DOI:
10.1039/D4SC05055J
(Edge Article)
Chem. Sci., 2025,
16, 3187-3197
Multi-dimensional bio mass cytometry: simultaneous analysis of cytoplasmic proteins and metabolites on single cells†
Received
29th July 2024
, Accepted 7th January 2025
First published on 8th January 2025
Abstract
Single-cell multi-dimensional analysis enables more profound biological insight, providing a comprehensive understanding of cell physiological processes. Due to limited cellular contents, the lack of protein and metabolite amplification ability, and the complex cytoplasmic environment, the simultaneous analysis of intracellular proteins and metabolites remains challenging. Herein, we proposed a multi-dimensional bio mass cytometry platform characterized by protein signal conversion and amplification through an orthogonal exogenous enzymatic reaction. Clustered regularly interspaced short palindromic repeats (CRISPR)/CRISPR-associated protein 9 (Cas9) gene editing technology was applied in the quantification of endogenous intracellular protein glycer-aldehyde-3-phosphate dehydrogenase (GAPDH) through exogenous luciferase Nanoluc (Nluc). The simultaneous detection of GAPDH and hundreds of metabolites at the single-cell level was realized for the first time. Semiquantitative analysis of GAPDH together with single-cell metabolomes under S-nitrosoglutathione (GSNO)-induced oxidative stress was investigated. Bioinformatics analysis revealed 16 metabolites that correlated positively with GAPDH expression upon oxidative stress, including long-chain fatty acids (palmitoleic acid, myristic acid, etc.) and UDP-N-acetylglucosamine (UDP-GlcNAc). Potential synergetic functions of GAPDH and UDP-GlcNAc-mediated oxidative stress responses were also elucidated. Our work proposes a novel strategy for the simultaneous quantitative analysis of single-cell intracellular proteins and metabolites, deepens the understanding of inherent anti-oxidative stress response mechanisms, and provides the molecular fundamentals for the study of inherent biological processes.
Introduction
Single-cell multi-dimensional analysis integrates various molecular data, such as epigenetics,1,2 chromatin conformation,3,4 protein expression,5 and metabolome information,6 providing insights into the intricate biological processes in cells. It unravels cellular events at the single-cell level, facilitating a comprehension of individual cell heterogeneity and spatiotemporal dynamics.7,8 In recent years, nucleic acid amplification-based single-cell conjoint analysis technologies, including genomics, transcriptomics, and epigenomics, have exhibited promising advancements towards maturity.9,10 However, it should be noted that gene information primarily reflects probabilities of disease susceptibility, whereas proteins and metabolites serve as direct indicators of vital activities and phenotypes,11 thereby offering more accurate reflections of pathological processes in diseases and cellular states.12,13 Nevertheless, the detection of metabolites and proteins at the single-cell level remains challenging due to their large diversity, low abundance, rapid turnover rates, wide dynamic ranges, and lack of amplification capabilities within current single-cell analysis platforms.14,15 Consequently, there is an urgent need for analytical techniques with high sensitivity and rapid response capabilities. Fluorescence-based flow cytometry has enabled multiplexed protein analysis in single cells with high throughput,16 and surface-competitive immunoassays have also been utilized in the quantification of proteins and specific metabolites.6,17,18 However, time-consuming antibody labeling and cell pretreatment have caused an impact on the real physiological state of cells, which may directly affect the information of endogenous metabolites. Due to its high resolution, sensitivity, and throughput, mass spectrometry is flourishing in single-cell proteomics and metabolomics.19,20 The parallel analysis of proteins and metabolites has been shown to be feasible for cells such as embryos,21,22 neurons,23 and tumor cells.24 Although such techniques enable high-throughput analysis of proteins and metabolites, targeted low-abundant protein analysis is still challenging. The complex micromanipulation technologies and sample preprocessing workflows are required, resulting in a relatively low cell analysis throughput. In addition, the spatiotemporal separation may cause the alteration of proteins and metabolites.25,26 Therefore, simultaneous analysis of proteins and metabolites in somatic cells is critical to obtain accurate and comprehensive cellular phenotypic understanding. Insufficient ionization efficiency of proteins puts forward a considerable challenge for direct protein quantification strategies. Our group has developed a multi-dimensional organic mass cytometry platform,14 realizing the detection of six cell surface proteins along with endogenous metabolites through immune-based MS signal amplification. In comparison to cell surface proteins, cytoplasmic proteins constitute approximately 70% of the cellular proteome and play a dominant role in fundamental cellular activities.27 Nevertheless, the current methods are limited in the cytoplasm because of the complex cellular environment that presents intricate redox circumstances and diverse chemical reactivity. Furthermore, the amplification capability is also restricted by the limited loading capacity of mass tags. Therefore, there is an urgent need for detection methods that exhibit excellent biological orthogonality and high sensitivity for cytoplasmic proteins and endogenous metabolites at single-cell resolution.
Glyceraldehyde-3-phosphate dehydrogenase (GAPDH), regarded as a moonlighting protein, has exhibited significant function in not only the classical glycolysis but also in the response to oxidative stress process.28–30 Under oxidative stress conditions, various oxidative posttranslational modifications (oxPTMs), such as sulfenylation and S-thiolation, induce alterations in GAPDH's conformation, subcellular localization, and interactions with multiple metabolic pathways.31,32 However, detailed metabolic change accompanied by GAPDH alteration at the single-cell level still remains unknown, and further exploration is needed to understand the synergistic regulation among numerous metabolic pathways in response to external oxidative stimulation.
Herein, we proposed a multi-dimensional bio mass cytometry approach to achieve simultaneous analysis of cytoplasmic protein GAPDH and hundreds of metabolites at the single-cell level. As illustrated in Scheme 1, the in vitro protein quantification is demonstrated by the fusion protein GAPDH-Nanoluc (GAPDH-Nluc) using the amount of product generated from substrate through monitoring the kinetics of the enzymatic reaction of Nluc, a luciferase that spontaneously emits light upon incubation with its substrate luciferin. To further characterize the expression of endogenous GAPDH, the clustered regularly interspaced short palindromic repeats (CRISPR)/CRISPR-associated protein 9 (Cas9) gene editing system33 is utilized to construct a GAPDH-Nluc cell line. Combined with the rapid enzyme reaction, the level of GAPDH in cells can be reflected by the product measured. The intracellular protein and the endogenous metabolites can be detected simultaneously within individual cells. Based on the multi-dimensional information, changes in GAPDH and metabolites under oxidative stress induced by S-nitrosoglutathione (GSNO) and probably synergistic effects among GAPDH and specific metabolic pathways were also investigated.
