Optoelectronic stimuli-driven switchable memristors with multilevel resistance states for neuromorphic vision sensors†
Received
2nd May 2024
, Accepted 7th October 2024
First published on 8th October 2024
Abstract
Advancements in next-generation artificial intelligence technology have revolutionized neuromorphic visual systems by surpassing the limitations of the visible light range. However, the intricate circuitry of artificial visual systems, which relies on traditional image sensors, processing units, and memory, poses significant obstacles to device computational speed, large-scale integration, and power consumption. In this research, synaptic devices were developed with novel optoelectronic two-terminal resistive random-access memory. In this manner, a robust neuromorphic visual system with synaptic behaviors and nonvolatile optical resistive switching achieved by optical stimuli can be developed. The proposed optoelectronic synapse exhibits a symmetric and linear conductance-update trajectory with several conductance states, enabling image-sensing memory functions and efficient neuromorphic visual image pattern recognition with robust fault resistance. The optoelectronic memristor logic function “OR” can also boost the information-sensing potential of neuromorphic visual sensors. This work aimed to advance the creation of cutting-edge optogenetic neuromorphic vision systems.
Introduction
The extraordinary ability of the human visual system to discern diverse entities has inspired the advancement of biomimetic visual systems with electronic mechanisms, enabling artificial vision in the forthcoming era of vision.1–3 An artificial visual system encompasses photoreceptors to capture visual inputs in digital images, a memory module to retain visual information, and a processing unit to execute intricate image-processing endeavors, such as pattern recognition and object detection.1,4–7 However, conventional artificial visual systems rely on discrete sensors for the artificial retina and in-memory computing devices for the artificial visual cortex, which cannot directly respond to optical stimuli. Instead, they depend on image sensor arrays to convert optical signals into electrical signals before passing them to neuromorphic chips for subsequent signal processing. This process requires additional time and energy to convert and transmit information between distinct functional components.8
Traditional von Neumann computers face obstacles in the modern era of artificial intelligence (AI) due to the inherent limitations imposed by the physical segregation of processing and memory units, which restrict computational speed and substantially increase energy consumption.9–11 Neuromorphic computing draws inspiration from the human brain, offering the benefits of robust fault tolerance, rapid computations, and low energy consumption needed for sophisticated learning, perception, recognition, and memory.12,13 Over the last few years, many artificial neuromorphic devices, including two-terminal memristors and three-terminal field-effect transistors, have been investigated to overcome the constraints imposed by the von Neumann architecture.12,14–18 Despite extensive simulation of fundamental synaptic functions, most synaptic devices modulate synaptic weight via electrical stimulation, which also limits processing speed due to the tradeoff between bandwidth connection density and interconnection.11,19 In contrast, optical stimuli present a potential operation mode for synaptic devices, offering advantages such as rapid computational speeds, reduced crosstalk, broad bandwidth, reduced energy consumption, simple parallel input, outstanding scalability, and efficient mitigation of interconnectivity problems.20,21 A physical architecture based on optoelectronic synapses is required to construct effective in-memory computing systems. Researchers have recently become interested in light-stimuli neuromorphic computing systems.22,23 Optoelectronic memristors can significantly contribute to integrating sensing, memory, and neuromorphic computing as the fundamental components of light stimuli in artificial neuromorphic computing systems. Exploring optoelectronic memristors is crucial for creating AI.
Polymethylmethacrylate (PMMA), a low-cost, high-transparency, and easily machinable dielectric polymer has garnered significant interest in application in electronic devices.24,25 The inherent properties of PMMA, such as its ability to be processed into thin films and its compatibility with various substrates, make it an excellent candidate for use in memristive devices. Previous studies have explored the resistive switching behavior in PMMA films and noted improvements in performance with the inclusion of inorganic or metal particles (e.g., ZnO) to enhance stability and switching parameters.26 ZnO semiconductor-based optoelectronic memristors are potential candidates for light-stimuli neuromorphic computer systems because of their high transparency, low cost, easy manufacturing, and broad bandgap. Oxides must be exposed to ultraviolet (UV) light to be optically stimulated. Interstitial zinc atoms and oxygen vacancies, typically considered inherent defects, are advantageous for light-controlled artificial synapses.27 However, size and shape alterations complicate the successful use of conductive filaments (CFs) in light-stimuli synaptic devices. The human brain contains approximately 1012 neurons and 1015 synapses that facilitate information transmission between neurons via synaptic connections. A synaptic device may accommodate ionic flow to resemble the behaviors observed in filament-based memristive devices. 2-Iodofluorene (2IF) is an organic semiconductor with a wide bandgap (4.8 eV), excellent transparency, and strong UV light responsiveness. Incorporating 2IF into the device structure significantly enhances the photoresponsiveness of the synaptic device. The synergy between ZnO and 2IF allows for efficient charge carrier generation and separation under UV illumination, facilitating robust and stable resistive switching behavior. To the best of our knowledge, no previous studies have addressed the impact of 2IF on optically controlled synaptic devices.
In this study, an optical stimuli synaptic device with a straightforward two-terminal structure of Ag/PMMA/ZnO/2IF/Ag for sensing UV light was engineered. Volatile and nonvolatile resistance switching modes were optically triggered to observe the optically tunable synaptic behaviors. The proposed device exhibits stable synaptic properties, such as high linearity, repetitive potentiation/depression cycles, prolonged DC endurance for neuromorphic computing, and multistate data storage contingency in response to incident light intensity. Thus, this device is suitable for image-sensing applications. Furthermore, simulations of neuromorphic visual systems enable the precise and energy-efficient recognition of handwritten digits. Given their outstanding performance, optoelectronic artificial neural networks (ANNs) are promising for next-generation in-sensor AI systems.
