DOI:
10.1039/D4TB01946F
(Paper)
J. Mater. Chem. B, 2025,
13, 1681-1689
Multiple aptamer recognition-based quantum dot lateral flow platform: ultrasensitive point-of-care testing of respiratory infectious diseases†
Received
29th August 2024
, Accepted 24th November 2024
First published on 5th December 2024
Abstract
Respiratory infectious diseases spread rapidly and have a wide range of impacts, posing a serious threat to public health security. The development of a sensitive, accurate, and rapid detection method for respiratory viruses is crucial for disease prevention and control. However, existing methods are inadequate in satisfying the demand for accurate and convenient detection simultaneously. Therefore, an ultrasensitive point-of-care testing (POCT) platform based on a multiple aptamer recognition-based quantum dot lateral flow immunoassay (MARQ-LFIA) was developed in this work. This platform consisted of multiple high-affinity aptamers for recognizing different sites on a respiratory infectious virus protein, enhancing the efficiency of virus identification in complex environments. By combining a multiple aptamer recognition strategy with quantum dot fluorescent technique to construct LFIA test strips and pairing them with a high-gain portable fluorescence reader, excellent detection sensitivity and specificity were achieved in the case of coronavirus disease 2019 (COVID-19). The limits of detection were 1.427 pg mL−1 and 1643 U mL−1 towards the nucleocapsid protein and inactivated viruses, respectively, indicating that MARQ-LFIA improved detection sensitivity compared to reported methods. More critically, by testing thirty COVID-19 positive and twenty negative patient samples, the positive detection rate increased from 55.17% to 86.67% compared with commercially similar products. The universality of MARQ-LFIA was also investigated for diagnosing influenza B. We believe that MARQ-LFIA can be a promising POCT tool with potential applications in the areas of public health for the growing demand for precision diagnosis and treatment.
Introduction
Globally, respiratory infectious diseases have posed a serious threat to the safety of human health and the development of society, especially with the outbreak of coronavirus disease 2019 (COVID-19).1,2 Given the complexity, variability, and strong infectiousness of respiratory diseases, there is an urgent need to establish a universal detection platform that is capable of quickly, sensitively, and specifically identifying these diseases.3–5 During the COVID-19 pandemic, nucleic acid amplification testing (NAAT) by virtue of real-time polymerase chain reaction (RT-PCR) technology was widely recognized as the gold standard for detecting severe acute respiratory syndrome coronavirus 2 (SARS-CoV-2, which causes COVID-19) because of its high accuracy and sensitivity.6–8 However, molecular-level diagnosis requires specialized equipment and skilled personnel, which are not suitable for point-of-care testing (POCT), especially in the midst of an outbreak and harsh environments.9,10 In contrast, the lateral flow immunoassay (LFIA), which relies on sandwich immunoassay technique to identify pathogenic microbial antigens, has gradually been applied in various fields of respiratory infection detection because of its shorter detection time and lack of environmental and equipment limitations.11–13 However, hitherto, the LFIA for rapid antigen detection can only be used as a supplementary method to NAAT as it suffers from insufficient sensitivity. The recognition affinity between the antibody and the virus antigen, as well as naked-eye detection, are significant obstacles limiting the sensitivity of LFIA. Furthermore, it cannot be ignored that the “antibody–antigen–antibody” sandwich structure of LFIA's recognition patterns faces challenges, such as overlapping recognition sites, numerous nonspecific cross-reactions and considerable between-batch variations of antibody pairs.14–17 Therefore, a novel ultrasensitive sensing strategy with high recognition affinity, strong specificity, and rapid acquisition is essential for the detection of various respiratory infectious diseases.
Aptamers are single-stranded oligonucleotides obtained from the systematic evolution of ligands by exponential enrichment (SELEX) technology, with lengths of about 20–120 nucleotides.18–22 Compared to antibodies, aptamers offer several advantages, including small steric hindrance, broad range of targets, and superior stability. As a result, they have been widely applied in research fields of disease diagnosis and treatment.23–28 The simultaneous incorporation of aptamers and antibodies, which exhibit distinct biochemistry characteristics, into the sandwich-type immune recognition can ensure mutual enhancement in various analytical applications. Numerous aptamer–antibody hybrid analysis systems have been developed, showing improved detection sensitivity for pathogenic microorganism antigens compared to traditional full antibody analysis systems.29–34 However, these advancements still fall short of the high sensitivity requirements of POCT, and are constrained by their limited qualitative or semi-quantitative detection capabilities. The combinatorial application of different aptamers can significantly improve the recognition and binding ability for targets.35–39 Despite this potential, there have been relatively few studies devoted to developing the multiple aptamers combination in LFIA design to improve its detection performance. Therefore, we anticipate that the design and composition of multiple aptamers recognition, along with aptamer–antibody hybrid analysis systems, could pave the way for the highly sensitive detection of pathogenic microorganism antigens.
Building upon this foundation, the introduction of a fluorescent labeling technique of LFIA can essentially improve the signal detection sensitivity beyond naked-eye judgment methods.40–45 Among the various luminescent materials, quantum dots (QDs) stand out due to their high fluorescence intensity, tunable emission wavelength, large Stokes shift, prolonged fluorescence lifetime, and abundant surface chemical modification sites. These unique characteristics make QDs one of the premier choices for fluorescent labeling applications.46–49 However, the single QD-assembled signal source still limits the luminescence intensity and detection sensitivity. Dendritic mesoporous silica nanoparticles (DMSNs) have emerged as special nanomaterials, which are distinguished from traditional mesoporous silica by their three-dimensional dendritic framework, central radial mesoporous structure, larger specific surface area, and higher loading capacity.50,51 The integration of QDs with DMSNs has resulted in the formation of QDs fluorescent microspheres, exhibiting enhanced fluorescence effects and significantly improved detection sensitivity compared to conventional QDs.52,53 Consequently, the synergy between the QD fluorescence immunochromatography platform and the multiple aptamers–antibody hybrid system is expected to further improve the sensitivity and specificity of virus antigen detection.
