DOI:
10.1039/D4NR03761H
(Minireview)
Nanoscale, 2025,
17, 1936-1953
Advances in simulating dilute alloy nanoparticles for catalysis
Received
13th September 2024
, Accepted 1st December 2024
First published on 2nd December 2024
Abstract
Dilute alloy (DA) catalysts, including single-atom alloys (SAAs), which are comprised of trace amounts of an active promoter metal dispersed on the surface of a selective host metal, offer exceptional activity and selectivity while utilizing precious metals more efficiently. Although most SAA and DA applications have focused on partial hydrogenation and oxidation reactions, their use has steadily expanded into more complex thermo-, photo-, and electro-catalytic processes. This progress has been largely driven by mechanistic insights derived from computational chemistry and is expected to accelerate with the advancement of artificial intelligence. This minireview discusses novel advances in simulating SAAs and DAs for catalysis applications, including ab initio calculations, multiscale modeling, and machine learning. Emphasis is placed on the impact of reaction conditions, promoter ensembles, and nanoparticle morphology on the stability and catalytic performance of SAAs and DAs. Finally, a perspective is offered on potential future directions of SAA and DA simulations and their extension to other systems with distinct, well-defined active sites.
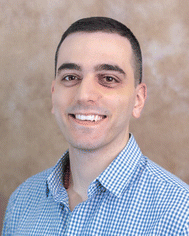 John N. El Berch | John El Berch received his BS degree in Chemical Engineering (2021) from Simon Bolivar University in Caracas, Venezuela. He is currently pursuing his PhD in Chemical Engineering at the University of Pittsburgh, where he investigates novel catalysts for carbon dioxide utilization by combining density functional theory calculations and machine learning. |
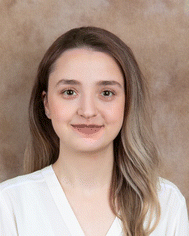 Maya Salem | Maya Salem earned her B.S. in Chemical Engineering from North Carolina State University in 2019. She recently completed her Ph.D. in Chemical Engineering at the University of Pittsburgh in 2024. Her research focused on computationally investigating single atom alloy stability, utilizing density functional theory and machine learning. |
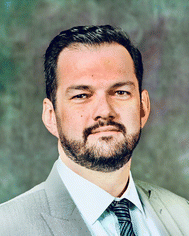 Giannis Mpourmpakis | Giannis Mpourmpakis is a Full Professor of Chemical Engineering at the National Technical University of Athens and an Adjunct Professor at the University of Pittsburgh. He received BS and PhD degrees in Chemistry from the University of Crete, and he worked as a Marie Curie fellow and senior scientist in the Chemical Engineering Department at the University of Delaware. He has been recognized for his cutting-edge research with prestigious awards, including the 2017 NSF CAREER award and the 2019 Bodossaki Foundation Young Scientist Prize. His research focuses on the first-principles-based multiscale modeling of nanomaterials for energy and environmental applications. |
Introduction
Metal alloying has been used to improve the structural and electronic properties of heterogeneous catalysts for over a century.1 Additionally, given the differences between the surface and bulk compositions of alloys,2 metal alloying can decrease the use of precious, active (usually Pt-group) metals by diluting and dispersing them on the surface of cheaper but less active host metals (e.g. coinage metals). Previous studies have shown that even trace amounts of promoter metals (<1% of the catalyst composition) can enhance the catalytic properties of the host.3,4 Thus, significant efforts have focused on developing and understanding the function of dilute alloys (DAs) for catalysis applications,5,6i.e., alloys with much higher host concentrations relative to the promoter, with the latter being generally dispersed in small surface clusters. Among DAs, single-atom alloys (SAAs), in which isolated, single promoter atoms are scattered on the surface of the host, have gained notable attention due to their unique bifunctionality, combining the high catalytic activity of the isolated promoter atoms with the weak intermediate binding of the host.7–13
In the design of SAA and DA catalysts, computational chemistry calculations, mainly based on density functional theory (DFT), have been pivotal in understanding the governing physicochemical phenomena leading to their desired functionality. DFT calculations have shown how, due to their bifunctionality, SAAs can escape the linear scaling relationships (LSR) that traditionally constrain the performance of mono- and bi-metallic catalysts.11–13 These include the Brønsted–Evans–Polanyi (BEP) relationships,14–17 the thermochemical scaling relationships,18–20 and the d-band model,21–23 all of which are well-known linear relationships for catalyst design. Moreover, DFT calculations of segregation (Eseg), i.e., migration of promoter atoms from bulk to surface sites, and aggregation energies (Eagg), i.e., formation of surface clusters from dispersed promoter metal atoms, have provided thermodynamic grounds on the stability of SAAs and DAs, both under vacuum and in the presence of adsorbates (e.g., reaction intermediates).24–26
Furthermore, pairing DFT with multiscale modeling techniques has provided kinetic insights into catalytic events on the catalyst surface. For instance, by combining experimental work with DFT-parametrized kinetic Monte Carlo (kMC) simulations, Marcinkowski et al.27 unveiled how spectator CO molecules can block the active Pd sites on a Pd-promoted Cu SAA, acting as a “molecular cork” that inhibits the uptake and desorption of surface H atoms. Variations of the molecular cork effect have also been investigated via DFT-parametrized kMC, including the desorption of hydrogen on Pt-promoted Cu SAAs,28 as well as the coupling of methyl groups from methyl iodide on Ni- and Pd-promoted Au SAAs.29 Additionally, based on the molecular cork effect, Pt- and Pd-promoted Cu SAAs have been proposed as hydrogen carriers.30 Moreover, molecular dynamics (MD) simulations by Gu et al.31–33 have shed light on the H2 spillover mechanism on Pt-promoted Cu SAAs. Initial ab initio MD simulations showed how, following a dissociation step on the Pt site, one H atom migrates to the Cu host while the other atom remains trapped in the vicinity of the promoter, inhibiting the dissociation of additional H2 molecules.31 To extend the timescale of the initial simulations, the potential energy surface was defined using a DFT-trained neural network (NN),32 which further revealed how collisions with incoming H2 molecules enable the spillover of the trapped H* atoms.33
It becomes apparent that SAA and DA catalysts are ideal systems for computational research because of the well-defined nature of active sites and the multiscale nature of catalytic events, thus enabling a gamut of computational techniques to be applied for their study. In this minireview, we cover recent computational insights and advances in the design of SAA and DA nanoparticles (NPs) for catalysis applications (Fig. 1). Moving forward, we use the PM–HM notation to describe SAA and DAs, where PM corresponds to the less-abundant promoter metal, while HM refers to the host metal (e.g., RuCo corresponds to Ru-promoted Co). Special attention is given to the effect of promoter-ensembles, NP morphology effects, and the emerging role of machine learning (ML) in accelerating the discovery of DA catalysts. Although we include very select experimental observations, this review article primarily focuses on computational advances guiding researchers on what is the state-of-the-art on simulations of SAAs and DAs. Hence, we point readers with an experimental interest in SAAs and DAs to remarkable reviews related to their synthesis,10,34,35 structural analysis,10,36 and experimental applications.10,34–36
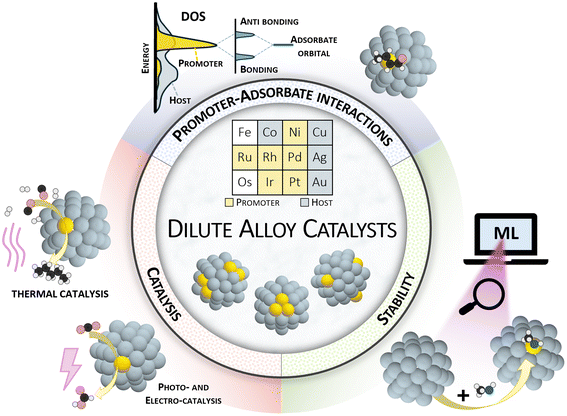 |
| Fig. 1 Graphical overview of recent developments in SAA and DA computational research, including insights dictating the promoter–adsorbate interactions, rapid screening of segregation trends, and catalyst discovery for thermo-, electro-, and photo-catalytic applications. | |
Key insights dictating promoter–adsorbate interactions
Unique electronic structure of SAAs and DAs and its implications on adsorption
The unique properties of SAA catalysts stem from the poor electronic mixing between the isolated promoter atoms and the host-metal matrix. By analyzing the d-band projected density of states (PDOS) of Ni, Pt, Pd, Ir, and Rh-promoted SAAs having Ag, Au, and Cu as hosts, Thirumalai and Kitchin37 showed how this poor mixing led to narrow peaks close to the Fermi level on the promoter atoms. This narrow feature has been experimentally validated by Greiner et al.38 on a CuAg SAA and later by Hartwig et al.39 on a PdAg SAA. Hence, the isolated promoter atoms exhibit free-atom-like electronic structures, which interact with adsorbates similar to organometallic complexes (i.e., through bonding and antibonding molecular orbitals).37,38 Conversely, on mono- and intermetallic catalysts that follow the d-band model, adsorbate states are broadened and shifted from coupling with metallic sp-states, forming bonding and antibonding states by coupling with the metallic d-states.40,41
The narrow d-band feature of SAA promoters helps explain the high selectivity of SAA catalysts in partial hydrogenation reactions.42,43 In this regard, Spivey and Holewinski44 revealed how the location of the d-band promoter peak determines the selective hydrogenation of crotonaldehyde (a model α,β unsaturated aldehyde) on SAAs with Cu, Ag, and Au hosts (Fig. 2A and B). The hydrogenation of crotonaldehyde can occur via its carbonyl group, leading to an unsaturated alcohol, or via its alkene group, leading to a saturated aldehyde (Fig. 2B). Depending on the location of the sharp promoter d-band peak, the molecular orbitals associated with each hydrogenation pathway can be selectively hybridized (Fig. 2A). Thus, late transition metal (TM) promoters favor alkene hydrogenation, whereas earlier TMs favor carbonyl hydrogenation. Similar trends were observed by Ngan and Sautet,45 when investigating the selective hydrogenation of acrolein (a different α,β unsaturated aldehyde) on Cu-based SAAs, i.e., early TMs hydrogenate the carbonyl group, whereas late TMs hydrogenate the alkene group. Additional work by Fung et al.46 on Cu-based SAAs showed how the narrow d-band peaks can effectively hybridize with the C–H σ and σ* orbitals of methane, enabling its low-temperature activation. Furthermore, CH4 chemisorption was found to be favored over early TM promoters, which have energetically high (electron-accepting) d-band centers.