Results and discussion
Device structure and optical characteristics
Fig. 1(a) depicts synaptic devices replicating synaptic functions. These devices are electrically stimulated, optically stimulated, and photoelectric synergetic synaptic devices based on electrical and optical signal stimulation. Fig. 1(b) shows a schematic of two-terminal optoelectronic memristors based on PMMA/ZnO/2IF. This device employs 2IF as the charge-trapping layer and responds to light stimuli for both inhibitory and excitatory synaptic behaviors. For the ZnO film to serve as the active layer, the trapped holes/electrons in 2IF may linearly change the conductance of ZnO. Thus, the conductance update trajectory must have a nearly linear relationship. Fig. 1(c) shows the developed optoelectronic PMMA/ZnO/2IF film demonstrating the potential of PMMA/ZnO/2IF for large-scale applications. We investigated optoelectronic characteristics using individual devices, and neural network simulations were based on these characteristics. The prepared PMMA/ZnO/2IF films were examined via X-ray diffraction (XRD). The patterns revealed that the films were amorphous (Fig. S1, ESI†). Fig. 1(d) and (e) show the diagram of the UV-assisted ZnO NP-activated synapse array and its potential application as a helmet screen for quick actions. Fig. 1(f) shows the Raman spectrum of PMMA/ZnO/2IF, in which multiple peaks are observed. The Raman bands at 472, 596, 807, 979, 1115, 1158, 1446, 1728, 2842, and 2947 cm−1 are attributed to ZnO, vs(C–C–O), vs(C–C–O), O–CH3, v(C–C), va(C–O–C), δa(C–H) of α-CH3, v(C
O), O–CH3, and vs(C–H) of O–CH3 with vs(C–H) of α-CH3 and va(CH2), respectively.28 The peak at 472 cm−1 corresponds to the nonpolar optical phonon mode of ZnO.29 The Raman peaks corresponding to vs(C–C–O), O–CH3, v(C
O), and vs(C–H) typically represent symmetric stretching vibrations in the polymer chain. The γa(C–O–C) peak typically represents the asymmetric stretching vibration, and the δa(C–H) peak of α-CH3 represents the chemical shift of the stretching vibration in fluorine derivatives.30 The Raman spectra indicate a high degree of uniformity on a macroscopic scale. This uniformity is crucial for device performance and reliability and ensuring the reproducibility of electrical properties. While filament formation may inherently involve stochastic processes, the observed uniformity and reproducibility across the material suggest that filament formation is likely to occur consistently across all devices. The material composition and structural characteristics are consistent across the device, suggesting reliable electrical behavior and enhanced device-to-device reproducibility. The Raman spectra of PMMA, PMMA:ZnO, PMMA/ZnO/2IF, and 2IF and their corresponding bands are shown in Fig. S2 (ESI†). Fig. 1(g) shows the strong absorption peaks in the UV region with and without a 2IF-doped PMMA/ZnO device. This absorption eventually fades to zero for wavelengths exceeding 600 nm. The results were determined based on density functional theory (DFT). The 2IF material has strong absorbance in the UV range, as shown in Fig. S3(b) (ESI†). Overall, optical synapses based on PMMA/ZnO/2IF may be activated by light irradiation at a wavelength of 365 nm.
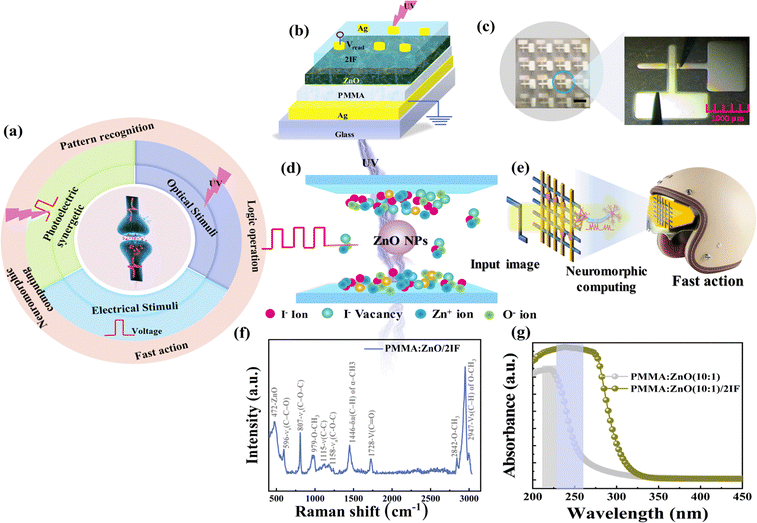 |
| Fig. 1 Characterizations of the optoelectronic memristor based on the PMMA/ZnO/2IF devices. (a) Schematic of biological neurons and applications. (b) Schematic of the optoelectronic memristor. (c) Photograph of a 2.5 cm × 2.5 cm PMMA/ZnO/2IF film. (d) UV-assisted ZnO NP activated synapse array. (e) The helmet screen can be designed as a synapse array. When external stimuli enter the synapse array, it can immediately recognize dangerous things and signal the central processing unit (CPU) to prompt the driver to take quick action to avoid them. The process involves the camera taking pictures and sending the signals to the CPU, recognizing the danger and acting accordingly. This architecture allows for faster responses compared to traditional methods. (f) Raman spectra of PMMA/ZnO/2IF on a glass substrate. (g) Optical absorption spectra of PMMA/ZnO/2IF and pristine PMMA/ZnO. | |
Optoelectronic tunable synaptic modulator
Fig. 2(a) shows a schematic of the PMMA/ZnO/2IF-based two-terminal optoelectronic memristor synapse. An initial electrical analysis was performed by grounding the Ag electrode and switching the voltage on the bottom Ag electrode. While the device remains stable in the high-resistance state over a voltage window of −3 V to 2.5 V under dark conditions, switching between high- and low-resistance states occurs within a more restricted voltage range, as demonstrated in Fig. 2(b). We have performed a detailed analysis of the set and reset voltages of our PMMA/ZnO/2IF-based devices both under UV light and in the absence of light, as shown in Table S1 (ESI†). The current–voltage (I–V) characteristics of the various concentrations of the PMMA/ZnO memristor and PMMA/ZnO/2IF and the corresponding set/reset and ON/OFF ratios are shown in Fig. S4 (ESI†). The device entails bipolar switching behavior with sudden SET and RESET. Fig. 2(b) shows the I–V curves of the PMMA/ZnO/2IF device under dark and UV light conditions (365 nm, 900 μW cm−2) using an optoelectronic resistive random-access memory (RRAM). The HRS of the system was maintained at a low current level when kept in the dark at room temperature. When a positive (negative) voltage sweep was applied to the device, a SET (RESET) transition was observed at a voltage of 2.4 V (1.5 V), and the ON/OFF ratios were determined at 4.52 V for the ON state and 1.2 V for the OFF state. By contrast, when exposed to UV light of 365 nm wavelength (UV LED: 900 μW cm−2), an