 |
| Scheme 1 The workflow of multi-dimensional bio mass cytometry. (a) In vitro validation. Through an Nluc fusion protein-mediated enzymatic reaction, the amount of product was positively correlated with the concentration of enzyme. (b) CRISPR-based Nluc knock-in cell line construction. Cas9, template DNAs, and sgRNA plasmids were transfected, and then genotype validation was performed to ensure the successful knock-in of Nluc. Through monoclonal sorting and following phenotypic verification, an Nluc knock-in cell line was obtained. (c) Single-cell multi-dimensional analysis. Metabolites and target proteins were detected simultaneously using single-cell mass cytometry. (d) GSNO oxidation-stress analysis. GSNO oxidation stress application was further studied with the help of bioinformatics tools. | |
Results and discussion
In vitro validation
In order to validate the quantification capability of the strategy, we conducted in vitro enzyme activity experiments. Firstly, the active fusion protein GAPDH-Nluc was successfully expressed and purified based on MS identification and SDS-PAGE results (Fig. S1†). Subsequently, a non-contact electrospray MS device other than conventional contact mode was opted for subsequent enzyme kinetics analysis (Fig. S2†), considering factors such as salt tolerance of existing ionization methods, probability of cell blockage, and compatibility with flow cytometric devices (Fig. S2 and S3†). A Cu wire was enwound at the spray needle to establish electrical contact with the MS built-in electricity supply system (Fig. S2†). Fig. 1a demonstrates that within a concentration range of 0.26–26 μM (covering the intracellular substrate concentration range), a good linearity between MS response and the concentration of furimazine as substrates in cell lysate is obtained, indicating that the non-contact electrospray device exhibits excellent substrate detection capability under complex environments. Based on this result, we monitored the enzymatic reaction under different enzyme concentrations (covering the intracellular GAPDH concentration range) by adding the substrate in excess to simulate the enzymatic reaction process in cell lysate. The MS responses of the product and substrate during the reaction are shown in Fig. 1b and c, respectively. With an increase in enzyme concentration, the product generation rate gradually improves. When the enzyme concentration exceeds 1.6 mg L−1, the peak of product generation can be achieved within 2 minutes. It is worth noting that after reaching its peak concentration, there may be a slight decrease followed by eventual stabilization in product concentration due to the reported inverse reactions observed in previous studies.34–36 The same conclusion is also reached regarding substrate consumption as depicted in Fig. 1c. Based on these findings, we analyzed the correlation between enzyme concentration and product production at a reaction time of 2 minutes, as illustrated in Fig. 1d. In order to better estimate the number of proteins in single cells, we converted the absolute concentration of proteins into the number of copies of proteins in a single cell according to previous literature.37 There is a piecewise linear relationship between the product production and the protein copy number, which validates the quantification capacity of our strategy. The detection limit of the method can be as low as 400 copies per cell. The results indicate that there are probably different catalytic reaction rate constants in the range of high concentration and low concentration of proteins (copy number of 50
000 as the dividing point).
 |
| Fig. 1 Enzymatic reaction kinetics monitoring using non-contact electrospray MS. (a) Standard curve of substrate furimazine at different concentrations in cell lysate. (b) MS response of product during enzymatic reaction under different enzyme concentrations. (c) MS response of substrate during enzymatic reaction under different enzyme concentrations (low enzyme concentration is shown on the top right). (d) Linear correlation between intensity of the product and simulated intracellular enzymatic concentration at monitoring time point of 2 min (n = 3 independent experiments; error bars: mean ± s day). | |
In summary, we simulated the enzymatic reaction process in cellular contents and confirmed that the MS response of the product was positively correlated with the enzyme concentration at the specific reaction time, and good linearity within a specific range was observed. The strong signal amplification ability offers the probability for the quantification of proteins with low copy number in cells. The results lay a foundation for subsequent intracellular protein quantification.
CRISPR-based Nluc knock-in cell line construction
After confirming the quantification capability of the strategy in vitro, we introduced the system into cells for intracellular protein verification. To characterize the abundance of endogenous GAPDH expression and maintain its original transcriptional regulatory mechanism, CRISPR-Cas9 gene editing technology was utilized to achieve site-specific Nluc knock-in at the GAPDH locus. The main workflow is illustrated in Fig. 2a, where Cas9 nuclease initially induces a double-strand break (DSB) at specific sites under the guidance of single guide RNA (sgRNA). In the presence of donor DNA fragments, Nluc is inserted into the C-terminal region of GAPDH through the intracellular homologous recombination repair mechanism. Initially, five sgRNA sequences were designed (Table S1†) targeting the last exon of GAPDH, and their targeted cleavage efficiency was evaluated using the T7E1 assay as shown in Fig. 2b. Considering the overall editing performance, sgRNA-1 and sgRNA-2 were subsequently selected as guide RNAs. Compared to the control group, gene-edited cell samples exhibited significant luminescent intensity, indicating successful insertion (Fig. 2c), and normal expression of Nluc was further confirmed by PCR verification (primer sequence in Table S1†). Through fluorescence-activated cell sorting (FACS) followed by monoclonal culture techniques, an excellent luminescent phenotype #76 cell line from the sgRNA-1 edited sample was finally obtained (Fig. 2d). PCR and sequencing results demonstrated successful generation of the Nluc knock-in cell line (sequence in Table S2†). qPCR and enzyme activity assays confirmed the endogenous expression level as well as the enzyme activity of GAPDH in the #76 cell line (Fig. S4 and S5,† primer sequences in Table S1†), which could be employed for endogenous GAPDH characterization and subsequent single-cell mass cytometry analysis. In order to further confirm the quantitative capability of this method for low-abundance proteins within single cells, similarly, we also applied this strategy to establish a TP53-cypridina luciferase (Cluc) cell line (Fig. S6†) based on the enzymatic reaction of Cluc, thereby demonstrating that this method also has the ability to quantify low-abundance proteins in single cells.
 |
| Fig. 2 Nluc knock-in cell line construction. (a) Workflow of CRISPR-Cas9-mediated Nluc knock-in process. (b) Comparison of the site-specific edition efficiency of five sgRNAs (represented by numbers 1–5) on the genome. (c) PCR (top right) and luminescence test results for Nluc knock-in verification (n = 3 independent experiments; error bars represent mean ± s day). (d) PCR (top right) and luminescence test results for the obtained monoclones. | |
Single-cell mass cytometry analysis
With the obtained Nluc knock-in cell line, we conducted single-cell mass cytometry analysis using a modified non-contact electrospray-based organic mass cytometry platform.14 The cells were incubated with the substrate for a specific duration, followed by removal of excess substrate, washing, fixation, and monodispersion prior to MS analysis. To achieve optimal intracellular signal amplification performance, we optimized the substrate incubation time (Fig. S7†), and a 10 minutes incubation was selected for subsequent monitoring. A representative cellular MS spectrum is shown in Fig. S8.† The total ion chromatogram (TIC) and extraction ion chromatogram (EIC) of glucose are presented in Fig. 3a and b, respectively. Single pulse peaks were observed in the TIC, which closely matched the pulse peaks in the EIC of endogenous cellular metabolites, such as glucose, indicating the occurrence of single-cell events and good monodispersity of cells. Furthermore, only cells incubated with substrates exhibited distinct EIC signals corresponding to both substrates and products; no such signals were observed in the control group (Fig. 3a and S9†). The fixation process prevents the leakage of enzymatic reaction products from the cells (Fig. S10†), which also facilitates metabolite detection without salt disturbance (Fig. S11†). In addition, both negative and positive modes enabled the detection of products and substrates (Fig. S12†), thereby expanding the scope of metabolite profiling. Hence, our strategy possesses the capability to detect substrates and products at the single-cell level while demonstrating the orthogonality of the reaction in complex cytoplasmic environments. To validate the quantification results obtained through single-cell mass cytometry, an insulin treatment model was employed. According to relevant literature,38–40 the promoter region of GAPDH contains numerous cis-regulatory elements such as hypoxia response elements (HREs) and insulin response elements (IREs). Consequently, under conditions of hypoxia and insulin treatment, the inducible transcription factor HIF complex binds to this region, thereby enhancing transcriptional expression levels. Simultaneously, ethanol can inhibit this signaling pathway,40 resulting in the decrease in GAPDH expression. The quantification results obtained through single-cell mass cytometry are depicted in Fig. 3c. With increasing concentrations of insulin, there is a significant increase in product intensity; however, upon ethanol treatment, the response decreases, which is consistent with existing theories. Western blotting conducted on bulk samples also exhibited similar changes (Fig. 3d), indicating that our strategy possesses excellent quantification capabilities for intracellular proteins at the single-cell level.