Herein, we developed a novel multiple aptamers recognition based quantum dots lateral flow immunoassay (MARQ-LFIA) platform for the ultrasensitive POCT of respiratory infectious diseases (Fig. 1). MARQ-LFIA was constructed based on a strategy that combined multiple aptamers recognition with the QDs fluorescence immunochromatography technique. In this study, COVID-19 was selected as a research model, employing multiple aptamers that bind to different sites on the nucleocapsid (N) protein of SARS-CoV-2. By integrating these aptamers with QDs, we created a high-affinity capture probe capable of significantly enhancing the detection accuracy. Multiple combinations of aptamers enhanced the recognition, while the fluorescence QDs LFIA platform further improved the detection output signal. By developing the high-gain portable fluorescence reader for signal intensity acquisition, MARQ-LFIA demonstrated remarkable detection sensitivity and specificity. Compared to reported LFIA-based sensors and commercial kits, MARQ-LFIA improved the detection sensitivity (1.427 pg mL−1 and 1643 U mL−1) and clinical sample detection capability of COVID-19 positive patients (55.17% to 86.67%). To explore the universality of our platform, we also established another MARQ-LFIA for investigating the diagnosis capability of influenza B.
 |
| Fig. 1 Principle of the MARQ-LFIA platform for SARS-CoV-2 detection. | |
Experimental section
Acquisition and characteristic of multiple high-affinity aptamers
Multiple high-affinity aptamers for the SARS-CoV-2 N protein and influenza B virus nucleoprotein were obtained by magnetic beads-based SELEX using a previously reported method.54 In brief, 10 μL target protein (500 μg mL−1) was coupled on the surface of magnetic beads (protein-MB) through the classic 1-ethyl-(3-dimethylaminopropyl) carbodiimide (EDC)/N-hydroxybutanediimide (NHS) protocol. After 95 °C denaturation, 2 OD of the DNA library was incubated with the target virus protein-MB in binding buffer (50 mM Tris–HCl, 5 mM MgCl2, 120 mM NaCl, 0.2% Tween-20), which simulated the clinical detection environment. The supernatant was then removed by magnetic separation, and the beads were washed with 200 μL DPBS. PCR was used to recover and amplify the bound DNA. After about ten rounds of selection, the high-throughput sequencing (HTS) technique was utilized to access the sequence information of the final enriched DNA pool. The top twenty sequences with the highest enrichment were synthesized, and the affinity was confirmed by surface plasmon resonance (SPR) analysis. The sequences of the DNA libraries and PCR primers during the entire selection are shown in Tables S1 and S2 (ESI†).
Synthesis of the fluorescence nanoprobe ApMQS
The synthesis procedures of DMSN and DMSN/QDs/SiO2 (MQS) are shown in the ESI.† The carboxyl-terminated MQS was functionalized with SA through the classical carbodiimide coupling reaction. In brief, a 20-μL volume of MQS (5 mg mL−1) in 180 μL MES buffer was first activated by adding 50 μL EDC (64 mM) and 75 μL NHS (10 mg mL−1), and reacted for 20 min. Then, 25 μg SA was added and incubated for 2 h with gentle agitation. After centrifugation at 10
000 rpm for 5 min, MQS-SA was washed with 1 × Tris–borate–sodium Tween-20 (TBST, pH 7.4) three times. A 2.5-μL volume of biotinylated aptamers (100 μM) was incubated with 5 μL MQS-SA for 30 min. Aptamers-MQS (ApMQS) were harvested by centrifugation, redispersed in phosphate buffered saline to form the storage dispersion, and kept at 4 °C.
Construction of the MARQ-LFIA platform
MAQF-LFIA consists of four components: the sample pad, conjugate pad, NC membrane, and absorbent pad. Using a dispenser (HGS510-1B; Autokun, Hangzhou, China), anti-virus N protein antibody (1 mg mL−1) and anti-SA antibody (0.5 mg mL−1) were immobilized onto the NC membrane (0.8 cm and 1.7 cm from the side of the NC membrane; 30 × 2.5 cm2) at 1 μL cm−1 respectively. The final resuspended ApMQS conjugate solution was loaded onto the conjugate pad (30 × 1 cm2) at 4 μL cm−1, and dried in a desiccator at 37 °C. After incubation, the conjugate pad and sample pad (30 × 1 cm2) were attached to the bottom of the NC membrane with overlaps of 1.5 and 2.5 mm, respectively. The absorbent pad (30 × 2.6 cm2) was attached to the top side of the NC membrane with a 2 mm overlap. The combined strips were subsequently cut into 4 mm wide strips using a cutting machine.
The MARQ-LFIA platform for virus protein detection
We evaluated the performance of the MAQF-LFIA platform at the level of virus N proteins, and examined the efficacy of an assay by diluting the N proteins with an extraction buffer (50 mM Tris–HCl, 5 mM MgCl2, 120 mM NaCl, 0.02% Tween-20, pH 7.5). An 80-μL volume of sample was added to the strip with 15 min detection time, and the signal intensity was subsequently acquired from the portable fluorescence reader. The dilution ranged from 500 fg mL−1 to 10 ng mL−1 for the SARS-CoV-2 N protein, and the dilution range of the influenza B virus nucleoprotein was 1 pg mL−1 to 10 ng mL−1, for obtaining a standard curve. Based on the linear graph obtained from the experiment, the limit of detection (LOD) was calculated using the formula LOD = 3δ/S (where δ is the standard deviation of the blank and S is the slope of the standard curve).
The MARQ-LFIA platform for inactivated virus detection
Inactivated SARS-CoV-2 and influenza B virus were from the National Reference Panel for 2019-nCoV (SARS-CoV-2) Antigen Detection Kit and the 2nd National Reference Panel for Influenza B Viral Antigens Detection Kit, respectively, which were acquired from the National Institutes for Food and Drug Control (Beijing China). After the reference samples were taken out and restored to room temperature, 0.5 mL distilled water was added to re-dissolve the samples. The minimum LOD reference sample diluted by binding buffer was detected to obtain the titration curve and LOD, and the diluted positive reference sample and negative reference sample were measured to obtain the specificity of MAQF-LFIA.