abrupt increase in current implies a resistive transition from the HRS to LRS at a lower voltage. Without UV light, this transition occurs at a higher voltage, as the device still exhibits resistive switching but with increased voltage due to lower photoconductivity. Consequently, the optoelectronic memristor shifted to a relatively low set (reset) and a wide ON/OFF ratio at 1.2 (0.8) and 5.40 V, respectively. The observed reduction in the SET voltage under UV illumination can be attributed to the enhanced photoconductivity of the PMMA/ZnO/2IF-based memristor. When exposed to UV light, the 2IF layer absorbs photons and generates electron–hole pairs, significantly increasing charge carriers within the device. These additional carriers reduce the potential filament formation barrier, lowering the voltage required to initiate the SET process. This photogate effect facilitates the migration of Ag+ ions and the subsequent formation of conductive filaments at a reduced SET voltage. The device could read the current level at a subthreshold voltage of 0.1 V while switching between the HRS and LRS using the SET and RESET voltage pulses. This change from the HRS to LRS is equivalent to a “writing” function in the storage of data devices. The UV light performance indicates that the LRS can be well preserved even after removing the UV light, further demonstrating the nonvolatile nature of the PMMA/ZnO/2IF-based device. However, to return the device to its HRS, the applied voltage must be adjusted to a specific negative value, which ensures the device exhibits optimal characteristics such as low leakage current and high stability. The change from the LRS to HRS in digital memory systems corresponds to “erasing” a process. The device remains in the HRS when a continuous voltage sweep within the −3 to 2.5 V range is applied. In the device proposed in this study, reverse bias was needed for resistance switching between the LRS and HRS or vice versa; incidentally, RRAM devices frequently entail bipolar resistive switching behaviors.31,32 Furthermore, previous studies have demonstrated that RRAM devices with top electrodes scaled to the nanoscale maintain equivalent switching capabilities.33,34 This configuration provides the opportunity for ultrahigh integrated ANNs. The device also entailed highly reliable switching between progressive SET and RESET.
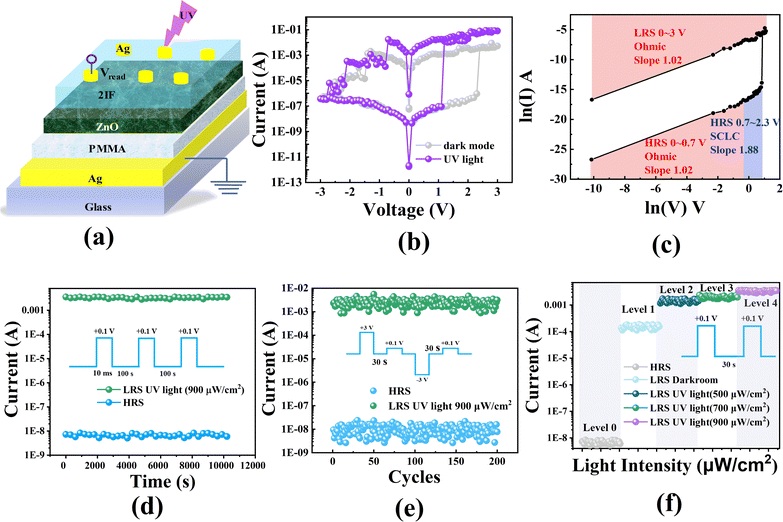 |
| Fig. 2 (a) Schematic of the memristors used for optical measurements. (b) Optoelectronic resistive switching properties of memristors with and without UV light. (c) Fitted I–V curve of the memristor's set procedure. (d) Electrical reliability test of memristors at >104 s for PMMA/ZnO/2IF. (e) Endurance test of memristors for 200 cycles of PMMA/ZnO/2IF. (f) Variations in multilevel PMMA/ZnO/2IF device states with light intensity and electrical pulse. Note: the device has an extremely high multilayer memory storage capacity based on the intensity of incoming light. | |
Fig. S5(c) (ESI†) shows the electrical resistive switching properties of the memristor for the PMMA/ZnO/2IF device. Different hypotheses were considered for the positive parts of the I–V curve to understand the potential principles of conduction behavior. Fig. 2(c) shows the conduction in the HRS region interrupting the ohmic conduction (I ∝ V) and space-charge-limited current (SCLC) (I ∝ V2). The SCLC model equation is expressed as SCLC = αVβ, where α is a constant, V is the applied voltage, and β is the exponent.35 The slope in the lower voltage region is ∼1.02. Therefore, ohmic conduction dominates the charge carrier transfer. The current increases exponentially in the intermediate voltage region as I ∝ Vm (m is ∼2). Therefore, the SCLC dominates the carrier transport mechanism. The substantial concentration of free-charge carriers may explain the increase in values (1.88) at this location with increasing light intensity. In the LRS, Ohm's law obeys the plot of ln(I) versus ln(V). Hence, ohmic conduction predominates in the LRS.
The addition of UV light causes the resistance in the LRS to decrease. The difference in the resistance states of the RRAM device highlights the prospective application of UV light for data encryption technologies. This optically tunable resistance effect may also be utilized in multilevel memory cells, boosting the information stored in a single cell.36Fig. 2(d) shows the capacity for data retention of the multilayer UV-assisted RRAM device. The ON and OFF currents did not significantly decrease after 104 s, demonstrating robust and durable stability. A reliability endurance test was also performed using SET and RESET cycles. The results are shown in Fig. 2(e). Using the SET optical stimulus (3 V, with UV light of 365 nm wavelength and 900 μW cm−2 intensity) and the RESET optical stimulus (−3 V, removal of UV light), the device was switched between the HRS and LRS throughout the measurement. A reading voltage of 0.1 V was used to determine the current levels. The electrical pulses are necessary to switch between the LRS and HRS fully, while UV light can modulate the resistance state to enhance the overall switching behavior. The devices did not show apparent volatility across the 200 cycles under ambient conditions; this issue has long been a concern in the memory sector.37,38Fig. 2(f) shows the different resistive states (LRS1 to LRS4) under various illumination irradiances. The UV-modulated resistive state of LRS devices may reach distinct conditions for multilevel data storage, indicating their excellent capability for neuromorphic computing applications because of their long-term durability and stability. The dark mode retention and endurance are shown in Fig. S6 (ESI†).