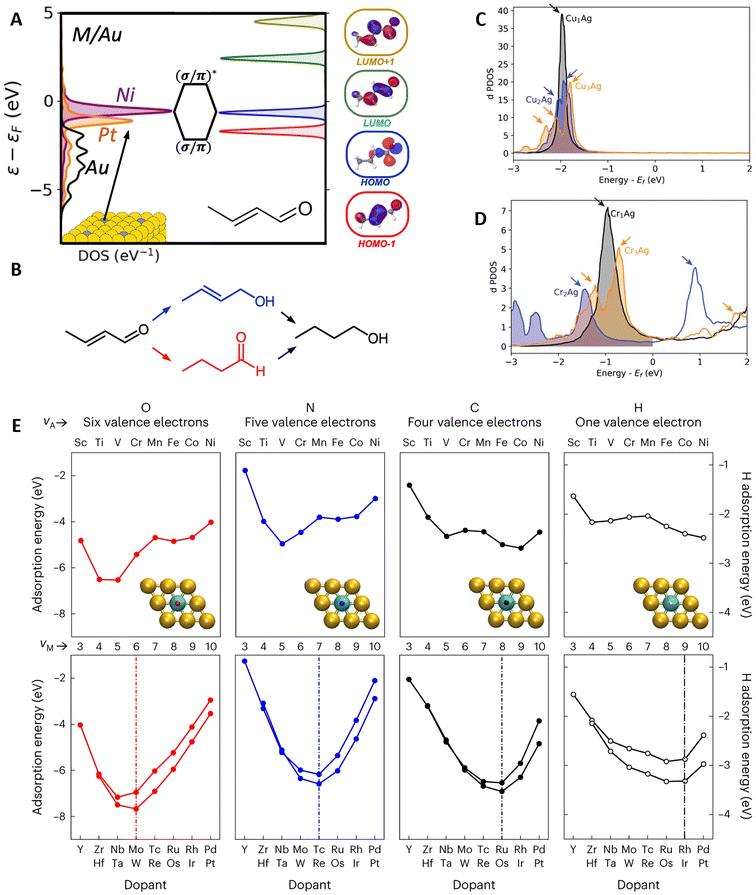 |
| Fig. 2 Insights on promoter–adsorbate interactions. (A) Effect of the promoter sharp peak (in the figure Ni or Pt) on the d-band PDOS and the hybridization of the molecular orbitals of crotonaldehyde. (B) The impact of the peak's location on the reduction pathways of crotonaldehyde (blue and red pathways color-matching the molecular orbitals in (A) related to the different binding configurations), reprinted (adapted) with permission from ref. 44, copyright 2021 American Chemical Society. Broadening and splitting of the sharp peak in the d-band PDOS with increasing ensemble sizes (monomers, dimers, and trimers) of (C) CuAg and (D) CrAg DAs, with arrows indicating the location of PDOS peaks, reproduced from ref. 47 with the permission of AIP Publishing. (E) Periodic trends for Eads of different adsorbates, highlighting the 10-electron-count rule and the deviations in 3d metal promoters. In the figure, νA and νM correspond to the valence electrons of the adsorbate and promoter metal, respectively, reproduced with permission from ref. 50. | |
Moving beyond the SAA into the DA limit, Monasterial et al.47 investigated how the d-band PDOS and hydrogen adsorption change with increasing promoter-ensemble sizes (i.e., going from isolated metal promoter atoms to small promoter clusters) on Ag, Au, Cu, and Pd hosts. Overall, hybridization between promoter atoms broadens and splits the sharp d-band feature into new peaks. The number of new peaks generally matches the number of promoter atoms in the ensemble, e.g., a trimer has three peaks (Fig. 2C and D). Meanwhile, the resulting PDOS broadening depends on the promoter–promoter and host–promoter hybridization. Notably, when this broadening occurs across the Fermi level (Fig. 2D), it can lead to non-monotonic changes in hydrogen adsorption energy (Eads), i.e., Eads does not scale linearly with the number of grouped promoters, despite hydrogen binding on the hollow site. This behavior is unique to DAs and contrary to their intermetallic counterparts. In a different study, Papanikolaou and Stamatakis48 observed a similar PDOS peak broadening and splitting trend in NiCu SAA and DAs (Ni dimers and trimers). Furthermore, increasing the number of Ni atoms decreased the activation barrier (Eact) for CO, NO, and N2 dissociation. This reduction could be attributed to an increased electron back-donation to the antibonding orbitals of the molecules, as the PDOS peaks of the DAs are closer to the Fermi level compared to that of the SAA case.
Promoter-based trends in adsorption and spillover
In general, the preferred binding site/configuration of adsorbed species on SAAs depends on their number of dangling bonds. Species with a single dangling bond or lone pairs of electrons favor top-site adsorption, while species with two or three dangling bonds favor bridge- or hollow-site adsorption.12,13 Furthermore, Eads is determined by the group number (i.e., number of valence electrons) of the promoter, often leading to U-shaped relationships.46,47,49 Motivated by the similarities between SAAs and molecular systems, Schumann et al.50 rationalized these U-shaped relationships using a simple 10-electron count rule (Fig. 2E). The 10-electron rule predicts a maximum Eads when the interacting valence electrons of the adsorbate and the valence electrons of the promoter add up to ten, i.e., when the electrons of the adsorbate fill and saturate the d-orbitals of the promoter. It is worth noting that an adsorbate's number of interacting electrons is not necessarily equal to the number of valence electrons. For example, C* has two interacting electrons but four valence electrons, thus binding preferably on group-8 promoters (third column in Fig. 2E). Deviations from the rule can be found in 3d promoters due to spin effects and for adsorbates interacting non-covalently, like H2O and NH3, which bind through electrostatic interactions.50
Besides adsorption, spillover (i.e., the diffusion, from promoter to host sites, of small adsorbed species such as hydrogen atoms) is critical for the bifunctional operation of SAA catalysts.11 Although spillover is entropically favored,12 Schumann et al.51 found that host atoms neighboring the promoter have weaker Eads than host atoms farther away, inhibiting the diffusion of adsorbates outside promoter-neighboring sites. Additional kMC simulations and experiments confirmed the role of this weak adsorption ring in preventing the diffusion of adsorbates deep into the host matrix. Remarkably, the discrepancy in Eads between host atoms decreases monotonically with the chemical group of the promoter, e.g., O* binding is ∼0.20 eV less favorable on Cu sites close to a Ru promoter compared to Cu sites far from it; nonetheless, this difference reduces to ∼0.05 eV when Pd is the promoter. Once more, exceptions are found for 3d promoters due to spin effects and for promoters with less favorable Eads compared to the host.
To accelerate the prediction of spillover behavior, Réocreux et al.52 developed a simplified linear model to compute the spillover energy (SOE), i.e., the difference in formation energies of adsorbed species on host sites versus promoter sites. The model predicts DFT-obtained SOEs with a mean absolute error of 0.06 eV using two descriptors: (1) the charge of the promoter, relevant for saturated species that bind through electrostatic interactions, and (2) the SOE of C*, relevant for unsaturated species that bind through covalent bonds. Later work by Hannagan et al.53 used the SOE as a key factor in designing NiCu SAA catalysts for formic acid decomposition. Moreover, SOE calculations provided insights into the poor enhancement provided by Pt promoters, which, like Ni, facilitate the rate-limiting C–H activation step but, unlike Ni, are limited by the unfavorable reverse spillover of formate intermediates from the Cu host.
Stability of SAAs and DAs
Thermodynamic stability of SAAs and DAs
As previously mentioned, DFT-calculated Eseg and Eagg values are used to predict the formation of isolated active sites on the surface both under vacuum and in the presence of adsorbates.10,26 The stability of DA and SAA NPs is influenced by several factors, including surface energies, atomic radii, metal cohesive energies (CE), and promoter concentrations.54–57 Additionally, metal–metal and adsorbate–metal interactions have been shown to dictate the formation of stable SAAs.24,56,58 In this section, we particularly focus on how adsorbates, solvents, and applied potentials (in electrochemical applications) affect the overall stability of DAs and SAAs.
Metal–adsorbate interactions can lead to reverse segregation trends and/or the formation of clusters in SAAs.24,26,56,59–61 To further understand these effects, Ouyang et al.62 employed a combination of experiments and Monte Carlo (MC) simulations (parametrized using a DFT-fitted cluster expansion model) to study how the chemical ordering (i.e., how different metal atoms occupy the lattice positions) of PdAu NPs can be tailored by varying the CO partial pressure and temperature. The strong binding between Pd and CO enhances the thermodynamic stability of Pd atoms on the surface of the alloy, promoting their segregation to the surface. Furthermore, at low CO coverage, the strong Pd-CO interaction favors the formation of Pd aggregates. On the other hand, at high CO coverages, CO–CO lateral interactions prevent Pd aggregation, dispersing them into single atoms, which is crucial in enhancing the selectivity of acetaldehyde in the partial dehydrogenation of ethanol. It is worth noting that segregation is not always induced in the presence of adsorbates, as demonstrated by Yu et al.61 on NiCu(111) SAAs. DFT-calculated Eseg revealed Ni segregation is favored in the presence of CO and O, whereas Ni stays in the bulk when exposed to H.
Additionally, solvent environments can influence the thermodynamic stability of DAs,63 as solvent molecules can coordinate with the surface of metallic NPs.64 For instance, by combining DFT calculations and experiments, Doronin et al.65 revealed that in aqueous environments, water molecules increase the tendency of Ag to segregate to the surface of AgAu alloys (Fig. 3A). Interestingly, the effects of solvent molecules on the segregation behavior can become more pronounced under electrochemical conditions, as externally applied potentials can affect the stability of solvent molecules and intermediates on the surface.66 In this regard, Weitzner et al.67 investigated the stability of ten Cu(100) based SAAs via a hybrid atomistic-continuum approach. Surface-level phenomena were studied by pairing DFT with effective screening medium (ESM) and the electrolytic environment (1 M KOH) was implicitly considered through the reference interaction site method (RISM). Interfacial effects in the ESM-RISM scheme are bridged using a grand canonical scheme, adjusting the number of electrons and electrolyte ions according to the applied potential. Importantly, the segregation trends were found to vary across promoters when a reducing potential was applied (Fig. 3B). For instance, in the absence of adsorbates, the surface segregation of Al, Pt, and Ga promoters becomes less favorable with increasing reducing potentials, suggesting a sub-surface migration at strong cathodic conditions. Remarkably, comparing these results with a separate set of Eseg calculations conducted in the absence of the electrolyte layer, similar segregation trends were found upon CO and OH adsorption (e.g., OH drives the segregation of oxyphilic promoters like Al).
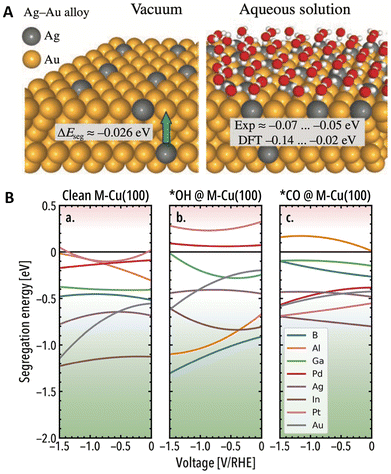 |
| Fig. 3 Impact of (A) aqueous solvent environments on the segregation behavior of AgAu(100) and (111) surfaces, reprinted from the Lancet (ref. 65), copyright 2020, with permission from Elsevier. (B) Applied potential effects on the surface segregation of Cu-based (100) DAs in the absence of adsorbates (a), and in the presence of OH* (b) and CO* (c) in 1 M KOH (different colors represent different promoters). Positive Eseg values indicate the promoter prefers to remain in the bulk, whereas negative to segregate to the surface, reproduced from ref. 67 with the permission of AIP Publishing. | |
Accelerated methods for modeling the stability of SAAs and DAs
ML has significantly advanced the study of SAAs by accelerating the prediction of formation energies,68Eseg,69–71 and Eagg
72 with DFT-level accuracy. Thus far, predictive models have primarily focused on the effects of catalysis-relevant adsorbates, such as CO and H, on the segregation behavior.71,73 For instance, Hipolito and coworkers74 focused on identifying stable SAAs for ORR by developing a linear support vector machine regression model in the presence of O and OH groups.74 However, one can view the SAA and DA NPs from two different perspectives, synthesis and applications, hence, the role of commonly used ligands in colloidal NP synthesis on the segregation behavior is also important. Work by Salem et al.75 aimed to examine the effect of amine and thiol groups used in colloidal NP synthesis on Eseg trends. They developed a NN multilayer perceptron model to predict Eseg in promoter–host metal combinations of d8- (Ni, Pd, Pt) and d9- (Ag, Au, Cu) SAAs on (100) and (111) facets, incorporating features that account for adsorbate effects (Eads on a single metal atom), structural effects (CN and atomic radius), electronic properties (electron affinity), and cohesion of metal in the bulk (CEbulk) – inspired by the bond-centric model,57 previously used to measure the thermodynamic stability in terms of the CE of multi-metallic NPs as a function of morphology and composition.
Similar features used by Salem et al.76 in kernel ridge regression Eseg model, trained on Pt-group metal (111), (100), (110), and (210) surfaces showed exceptional extrapolation capability to SAA NPs of varying sizes (147, 309, and 561 atoms), promoter positions (CN = 7, 8, and 9), and a metal host (Cu) that was not present in the model's training set (Fig. 4A). Similarly, Ghosh and coworkers77 employed a random forest classifier to determine the chemical ordering in a 55-atom icosahedral binary-alloyed metallic NP consisting of wide range of metal groups, based on the change in the CE, radius, CN, and magnetism. Their model successfully determined core–shell preferences from mixed and Janus orderings, demonstrating the significance of these features in capturing the stability in SAAs.
