DOI:
10.1039/D4SD00360H
(Critical Review)
Sens. Diagn., 2025, Advance Article
Minimally and non-invasive glucose monitoring: the road toward commercialization
Received
4th December 2024
, Accepted 21st January 2025
First published on 21st January 2025
Abstract
The increasing global prevalence of diabetes has led to significant advancements in glucose monitoring technologies. Since the introduction of enzyme-based glucose biosensors in the 1960s, these devices have evolved to enable real-time and dynamic glucose monitoring, with electrochemical biosensors playing a crucial role. Recent innovations have expanded glucose sensing into non-invasive and minimally invasive methods, utilizing optical, millimeter wave, ultrasound, and bioimpedance techniques to provide user-friendly and painless alternatives. This review examines the current state and future prospects of glucose monitoring technologies, particularly focusing on wearable sensors for in vivo applications. It explores the key mechanisms of electrochemical and alternative sensing methods, highlighting their evolution, adaptability to different biofluids, and integration into multiplexed systems for improved diabetes management. Emerging wearable devices offer continuous and real-time feedback, which is essential for effective glucose regulation. The review also addresses challenges such as biocompatibility, accuracy in fluctuating physiological conditions, and external factors that can affect sensor performance. Furthermore, it analyzes the commercial landscape, from established continuous glucose monitors to next-generation technologies, highlighting opportunities for personalized care. The aim of this review is to guide future research in developing advanced and efficient glucose monitoring solutions tailored to meet patient needs.
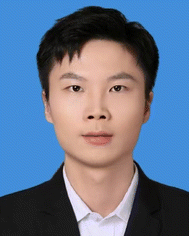 Shunhua Min | Shunhua Min is currently a master's student at Shenzhen University. His research focuses on the development of biosensing systems for wearable applications. After graduating from the undergraduate program, he continued to engage in research work at the Guangdong Laboratory of Artificial Intelligence and Digital Economy (SZ) in 2023. |
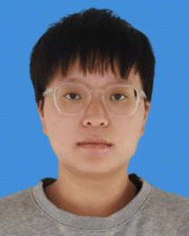 Haoyang Geng | Haoyang Geng is currently a Master's student in the Computer Science and Technology program at the Guangdong Laboratory of Artificial Intelligence and Digital Economy (SZ). Her research focuses on wearable electronic devices and flexible biosensors. |
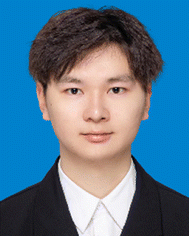 Yuheng He | Yuheng He is a Master's student at Shenzhen University, specializing in sensors and algorithms for human vital sign monitoring. He earned his Bachelor's degree in Software Engineering from Sichuan University of Science and Engineering and is currently pursuing a Master's degree in Computer Science at Shenzhen University. His research aims to develop advanced sensor technologies and algorithms to improve the accuracy and efficiency of health monitoring systems. |
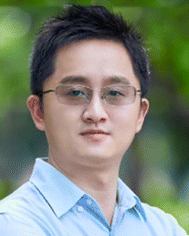 Tailin Xu | Tailin Xu is an associate professor at the Institute of Advanced Science, Shenzhen University. He joined the Beijing Key Laboratory for Bioengineering & Sensing Technology research group at University of Science & Technology Beijing to pursue his PhD degree under the supervision of Prof. Xueji Zhang and Prof. Shutao Wang, where he studied until 2017. In 2013, he joined Joseph Wang's group as a joint PhD student at the University of California, San Diego, where he studied until 2015. His research interests include wearable sensors, biointerfaces and functional nanomotors. |
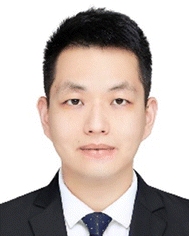 Qingzhou Liu | Qingzhou Liu is an associate professor at Guangdong Laboratory of Artificial Intelligence and Digital Economy (SZ), Shenzhen. He obtained his PhD from University of Southern California. He has worked at Huawei Inc. as a Sr. scientist. His research interests include wearable sensors, mimetic bioelectronics and biomedical instruments. |
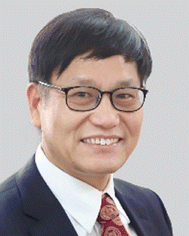 Xueji Zhang | Xueji Zhang is a professor of Shenzhen University. He obtained his PhD from Wuhan University in 1994. He was a postdoc fellow at the National Institute of Chemistry, Slovenia, Swiss Federal Institute of Technology (ETH), Zurich, and New Mexico State University from 1995 to 1999. He has worked at World Precision Instruments Inc. as a Sr. scientist, head of the chemistry department, and Sr. Vice President of Science since 1998. His research centers on electrochemistry, bioanalysis, author biographies click here to view linked references micro/nanosensors, and biomedical instrumentation. |
1. Introduction
Diabetes mellitus (DM) has become a significant global health issue. Currently, over 422 million people worldwide are affected by this chronic metabolic disease, which means approximately one in ten adults is affected, and this proportion continues to grow. Diabetes seriously affects human health, and poorly controlled blood sugar levels can lead to various complications such as neuropathy, cardiovascular diseases, renal failure, and retinopathy, causing great suffering to patients and even jeopardizing their lives.1 Diabetes is caused by insufficient insulin production by the pancreas or inadequate utilization of insulin by the body's cells.2 DM can be roughly classified into type 1 (T1D), type 2 (T2D), and gestational diabetes based on the way and time of onset.3 Type 1 diabetes is caused by insufficient insulin production, leading to a sudden drop in blood sugar levels, while type 2 diabetes results from inadequate insulin utilization, causing a rise in blood sugar levels.4 Gestational diabetes is a medical complication occurring during pregnancy.5 Current clinical methods cannot cure diabetes, and the main ways to alleviate and control it include insulin injections, taking antidiabetic medications, maintaining physical activity, healthy eating, weight management, and regular blood sugar monitoring.6
For diabetes, a progressive disease, early diagnosis, effective treatment, and ongoing management are crucial for slowing disease progression and helping patients maintain optimal quality of life.7 Accurate diagnosis and comprehensive management are essential for effective treatment and the quality of life of diabetes patients. Various invasive and non-invasive technologies used for diagnosing and managing diabetes each have their advantages and disadvantages. While invasive methods like self blood glucose monitoring (SBGM) and continuous glucose monitoring (CGM) provide precise blood sugar measurements, they can cause discomfort and carry the risk of infection and tissue damage.8 Minimally invasive and non-invasive methods, designed to reduce patient discomfort and enhance compliance, have received significant attention and development in recent years. The importance of efficient blood sugar monitoring technologies extends beyond medical treatment, affecting lifestyle changes, treatment decisions, and overall health.9 By continuously or periodically testing blood sugar levels, the impact of medications, diet, and exercise on blood sugar levels can be more effectively assessed. This enables patients to timely adjust their treatment plans, keep blood sugar within target ranges, reduce or delay the onset of diabetes complications, and improve their quality of life.10
The field of minimally invasive and non-invasive blood glucose monitoring technology has garnered significant attention and is continuously evolving. Researchers are dedicated to developing a method for continuous blood glucose level monitoring to achieve minimal or painless experiences, while integrating artificial intelligence technology to provide comprehensive glucose assessment.11 This review evaluates minimally invasive and non-invasive blood glucose monitoring technologies, detailing their mechanisms, advantages, limitations, and clinical applications. It aims to enhance understanding of both current and emerging technologies, providing insights for researchers, clinicians, and industry stakeholders. By examining the development of glucose monitoring technologies, this review highlights future directions and innovative opportunities, contributing to better diabetes management and improved patient outcomes.
In recent years, there has been a surge in the demand for innovative blood glucose monitoring methods. Scientists and researchers have been actively exploring and developing a range of new non-invasive and minimally invasive blood glucose monitoring technologies, encompassing areas such as sweat,12,13 tears,14,15 saliva,16,17 interstitial fluid,18,19 blood vessels,20,21 and physical methods,22,23 as shown in the Fig. 1. These new technologies not only offer more convenient and comfortable blood glucose monitoring methods but also have numerous potential advantages over traditional finger-prick blood glucose monitoring. In the following section, we will provide a detailed introduction to these new technologies.
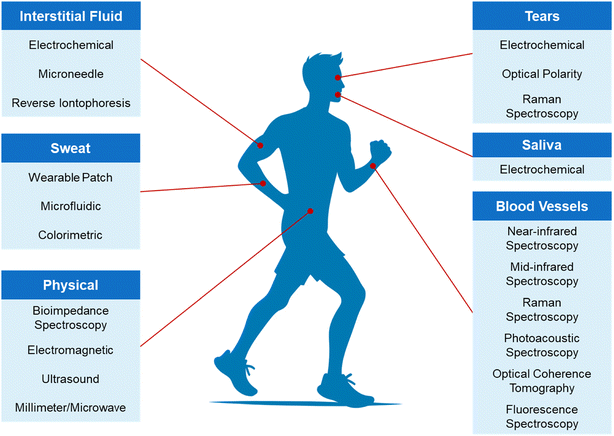 |
| Fig. 1 Classification of minimally and non-invasive glucose sensing techniques. | |
2. Glucose monitoring methods
The key to effectively managing diabetes lies in understanding and monitoring blood sugar levels. For a healthy individual, the blood sugar concentration should fall within the range of 70 mg dL−1 (3.9 mmol L−1) to 180 mg dL−1 (10 mmol L−1).24 Biomarkers like glycated hemoglobin (HbA1c) and glycated albumin provide valuable insights into blood sugar levels, enabling personalized diabetes management.25 Tracking the glycemic index of food intake is crucial, as it directly impacts blood sugar levels.26 Foods with a high glycemic index lead to rapid spikes in blood sugar, while those with a low glycemic index result in slower, steadier increases. Therefore, carefully monitoring calorie intake and carbohydrate consumption is vital for effective diabetes management. Additionally, continuous measurement of key diabetes biomarkers, such as insulin and ketones, is essential for successful diabetes management.27 It's important to note that insulin, a hormone that regulates glucose uptake into cells, plays a critical role in diabetes management.28 Keeping insulin levels in check is crucial, as excessive insulin secretion is common in the early stages of type 2 diabetes.29 By proactively monitoring and adjusting insulin levels, individuals can significantly improve their diabetes management and overall quality of life. Moreover, maintaining a healthy level of physical activity and fitness can positively influence basal metabolic rate and glucose metabolism, ultimately contributing to better diabetes management.30
2.1. Conventional methods
The self-monitoring of blood glucose (SMBG) is a vital component of diabetes management. It involves collecting a small blood sample from the fingertip and using electrochemical methods to analyze the glucose level, providing immediate information about the user's blood sugar level.31 Traditional portable blood glucose monitoring devices consist of three main parts: an electrochemical sensor, a measuring instrument, and a display. After obtaining a blood sample, the glucose oxidase in the electrochemical sensor reacts with the blood, producing an electric current signal that is directly proportional to the glucose concentration.32 This result is displayed on the device's screen for the user's reference and record. Despite its effectiveness, this method has limitations. It requires multiple daily blood collections using needles, causing discomfort and potential infection. Additionally, it does not provide continuous blood glucose monitoring data. Therefore, an ideal blood glucose testing method should offer continuous monitoring and be minimally invasive or non-invasive.
The rapid advancements in sensor and enzyme technology have given rise to the revolutionary continuous glucose monitoring technology. CGM systems represent a cutting-edge approach to glucose monitoring, involving the continuous tracking of a patient's glucose levels through the implantation of a sensor under the skin.33,34 Comprising a sensor, transmitter, and display device, the CGM system functions by implanting the sensor in subcutaneous tissue to monitor glucose levels in the interstitial fluid. This sensor reacts with glucose molecules, generating a current signal through an electron transfer. The transmitter then transmits the data to an external device, where real-time blood glucose values are calculated using algorithms, enabling users to stay informed about their blood glucose levels at all times.35 The key advantage of CGM lies in its ability to provide 24 hour continuous monitoring, timely detection of glucose fluctuations and trends, and alerts for high and low blood glucose levels, thereby aiding in the prevention of severe blood glucose fluctuations.36 Notably, CGM is adept at detecting covert high and low blood glucose levels that traditional testing methods may miss, particularly in monitoring postprandial hyperglycemia and nocturnal asymptomatic hypoglycemia.37 However, it is important to note that the sensors of the CGM system require regular replacement (approximately every 14 days) and entail high usage costs. Furthermore, CGM systems carry the risk of infection and sensor failure due to long-term wear, as well as potential inaccuracies in blood glucose data caused by physical activity, collisions, and medication interference.
The traditional methods for blood sugar testing, such as the oral glucose tolerance test (OGTT) and the hemoglobin A1c (HbA1c) test, are widely used for diagnosing diabetes and evaluating blood sugar levels.38,39 While the OGTT provides a comprehensive assessment of glucose tolerance and insulin response, it is time-consuming and may cause discomfort for some patients.40 On the other hand, the HbA1c test offers a convenient and reliable way to measure long-term blood sugar control but may not capture short-term fluctuations and could be affected by certain health conditions.41 Each method has its advantages and limitations, and understanding these differences can help individuals and healthcare professionals make informed decisions about managing diabetes and monitoring blood sugar levels effectively.
In conclusion, traditional methods of blood glucose monitoring include self-monitoring, continuous monitoring, oral glucose tolerance testing, and glycated hemoglobin testing. While these methods play a crucial role in the diagnosis and management of diabetes, they still have inherent limitations and drawbacks. Therefore, the development of new blood glucose monitoring technologies is particularly necessary. The development of new technologies should focus on improving the shortcomings of existing technologies and exploring innovative blood glucose monitoring methods, such as non-invasive/minimally invasive monitoring technologies based on optical, acoustic, or biosensors. This will further enhance device comfort and wearability, reduce usage costs, and improve accuracy and stability.
2.2. Electrochemical methods
The development of electrochemical glucose biosensors has evolved through four main stages based on different electron transfer mechanisms, including the classic glucose oxidase (GOx) electrode sensor, the mediator GOx electrode sensor, and the direct GOx electrode sensor, and non-enzyme glucose sensor, as shown in Fig. 2.
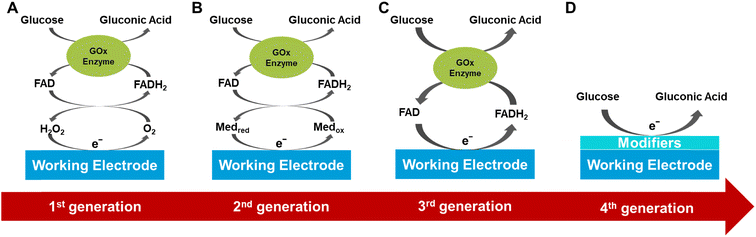 |
| Fig. 2 Schematic representation of different generations of electrochemical glucose sensors' sensing mechanisms. (A) Classic glucose oxidase electrode sensor, (B) mediator GOx electrode sensor, (C) direct GOx electrode sensor, and (D) non-enzyme glucose sensor. | |
The first generation of glucose sensors used glucose oxidase and oxygen as the enzyme's co-factor to measure glucose concentration by detecting the hydrogen peroxide (H2O2) produced during the glucose oxidation reaction.42,43 However, these sensors had limitations as they relied on the availability of oxygen in the environment and were prone to interference from other electroactive substances. To overcome these limitations, researchers made various improvements such as using diffusion-limiting polymer membranes, oxygen-rich carbon pastes, and enzyme modifications.44 Although these improvements partially addressed the oxygen dependence and interference issues, they still restricted the application of first-generation sensors.
The second generation of enzyme-based biosensors introduced synthetic redox mediators to replace natural oxygen as the electron acceptor, thus overcoming the dependency on oxygen.45 These redox mediators facilitate rapid and reversible electron transfer between the enzyme's redox center and the electrode surface, reducing the operating potential, eliminating oxygen dependence, and minimizing interference.46,47 Ferrocene was one of the earliest discovered redox mediators used for glucose oxidase, and second-generation glucose sensors achieved significant commercial success in the field of personal glucose test strips.48 Ideal redox mediators should allow selective electrochemical reactions at lower potentials, exhibit high chemical stability, rapid response with the enzyme (relative to oxygen), and low solubility in aqueous solutions. Other common redox mediators include quinones, tetrathiafulvalene, methylene blue, ferricyanide, thionine, transition metal complexes, and ruthenium-containing redox hydrogels.49,50 Although second-generation sensors performed well in ex vivo glucose testing, concerns exist regarding the leaching of redox mediators in continuous in-vivo monitoring applications, which could lead to stability and toxicity issues.