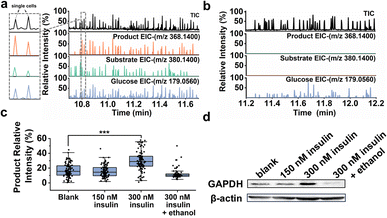 |
| Fig. 3 Single-cell mass spectrometry quantification results. TIC and EICs of product (m/z 368.1400), substrate (m/z 380.1400), and glucose (m/z 179.0560) in Nluc knock-in cells in negative mode (a) with substrate incubation and (b) without substrate incubation. (c) Box plots of product relative intensity changes of single cells when treated with different concentrations of insulin and ethanol (cell number = 400; black lines indicate means; two-way ANOVA; the box plot shows the median, 0.25 and 0.75 quantiles, and whiskers extend to points within the 1.5 interquartile range of lower and upper quartiles). *** denoted p values <0.001). (d) Western blot assay of GAPDH in Nluc knock-in cells that were treated with different concentrations of insulin and ethanol. β-actin was used as a loading control. | |
Single cell multi-dimensional analysis of GSNO oxidation stress
Metabolic profiling accompanied by quantification of GAPDH expression on single cells under oxidative stress induced by GSNO was conducted using this multi-dimensional bio mass cytometry. GSNO is considered a major intracellular nitric oxide donor that can induce trans-nitrosylation and glutathione conversion of GAPDH at the post-translational modification level, thereby affecting the intranuclear transport of GAPDH and triggering the apoptosis process.32,41–43 Additionally, NO can also influence the transcriptional level of GAPDH expression.44 Initially, we incubated Nluc knock-in cell lines under different concentrations of GSNO. Oxidative stress significantly altered the metabolic pattern (Fig. 4a and S13–S15†), leading to distinct cell clustering (Fig. S16†) and increasing cell heterogeneity (Fig. S14 and S16†), which cannot be exhibited in bulk sample studies. Specifically, as oxidative pressure increased, the TCA cycle and amino acid metabolism pathways were gradually activated. Interestingly, fatty acid metabolism was most affected by oxidative stress (Fig. S17†), with some lipid hormones and long-chain fatty acids initially increasing in expression before subsequently decreasing, consistent with the GAPDH expression, which demonstrates that this platform can provide the molecular information of the dynamic process at the single-cell level. It has been reported that under conditions of oxidative stress, the upregulation of GAPDH expression triggers an autophagic response to ensure the generation of glycolytic ATP for cellular protection against oxidative damage.44–46 Excessive oxidative stress, however, can ultimately impair the capability of oxidative defense, as evidenced by the down-regulation of GAPDH. The addition of GSH alleviated the oxidative stress and upregulated the expression of GAPDH, indicating that the impact of oxidative stress is reversible. Intragroup correlation analysis (Fig. 4b) identified metabolites most closely related to the trend of GAPDH change. Furthermore, trend analysis (Fig. 4c) reveals multiple metabolic patterns. The end products of glycolysis, pyruvate and lactate, as well as some metabolites related to the TCA cycle, showed a decreasing trend as oxidative stress levels increased, which is consistent with the relating literature.47,48 Correspondingly, the intermediate metabolites exhibited an upward trend,49 suggesting that the increase in oxidative stress may have a significant and irreversible impact on the activity of downstream metabolic enzymes. Additionally, some amino acids, such as aspartate and glutamine, displayed a trend of initially increasing followed by decreasing, which has not been reported yet. The changes in the levels of GAPDH were generally consistent with those of certain long-chain fatty acids. Combined with intragroup correlation analysis and trend analysis, we identified a total of 16 metabolites that exhibited the closest association with changes in GAPDH levels. These metabolites include fatty acids, amino acid derivatives, and metabolites involved in glycosylation modification (Table S3†). Five long-chain fatty acids, including palmitic acid, pentadecanoic acid, myristic acid, linoleic acid, and UDP-N-acetylglucosamine (UDP-GlcNAc), were selected as the specific metabolites, based on the literature50–53 (Fig. 4d). Western blotting of key enzymes related to these pathways also demonstrated a similar change pattern as observed for GAPDH expression levels, which also correspond to wild-type 293T (Fig. S18†), confirming a close relationship between these metabolites and protein expression (Fig. 4e). Conventional liquid chromatography-mass spectrometry (LC-MS) assay also exhibits similar results (Fig. S19†). The MS/MS spectrum of representative metabolites is presented in Fig. S20.† However, there are some inconsistencies with amino acids, such as lysine and serine, which reflect possible metabolism pathway heterogeneity. It is noteworthy that UDP-GlcNAc serves as a donor for O-linked GlcNAc modification,51 which exerts profound effects on the oxidative stress response through the regulation of specific kinase glycolation.50 Moreover, UDP-GlcNAc itself is derived from the hexosamine biosynthetic pathway (HBP), which directly links to the glycolytic pathway of upstream GAPDH. Fatty acid metabolism is connected to HBP through the supply of synthetic substrate acetyl-CoA. Therefore, we hypothesize that GAPDH, instead of functioning solely as a glycolytic enzyme, may be involved in mediating the oxidative stress response mechanism mediated by UDP-GlcNAc. The detailed molecular mechanisms underlying this regulation remain to be further explored.
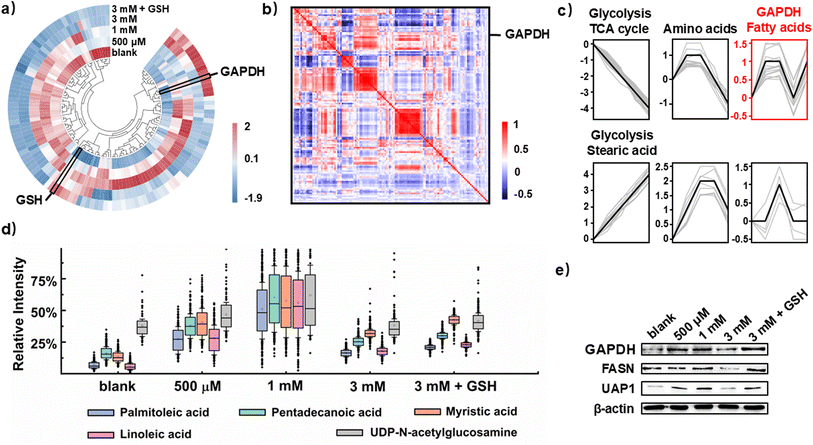 |
| Fig. 4 Application of GSNO-induced oxidative stress. (a) Circular heat maps of metabolites under different concentrations of GSNO and GSH. (b) Correlation matrix of metabolites within all groups. (c) Trend analysis of metabolites within all groups. Detected metabolites were mainly divided into 6 trends according to content change patterns. Change patterns of metabolites closely related to GAPDH are highlighted with a red frame. (d) Box plots of relative abundance of specific fatty acids and UDP-GlcNAc in single cells with treatment of different concentrations of GSNO and GSH (cell number = 400; black lines indicate means; the box plot shows the median, 0.25 and 0.75 quantiles, and whiskers extend to points within 1.5 interquartile ranges of lower and upper quartiles). (e) Western blot assay of GAPDH, FASN (fatty acid synthase), and UAP1 (UDP-N-acetylglucosamine pyrophosphorylase 1) in cells that were treated with GSNO and GSH. β-actin was used as a loading control. | |
Conclusions
In summary, we have developed a multi-dimensional bio mass cytometry platform for the simultaneous analysis of intracellular proteins and metabolites at the single-cell level. By utilizing the enzymatic kinetics of luciferase Nluc, we have transformed the quantification of intracellular proteins into the detection of enzymatic reaction products, thereby achieving signal transformation and amplification. To accurately determine the abundance of endogenous protein GAPDH, we introduced the CRISPR-Cas9 gene editing system to precisely insert Nluc at the C-terminal of GAPDH. Both in vitro enzymatic experiments and single-cell mass cytometry validated the accuracy of our method for protein quantification. Finally, we applied this multi-modal bio mass cytometry platform to investigate GSNO-induced oxidative stress and revealed significant metabolic changes at the single-cell level. We identified 16 metabolites that positively correlated with changes in GAPDH abundance. Notably, long-chain fatty acids and UDP-GlcNAc showed close correlation in terms of both protein levels and metabolite levels, suggesting the possibility of a synergistic anti-oxidation function between GAPDH and UDP-GlcNAc-mediated oxidative stress response. In conclusion, our method expands the toolbox for single-cell multi-modal mass spectrometry methodology and provides insights into understanding the mechanisms of cellular apoptosis, aging, and oxidative stress response at the single-cell level.