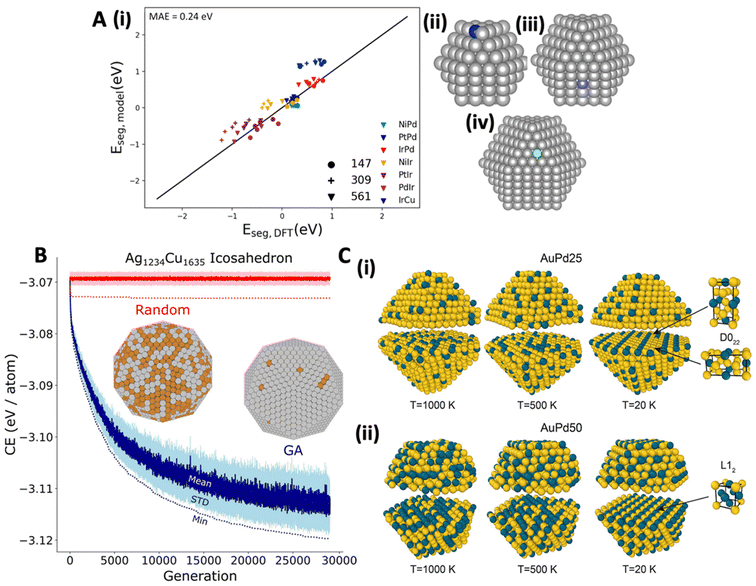 |
| Fig. 4 (A) (i) Eseg predictions based on a five-feature, second order polynomial kernel ridge regression model for SAA NPs consisting of Pd, Ir, and Cu host metals. Marker styles represent the different NP sizes, while different colors represent the various SAA combinations; for instance, NiIr would indicate that Ni is the promoter and Ir is the host. Additionally, panels (ii) to (iv) illustrate the NP systems included in the extrapolation test, specifically, (ii) promoter on a CN = 7 (blue) site using 147-atom cuboctahedra, (iii) promoter on a CN = 8 (purple) site in a 309-atom cuboctahedra, and (iv) promoter on a CN = 9 (green) site in a 561-atom cuboctahedra, reproduced with permission from ref. 76. (B) Comparison between GA optimization and random search on an icosahedral Ag1234Cu1635 NP, represented by blue and red, respectively. The darker solid lines and lighter shaded areas show the mean and standard deviation for each generation, while dotted lines highlight the lowest CE at each generation, with the minimum CE structures illustrated in the figure, reproduced with permission from ref. 80, copyright 2020 American Chemical Society. (C) Chemical ordering of PdAu NPs as a function of temperature and composition (i) AuPd25 and (ii) AuPd50, reproduced with permission from ref. 87, copyright 2024 American Chemical Society. | |
While ML provides powerful tools for predicting Eseg and Eagg based on metal and adsorbate descriptors, it can also be used to rapidly explore the chemical ordering (i.e., stability) in SAA and DA NPs as a function of morphology and composition through the use of genetic algorithms (GA) and further address temperature effects with MC simulations.78–86 Since the CE of the metals and the exposed surface coordination have emerged as important descriptors of stability in SAAs and DAs, one can implement the bond-centric model57 with a GA80 to rapidly (i.e., within minutes) predict the chemical ordering of SAA and DA NPs80,81 (i.e. if a promoter metal will reside on the NP surface of the metal host and at what positions, Fig. 4B). Ter-Oganessian et al.87 combined MD with MC simulations, using a precise ReaxFF potential, to effectively depict bulk and surface ordering, as well as shape restructuring in PdAu NPs at various compositions, temperatures, and NP sizes (Fig. 4C). They found that at low temperatures (20 K), AuPd25 and AuPd50 NPs formed bulk-ordered arrangements, contrasting with more disordered states at 1000 K. Additionally, they also observed that composition plays a role in the arrangement of Au and Pd, revealing that in the AuPd25, D022 bulk order was formed, while L12 was observed in the AuPd50 case (Fig. 4C). Compositional dependent chemical orderings on PdAu NPs have been also reported by Loevlie et al.81 as well as Ekborg-Tanner and Erhart.59
SAA and DA surface kinetics
In the formation of SAAs via vapor deposition, kinetics play a crucial role in determining the final structure and composition of the alloy. During vapor deposition, promoter atoms are deposited onto a host surface where they can diffuse and interact to form the alloy.88 The kinetics of this process, including the rate of deposition, surface diffusion of atoms, and interactions between different species, significantly influence the formation of SAAs.89 In this regard, Wang and coworkers90 used DFT and scanning tunneling microscopy to study the different mechanisms (diffusion hoping, atomic exchange, vacancy formation, and atomic swapping; see Fig. 5A) occurring in Rh alloying on various Cu surfaces. They found that Rh atoms stay above step edges on Cu(111) and alloy directly into the terrace on Cu(100) and Cu(110). The lowest diffusion barrier for Rh was observed on Cu(111), while atomic exchange was favored on Cu(100) and Cu(110). Lower vacancy formation barriers on Cu(100), Cu(110), and Cu(211) compared to Cu(111) suggest that Rh atoms are kinetically trapped above step edges on Cu(111), while more readily integrating into the lattice on other surfaces, promoting the formation of SAAs (Fig. 5A). The impact of vacancies in the anchoring of SAA promoters has also been investigated for other synthesis methods, including metal vacancies during galvanic replacement,91 as well as O-vacancies during temperature annealing.92
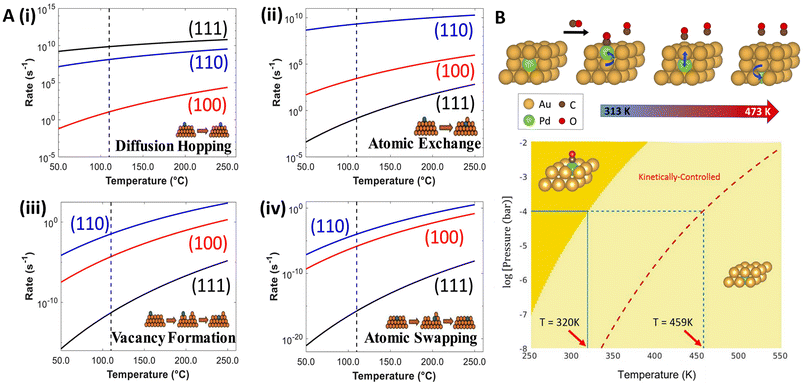 |
| Fig. 5 (A) Predicted rates under vacuum conditions for Cu-hosted Rh (i) hop diffusion, (ii) atomic exchange, (iii) vacancy formation (with a Rh adatom nearby), and (iv) atomic swapping, respectively, reproduced from ref. 90 with the permission of AIP Publishing. (B) DFT-constructed phase diagram, showing two phases: CO adsorption on Pd monomer (dark yellow) and subsurface Pd with no adsorption (light yellow). At the experimental CO pressure of 0.1 mbar, the thermodynamic transition, i.e., CO desorption and Pd migrating to the subsurface, is expected to occur at 320 K (blue solid line); nonetheless, Pd remains kinetically trapped until a temperature of 459 K (blue dashed line) is reached, reproduced from ref. 94, copyright 2022 American Chemical Society. | |
In another study focusing on the segregation dynamics in NiCu(100) alloys during annealing, Garza et al.93 performed several DFT-parametrized multiscale simulations (MD, parallel trajectory splicing, and kMC) to explore segregation across various timescales. MD simulations covered picosecond to nanosecond scales, revealing rapid vacancy diffusion and initial segregation patterns. To simulate longer timescales (up to microseconds), parallel trajectory splicing was used, which is crucial for understanding how vacancies reintegrate into the subsurface layers. kMC methods extended the analysis to second scales. The results showed that Ni migrates from the top layers during annealing, driven primarily by lattice vacancies. The alloy equilibrium composition that was reached within seconds, matched experimental observations with low Ni concentrations on the top layer and higher in the bulk.
The kinetics of surface segregation are also influenced by the presence of adsorbates. Zhou and coworkers94 investigated the dynamics of surface restructuring in PdAu(111) induced by CO using experiments and DFT. At 0.1 mbar CO pressure, they observed that below 320 K, Pd monomers are stabilized on the surface by CO (Fig. 5B), which aligns with findings from Ouyang et al.62 However, above 320 K, the entropic contribution of CO desorption leads to the dispersion of Pd into the subsurface as opposed to maintaining the Pd monomer on the surface, as indicated by the light-yellow region in Fig. 5B. In the kinetically influenced regime, bounded by the red dashed line, Pd monomers are trapped on the Au surface as CO desorption is unfavorable. This regime reflects the interplay of kinetic pathways influencing the persistence of CO-bound Pd monomers on the surface. Ultimately, above 459 K (dashed blue line in Fig. 5B), complete CO desorption occurs; thus, Pd is no longer favored at the surface, aligning with experimental findings. In related studies, Liu et al.95 combined DFT with operando X-ray absorption fine structure spectroscopy to study the reconstruction of CuAu NP surfaces under electrochemical conditions, finding that isolated Cu atoms migrate from the corners to the (100) plane based on the reduced Cu–O CN (from 0.9 to 0.6) and higher Cu–Au CN (going from 5 to 8.3) under reduction potentials, impacting Cu–Au interactions.
Advances in the design of SAA and DA catalysts
Morphology effects on DA catalysis
A common assumption in the design of SAA catalysts is the facile spillover of reactants from promoter to host sites, where subsequent reaction steps occur. DFT calculations are usually limited to the most stable host facet (e.g., (111) for fcc metals), neglecting the effects of surface coordination on the activity of host and promoter sites. To elucidate how surface coordination impacts the reactivity of Pt-group promoters on Cu-, Ag-, and Au-based SAAs, Papanikolaou et al.48 compared the Eads of N2, NO, and O on top of the promoter sites on the (100) and (111) facets (Fig. 6A). Contrary to the behavior observed on monometallic Pt-group metal surfaces, the SAA catalysts exhibited low structure sensitivity, as similar Eads were found on both facets. Remarkably, a systematic bias of ∼0.1 eV favoring the less coordinated (100) facet was observed, attributed to differences in the electronic mixing of promoter and host atoms across facets. Similar trends were found for the dissociation barriers of NO, CO2, and N2, i.e., small differences between the two SAA surfaces and lower Eact on the (100) facet.
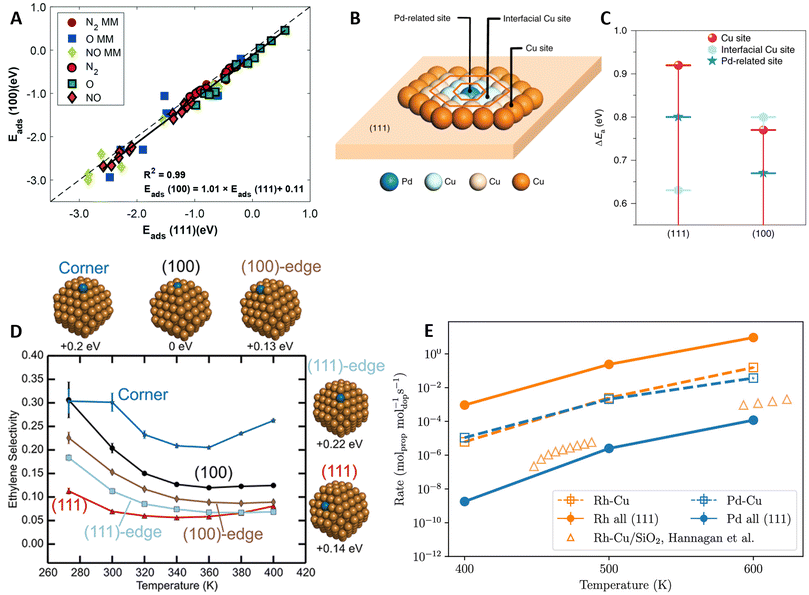 |
| Fig. 6 Coordination and NP shape effects in the catalytic activity of SAA and DAs. (A) Parity plot between the top-site NO, N2, and O Eads computed on the (100) and (111) facets of SAA. For comparison, Eads on the preferred sites of the corresponding monometallic (MM: Pt, Pd, Rh, Ni, Ag, Au, and Cu) surfaces are included, reproduced from ref. 48 with permission from the Royal Society of Chemistry. (B) Different active sites investigated and (C) corresponding Eact for the hydrogenation of phenylacetylene (PhC CH) into styrene on the (100) and (111) facets of PdCu SAA, reproduced from ref. 96 with permission of Springer Nature. (D) Selectivity changes for the partial hydrogenation of acetylene with the promoter location on PdCu SAA NPs (energies of the studied NPs relative to the minimum energy configuration are indicated below the particles), reproduced with permission from ref. 99, copyright 2019 American Chemical Society. (E) Changes in propane dehydrogenation activity with varying promoter locations, comparing RhCu and PdCu SAAs with promoters located on the (111) terrace sites (labeled “dopant all (111)), vs. having the promoters on their most probable NP sites (labeled “dopant-Cu”), with theoretical results from ref. 101, reproduced with permission from ref. 100. | |
In a separate study, Jiang et al.96 explored the effects of surface coordination on spillover hydrogenation reactions. Specifically, the partial hydrogenation of phenylacetylene (PhC
CH) to styrene was investigated on the (100) and (111) facets of PdCu SAAs. Experiments revealed that hydrogen spillover from the Pd atoms into the Cu host was surface insensitive; however, the subsequent alkyne hydrogenation step was hindered on the (111) facet due to the low activity of the host sites. DFT-calculated PhC
CH hydrogenation barriers (Fig. 6B and C) corroborated the experimental trends, with an Eact of 0.92 eV on the Cu(111) facet compared to 0.77 eV on the (100) facet. Additionally, on the (111) facet, Eact values were considerably lower close to the promoter (Pd sites and interfacial Cu sites), verifying that hydrogenation likely occurs in the vicinity of the Pd atoms, thus requiring higher Pd loadings compared to the (100) SAA.