The third generation of glucose sensors eliminated the need for redox mediators and relied on direct electron transfer (DET) mechanisms.51,52 These sensors depend on the direct electron transfer between the enzyme's active site (e.g., flavin adenine dinucleotide (FAD)) and the electrode. And one of them combines a three-dimensional layered porous gold hydrogel–enzyme electrode with high biocompatibility, activity, and flexibility with high-precision, mass-producible soft-MEMS technology.53 However, the thick insulating protein layer of the enzyme (e.g., approximately 13 Å for GOx) may hinder electron transfer, resulting in a direct electron tunneling effect from the FAD redox center to the electrode.54 Research on these sensors is ongoing, but compared to sensors based on oxygen or redox mediators, third-generation sensors have not yet been widely adopted. The main challenge of third-generation sensors lies in achieving stable direct electron transfer while maintaining the enzyme's activity and stability. Although these sensors theoretically offer higher sensitivity and specificity, their complex manufacturing processes and higher costs limit their widespread use.55
The fourth generation of glucose sensors, based on non-enzymatic electrodes modified with nanomaterials capable of conducting enzyme-like reactions under extreme conditions, has improved the performance of glucose sensors.56 There have been numerous reports on non-enzymatic glucose sensors based on nanostructured metal, alloys, metal oxides, conductive polymers, MOFs, and other modified electrodes.57,58 The strategy designed by Li showed outstanding electrocatalytic activity and stability during glucose oxidation under neutral conditions.59Although enzymatic glucose sensors have successfully addressed the oxygen issue, concerns about the stability of natural enzymes remain. Non-enzymatic glucose sensors have several advantages over enzymatic sensors, including cost-effectiveness, high sensitivity, and long-term stability.60 However, the performance of metal-based sensors can be significantly affected by the absorption of reaction intermediates during the glucose oxidation process. Therefore, the choice and structure of nanomaterials have a significant impact on the performance of non-enzymatic sensors.61 To enhance glucose catalysis efficiency, efficient and synergistic nanomaterial combinations should be selected for glucose sensors.50
In summary, each generation of glucose sensors has its advantages and disadvantages. The first-generation technology is simple and cost-effective but is limited by oxygen dependence and interference issues. The second-generation technology overcomes oxygen dependence issues by introducing redox mediators but faces stability and toxicity issues related to mediator leaching. The third-generation technology, based on direct electron transfer, theoretically offers higher sensitivity and specificity but faces challenges in manufacturing and cost. The fourth-generation non-enzymatic sensor technology has stability, consistency, and selectivity issues related to nanomaterial detection of glucose. Future research efforts should focus on further optimizing these technologies to achieve more efficient, reliable, and convenient glucose monitoring.
2.2.1 Sweat-based glucose sensors. Sweat is a biological fluid secreted by the sweat glands in the skin's epidermal layer.62 Due to its rich chemical composition and easy accessibility, it has become an attractive alternative biofluid for non-invasive biomarker monitoring associated with epidermal wearable sensing devices.63 The glucose content in sweat is correlated with blood glucose levels, with glucose concentration in sweat varying within the 1 μM to 1 mM range.64 The working principle is using electrochemical sensors to detect glucose content in sweat. Once collected and corrected for individual variances, the glucose in sweat has been proven to accurately reflect the glucose level in the blood.65 One method involves integrating a glucose sensor onto a patch, with the sensing platform directly in contact with sweat on the skin's surface.66,67 Another method involves integrating it with a microfluidic channel containing pre-sampled sweat to transfer sweat to the sensing element.68In recent years, researchers have developed several wearable sensing devices for continuous measurement of sweat glucose levels.46 Fig. 3A shows a sweat glucose sensor integrated with a flexible self-powered watch, with the sensor conforming to the skin and directly measuring glucose in skin sweat.69 Fig. 3B utilizes tungsten cobalt (CoWO4)/CNT and polyaniline/CNT nanocomposite materials coated on a CNT–AuNS working electrode on a stretchable silicon substrate, with silver chloride nanowires serving as the reference electrode, used for wearable sweat, glucose, and pH sensing.70 Fig. 3C displays a three-electrode electrochemical platform based on elastic gold fibers, functionalized with Prussian blue and glucose oxidase for the working electrode, and modified with Ag/AgCl as the reference electrode, maintaining good sensing performance even at 200% strain.71 Fig. 3D reports a flexible wristband sensor array for multiplexed measurement of sweat glucose, directly connected to the skin to simultaneously measure electrolytes and metabolites, enabling measurement of body status during exercise.72 Fig. 3E shows a wearable glucose colorimetric sensor based on a microfluidic chip, where microchannels guide sweat excreted from the skin to microchambers containing pre-embedded glucose oxidase (GOD)-peroxidase-ortho-phenylenediamine reagents for detecting glucose in sweat.68 Fig. 3F demonstrates a wearable patch comprising glucose, pH, humidity, and temperature sensors, along with feedback-based drug delivery micro-needles.73 The patch is validated for estimating actual glucose concentration in sweat during exercise and delivering diabetes medication in a mouse model. Fig. 3G transports sweat from microfluidic channels to the glucose sensor with a module for sensor signal acquisition, processing, wireless transmission, and data display, demonstrating high stability over 10 hours.74 Fig. 3H introduces pH and temperature correction to accurately estimate sweat glucose concentration in the microfluidic device. Patch-type sweat sensors typically use soft materials attached to the skin surface, enabling direct contact with sweat and measurement of the concentration of biomolecules in it.75
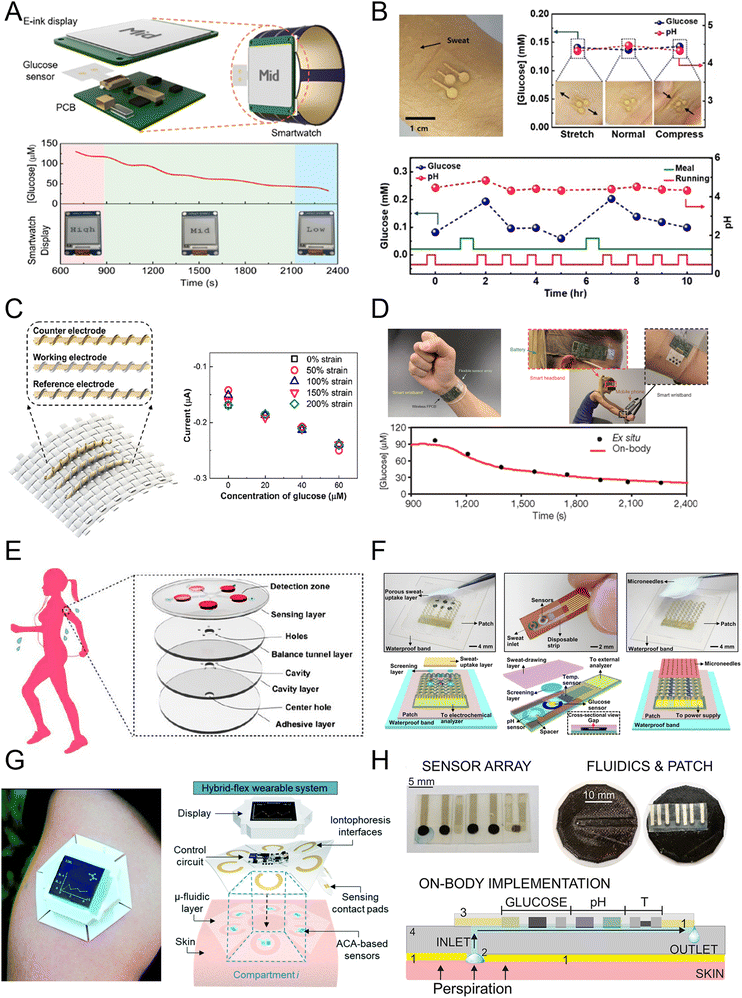 |
| Fig. 3 Electrochemical sweat glucose sensors including (A) sensors integrated in a watch (reprinted with permission from ref. 69. Copyright 2019 American Chemical Society), (B) sensors on a flexible substrate (reprinted with permission from ref. 70. Copyright 2018 American Chemical Society), (C) stretchable glucose sensor (reprinted with permission from ref. 71. Copyright 2019 American Chemical Society), (D) multiplexed sensing platform (reprinted with permission from ref. 72. Copyright 2016 Nature Publishing Group), (E) glucose sensor patch (reprinted with permission from ref. 68. Copyright 2019 American Chemical Society), (F) closed loop sensor system (reprinted with permission from ref. 73. Copyright 2017 American Association for the Advancement of Science), (G) microfluidic glucose sensor patch (reprinted with permission from ref. 74. Copyright 2020 Royal Society of Chemistry), (H) microfluidic sensor platform (reprinted with permission from ref. 75. Copyright 2020 American Chemical Society). | |
These patch-type sensors are suitable for prolonged wear, offering good comfort and durability, but may experience displacement or detachment in highly dynamic environments. Microfluidic sensors use microfluidic channel structures for precise control of sweat collection and analysis, enabling finer and controllable sample processing, achieving higher detection sensitivity and selectivity with high accuracy and stability. The advantage of sweat glucose monitoring technology lies in its non-invasive nature, allowing continuous monitoring at home or in daily life without the discomfort associated with traditional fingerstick blood sampling. However, sweat glucose monitoring technology is not suitable for all populations. For example, for individuals with dry skin or those who do not sweat easily, the glucose content in sweat may not accurately reflect blood glucose levels.76 Additionally, the glucose content in sweat does not always perfectly match blood glucose levels, indicating the need for further research and improvement to enhance the technology's accuracy and reliability.
2.2.2 ISF-based glucose sensors. Extracting interstitial fluid (ISF) from subcutaneous tissue to monitor blood glucose presents an appealing method for glucose detection.77,78 ISF closely correlates with biomarker concentrations in blood, providing reliable diagnostic information.79 The methods for accessing ISF include reverse iontophoresis (RI) and microneedle (MN) technology. RI induces iontophoretic flow by applying a weak electric current (0.2–0.5 mA cm−2) to the skin, promoting the migration of ions, analytes, and metabolites.80 Due to the negative charge of the skin physiologically, positively charged and neutral ions migrate to the cathode, while negatively charged molecules migrate to the anode. Glucose molecules have a neutral charge, thus they are collected for quantitative analysis on the cathode side during RI. Microneedle technology uses micro-scale needles to directly or indirectly (post-extraction sensing) monitor glucose concentration in ISF.81In the year 2000, researchers conducted a study on the collection of ISF using reverse iontophoresis for glucose detection (Fig. 4A).82 After applying a current of 0.25 mA cm−2, glucose from ISF was extracted into a sensing unit containing buffered saline solution. In 2011, the most convenient and practical microneedle-assisted glucose detection format based on two-component assembly was introduced, creating enzyme electrodes by immobilizing glucose oxidase and poly(aniline) conducting polymer film on a solid microneedle electrode (Fig. 4B).83 Fig. 4C shows an integrated system of RI and electrochemical sensing using a graphene miniaturized pixel array. This design utilizes channels in hair follicles to avoid sample dilution issues, enabling more accurate glucose monitoring.84 Fig. 4D demonstrates a hollow microneedle sensing system that simultaneously monitors blood glucose and ketones, allowing for better management of diabetes by detecting multiple glucose-related biomarkers.18 Fig. 4E presents a flexible, epidermal-wearable reverse iontophoresis glucose sensing system.85 This system integrates ultra-thin skin-like biosensors and electrochemical dual channels powered by paper batteries, diverting blood glucose from the blood vessels to the skin surface for detection. Fig. 4F developed a multiplex sensing system for various health-related markers, providing real-time analysis of glucose, lactate, and alcohol concentrations in ISF, applicable in various scenarios such as exercise, meals, and drinking.86
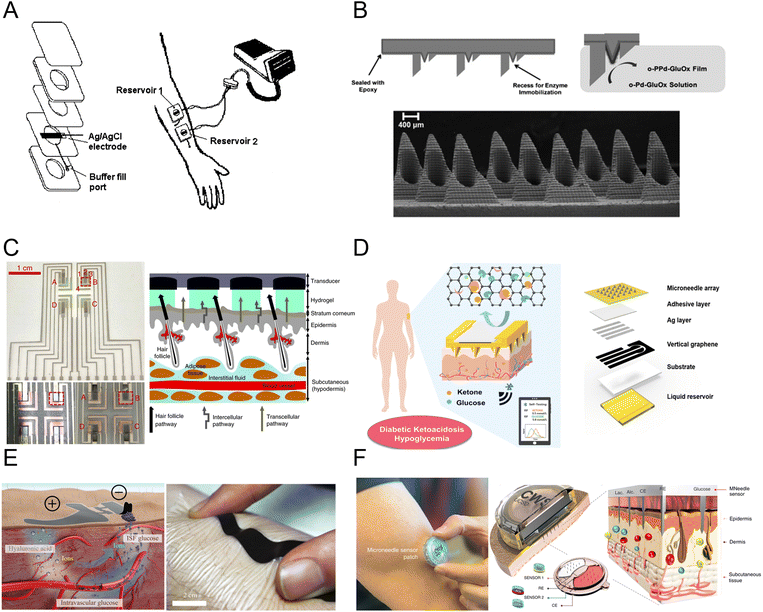 |
| Fig. 4 Electrochemical glucose sensors based on interstitial fluid (ISF). (A) First reverse iontophoresis (RI)-based glucose sensor (reprinted with permission from ref. 82. Copyright 2000 Wiley Inc.), (B) bicomponent microneedle (MN) array glucose sensor (reprinted with permission from ref. 83. Copyright 2011 Wiley Inc.), (C) fully integrated RI glucose sensing system (reprinted with permission from ref. 84. Copyright 2018 Springer Nature), (D) MN-based glucose and ketone sensors (reprinted with permission from ref. 18. Copyright 2024 American Chemical Society), (E) skin-like RI-based glucose sensor patch (reprinted with permission from ref. 85. Copyright 2017 American Association for the Advancement of Science), (F) multiplexed MN-based sensing system for glucose, lactate, and alcohol monitoring (reprinted with permission from ref. 86. Copyright 2022 Springer Nature). | |
ISF wearable sensors, using technologies like RI and microneedles, achieve the detection of biomarkers in ISF with advantages such as minimal invasiveness, effectiveness, and convenience, providing a reliable and comfortable option for glucose monitoring. Although ISF-based glucose detection technologies demonstrate tremendous potential for glucose monitoring, they still face challenges in commercial applications, such as the need for hydrogel membranes in RI to avoid direct contact between the skin and the iontophoresis region, as well as the potential skin irritation and discomfort associated with RI and MN.