The MARQ-LFIA platform for clinical samples evaluation
Nasal swab samples (thirty cases) from COVID-19 positive patients and samples (fifty-seven cases) from influenza B positive patients were supplied by Zhejiang Provincial Center for Disease Control and Prevention (Hangzhou China). At the same time, nasal swab samples (forty cases) from healthy individuals were randomly selected as negative controls. Clinical samples were taken out and restored to room temperature, and diluted in half by the extraction buffer. The sample was not used for testing if it contained large amounts of mucus or turbidity.
Results and discussion
Acquisition and characteristic of multiple high-affinity aptamers
SELEX technology is a process that emulates the mechanisms of natural selection and evolution, which enriches aptamers that bind to the target molecule specifically through iterative cycles of selection and amplification from a DNA library comprising a vast array of random sequences. In this study, we utilized a modified SELEX process to obtain high-affinity aptamers. As shown in Fig. 2A, the SELEX process comprises the preparation of a random sequence DNA library, incubation with the target molecule, complex separation, elution and amplification, cyclic selection, and identification. Especially, in order to achieve precise recognition and binding of the target in corresponding environments (such as human nasopharyngeal swabs, sputum, and sample extraction buffer), it was crucial to emulate the complex buffer systems by virtue of designing the selection environment and stress under the SELEX process. Herein, we chose the N protein of SARS-CoV-2 as the specific target for aptamer selection and COVID-19 detection, owing to its high abundance, conservation, early expression during infection, strong immunogenicity and structural simplicity.55
 |
| Fig. 2 (A) The modified SELEX process for aptamer selection. (B) The predicted secondary structures of Scov1, Scov2 and Scov3 using the RNAfold web. SPR binding-dissociation kinetic curve of Scov1 (C), Scov2 (D) and Scov3 (E). The red line represents the fitted data. (F)–(H) SPR sensorgrams of Scov1, Scov2 and Scov3 binding kinetics to the immobilized N protein. Each kinetic curve starts with the first aptamer flowing through for 90 s, followed by a 2 min run of running buffer, and finally the second aptamer being injected into the same channel for 2 min. | |
As a result, our process ultimately yielded three aptamers specifically related to the N protein, termed Scov1, Scov2, and Scov3, respectively (Table S1, ESI†). The secondary structures of these aptamers were predicted using the RNAfold web (https://rna.tbi.univie.ac.at) server, which is based on the principle of minimum folding energy. As shown in Fig. 2B, the predicted secondary structures of these aptamers showed stable stem hairpin, loop and pseudoknot structures, which may play important roles in the binding of DNA aptamers to the N protein. The predicted secondary structures with the minimum folding energy suggested that these aptamers were capable of forming stable complexes with the N protein. The respective minimum Gibbs free energy change (ΔG) of Scov1, Scov2, and Scov3 were calculated to be −9.11, −7.18, and −8.75 kcal mol−1, respectively, indicating favourable binding thermodynamics. In addition, the binding-dissociation kinetic curve for these aptamers were stable and reproducible, exhibiting a high degree of concordance with the fitted results (Fig. 2C–E). The binding dissociation constants (KD) of the aptamers with the target were calculated by the binding rate constants (ka) and dissociation rate constants (kd) with the formula KD = ka/kd.33 The relevant parameters of Scov1, Scov2, and Scov3 are illustrated in Table S3 (ESI†) with the respective affinities of 2.41 nM, 3.24 nM, and 4.31 nM, indicating that all three aptamers had a relatively stable binding with the N protein.
Competitive experiments conducted through surface plasmon resonance (SPR) were subsequently performed to investigate the potential for multisite binding between different aptamers and the N protein. As shown in Fig. 2F, signals of up to 100 response units (RU) could be observed after Scov1 flowed into the channel in the initial run. The second aptamer Scov2 subsequently flowed through the same channel, and there was a significant change in the response signal (approximately 250 RU). These results demonstrated that Scov1 bound to a different site of the N protein with Scov2. During the competitive experiments comparing Scov1 with Scov3 (Fig. 2G) and Scov2 with Scov3 (Fig. 2H), similar results were also observed, further confirming that Scov1, Scov2, and Scov3 exhibited distinct binding sites of the N protein. This result strongly suggests that Scov1 and Scov2 bind to different sites on the N protein, supporting the hypothesis of multisite binding.
Synthesis and characteristic of the fluorescence nanoprobe ApMQS
In order to obtain high-performance signal output to overcome the poor sensitivity caused by naked-eye detection, we combined the QDs fluorescent technique with the multiple aptamers recognition strategy to construct a concordance high-fluorescence nanoprobe, termed ApMQS. The ApMQS construction strategy involved four steps (Fig. 3A): (i) the immobilization of hydrophobic QDs on dendritic mesoporous silica spheres, (ii) phase-transfer of the nanoassemblies by organosilica encapsulation, (iii) growth of the outer silica shell and surface functionalization of the streptavidin (SA) and (iv) the modification of aptamers.
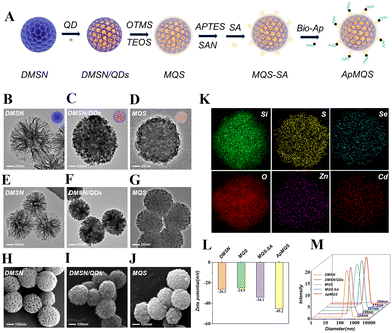 |
| Fig. 3 (A) Schematic of the synthesis route of the high-performance fluorescent nanoprobe ApMQS. (B)–(J) TEM and SEM results of the high-performance fluorescent material MQS synthesized at different stages. TEM images of DMSN (B), DMSN/QD (C), and MQS (D) at 100 K magnification. TEM images of DMSN (E), DMSN/QD (F), and MQS (G) at 50 K magnification. SEM images of DMSN (H), DMSN/QD (I), and MQS (J) at 25 K magnification. (K) Resulting graphs of the EDS mapping of MQS. The zeta (ζ) potential (L) and the hydrodynamic diameter distributions (M) of ApMQS in different stages. | |
DMSN was synthesized using hexadecyltrimethylammonium bromide (CTAB) and sodium salicylate (NaSal) for the unique central radial channels. The well-defined pores and channels of the DMSN spheres were illustrated in Fig. 3B and 3E from TEM images and Fig. 3H from SEM images. Due to the presence of wide radial pores, the inner surface of DMSN was accessible for loading nanoparticles. With thiol-groups grafting, the QD surface metal was anchored onto the silica surface using (3-mercaptopropyl) trimethoxysilane (MPTS). As shown in Fig. 3C and 3F, the robust coordination between the alkylthiols and the metal atoms resulted in the complete occupation of pore walls by the oleic acid-capped QDs, probably triggering through the partial exchange of oleic acid ligands with mercaptopropyl groups. The same result was indicated in the SEM image (Fig. 3I), with the radial pores occupied by QDs. After silanization with n-hexane octyl trimethoxysilane (OTMS) on these hydrophobic DMSN/QDs assemblies and encapsulation with an organosilica/silica bilayer, MQS with a dense silica shell were obtained, as the growth of the silica layer smoothed the porous surface (Fig. 3D, G and J).