Optically-assisted resistive switching characterization
The optoelectronic characteristics of the PMMA/ZnO/2IF-based memristor were examined by conductive atomic force microscopy (C-AFM) under UV irradiation. Two regions were selected for each area (2.5 μm × 2.5 μm) to read the resistance phases of the film under various conditions (Fig. 3(a)). Fig. 3(a) presents the surface layer analysis of the PMMA/ZnO/2IF device under a SET voltage of 1 V in the dark, with current measurements at specific compliance currents. The resulting current was within the nanoamp range. A peak current of 17.4 nA was observed in the PMMA/ZnO/2IF thin film, corresponding to a resistance of approximately 57.5 MΩ. The minor peaks detected in CAFM suggest potential CF formation sites, with the maximum current limited to 17.4 nA. These nascent CFs are inherently unstable, often leading to a reversion of the film to its HRS. Then, the SET operation with a 1 V voltage and 900 μW cm−2 UV light was simultaneously conducted for the PMMA/ZnO/2IF thin film. Fig. 3(b) shows a comparative map of the C-AFM current, in which the current signal approaches 115.0 nA, corresponding to a resistance value of approximately 8.7 MΩ. The UV light-induced higher conduction current indeed helps in the formation of CFs. The current map of the PMMA/ZnO/2IF surface has several CF spots due to the emergence of local electron–hole pairs. Thus, PMMA/ZnO/2IF can be utilized to guarantee reliable memristors for memory switching. We also examine the AFM tomography images of the top surface at various stages of the structure, as depicted in Fig. S7 (ESI†). The details of the CF band mechanism are explained in the following sections.
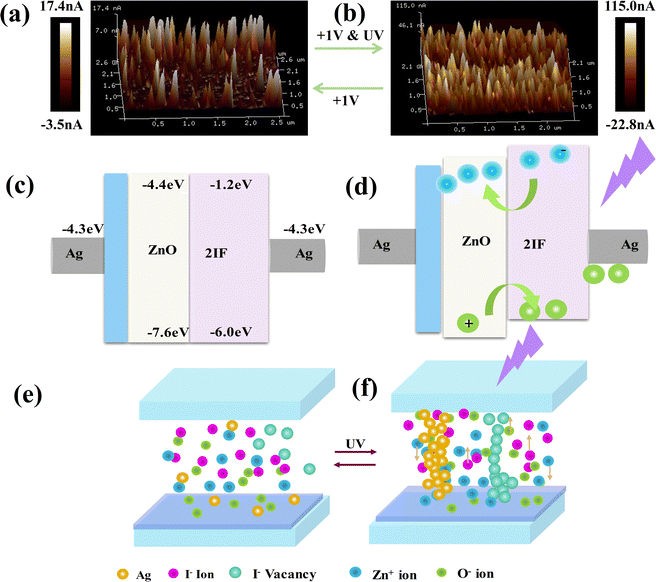 |
| Fig. 3 (a) and (b) C-AFM electronic/photonic excitation and carrier conduction under various test conditions. (c) and (d) Energy band diagram of the device in the dark and in a 365 nm UV environment. (e) and (f) Corresponding conducting state of the RRAM device. | |
Fig. 3(c) shows the CF model used for the PMMA/ZnO/2IF bilayer device. Fig. 3(c)–(f) further illustrate the potential resistive switching processes and the related variations in energy levels. The work function of the top and bottom electrodes of Ag is 4.3 eV.39 The n-type semiconductor ZnO has a large bandgap (3.2 eV),40 excellent transparency, and abundant oxygen vacancies.41 2IF is an organic light-sensitive semiconductor with a bandgap of 4.8 eV. The optimized HOMO–LUMO energy level diagram obtained by DFT calculations is shown in Fig. S3(c) (ESI†). The optoelectronic RRAM photoresponse characteristics can be explained as follows: a photoresponsive 2IF layer absorbs most of the incident light striking the 365 nm-illuminated device. A photovoltaic effect is produced when 2IF molecules are excited by UV photon absorption, which creates electron–hole pairs. Considering the favorable energy level alignment between ZnO and the photosensitive material 2IF, excited electrons are driven to much higher energy levels, leaving holes at the 2IF level. The excited electrons emitted by 2IF can enter the ZnO layer at the LUMO level. Defects or vacancies in the ZnO lattice can trap electrons injected into the ZnO layer. Electrons trapped in the photosensitive material and electrons injected from the ZnO layer may migrate to the Ag electrode. Under an external bias, Ag is ionized into Ag+ cations. The applied voltage stimulates Ag+ migration to the cathode through the Zn active layer. Eventually, after absorbing charge carriers, Ag+ is reduced to neutral Ag atoms, which nucleate to form nanoscale Ag filaments. With 2IF, the more photogenerated carriers are effectively separated and trapped, which enhances the photoresponse of the device. The 2IF material, being UV-sensitive, generates additional electron–hole pairs when exposed to UV light, contributing to the improved performance of the optoelectronic memristor. The photogate effect is crucial for the subsequent steps of vacancy filament and metal filament production. The collected electrons have the potential to form CFs under specific circumstances, creating a low-resistance channel for charge transport between the top and bottom electrodes. According to the device programming, the RRAM device is in one particular resistive state before illumination, which might be either high resistance (off state) or low resistance (on state). Iodine ions (I−) can migrate toward the direction of the positively biased electrode (i.e., the Ag electrode on top) because they are negatively charged. However, the migration of I− ions alone is insufficient to form a robust filament capable of sustaining high current conduction. Similarly, zinc ions (Zn+) may travel toward a negatively biased electrode (i.e., the Ag electrode at the bottom).