2.2.3 Tear-based glucose sensors. Tears, as a non-invasive body fluid for glucose monitoring, are an attractive option due to their easy accessibility, continuous flow, and the correlation between tear glucose and blood glucose mediated by diffusion from the lacrimal artery.87 Based on these characteristics, the development of wearable tear glucose sensors has garnered widespread attention.88 Tear glucose sensors are primarily based on optical and electrochemical detection, with advantages such as rapid response, high sensitivity, and accuracy.89Early designs of tear glucose sensors aimed to achieve flexible and miniaturized structures to ensure user safety and comfort, since the eye is a delicate organ.14 In 2011, Parviz's team first described an electrochemical contact lens for tear glucose detection, as shown in Fig. 5A.90 This sensor composes of a transparent and soft polyethylene terephthalate substrate, titanium/palladium/platinum metal layers, and a titanium dioxide sol–gel membrane immobilized with glucose oxidase, exhibited high sensitivity and a good linear range with a detection limit below 0.01 mM. Fig. 5B presents a tear-based sensor developed using a graphene–silver nanowire hybrid structure in a non-invasive manner. This structure improved electrical and mechanical properties, and the transconductivity of this hybrid structure was minimal, rendering it suitable for use as an electrode in the fabrication of passive electronic components.91 Google and Novartis proposed developing a smart contact lens for continuous tear glucose monitoring, as shown in Fig. 5C.92 However, due to a lack of supporting evidence on the correlation between tear and blood glucose, the project was halted in 2018. NovioSense developed a spring-like miniaturized tear glucose sensor, reporting accuracy comparable to commercial CGM devices in phase II clinical trials, as shown Fig. 5D.93 Fig. 5E presents an integrated sensor combining glucose detection and intraocular pressure measurement.94 The smart contact lens, incorporating an LED display, enabled real-time tear glucose readings by switching off the LED when glucose levels in tears reached a threshold. Fig. 5F developed smart contact lenses can noninvasively monitor glucose levels in tears and deliver on-demand drugs for diabetic retinopathy treatment.95
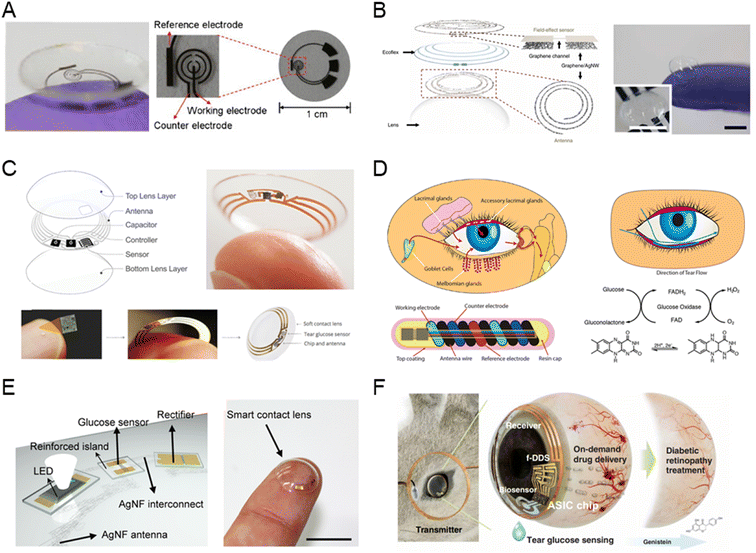 |
| Fig. 5 Tear-based glucose sensor. (A) An electrochemical glucose sensor integrated on a contact lens (reprinted with permission from ref. 90. Copyright 2011 Elsevier.), (B) graphene–silver nanowire based glucose sensor (reprinted with permission from ref. 91. Copyright 2017 Springer Nature.), (C) Google X's smart contact lens (images adapted from https://sites.google.com/site/smartcontactlens/. Reprinted with permission from ref. 92. Copyright 2015 WILEY-VCH Verlag GmbH & Co.), (D) spring-like glucose sensor developed by NovioSense (reprinted with permission from ref. 93. Copyright 2018 American Chemical Society), (E) contact lens for real-time glucose sensing integrated with LED for data feedback (reprinted with permission from ref. 94. Copyright 2018 American Association for the Advancement of Science), (F) contact lens for glucose detection and on-demand drug delivery (reprinted with permission from ref. 95. Copyright 2020 American Association for the Advancement of Science). | |
Although the field of tear glucose sensors has progressed rapidly, certain barriers must be addressed before practical application. Firstly, sensor safety is a primary concern, necessitating toxicity evaluation for human use. Additionally, the optical transparency and mechanical stretchability of the sensors require further optimization. Power supply also remains an issue, as many devices rely on external power sources, highlighting the future need for self-powered smart contact lenses. Finally, large-scale validation studies are essential to establish the correlation between tear and blood glucose concentrations.
2.2.4 Saliva-based glucose sensors. Saliva has emerged as a strong candidate for non-invasive monitoring because it can be easily accessed without the need for skin punctures or causing adverse effects.96,97 It contains a variety of substances, including secretions from salivary glands, microbial components, and blood constituents that permeate through intercellular or transcellular pathways.98 As a result, the concentrations of analytes in saliva often correlate with those found in blood.99 However, it's important to note that the concentrations of these analytes in saliva are typically lower, despite saliva samples being generally larger than other fluids, such as interstitial fluid or blood.100 These characteristics, along with its accessibility and compositional diversity, position saliva as a valuable focus in fields such as biomedicine, nutrition, and health monitoring.As early as 1991, elevated glucose levels were observed in the saliva samples of diabetic patients, which opened up the possibility for non-invasive monitoring that avoids traditional needle pricks and blood draws.17 The wearable device designed to estimate salivary glucose was developed in the form of a salivary biosensor integrated into a mouthguard, as shown in Fig. 6A.101 This sensor consists of a platinum electrode modified with glucose oxidase, which detects glucose in artificial saliva through electrochemical methods. This allows for real-time, non-invasive glucose monitoring via a wireless measurement system. Fig. 6B illustrates a mouthguard biosensor specifically designed for non-invasive monitoring of salivary glucose. The biosensor is constructed on the surface of a mouthguard made from dental polyethylene terephthalate glycol (PETG), achieving high sensitivity for detecting the range of human salivary glucose levels.102 Fig. 6C presents a novel method for oral glucose detection, which involves a modified membrane that is sensitive to changes in pH, temperature, and glucose levels.103 This membrane was placed between two resonators and demonstrated by being attached to a human tooth. The sensor, based on a broadside-coupled split-ring resonator, includes a silk membrane along with a responsive hydrogel layer. The hydrogel expands by absorbing surrounding solvents, altering its thickness and dielectric constant, which in turn changes the resonance frequency and signal amplitude. This frequency shift in response to the presence of glucose highlights the sensitivity of the functionalized membrane to biomolecules such as glucose.
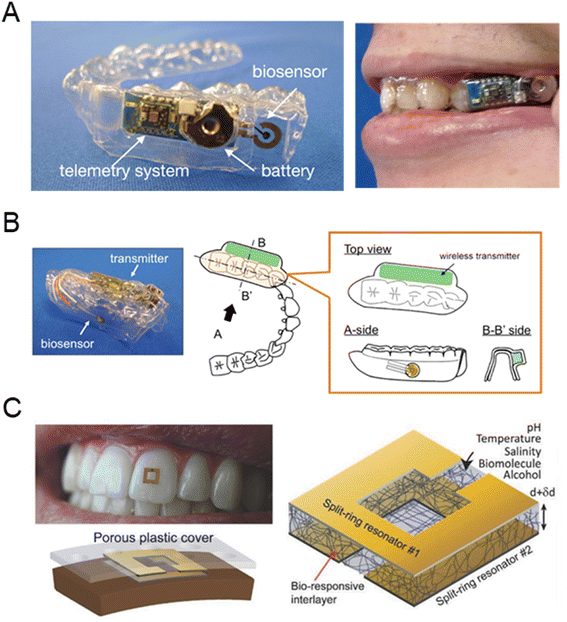 |
| Fig. 6 Saliva-based glucose sensor. (A) Wearable device for saliva glucose estimation (reprinted with permission from ref. 101. Copyright 2020 American Chemical Society), (B) mouthguard biosensor with telemetry system for glucose monitoring (reprinted with permission from ref. 102. Copyright 2016 Elsevier.), (C) wearable sensor on human teeth for the detection of biomolecules (reprinted with permission from ref. 103. Copyright 2018 Wiley-VCH Verlag GmbH & Co). | |
Detecting glucose in saliva with small wearable devices has the potential to create non-invasive methods for accessing biofluid data and detecting other analytes. However, challenges such as interference from food, device damage during eating, and sensor stability due to biofouling remain. User comfort is vital, as oral devices can be uncomfortable. While mouthguard-based sensors are beneficial for athletes, they are often impractical for daily use. Integrating devices into dental prosthetics or children's pacifiers offers a more comfortable solution. Future advancements should focus on miniaturizing these devices and enabling autonomous operation without external power, facilitating continuous glucose monitoring. Additionally, large-scale studies are necessary to accurately correlate saliva and blood glucose levels.
2.3. Optical methods
Optical sensors have emerged as a promising platform for non-invasive glucose monitoring, offering distinct advantages over traditional electrochemical biosensors by utilizing photon detection rather than electron transfer mechanisms.104 These sensors allow for continuous, real-time monitoring of glucose levels in biofluids through techniques such as near-infrared, mid-infrared, fluorescence, and Raman spectroscopy. Their ability to provide label-free, noninvasive detection with internal calibration has garnered significant attention, positioning optical sensors as a critical technology for developing reliable, user-friendly, and continuous glucose monitoring systems.105
2.3.1 NIR/MIR spectroscopy. In non-invasive blood glucose monitoring, near-infrared (NIR, 680–2500 nm) and mid-infrared (MIR, 2500–25
000 nm) wavelengths provide relatively ideal detection spectra,46 since ultraviolet light can be easily absorbed by DNA and proteins, while visible light is primarily absorbed by hemoglobin, and infrared light is absorbed by water.106 This allows for the analysis of molecular vibrations and rotations, leading to glucose concentration estimations.107 Analyzing these spectral features enables indirect measurements of blood glucose levels.108NIR and MIR spectroscopy, due to their non-invasive nature, significantly reduce patient discomfort and offer the capability to detect minor fluctuations in glucose concentration in biofluids, thereby facilitating continuous and real-time monitoring.109 NIR is remarkably versatile due to its ability to operate in reflection, interaction, and transmission modes. However, it faces challenges from physiological variables such as tissue thickness, temperature, and ambient light. Meanwhile, MIR spectroscopy excels in providing sharp response peaks with lower scattering, enhancing detection accuracy, but is constrained to reflection mode due to limited tissue penetration.110 The incorporation of advanced calibration models and multivariate analysis further enhances measurement precision.111
Fig. 7A presents a method using NIR spectroscopy for non-invasive, rapid blood glucose measurement in diabetic and normal rats. Comparing partial least squares (PLS) regression and artificial neural networks (ANN) showed both methods to be robust, accurate, and repeatable.112 Fig. 7B shows a semi-invasive NIR sensor integrating a microfluidic chip and microdialysis for continuous glucose monitoring, demonstrating a detection limit of 20 mg dL−1 in vitro and a MARE of 13.8% in vivo.113 As shown in Fig. 7C, MIR passive spectroscopic imaging enables remote glucose measurement by detecting glucose-induced luminescence from body thermal radiation. Wrist emission spectra correlated strongly with invasive glucose measurements over 60 minutes, offering the potential for real-time monitoring of diabetic patients to detect hypoglycemia or hyperglycemia.114 As shown in Fig. 7D, a MIR photoacoustic system using a single-wavelength quantum cascade laser at 1080 cm−1 has been developed for non-invasive glucose monitoring. Tested with artificial skin phantoms, it achieved a detection sensitivity of ±25 mg dL−1 across normal and hyperglycemic ranges.115
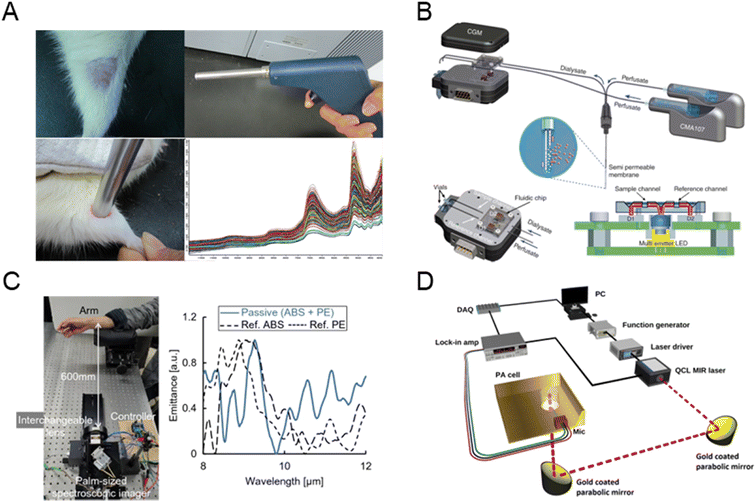 |
| Fig. 7 NIR/MIR spectroscopy-based glucose sensor. (A) Noninvasive in vivo blood glucose monitoring using NIR spectroscopy. Adapted with permission.112 Copyright 2017, Elsevier. (B) NIR sensor integrating a microfluidic chip and microdialysis for continuous glucose monitoring (reprinted with permission from ref. 113. Copyright 2014 Elsevier). (C) MIR imaging of the wrist for non-invasive glucose sensing (reprinted with permission from ref. 114. Copyright 2022 Springer Nature). (D) The setup used for glucose detection using MIR and PA spectroscopy (reprinted with permission from ref. 115. Copyright 2023 Springer Nature). | |
NIR and MIR spectroscopy techniques offer key advantages in glucose detection due to their non-invasive nature, high sensitivity, and specificity, eliminating the need for blood samples and thus reducing patient discomfort.116 They can detect minor glucose fluctuations and distinguish between various biomolecules, minimizing interference from other substances during detection. However, these techniques face challenges, as changes in blood glucose often produce only small spectral signal variations, requiring highly sensitive instruments for accurate detection. Additionally, interference from other sample components can complicate spectral analysis, while the high costs of equipment and the necessity for specialized personnel for operation and data analysis present further obstacles to widespread clinical use.117
2.3.2 Raman spectroscopy. Raman spectroscopy for non-invasive measuring blood glucose concentration is based on the changes in the frequency of scattered light caused by glucose.118 Its principle involves using a laser to illuminate the sample, which produces a Raman scattering spectrum.119 Specifically, when exposed to laser light, glucose molecules in the blood will vibrate and rotate, producing a distinct Raman spectrum with various peaks corresponding to different molecular vibration modes, such as C–C and C–H bonds.21 By measuring the intensity and frequency of these Raman peaks, the blood glucose concentration in the sample can be inferred.120 Due to the unique Raman spectral lines of glucose, this method provides high measurement accuracy.121 However, the glucose signal can be easily masked by the signals from collagen and triglycerides in the dermis.Fig. 8A presents a surface-enhanced Raman spectroscopy (SERS) sensor using a low-cost poly(methyl-methacrylate) (PMMA) microneedle array for in situ intradermal glucose detection, calibrated in skin phantoms and tested in diabetic mice.122 The sensor demonstrated rapid glucose measurement in interstitial fluid with minimal invasiveness, showing potential for painless glucose monitoring in diabetic patients. Fig. 8B illustrates fast and continuous glucose sensing using SERS emission shifts of mercaptophenylboronic acid (MPBA), demonstrating reversible MPBA–glucose bonding for stable performance over 30 days in the physiologically relevant range.123 This approach was further validated through intraocular glucose measurements in ex vivo rabbit eyes, achieving accuracy within ±0.5 mM of commercial sensors. Fig. 8C demonstrates the direct observation of glucose-specific Raman peaks in swine glucose clamping experiments, confirming a linear relationship between Raman peak intensities and reference glucose concentrations.121 A robust prediction method for glucose concentration, incorporating both glucose and skin-related Raman peaks, was developed and tested across single and multiple subject recordings.