The elemental (Si, S, Se, O, Zn and Cd) distribution was determined by energy dispersive spectrometry (EDS) mapping, which confirmed the assembly of QDs in DMSN and their encapsulation with the silica shell (Fig. 3K). In this structure, S, Cd, Zn and Se form the core–shell structure of the QDs, while Si and O are the most abundant elements in MQS due to the predominant presence of SiO2 in the microsphere framework. After surface functionalization with SA and labelling with biotinylated aptamers, ApMQS was obtained (Fig. 3A).
The UV-Vis absorption spectrum and the fluorescence emission spectrum of ApMQS are shown in Fig. S1 (ESI†). ApMQS exhibited a distinct absorption peak at the wavelength of 365 nm. Under excitation at 365 nm, ApMQS emitted fluorescence at 625 nm. To ensure the whole synthesis proceeded as expected, the surface zeta (ζ) potential and the hydrodynamic diameter at each stage were measured using a ζ potential analyzer and a dynamic light scattering (DLS) particle size analyzer, respectively. As shown in Fig. 3L, the initial ζ potential value of DMSN was −26.3 mV. When the organic silica layer was formed on MQS, the ζ potential reached −24.9 mV, which is close to that of DMSN. After coupling with SA and aptamers which carried negative electricity, the ζ potential of ApMQS decreased to −45.2 mV. Throughout the synthesis of ApMQS, DMSN continuously loaded QDs, coupled with SA, and reacted with aptamers, resulting in a progressive increase in hydrodynamic diameter from 224 nm of DMSN to 289 nm in ApMQS (Fig. 3M). This change in diameter was consistent with observations from the TEM and SEM results, indicating the successful preparation of the high-performance fluorescence nanoprobe ApMQS. Furthermore, after coupling with three different aptamers (Scov1, Scov2, Scov3), there was no significant difference in the hydrated particle sizes of the three ApMQSs (Fig. S2, ESI†).
Construction and principle of the MARQ-LFIA platform
The MAQR-LFIA POCT system was constructed by ApMQS-based LFIA strips. The strips consist of the sample pad, conjugate pad, nitrocellulose (NC) membrane and absorbent pad (Fig. 1): (i) the sample pad is the initial point of contact for the sample added to the strip, absorbing and transferring the sample to the conjugate pad. In the detection process of MAQR-LFIA, a nasal swab collected from a COVID-19 positive patient was dispersed in the extraction buffer to separate the N protein. (ii) The conjugate pad contains the functionalized ApMQS to identify and capture the SARS-CoV-2 N protein, initiating the detection reaction, which is the most important step in the whole detection process. (iii) The NC membrane is the central part of the test strip where separation and detection occur, and contains immobilized capture antibodies that are arranged in the test (T) line and control (C) line. Finally, the sandwich complex (ApMQS-N protein–antibody) was formed at the T line, which directly determined the output of the final signal (Fig. 1). (iv) The absorbent pad serves as the endpoint for the test, and can ensure consistent flow and collect excess sample. These components work in harmony to provide a rapid, reliable diagnostic test. After 15 min detection time, the quantitative and more accurate analysis was achieved by using a high-gain portable fluorescence reader (Fig. 1). The internal LED of the portable fluorescence reader emitted light at a wavelength of 365 nm, and excited the ApMQS's fluorescence signals. The fluorescence emission signal from the T and C lines was filtered by a 625 nm pass filter and detected continuously by a photodiode. The photodiode collected and transformed fluorescence signals into data signals. The ratio of the integrated areas under the T and C lines (T and C values) was obtained from the scan curve, and used to quantify the N protein concentration (Fig. 1). The appearance and internal structure of the portable fluorescence reader are shown in Fig. S3 (ESI†).
The MARQ-LFIA platform for N protein detection
To evaluate the performance of MARQ-LFIA in COVID-19 detection, an assay was performed using the N protein. Notably, the fluorescence signal of the T line was gradually enhanced with the increase of N protein concentration (Fig. S4, ESI†). The visualization results were consistent with the portable fluorescence reader detection results (Fig. S5, ESI†). The migration of ApMQS on the T line at different N protein concentrations was confirmed through fluorescence imaging photographs and SEM images (Fig. 4A). In the positive test with a concentration of 5 ng mL−1 N protein, the T line exhibited a strong fluorescence signal under UV light, while the SEM image showed a substantial accumulation of ApMQS-N protein immune complex on the T line (Fig. 4A), confirming the efficient capture and detection capability of our MARQ-LFIA system. When the N protein concentration was reduced to 500 pg mL−1, the quantity of ApMQS captured by the T line decreased, accompanied with the attenuation of the fluorescence signal (Fig. 4B). For the negative test, no ApMQS was detected and there was no fluorescence signal on the T line, which was consistent with the UV light photographs (Fig. 4C). All of the above data demonstrated the feasibility of using MARQ-LFIA for the N protein detection.