Emulation of synaptic plasticity
In the context of biological neural networks (BNNs), excitatory or inhibitory neurotransmitters can be released at the presynapse in response to stimulation, and these neurotransmitters subsequently connect to postsynaptic receptors to form excitatory postsynaptic currents (EPSCs) or inhibitory postsynaptic currents,42 as shown in Fig. 4(a). An optoelectronic memristor postsynaptic current can be produced when optical stimuli are applied, allowing many crucial forms of synaptic plasticity. Postsynaptic neurons contain EPSCs, which are essential for controlling the excitation activity of neurons. The PMMA/ZnO/2IF-based device proposed in this work can simulate excitatory synaptic responses in an optical stimulus owing to its versatile photoresponse with prolonged decay time. Fig. 4(b) shows EPSCs triggered by optical stimuli at 365 nm wavelength of 900 μW cm−2 and electrical stimuli at 1 V, with 50 pulses at 10 ms intervals, showing the response to varying pulse conditions. Here, a reading voltage of 1 V was used to measure the current. When the light illumination is turned off, the device current might continue to flow at a high level rather than instantly reverting to its previous state. Subsequently, the spatial Fermi distribution of the trapped photogenerated charges in the charge-trapping layers, such as the holes in 2IF and the electrons in ZnO, causes the trapped charges close to the interface to be released much more quickly than the charges farther from the interface. Hence, the photocurrent of the PMMA/ZnO/2IF device slowly decayed (∼80 ms) even after removing the external light. This phenomenon indicates its potential for optical sensing and storage without data conversion or transmission.
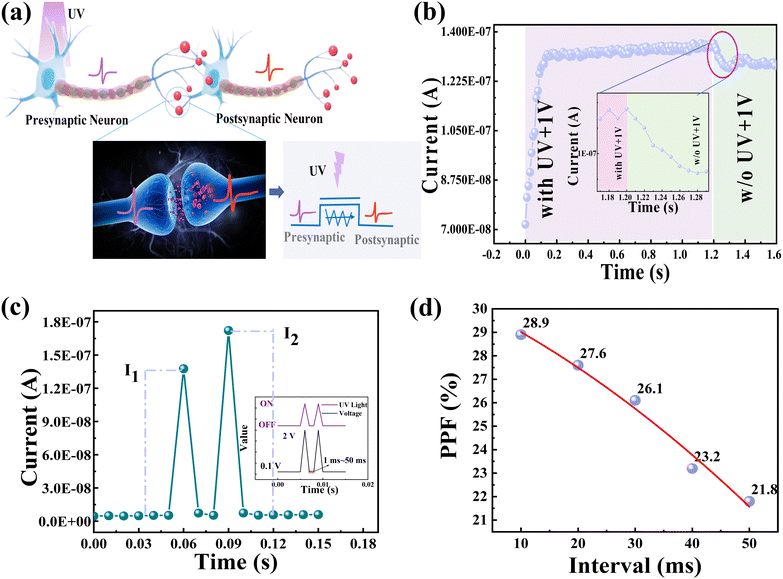 |
| Fig. 4 PMMA/ZnO/2IF-based entirely light-modulated synaptic features. (a) Schematic of a synapse and a BNN. The electrical circuit for the optical synapses is depicted in the right panel. (b) A UV pulse triggers the EPSC. (c) A postsynaptic current is generated by two successive light pulses delayed by 10 ms. (d) The photonic PPF interval between successive pulses and the simulated curve. | |
In biology, paired-pulse facilitation (PPF) is a crucial form of short-term plasticity that helps excitatory synapses identify and decode temporal data in visual synapses.43,44 In this work, we observed the EPSC when two subsequent pulses were applied with varying pulse intervals (Δt, 10–50 ms). The PPF behavior of the PMMA/ZnO/2IF-based optical synapse was demonstrated by the first and second pulses.45 The EPSC triggered by the second spike was much greater compared to the first spike (Fig. 4c). This enhancement can be attributed to the accumulation of trapped electrons in the ZnO films and trapped holes in the 2IF films (Fig. 4d). The following equations were used to calculate this phenomenon:46
| PPF = |(I2 − I1)|/I1 × 100% | (1) |
where
I1 and
I2 represent the currents measured at the first and second pulse stimuli, respectively.
Neuromorphic visual system and image reorganization
Fig. 5(a) shows the sensing, memorization, and analytical results of visible information in the human visual system. The retina views visible information from the external environment before this information is transmitted to the visual cortex in the brain for memory processing.47Fig. 5(b) shows the simulation of a three-layer ANN with one hidden layer. Here, the backpropagation technique was used to perform supervised learning on handwritten digit recognition, especially on images (resolution: 28 × 28 pixels) derived from the Modified National Institute of Standards and Technology (MNIST) dataset. As shown in Fig. 5(c), the synaptic weight of the network can be expressed as the difference in conductance between two equivalent optical synapses, i.e., W = G+ − G−.48,49 The accuracy and efficiency of neuromorphic computing rely heavily on the linearity and symmetry of the weight update trajectory, and the quantity of effective conductance states.49,50 The long-term potentiation (LTP) and long-term depression (LTD) curve features were initially investigated to determine the potential of the proposed optical synapse for application in computational neuroscience. LTP/LTD means the weight increase/decrease behaviors for the synaptic device after continuous multiple stimuli. They usually reflect the learning/forgetting ability of neuro devices.51 Fig. S8(a) (ESI†) shows the device subjected to 20 successive laser pulses with a wavelength of 365 nm. The same potentiation and depression pulses may be utilized to increase or decrease the conductance of the memristive synapse. The following equations were used to assess the linearity and symmetry of LTP/LTD for the PMMA/ZnO/2IF-based artificial synapses:51 | GLTP = B(1 − e(−P/A)) + Gmin | (2) |
| GLTD = B(1 − e(P−Pmax/A)) + Gmax | (3) |
| 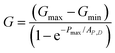 | (4) |
|  | (5) |
where GLTP and GLTD represent the conductances of potentiation and depression, respectively; Gmax, Gmin, P, and Pmax represent the maximum conductance, minimum conductance, number of pulses, and maximum number of pulses necessary for the device to switch between the minimum and maximum conductance states, respectively; B is a fitting constant; AP and D represent the nonlinearity (NL) values for potentiation or depression, respectively; Gp and Gd denote the conductance of LTP and LTD, respectively; and n represents the number of pulses. Fig. S8(b) (ESI†) shows the NL values for potentiation and depression obtained via MATLAB simulations. The NL values for the LTP and LTD curves were calculated to be 3.88 and −4.1, respectively. The asymmetry ratio between the LTP and LTD curves calculated according to eqn (5) was 0.27. The dynamic range (Gmax/Gmin) LTP and LTD curves (2.23) were obtained after 20 pulses. The modification of gate tuning considerably affects numerous aspects of artificial synaptic memristors, including multilevel states, linearity, dynamic range, and symmetry, thus directly influencing the accuracy of identifying patterns in ANNs.52,53 A significant density of efficient multilevel states is widely considered an essential factor for increasing network durability and training. A large conductance (G) range can increase the consumption of artificial synapse power.54 The outstanding linearity and symmetrical nature observed in the LTP/LTD curves can be explained by the modulation of the ZnO channel conductance via the strong interaction between trapped charges within the 2IF and ZnO films. This impact becomes more apparent when many effective conductance states are present.