Overall, the coordination of surface atoms is influenced by the morphology of the NP catalyst. Under reaction conditions, an ensemble of NP sizes and shapes is obtained, which can lead to variations in the catalytic activity compared to the most stable NP structure.97 Furthermore, by pairing BEP relationships with kMC simulations, Jørgensen and Grönbeck98 highlighted how the catalytic activity of NPs deviates from that of extended crystallographic planes due to kinetic couplings, i.e., the communication of different NP active sites through rapid adsorbate diffusion, which leads to non-linear additive rates. Focusing on the partial hydrogenation of acetylene into ethylene on a PdCu SAA, octahedral NPs exhibited considerably lower ethylene selectivity compared to the (111) extended surface (∼100% selective).99 Moreover, the predicted C2H4 selectivity varied based on the location of the Pd promoter on the NP (Fig. 6D), highlighting the importance of NP morphology in catalyst design.
Similarly, Bunting et al.100 investigated how different promoter locations affect the propane dehydrogenation activity of RhCu and PdCu SAA NPs. Using a DFT-trained ML potential, MD simulations with transmutation MC swaps revealed that Rh favors bulk segregation, whereas Pd remains on the surface of the NPs. Hence, although lower Eact values were found on Rh sites compared to Pd, the larger amount of surface Pd sites led to comparable dehydrogenation rates between the two SAA NPs (Fig. 6E). It is worth noting that, for RhCu, the microkinetic modeling (mkm) predictions were higher compared to experimental rates from a separate study,101 likely due to DFT limitations. Lastly, dehydrogenation rates were computed on idealized SAA NPs with the promoters on (111) terraces. For RhCu, this idealization resulted in a higher rate due to the higher presence of Rh atoms in the model surface, whereas, on PdCu, it underestimated the rate as less stable (but more reactive) facets were excluded from the mkm simulations.
Designing DAs for thermal catalysis
Thermocatalytic applications of DAs have typically focused on selective (de)hydrogenation and oxidation reactions.5,10 The design process often combines surface science experiments on well-defined surfaces, characterization and testing experiments on the synthesized catalyst, in addition to computational chemistry calculations using DFT and multiscale modeling techniques such as kMC or mkm. Recent examples include the role of hydrogen coverage on the selective (de)hydrogenation of ethyl (C2H5) over PtCu SAAs,102 the isomerization of 1-hexene into 2-hexene on PdAu DAs,103 the enhanced H2 splitting on TiCu SAAs,104 and the non-oxidative ethanol dehydrogenation on NiCu SAAs.105
Expanding on these applications, RuCo SAAs have been explored for Fischer–Tropsch synthesis106–108 (FTS: (2n + 1)H2 + nCO → CnH2n+2 + nH2O) i.e., the polymerization of CHx groups into long-chain hydrocarbons.109 This thermal process has potential applications in providing fuels for sectors difficult to electrify, such as aviation.110 A current challenge in FTS for CO2 utilization lies in separating the CO2 effluent from the upstream reverse water gas shift step,111–114 which can otherwise hydrogenate into methane.115,116 In this context, temperature-programmed reactor studies by Liu et al.107 demonstrated that RuCo SAAs can reactively separate CO from a 50/50 CO/CO2 mixture via FTS at mild temperatures (Fig. 7A). DFT-computed Eads confirmed the SAA potential to separate the mixture, favoring CO chemisorption and limiting CO2 to being physisorbed (Fig. 7B and C). Interestingly, Ru-promotion was not experimentally found to enhance the FTS activity or selectivity, which was verified by comparing the Eact of various elementary steps on promoter and host sites. Instead, the role of the Ru atoms was found to maintain the catalyst in an active, reduced phase. Similar reducibility improvements (caused by an enhanced H2 spillover) have been reported for PtNi SAAs,117,118 demonstrating the capacity of promoters to minimize surface oxidation.
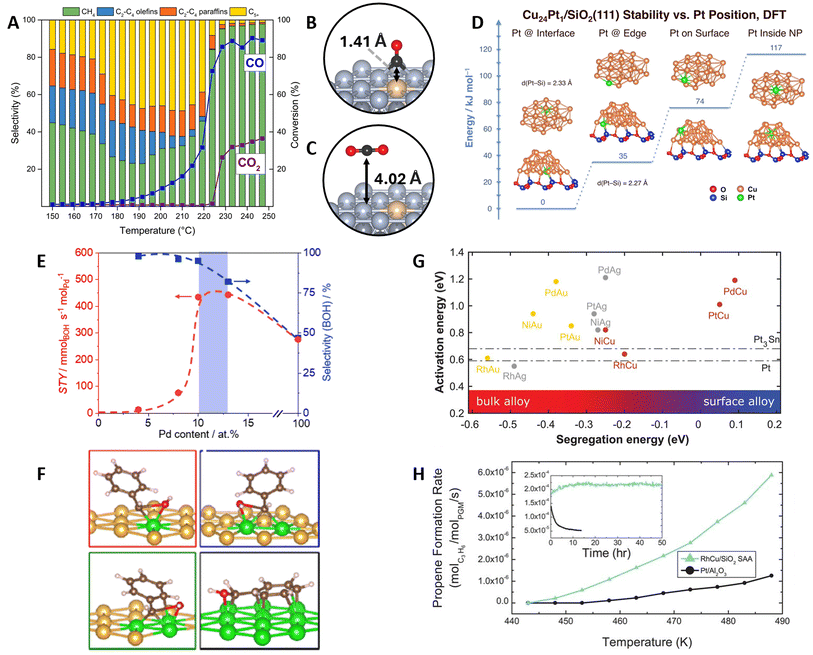 |
| Fig. 7 DA applications in heterogeneous thermal catalysis. (A) Programmed temperature reactor studies on the reactive separation of CO/CO2 mixtures over RuCo SAA (reaction conditions: P = 300 psig, H2 : COx ratio = 2.5 : 1, COx = 50% CO/50% CO2, GHSV = 84 000 mL h−1 g−1, T = 150–250 °C, 10 °C h−1). Side views and vertical distances for (B) CO and (C) CO2 adsorption on the RuCo SAA surface, reproduced with permission from ref. 107. (D) DFT-calculated formation energies of Cu24Pt1 nanoclusters supported on SiO2(111) showing the stabilization of the Pt promoter on the inner SAA-support interface, reproduced with permission from ref. 120. (E) Space–time yield (STY) and BOH selectivity as a function of Pd content on PdAu DAs (reaction conditions: T = 323 K, P = 5 bar, F(H2) = 50 mL STP per min, F(BA) = 0.05 mL min−1, Wcat = 40 mg) and (F) changes in PhCHOH* C–O cleavage transition state over PdAu(111) DAs (Pd monomer, dimer, trimer) and Pd(111), reproduced with permission from ref. 125, copyright 2023 American Chemical Society. (G) Computational screening of SAA catalysts for nonoxidative propane dehydrogenation using the Eact of the first C–H cleavage of CH4 and the Eseg as design parameters. Dash-dotted lines correspond to the Eact on Pt(111) and Pt3Sn(111) (2 × 2). (H) Reactor studies comparing the selected RhCu/SiO2 SAA catalyst with Pt/Al2O3 (reaction conditions: 1.4 kPa of propane and 0.7 kPa of hydrogen; 90 mL min−1 flow rate. Formation rate was normalized per mole of Pt-group metal). The figure inset corresponds to the stability test performed for 50 h at T = 623 K, reproduced from ref. 101 with permission of the American Association for the Advancement of Science. | |
Concerning the role of support effects in DA catalysts, Guan et al.119 highlighted how metal–support interactions between MXene and RuNi SAA NPs enhance the hydrolysis of ammonia-borane (AB: NH3BH3), resulting in uniquely higher activities compared to other RuNi SAAs, as well as MXene-supported Ru single-atom catalysts and NPs. DFT calculations confirmed that lower Eact were found at the support-NP junction. Further Bader charge analysis revealed an electron distribution around the junction, resulting in an electron accumulation around interfacial Ru atoms, thereby favoring the binding and splitting of AB and H2O. In a separate study on the hydrogenation of unsaturated aldehydes into unsaturated alcohols, Han et al.120 showed how the Pt atoms of Si2O-supported PtCu SAA NPs are stabilized at the inner metal–support interface. Remarkably, despite the Pt atoms not being exposed on the catalyst surface, experiments demonstrated that the PtCu NPs maintained a higher selectivity compared to the pure metals. The stability of the Pt atoms on the NP-support interface, due to the formation of Pt-Si bonds, was verified by DFT-computed formation energies of both free and supported Cu24Pt1 nanoclusters (Fig. 7D). Moreover, potential energy surface and H2Eact calculations unveiled that, even when located two layers below the surface, the Pt atoms can modify the electronic properties of the Cu surface and enhance H2 spillover.
Another key aspect in the design of DA catalysts is to identify the optimal promoter ensemble size. As previously discussed, promoter–promoter hybridization broadens and splits the d-states going from SAAs to DAs,47,48 usually leading to higher activities at the expense of selectivity. In this context, several articles have investigated the ensemble size effects on the catalytic performance of PdAu DAs. For instance, in the selective hydrogenation of 1-hexyne into 1-hexene, experiments showed that isolated surface Pd atoms increase the activity of the Au host while maintaining a high selectivity.121–123 However, high Pd loadings lead to the formation of Pd clusters, which decreases both activity and selectivity.122,124 Using acetylene as a proxy to study the triple bond of 1-hexyne, Foucher et al.124 performed DFT and mkm simulations on PdAu(111) DAs of increasing Pd-ensemble sizes (monomers, dimers, and trimers). Comparing the binding of hydrogenation reactants and intermediates, more exergonic Gibbs free energies of formation were found by increasing the ensemble size. Moreover, degree of rate control analysis showed hydrogen dissociation to be the rate-limiting step. Hence, as the cluster size increases, the adsorption of acetylene is enhanced, blocking the Pd sites for hydrogen spillover. This was reflected in the mkm-predicted reaction rates, as the rates of Pd dimers and trimers were 10% and 2%, respectively, of the rate of Pd monomers.