Experimental section
Chemicals and materials
293T cells were obtained from the Wensheng Wei Laboratory (Peking University). Dulbecco's modified eagle medium (DMEM)/high glucose (+0.584 g per L L-glutamine, + 4.5 g per L glucose, + 3.7 g per L sodium bicarbonate, + 0.110 g per L sodium pyruvate, + 80 U per mL penicillin, + 0.08 mg per mL streptomycin) was purchased from Thermo Fisher Scientific Life Technologies (USA). Trypsin–EDTA digestion solution (0.25%) was purchased from Solarbio Biotech. Co., Ltd. (Beijing, China). Phosphate buffer saline (PBS 1×) was purchased from Hyclone (Logan, UT, USA). Certified fetal bovine serum (FBS) was purchased from BI (Kibbutz Beit-Haemek, Israel). Human insulin was purchased from HarveyBio (Beijing, China).
Methanol (HPLC grade) was purchased from Fisher Scientific (USA). Ethanol (AR grade) was purchased from Modern Oriental Fine Chemistry (Beijing, China). Acetone (AR grade) was purchased from Tong Guang fine chemicals company (Beijing, China). Purified water was from Hangzhou Wahaha Group (Hangzhou, China). Furimazine was purchased from TargetMol (Shanghai, China). GSNO was purchased from Enzo Life Sciences (USA). Ordering silica capillaries (150 μm i.d. and 360 μm o.d.) were obtained from Ruifeng Chromatographic Devices Co., Ltd. (Hebei, China). Electrospray emitters were made of fused silica capillaries (360 μm i.d. and 150 μm o.d.), obtained from Ruifeng Chromatographic Devices Co., Ltd. (Hebei, China).
All the primers were ordered from Beijing Ruiboxingke Biotechnology Co., Ltd. (Beijing, China). DNA template was cloned from the protein purification vector. X-tremeGEN HP DNA Transfection Reagent was purchased from Roche Pharmaceuticals Co., Ltd. (Shanghai, China). Opti-MEM medium was purchased from Thermo Fisher Scientific Life Technologies (USA). Universal DNA purification kit was purchased from TIANGEN (Beijing, China). RIPA lysis buffer was purchased from Solarbio (Beijing, China). Trans Taq DNA polymerase high fidelity was purchased from TransGen (Beijing, China).
Protein purification vector pET-His/hGAPDH:3 × GGGGS:Nluc was designed and synthesized by VectorBuilder Inc. (Guangzhou, China). Escherichia coli strain BL21 was used for GAPDH-Nluc fusion protein expression. The binding buffer was composed of 50 mM Tris–HCl, 2.5 mM EDTA, 2 mM DTT, 500 mM NaCl, and 15 mM imidazole (pH = 8.0). The elution buffer was composed of 50 mM Tris–HCl, 2.5 mM EDTA, 2 mM DTT, 500 mM NaCl, and 300 mM imidazole (pH = 8.0). Superdex 75 increase prepacked columns were purchased from GE Healthcare (USA).
Anti-FASN antibody and anti-UAP1 antibody were purchased from abcam (USA). Anti-GAPDH antibody and anti-actin antibody were purchased from Proteintech Group (USA). BCA Protein Concentration Assay Kit, Triton X-100, 30% Acr-Bis (29
:
1) solution, and 6 × SDS-PAGE sample loading buffer were purchased from Beyotime (Shanghai, China). 10% SDS solution was purchased from Solarbio (Beijing, China). Ammonium persulfate was purchased from Macklin (Shanghai, China). Clarity Western ECL Substrate was purchased from Bio-rad (California, USA). Bovine Serum Albumin was purchased from yuanye Bio-Technology Co., Ltd (Shanghai, China), Tween-20 was purchased from Beijing Chemical Reagent Research Co., Ltd. (Beijing, China), secondary antibodies of HRP-Goat Anti-Rabbit IgG (H + L) were purchased from Biodragon (Shanghai, China), and the stripping buffer was purchased from Cowin Biotech Co., Ltd (Suzhou, China).
Cell culture and harvest
GAPDH-Nluc knock-in 293T cells were cultured with DMEM supplemented with 20% FBS, 80 U per mL penicillin, and 80 μg per mL streptomycin in an incubator (5% CO2, 37 °C). At the logarithmic growth phase, the medium was removed, followed by cell rinsing once using PBS, and then dissociation by trypsin solution within 2 min. The supernatant was removed by centrifugation (1250 rpm, 5 min). Furimazine PBS solution (26 μM) was then added into cell deposition and resuspended thoroughly. After 5 min of dark incubation (5% CO2, 37 °C), the remaining substrate solution was removed by centrifugation (1250 rpm, 3 min), and cells were fixed by pre-cooled acetone for 3 min, followed by washing with a cold 80% methanol/H2O solution twice (1250 rpm, 5 min), and finally suspended in cold 80% methanol/H2O. The cell number was determined using a cell counter. The final cell suspension needed to be analyzed by mass spectrometer immediately.
Protein purification
GAPDH-Nluc fusion protein was expressed in Escherichia coli strain BL21 using purification plasmid pET-His/hGAPDH:3 × GGGGS:Nluc. Recombinant cells were cultivated at 37 °C in LB medium. At an OD600 of 0.6–0.8, expression was induced by the addition of 0.4 mM IPTG, and cultures were grown at 16 °C for 12 h. E. coli cells were resuspended in binding buffer, lysed by sonication supplemented with 1 mM PMSF, and sedimented at 15
000 rpm for 30 min. The supernatant lysates were chromatographed on a Ni2+ column. Protein fractions were eluted with the elution buffer with five column volumes and then exchanged into PBS solution, pH 7.3. Fractions were concentrated and further purified on Superdex 75 increase prepacked columns. SDS-PAGE results are presented in Fig. S1a,† which implies successful purification of the GAPDH-Nluc fusion protein. MS/MS analysis and in vitro enzymatic experiments also confirmed the successful purification of active fusion protein.
The transformation of GAPDH-Nluc protein absolute concentration to protein copies was estimated from the following equation:
where single-cell volume was approximately set as 1 pL according to the relevant literature,
37,54,55 the molecular weight of GAPDH-Nluc was 57 kDa, and the copy number of GAPDH-Nluc fusion protein in single cells was
n.