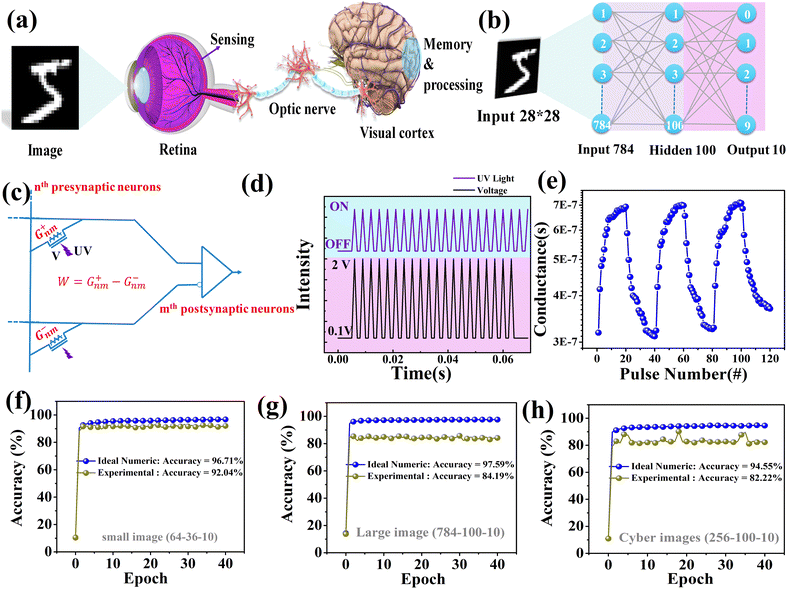 |
| Fig. 5 Simulations of PMMA/ZnO/2IF based on a neuromorphic visual system for image recognition. (a) Schematic of the human visual system, memory, and processing. (b) Biological three-layer neural network used to recognize 28 × 28-pixel handwritten digits. (c) Schematic of the simplified electrical circuit for an optical synapse. (d) Optoelectronic synaptic device subjected to optical and electrical pulses for LTP/LTD emulation. (e) Optoelectronic artificial synapse simulating the optical and electrical characteristics of LTP/LTD. (f)–(h) Recognition accuracy evolution with training epochs for small and large handwritten digits and for cyber-images of PMMA/ZnO/2IF. | |
Fig. 5(d) shows the cyclic endurance stability of the long-term potentiation and depression properties under ideal pulse settings. The experimental setup for measuring LTP/LTD typically differs from the static characterization of memristors. It is intricately linked to the intrinsic properties of the material. Selecting an appropriate pulse amplitude to prevent device breakdown due to excessive current in the LRS while preserving the LTP/LTD characteristics is crucial. For the memristor under investigation, the pulse amplitude is generally situated within the transition region between the HRS and LRS. Accordingly, we employed a pulse amplitude of 2 V/−1 V for the LTP/LTD assessments. The device was rapidly subjected to 20 electrical pulses of 2 V and optical spikes with a wavelength of 365 nm, followed by an additional 20 electrical pulses of −1 V and 20 optical spikes. As shown in Fig. 5(e), the range of memristor synapsis conductance increased (decreased) with a series of potentiation (depression) pulses and with tiny cycle-to-cycle fluctuations across the whole cycle. This phenomenon indicates electrical/optical durability and accurate synaptic weight tunability. The features of memristor synapses can imitate the potentiation and depression processes in the human brain. Fig. 5(f)–(h) show the improvement in recognition accuracy for handwritten digits as a function of the number of training epochs. The neural network size for the cyber-file datasets (images, documents, and websites) was 256 × 512 × 10, while that for the large (small) digit datasets was 784 × 300 × 10 (64 × 36 × 10). After 40 epochs, the recognition accuracies of the BNN reached 82.2% and 84.19% (92.04%) for the cyber-images and large (small) digits, respectively. Combined with the ideal floating-point numeric accuracy, this approach yields limits of 94.55% and 97.59 (96.71%) for neuromorphic algorithms for cyber-type and large (small) digits, respectively. The considerable improvement in recognition accuracy demonstrated the strong fault tolerance of PMMA/ZnO/2IF-based optoelectronic synapses. The simulated calculations based on neuromorphic recognition accuracy under dark mode conditions and for an optical stimulus over an entire cycle are shown in Fig. S9 and S10 (ESI†), respectively. These synapses offer significant potential for advancements in neuromorphic visual sensor applications.