In a separate study on PdAu DAs, Kaiser et al.125 tuned the Pd-ensemble size for benzaldehyde (PhCHO) hydrogenation to benzyl alcohol (BOH). Experiments identified an optimal hydrogenation selectivity on small clusters, around 10 and 12 at% of Pd (Fig. 7E). Isolated Pd atoms exhibited minimal activity in PhCHO hydrogenation, whereas large clusters led to the hydrogenolysis of the C–O bond and the production of toluene, decreasing selectivity to BOH. DFT calculations revealed an increase in the binding strength of PhCHO hydrogenation intermediates with the Pd ensemble size, as larger parts of the aromatic ring could be stabilized (Fig. 7F). Additionally, lower C–O dissociation Eact were found by increasing the ensemble size, thereby explaining the selectivity decline at higher Pd loadings. Other studies on promoter ensemble size effects include the tuning of NiAu and NiCu DAs for ethanol dehydrogenation126 and N2O decomposition,127 respectively, as well as TiCu DAs for ethanol deoxygenation into ethylene128 and MoNi DAs for the hydrodeoxygenation of furfural into 2-methyl furan.129
Moving beyond the experimental design, historically driven by chemical intuition and reliant on trial-and-error testing, the advances in high-throughput computational chemistry calculations have enabled the in silico design of SAA and DA catalysts.130 Through DFT-derived LSR and mkm, Zhang et al.131 screened DA catalysts featuring Ag and Cu hosts, as well as TM promoter monomers and dimers for ammonia synthesis. The formation energy of N* was used as a descriptor for the LSRs, which were paired with mkm to predict turnover frequencies. Overall, higher turnover frequencies for ammonia synthesis were found on promoter dimers compared to monomers, as ensemble effects facilitate the rate-limiting N2 dissociation. Hence, the predicted turnover frequency of the best-performing DA (MoAg, having Mo dimers) was three orders of magnitude higher compared to the best-performing SAA (NbAg). Remarkably, the predicted activity of the MoAg DA catalyst surpassed that of commercial Ru catalysts. The stability of the top-performing catalysts was verified through a combination of formation energy calculations, diffusion barrier calculations for promoter migration to subsurface and adatom sites, and ab initio MD simulations. Similar work involves the screening of SAA and DAs for (de)hydrogenation reactions,132,133 methane dry reforming,134 direct oxidation into methanol,135 and hydrodeoxygenation reactions for bio-oil upgrading.136,137
A great example of simulations leading to experimental validation is from Hannagan et al.101 on designing SAA catalysts for non-oxidative propane dehydrogenation. SAAs consisting of coinage-metal hosts (Au, Cu, Ag) and Pt-group metal promoters were screened based on their segregation trends and methane C–H dissociation activity (Fig. 7G). Among these, RhCu was selected as it showed a low C–H dissociation barrier comparable to industrial Pt and Pt3Sn catalysts, and the most favorable Eseg among the Rh-promoted SAAs. Further analysis of the energetics for the full dehydrogenation of methane unveiled similar activities between RhCu SAA and Pt, but a weaker binding of coke-related intermediates (CH2 and CH) on the SAA. Subsequent reactor experiments demonstrated higher propane dehydrogenation rates and coking resistance on RhCu/SiO2 SAA compared to Pt/Al2O3 (Fig. 7H). Moreover, the SAA maintained a Cu-like selectivity of 100% compared to ∼80% on the Pt catalyst.
SAA application in photo- and electrochemistry
Amidst the ongoing efforts to decarbonize the chemical industry through process electrification,138,139 SAAs have emerged as promising candidates to replace traditional noble metal electrode catalysts.140,141 In this context, DFT calculations have provided valuable insights into catalytic activity and selectivity through the computational hydrogen electrode approximation,142,143 which enables mapping the influence of externally applied potentials on the reaction free energies of elementary steps that include the transfer of a proton–electron pair.
In this way, DFT screening has been used to tune the selectivity of Cu-based alloys for CO2 electrochemical reduction reaction (CO2RR), as Cu is the only known metal catalyst to form C2+ products.144 By comparing the stability of CO2RR intermediates on twelve Cu(111)-based SAAs, Zhi et al.145 found the O* and H* formation energies on the promoter site to describe the selectivity toward C1 products requiring two electron-transfer steps (HCOOH/CO) or more (CH4/CH3OH), in addition to C2+ products (Fig. 8A). The changes in selectivity were explained by the electronic structure of the SAA catalysts, as the O* and H* formation free energies correlate with the d-band center of the promoter atoms. Later work by Jiang et al.146 explored the changes in C1-product selectivity of Cu(111)-based SAAs promoted with 3d TMs. Except for the late TMs, the investigated SAAs were found to activate CO2. Moreover, the OH* formation free energy was found to describe the reaction free energies of common potential-determining steps. Cu-based SAAs have also been explored for other reduction reactions, including the design of a WCu SAA catalyst for the co-reduction of CO2 and N2 to produce acetamide,147 as well as, a PdCu SAA for the reduction of nitrate148 and nitric oxide149 to ammonia. Lastly, interested readers in broader electrochemical applications are encouraged to consider additional DFT and experimental work on the design of SAA catalysts for fuel cell applications150–152 and Li2O2 battery electrodes.153
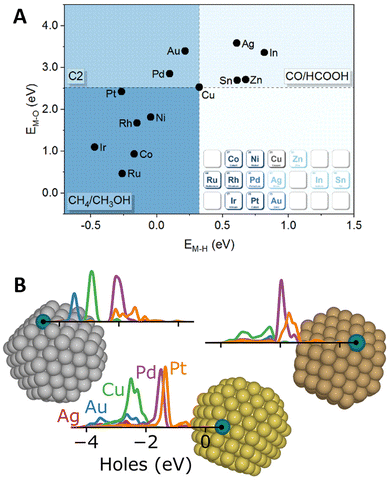 |
| Fig. 8 Novel SAA applications in electrocatalysis and photocatalysis. (A) Favored electrocatalysis products of Cu-based SAAs based on formation free energies of O* (M–O) and H* (M–H) on the promoter site, reproduced from the Lancet (ref. 145) with permission from Elsevier. (B) Illustration highlighting the localized hot-hole distributions projected on the promoter atoms (labeled on the figure) of (from left to right) Ag, Au, and Cu NPs, reproduced with permission from ref. 154. | |
In the field of photocatalysis, SAAs have been investigated both as plasmonic catalysts and as co-catalysts for semiconductor materials. Addressing the role of promoters (Ag, Au, Cu, Pd, and Pt) in the plasmonic hot-carrier generation of Ag-, Au- and Cu-based SAAs, Sorvisto et al.154 modeled the plasmonic-catalytic properties of 201-atom octahedral NPs (having one promoter atom on a facet or corner site) using time-dependent DFT. Although the promoters did not affect the overall plasmonic response of the NPs, local changes were observed. By analyzing the hot-carrier distributions projected on the promoter sites, the localized promoter states were found to lead to peaked hot-hole distributions (Fig. 8B), with less impact on the distribution of hot electrons. The local hot-hole distributions were further found to change with the location of the promoter atoms on the NP (corner vs. terrace) and the NP size, when performing additional calculations on 309-atom Ag NPs (icosahedral and octahedral). Besides modifying local hot-carrier distributions, promoter atoms can facilitate elementary steps, boosting the overall plasmonic-catalyzed rates (i.e., “antenna-reactor” catalysts). For example, Lu et al.155 paired experiments with DFT calculations and unveiled the role of Co promoters in the activity of CoCu SAAs for the light-driven production of hydrogen from methanol and water.
Regarding the use of SAAs as co-catalysts, Wang et al.156 showed that AuAg SAAs enhanced the photo-oxidative coupling of methane into ethane over ZnO. Experiments demonstrated a higher activity of the SAA catalyst compared to Au and Ag NPs. Moreover, Au-loading tests revealed a uniquely high activity of isolated Au atoms in contrast to Au ensembles. DFT-calculated reaction energetics shed light on the synergy between the Ag host, relevant for *CH3 coupling, and the electron-deficient Au isolated atoms. The latter were found to facilitate the diffusion of *CH3 on the SAA NP, as well as the production of the *OOH intermediate, critical for the C–H activation of methane. Analogous work by Pan et al.157 developed a PdPt SAA co-catalyst for photocatalytic water splitting over UiO-66-NH2 metal–organic frameworks, exhibiting a higher H2 production rate compared to PdPt intermetallic alloys.
ML screening of novel catalysts
The increasing momentum in the development of ML is revolutionizing the screening of heterogeneous catalysts while holding the promise of dramatically reducing if not replacing the computationally intense DFT calculations in the future.158–160 In the field of SAAs, various ML regression models (mostly gradient-boosted trees) have been recently trained from DFT data to predict Eads and Eact with high accuracy.73,161–168 Nonetheless, the two obstacles hindering the screening of heterogeneous catalysts with ML are the limited amount of available training data and the high computational cost of generating the data.169,170 A solution to this can be found in active learning models, which identify the most informative data points to test (e.g., with select DFT calculations) and incorporate them into the model's training set, thereby iteratively improving the screening process.169,170
In this regard, Kayode et al.171 benchmarked the efficiency of Bayesian optimization in screening SAAs for alkane conversions and CO2RR, as well as bimetallic alloys for HER. In all cases, the Eads of activity descriptors were used as targets. Gaussian process regression was chosen for the surrogate model, used to identify (through an acquisition function) the promoter–host combinations with the highest improvements. After initializing the algorithm with a random set of catalysts, it iteratively tested and learned from promising candidates until an optimal Eads was found (or equivalently, an iteration threshold was reached). The algorithm identified HfCu SAA for alkane transformations, Y-promoted Ag, Cu, and Ag SAAs for CO2RR, and intermetallic AgIr for HER. Moreover, the algorithm was both efficient, requiring substantially fewer DFT calculations compared to random search, and robust, as variations in the features or the initial random sample resulted in similar numbers of iterations. A different study by Feng et al.172 also leveraged an active learning framework to screen across the (111), (100), (110), and (320) facets of Au, Ag, and Cu-based SAAs for the selective hydrogenation of crotonaldehyde into crotyl alcohol. The top performing TiAu(320) and (111) SAA surfaces were further investigated using mkm, corroborating their high selectivity and near 100% conversion.
Conclusion and outlook
DAs and SAAs have gained tremendous attention over the years due to their distinct properties, leading to enhanced catalytic performance. In this minireview, we reviewed how electronic structure, thermodynamics and kinetics affect the stability and catalytic behavior of DAs and SAAs using DFT, multi-scale modeling, and ML. Regarding their stability, we specifically highlighted that the promoter–adsorbate interactions and external fields can alter the segregation and aggregation behavior in SAAs. Additionally, we discussed how kinetics may impose different stability trends compared to thermodynamics leading to metastable structures. We then dived into recent applications of ML in predicting the stability of DAs and SAAs, highlighting the emergence of physicochemical descriptors that dictate the segregation and aggregation trends. Regarding catalytic applications we discussed how the activity of DAs and SAAs is determined by the NP morphology, promoter ensembles, and surface coordination. Furthermore, we presented thermo-, electro-, and photocatalytic applications, extending beyond the spillover hydrogenation reactions commonly investigated on SAAs. The integration of computational chemistry with ML has paved the way in the accelerated screening of DA and SAA catalysts. Looking forward, we present an outlook emphasizing on the importance of using transfer learning to accurately capture adsorbate configurations in SAAs and DAs and understanding the implications of dual atom alloys (DAAs) emerging from the presence of two different heteroatom promoters on a single host metal.
Improving ML predictions through accurate adsorbate configuration representations
Although great progress has been made in ML-predicted Eads in SAA and DA catalysts, solely relying on surface-level features can result in significant prediction errors due to the diverse binding configurations of adsorbates.162,163 In this regard, graph-based models offer an alternative solution by accurately representing adsorbate configurations, which can then be used to compute the energy and forces of a given state. These can then be fed into an optimization algorithm (e.g., BFGS173) to find the most stable adsorption configuration. However, given the limited configuration space for SAAs (compared to bimetallic catalysts of various compositions), training complex models directly on this data can be challenging. Recent progress in developing graph-based models for intermetallic catalysts, especially in transfer learning algorithms (in which the weights of a model trained on an analogous dataset are fine-tuned with new data) is promising to reduce the amount of training data needed.174 In this sense, the pre-trained weights, which capture general trends, can be fine-tuned to adapt to a new target.175 Such approaches have been used to predict materials properties using graph convolutional networks176 as well as XGBoost regression in bimetallic alloys (including SAAs).177 For instance, the EquiformerV2 model, trained on the Open Catalyst Project database,178 has been successfully extended to predict Eads of OH and O on high-entropy Ag–Ir–Pd–Pt–Ru alloys.179 Hence, we envision that similar extensions could be applied using other graph-based models within the context of SAA and DAs.
Dual-atom alloy catalysts and systems with isolated active sites
The great advancements made in understanding the behavior and applications of SAAs and DAs have paved the way for exploring more complex systems, such as DAAs (i.e., trimetallic systems where one metal acts as the host and the other two metals act as promoters). These systems provide additional tunability and unique properties due to the interactions between two different active sites,180 leading to enhanced performance in catalytic applications such as ethanol dehydrogenation181 and urea electrosynthesis from nitrate and carbon dioxide.182 However, they encounter great challenges in their synthesis.