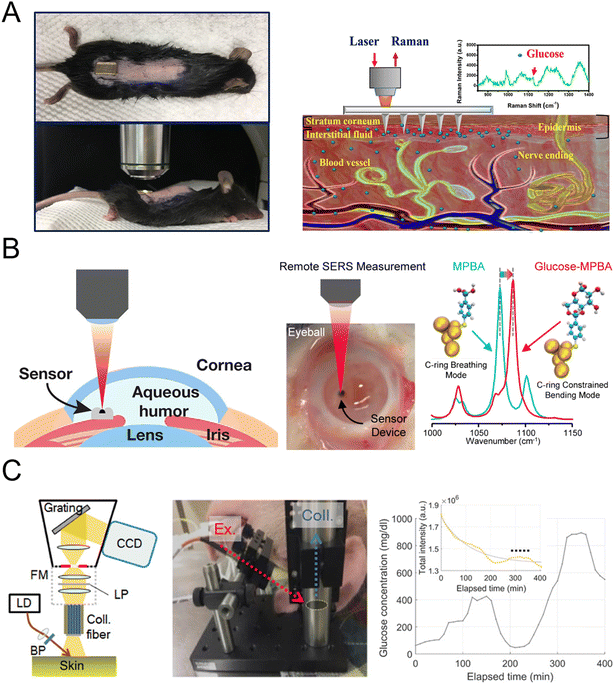 |
| Fig. 8 Raman-based glucose sensor. (A) Surface-enhanced Raman spectroscopy using microneedle array for in situ glucose detection (reprinted with permission from ref. 122. Copyright 2020 American Chemical Society). (B) Continuous glucose sensing using SERS emission shifts (reprinted with permission from ref. 123. Copyright 2018 American Chemical Society). (C) Direct observation of glucose fingerprint using in vivo Raman spectroscopy (reprinted with permission from ref. 121. Copyright 2020 American Association for the Advancement of Science). | |
The advantages of the Raman spectroscopy blood glucose detection technique include non-invasiveness, high sensitivity, and high specificity. Since it does not require blood samples, it minimizes discomfort for patients and is capable of detecting minute changes in glucose concentration. Furthermore, Raman spectroscopy's high specificity allows it to distinguish between different biomolecules, thereby reducing interference from other substances in the detection results.124 However, there are challenges associated with Raman spectroscopy for blood glucose detection. First, the relatively low concentration of glucose in the blood necessitates highly sensitive instruments to detect weak Raman signals. Additionally, interference from other components in the sample may affect the interpretation of Raman spectra and the accuracy of glucose measurements. Moreover, Raman spectrometers are expensive and require trained personnel for operation and data analysis, which limits their wider adoption and application in clinical practice.125
2.3.3 Optical coherence tomography (OCT). Optical coherence tomography (OCT) is a promising non-invasive technique for monitoring blood glucose levels using optical interference principles.126 Coherent light is directed onto the skin, and the backscattered light interacts with a reference signal to produce an interference pattern. By analyzing this pattern, OCT provides insights into tissue reflectivity and scattering, enabling the indirect estimation of glucose concentrations.127 Changes in glucose levels alter the refractive index and decrease the scattering coefficient, offering measurable optical markers for glucose monitoring.128The advantages of OCT include its non-invasive nature, high spatial and temporal resolution, real-time monitoring capability, and depth-resolving ability, making it suitable for various tissue types. These features allow OCT to detect glucose at different tissue layers, theoretically unaffected by physiological factors such as heart rate, blood pressure, osmolytes, or red blood cell concentration.129 However, the subtle effect of glucose concentration on optical properties poses a significant challenge, requiring highly sensitive equipment to detect minor signal variations. Furthermore, tissue-specific optical characteristics, such as vascular density and refractive index, along with motion artifacts and temperature fluctuations, can compromise measurement accuracy. While OCT offers depth-resolved glucose monitoring, these limitations hinder its development into reliable continuous monitoring devices. High equipment costs and the need for precise calibration further restrict its broader clinical adoption.130
2.3.4 Optical polarimetry (OP). Optical polarimetry for blood glucose detection is a non-invasive technique that relies on the optical rotation effect.131 This method measures changes in the optical rotation angle of polarized light passing through a sample to indirectly infer blood glucose levels. In this approach, glucose molecules in the blood interact with optically active substances, causing a shift in the rotation angle of polarized light. By measuring this change in optical rotation, glucose concentrations in the blood can be estimated.132The advantages of optical polarimetry for glucose monitoring include non-invasiveness, ease of use, and real-time results. It reduces patient discomfort by eliminating the need for blood samples, making it suitable for both clinical and home settings. Additionally, this method requires minimal sample preparation and can work with various biological samples. However, there are challenges.133 Other blood components, such as hemoglobin and cholesterol, can interfere with optical rotation measurements. Since glucose-induced changes in optical rotation are minor, highly sensitive equipment is needed to detect these subtle shifts. The technology is also costly and can be affected by environmental factors like light intensity and temperature, which may impact measurement accuracy and stability. Further research is needed to reduce delay times and address the technique's high sensitivity to movement, temperature, interfering compounds, and pH levels.134
2.3.5 Fluorescence. Fluorescence-based glucose sensing relies on fluorophores emitting light at specific wavelengths after absorbing radiation, with fluorescence intensity or decay time correlating to glucose concentration.135 To enhance selectivity and reversibility, intermediary molecules like enzymes, boronic acid derivatives, or quantum dots (QDs) are used, enabling techniques such as fluorescence resonance energy transfer (FRET) or ratiometric sensing.136Advanced designs, including carbon dots (CDs), fluorescent probes, and dual enzymatic reactions, improve sensitivity and allow glucose quantification in biofluids like blood and tears. These methods leverage changes in fluorescence ratios caused by glucose oxidation products, offering high sensitivity, specificity, and visual feedback, with detection limits reaching as low as 0.08 μM. While ratiometric fluorescence biosensors provide gradient visual feedback for glucose levels, quantitative precision remains a challenge for further development.137
Fig. 9A presents a fluorescent and colorimetric glucose sensor using metal oxide hybrid nitrogen-doped carbon dots (MFNCDs), leveraging their peroxidase-like activity to catalyze glucose oxidation and enable quantitative detection with high sensitivity. The sensor exhibited excellent biocompatibility, low cytotoxicity, and potential for on-site glucose analysis via smartphone integration, making it suitable for real serum applications.138 Fig. 9B shows smart contact lenses with flexible hydrogels have been developed for real-time, non-invasive glucose monitoring in tears, utilizing a glucose-sensitive fluorescent probe to detect concentrations ranging from 23 μM to 1.0 mM. The lenses enable smartphone-based RGB signal quantification, offering a biosafe and painless platform for continuous glucose monitoring.139 As shown in Fig. 9C, aniline-functionalized graphene quantum dots (a-GQDs) modulated with phenyl boric acid (PBA) were developed as a fluorescent glucose sensor, offering high sensitivity and selectivity through fluorescence quenching. The sensor's versatility was demonstrated with paper-based devices for portable glucose detection, fiber optic sensors for fast on-site applications, and wearable hydrogel films, all showcasing excellent performance and biocompatibility.140 In Fig. 9D, a wearable skin pad based on a ratiometric fluorescent nanohybrid has been developed for non-invasive, visual monitoring of sweat glucose, utilizing a red-to-blue fluorescence transition triggered by glucose oxidation. This biocompatible device demonstrates a strong correlation between fluorescence change and sweat glucose levels, enabling accurate detection of hyperglycemia in clinical tests.141
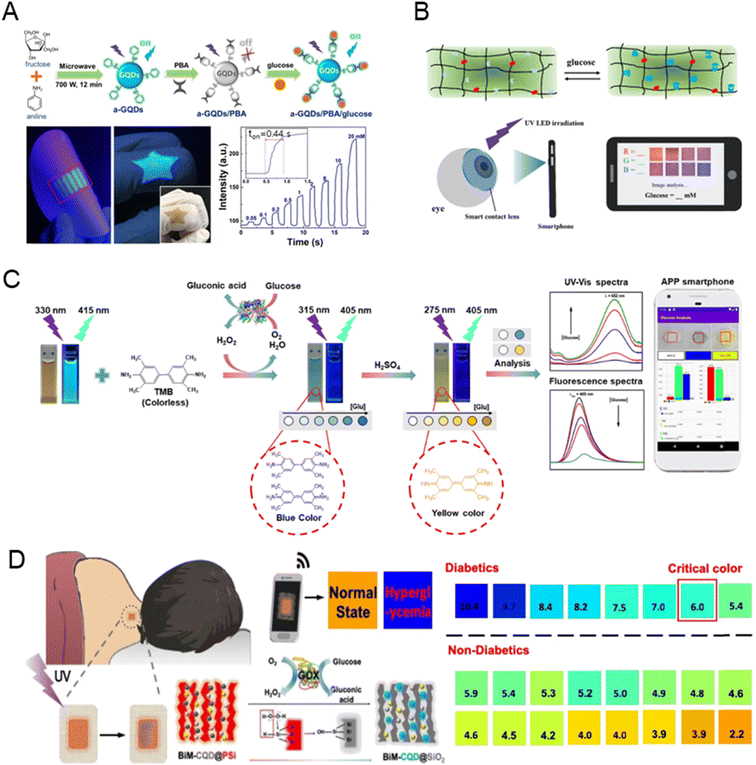 |
| Fig. 9 Fluorescence-based glucose sensor. (A) Paper- and fiber optic-based fluorescent sensor for glucose detection (reprinted with permission from ref. 138. Copyright 2021 Elsevier), (B) wearable fluorescent contact lenses for monitoring glucose (reprinted with permission from ref. 139. Copyright 2022 Elsevier). (C) Paper-based colorimetric and fluorescent glucose sensor (reprinted with permission from ref. 140. Copyright 2021 Elsevier), (D) ratiometric fluorescent nanohybrid for sweat glucose monitoring (reprinted with permission from ref. 141. Copyright 2020 Americal Chemical Society). | |
However, challenges exist, such as cell or protein buildup around the sensor or fibrous encapsulation post-implantation, which can reduce sensor accuracy and eventually cause it to fail in vivo. Enhancing the biocompatibility of these miniature biosensors remains a key focus in advancing implantable sensor technology.
Fluorescence glucose detection also has potential for commercial applications. Its operation involves fluorescent probes that either enhance or quench fluorescence when binding with glucose molecules, allowing quantitative glucose analysis through fluorescence signal changes.142 Commercially, several fluorescence-based glucose monitoring products are available, including portable devices and earring-style monitors, providing convenient glucose monitoring options for users.
This technology offers accurate glucose monitoring, with specific fluorescent probes ensuring high sensor specificity. Fluorescent materials like quantum dots and carbon dots are also commonly used in glucose detection, providing high fluorescence efficiency and photostability, which help enhance the accuracy and consistency of measurements.143 Yet, challenges remain. The accuracy and stability of fluorescence glucose detection still need improvement, especially as dynamic glucose changes can introduce errors. Environmental factors, such as light intensity and temperature, can affect the fluorescence signal and impact measurement accuracy. Additionally, the high cost of some commercial fluorescence-based glucose monitors may limit broader accessibility and adoption.
2.4. Other methods
2.4.1 Millimeter-wave (mmW) and microwave (MW). Millimeter wave (mmW) and microwave (MW) technologies are emerging techniques for non-invasive glucose monitoring.144,145 These methods utilize specific frequency ranges in the electromagnetic spectrum, particularly within dielectric spectroscopy. The frequencies used can penetrate deep into tissue, overcoming the limitations of optical methods, and enable accurate glucose detection through their interaction with glucose molecules. Glucose interacts with electromagnetic waves in various ways, including reflection, transmission, vibration (in resonant systems), and radar effects, depending on the frequency employed. This interaction allows for the determination of glucose concentration based on changes in energy levels.146The main advantages of mmW and MW sensing techniques include high sensitivity, rapid response times, flexibility, low power consumption, portability, and ease of manufacture.147 These technologies do not require precise alignment and are cost-effective for real-time measurements. The mmW spectrum, particularly between 30 and 300 GHz, has shown promise for in vivo glucose monitoring through skin tissue, with certain frequency bands proving to be particularly effective.148
However, it's important to note that while mmW and MW techniques are promising, their penetration depth could potentially cause tissue damage with repeated exposure, which limits their usefulness for continuous glucose monitoring.149 These sensing methods rely on the dielectric properties of biological tissues, which vary with fluctuations in glucose levels. Key interactions that enable glucose concentration detection include reflection, transmission, absorption, and resonant perturbation. Advances in micro-fabrication techniques are enhancing sensor designs, improving their sensitivity and accuracy. Nonetheless, challenges remain, such as the impact of environmental factors (like temperature and movement) and variations in tissue, necessitating ongoing refinement for practical and reliable glucose monitoring.
2.4.2 Ultrasound. The ultrasound method for non-invasive glucose monitoring utilizes the propagation time of ultrasound waves through biological media, where variations in glucose concentration influence wave speed.150,151 Specifically, increased glucose levels result in faster transmission speeds due to changes in acoustic impedance, which is affected by the density and compressibility of the tissue. This method offers several advantages, including the ability to penetrate deep into tissues, high sensitivity, and resilience to variations in skin color, making it a promising technology for glucose monitoring.While the ultrasound technique has limitations, such as high costs and sensitivity to temperature and pressure fluctuations, it remains a compelling option. The integration of ultrasound with other techniques, particularly near-infrared spectroscopy, enhances its potential for accurate and reliable glucose monitoring.152 Additionally, advancements in ultrasound-assisted mid-infrared spectroscopic imaging, which generates standing waves to create a reflective plane at a depth of approximately 100 micrometers, help mitigate issues related to water absorption and ultrasound attenuation. Despite these advancements, the impact of environmental temperature on measurement accuracy continues to pose challenges. Ongoing research and refinement are essential to improve the precision and reliability of this method for practical applications in glucose monitoring.153
2.4.3 Bioimpedance spectroscopy. Bioimpedance spectroscopy (BIS) measures glucose levels by applying an alternating electrical current to the skin and analyzing the resulting voltage or conductivity.153 At low frequencies (less than 5 kHz), BIS measures impedance, which includes resistance and reactance, as the electrical current interacts with various tissues and fluids. It detects changes in electrical properties, such as blood volume or the conductivity of red blood cells (RBCs), which are affected by variations in glucose concentration.154 These fluctuations are connected to changes in sodium (Na+) and potassium (K+) ion concentrations in plasma, which alter the permittivity and conductivity of RBC membranes.155BIS has several advantages, including being inexpensive, safe, reliable, fast, and compact, making it suitable for wearable applications. Recent advancements include the development of a biocompatible wearable ring and wrist device that can collect bioimpedance data while compensating for skin temperature and motion, addressing traditional challenges related to instability from sweat or movement.156 However, BIS also faces limitations, such as the difficulty in accurately predicting cell impedance due to cell membrane capacitance and sensitivity to external factors like sweat and temperature fluctuations. Despite these challenges, BIS shows promise as an accurate, real-time, and continuous glucose monitoring technique, especially with ongoing innovations in wearable device design aimed at enhancing stability and precision.157
3. Commercial products
The early reverse iontophoresis technology began in the early 2000s. Researchers used Plexiglas blocks to simulate the geometric structure of water gel pads for extracting glucose from interstitial fluid. By applying a current density of 0.25 mA cm−2 for 15 minutes, glucose was successfully extracted from the ISF into a sensor unit containing a buffered saline solution, and was detected electrochemically using an enzyme biosensor. This preliminary research laid the foundation for further development of non-invasive monitoring platforms. The GlucoWatch Biographer developed by Cygnus, Inc. was the first commercially approved non-invasive continuous glucose monitoring device by the FDA. The device integrates two conductive terminals, two gel pads loaded with glucose oxidase, a temperature sensor, a skin conductivity sensor, and an electrochemical sensor. It samples glucose from the ISF every 20 minutes for up to 12 hours. While the GlucoWatch showed good correlation in blood glucose monitoring, its long warm-up time (2–3 hours), daily calibration requirement, and inability to effectively detect hypoglycemic events in infants led to its market withdrawal. In recent years, the Wizmi™ NIR device has employed near-infrared spectroscopy (NIR) technology to measure blood glucose levels on wrist skin. In a prospective clinical trial, this technology demonstrated good system accuracy and high precision data among 32 healthy pregnant women.158 Additionally, the Tensor Tip CoG NIR device also uses NIR technology but measures blood glucose levels on fingertip skin. Although specific data has not been disclosed, this technology has achieved positive results in validating system accuracy.159 The C8 RI device offers convenient non-invasive blood glucose monitoring through the skin at the waist, but lacks specific technical details and clinical trial data.160 In contrast, the Gluco Beam™ RI provides high accuracy blood glucose data by measuring ISF at the fingertip for diabetic patients.161 This device utilizes ISF measurement technology and has shown good accuracy in practical clinical applications. The GlucoWatch G2 RI device can measure ISF on the wrist and provide continuous blood glucose data suitable for children and diabetic patients, with its performance validated in multiple clinical studies.162 The Sugar Beat™ RI adopts patch technology for minimally invasive measurements in ISF, suitable for diabetic patients, and has displayed MARD values ranging from 8% to 17%, meeting the standards for minimally invasive blood glucose monitoring.163 There are also devices that utilize other principle technologies for blood glucose measurement, which perform well. For example, the Gluco Track device combines electromagnetic waves, ultrasound, and multiple technologies to provide highly accurate blood glucose measurements and demonstrates good system accuracy.164 The Novio Sense device measures blood glucose in tear fluid using enzymatic methods to reduce discomfort for patients, targeted at certain diabetic patients.165 The Gluco Track from Integrity Applications Ltd. (Ashdod, Israel) and the egm1000™ earlobe device from Evia Medical Technologies Ltd. (Webridge, UK) aim to intermittently estimate blood glucose levels in type 2 diabetes patients, combining three different technologies, including ultrasound, electromagnetic RF waves, and thermography. Specifically, the egm1000™ has obtained CE certification and is currently available for purchase.166 The combination of multiple technologies can significantly enhance accuracy by reducing the chances of error and identifying and implementing corrections for environmental or physiological parameters that affect accuracy, both benefiting from advancements in machine learning to improve precision. The NIRLUS device offers high accuracy blood glucose measurement suitable for healthy individuals and diabetic patients.167 However, in multiple studies, this device showed MARD values of approximately 8% to 17% among diabetic populations; although in line with minimally invasive CGM levels, overall accuracy still needs improvement to better fit the requirements of different blood glucose ranges and populations. Clinical applications of sweat glucose monitoring technology in recent years, several companies and research teams have been dedicated to developing sweat glucose monitoring technology, which offers a painless, non-invasive method of monitoring blood glucose levels by detecting glucose levels in sweat. For instance, Persperion, a licensed company that has secured $4 million in seed funding, is developing a sensor that detects blood glucose through sweat, aiming for market launch in the coming years. Although current technologies need further research and development to improve accuracy and reliability, their potential lies in providing better blood glucose management and monitoring solutions for diabetic patients. Regarding tear fluid glucose detection, NovioSense has developed a miniaturized device that can be placed inside the eye to measure glucose levels in tear fluid for real-time monitoring. However, Google once attempted a similar project using smart contact lenses but abandoned it due to accuracy issues. Recent research has proposed detecting glycated albumin (GA) in tear fluid as a potential non-invasive method for monitoring blood glucose levels in diabetic patients; however, this technology is still in the exploratory stage. Additionally, DiaMonTech has developed a non-invasive device that uses infrared light to measure glucose levels in the skin.168 The GlucoWise sensor is designed to be placed between the thumb and index finger for blood glucose measurement and is still in development, with plans to integrate machine learning for improved accuracy. NovioSense is also working on a sensor that can be placed beneath the lower eyelid to monitor blood glucose levels in tear fluid, with data wirelessly transmitted to smartphones. At the same time, Indigo is developing a single sensor that is designed to measure a large number of metabolites. The small continuous multi-metabolite monitoring (CMM) sensor is implanted just under the skin and continuously measures metabolites in the body delivering best-in-class data to aid the management of chronic diseases.169 Biolinq170 provides painless blood glucose monitoring through sensing technology, while One Drop171 combines real-time blood glucose monitoring and intelligent health management to provide users with personalized recommendations. In addition, related studies such as Bai,172 Wang173 and Sun174 demonstrated the potential of these technologies to improve accuracy, stability, and adaptability to physiological changes, further supporting the application prospects of non-invasive blood glucose monitoring. The development of these new technologies demonstrates significant potential for providing accurate, non-invasive, and continuous blood glucose monitoring, helping to improve diabetes management and reduce the discomfort and risks associated with traditional blood glucose monitoring methods. The tissue interstitial fluid blood glucose monitoring method based on microneedle technology uses tiny, shallow microneedles to penetrate the skin and access subcutaneous interstitial fluid for blood glucose monitoring, offering a solution for continuous and real-time monitoring without the need for fingertip blood draws. Nonetheless, the accuracy and reliability of microneedle technology still require additional research and development to ensure its effectiveness in practical applications. Commercialized glucose monitoring devices are summarized in Table 1.