Advancing optical logic
Synaptic integration of logic operations is highly desirable because it can considerably improve the ability of neuromorphic computing to process information. Fig. 6(a) shows the device performing “OR” optical logic operations owing to the photoresponse of the optical synapse that relies on PMMA/ZnO/2IF. Fig. 6(b) shows the setup for an optical logic operation. The two optical inputs, A and B, represent signals “0” and “1” in the absence and presence of light, respectively. The logic functions were established by modulating the output current level with an additional input. For example, optical pulses at 365 nm are considered high input, while those at 532 or 632 nm are low input. The memristors can compile the logical outcome (OUT). The output of the present OR gate shifts to high (logic “1”) when one of the inputs (A or B) is high. The outcome does not shift to low (the logic of “0”) until all inputs are low. Fig. 6(c) shows a single unit confirming the adjustable logic gate “OR.” These results provide a pathway toward incorporating in-sensor computing and parallel functioning within large-scale neuromorphic visual systems.
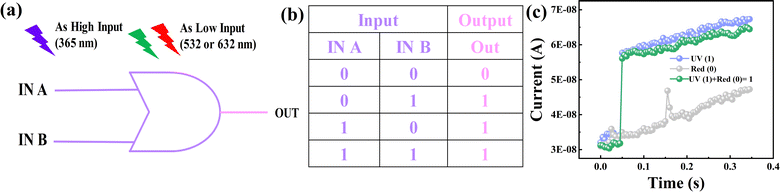 |
| Fig. 6 Optical logic operations for an optical synapse based on PMMA/ZnO/2IF. (a) Schematic of the “OR” logic operator. (b) Truth table for the “OR” optoelectronic memristor array with light pulses employed for modifying weights. (c) Realization of the “OR” logic function with 632 nm light (red(0)) as the low-level input and 365 nm light as the high-level input, respectively. UV(1) refers to OR gate output with only a 365 nm light input (high input), and Red(0) refers to OR gate output with only a 632 nm light input (low input). UV(1) + Red(0) presents the OR gate output simultaneously with 365 nm and 632 nm light inputs. The output raised high when 365 nm UV light was one of the inputs, consisting of the OR gate function. | |
Conclusions
An efficient optoelectronic PMMA/ZnO/2IF-based memristor array and an artificial visual system were constructed for in-sensor computing. The device could be regarded as a nonvolatile memristor used to perform memory tasks via optical stimulation. The optoelectronic memristors demonstrated that optical stimuli can alter both optical and electrical conductance when used in conjunction with electrical stimuli. UV light alone does not form conductive filaments easily; it assists in generating electron–hole pairs that enhance the device response. This combined approach successfully emulates EPSCs and PPFs. The optical stimuli amplified the ON/OFF ratios and enabled multilevel data storage. The visible information in the ANN for sensing memory and processing was simulated using optical synapses, proficiently identifying handwritten digits and cybersecurity images. The proposed system achieved outstanding fault tolerance and a recognition accuracy of 84.14 (92.04%) for large (small) images and 82.2% for cyber-images. Logic processing operations (“ORs”) for preventing data conversion and transmission were also demonstrated. The optoelectronic memristor array exhibited excellent potential for application in neuromorphic visual systems. The circuitry can be reduced, and the power consumption can be minimized. These advantages can aid in the efficient processing of abundant visual information from the natural world.
Experimental section
Device fabrication
The optoelectronic memristor was prepared on transparent glass (2.5 cm × 2.5 cm) as the base material. First, acetone, isopropanol, and deionized (DI) water were used to clean the substrate. Nitrogen gas was used to dry the substrate. A shadow mask (0.2 cm × 0.1 cm) was adhered to the cleaned glass substrate with heat-resistant tape to establish the position of the bottom electrode. A 100 nm thick Ag electrode was generated by a thermal coater (VPC-260F) at a vacuum gas pressure of 2 × 10−5 torr. Second, PMMA/ZnO composites with different ratios (10
:
1, 30
:
1, 50
:
1, and 100
:
1) were applied onto the substrate via spin coating at 3000 rpm for 30 seconds to prepare a resistive layer. The substrate and the bottom electrode were positioned inside a nitrogen-filled glove box with 20% humidity. Third, the coated sample was heated at 160 °C on a hot plate for 2 hours to achieve a resistive layer thickness of 200 nm. Fourth, a 100 nm-thick coating of 2IF was deposited with a thermal coater under a vacuum gas pressure of 2 × 10−5 torr. Finally, RF sputtering (SB-12) was conducted at room temperature to develop a 150 nm-thick Ag top electrode. The deposition procedure used a shadow mask at a vacuum gas pressure of 2 × 10−2 torr.
Device measurements
A Keithley 2636A probe station was used to analyze the electrical and optoelectronic properties of the optical synapse. A Tektronix AFG31052 arbitrary waveform generator was operated under ambient conditions at room temperature. A UV light source with a wavelength of 365 nm was used to illuminate the sample for optical measurements. XRD (Bruker D8-Discover) with Cu Kα radiation was used to characterize the crystalline structure of the PMMA/ZnO/2IF-based memristors. A Raman apparatus (Tokyo Instrument, Inc.) was used to examine the molecular composition of the materials. CAFM (Dimension ICON XR) was used to examine the surface of the PMMA/ZnO/2IF-based memristors. A UV-vis apparatus (Hitachi U3900) was used to examine the absorbance spectrum of the prepared PMMA/ZnO/2IF-based memristors.
Neural network simulation
The simulation of the ANN for recognition was conducted on the Cross-Sim platform.55 In Cross-Sim simulations, three categories of datasets exist: large (28 × 28 pixels) handwritten digits from the MNIST dataset,56 small handwritten digits (8 × 8 pixels) from image versions,57 and the Sandia cyberfile format with 256 byte-pair statistical features.58 A three-layer neural network was used to train the methods for classifying the datasets via backpropagation. An additional 10
000-image testing set was inputted for recognition after training by using a 60
000-image training set. An updated device conductance was used to derive the simulation parameters. For W = G+ − G−, the synaptic weight denotes the conductance difference between two equivalent synaptic devices. In this manner, positive and negative synaptic weights could be simulated.
Author contributions
Y. W. supervised the project. Y. W. and P. S. conceived and designed the project. M. L. performed the experiments, including both fabrication and characterization. S. A. performed the experiments. P. S. performed the simulations. P. S. and Y. W. analyzed the data. P. S. wrote the original manuscript. P. S. and Y. W. revised the final manuscript. All the authors discussed the results and commented on the manuscript.