Extending insights from SAAs to DAAs, Zhang, and coworkers180 employed DFT to reveal that while SAAs display free-atom-like electronic states, DAAs show hybridization of these states, forming molecular-like states. This hybridization occurs from the interaction of the promoter atoms in the DAA, resulting in new electronic properties that are absent in SAAs (the latter presented in Fig. 2A). In addition to DFT, MD simulations along with multi-scale modeling, such as mkm and kMC, have been used to examine the dynamic behavior of SAAs and DAs under various conditions such as temperature, pressure, and in the presence of adsorbates. These tools provide valuable insights into the mobility of the active sites, which in turn affects their catalytic performance. The use of ML, which has opened new avenues in accelerating the screening of SAA and DA catalysts by predicting properties such as Eseg and Eads, can further enhance the efficiency and accuracy of identifying promising DAA catalysts in terms of their stability and catalytic activity. As a final take-home message, the complexity of SAAs and DAs can increase by changing the number of different metal promoters and designing novel nanocatalyst architectures. However, one must be very careful with the material limit (e.g. metal composition, ensemble sizes, etc.) where these systems lose their unique electronic structure and start resembling the behavior of bimetallic and/or multimetallic systems. In this regard, simulation strategies for SAA and DA screening for catalytic applications (and beyond) should be developed on systems that exhibit these unique electronic properties and the latter should be integrated as critical information on optimizing the application performance.
Data availability
No primary research results, software or code have been included and no new data were generated or analysed as part of this review.
Conflicts of interest
There are no conflicts to declare.
Acknowledgements
This work was supported by the US Department of Defense, Office of Naval Research under the award number: N00014-21-1-2246. The authors acknowledge computational support from the Center for Research Computing at the University of Pittsburgh, RRID:SCR_022735, through the resources provided.
References
- Y. Nakaya and S. Furukawa, Chem. Rev., 2023, 123, 5859–5947 CrossRef CAS PubMed.
-
W. M. H. Sachtler and R. A. V. Santen, in Advances in Catalysis, ed. D. D. Eley, H. Pines and P. B. Weisz, Academic Press, 1977, vol. 26, pp. 69–119 Search PubMed.
- H. C. de Jongste and V. Ponec, J. Catal., 1980, 63, 389–394 CrossRef CAS.
- E. Iglesia, S. L. Soled, R. A. Fiato and G. H. Via, J. Catal., 1993, 143, 345–368 CrossRef CAS.
- J. D. Lee, J. B. Miller, A. V. Shneidman, L. Sun, J. F. Weaver, J. Aizenberg, J. Biener, J. A. Boscoboinik, A. C. Foucher, A. I. Frenkel, J. E. S. van der Hoeven, B. Kozinsky, N. Marcella, M. M. Montemore, H. T. Ngan, C. R. O'Connor, C. J. Owen, D. J. Stacchiola, E. A. Stach, R. J. Madix, P. Sautet and C. M. Friend, Chem. Rev., 2022, 122, 8758–8808 CrossRef CAS.
- M. Luneau, J. S. Lim, D. A. Patel, E. C. H. Sykes, C. M. Friend and P. Sautet, Chem. Rev., 2020, 120, 12834–12872 CrossRef CAS PubMed.
- H. L. Tierney, A. E. Baber, J. R. Kitchin and E. C. H. Sykes, Phys. Rev. Lett., 2009, 103, 246102 CrossRef.
- G. Kyriakou, M. B. Boucher, A. D. Jewell, E. A. Lewis, T. J. Lawton, A. E. Baber, H. L. Tierney, M. Flytzani-Stephanopoulos and E. C. H. Sykes, Science, 2012, 335, 1209–1212 CrossRef CAS PubMed.
- G. Giannakakis, M. Flytzani-Stephanopoulos and E. C. H. Sykes, Acc. Chem. Res., 2019, 52, 237–247 CrossRef CAS.
- R. T. Hannagan, G. Giannakakis, M. Flytzani-Stephanopoulos and E. C. H. Sykes, Chem. Rev., 2020, 120, 12044–12088 CrossRef CAS PubMed.
- M. T. Darby, M. Stamatakis, A. Michaelides and E. C. H. Sykes, J. Phys. Chem. Lett., 2018, 9, 5636–5646 CrossRef CAS PubMed.
- M. T. Darby, R. Réocreux, E. C. H. Sykes, A. Michaelides and M. Stamatakis, ACS Catal., 2018, 8, 5038–5050 CrossRef CAS.
- W. Li, S. E. Madan, R. Réocreux and M. Stamatakis, ACS Catal., 2023, 13, 15851–15868 CrossRef CAS.
- V. Pallassana and M. Neurock, J. Catal., 2000, 191, 301–317 CrossRef CAS.
- Z.-P. Liu and P. Hu, J. Chem. Phys., 2001, 114, 8244–8247 CrossRef CAS.
- A. Logadottir, T. H. Rod, J. K. Nørskov, B. Hammer, S. Dahl and C. J. H. Jacobsen, J. Catal., 2001, 197, 229–231 CrossRef CAS.
- T. Bligaard, J. K. Nørskov, S. Dahl, J. Matthiesen, C. H. Christensen and J. Sehested, J. Catal., 2004, 224, 206–217 CrossRef CAS.
- J. Greeley, Annu. Rev. Chem. Biomol. Eng., 2016, 7, 605–635 CrossRef PubMed.
- F. Abild-Pedersen, J. Greeley, F. Studt, J. Rossmeisl, T. R. Munter, P. G. Moses, E. Skúlason, T. Bligaard and J. K. Nørskov, Phys. Rev. Lett., 2007, 99, 016105 Search PubMed.
- M. M. Montemore and J. W. Medlin, Catal. Sci. Technol., 2014, 4, 3748–3761 Search PubMed.
- B. Hammer and J. K. Nørskov, Surf. Sci., 1995, 343, 211–220 CAS.
- B. Hammer and J. K. Norskov, Nature, 1995, 376, 238–240 CAS.
-
B. Hammer and J. K. Nørskov, in Advances in Catalysis, Academic Press, 2000, vol. 45, pp. 71–129 Search PubMed.
- K. G. Papanikolaou, M. T. Darby and M. Stamatakis, J. Phys. Chem. C, 2019, 123, 9128–9138 Search PubMed.
- Q. Fu and Y. Luo, J. Phys. Chem. C, 2013, 117, 14618–14624 Search PubMed.
- M. T. Darby, E. C. H. Sykes, A. Michaelides and M. Stamatakis, Top. Catal., 2018, 61, 428–438 Search PubMed.
- M. D. Marcinkowski, A. D. Jewell, M. Stamatakis, M. B. Boucher, E. A. Lewis, C. J. Murphy, G. Kyriakou and E. C. H. Sykes, Nat. Mater., 2013, 12, 523–528 CrossRef CAS PubMed.
- M. T. Darby, F. R. Lucci, M. D. Marcinkowski, A. J. Therrien, A. Michaelides, M. Stamatakis and E. C. H. Sykes, J. Phys. Chem. C, 2019, 123, 10419–10428 Search PubMed.
- P. Kress, R. Réocreux, R. Hannagan, T. Thuening, J. A. Boscoboinik, M. Stamatakis and E. C. H. Sykes, J. Chem. Phys., 2021, 154, 204701 CrossRef CAS PubMed.
- S. Simpson, JCIS Open, 2021, 3, 100013 Search PubMed.
- K. Gu, F. Wei, Y. Cai, S. Lin and H. Guo, J. Phys. Chem. Lett., 2021, 12, 8423–8429 Search PubMed.
- K. Gu, C. Li, B. Jiang, S. Lin and H. Guo, J. Phys. Chem. C, 2022, 126, 17093–17101 Search PubMed.
- K. Gu and S. Lin, Angew. Chem., Int. Ed., 2023, 62, e202312796 CAS.
- B. Geng, L. Zhang, F. Wang, X. Wang, S. Song and H. Zhang, Eur. J. Inorg. Chem., 2023, 26, e202300052 CrossRef CAS.
- J. Mao, J. Yin, J. Pei, D. Wang and Y. Li, Nano Today, 2020, 34, 100917 CrossRef CAS.
- T. Zhang, A. G. Walsh, J. Yu and P. Zhang, Chem. Soc. Rev., 2021, 50, 569–588 RSC.
- H. Thirumalai and J. R. Kitchin, Top. Catal., 2018, 61, 462–474 CrossRef CAS.
- M. T. Greiner, T. E. Jones, S. Beeg, L. Zwiener, M. Scherzer, F. Girgsdies, S. Piccinin, M. Armbrüster, A. Knop-Gericke and R. Schlögl, Nat. Chem., 2018, 10, 1008–1015 CrossRef CAS.
- C. Hartwig, K. Schweinar, T. E. Jones, S. Beeg, F.-P. Schmidt, R. Schlögl and M. Greiner, J. Chem. Phys., 2021, 154, 184703 CrossRef CAS PubMed.
- J. K. Nørskov, F. Abild-Pedersen, F. Studt and T. Bligaard, Proc. Natl. Acad. Sci. U. S. A., 2011, 108, 937–943 CrossRef.
- L. G. M. Pettersson and A. Nilsson, Top. Catal., 2014, 57, 2–13 Search PubMed.
- F. R. Lucci, J. Liu, M. D. Marcinkowski, M. Yang, L. F. Allard, M. Flytzani-Stephanopoulos and E. C. H. Sykes, Nat. Commun., 2015, 6, 8550 Search PubMed.
- M. J. Islam, M. Granollers Mesa, A. Osatiashtiani, J. C. Manayil, M. A. Isaacs, M. J. Taylor, S. Tsatsos and G. Kyriakou, Appl. Catal., B, 2021, 299, 120652 Search PubMed.
- T. D. Spivey and A. Holewinski, J. Am. Chem. Soc., 2021, 143, 11897–11902 Search PubMed.
- H. T. Ngan and P. Sautet, J. Am. Chem. Soc., 2024, 146, 2556–2567 CAS.
- V. Fung, G. Hu and B. Sumpter, J. Mater. Chem. A, 2020, 8, 6057–6066 CAS.
- A. P. Monasterial, C. A. Hinderks, S. Viriyavaree and M. M. Montemore, J. Chem. Phys., 2020, 153, 111102 CAS.
- K. G. Papanikolaou and M. Stamatakis, Catal. Sci. Technol., 2020, 10, 5815–5828 Search PubMed.
- T. Dai, Z. Wang, X. Lang and Q. Jiang, J. Mater. Chem. A, 2022, 10, 16900–16907 Search PubMed.
- J. Schumann, M. Stamatakis, A. Michaelides and R. Réocreux, Nat. Chem., 2024, 16, 749–754 CAS.
- J. Schumann, Y. Bao, R. T. Hannagan, E. C. H. Sykes, M. Stamatakis and A. Michaelides, J. Phys. Chem. Lett., 2021, 12, 10060–10067 CAS.
- R. Réocreux, E. C. H. Sykes, A. Michaelides and M. Stamatakis, J. Phys. Chem. Lett., 2022, 13, 7314–7319 Search PubMed.
- R. T. Hannagan, H. Y. Lam, R. Réocreux, Y. Wang, A. Dunbar, V. Lal, V. Çınar, Y. Chen, P. Deshlahra, M. Stamatakis, N. M. Eagan and E. C. H. Sykes, J. Phys. Chem. Lett., 2023, 14, 10561–10569 CAS.
- L. Farsi and N. A. Deskins, Phys. Chem. Chem. Phys., 2019, 21, 23626–23637 CAS.
- Y. Xu, G. Wang, P. Qian and Y. Su, J. Mater. Sci., 2022, 57, 7384–7399 CrossRef CAS.
- K. G. Papanikolaou, M. T. Darby and M. Stamatakis, ACS Catal., 2020, 10, 1224–1236 CrossRef CAS.
- Z. Yan, M. G. Taylor, A. Mascareno and G. Mpourmpakis, Nano Lett., 2018, 18, 2696–2704 CrossRef CAS PubMed.
- J. Yin, M. Ehara and S. Sakaki, Phys. Chem. Chem. Phys., 2022, 24, 10420–10438 RSC.
- P. Ekborg-Tanner and P. Erhart, J. Phys. Chem. C, 2021, 125, 17248–17260 CrossRef CAS.
- Q. Wang, B. Zhu, F. Tielens, D. Tichit and H. Guesmi, Appl. Surf. Sci., 2021, 548, 149217 CrossRef CAS.
- Y. Yu, Z. Liu, W. Huang, S. Zhou, Z. Hu and L. Wang, J. Phys. Chem. Solids, 2022, 171, 111021 CrossRef CAS.