Table 1 Commericialized glucose monitoring devices
Device |
Principle |
Measurement site |
Measurement range |
Target population |
Operational duration |
MARD value |
Wizmi™158 |
NIR |
Wrist skin |
40–300 mg dL−1 |
Healthy individuals |
Up to 24 hours |
7.23% |
Tensor Tip CoG159 |
NIR |
Finger skin |
3.89–24.4 mmol L−1 |
Healthy individuals & diabetics |
Continuous during use |
14.5–18.1% |
C8 (ref. 160) |
Raman spectroscopy |
Waist skin |
— |
Healthy individuals |
Not specified |
— |
Gluco Beam™161 |
RI |
Finger ISF (interstitial fluid) |
— |
Healthy individuals |
Up to 12 hours |
— |
GlucoWatch G2 (ref. 162) |
RI |
Wrist ISF |
41–279 mg dL−1 |
Healthy individuals |
Up to 12 hours |
— |
Sugar Beat©163 |
RI |
ISF |
— |
Healthy individuals |
Up to 14 days |
8–17% |
K'Watch175 |
RI |
ISF |
— |
Healthy individuals |
Up to 7 days |
— |
Gluco Track164 |
Electromagnetic, ultrasound, MHC |
— |
4.17%–27.8 mmol L−1 |
Healthy individuals |
Continuous during use |
22.7% |
NovioSense165 |
Enzymatic |
Tear fluid |
— |
Animal models |
1–2 weeks |
— |
NIRLUS©167 |
— |
— |
70–300 mg dL−1 |
Healthy individuals |
Continuous during use |
10.7 ± 0.5% |
GWave176 |
RF |
— |
— |
Type 2 diabetes (5 cases) |
Not specified |
−4.787 mg dL−1 |
Dexcom177 |
CGM |
Subcutaneous |
40–400 mg dL−1 |
Diabetics |
10 days |
9.0% |
Abbott (Freestyle Libre)178 |
CGM |
Subcutaneous |
40–500 mg dL−1 |
Diabetics |
14 days |
9.4% |
Medtronic (Guardian Series)179 |
CGM |
Subcutaneous |
40–400 mg dL−1 |
Diabetics |
7 days |
8.7% |
Senseonics (Eversense)180 |
CGM (implantable) |
Subcutaneous (implantable) |
40–400 mg dL−1 |
Diabetics |
90 days |
8.5% |
DiaMonTech168 |
CGM |
ISF |
— |
Healthy individuals |
Continuous during use |
— |
GlucoWise181 |
— |
Finger skin |
— |
|
Continuous during use |
— |
Indigo169 |
CGM (implantable) |
ISF |
— |
Healthy individuals |
1 years |
— |
Biolinq |
CGM |
Subcutaneous |
— |
Healthy individuals |
— |
— |
One Drop |
CGM |
— |
— |
Diabetics |
— |
— |
Here are a few suggestions for new commercial products:
1. User experience and comfort: non-invasive devices offer the advantage of no skin punctures, reducing discomfort. New designs should prioritize comfort and ease of use, with wearable options like smart rings or contact lenses potentially appealing to younger or less frequent sensor-replacement users.
2. Accuracy and reliability: despite the appeal of non-invasive technologies, accuracy is critical. New devices must undergo rigorous clinical trials to prove reliability in varying conditions, especially for patients who use glucose data to adjust insulin.
3. Price and affordability: existing CGMs are expensive, limiting accessibility. Non-invasive devices could be more competitive with lower costs, achieved through optimized production and insurance partnerships for better pricing.
4. Personalization and intelligence: with AI and big data, future devices could offer personalized management, predicting trends and warning of blood sugar issues, enhancing user satisfaction and loyalty.
In short, for non-invasive devices to stand out, they must innovate in technology, user experience, pricing, and personalized services. As technology advances, non-invasive blood glucose monitoring has strong potential to become a key tool in diabetes management.
4. Multiplexing techniques in non-invasive glucose monitoring
Multiplexing techniques have become essential in non-invasive glucose monitoring, enabling the integration of multiple sensing modalities to achieve higher accuracy, sensitivity, and reliability. By combining different measurement principles, these approaches address the inherent limitations of single-sensor systems, such as low SNR, interference from other biological components, and sensitivity to physiological variability. We propose various multiplexing methods, including advanced combinations of spectroscopy, bioimpedance, photoplethysmography, and ultrasound, highlighting their principles, advantages, challenges, and potential for wearable applications.
Near-infrared spectroscopy + ultrasound
Near-infrared spectroscopy combined with ultrasound presents a compelling solution for glucose monitoring by optimizing both optical and acoustic measurement techniques. NIR offers non-invasive glucose estimation by measuring absorption at specific wavelengths, while ultrasound provides deep tissue penetration, improving the overall accuracy of glucose measurements. To enhance the system's SNR, researchers have developed compact quantum cascade lasers (QCLs) and tunable semiconductor lasers, narrowing the spectral width to mere nanometers. This precision reduces the influence of overlapping spectral components, which significantly improves measurement accuracy for glucose and other analytes, such as cholesterol and triglycerides. By measuring these trace components, the system can indirectly eliminate interfering variables, isolating glucose concentrations with greater reliability.
The NIR-ultrasound multiplexing approach is particularly advantageous for non-invasive applications due to its high sensitivity and tissue penetration depth, offering a robust solution for monitoring glucose in individuals with varying physiological conditions. However, as with any optical method, environmental factors and device complexity pose challenges, which are mitigated through careful device calibration and refinement of optical components.
Raman spectroscopy + bioimpedance spectroscopy
Raman spectroscopy, known for its high specificity due to unique glucose spectral peaks, can achieve precise glucose detection. With recent advances in Raman chip technology, Raman spectroscopy is now more accessible for commercial application. However, Raman-based systems often encounter stability issues under fluctuating environmental conditions, which can be addressed by combining them with bioimpedance spectroscopy (BIS).
BIS measures electrical impedance in body tissues, providing supplementary data on skin temperature and movement, which can compensate for the variability in optical measurements. By monitoring electrical impedance fluctuations, BIS captures real-time data on changes in blood volume and tissue properties that correlate with glucose levels.
The integration of Raman spectroscopy and BIS has promising applications in wearable glucose monitors, such as rings and wristbands, which could leverage both techniques to provide highly accurate, continuous, and real-time glucose readings. This multiplexed system's major advantage lies in its resilience to the physiological and environmental factors that commonly disrupt single-sensor devices. For instance, by accounting for variations in skin temperature and tissue hydration, this combined system can maintain consistent accuracy across different conditions.
Silicon photonics + photoplethysmography
Silicon photonics combined with PPG represents a low-cost and highly adaptable solution for non-invasive glucose monitoring. Silicon photonic chips emit laser light at targeted wavelengths, allowing for glucose estimation by analyzing the returned spectra from specific subcutaneous layers. Meanwhile, PPG measures blood volume changes through light absorption, providing a secondary data source relevant to glucose fluctuations.
The real-time blood flow dynamics captured by PPG offer critical contextual information that complements glucose readings from silicon photonics. For example, while photonic measurements provide baseline glucose levels, PPG tracks pulsatile changes in blood flow associated with glucose metabolism. This dual approach not only enhances the accuracy of glucose monitoring but also allows for real-time feedback, making it suitable for wearable devices.
One of the key advantages of this multiplexed technique is its low implementation cost, as it requires only minor modifications to existing wearable platforms. This versatility makes it an ideal candidate for integration into popular consumer wearables, such as smartwatches, potentially enabling large-scale adoption. Apple Watch, for instance, has been exploring similar methods, underscoring the viability of this technique for mass-market glucose monitoring.
Multiplexing techniques significantly improve non-invasive glucose monitoring but add complexity in design, data processing, and calibration. Advanced algorithms are needed to interpret data across sensing modalities, requiring machine learning for accurate calibration in diverse conditions. Environmental factors like temperature and light sensitivity affect optical and acoustic signals, while device miniaturization and low power consumption are essential for wearable comfort. Physiological variability further complicates accuracy, despite multiplexing's complementary data benefits, and the high cost of specialized components makes balancing performance with affordability crucial for widespread adoption.
The future of multiplexing in glucose monitoring will focus on refining sensor integration, data processing, and wearable design. Key advancements include adaptive machine learning to identify patterns and reduce noise, minimizing the need for invasive calibrations. Biocompatible materials and flexible electronics could enable comfortable wearables like smart rings and contact lenses, transforming continuous monitoring. Integrating multiplexed sensors with mobile devices for real-time analysis could also lower costs, making these technologies more accessible. Additionally, self-calibrating systems that adapt to each user's physiology would improve accuracy and convenience for long-term use. Together, these developments will enhance the accuracy, comfort, and affordability of non-invasive glucose monitoring.
5. Conclusions
First, we summarize the above glucose detection methods in Table 2. Furthermore, non-invasive glucose monitoring technologies (e.g., optical methods, millimeter wave sensing, and ultrasound) have the advantage of not requiring blood sampling, but face significant challenges in terms of accuracy and reliability, compared to non-invasive and traditional chemical technologies. Factors such as skin pigmentation, moisture, body composition, and environmental conditions (e.g., temperature and humidity) can affect their performance, resulting in greater variability and measurement errors compared to electrochemical sensors. While electrochemical biosensors remain the gold standard in clinical applications for their high accuracy (within 5–15% RMSE), real-time monitoring, and reliability, they are invasive and require frequent calibration. Non-invasive methods hold the promise of improving patient comfort, but are currently limited by reduced accuracy, individual variability, and the need for significant advances in sensor technology, calibration algorithms, and understanding of physiological factors. Further innovation is necessary for these technologies to complement or compete with traditional methods in clinical applications. Then, in this exploration of advanced glucose monitoring technologies, we have examined the evolution and potential of multiplexing techniques in non-invasive glucose monitoring. Multiplexing, which integrates multiple sensing modalities such as NIR spectroscopy, ultrasound, Raman spectroscopy, bioimpedance, and photoplethysmography, represents a significant leap forward, enhancing measurement accuracy, sensitivity, and robustness against physiological variability. Meanwhile, integrating glucose monitoring with other biomarkers into multiplexed systems has great potential to enhance disease management, especially in conditions such as diabetes, metabolic syndrome, and other chronic diseases. Lactate is an important biomarker of metabolic activity, and monitoring lactate can provide insight into cellular oxygenation and anaerobic metabolism. Elevated lactate levels may indicate tissue hypoxia or metabolic stress, which is particularly important in critical care settings or for patients with conditions such as sepsis or lactic acidosis. While integrating glucose monitoring with other biomarkers holds great promise, several technical challenges must be overcome to achieve accurate, reliable, and real-time measurements from multiple sensors in a single device. One of these challenges is the potential interference between different sensing targets, where glucose sensors can be affected by other molecules or substances present in the sample, resulting in inaccurate readings. To mitigate this, robust calibration methods and the use of selective sensors are required. Furthermore, multiplexed systems generate complex data from multiple sensing modalities, which requires sophisticated signal processing and data fusion algorithms to ensure accurate and meaningful information is extracted. Developing algorithms that can process and interpret data from different biomarkers in real time is a major challenge.
Table 2 Glucose sensing methods
Method |
Category |
Advantage |
Disadvantage |
Conventional methods |
Self-monitoring of blood glucose (SMBG) |
Real-time, accurate |
Frequent blood sampling required, discomfort, high risk of infection |
Continuous glucose monitoring (CGM) |
Continuous monitoring, early detection of glucose fluctuations |
High cost, sensor replacement needed every 14 days, risk of infection and sensor malfunction due to long-term wear |
Oral glucose tolerance testing (OGTT) |
Comprehensive evaluation of glucose tolerance and insulin response |
Time-consuming, potential discomfort for some patients, may not capture short-term fluctuations, affected by certain health conditions |
Glycosylated hemoglobin (HbA1c) measurement |
Convenient and reliable method to measure long-term blood sugar control |
May not capture short-term fluctuations, affected by certain health conditions |
Electrochemical methods |
Sweat-based glucose sensors |
Non-invasive, user-friendly, cost-effective |
Less accurate than other methods, affected by factors such as sweat composition and skin temperature |
ISF-based glucose sensors |
Non-invasive, provides reliable diagnostic information, accessible through simple procedures |
Limited accuracy |
Tear-based glucose sensors |
Non-invasive, real-time monitoring of tear glucose levels |
Safety issues and power supply challenges |
Saliva-based glucose sensors |
Non-invasive, easy sample collection, high sensitivity and specificity |
Limited accuracy |
Optical method |
NIR/MIR spectroscopy |
Non-invasive, fast measurement time, wide range of applications, high sensitivity and specificity |
Limited accuracy in certain situations, expensive equipment required, need for calibration and validation studies |
Raman spectroscopy |
High specificity, non-destructive, can detect multiple analytes simultaneously |
Low sensitivity, requires specialized instruments and expertise, prone to interference from other substances |
Optical coherence tomography (OCT) |
Non-invasive, high-resolution imaging, real-time monitoring, provides structural information about biological tissues |
Limited penetration depth, expensive equipment, interpretation of images requires expertise |
Optical polarimetry (OP) |
High sensitivity and specificity, can detect subtle changes in the polarization state of light, non-destructive measurement method |
Requires specialized equipment and expertise to interpret results, limited applicability in certain environments or situations |
Fluorescence |
Highly sensitive and specificity, provides visual feedback, can be used for quantitative analysis |
Can be affected by environmental factors such as temperature and pH |
Other methods |
Millimeter-wave (mmW) and microwave (MW) |
Non-invasive, high spatial resolution, suitable for monitoring physiological parameters in real-time |
Limited penetration depth, susceptibility to motion artifacts, signal interference from external sources |
Ultrasound |
Non-invasive, high spatial resolution, suitable for monitoring deep tissue structures |
High cost, sensitive to temperature and pressure fluctuations |
Bioimpedance spectroscopy |
Non-invasive, easy to use, provides information on tissue composition and hydration status |
Limited accuracy and sensitivity |
However, non-invasive glucose monitoring technology still has many issues that need to be addressed and solved.
