Tang and L. H. Skibsted, J. Agric. Food Chem., 2016, 64, 4376–4389 CrossRef CAS PubMed.
- S. Peng and C. Wu, Macromolecules, 1999, 32, 585–589 CrossRef CAS.
- H. I. Okur, J. Hladílková, K. B. Rembert, Y. Cho, J. Heyda, J. Dzubiella, P. S. Cremer and P. Jungwirth, J. Phys. Chem. B, 2017, 121, 1997–2014 CrossRef CAS PubMed.
- N. Schwierz, D. Horinek, U. Sivan and R. R. Netz, Curr. Opin. Colloid Interface Sci., 2016, 23, 10–18 CrossRef CAS.
- L. A. Ferreira, V. N. Uversky and B. Y. Zaslavsky, J. Mol. Liq., 2019, 277, 123–131 CrossRef CAS.
- A. Nomoto, S. Nishinami and K. Shiraki, Biophys. Chem., 2022, 287, 106831 CrossRef CAS PubMed.
- C. Nacar, Protein J., 2020, 39, 21–32 CrossRef CAS PubMed.
- D. Biriukov and Z. Futera, J. Phys. Chem. C, 2021, 125, 7856–7867 CrossRef CAS.
- K. Konno, E. Ito, J. Noh and M. Hara, Jpn. J. Appl. Phys., 2006, 45, 405 CrossRef CAS.
- E. Bedford, V. Humblot, C. Méthivier, C.-M. Pradier, F. Gu, F. Tielens and S. Boujday, Chem. – Eur. J., 2015, 21, 14555–14561 CrossRef CAS PubMed.
- S. Ambadipudi, J. Biernat, D. Riedel, E. Mandelkow and M. Zweckstetter, Nat. Commun., 2017, 8, 275 CrossRef.
- M. Hardenberg, A. Horvath, V. Ambrus, M. Fuxreiter and M. Vendruscolo, Proc. Natl. Acad. Sci. U. S. A., 2020, 117, 33254–33262 CrossRef CAS PubMed.
- S. M. Cascarina, M. R. Elder and E. D. Ross, PLoS Comput. Biol., 2020, 16, e1007487 CrossRef PubMed.
- P. Y. Chou and G. D. Fasman, Biochemistry, 1974, 13, 211–222 CrossRef CAS PubMed.
- M. Kudou, K. Shiraki, S. Fujiwara, T. Imanaka and M. Takagi, Eur. J. Biochem., 2003, 270, 4547–4554 CrossRef CAS PubMed.
- A. J. Michael, J. Biol. Chem., 2018, 293, 18693–18701 CrossRef CAS PubMed.
Footnote |
† Electronic supplementary information (ESI) available: Analysis details of output voltage and self-assembled monolayers. See DOI: https://doi.org/10.1039/d4lf00310a |
|
This journal is © The Royal Society of Chemistry 2025 |
Click here to see how this site uses Cookies. View our privacy policy here.