- M. Ouyang, K. G. Papanikolaou, A. Boubnov, A. S. Hoffman, G. Giannakakis, S. R. Bare, M. Stamatakis, M. Flytzani-Stephanopoulos and E. C. H. Sykes, Nat. Commun., 2021, 12, 1549 CrossRef CAS PubMed.
-
G. Agrahari, A. Sarkar and A. Chatterjee, Effect of Solvent on Segregation Behavior in Pt-Ni Bimetallic Alloy, Singapore, 2020 Search PubMed.
- W. Li, M. G. Taylor, D. Bayerl, S. Mozaffari, M. Dixit, S. Ivanov, S. Seifert, B. Lee, N. Shanaiah, Y. Lu, L. Kovarik, G. Mpourmpakis and A. M. Karim, Nanoscale, 2021, 13, 206–217 RSC.
- S. V. Doronin, Mendeleev Commun., 2020, 30, 288–290 CrossRef CAS.
- J. S. Jirkovsky, I. Panas, S. Romani, E. Ahlberg and D. J. Schiffrin, J. Phys. Chem. Lett., 2012, 3, 315–321 CrossRef CAS.
- S. E. Weitzner, S. A. Akhade, A. R. Kashi, Z. Qi, A. K. Buckley, Z. Huo, S. Ma, M. Biener, B. C. Wood, K. P. Kuhl, J. B. Varley and J. Biener, J. Chem. Phys., 2021, 155, 114702 CrossRef CAS PubMed.
- K. K. Rao, Q. K. Do, K. Pham, D. Maiti and L. C. Grabow, Top. Catal., 2020, 63, 728–741 CAS.
- D. Ologunagba and S. Kattel, Energies, 2020, 13, 2182 CAS.
- D. Shin, G. Choi, C. Hong and J. W. Han, Mol. Catal., 2023, 541, 113096 CrossRef CAS.
- G. A. Sulley, J. Hamm and M. M. Montemore, JPhys Energy, 2023, 5, 015002 CrossRef CAS.
- Z. Lu, S. Yadav and C. V. Singh, Catal. Sci. Technol., 2020, 10, 86–98 CAS.
- Z.-K. Han, D. Sarker, R. Ouyang, A. Mazheika, Y. Gao and S. V. Levchenko, Nat. Commun., 2021, 12, 1833 CAS.
- A. N. P. Hipolito, M. A. Palmero, V. Z. Ordillo, K. Shimizu, D. B. Putungan, A. B. Santos-Putungan, J. D. Ocon, S. Watanabe, K. E. S. Pilario and A. A. B. Padama, Comput. Mater. Sci., 2024, 232, 112607 CrossRef CAS.
- M. Salem, D. J. Loevlie and G. Mpourmpakis, J. Phys. Chem. C, 2023, 127, 22790–22798 CrossRef CAS.
- M. Salem, M. J. Cowan and G. Mpourmpakis, ACS Omega, 2022, 7, 4471–4481 CrossRef CAS PubMed.
- A. Ghosh, S. Datta and T. Saha-Dasgupta, J. Phys. Chem. C, 2022, 126, 6847–6853 CrossRef CAS.
- S. Han, G. Barcaro, A. Fortunelli, S. Lysgaard, T. Vegge and H. A. Hansen, npj Comput. Mater., 2022, 8, 121 Search PubMed.
- Y. Yang, Z. Zhao, J. Zhu and D. Cheng, J. Cluster Sci., 2020, 31, 609–614 CAS.
- J. Dean, M. J. Cowan, J. Estes, M. Ramadan and G. Mpourmpakis, ACS Nano, 2020, 14, 8171–8180 CAS.
- D. J. Loevlie, B. Ferreira and G. Mpourmpakis, Acc. Chem. Res., 2023, 56, 248–257 Search PubMed.
- J. Jung, S. Ju, P.-H. Kim, D. Hong, W. Jeong, J. Lee, S. Han and S. Kang, ACS Catal., 2023, 13, 16078–16087 CAS.
- A. Front and C. Mottet, Theor. Chem. Acc., 2021, 141, 2 Search PubMed.
- M. Hua, X. Tian, S. Li, A. Shao and X. Lin, Phys. Chem. Chem. Phys., 2022, 24, 19488–19501 Search PubMed.
- K. Yun, H.-S. Nam and S. Kim, Phys. Chem. Chem. Phys., 2020, 22, 7787–7793 RSC.
- M. Hua, X. Tian, S. Li, X. Zhang, A. Shao, L. Song and X. Lin, Phys. Chem. Chem. Phys., 2022, 24, 2251–2264 RSC.
- N. V. Ter-Oganessian, Y. V. Rusalev, A. V. Motseyko, A. A. Guda and A. V. Soldatov, J. Phys. Chem. C, 2024, 128, 3054–3063 CrossRef CAS.
- F. R. Lucci, T. J. Lawton, A. Pronschinske and E. C. H. Sykes, J. Phys. Chem. C, 2014, 118, 3015–3022 CrossRef CAS.
- D. A. Patel, P. L. Kress, L. A. Cramer, A. M. Larson and E. C. H. Sykes, J. Chem. Phys., 2019, 151, 164705 CrossRef PubMed.
- Y. Wang, K. G. Papanikolaou, R. T. Hannagan, D. A. Patel, T. A. Balema, L. A. Cramer, P. L. Kress, M. Stamatakis and E. C. H. Sykes, J. Chem. Phys., 2020, 153, 244702 CrossRef CAS PubMed.
- H. Zhang, L. Lu, K. Kawashima, M. Okumura, M. Haruta and N. Toshima, Adv. Mater., 2015, 27, 1383–1388 CrossRef CAS PubMed.
- Y.-R. Wang, Q. Zhuang, R. Cao, Y. Li, F.-Y. Gao, Z.-R. Li, Z. He, L. Shi, Y.-F. Meng, X. Li, J.-L. Wang, Y. Duan, M.-R. Gao, X. Zheng and S.-H. Yu, Nano Lett., 2022, 22, 4232–4239 CAS.
- R. B. Garza, J. Lee, M. H. Nguyen, A. Garmon, D. Perez, M. Li, J. C. Yang, G. Henkelman and W. A. Saidi, J. Chem. Theory Comput., 2022, 18, 4447–4455 CrossRef CAS PubMed.
- C. Zhou, H. T. Ngan, J. S. Lim, Z. Darbari, A. Lewandowski, D. J. Stacchiola, B. Kozinsky, P. Sautet and J. A. Boscoboinik, J. Am. Chem. Soc., 2022, 144, 15132–15142 CAS.
- X. Liu, C. Ao, X. Shen, L. Wang, S. Wang, L. Cao, W. Zhang, J. Dong, J. Bao, T. Ding, L. Zhang and T. Yao, Nano Lett., 2020, 20, 8319–8325 CAS.
- L. Jiang, K. Liu, S.-F. Hung, L. Zhou, R. Qin, Q. Zhang, P. Liu, L. Gu, H. M. Chen, G. Fu and N. Zheng, Nat. Nanotechnol., 2020, 15, 848–853 CAS.
- R. Cheula, M. Maestri and G. Mpourmpakis, ACS Catal., 2020, 10, 6149–6158 CAS.
- M. Jørgensen and H. Grönbeck, ACS Catal., 2019, 9, 8872–8881 CrossRef.
- M. Jørgensen and H. Grönbeck, J. Am. Chem. Soc., 2019, 141, 8541–8549 CrossRef.
- R. J. Bunting, F. Wodaczek, T. Torabi and B. Cheng, J. Am. Chem. Soc., 2023, 145, 14894–14902 CrossRef CAS.
- R. T. Hannagan, G. Giannakakis, R. Réocreux, J. Schumann, J. Finzel, Y. Wang, A. Michaelides, P. Deshlahra, P. Christopher, M. Flytzani-Stephanopoulos, M. Stamatakis and E. C. H. Sykes, Science, 2021, 372, 1444–1447 CrossRef CAS.
- R. Réocreux, P. L. Kress, R. T. Hannagan, V. Çınar, M. Stamatakis and E. C. H. Sykes, J. Phys. Chem. Lett., 2020, 11, 8751–8757 CrossRef.
- J. E. S. van der Hoeven, H. T. Ngan, G. Yan, J. Aizenberg, R. J. Madix, P. Sautet and C. M. Friend, J. Phys. Chem. C, 2022, 126, 15710–15723 CrossRef CAS.
- J. D. Lee, Z. Qi, A. C. Foucher, H. T. Ngan, K. Dennis, J. Cui, I. I. Sadykov, E. J. Crumlin, P. Sautet, E. A. Stach, C. M. Friend, R. J. Madix and J. Biener, J. Am. Chem. Soc., 2022, 144, 16778–16791 CrossRef CAS.
- D. A. Patel, G. Giannakakis, G. Yan, H. T. Ngan, P. Yu, R. T. Hannagan, P. L. Kress, J. Shan, P. Deshlahra, P. Sautet and E. C. H. Sykes, ACS Catal., 2023, 13, 4290–4303 CrossRef CAS.
- G. Meng, J. Sun, L. Tao, K. Ji, P. Wang, Y. Wang, X. Sun, T. Cui, S. Du, J. Chen, D. Wang and Y. Li, ACS Catal., 2021, 11, 1886–1896 Search PubMed.
- R. Liu, J. N. El Berch, S. House, S. W. Meil, G. Mpourmpakis and M. D. Porosoff, ACS Catal., 2023, 13, 2449–2461 Search PubMed.
- J. Zhao, J. Liu, Z. Li, K. Wang, R. Shi, P. Wang, Q. Wang, G. I. N. Waterhouse, X. Wen and T. Zhang, Nat. Commun., 2023, 14, 1909 Search PubMed.
- H. Schulz, Top. Catal., 2003, 26, 73–85 Search PubMed.
-
O. C. Anika, S. G. Nnabuife, A. Bello, E. R. Okoroafor, B. Kuang and R. Villa, Carbon Capture Science & Technology, 2022, vol. 5, p. 100072 Search PubMed.
- M. Juneau, D. Yaffe, R. Liu, J. N. Agwara and M. D. Porosoff, Nanoscale, 2022, 14, 16458–16466 Search PubMed.
- M. Dixit, X. Peng, M. D. Porosoff, H. D. Willauer and G. Mpourmpakis, Catal. Sci. Technol., 2017, 7, 5521–5529 Search PubMed.
- M. D. Porosoff, J. W. Baldwin, X. Peng, G. Mpourmpakis and H. D. Willauer, ChemSusChem, 2017, 10, 2408–2415 CrossRef CAS.
- M. Juneau, M. Vonglis, J. Hartvigsen, L. Frost, D. Bayerl, M. Dixit, G. Mpourmpakis, J. R. Morse, J. W. Baldwin, H. D. Willauer and M. D. Porosoff, Energy Environ. Sci., 2020, 13, 2524–2539 Search PubMed.
- R. Liu, D. Leshchev, E. Stavitski, M. Juneau, J. N. Agwara and M. D. Porosoff, Appl. Catal., B, 2021, 284, 119787 Search PubMed.
- R. Liu, Z. Ma, J. D. Sears, M. Juneau, M. L. Neidig and M. D. Porosoff, J. CO2 Util., 2020, 41, 101290 CrossRef CAS.
- S. Kim, J. Lauterbach and E. Sasmaz, ACS Catal., 2021, 11, 8247–8260 CrossRef CAS.
- S. Kim and E. Sasmaz, ChemCatChem, 2022, 14, e202200568 CrossRef CAS.
- S. Guan, Z. Yuan, Z. Zhuang, H. Zhang, H. Wen, Y. Fan, B. Li, D. Wang and B. Liu, Angew. Chem., Int. Ed., 2024, 63, e202316550 CrossRef CAS.
- T. Han, Y. Li, T. Wu, D. M. Meira, S. Xiang, Y. Cao, I. Lee, X.-G. Zhou, D.-E. Jiang, A. I. Frenkel and F. Zaera, ACS Catal., 2024, 14, 7157–7165 CrossRef CAS.
- J. Liu, J. Shan, F. R. Lucci, S. Cao, E. C. H. Sykes and M. Flytzani-Stephanopoulos, Catal. Sci. Technol., 2017, 7, 4276–4284 RSC.
- M. Luneau, T. Shirman, A. C. Foucher, K. Duanmu, D. M. A. Verbart, P. Sautet, E. A. Stach, J. Aizenberg, R. J. Madix and C. M. Friend, ACS Catal., 2020, 10, 441–450 CrossRef CAS.