About how to solve the critical problem of accuracy under fluctuating physiological conditions. It can be solved from the following aspects. Multiplexing technology: by combining multiple sensing modes, errors from a single sensor under complex physiological conditions can be reduced. Machine learning and adaptive algorithms: the machine learning models allows the system to dynamically calibrate based on individual physiological characteristics, minimizing measurement deviations caused by environmental factors like temperature and humidity. Advancements in sensor materials: the use of biocompatible materials and flexible electronic devices can reduce poor contact between the sensor and the skin, ensuring more stable signal transmission.
Non-invasive glucose monitoring is also affected by a variety of external factors. For example, temperature fluctuation is one of the main environmental factors that affect the accuracy of wearable sensors. Temperature changes can affect reaction rates, signal drift, and sensor sensitivity. To mitigate the effects of temperature, modern sensors are often equipped with temperature compensation. We recommend real-time monitoring of ambient temperature through a temperature sensor and dynamically adjusting the measured value through a compensation algorithm. Sweat caused by physiological factors such as exercise, weather, or stress can interfere with sensor readings, especially for electrochemical sensors. The concentration of electrolytes in sweat may change the electrochemical environment around the sensor, resulting in inaccurate readings. To address sweat interference, some wearable sensors now include sweat monitoring capabilities that allow algorithm-based compensation. Another effective strategy is to use waterproof coatings or hydrophobic materials to reduce sweat accumulation and minimize its impact on sensor performance. Food intake, especially high-glycemic index foods, can cause rapid fluctuations in blood glucose levels. These fluctuations are often reflected in the interstitial fluid, which is where many wearable glucose sensors work. We recommend designing sensors to automatically recalibrate after meals based on the expected post-meal blood glucose curve. Using machine learning algorithms to simulate an individual's post-meal blood sugar response, combined with historical data, can improve the accuracy of post-meal measurements.
In general, non-invasive glucose monitoring technology still has great development prospects. As long as we continue to pioneer and innovate, believe there will be better development.
Data availability
No primary research results, software or code have been included and no new data were generated or analyzed as part of this review.
Author contributions
S. M. conducted the statistical analysis of the current development status and completed the proofreading of the manuscript. Y. H. performed the statistical analysis and review of commercial products. H. G. explored and discussed future research directions. Q. L. led conceptualization, writing and analyses. T. X. and X. Z. assisted with analyses, writing and editing.
Conflicts of interest
The authors declare no conflict of interest.
Acknowledgements
This work was supported by funding from National Natural Science Foundation of China (22234006 and 62301325), Shenzhen University 2035 Program for Excellent Research (8690100000221), Joint Fund of the Ministry of Education for Equipment Preresearch (8091B022142), and Shenzhen Overseas Talent Program.
References
- A. D. Association, Standards of medical care in diabetes—2022 abridged for primary care providers, Clin. Diabetes, 2022, 40(1), 10–38 CrossRef PubMed.
- G. M. Reaven, Role of insulin resistance in human disease, Diabetes, 1988, 37(12), 1595–1607 CrossRef CAS PubMed.
- A. D. Association, Diagnosis and classification of diabetes mellitus, Diabetes Care, 2014, 37(Supplement_1), S81–S90 CrossRef PubMed.
- Z. Fu, E. R. Gilbert and D. Liu, Regulation of insulin synthesis and secretion and pancreatic Beta-cell dysfunction in diabetes, Curr. Diabetes Rev., 2013, 9(1), 25–53 CrossRef PubMed.
- H. D. McIntyre, P. Catalano, C. Zhang, G. Desoye, E. R. Mathiesen and P. Damm, Gestational diabetes mellitus, Nat. Rev. Dis. Prim., 2019, 5(1), 47 CrossRef PubMed.
- L. Ang, M. Jaiswal, C. Martin and R. Pop-Busui, Glucose control and diabetic neuropathy: lessons from recent large clinical trials, Curr. Diabetes Rep., 2014, 14, 1–15 CAS.
- J. B. Buse, D. J. Wexler, A. Tsapas, P. Rossing, G. Mingrone, C. Mathieu, D. A. D'Alessio and M. J. Davies, 2019 update to: management of hyperglycemia in type 2 diabetes, 2018. A consensus report by the American Diabetes Association (ADA) and the European Association for the Study of Diabetes (EASD), Diabetes Care, 2020, 43(2), 487–493 CrossRef CAS PubMed.
- B. Patel and R. Priefer, Infections associated with diabetic-care devices, Diabetes Metab. Syndr., 2021, 15(2), 519–524 CrossRef CAS PubMed.
- S. A. Siddiqui, Y. Zhang, J. Lloret, H. Song and Z. Obradovic, Pain-free blood glucose monitoring using wearable sensors: Recent advancements and future prospects, IEEE Rev. Biomed. Eng., 2018, 11, 21–35 Search PubMed.
- S. Clarke and J. Foster, A history of blood glucose meters and their role in self-monitoring of diabetes mellitus, Br. J. Biomed. Sci., 2012, 69(2), 83–93 CrossRef CAS PubMed.
- S. Ellahham, Artificial intelligence: the future for Diabetes Care, Am. J. Med., 2020, 133(8), 895–900 CrossRef CAS PubMed.
- J. Moyer, D. Wilson, I. Finkelshtein, B. Wong and R. Potts, Correlation between sweat glucose and blood glucose in subjects with diabetes, Diabetes Technol. Ther., 2012, 14(5), 398–402 CrossRef CAS PubMed.
- X. He, S. Yang, Q. Pei, Y. Song, C. Liu, T. Xu and X. Zhang, Integrated smart janus textile bands for self-pumping sweat sampling and analysis, ACS Sens., 2020, 5(6), 1548–1554 CrossRef CAS PubMed.
- J. T. La Belle, A. Adams, C.-E. Lin, E. Engelschall, B. Pratt and C. B. Cook, Self-monitoring of tear glucose: the development of a tear based glucose sensor as an alternative to self-monitoring of blood glucose, Chem. Commun., 2016, 52(59), 9197–9204 RSC.
- J. R. Sempionatto, L. C. Brazaca, L. García-Carmona, G. Bolat, A. S. Campbell, A. Martin, G. Tang, R. Shah, R. K. Mishra and J. Kim, Eyeglasses-based tear biosensing system: Non-invasive detection of alcohol, vitamins and glucose, Biosens. Bioelectron., 2019, 137, 161–170 CrossRef CAS PubMed.
- X. Jin, A. Cai, T. Xu and X. Zhang, Artificial intelligence biosensors for continuous glucose monitoring, Interdiscip. Mater., 2023, 2(2), 290–307 Search PubMed.
- W. Zhang, Y. Du and M. L. Wang, Noninvasive glucose monitoring using saliva nano-biosensor, Sens. Biosensing Res., 2015, 4, 23–29 CrossRef.
- Q. Wang, Q. Liu, G. Zhong, T. Xu and X. Zhang, Wearable Vertical Graphene-Based Microneedle Biosensor for Real-Time Ketogenic Diet Management, Anal. Chem., 2024, 96(21), 8713–8720 CrossRef CAS PubMed.
- E. De la
Paz, A. Barfidokht, S. Rios, C. Brown, E. Chao and J. Wang, Extended noninvasive glucose monitoring in the interstitial fluid using an epidermal biosensing patch, Anal. Chem., 2021, 93(37), 12767–12775 CrossRef PubMed.
- C. D. Malchoff, K. Shoukri, J. I. Landau and J. M. Buchert, A novel noninvasive blood glucose monitor, Diabetes Care, 2002, 25(12), 2268–2275 CrossRef CAS PubMed.
- R. Pandey, S. K. Paidi, T. A. Valdez, C. Zhang, N. Spegazzini, R. R. Dasari and I. Barman, Noninvasive monitoring of blood glucose with Raman spectroscopy, Acc. Chem. Res., 2017, 50(2), 264–272 CrossRef CAS PubMed.
- G. Freckmann, S. Pleus, M. Grady, S. Setford and B. Levy, Measures of accuracy for continuous glucose monitoring and blood glucose monitoring devices, J. Diabetes Sci. Technol., 2019, 13(3), 575–583 CrossRef CAS PubMed.
- A. Ciudin, C. Hernández and R. Simó, Non-invasive methods of glucose measurement: current status and future perspectives, Curr. Diabetes Rev., 2012, 8(1), 48–54 CrossRef CAS PubMed.
- M. J. Davies, V. R. Aroda, B. S. Collins, R. A. Gabbay, J. Green, N. M. Maruthur, S. E. Rosas, S. Del Prato, C. Mathieu and G. Mingrone, Management of hyperglycemia in type 2 diabetes, 2022. A consensus report by the American Diabetes Association (ADA) and the European Association for the Study of Diabetes (EASD), Diabetes Care, 2022, 45(11), 2753–2786 CrossRef CAS PubMed.
- S. E. Inzucchi, R. M. Bergenstal, J. B. Buse, M. Diamant, E. Ferrannini, M. Nauck, A. L. Peters, A. Tsapas, R. Wender and D. R. Matthews, Management of hyperglycemia in type 2 diabetes, 2015: a patient-centered approach: update to a position statement of the American Diabetes Association and the European Association for the Study of Diabetes, Diabetes Care, 2015, 38(1), 140–149 CrossRef PubMed.
- F. X. Pi-Sunyer, Glycemic index and disease, Am. J. Clin. Nutr., 2002, 76(1), 290S–298S CrossRef CAS PubMed.
- K. T. Nguyen, N. Y. Xu, J. Y. Zhang, T. Shang, A. Basu, R. M. Bergenstal, K. Castorino, K. Y. Chen, D. Kerr and S. K. Koliwad, Continuous ketone monitoring consensus report 2021, J. Diabetes Sci. Technol., 2022, 16(3), 689–715 CrossRef PubMed.
- M. S. Rahman, K. S. Hossain, S. Das, S. Kundu, E. O. Adegoke, M. A. Rahman, M. A. Hannan, M. J. Uddin and M.-G. Pang, Role of insulin in health and disease: an update, Int. J. Mol. Sci., 2021, 22(12), 6403 CrossRef CAS PubMed.
- P.-J. Guillausseau, T. Meas, M. Virally, M. Laloi-Michelin, V. Médeau and J.-P. Kevorkian, Abnormalities in insulin secretion in type 2 diabetes mellitus, Diabetes Metab., 2008, 34, S43–S48 CrossRef CAS PubMed.
- S. R. Colberg, R. J. Sigal, J. E. Yardley, M. C. Riddell, D. W. Dunstan, P. C. Dempsey, E. S. Horton, K. Castorino and D. F. Tate, Physical activity/exercise and diabetes: a position statement of the American Diabetes Association, Diabetes Care, 2016, 39(11), 2065 CrossRef PubMed.
- J. Hortensius, R. J. Slingerland, N. Kleefstra, S. J. Logtenberg, K. H. Groenier, S. T. Houweling and H. J. Bilo, Self-monitoring of blood glucose: the use of the first or the second drop of blood, Diabetes Care, 2011, 34(3), 556–560 CrossRef CAS PubMed.
- J. Wang, Electrochemical glucose biosensors, Chem. Rev., 2008, 108(2), 814–825 CrossRef CAS PubMed.
- M. Mansour, M. S. Darweesh and A. Soltan, Wearable devices for glucose monitoring: A review of state-of-the-art
technologies and emerging trends, Alexandria Eng. J., 2024, 89, 224–243 CrossRef.
- I. Lee, D. Probst, D. Klonoff and K. Sode, Continuous glucose monitoring systems-Current status and future perspectives of the flagship technologies in biosensor research, Biosens. Bioelectron., 2021, 181, 113054 CrossRef CAS PubMed.
- L. Hoeks, W. Greven and H. De Valk, Real-time continuous glucose monitoring system for treatment of diabetes: a systematic review, Diabetic Med., 2011, 28(4), 386–394 CrossRef CAS PubMed.
- J. P. Bantle and W. Thomas, Glucose measurement in patients with diabetes mellitus with dermal interstitial fluid, J. Lab. Clin. Med., 1997, 130(4), 436–441 CrossRef CAS PubMed.
- Z.-Y. Zhang, L.-F. Miao, L.-L. Qian, N. Wang, M.-M. Qi, Y.-M. Zhang, S.-P. Dang, Y. Wu and R.-X. Wang, Molecular mechanisms of glucose fluctuations on diabetic complications, Front. Endocrinol., 2019, 10, 640 CrossRef PubMed.
- M. J. Picón, M. Murri, A. Muñoz, J. C. Fernández-García, R. Gomez-Huelgas and F. J. Tinahones, Hemoglobin A1c versus oral glucose tolerance test in postpartum diabetes screening, Diabetes Care, 2012, 35(8), 1648–1653 CrossRef PubMed.
- N. Alqahtani, W. A. G. Khan, M. H. Alhumaidi and Y. A. A. R. Ahmed, Use of glycated hemoglobin in the diagnosis of diabetes mellitus and pre-diabetes and role of fasting plasma glucose, oral glucose tolerance test, Int. J. Prev. Med., 2013, 4(9), 1025 Search PubMed.
- A. Mari, G. Pacini, E. Murphy, B. Ludvik and J. J. Nolan, A model-based method for assessing insulin sensitivity from the oral glucose tolerance test, Diabetes Care, 2001, 24(3), 539–548 CrossRef CAS PubMed.
- S. I. Sherwani, H. A. Khan, A. Ekhzaimy, A. Masood and M. K. Sakharkar, Significance of HbA1c test in diagnosis and prognosis of diabetic patients, Biomarker Insights, 2016, 11, 95–104 CrossRef CAS PubMed , BMI. S38440.
- M. Harun-Or-Rashid, M. N. Aktar, V. Preda and N. Nasiri, Advances in electrochemical sensors for real-time glucose monitoring, Sens. Diagn., 2024, 3, 893–913 RSC.
- H.-C. Wang and A.-R. Lee, Recent developments in blood glucose sensors, J. Food Drug Anal., 2015, 23(2), 191–200 CrossRef CAS PubMed.
- H. Teymourian, A. Barfidokht and J. Wang, Electrochemical glucose sensors in diabetes management: an updated review (2010–2020), Chem. Soc. Rev., 2020, 49(21), 7671–7709 RSC.
- P. Bollella and E. Katz, Enzyme-based biosensors: Tackling electron transfer issues, Sensors, 2020, 20(12), 3517 CrossRef CAS PubMed.
- I. Ahmed, N. Jiang, X. Shao, M. Elsherif, F. Alam, A. Salih, H. Butt and A. K. Yetisen, Recent advances in optical sensors for continuous glucose monitoring, Sens. Diagn., 2022, 1(6), 1098–1125 RSC.
- S. H. Lee, D. S. Choi, S. K. Kuk and C. B. Park, Photobiocatalysis: activating redox enzymes by direct or indirect transfer of photoinduced electrons, Angew. Chem., Int. Ed., 2018, 57(27), 7958–7985 CrossRef CAS PubMed.
- M. Saleem, H. Yu, L. Wang, H. Khalid, M. Akram, N. M. Abbasi and J. Huang, Review on synthesis of ferrocene-based redox polymers and derivatives and their application in glucose sensing, Anal. Chim. Acta, 2015, 876, 9–25 CrossRef CAS PubMed.
- A. Chaubey and B. Malhotra, Mediated biosensors, Biosens. Bioelectron., 2002, 17(6–7), 441–456 CrossRef CAS PubMed.
- A. Khan, E. DeVoe and S. Andreescu, Carbon-based electrochemical biosensors as diagnostic platforms for connected decentralized healthcare, Sens. Diagn., 2023, 2(3), 529–558 RSC.
- S. A. Patil, C. Hägerhäll and L. Gorton, Electron transfer mechanisms between microorganisms and electrodes in bioelectrochemical systems, Bioanal. Rev., 2012, 4, 159–192 CrossRef.
- M. Zhao, Y. Gao, J. Sun and F. Gao, Mediatorless glucose biosensor and direct electron transfer type glucose/air biofuel cell enabled with carbon nanodots, Anal. Chem., 2015, 87(5), 2615–2622 CrossRef CAS PubMed.
- G. Li, J. Hao, W. Li, F. Ma, T. Ma, W. Gao, Y. Yu and D. Wen, Integrating Highly Porous and Flexible Au Hydrogels with Soft-MEMS Technologies for High-Performance Wearable Biosensing, Anal. Chem., 2021, 93(42), 14068–14075, DOI:10.1021/acs.analchem.1c01581.
- I. Lee, N. Loew, W. Tsugawa, C.-E. Lin, D. Probst, J. T. La Belle and K. Sode, The electrochemical behavior of a FAD dependent glucose dehydrogenase with direct electron transfer subunit by immobilization on self-assembled monolayers, Bioelectrochemistry, 2018, 121, 1–6 CrossRef CAS PubMed.