 |
| Fig. 4 Photographs (left) and SEM images (right) of the MARQ-LFIA membrane under the N protein concentrations of 5 ng mL−1 (A), 500 pg mL−1 (B), 0 pg mL−1 (C). (D) Detection results of different combinations of Scov1, Scov2 and Scov3 under 100 pg mL−1 and 5 pg mL−1 N protein concentration. (T and C represent the detection values of the test and control lines under the corresponding N protein concentration, T0 and C0 represent the detection values of the T test and control lines under the blank control, respectively). (E) The standard curve of the SARS-CoV-2 N protein concentration and (T/T0)/(C/C0) value. (F) The detection results of SARS-CoV-2 and other control virus N protein (1 ng mL−1 for the SARS-CoV-2 N protein and 1 μg mL−1 for others. The red line represents the cut-off value as 1.110, where the cut-off = average of negative samples + 3 × standard deviation). (G) The standard curve of the inactivated SARS-CoV-2 concentration and (T/T0)/(C/C0) value. (H) The detection results of the positive reference P1 and negative references N1-N20 in the National Reference Panel for 2019-nCoV (SARS-CoV-2) Antigen Detection Kit. (I) The clinical positive samples detection results of the MARQ-LFIA and two commercially available SARS-CoV-2 antigen LFIA kits A (latex method) and B (fluorescence method). Error bars represent the standard deviation of three parallel experiments. * p ≤ 0.05, ** p ≤ 0.01, *** p ≤ 0.001. | |
To further enhance the sensitivity of MARQ-LFIA, we investigated the effect of the multiple aptamers recognition strategy on the detection performance. As shown in Fig. 4D and Fig. S6 (ESI†), among the results for single aptamer detection, Scov1 showed the best performance compared to Scov2 or Scov3 at a concentration of 100 pg mL−1 N protein, which was consistent with the previously obtained SPR results. Among all combinations using two or three aptamers, the group Scov1/2/3 exhibited the most outstanding fluorescence signal under 100 pg mL−1 N protein, representing the best sensitivity. This improvement was attributed to the different binding sites of these three aptamers for the N protein, providing maximum recognition efficiency. We also conducted low concentration detection under 5 pg mL−1 N protein, which further demonstrated the enhanced detection performance due to the multiple aptamer recognition strategy (Fig. 4D). All t-test results for the analysis of significant differences shown in Fig. 4D can be found in Table S5–S8 (ESI†). Additionally, we utilized AuNPs-LFIA as a visualized platform to provide more intuitive evidence. As shown in Fig. S7 (ESI†), among the different combinations of Scov1, Scov2 and Scov3, only Scov1/2/3 exhibited the highest colorimetric signal. We believe that the multiple ApMQS interacted with different binding sites on the target simultaneously, increasing the signal labeling of a single target molecule, which resulted in enhanced T line signal and improved sensitivity (Fig. 1). Unless otherwise specified, the MARQ-LFIA of multiple ApMQS containing Scov1/2/3 was used in the following studies.
Then, we further performed sensitivity and specificity tests on the MARQ-LFIA by detecting the N protein. The calculated LOD for the detection of N protein was 1.427 pg mL−1 (Fig. 4E). Compared with the reported studies, our MARQ-LFIA platform-based multiple aptamers recognition strategy and QDs fluorescence technique had better sensitivity than other LFIA platforms for the detection of SARS-CoV-2 antigens (Table 1).
Table 1 Comparison results of representative LFAs for the detection of the SARS-CoV-2 N protein
Recognition probe |
LOD |
Method |
Ref. |
Antibody–antibody |
2 ng mL−1 |
Colloidal gold LFIA |
29
|
Aptamer–antibody |
0.1 ng mL−1 |
Hybrid-LFS |
31
|
Aptamer–antibody |
2.89 pg mL−1 |
Conjugated gold nanoparticles LFIA |
32
|
Aptamer–antibody |
1 ng mL−1 |
Colloidal gold LFIA |
33
|
Antibody–antibody |
0.65 ng mL−1 |
Latex LFIA |
34
|
Multiple aptamers–antibody |
1.427 pg mL−1 |
QDs fluorescence LFIA
|
This work
|
The MARQ-LFIA platform for inactivated SARS-CoV-2 detection
Next, we performed the detection of inactivated virus using the MARQ-LFIA platform. The LOD for the detection of inactivated SARS-CoV-2 was 1643 U mL−1 (Fig. 4G). Using the standard addition method, we added 105 U mL−1 of inactivated SARS-CoV-2 to diluted nasal swab samples from five healthy individuals, and the detection results are shown in Fig. S8 (ESI†). The detection results indicated excellent recovery rates ranging from 96.96% to 105.64% (Table S9, ESI†). A Welch t-test analysis was utilized to evaluate the differences in the detection results, obtaining a p-value of 0.7019 (greater than 0.05) (Table S10, ESI†). This indicated that there was no statistically significant difference between the detection results and the theoretical values, thereby confirming the high accuracy of detecting inactivated SARS-CoV-2 in actual samples. In addition, the results obtained with MARQ-LFIA were comparable to the negative controls in the test of other virus proteins (influenza A virus H1N1, influenza A virus H3N2, coronavirus OC43, coronavirus 229E, coronavirus NL63, coronavirus HKU1), and inactivated respiratory viruses (influenza virus, adenovirus, coronavirus, enterovirus, Bordetella pertussis, Streptococcus pneumoniae and other respiratory pathogens from the National Reference Panel for SARS-CoV-2 Antigen Detection Kit) (Table S13, ESI†), demonstrating excellent specificity (Fig. 4F and H).
The MARQ-LFIA platform for clinical samples evaluation
To demonstrate the accuracy and sensitivity of MARQ-LFIA in clinical diagnosis, a comparative clinical study was conducted involving thirty COVID-19 positive samples and twenty negative samples from healthy individuals. The results were compared with clinical PCR results from the Zhejiang Provincial Center for Disease Control and Prevention. The detection results were presented in Table S11 (ESI†). The clinical sensitivity (true positive rate) of MARQ-LFIA was 86.67%, the clinical specificity (true negative rate) was 100.00%, and the clinical accuracy (overall agreement rate) was 92.00%. The kappa value for the consistency analysis was 0.8391. Therefore, there was positive consistency between the detection results from the MARQ-LFIA and PCR platform. Moreover, commercially available COVID-19 antigen immunochromatographic test kits A (latex method) and B (fluorescence method) were used to diagnose positive samples. As shown in Fig. 4I, MARQ-LFIA increased the positive detection rate from 55.17% to 86.67% compared with commercial similar products. Therefore, MARQ-LFIA demonstrated strong applicability in clinical diagnosis.