CRISPR-Cas9 based Nluc knock-in cell line construction
Detailed gene editing workflow refers to relevant protocols.56,57 Briefly, oligonucleotides for sgRNA templates were synthesized, annealed, and inserted into the corresponding vectors. Golden gate assembly was used to assemble the constructed vectors into the CRISPR/Cas9 vector. Oligonucleotide sequences of sgRNA and primers are shown in Table S1.† The sgRNA with the highest editing efficiency was selected using the T7E1 assay. We synthesized double-stranded template DNA donor specific to the cleavage site and co-transfected it with sgRNA expression plasmid into 293T cells. Successfully transfected cells were cultured as single clones using fluorescence-activated cell sorting (FACS). After several weeks, luminescence assays were conducted to screen for cells exhibiting successful insertion of Nluc, followed by expansion of their cultivation. Further genotype identification and sequencing experiments confirmed the successful acquisition of 293T cells with the GAPDH-Nluc genotype.
GSNO oxidation stress model construction
Cells were maintained under optimal growth conditions in a humidified incubator in DMEM, 20% fetal bovine serum, 50 U per mL penicillin, and 50 μg per mL streptomycin, at 37 °C and 5% CO2 in the logarithmic growth phase. Five plates of GAPDH-Nluc 293T cell lines (6 cm dishes) were treated with PBS solutions of GSNO, achieving final concentrations of 0, 500 μM, 1 mM, and 3 mM, respectively. The media were gently agitated to ensure thorough mixing and incubated at 37 °C for 5 hours. One plate with a final concentration of 3 mM GSNO was subsequently supplemented with a GSH solution at a final concentration of 3 mM and incubated at 37 °C for an additional 3 hours to form a replenishment group. Following incubation, the media were removed, and the cells were washed twice with PBS, making them suitable for subsequent assays, including single-cell mass spectrometry, LC-MS, and Western blot analysis.
Single cell MS analysis
The mass cytometric analysis of cells was similar to organic mass cytometry,58 as follows (Fig. S2†): the prepared cell solution (∼8 × 104 mL) was injected by an MS built-in injection syringe at a flow rate of 1–3 μL min−1, and the cells were mono-dispersed by an ordering capillary (150 μm i.d. and 360 μm o.d.) twined into 6 loops with diameters of 6 cm. In particular, a homemade non-contact ESI emitter was used for cellular content ionization. In detail, nearly 5 cm of copper wire was surrounded with an emitter that had a faded outer coating layer. MS analysis was performed with positive and negative modes, respectively. After each test or between two tests, the flow system required washing using methanol and 80% methanol/H2O in turn with a flow rate of 20 μL min−1 for 10 minutes each.
Electrospray emitters were made of fused silica capillaries (50 μm i.d. and 150 μm o.d.). The tip of the capillary was firstly disposed of on fire and then washed with ethanol to peel off the coating of the capillary. No extra pulling was needed. The spray electric field was provided by the MS built-in DC electrical power supply system, and electric contact was realized through the connection of the surrounding copper wire outside of the emitters with a nanospray ion source (Thermo Fisher Scientific, CA, USA). All the mass cytometric experiments were conducted on Orbitrap MS (Q Exactive plus, Thermo Fisher Scientific, CA, USA). The parameters of full scan MS mode were set as follows: mode—negative; scan range—m/z 80–1200; resolution—35000; microscans—1; AGC target—1e6; maximum inject time—50 ms; sheath gas flow rate—0; aux gas flow rate—0; sweep gas flow rate—0; spray voltage—4 kV capillary temperature—320 °C; tube lens voltage—60. Xcalibur software (version 4.0) was used to control the MS system, data collection, and subsequent analysis.
Single-cell events were selected according to the signal-to-noise ratio, characteristic mass peaks, and monodispersity. Only cells with a total ion current (TIC) of pulse peak >5 times the signal-to-noise ratio, characteristic mass peaks of cell-specific metabolites, and good monodispersity could be chosen. The mass spectra with the highest total ion current was selected to represent single cells. The intensities of identified metabolites in each mass spectrum of a single cell were assembled into a data matrix. The MS intensities of metabolites in each mass spectrum were normalized by the total ion current (TIC). Characteristic MS peaks were defined as totally new MS peaks that appeared in sample spectra compared with control group spectra. The metabolites were identified with the available literature,59–65 which provided single-cell metabolite identification lists with MS or MS/MS information. The single-cell data were performed using the OmicShare online platform for data analysis (https://www.omicshare.com/tools), including correlation analysis, volcano plots, data visualization and clustering by uniform manifold approximation and projection (UMAP), trend analysis, circular heat maps, and KEGG enrichment pathway analysis.
Western blot assay
Cell lysates were resolved on 10% SDS/PAGE gels for electrophoresis and transferred to PVDF membranes (Millipore) by a Trans-blot Turbo transfer system (Bio-rad). After blocking with 5% BSA at 37 °C for 1 h, probed with primary antibody overnight at 4 °C, the membrane was incubated with goat anti-mouse IgG HPR secondary antibody for 1 h at room temperature. Clarity Western ECL Substrate Kit (Bio-rad) and Chemi-doc system (Bio-rad) were used to detect protein bands.
TP53 validation model.
The methods of inducing changes of TP53 protein expression were referred to the relevant literature.66–68 In brief, the culture medium was first removed, and the cells were irradiated in a 3 cm dish under ultraviolet light for 20 minutes, followed by medium incubation for 2 h. The culture medium was sucked off, and the cells were washed with PBS. The cells were either scraped off and transferred into a 1.5 mL centrifuge tube for centrifugation to collect cell precipitation, which was deposited in a refrigerator at −20 °C for WB, or the washed cells were subjected to a single-cell mass cytometry assay (the substrate was cypridina luciferin, a Cluc luciferin substrate).
qPCR quantification.
Total RNA was extracted using the TaKaRa MiniBEST universal RNA extraction kit (ranging from 100 to 150 μg), which was quantified using a NanoDrop 1000 spectrophotometer (Thermo Scientific), as per the manufacturer's instructions, to a 70 μL final volume. Reversal transcription was carried out using the TaKaRa PrimeScript RT reagent kit. The expected amplicon size was 150 bp, and the primer sequences are listed in Table S1.† TB Green Premix Ex Taq was used as the fluorescence dye. PCR cycling was performed at 95 °C, 2 30 s, followed by 40 cycles of 95 °C, 5 s, and 60 °C, 30 s, on an AriaMx (Agilent), with the fluorescence acquired at the end of each cycle. A melt curve performed at the end of the amplification was undertaken to confirm that there was only a single product amplified in each reaction.
GAPDH enzyme activity assay.
The activity of GAPDH in the supernatant was measured according to the manufacturer's instructions (Solarbio, Beijing, China). The NanoDrop 1000 spectrophotometer (Thermo Scientific) was preheated to 37 °C, and the wavelength was set to 340 nm. The samples were fully mixed with the working solution provided by the GAPDH detection kits (Solarbio, Beijing, China) in a 1 cm cuvette. At 340 nm wavelength, the absorbance values A1 and A2 of the sample mixture before and after incubation at 37 °C for 5 minutes were measured, respectively. Finally, the activity of the enzyme was calculated. Blank indicates ddH2O was added instead of cell lysate.
LC-MS/MS analysis.
293T cells were seeded in 6 cm cell culture plates with Dulbecco Modified Eagle's Medium (DMEM) containing 10% fetal bovine serum (FBS) and 1% penicillin/streptomycin. Cells were grown to 80% confluence and washed using 1 mL of PBS. Cell plates were placed on the dry ice and fast quenched with 1 mL of MeOH
:
ACN
:
H2O (2
:
2
:
1, v/v/v, pre-cooled in a −80 °C refrigerator) solvent mixture. The plates were incubated at −80 °C for 40 min. Cells were scraped from the plate and transferred to a 1.5 mL centrifuge tube. The samples were vortexed for 1 min, followed by 15 min of centrifugation using 16
200
×
g at 4 °C. The supernatant was taken and evaporated to dryness in a vacuum concentrator. Dry extracts were reconstituted in 100 μL of ACN
:
H2O (1
:
1, v/v, containing internal standard L-4-chlorophenylalanine), followed by 10 min sonication (50 Hz, 4 °C) and 15 min centrifugation using 16
200
×
g at 4 °C to remove insoluble material. Supernatants were finally transferred to HPLC glass vials and stored at −20 °C prior to LC/MS analysis.