- B. J. Van Enter and E. Von Hauff, Challenges and perspectives in continuous glucose monitoring, Chem. Commun., 2018, 54(40), 5032–5045 RSC.
- G. A. Naikoo, T. Awan, H. Salim, F. Arshad, I. U. Hassan, M. Z. Pedram, W. Ahmed, H. L. Faruck, A. A. Aljabali and V. Mishra, Fourth-generation glucose sensors composed of copper nanostructures for diabetes management: A critical review, Bioeng. Transl. Med., 2022, 7(1), e10248 CrossRef CAS PubMed.
- B. A. Hussein, A. A. Tsegaye, G. Shifera and A. M. Taddesse, A sensitive non-enzymatic electrochemical glucose sensor based on a ZnO/Co 3 O 4/reduced graphene oxide nanocomposite, Sens. Diagn., 2023, 2(2), 347–360 RSC.
- J. Liu, J. Shen, S. Ji, Q. Zhang and W. Zhao, Research progress of electrode materials for non-enzymatic glucose electrochemical sensors, Sens. Diagn., 2023, 2(1), 36–45 RSC.
- G. Li, C. Wang, Y. Chen, F. Liu, H. Fan, B. Yao, J. Hao, Y. Yu and D. Wen, Dual Structural Design of Platinum-Nickel Hydrogels for Wearable Glucose Biosensing with Ultrahigh Stability, Small, 2023, 19(16), 2206868 CrossRef CAS PubMed.
- M. H. Hassan, C. Vyas, B. Grieve and P. Bartolo, Recent advances in enzymatic and non-enzymatic electrochemical glucose sensing, Sensors, 2021, 21(14), 4672 CrossRef CAS PubMed.
- D. Thatikayala, D. Ponnamma, K. K. Sadasivuni, J.-J. Cabibihan, A. K. Al-Ali, R. A. Malik and B. Min, Progress of advanced nanomaterials in the non-enzymatic electrochemical sensing of glucose and H2O2, Biosensors, 2020, 10(11), 151 CrossRef CAS PubMed.
- L. B. Baker, Physiology of sweat gland function: The roles of sweating and sweat composition in human health, Temperature, 2019, 6(3), 211–259 CrossRef PubMed.
- L. B. Baker and A. S. Wolfe, Physiological mechanisms determining eccrine sweat composition, Eur. J. Appl. Physiol., 2020, 120, 719–752 CrossRef CAS PubMed.
- E. V. Karpova, E. V. Shcherbacheva, A. A. Galushin, D. V. Vokhmyanina, E. E. Karyakina and A. A. Karyakin, Noninvasive diabetes monitoring through continuous analysis of sweat using flow-through glucose biosensor, Anal. Chem., 2019, 91(6), 3778–3783 CrossRef CAS PubMed.
- J. R. Sempionatto, J.-M. Moon and J. Wang, Touch-based fingertip blood-free reliable glucose monitoring: Personalized data processing for predicting blood glucose concentrations, ACS Sens., 2021, 6(5), 1875–1883 CrossRef CAS PubMed.
- P.-H. Lin, S.-C. Sheu, C.-W. Chen, S.-C. Huang and B.-R. Li, Wearable hydrogel patch with noninvasive, electrochemical glucose sensor for natural sweat detection, Talanta, 2022, 241, 123187 CrossRef CAS PubMed.
- Q. Liu, Y. Liu, F. Wu, X. Cao, Z. Li, M. Alharbi, A. N. Abbas, M. R. Amer and C. Zhou, Highly sensitive and wearable In2O3 nanoribbon transistor biosensors with integrated on-chip gate for glucose monitoring in body fluids, ACS Nano, 2018, 12(2), 1170–1178 CrossRef CAS PubMed.
- J. Xiao, Y. Liu, L. Su, D. Zhao, L. Zhao and X. Zhang, Microfluidic chip-based wearable colorimetric sensor for simple and facile detection of sweat glucose, Anal. Chem., 2019, 91(23), 14803–14807 CrossRef CAS PubMed.
- J. Zhao, Y. Lin, J. Wu, H. Y. Y. Nyein, M. Bariya, L.-C. Tai, M. Chao, W. Ji, G. Zhang and Z. Fan, A fully integrated and self-powered smartwatch for continuous sweat glucose monitoring, ACS Sens., 2019, 4(7), 1925–1933 CrossRef CAS PubMed.
- S. Y. Oh, S. Y. Hong, Y. R. Jeong, J. Yun, H. Park, S. W. Jin, G. Lee, J. H. Oh, H. Lee and S.-S. Lee, Skin-attachable, stretchable electrochemical sweat sensor for glucose and pH detection, ACS Appl. Mater. Interfaces, 2018, 10(16), 13729–13740 CrossRef CAS PubMed.
- Y. Zhao, Q. Zhai, D. Dong, T. An, S. Gong, Q. Shi and W. Cheng, Highly stretchable and strain-insensitive fiber-based wearable electrochemical biosensor to monitor glucose in the sweat, Anal. Chem., 2019, 91(10), 6569–6576 CrossRef CAS PubMed.
- W. Gao, S. Emaminejad, H. Y. Y. Nyein, S. Challa, K. Chen, A. Peck, H. M. Fahad, H. Ota, H. Shiraki and D. Kiriya, Fully integrated wearable sensor arrays for multiplexed in situ perspiration analysis, Nature, 2016, 529(7587), 509–514 CrossRef CAS PubMed.
- H. Lee, C. Song, Y. S. Hong, M. Kim, H. R. Cho, T. Kang, K. Shin, S. H. Choi, T. Hyeon and D.-H. Kim, Wearable/disposable sweat-based glucose monitoring device with multistage transdermal drug delivery module, Sci. Adv., 2017, 3(3), e1601314 CrossRef PubMed.
- H. Hojaiji, Y. Zhao, M. C. Gong, M. Mallajosyula, J. Tan, H. Lin, A. M. Hojaiji, S. Lin, C. Milla and A. M. Madni, An autonomous wearable system for diurnal sweat biomarker data acquisition, Lab Chip, 2020, 20(24), 4582–4591 RSC.
- A. Wiorek, M. Parrilla, M. Cuartero and G. N. A. Crespo, Epidermal patch with glucose biosensor: pH and temperature correction toward more accurate sweat analysis during sport practice, Anal. Chem., 2020, 92(14), 10153–10161 CrossRef CAS PubMed.
- S. Robinson and A. H. Robinson, Chemical composition of sweat, Physiol. Rev., 1954, 34(2), 202–220 CrossRef CAS PubMed.
- S. Mitragotri, M. Coleman, J. Kost and R. Langer, Analysis of ultrasonically extracted interstitial fluid as a predictor of blood glucose levels, J. Appl. Physiol., 2000, 89(3), 961–966 CrossRef CAS PubMed.
- M. Friedel, I. A. Thompson, G. Kasting, R. Polsky, D. Cunningham, H. T. Soh and J. Heikenfeld, Opportunities and challenges in the diagnostic utility of dermal interstitial fluid, Nat. Biomed. Eng., 2023, 7(12), 1541–1555 CrossRef PubMed.
- J. Heikenfeld, A. Jajack, B. Feldman, S. W. Granger, S. Gaitonde, G. Begtrup and B. A. Katchman, Accessing analytes in biofluids for peripheral biochemical monitoring, Nat. Biotechnol., 2019, 37(4), 407–419 CrossRef CAS PubMed.
- R. O. Potts, J. A. Tamada and M. J. Tierney, Glucose monitoring by reverse iontophoresis, Diabetes/Metab. Res. Rev., 2002, 18(S1), S49–S53 CrossRef CAS PubMed.
- A. El-Laboudi, N. S. Oliver, A. Cass and D. Johnston, Use of microneedle array devices for continuous glucose monitoring: a review, Diabetes Technol. Ther., 2013, 15(1), 101–115 CrossRef CAS PubMed.
- M. Tierney, H. Kim, M. Burns, J. Tamada and R. Potts, Electroanalysis of glucose in transcutaneously extracted samples, Electroanalysis, 2000, 12(9), 666–671 CrossRef CAS.
- J. R. Windmiller, G. Valdés-Ramírez, N. Zhou, M. Zhou, P. R. Miller, C. Jin, S. M. Brozik, R. Polsky, E. Katz and R. Narayan, Bicomponent microneedle array biosensor for minimally-invasive glutamate monitoring, Electroanalysis, 2011, 23(10), 2302–2309 CrossRef CAS.
- L. Lipani, B. G. Dupont, F. Doungmene, F. Marken, R. M. Tyrrell, R. H. Guy and A. Ilie, Non-invasive, transdermal, path-selective and specific glucose monitoring via a graphene-based platform, Nat. Nanotechnol., 2018, 13(6), 504–511 CrossRef CAS PubMed.
- Y. Chen, S. Lu, S. Zhang, Y. Li, Z. Qu, Y. Chen, B. Lu, X. Wang and X. Feng, Skin-like biosensor system via electrochemical channels for noninvasive blood glucose monitoring, Sci. Adv., 2017, 3(12), e1701629 CrossRef PubMed.
- F. Tehrani, H. Teymourian, B. Wuerstle, J. Kavner, R. Patel, A. Furmidge, R. Aghavali, H. Hosseini-Toudeshki, C. Brown and F. Zhang, An integrated wearable microneedle array for the continuous monitoring of multiple biomarkers in interstitial fluid, Nat. Biomed. Eng., 2022, 6(11), 1214–1224 CrossRef CAS PubMed.
- D. Pankratov, E. González-Arribas, Z. Blum and S. Shleev, Tear based bioelectronics, Electroanalysis, 2016, 28(6), 1250–1266 CrossRef CAS.
- L. Yu, Z. Yang and M. An, Lab on the eye: A review of tear-based wearable devices for medical use and health management, BioSci. Trends, 2019, 13(4), 308–313 CrossRef CAS PubMed.
- Z. Peng, X. Xie, Q. Tan, H. Kang, J. Cui, X. Zhang, W. Li and G. Feng, Blood glucose sensors and recent advances: A review, J. Innovative Opt. Health Sci., 2022, 15(02), 2230003 CrossRef CAS.
- H. Yao, A. J. Shum, M. Cowan, I. Lähdesmäki and B. A. Parviz, A contact lens with embedded sensor for monitoring tear glucose level, Biosens. Bioelectron., 2011, 26(7), 3290–3296 CrossRef CAS PubMed.
- J. Kim, M. Kim, M.-S. Lee, K. Kim, S. Ji, Y.-T. Kim, J. Park, K. Na, K.-H. Bae and H. Kyun Kim, Wearable smart sensor systems integrated on soft contact lenses for wireless ocular diagnostics, Nat. Commun., 2017, 8(1), 14997 CrossRef PubMed.
- N. M. Farandos, A. K. Yetisen, M. J. Monteiro, C. R. Lowe and S. H. Yun, Contact lens sensors in ocular diagnostics, Adv. Healthcare Mater., 2015, 4(6), 792–810 CrossRef CAS PubMed.
- A. E. Kownacka, D. Vegelyte, M. Joosse, N. Anton, B. J. Toebes, J. Lauko, I. Buzzacchera, K. Lipinska, D. A. Wilson and N. Geelhoed-Duijvestijn, Clinical evidence for use of a noninvasive biosensor for tear glucose as an alternative to painful finger-prick for diabetes management utilizing a biopolymer coating, Biomacromolecules, 2018, 19(11), 4504–4511 CrossRef CAS PubMed.
- J. Park, J. Kim, S.-Y. Kim, W. H. Cheong, J. Jang, Y.-G. Park, K. Na, Y.-T. Kim, J. H. Heo and C. Y. Lee, Soft, smart contact lenses with integrations of wireless circuits, glucose sensors, and displays, Sci. Adv., 2018, 4(1), eaap9841 CrossRef PubMed.
- D. H. Keum, S.-K. Kim, J. Koo, G.-H. Lee, C. Jeon, J. W. Mok, B. H. Mun, K. J. Lee, E. Kamrani and C.-K. Joo, Wireless smart contact lens for diabetic diagnosis and therapy, Sci. Adv., 2020, 6(17), eaba3252 CrossRef CAS PubMed.
- Y. Du, W. Zhang and M. L. Wang, An on-chip disposable salivary glucose sensor for diabetes control, J. Diabetes Sci. Technol., 2016, 10(6), 1344–1352 CrossRef CAS PubMed.
- V. Mani, T. Beduk, W. Khushaim, A. E. Ceylan, S. Timur, O. S. Wolfbeis and K. N. Salama, Electrochemical sensors targeting salivary biomarkers: A comprehensive review, TrAC, Trends Anal. Chem., 2021, 135, 116164 CrossRef CAS.
- G. Varga, Physiology of the salivary glands, Surgery, 2012, 30(11), 578–583 Search PubMed.
- M. Yamaguchi, M. Mitsumori and Y. Kano, Noninvasively measuring blood glucose using saliva, IEEE Eng. Med. Biol. Mag., 1998, 17(3), 59–63 CrossRef CAS PubMed.
- S. Gupta, S. V. Sandhu, H. Bansal and D. Sharma, Comparison of salivary and serum glucose levels in diabetic patients, J. Diabetes Sci. Technol., 2014, 9(1), 91–96 CrossRef PubMed.
- T. Arakawa, K. Tomoto, H. Nitta, K. Toma, S. Takeuchi, T. Sekita, S. Minakuchi and K. Mitsubayashi, A wearable cellulose acetate-coated mouthguard biosensor for in vivo salivary glucose measurement, Anal. Chem., 2020, 92(18), 12201–12207 CrossRef CAS PubMed.
- T. Arakawa, Y. Kuroki, H. Nitta, P. Chouhan, K. Toma, S.-I. Sawada, S. Takeuchi, T. Sekita, K. Akiyoshi and S. Minakuchi, Mouthguard biosensor with telemetry system for monitoring of saliva glucose: A novel cavitas sensor, Biosens. Bioelectron., 2016, 84, 106–111 CrossRef CAS PubMed.
- P. Tseng, B. Napier, L. Garbarini, D. L. Kaplan and F. G. Omenetto, Functional, RF-trilayer sensors for tooth-mounted, wireless monitoring of the oral cavity and food consumption, Adv. Mater., 2018, 30(18), 1703257 CrossRef PubMed.
- M.-S. Steiner, A. Duerkop and O. S. Wolfbeis, Optical methods for sensing glucose, Chem. Soc. Rev., 2011, 40(9), 4805–4839 RSC.
- A. K. Yetisen, N. Jiang, A. Fallahi, Y. Montelongo, G. U. Ruiz-Esparza, A. Tamayol, Y. S. Zhang, I. Mahmood, S. A. Yang and K. S. Kim, Glucose-sensitive hydrogel optical fibers functionalized with phenylboronic acid, Adv. Mater., 2017, 29(15), 1606380 CrossRef PubMed.
- B. Chance, M. Cope, E. Gratton, N. Ramanujam and B. Tromberg, Phase measurement of light absorption and scatter in human tissue, Rev. Sci. Instrum., 1998, 69(10), 3457–3481 CrossRef CAS.
- A. Sakudo, Near-infrared spectroscopy for medical applications: Current status and future perspectives, Clin. Chim. Acta, 2016, 455, 181–188 CrossRef CAS PubMed.
- J. Kottmann, J. M. Rey and M. W. Sigrist, Mid-Infrared photoacoustic detection of glucose in human skin: towards non-invasive diagnostics, Sensors, 2016, 16(10), 1663 CrossRef PubMed.
- K. Maruo, M. Tsurugi, J. Chin, T. Ota, H. Arimoto, Y. Yamada, M. Tamura, M. Ishii and Y. Ozaki, Noninvasive blood glucose assay using a newly developed near-infrared system, IEEE J. Sel. Top. Quantum Electron., 2003, 9(2), 322–330 CrossRef CAS.
- J. Y. Sim, C.-G. Ahn, E.-J. Jeong and B. K. Kim, In vivo microscopic photoacoustic spectroscopy for non-invasive glucose monitoring invulnerable to skin secretion products, Sci. Rep., 2018, 8(1), 1059 CrossRef PubMed.
- R. Kasahara, S. Kino, S. Soyama and Y. Matsuura, Noninvasive glucose monitoring using mid-infrared absorption spectroscopy based on a few wavenumbers, Biomed. Opt. Express, 2017, 9(1), 289–302 CrossRef PubMed.