The universality of the MARQ-LFIA platform
To validate the universality of the MARQ-LFIA platform, we evaluated its detection performance for the influenza B virus nucleoprotein (Fig. 5A). Three distinct high-affinity aptamers related to the influenza B virus nucleoprotein were successfully acquired, designated as FluB1, FluB2, and FluB3 (Table S2, ESI†), through the aforementioned SELEX method. The secondary structures of FluB1, FluB2, and FluB3, which could bind to nucleoproteins, were predicted at the most stable state in Fig. 5B. The affinity curves for these aptamers are presented in Fig. 5C–E. The relevant parameters of FluB1, FluB2, and FluB3 are illustrated in Table S4 (ESI†) with the respective affinities of 7.88 nM, 6.29 nM, and 8.53 nM. These results indicated a relatively stable bonding strength between all three aptamers and the nucleoprotein, contributing to further ultrasensitive detection. The MQS was subsequently coupled with FluB1/2/3 to obtain the high-fluorescence nanoprobe ApMQS, and the MARQ-LFIA platform for influenza B detection was constructed by combining multiple ApMQS with the immunochromatographic assay technique (Fig. 5A).
 |
| Fig. 5 (A) Principle of influenza B virus nucleoprotein using the MARQ-LFIA platform. (B) The predicted secondary structures of FluB1, FluB2 and FluB3 using the RNAfold web. SPR binding-dissociation kinetic curve of FluB1 (C), FluB2 (D) and FluB3 (E). The red line represents the fitted data. (F) The standard curve of the inactivated influenza B virus concentration and (T/T0)/(C/C0) value. (G) The fluorescence values of positive reference P1 and negative references N1–N20 in the 2nd National Reference Panel for Influenza B Viral Antigen Detection Kit (the red line represents the cut-off value as 1.187, where the cut-off = average of negative samples + 3 × standard deviation). (H) The clinical positive samples detection results of the MARQ-LFIA and the commercially available influenza B antigen LFIA kits. Error bars represent the standard deviation of three parallel experiments. | |
Using the MARQ-LFIA platform, we conducted assays on virus nucleoprotein, inactivated virus and clinical samples. The LOD for the influenza B virus nucleoprotein was 2.742 pg mL−1 (Fig. S9, ESI†), and the LOD for the inactivated virus was 7274 U mL−1 (Fig. 5F). Furthermore, compared to other inactivated respiratory viruses (rhinovirus, adenovirus, coronavirus, enterovirus, Bordetella pertussis, Streptococcus pneumoniae and other respiratory pathogens from the 2nd National Reference Panel for Influenza B Viral Antigens Detection Kit) (Table S14, ESI†), the MARQ-LFIA exhibited excellent detection specificity for influenza B (Fig. 5G). At the clinical sample level, we tested fifty-seven positive samples from patients with influenza B and twenty negative samples from healthy individuals. The clinical sensitivity (true positive rate) was 77.19%, significantly eclipsing the 23.81% rate associated with similar commercial products available on the market (Fig. 5H). The clinical specificity (true negative rate) was 100.00% and the clinical accuracy (overall agreement rate) was 83.11% (Table S12, ESI†). These results demonstrated that the MARQ-LFIA platform had excellent performance in the detection of influenza B, confirming its universality in respiratory infectious disease detection.
Conclusions
We have developed a MARQ-LFIA platform for the highly sensitive and specific rapid detection of various respiratory infectious viruses, including COVID-19 and influenza B. We identified three high-affinity aptamers targeting the SARS-COV-2 N protein (Scov1, Scov2, Scov3) and influenza B virus nucleoprotein (FluB1, FluB2, FluB3), respectively. By integrating QDs fluorescent technique with the multiple aptamers recognition strategy, we constructed a high-fluorescence nanoprobe ApMQS, which was successfully applied to the construction of a high-performance MARQ-LFIA platform. In assessing MARQ-LFIA's capability to detect COVID-19, we combined it with a high-gain portable fluorescence reader. The LODs for the N protein and inactivated virus were 1.427 pg mL−1 and 1643 U mL−1, respectively, showcasing its enhanced sensitivity compared to previously reported LFIA-based sensors. MARQ-LFIA also exhibited exceptional specificity in detecting COVID-19, as confirmed through tests against other respiratory viruses. Compared with commercial kits, our MARQ-LFIA platform demonstrated superior performance in the detection of clinical samples, with an increase in positive patient detection from 55.2% to 86.7%. For the universality study, another MARQ-LFIA was established for investigating diagnosis and showed excellent performance in the detection of influenza B. The MARQ-LFIA platform is rapid, convenient, ultrasensitive, and specific, making it suitable for the rapid detection of respiratory infectious diseases in diverse settings, such as homes, customs airports, grassroots communities, rural areas, and emergency departments. This contributes significantly to enhancing society's ability to respond to major diseases and emerging public health safety challenges.
Data availability
The data supporting this article have been included as part of the ESI.†
Conflicts of interest
There are no conflicts to declare.
Acknowledgements
This work was financially supported by the National Key Research and Development Program of China (2023YFC3040800), the National Natural Science Foundation of China (22304160), Hangzhou Institute of Medicine Chinese Academy of Sciences (2024ZZBS12), and Joint Funds for the innovation of science and Technology, Fujian province (2023Y9267).
References
- H. C. Maltezou, A. Papanikolopoulou, S. Vassiliu, K. Theodoridou, G. Nikolopoulou and N. V. Sipsas, Viruses, 2023, 15, 865 CrossRef CAS PubMed.