- M. Luneau, E. Guan, W. Chen, A. C. Foucher, N. Marcella, T. Shirman, D. M. A. Verbart, J. Aizenberg, M. Aizenberg, E. A. Stach, R. J. Madix, A. I. Frenkel and C. M. Friend, Commun. Chem., 2020, 3, 46 CrossRef PubMed.
- A. C. Foucher, H. T. Ngan, T. Shirman, A. Filie, K. Duanmu, M. Aizenberg, R. J. Madix, C. M. Friend, J. Aizenberg, P. Sautet and E. A. Stach, ACS Appl. Nano Mater., 2023, 6, 22927–22938 Search PubMed.
- S. K. Kaiser, J. E. S. van der Hoeven, G. Yan, K. R. G. Lim, H. T. Ngan, S. Garg, M. Karatok, M. Aizenberg, J. Aizenberg, P. Sautet, C. M. Friend and R. J. Madix, ACS Catal., 2023, 13, 12092–12103 Search PubMed.
- G. Giannakakis, P. Kress, K. Duanmu, H. T. Ngan, G. Yan, A. S. Hoffman, Z. Qi, A. Trimpalis, L. Annamalai, M. Ouyang, J. Liu, N. Eagan, J. Biener, D. Sokaras, M. Flytzani-Stephanopoulos, S. R. Bare, P. Sautet and E. C. H. Sykes, J. Am. Chem. Soc., 2021, 143, 21567–21579 CrossRef CAS PubMed.
- K. G. Papanikolaou and M. Stamatakis, Catal. Sci. Technol., 2021, 11, 3681–3696 RSC.
- J. Shi, H. T. Ngan, P. Sautet and J. F. Weaver, ACS Catal., 2023, 13, 11244–11255 Search PubMed.
- L. Chen, Y. Shi, C. Chen, H. Feng, S. Wang, X. Zhang and M. Wei, J. Phys. Chem. C, 2022, 126, 4319–4328 CrossRef CAS.
- R. Réocreux and M. Stamatakis, Acc. Chem. Res., 2022, 55, 87–97 CrossRef PubMed.
- Y. Zhang, S. Li, C. Sun, P. Wang, Y. Yang, D. Yi, X. Wang and J. Yao, ACS Catal., 2022, 12, 9201–9212 CrossRef CAS.
- S. Liu, N. Govindarajan and K. Chan, ACS Catal., 2022, 12, 12902–12910 CrossRef CAS.
- Y. Zhang, B. Wang, M. Fan, D. Li and R. Zhang, Fuel, 2021, 306, 121641 CrossRef CAS.
- S. Chen, J. Zaffran and B. Yang, ACS Catal., 2020, 10, 3074–3083 CrossRef CAS.
- R. J. Bunting, P. S. Rice, Z. Yao, J. Thompson and P. Hu, Chem. Commun., 2022, 58, 9622–9625 RSC.
- R. Liu and W. An, Catal. Sci. Technol., 2021, 11, 7047–7059 Search PubMed.
- S. AlAreeqi, D. Bahamon, K. Polychronopoulou and L. F. Vega, Fuel Process. Technol., 2024, 253, 108001 Search PubMed.
- R. Xia, S. Overa and F. Jiao, JACS Au, 2022, 2, 1054–1070 CAS.
- K. M. Van Geem and B. M. Weckhuysen, MRS Bull., 2021, 46, 1187–1196 CrossRef.
- Q. Gao, X. Han, Y. Liu and H. Zhu, ACS Catal., 2024, 14, 6045–6061 CrossRef CAS.
- Y. Da, R. Jiang, Z. Tian, X. Han, W. Chen and W. Hu, SmartMat, 2023, 4, e1136 Search PubMed.
- J. K. Nørskov, J. Rossmeisl, A. Logadottir, L. Lindqvist, J. R. Kitchin, T. Bligaard and H. Jónsson, J. Phys. Chem. B, 2004, 108, 17886–17892 CrossRef.
- D. R. Alfonso, D. N. Tafen and D. R. Kauffmann, Catalysts, 2018, 8, 424 Search PubMed.
- S. Nitopi, E. Bertheussen, S. B. Scott, X. Liu, A. K. Engstfeld, S. Horch, B. Seger, I. E. L. Stephens, K. Chan, C. Hahn, J. K. Nørskov, T. F. Jaramillo and I. Chorkendorff, Chem. Rev., 2019, 119, 7610–7672 Search PubMed.
- X. Zhi, Y. Jiao, Y. Zheng, A. Vasileff and S.-Z. Qiao, Nano Energy, 2020, 71, 104601 Search PubMed.
- J.-C. Jiang, J.-C. Chen, M.-D. Zhao, Q. Yu, Y.-G. Wang and J. Li, Nano Res., 2022, 15, 7116–7123 Search PubMed.
- J. Wang, S. Li, Q. Liu, K. Zhao, Y. Yang and X. Wang, ACS Appl. Mater. Interfaces, 2023, 15, 53436–53445 Search PubMed.
- C. Du, S. Lu, J.-A. Wang, X. Wang, M. Wang, H. M. Fruehwald, L. Wang, B. Zhang, T. Guo, J. P. Mills, W. Wei, Z. Chen, Y. Teng, J. Zhang, C.-J. Sun, H. Zhou, R. D. L. Smith, B. Kendall, G. Henkelman and Y. A. Wu, ACS Catal., 2023, 13, 10560–10569 CrossRef CAS.
- K. Chen, J. Xiang, Y. Guo, X. Liu, X. Li and K. Chu, Nano Lett., 2024, 24, 541–548 CrossRef CAS.
- L. Chen, X. Liang, D. Wang, Z. Yang, C.-T. He, W. Zhao, J. Pei and Y. Xue, ACS Appl. Mater. Interfaces, 2022, 14, 27814–27822 CrossRef CAS.
- J. Mao, C.-T. He, J. Pei, Y. Liu, J. Li, W. Chen, D. He, D. Wang and Y. Li, Nano Lett., 2020, 20, 3442–3448 CrossRef CAS.
- M. T. Darby and M. Stamatakis, ChemPhysChem, 2021, 22, 499–508 CrossRef CAS.
- E. Zhang, A. Dong, K. Yin, C. Ye, Y. Zhou, C. Tan, M. Li, X. Zheng, Y. Wang, X. Gao, H. Li, D. Wang and S. Guo, J. Am. Chem. Soc., 2024, 146, 2339–2344 CrossRef CAS.
- D. Sorvisto, P. Rinke and T. P. Rossi, J. Phys. Chem. C, 2023, 127, 8585–8590 CrossRef CAS.
- W.-N. Lu, S. Luo, Y. Zhao, J. Xu, G. Yang, E. Picheau, M. Han, Q. Wang, S. Li, L. Jia, M.-X. Ling, T. Kako and J. Ye, Appl. Catal., B, 2024, 343, 123520 CrossRef CAS.
- Y. Wang, G. Hong, Y. Zhang, Y. Liu, W. Cen, L. Wang and Z. Wu, Angew. Chem., Int. Ed., 2023, 62, e202310525 CrossRef CAS PubMed.
- Y. Pan, Y. Qian, X. Zheng, S.-Q. Chu, Y. Yang, C. Ding, X. Wang, S.-H. Yu and H.-L. Jiang, Natl. Sci. Rev., 2021, 8, nwaa224 CrossRef CAS PubMed.
- S. Ma and Z.-P. Liu, ACS Catal., 2020, 10, 13213–13226 CrossRef CAS.
- P. S. Lamoureux, K. T. Winther, J. A. Garrido Torres, V. Streibel, M. Zhao, M. Bajdich, F. Abild-Pedersen and T. Bligaard, ChemCatChem, 2019, 11, 3581–3601 CrossRef.
- J. R. Kitchin, Nat. Catal., 2018, 1, 230–232 CrossRef.
- H. Feng, H. Ding, S. Wang, Y. Liang, Y. Deng, Y. Yang, M. Wei and X. Zhang, ACS Appl. Mater. Interfaces, 2022, 14, 25288–25296 CrossRef CAS.
- J. Shirani, H. D. M. Pham, S. Yuan, A. B. Tchagang, J. J. Valdés and K. H. Bevan, J. Phys. Chem. C, 2023, 127, 12055–12067 CrossRef CAS.
- J. Shirani, J. J. Valdes, A. B. Tchagang and K. H. Bevan, J. Phys. Chem. C, 2024, 128, 4483–4496 CrossRef CAS.
- D. Wang, R. Cao, S. Hao, C. Liang, G. Chen, P. Chen, Y. Li and X. Zou, Green Energy Environ., 2023, 8, 820–830 CrossRef CAS.
- X. Zong and D. G. Vlachos, J. Chem. Inf. Model., 2022, 62, 4361–4368 CrossRef CAS.
- A. Kumar, J. Iyer, F. Jalid, M. Ramteke, T. S. Khan and M. A. Haider, ChemCatChem, 2022, 14, e202101481 CrossRef CAS.
- S. Saxena, T. S. Khan, F. Jalid, M. Ramteke and M. A. Haider, J. Mater. Chem. A, 2020, 8, 107–123 RSC.
- S. Sun, G. Sun, C. Pei, Z.-J. Zhao and J. Gong, J. Phys. Chem. C, 2021, 125, 18708–18716 CrossRef CAS.
- T. Mou, H. S. Pillai, S. Wang, M. Wan, X. Han, N. M. Schweitzer, F. Che and H. Xin, Nat. Catal., 2023, 6, 122–136 CrossRef.
- J. A. Esterhuizen, B. R. Goldsmith and S. Linic, Nat. Catal., 2022, 5, 175–184 CrossRef.
- G. O. Kayode, A. F. Hill and M. M. Montemore, J. Mater. Chem. A, 2023, 11, 19128–19137 RSC.
- H. Feng, M. Zhang, Z. Ge, Y. Deng, P. Pu, W. Zhou, H. Yuan, J. Yang, F. Li, X. Zhang and Y.-W. Zhang, ACS Appl. Mater. Interfaces, 2023, 15, 55903–55915 CrossRef CAS.
- J. D. Head and M. C. Zerner, Chem. Phys. Lett., 1985, 122, 264–270 CrossRef CAS.
- L. Yang, S. Hanneke and J. Carbonell, Mach. Learn., 2013, 90, 161–189 Search PubMed.
-
A. Ramdan, A. Heryana, A. Arisal, R. B. S. Kusumo and H. F. Pardede, Transfer Learning and Fine-Tuning for Deep Learning-Based Tea Diseases Detection on Small Datasets, 2020 International Conference on Radar, Antenna, Microwave, Electronics, and Telecommunications (ICRAMET), Tangerang, Indonesia, 2020, pp. 206–211, DOI:10.1109/ICRAMET51080.2020.9298575.
- C. Chen and S. P. Ong, npj Comput. Mater., 2021, 7, 173 Search PubMed.
- X. Li, B. Li, Z. Yang, Z. Chen, W. Gao and Q. Jiang, J. Mater. Chem. A, 2022, 10, 872–880 RSC.
- L. Chanussot, A. Das, S. Goyal, T. Lavril, M. Shuaibi, M. Riviere, K. Tran, J. Heras-Domingo, C. Ho, W. Hu, A. Palizhati, A. Sriram, B. Wood, J. Yoon, D. Parikh, C. L. Zitnick and Z. Ulissi, ACS Catal., 2021, 11, 6059–6072 CAS.
- C. M. Clausen, J. Rossmeisl and Z. W. Ulissi, J. Phys. Chem. C, 2024, 128, 11190–11195 CAS.
- S. Zhang, E. C. H. Sykes and M. M. Montemore, Chem. Sci., 2022, 13, 14070–14079 RSC.
- P. L. Kress, S. Zhang, Y. Wang, V. Çınar, C. M. Friend, E. C. H. Sykes and M. M. Montemore, J. Am. Chem. Soc., 2023, 145, 8401–8407 CrossRef CAS PubMed.
- K. Chen, D. Ma, Y. Zhang, F. Wang, X. Yang, X. Wang, H. Zhang, X. Liu, R. Bao and K. Chu, Adv. Mater., 2024, 36, 2402160 CrossRef CAS.
|
This journal is © The Royal Society of Chemistry 2025 |
Click here to see how this site uses Cookies. View our privacy policy here.