Metabolomics data of 293T cell samples were acquired using a UHPLC system (ACQUITY UPLC H-class, Waters) coupled to an orbitrap mass spectrometer (Q-Exactive Plus, Thermo Scientific). The Waters ACQUITY UPLC BEH C8 column (particle size, 1.7 μm; 100 mm (length) × 2.1 mm (i.d.)) was used for LC separation for the HILIC mode. Column temperature was kept at 50 °C. Mobile phases A = 0.1% ammonium hydroxide in acetonitrile
:
water = 95
:
5 solution and B = 0.1% ammonium hydroxide in acetonitrile were used for ESI negative mode. The linear gradient was eluted from 0% B (0–1 min), 0% B to 40% B (1–4 min), 40% B to 80% B (4–14 min), 80% B to 99% B (14–20 min), 99% B (20–25 min), and 0% B (25–30 min). The flow rate was 0.3 mL min−1. The sample injection volume was 10 μL.
All the mass cytometric experiments were conducted on an Orbitrap MS (Q-Exactive Plus, Thermo Fisher Scientific, CA, USA). The parameters of the full-scan MS mode were set as follows: mode, negative; scan range, m/z 80–1200; resolution, 35
000; microscans, 1; AGC target, 1 × 106; maximum injection time, 50 ms; sheath gas flow rate, 30; aux gas flow rate, 0; sweep gas flow rate, 50; spray voltage, 3.5 kV; capillary temperature, 320 °C; and tube lens voltage, 60. For metabolite identification, the full MS-ddMS2 acquisition mode was used. For the full MS process, the parameters were set as follows: m/z 80–1200; resolution—35000; microscans—1; AGC target—3e6; and maximum injection time—100 ms. For the ddMS2 process, the parameters were set as follows: resolution—17500; AGC target—1e5; maximum IT—50 ms; loop count/top N—7; isolation window—0.4 m/z; NCE—30, 40, 50; minimum AGC target—8e3; excluding isotopes—on; dynamic exclusion—20 s. Xcalibur software (version 4.0) was used for the control of the MS system, data collection, and subsequent analysis.
Statistical analysis.
Trend analysis can classify metabolites with similar change characteristic patterns within a changing trend. We used the OmicShare online tool (https://www.omicshare.com/tools/) for analysis. Metabolite abundance was normalized with log
2(FC); P < 0.05 was considered statistically significant. Short time-series expression mine software (STEM) (profiles ≤20 and FC ≥2) simulates the possible change trends and then calculates the correlation coefficient between each factor and the preset trend and divides the factors into the most similar trends. The top six trends were exhibited in the main text. Intra-group correlation analysis was used to calculate pairwise correlations for all metabolites within single cells and draw correlation heat maps. The correlation index was calculated by Spearman's correlation method using the OmicShare tools.
Data availability
The data supporting this article have been included as part of the ESI.†
Author contributions
Y. B. carried out project administration, funding acquisition, as well as writing – reviewing and editing. S. J. Q. proposed the strategy, designed and performed the experiments and data analysis. W. S. W. and X. Y. Z. offered instruction on gene edition experiment. Y. Z. and D. Y. M. contributed to the instrument construction. All authors discussed the results and contributed to the final manuscript.
Conflicts of interest
There are no conflicts to declare.
Acknowledgements
We acknowledge financial support from the National Natural Science Foundation of China (No. 22125401 and 22074003) and the National Key R&D Program of China (2022YFC3400700). We thank the public instrument platform of the College of Urban and Environmental Sciences at Peking University for assistance with qPCR validation and Western blot assays.
References
- A. C. Adey, Single-cell multiomics to probe relationships between histone modifications and transcription, Nat. Methods, 2021, 18, 602–603 CrossRef CAS PubMed.
- M. P. Meers, G. Llagas, D. H. Janssens, C. A. Codomo and S. Henikoff, Multifactorial profiling of epigenetic landscapes at single-cell resolution using MulTI-Tag, Nat. Biotechnol., 2022, 41, 708–716 Search PubMed.
- E. Fiskin, C. A. Lareau, L. S. Ludwig, G. Eraslan, F. Liu, A. M. Ring, R. J. Xavier and A. Regev, Single-cell profiling of proteins and chromatin accessibility using PHAGE-ATAC, Nat. Biotechnol., 2021, 40, 374–381 CrossRef PubMed.
- Y. Muto, P. C. Wilson, N. Ledru, H. Wu, H. Dimke, S. S. Waikar and B. D. Humphreys, Single cell transcriptional and chromatin accessibility profiling redefine cellular heterogeneity in the adult human kidney, Nat. Commun., 2021, 12, 2190 CrossRef CAS PubMed.
- M. Stoeckius, C. Hafemeister, W. Stephenson, B. Houck-Loomis, P. K. Chattopadhyay, H. Swerdlow, R. Satija and P. Smibert, Simultaneous epitope and transcriptome measurement in single cells, Nat. Methods, 2017, 14, 865–868 CrossRef CAS PubMed.
- M. Xue, W. Wei, Y. Su, J. Kim, Y. S. Shin, W. X. Mai, D. A. Nathanson and J. R. Heath, Chemical methods for the simultaneous quantitation of metabolites and proteins from single cells, J. Am. Chem. Soc., 2015, 137, 4066–4069 CrossRef CAS.
- K. Vandereyken, A. Sifrim, B. Thienpont and T. Voet, Methods and applications for single-cell and spatial multi-omics, Nat. Rev. Genet., 2023, 24, 494–515 CrossRef CAS.
- C. Zhu, S. Preissl and B. Ren, Single-cell multimodal omics: the power of many, Nat. Methods, 2020, 17, 11–14 CrossRef CAS PubMed.
- V. Marx, How single-cell multi-omics builds relationships, Nat. Methods, 2022, 19, 142–146 CrossRef CAS.
- M. Y. Y. Lee and M. Li, Integration of multi-modal single-cell data, Nat. Biotechnol., 2024, 42, 190–191 CrossRef CAS PubMed.
- R. Zenobi, Single-cell metabolomics: analytical and biological perspectives, Science, 2013, 342, 1243259 CrossRef CAS PubMed.
- M. Fessenden, Metabolomics: small molecules, single cells, Nature, 2016, 540, 153–155 Search PubMed.
- J. M. Perkel, Proteomics at the single-cell level, Nature, 2021, 597, 580–582 Search PubMed.
- S. Xu, M. Liu, Y. Bai and H. Liu, Multi-dimensional organic mass cytometry: simultaneous analysis of proteins and metabolites on single cells, Angew. Chem., Int. Ed., 2021, 60, 1806–1812 CrossRef CAS PubMed.
- L. Zhang and A. Vertes, Single-cell mass spectrometry approaches to explore cellular heterogeneity, Angew. Chem., Int. Ed., 2018, 57, 4466–4477 Search PubMed.
- A. Agarwal, D. Khushalani, A. Harkare and R. Agrawal, A review of FACS: fluorescence activated cell sorting system, Biosci. Biotechnol. Res. Commun., 2020, 13, 436–439 CrossRef.