- U. Sani, O. M. Alatawi, N. M. Halawani, J. A. Gould, J. G. Knight and F. Cucinotta, Front. Chem., 2022, 10, 921112 CrossRef CAS PubMed.
- L. Bourouiba, Annu. Rev. Biomed. Eng., 2021, 23, 547–577 CrossRef CAS PubMed.
- E. J. Chow, T. M. Uyeki and H. Y. Chu, Nat. Rev. Microbiol., 2023, 21, 195–210 CAS.
- J. Lessler, N. G. Reich, R. Brookmeyer, T. M. Perl, K. E. Nelson and D. A. Cummings, Lancet Infect. Dis., 2009, 9, 291–300 CrossRef PubMed.
- S. S. Alamri, A. Alsaieedi, Y. Khouqeer, M. Afeef, S. Alharbi, A. Algaissi, M. Alghanmi, T. Altorki, A. Zawawi and M. A. Alfaleh, PeerJ, 2023, 11, e15024 CrossRef PubMed.
- N. Minhas, Y. K. Gurav, S. Sambhare, V. Potdar, M. L. Choudhary, S. D. Bhardwaj and P. Abraham, PLoS One, 2023, 18, e0277867 CrossRef CAS PubMed.
- M. C. Oliveira, K. O. Scharan, B. I. Thomés, R. S. Bernardelli, F. B. Reese, A. C. Kozesinski-Nakatani, C. C. Martins, S. M. A. Lobo and Á. Réa-Neto, BMC Pulm. Med., 2023, 23, 81 CrossRef CAS PubMed.
- C. Onwuchekwa, L. M. Moreo, S. Menon, B. Machado, D. Curcio, W. Kalina, J. E. Atwell, B. D. Gessner, M. Siapka and N. Agarwal, J. Infect. Dis., 2023, 228, 173–184 CrossRef PubMed.
- J. F. Drexler, A. Helmer, H. Kirberg, U. Reber, M. Panning, M. Muller, K. Hofling, B. Matz, C. Drosten and A. M. Eis-Hubinger, Emerging Infect. Dis., 2009, 15, 1662–1664 CrossRef PubMed.
- M. Ricco, P. Ferraro, G. Gualerzi, S. Ranzieri, B. M. Henry, Y. B. Said, N. V. Pyatigorskaya, E. Nevolina, J. Wu, N. L. Bragazzi and C. Signorelli, J. Clin. Med., 2020, 9, 1515 CrossRef CAS PubMed.
- E. Ivanova Reipold, P. Easterbrook, A. Trianni, N. Panneer, D. Krakower, S. Ongarello, T. Roberts, V. Miller and C. Denkinger, BMC Infect. Dis., 2017, 17, 707 CrossRef PubMed.
- L. Ye, X. Xu, S. Song, L. Xu, H. Kuang and C. Xu, J. Mater. Chem. B, 2022, 10, 1786–1794 RSC.
- Z. Gao, H. Ye, D. Tang, J. Tao, S. Habibi, A. Minerick, D. Tang and X. Xia, Nano Lett., 2017, 17, 5572–5579 CrossRef CAS PubMed.
- Y. Wu, Y. Zhou, Y. Leng, W. Lai, X. Huang and Y. Xiong, Biosens. Bioelectron., 2020, 157, 112168 CrossRef CAS PubMed.
- Y. Zhou, Y. Wu, L. Ding, X. Huang and Y. Xiong, Trends Anal. Chem., 2021, 145, 116452 CrossRef CAS PubMed.
- T. Yan, Z. Zhang, Q. Zhang, X. Tang, D. Wang, X. Hu, W. Zhang, X. Chen and P. Li, Microchim. Acta, 2020, 187, 653 CrossRef CAS PubMed.
- S. L. Auwardt, Y. J. Seo, M. Ilgu, J. Ray, R. R. Feldges, S. Shubham, L. Bendickson, H. A. Levine and M. Nilsen-Hamilton, Sci. Rep., 2018, 8, 15712 CrossRef PubMed.
- A. Beck, L. Goetsch, C. Dumontet and N. Corvaia, Nat. Rev. Drug Discovery, 2017, 16, 315–337 CrossRef CAS PubMed.
- K. H. Cole and A. Luptak, Methods Enzymol., 2019, 621, 329–346 CAS.
- C. Ducrot and M. Piffoux, Mol. Ther.--Nucleic Acids, 2023, 33, 254–256 CrossRef CAS PubMed.
- J. Liu, Z. Cao and Y. Lu, Chem. Rev., 2009, 109, 1948–1998 CrossRef CAS PubMed.
- J. Hoinka, A. Berezhnoy, P. Dao, Z. E. Sauna, E. Gilboa and T. M. Przytycka, Nucleic Acids Res., 2015, 43, 5699–5707 CrossRef CAS PubMed.
- H. Li, P. Er Saw and E. Song, Cell. Mol. Immunol., 2020, 17, 451–461 CrossRef CAS PubMed.
- L. Li, S. Xu, H. Yan, X. Li, H. S. Yazd, X. Li, T. Huang, C. Cui, J. Jiang and W. Tan, Angew. Chem., Int. Ed., 2021, 60, 2221–2231 CrossRef CAS PubMed.
- Y. Wei, S. Long, M. Zhao, J. Zhao, Y. Zhang, W. He, L. Xiang, J. Tan, M. Ye and W. Tan, J. Am. Chem. Soc., 2023, 146, 319–329 CrossRef PubMed.
- L. Wu, Y. Wang, X. Xu, Y. Liu, B. Lin, M. Zhang, J. Zhang, S. Wan, C. Yang and W. Tan, Chem. Rev., 2021, 121, 12035–12105 CrossRef CAS PubMed.
- Y. Song, Y. Shi, M. Huang, W. Wang, Y. Wang, J. Cheng, Z. Lei, Z. Zhu and C. Yang, Angew. Chem., Int. Ed., 2019, 58, 2236–2240 CrossRef CAS PubMed.
- H. Y. Kim, J. H. Lee, M. J. Kim, S. C. Park, M. Choi, W. Lee, K. B. Ku, B. T. Kim, E. Changkyun Park, H. G. Kim and S. I. Kim, Biosens. Bioelectron., 2021, 175, 112868 CrossRef CAS PubMed.