- X. Jintao, Y. Liming, L. Yufei, L. Chunyan and C. Han, Noninvasive and fast measurement of blood glucose in vivo by near infrared (NIR) spectroscopy, Spectrochim. Acta, Part A, 2017, 179, 250–254 CrossRef PubMed.
- L. B. Mohammadi, T. Klotzbuecher, S. Sigloch, K. Welzel, M. Göddel, T. R. Pieber and L. Schaupp, In vivo evaluation of a chip based near infrared sensor for continuous glucose monitoring, Biosens. Bioelectron., 2014, 53, 99–104 CrossRef PubMed.
- T. Kitazaki, Y. Morimoto, S. Yamashita, D. Anabuki, S. Tahara, A. Nishiyama, K. Wada and I. Ishimaru, Glucose emission spectra through mid-infrared passive spectroscopic imaging of the wrist for non-invasive glucose sensing, Sci. Rep., 2022, 12(1), 20558 CrossRef CAS PubMed.
- A. Aloraynan, S. Rassel, M. R. Kaysir and D. Ban, Dual quantum cascade lasers for noninvasive glucose detection using photoacoustic spectroscopy, Sci. Rep., 2023, 13(1), 7927 CrossRef CAS PubMed.
- G. Mazarevica, T. Freivalds and A. Jurka, Properties of erythrocyte light refraction in diabetic patients, J. Biomed. Opt., 2002, 7(2), 244–247 CrossRef PubMed.
- J. Yadav, A. Rani, V. Singh and B. M. Murari, Prospects and limitations of non-invasive blood glucose monitoring using near-infrared spectroscopy, Biomed. Signal Process. Control, 2015, 18, 214–227 CrossRef.
- J. Lv, J. Wang, L. Yang, W. Liu, F. Haihao, P. K. Chu and C. Liu, Recent advances of optical fiber biosensors based on surface plasmon resonance: Sensing principles, Structures, and Prospects, Sens. Diagn., 2024, 3, 1369–1391 RSC.
- A. M. Enejder, T. G. Scecina, J. Oh, M. Hunter, W.-C. Shih, S. Sasic, G. L. Horowitz and M. S. Feld, Raman spectroscopy for noninvasive glucose measurements, J. Biomed. Opt., 2005, 10(3), 031114 CrossRef PubMed.
- J. Shao, M. Lin, Y. Li, X. Li, J. Liu, J. Liang and H. Yao, In vivo blood glucose quantification using Raman spectroscopy, PLoS One, 2012, 7(10), e48127 CrossRef CAS PubMed.
- J. W. Kang, Y. S. Park, H. Chang, W. Lee, S. P. Singh, W. Choi, L. H. Galindo, R. R. Dasari, S. H. Nam and J. Park, Direct observation of glucose fingerprint using in vivo Raman spectroscopy, Sci. Adv., 2020, 6(4), eaay5206 CrossRef CAS PubMed.
- J. Ju, C.-M. Hsieh, Y. Tian, J. Kang, R. Chia, H. Chang, Y. Bai, C. Xu, X. Wang and Q. Liu, Surface enhanced Raman spectroscopy based biosensor with a microneedle array for minimally invasive in vivo glucose measurements, ACS Sens., 2020, 5(6), 1777–1785 CrossRef CAS PubMed.
- D. Yang, S. Afroosheh, J. O. Lee, H. Cho, S. Kumar, R. H. Siddique, V. Narasimhan, Y.-Z. Yoon, A. T. Zayak and H. Choo, Glucose sensing using surface-enhanced Raman-mode constraining, Anal. Chem., 2018, 90(24), 14269–14278 CrossRef CAS PubMed.
- X. Sun, Glucose detection through surface-enhanced Raman spectroscopy: A review, Anal. Chim. Acta, 2022, 1206, 339226 CrossRef CAS PubMed.
- A. J. Berger, I. Itzkan and M. S. Feld, Feasibility of measuring blood glucose concentration by near-infrared Raman spectroscopy, Spectrochim. Acta, Part A, 1997, 53(2), 287–292 CrossRef PubMed.
- R. O. Esenaliev, K. V. Larin, I. V. Larina and M. Motamedi, Noninvasive monitoring of glucose concentration with optical coherence tomography, Opt. Lett., 2001, 26(13), 992–994 CrossRef CAS PubMed.
- M. G. Ghosn, V. V. Tuchin and K. V. Larin, Depth-resolved monitoring of glucose diffusion in tissues by using optical coherence tomography, Opt. Lett., 2006, 31(15), 2314–2316 CrossRef PubMed.
- K. V. Larin, M. S. Eledrisi, M. Motamedi and R. O. Esenaliev, Noninvasive blood glucose monitoring with optical coherence tomography: a pilot study in human subjects, Diabetes Care, 2002, 25(12), 2263–2267 CrossRef CAS PubMed.
- H. Ullah, F. Hussain and M. Ikram, Optical coherence tomography for glucose monitoring in blood, Appl. Phys. B:Lasers Opt., 2015, 120, 355–366 CrossRef CAS.
- L. R. De Pretto, T. M. Yoshimura, M. S. Ribeiro and A. Zanardi de Freitas, Optical coherence tomography for blood glucose monitoring in vitro through spatial and temporal approaches, J. Biomed. Opt., 2016, 21(8), 086007 CrossRef PubMed.
- B. H. Malik and G. L. Coté, Real-time, closed-loop dual-wavelength optical polarimetry for glucose monitoring, J. Biomed. Opt., 2010, 15(1), 017002 CrossRef PubMed.
- B. D. Cameron, J. S. Baba and G. L. Cote, Optical polarimetry applied to the development of a noninvasive in-vivo glucose monitor, in Optical Diagnostics of Biological Fluids V, SPIE, 2000, vol. 3923, pp. 66–77 Search PubMed.
- R. J. McNichols and G. L. Cote, Optical glucose sensing in biological fluids: an overview, J. Biomed. Opt., 2000, 5(1), 5–16 CrossRef CAS PubMed.
- G. Purvinis, B. D. Cameron and D. M. Altrogge, Noninvasive polarimetric-based glucose monitoring: an in vivo study, J. Diabetes Sci. Technol., 2011, 5(2), 380–387 CrossRef PubMed.
- J. C. Pickup, F. Hussain, N. D. Evans, O. J. Rolinski and D. J. Birch, Fluorescence-based glucose sensors, Biosens. Bioelectron., 2005, 20(12), 2555–2565 CrossRef CAS PubMed.
- D. C. Klonoff, Overview of fluorescence glucose sensing: a technology with a bright future, J. Diabetes Sci. Technol., 2012, 6(6), 1242–1250 CrossRef PubMed.
- L. Chen, E. Hwang and J. Zhang, Fluorescent nanobiosensors for sensing glucose, Sensors, 2018, 18(5), 1440 CrossRef PubMed.
- T. Van Tam, S. H. Hur, J. S. Chung and W. M. Choi, Novel paper-and fiber optic-based fluorescent sensor for glucose detection using aniline-functionalized graphene quantum dots, Sens. Actuators, B, 2021, 329, 129250 CrossRef.
- M. Deng, G. Song, K. Zhong, Z. Wang, X. Xia and Y. Tian, Wearable fluorescent contact lenses for monitoring glucose via a smartphone, Sens. Actuators, B, 2022, 352, 131067 CrossRef CAS.
- Y.-L. T. Ngo, P. L. Nguyen, J. Jana, W. M. Choi, J. S. Chung and S. H. Hur, Simple paper-based colorimetric and fluorescent glucose sensor using N-doped carbon dots and metal oxide hybrid structures, Anal. Chim. Acta, 2021, 1147, 187–198 CrossRef CAS PubMed.
- Y. Cui, W. Duan, Y. Jin, F. Wo, F. Xi and J. Wu, Ratiometric fluorescent nanohybrid for noninvasive and visual monitoring of sweat glucose, ACS Sens., 2020, 5(7), 2096–2105 CrossRef CAS PubMed.
- T. Kawanishi, M. Romey, P. Zhu, M. Holody and S. Shinkai, A study of boronic acid based fluorescent glucose sensors, J. Fluoresc., 2004, 14, 499–512 CrossRef CAS PubMed.
- E. A. Moschou, B. V. Sharma, S. K. Deo and S. Daunert, Fluorescence glucose detection: advances toward the ideal in vivo biosensor, J. Fluoresc., 2004, 14, 535–547 CrossRef CAS PubMed.
- P. H. Siegel, Y. Lee and V. Pikov, Millimeter-wave non-invasive monitoring of glucose in anesthetized rats, in 2014 39th International Conference on Infrared, Millimeter, and Terahertz waves (IRMMW-THz), IEEE, 2014, pp. 1–2 Search PubMed.
- A. E. Omer, G. Shaker, S. Safavi-Naeini, H. Kokabi, G. Alquié, F. Deshours and R. M. Shubair, Low-cost portable microwave sensor for non-invasive monitoring of blood glucose level: Novel design utilizing a four-cell CSRR hexagonal configuration, Sci. Rep., 2020, 10(1), 15200 CrossRef CAS PubMed.
- A. E. Omer, S. Safavi-Naeini, R. Hughson and G. Shaker, Blood glucose level monitoring using an FMCW millimeter-wave radar sensor, Remote Sens., 2020, 12(3), 385 CrossRef.
- S. Saha, H. Cano-Garcia, I. Sotiriou, O. Lipscombe, I. Gouzouasis, M. Koutsoupidou, G. Palikaras, R. Mackenzie, T. Reeve and P. Kosmas, A glucose sensing system based on transmission measurements at millimetre waves using micro strip patch antennas, Sci. Rep., 2017, 7(1), 6855 CrossRef PubMed.
- A. Kandwal, L. W. Liu, M. J. Deen, R. Jasrotia, B. K. Kanaujia and Z. Nie, Electromagnetic wave sensors for noninvasive blood glucose monitoring: Review and recent developments, IEEE Trans. Instrum. Meas., 2023, 72, 1–15 Search PubMed.
- H. Choi, J. Naylon, S. Luzio, J. Beutler, J. Birchall, C. Martin and A. Porch, Design and in vitro interference test of microwave noninvasive blood glucose monitoring sensor, IEEE Trans. Microwave Theory Tech., 2015, 63(10), 3016–3025 CAS.
- S. Lee, V. Nayak, J. Dodds, M. Pishko and N. B. Smith, Glucose measurements with sensors and ultrasound, Ultrasound Med. Biol., 2005, 31(7), 971–977 CrossRef PubMed.
- J. Kost, S. Mitragotri, R. A. Gabbay, M. Pishko and R. Langer, Transdermal monitoring of glucose and other analytes using ultrasound, Nat. Med., 2000, 6(3), 347–350 CrossRef CAS PubMed.
- S. Meyhöfer, B. Wilms, F. Ihling, A. Windjäger, H. Kalscheuer, A. Augustinov, V. Herrmann, H. Lehnert and S. M. Schmid, Evaluation of a near-infrared light ultrasound system as a non-invasive blood glucose monitoring device, Diabetes, Obes. Metab., 2020, 22(4), 694–698 CrossRef PubMed.
- G. Merino, Y. N. Kalia and R. H. Guy, Ultrasound-enhanced transdermal transport, J. Pharm. Sci., 2003, 92(6), 1125–1137 CrossRef CAS PubMed.
- D. D. Stupin, E. A. Kuzina, A. A. Abelit, A. K. Emelyanov, D. M. Nikolaev, M. N. Ryazantsev, S. V. Koniakhin and M. V. Dubina, Bioimpedance spectroscopy: basics and applications, ACS Biomater. Sci. Eng., 2021, 7(6), 1962–1986 CrossRef CAS PubMed.
- F. Sanai, A. S. Sahid, J. Huvanandana, S. Spoa, L. H. Boyle, J. Hribar, D. T.-Y. Wang, B. Kwan, S. Colagiuri and S. J. Cox, Evaluation of a continuous blood glucose monitor: a novel and non-invasive wearable using bioimpedance technology, J. Diabetes Sci. Technol., 2023, 17(2), 336–344 CrossRef CAS PubMed.
- B. G. Pedro, D. W. C. Marcôndes and P. Bertemes-Filho, Analytical model for blood glucose detection using electrical impedance spectroscopy, Sensors, 2020, 20(23), 6928 CrossRef CAS PubMed.
- C.-F. So, K.-S. Choi, T. K. Wong and J. W. Chung, Recent advances in noninvasive glucose monitoring, Med. Devices: Evidence Res., 2012, 45–52 CAS.
- E. Hadar, R. Chen, Y. Toledano, K. Tenenbaum-Gavish, Y. Atzmon and M. Hod, Noninvasive, continuous, real-time glucose measurements compared to reference laboratory venous plasma glucose values, J. Matern.-Fetal Neonat. Med., 2019, 32(20), 3393–3400, DOI:10.1080/14767058.2018.1463987.
- CoG - Hybrid Glucometer, 2024, https://www.cnogacare.co/cog-hybrid-glucometer (accessed) Search PubMed.
- C8 MediSensors, 2024, http://www.c8medisensors.com (accessed) Search PubMed.
- GlucoBeam Smart™, 2024, https://rspsystems.com/glucobeam-smart/ (accessed) Search PubMed.
- E. Tsalikian, C. Kollman, N. Mauras, S. Weinzimer, B. Weinzimer, D. Xing, R. Beck, K. Ruedy, W. Tamborlane and R. Fiallo-Scharer, GlucoWatch® G2™ Biographer (GW2B) Alarm Reliability DuringHypoglycemia in Children, Diabetes Technol. Ther., 2004, 6(5), 559–566 CrossRef PubMed.
- Sugar Beat™, 2024, https://nemauramedical.com/technology/ (accessed) Search PubMed.
- Glucotrack, 2024, https://glucotrack.com/ (accessed) Search PubMed.
- NovioSense, 2024, https://noviosense.com/ (accessed) Search PubMed.
- H. H. Mosli and B. M. Madani, Performance evaluation of egm1000™ non-invasive glucose monitoring device in patients with type 2 diabetes and subjects with prediabetes, IJMDC, 2021, 5(4) DOI:10.24911/IJMDC.2.1.7.
- NIRLUS, 2024, http://nirlus.com/ (accessed) Search PubMed.
- Diamontech, 2024, DiaMonTech (accessed) Search PubMed.
- Indigo, 2024, https://indigomed.com/ (accessed) Search PubMed.
- Biolinq, 2024, https://www.biolinq.com/ (accessed) Search PubMed.
- one drop, 2024, https://onedrop.today/ (accessed) Search PubMed.
- J. Bai, D. Liu, X. Tian, Y. Wang, B. Cui, Y. Yang, S. Dai, W. Lin, J. Zhu, J. Wang, A. Xu, Z. Gu and S. Zhang, Coin-sized, fully integrated, and minimally invasive continuous glucose monitoring system based on organic electrochemical transistors, Sci. Adv., 2024, 10(16), eadl1856 CrossRef CAS PubMed.
- Q. Wang, A. Molinero-Fernandez, Q. Wei, X. Xuan, A. Konradsson-Geuken, M. Cuartero and G. A. Crespo, Intradermal Lactate Monitoring Based on a Microneedle Sensor Patch for Enhanced In Vivo Accuracy, ACS Sens., 2024, 9(6), 3115–3125, DOI:10.1021/acssensors.4c00337.
- M. Sun, X. Pei, T. Xin, J. Liu, C. Ma, M. Cao and M. Zhou, A Flexible Microfluidic Chip-Based Universal Fully Integrated Nanoelectronic System with Point-of-Care Raw Sweat, Tears, or Saliva Glucose Monitoring for Potential Noninvasive Glucose Management, Anal. Chem., 2022, 94(3), 1890–1900, DOI:10.1021/acs.analchem.1c05174.
- K'Watch Glucose, 2024, https://www.pkvitality.com/ktrack-glucose/ (accessed) Search PubMed.
- GWave, 2024, https://www.hagartech.com/ (accessed) Search PubMed.
- Dexcom, 2024, https://www.dexcom.com/ (accessed) Search PubMed.
- FreeStyle Libre, 2024, https://cloud.myfreestyle.abbott.com.sg/ (accessed) Search PubMed.
- Medtronic, 2024, https://www.medtronicdiabetes.com/ (accessed) Search PubMed.
- Senseonics, 2024, https://www.senseonics.com/ (accessed) Search PubMed.
- GlucoWise, 2024, https://www.medicalexpo.com/prod/mediwise/product-105186-686902.html (accessed) Search PubMed.
|
This journal is © The Royal Society of Chemistry 2025 |
Click here to see how this site uses Cookies. View our privacy policy here.