- M. Xue, W. Wei, Y. Su, D. Johnson and J. R. Heath, Supramolecular probes for assessing glutamine uptake enable semi-quantitative metabolic models in single cells, J. Am. Chem. Soc., 2016, 138, 3085–3093 CrossRef CAS.
- Y. Su, M. E. Ko, H. Cheng, R. Zhu, M. Xue, J. Wang, J. W. Lee, L. Frankiw, A. Xu, S. Wong, L. Robert, K. Takata, D. Yuan, Y. Lu, S. Huang, A. Ribas, R. Levine, G. P. Nolan, W. Wei, S. K. Plevritis, G. Li, D. Baltimore and J. R. Heath, Multi-omic single-cell snapshots reveal multiple independent trajectories to drug tolerance in a melanoma cell line, Nat. Commun., 2020, 11, 2345 CrossRef CAS.
-
B. Shrestha, in Single Cell Metabolism: Methods and Protocols, ed. B. Shrestha, Springer New York, New York, NY, 2020, pp. 1–8, DOI:10.1007/978-1-4939-9831-9_1.
- R. Aebersold and M. Mann, Mass spectrometry-based proteomics, Nature, 2003, 422, 198–207 CrossRef CAS PubMed.
- C. Lombard-Banek, J. Li, E. P. Portero, R. M. Onjiko, C. D. Singer, D. O. Plotnick, R. Q. Al Shabeeb and P. Nemes, In vivo subcellular mass spectrometry enables proteo-metabolomic single-cell systems biology in a chordate embryo developing to a normally behaving tadpole (X. laevis), Angew. Chem., Int. Ed. Engl., 2021, 60, 12852–12858 Search PubMed.
- Y. He, H. Yuan, Y. Liang, X. Liu, X. Zhang, Y. Ji, B. Zhao, K. Yang, J. Zhang, S. Zhang, Y. Zhang and L. Zhang, On-capillary alkylation micro-reactor: a facile strategy for proteo-metabolome profiling in the same single cells, Chem. Sci., 2023, 14, 13495–13502 Search PubMed.
- Y. Li, H. Li, Y. Xie, S. Chen, R. Qin, H. Dong, Y. Yu, J. Wang, X. Qian and W. Qin, An integrated strategy for mass spectrometry-based multiomics analysis of single cells, Anal. Chem., 2021, 93, 14059–14067 CrossRef CAS.
- J. Wu, Q.-Q. Xu, Y.-R. Jiang, J.-B. Chen, W.-X. Ying, Q.-X. Fan, H.-F. Wang, Y. Wang, S.-W. Shi, J.-Z. Pan and Q. Fang, One-shot single-cell proteome and metabolome analysis strategy for the same single cell, Anal. Chem., 2024, 96, 5499–5508 CrossRef CAS PubMed.
- D. Shalon, R. N. Culver, J. A. Grembi, J. Folz, P. V. Treit, H. Shi, F. A. Rosenberger, L. Dethlefsen, X. Meng, E. Yaffe, A. Aranda-Diaz, P. E. Geyer, J. B. Mueller-Reif, S. Spencer, A. D. Patterson, G. Triadafilopoulos, S. P. Holmes, M. Mann, O. Fiehn, D. A. Relman and K. C. Huang, Profiling the human intestinal environment under physiological conditions, Nature, 2023, 617, 581–591 CrossRef CAS.
- S. Blasche, Y. Kim, R. A. T. Mars, D. Machado, M. Maansson, E. Kafkia, A. Milanese, G. Zeller, B. Teusink, J. Nielsen, V. Benes, R. Neves, U. Sauer and K. R. Patil, Metabolic cooperation and spatiotemporal niche partitioning in a kefir microbial community, Nat. Microbiol., 2021, 6, 196–208 CrossRef CAS PubMed.
- C. S. Hughes, A. A. Nuhn, L. M. Postovit and G. A. Lajoie, Proteomics of human embryonic stem cells, Proteomics, 2011, 11, 675–690 CrossRef CAS PubMed.
- M. A. Sirover, The role of posttranslational modification in moonlighting glyceraldehyde-3-phosphate dehydrogenase structure and function, Amino Acids, 2021, 53, 507–515 Search PubMed.
- V. I. Muronetz, M. V. Medvedeva, I. A. Sevostyanova and E. V. Schmalhausen, Modification of glyceraldehyde-3-phosphate dehydrogenase with nitric oxide: role in signal transduction and development of apoptosis, Biomolecules, 2021, 11, 1656 Search PubMed.
- V. I. Muronetz, A. K. Melnikova, L. Saso and E. V. Schmalhausen, Influence of oxidative stress on catalytic and non-glycolytic functions of glyceraldehyde-3-phosphate dehydrogenase, Curr. Med. Chem., 2020, 27, 2040–2058 Search PubMed.
- M.-A. Tossounian, B. Zhang and I. Gout, The writers, readers, and erasers in redox regulation of GAPDH, Antioxidants, 2020, 9, 1288 CrossRef CAS PubMed.
- V. F. Lazarev, I. V. Guzhova and B. A. Margulis, Glyceraldehyde-3-phosphate dehydrogenase is a multifaceted therapeutic target, Pharmaceutics, 2020, 12, 416 CrossRef CAS.
- P. D. Hsu, E. S. Lander and F. Zhang, Development and applications of CRISPR-Cas9 for genome engineering, Cell, 2014, 157, 1262–1278 CrossRef CAS PubMed.
- E. N. Harvey, Studies on the oxidation of luciferin without luciferase and the mechanism of bioluminescence, J. Biol. Chem., 1928, 78, 369–375 CrossRef CAS.
- S. M. Marques and J. C. G. Esteves da Silva, Firefly bioluminescence: a mechanistic approach of luciferase catalyzed reactions, IUBMB Life, 2009, 61, 6–17 CrossRef CAS PubMed.
- A. Schenkmayerova, M. Toul, D. Pluskal, R. Baatallah, G. Gagnot, G. P. Pinto, V. T. Santana, M. Stuchla, P. Neugebauer, P. Chaiyen, J. Damborsky, D. Bednar, Y. L. Janin, Z. Prokop and M. Marek, Catalytic mechanism for renilla-type luciferases, Nat. Catal., 2023, 6, 23–38 Search PubMed.
- A. K. Bryan, V. C. Hecht, W. Shen, K. Payer, W. H. Grover and S. R. Manalis, Measuring single cell mass, volume, and density with dual suspended microchannel resonators, Lab Chip, 2014, 14, 569–576 Search PubMed.
- M. C. Alexander, M. Lomanto, N. Nasrin and C. Ramaika, Insulin stimulates glyceraldehyde-3-phosphate dehydrogenase gene-expression through cis-acting dna-sequences, Proc. Natl. Acad. Sci. U. S. A., 1988, 85, 5092–5096 Search PubMed.
- N. Nasrin, L. Ercolani, M. Denaro, X. F. Kong, I. Kang and M. Alexander, An insulin response element in the glyceraldehyde-3-phosphate dehydrogenase gene binds a nuclear protein induced by insulin in cultured cells and by nutritional manipulations in vivo, Proc. Natl. Acad. Sci. U. S. A., 1990, 87, 5273–5277 Search PubMed.
- M. Alexander-Bridges, I. Dugast, L. Ercolani, X. F. Kong, L. Giere and N. Nasrin, Multiple insulin-responsive elements regulate transcription of the GAPDH gene, Adv. Enzyme Regul., 1992, 32, 149–159 Search PubMed.
- M. R. Hara, M. B. Cascio and A. Sawa, GAPDH as a sensor of NO stress, Biochim. Biophys. Acta, Mol. Basis Dis., 2006, 1762, 502–509 CrossRef CAS PubMed.