- J. H. Lee, M. Choi, Y. Jung, S. K. Lee, C. S. Lee, J. Kim, J. Kim, N. H. Kim, B. T. Kim and H. G. Kim, Biosens. Bioelectron., 2021, 171, 112715 CrossRef CAS PubMed.
- S. W. Wu, Y. J. Chen, Y. W. Chang, C. Y. Huang, B. H. Liu and F. Y. Yu, J. Nanobiotechnol., 2024, 22, 5 CrossRef CAS PubMed.
- J. Kim, S. Baek, J. Nam, J. Park, K. Kim, J. Kang and G. Yeom, Anal. Chem., 2024, 96, 1725–1732 CrossRef CAS PubMed.
- L. Zhang, X. Fang, X. Liu, H. Ou, H. Zhang, J. Wang, Q. Li, H. Cheng, W. Zhang and Z. Luo, Chem. Commun., 2020, 56, 10235–10238 RSC.
- B. D. Grant, C. E. Anderson, J. R. Williford, L. F. Alonzo, V. A. Glukhova, D. S. Boyle, B. H. Weigl and K. P. Nichols, Anal. Chem., 2020, 92, 11305–11309 CrossRef CAS PubMed.
- M. Sun, S. Liu, X. Wei, S. Wan, M. Huang, T. Song, Y. Lu, X. Weng, Z. Lin, H. Chen, Y. Song and C. Yang, Angew. Chem., Int. Ed., 2021, 60, 10266–10272 CrossRef CAS PubMed.
- X. Chang, C. Zhang, C. Lv, Y. Sun, M. Zhang, Y. Zhao, L. Yang, D. Han and W. Tan, J. Am. Chem. Soc., 2019, 141, 12738–12743 CrossRef CAS PubMed.
- X. Cao, S. Li, L. Chen, H. Ding, H. Xu, Y. Huang, J. Li, N. Liu, W. Cao, Y. Zhu, B. Shen and N. Shao, Nucleic Acids Res., 2009, 37, 4621–4628 CrossRef CAS PubMed.
- Y. S. Kim, J. Chung, M. Y. Song, J. Jurng and B. C. Kim, Biosens. Bioelectron., 2014, 54, 195–198 CrossRef CAS PubMed.
- Y. Huang, X. Yan, L. Zhao, X. Qi, S. Wang and X. Liang, Microchem. J., 2019, 150, 104179 CrossRef CAS.
- X. Gong, J. Cai, B. Zhang, Q. Zhao, J. Piao, W. Peng, W. Gao, D. Zhou, M. Zhao and J. Chang, J. Mater. Chem. B, 2017, 5, 5079–5091 RSC.
- X. Hu, P. Zhang, D. Wang, J. Jiang, X. Chen, Y. Liu, Z. Zhang, B. Z. Tang and P. Li, Biosens. Bioelectron., 2021, 182, 113188 CrossRef CAS PubMed.
- H. Wang, Y. Wang, W. Wang, Y. Zhang, Q. Yuan and J. Tan, Acc. Mater. Res., 2023, 4, 1083–1094 CrossRef CAS.
- Y. Liu, L. Teng, Y. Lyu, G. Song, X.-B. Zhang and W. Tan, Nat. Commun., 2022, 13, 2216 CrossRef CAS PubMed.
- Y. Wang, J. Guo, S. Liao, L. Xu, Q. Chen, G. Song, X. Zhang and W. Tan, Res. Square, 2021, 30, 1026824 Search PubMed.
- M. Chen, H. Li, Z. Shi, W. Peng, Y. Qin, R. Luo, D. Zhou, X. Gong and J. Chang, Biosens. Bioelectron., 2020, 165, 112278 CrossRef CAS PubMed.
- O. Chen, L. Riedemann, F. Etoc, H. Herrmann, M. Coppey, M. Barch, C. T. Farrar, J. Zhao, O. T. Bruns, H. Wei, P. Guo, J. Cui, R. Jensen, Y. Chen, D. K. Harris, J. M. Cordero, Z. Wang, A. Jasanoff, D. Fukumura, R. Reimer, M. Dahan, R. K. Jain and M. G. Bawendi, Nat. Commun., 2014, 5, 5093 CrossRef CAS PubMed.
- C. Y. Wen, H. Y. Xie, Z. L. Zhang, L. L. Wu, J. Hu, M. Tang, M. Wu and D. W. Pang, Nanoscale, 2016, 8, 12406–12429 RSC.
- X. Xu, A.-a Liu and D. Pang, Chem. Res. Chin. Univ., 2024, 40, 162–172 CrossRef CAS.
- S. Hong, Z. Yang, Q. Mou, Y. Luan, B. Zhang, R. Pei and Y. Lu, Biosens. Bioelectron., 2023, 220, 114880 CrossRef CAS PubMed.
- C. Lei, C. Xu, A. Nouwens and C. Yu, J. Mater. Chem. B, 2016, 4, 4975–4979 RSC.
- Y. Wu, R. D. Tilley and J. J. Gooding, J. Am. Chem. Soc., 2018, 141, 1162–1170 CrossRef PubMed.
- L. Huang, T. Liao, J. Wang, L. Ao, W. Su and J. Hu, Adv. Funct. Mater., 2018, 28, 1705380 CrossRef.
- F. Gao, Y. Liu, C. Lei, C. Liu, H. Song, Z. Gu, P. Jiang, S. Jing, J. Wan and C. Yu, Small Methods, 2021, 5, 2000924 CrossRef CAS PubMed.
- Z. Luo, L. He, J. Wang, X. Fang and L. Zhang, Analyst, 2017, 142, 3136–3139 RSC.
- Y. Peng, N. Du, Y. Lei, S. Dorje, J. Qi, T. Luo, G. F. Gao and H. Song, EMBO J., 2020, 39, e105938 CrossRef CAS PubMed.
|
This journal is © The Royal Society of Chemistry 2025 |
Click here to see how this site uses Cookies. View our privacy policy here.