- M. R. Hara, N. Agrawal, S. F. Kim, M. B. Cascio, M. Fujimuro, Y. Ozeki, M. Takahashi, J. H. Cheah, S. K. Tankou, L. D. Hester, C. D. Ferris, S. D. Hayward, S. H. Snyder and A. Sawa,
S-Nitrosylated GAPDH initiates apoptotic cell death by nuclear translocation following Siah1 binding, Nat. Cell Biol., 2005, 7, 665–674 Search PubMed.
- C. Tristan, N. Shahani, T. W. Sedlak and A. Sawa, The diverse functions of GAPDH: Views from different subcellular compartments, Cell. Signall., 2011, 23, 317–323 Search PubMed.
- J. Bereta and M. Bereta, Stimulation of glyceraldehyde-3-phosphate dehydrogenase mRNA levels by endogenous nitric oxide in cytokine-activated endothelium, Biochem. Biophys. Res. Commun., 1995, 217, 363–369 Search PubMed.
-
N. W. Seidler, in GAPDH: Biological Properties and Diversity, Springer, Dordrecht, 2013, vol. 985 Search PubMed.
- Y. Ito, P. J. Pagano, K. Tornheim, P. Brecher and R. A. Cohen, Oxidative stress increases glyceraldehyde-3-phosphate dehydrogenase mRNA levels in isolated rabbit aorta, Am. J. Physiol. Heart Circul. Physiol., 1996, 270, 81–87 CrossRef PubMed.
- V. Borutaite and G. C. Brown, Nitric oxide induces apoptosis via hydrogen peroxide, but necrosis via energy and thiol depletion, Free Radical Biol. Med., 2003, 35, 1457–1468 Search PubMed.
- J. Yan, X. Huang, D. Zhu and Y. Lou, Enhanced aerobic glycolysis by S-nitrosoglutathione via HIF-1 associated GLUT1/aldolase A axis in human endothelial cells, J. Cell. Biochem., 2017, 118, 2443–2453 CrossRef CAS.
- L. Wei, J. Zhang, S. Wei, C. Wang, Y. Deng, D. Hu, H. Liu, W. Gong, Y. Pan and W. Liao, Nitric oxide alleviates salt stress through protein S-nitrosylation and transcriptional regulation in tomato seedlings, Planta, 2022, 256, 101 CrossRef CAS.
- S. Hardivillé and G. W. Hart, Nutrient regulation of signaling, transcription, and cell physiology by O-GlcNAcylation, Cell Metab., 2014, 20, 208–213 CrossRef.
- K. Lim, B. H. Yoon and C. H. Ha, O-linked N-acetylglucosaminylation of Sp1 interferes with Sp1 activation of glycolytic genes, Biochem. Biophys. Res. Commun., 2015, 468, 349–353 CrossRef CAS PubMed.
- C. Batthyany, F. J. Schopfer, P. R. S. Baker, R. Duran, L. M. S. Baker, Y. Huang, C. Cervenansky, B. P. Branchaud and B. A. Freeman, Reversible post-translational modification of proteins by nitrated fatty acids in vivo, J. Biol. Chem., 2006, 281, 20450–20463 CrossRef CAS.
- Y. Lu, J. Bi, F. Li, G. Wang, J. Zhu, J. Jin and Y. Liu, Differential gene analysis of trastuzumab in breast cancer based on network pharmacology and medical images, Front. Physiol., 2022, 8, 942049 CrossRef PubMed.
- A. Mateus, P. Matsson and P. Artursson, Rapid measurement of intracellular unbound drug concentrations, Mol. Pharm., 2013, 10, 2467–2478 Search PubMed.
- C. M. Gillen and B. Forbush III, Functional interaction of the K-Cl cotransporter (KCC1) with the Na-K-Cl cotransporter in HEK-293 cells, Am. J. Physiol., 1999, 276, C328–C336 Search PubMed.
- F. A. Ran, P. D. Hsu, J. Wright, V. Agarwala, D. A. Scott and F. Zhang, Genome engineering using the CRISPR-Cas9 system, Nat. Protoc., 2013, 8, 2281–2308 CrossRef CAS PubMed.
- J. D. Sander and J. K. Joung, CRISPR-Cas systems for editing, regulating and targeting genomes, Nat. Biotechnol., 2014, 32, 347–355 Search PubMed.
- S. Xu, M. Liu, Y. Bai and H. Liu, Multi-dimensional organic mass cytometry: simultaneous analysis of proteins and metabolites on single cells, Angew. Chem., Int. Ed., 2021, 60, 1806–1812 Search PubMed.
- X. Gong, Y. Zhao, S. Cai, S. Fu, C. Yang, S. Zhang and X. Zhang, Single cell analysis with probe ESI-mass spectrometry: detection of metabolites at cellular and subcellular levels, Anal. Chem., 2014, 86, 3809–3816 Search PubMed.
- H. Zhu, G. Zou, N. Wang, M. Zhuang, W. Xiong and G. Huang, Single-neuron identification of chemical constituents, physiological changes, and metabolism using mass spectrometry, Proc. Natl. Acad. Sci. U. S. A., 2017, 114, 2586–2591 CrossRef CAS.
- H. Yao, H. Zhao, X. Zhao, X. Pan, J. Feng, F. Xu, S. Zhang and X. Zhang, Label-free mass cytometry for unveiling cellular metabolic heterogeneity, Anal. Chem., 2019, 91, 9777–9783 CrossRef CAS PubMed.
- R. Wang, H. Zhao, X. Zhang, X. Zhao, Z. Song and J. Ouyang, Metabolic discrimination of breast cancer subtypes at the single-cell level by multiple microextraction coupled with mass spectrometry, Anal. Chem., 2019, 91, 3667–3674 Search PubMed.
- Z. Fang, R. Wang, H. Zhao, H. Yao, J. Ouyang and X. Zhang, Mannose promotes metabolic discrimination of osteosarcoma cells at single-cell level by mass spectrometry, Anal. Chem., 2020, 92, 2690–2696 CrossRef CAS PubMed.
- Z. Li, Z. Wang, J. Pan, X. Ma, W. Zhang and Z. Ouyang, Single-cell mass spectrometry analysis of metabolites facilitated by cell electro-migration and electroporation, Anal. Chem., 2020, 92, 10138–10144 Search PubMed.
- Q. Liu, W. Ge, T. Wang, J. Lan, S. Martinez-Jarquin, C. Wolfrum, M. Stoffel and R. Zenobi, High-throughput single-cell mass spectrometry reveals abnormal lipid metabolism in pancreatic ductal adenocarcinoma, Angew. Chem., Int. Ed. Engl., 2021, 60, 24534 Search PubMed.
- K. Galichanin, Estimation of the variance components in TP53 mRNA expression in the rat lens after in vivo exposure to ultraviolet radiation B, Biomed. Hub, 2023, 8, 42–45 CrossRef PubMed.
- H. Arai, R. Wada, K. Ishino, M. Kudo, E. Uchida and Z. Naito, Expression of DNA damage response proteins in gastric cancer: comprehensive protein profiling and histological analysis, Int. J. Oncol., 2018, 52, 978–988 Search PubMed.
- R. Martin-Sanz, J. M. Sayagues, P. Garcia-Cano, M. Azcue-Mayorga, M. d. C. Parra-Perez, M. A. Pacios-Pacios, E. Pique-Duran and J. Feito, TP53 abnormalities and MMR preservation in 5 cases of proliferating trichilemmal tumours, Dermatopathology, 2021, 8, 147–158 CrossRef.
|
This journal is © The Royal Society of Chemistry 2025 |
Click here to see how this site uses Cookies. View our privacy policy here.