Data availability
The data supporting this article have been included as part of the ESI.†
Conflicts of interest
The authors declare no conflicts of interest.
Acknowledgements
This work was supported by the National Science and Technology Council, Taiwan (110-2221-E-018-010-MY2, 111-2622-E-018-005 and 112-2811-E-018-002).
References
- Y.-X. Hou, Y. Li, Z.-C. Zhang, J.-Q. Li, D.-H. Qi, X.-D. Chen, J.-J. Wang, B.-W. Yao, M.-X. Yu and T.-B. Lu, ACS Nano, 2020, 15, 1497–1508 CrossRef PubMed.
- C. Zhang, M. Chen, Y. Pan, Y. Li, K. Wang, J. Yuan, Y. Sun and Q. Zhang, Adv. Sci., 2023, 2207229 CrossRef CAS.
- K. A. Rokade, D. D. Kumbhar, S. L. Patil, S. S. Sutar, K. V. More, P. B. Dandge, R. K. Kamat and T. D. Dongale, Adv. Mater., 2024, 2312484 CrossRef CAS.
- C. Choi, J. Leem, M. Kim, A. Taqieddin, C. Cho, K. W. Cho, G. J. Lee, H. Seung, H. J. Bae and Y. M. Song, Nat. Commun., 2020, 11, 5934 CrossRef CAS PubMed.
- Y. Kim, A. Chortos, W. Xu, Y. Liu, J. Y. Oh, D. Son, J. Kang, A. M. Foudeh, C. Zhu and Y. Lee, Science, 2018, 360, 998–1003 CrossRef CAS PubMed.
- G. J. Lee, C. Choi, D. H. Kim and Y. M. Song, Adv. Funct. Mater., 2018, 28, 1705202 CrossRef.
- A. R. Patil, T. D. Dongale, R. K. Kamat and K. Y. Rajpure, Mater. Today Commun., 2023, 34, 105356 CrossRef CAS.
- H. Tan, Y. Zhou, Q. Tao, J. Rosen and S. van Dijken, Nat. Commun., 2021, 12, 1120 CrossRef CAS PubMed.
- M. A. Zidan, J. P. Strachan and W. D. Lu, Nat. Electron., 2018, 1, 22–29 CrossRef.
- H. Li, X. Jiang, W. Ye, H. Zhang, L. Zhou, F. Zhang, D. She, Y. Zhou and S.-T. Han, Nano Energy, 2019, 65, 104000 CrossRef CAS.
- C. M. Yang, T. C. Chen, D. Verma, L. J. Li, B. Liu, W. H. Chang and C. S. Lai, Adv. Funct. Mater., 2020, 30, 2001598 CrossRef CAS.
- T. Y. Wang, J. L. Meng, Z. Y. He, L. Chen, H. Zhu, Q. Q. Sun, S. J. Ding, P. Zhou and D. W. Zhang, Adv. Sci., 2020, 7, 1903480 CrossRef CAS.
- S. Seo, B.-S. Kang, J.-J. Lee, H.-J. Ryu, S. Kim, H. Kim, S. Oh, J. Shim, K. Heo and S. Oh, Nat. Commun., 2020, 11, 3936 CrossRef CAS PubMed.
- T.-Y. Wang, J.-L. Meng, M.-Y. Rao, Z.-Y. He, L. Chen, H. Zhu, Q.-Q. Sun, S.-J. Ding, W.-Z. Bao and P. Zhou, Nano Lett., 2020, 20, 4111–4120 CrossRef CAS PubMed.
- J.-L. Meng, T.-Y. Wang, L. Chen, Q.-Q. Sun, H. Zhu, L. Ji, S.-J. Ding, W.-Z. Bao, P. Zhou and D. W. Zhang, Nano Energy, 2021, 83, 105815 CrossRef CAS.
- H. Yeon, P. Lin, C. Choi, S. H. Tan, Y. Park, D. Lee, J. Lee, F. Xu, B. Gao and H. Wu, Nat. Nanotechnol., 2020, 15, 574–579 CrossRef CAS PubMed.
- D. S. Jeon, T. D. Dongale and T. G. Kim, J. Alloys Compd., 2021, 884, 161041 CrossRef CAS.
- H. Patil, H. Kim, S. Rehman, K. D. Kadam, J. Aziz, M. F. Khan and D.-k Kim, Nanomaterials, 2021, 11, 359 CrossRef CAS.
- T. Ahmed, S. Kuriakose, E. L. Mayes, R. Ramanathan, V. Bansal, M. Bhaskaran, S. Sriram and S. Walia, Small, 2019, 15, 1900966 CrossRef.
- C. Qian, S. Oh, Y. Choi, J.-H. Kim, J. Sun, H. Huang, J. Yang, Y. Gao, J.-H. Park and J. H. Cho, Nano Energy, 2019, 66, 104095 CrossRef CAS.
- F. Ma, Y. Zhu, Z. Xu, Y. Liu, X. Zheng, S. Ju, Q. Li, Z. Ni, H. Hu and Y. Chai, Adv. Funct. Mater., 2020, 30, 1908901 CrossRef CAS.
- L. Yin, W. Huang, R. Xiao, W. Peng, Y. Zhu, Y. Zhang, X. Pi and D. Yang, Nano Lett., 2020, 20, 3378–3387 CrossRef CAS PubMed.
- Y. Wang, Z. Lv, J. Chen, Z. Wang, Y. Zhou, L. Zhou, X. Chen and S. T. Han, Adv. Mater., 2018, 30, 1802883 CrossRef.
- Y. Lin, H. Xu, Z. Wang, T. Cong, W. Liu, H. Ma and Y. Liu, Appl. Phys. Lett., 2017, 110, 193503 CrossRef.
- J. Mangalam, S. Agarwal, A. Resmi, M. Sundararajan and K. Jinesh, Org. Electron., 2016, 29, 33–38 CrossRef CAS.
- D.-I. Son, D.-H. Park, W. K. Choi, S.-H. Cho, W.-T. Kim and T. W. Kim, Nanotechnology, 2009, 20, 195203 CrossRef PubMed.
- X. Zhao, Y. Li, C. Ai and D. Wen, Materials, 2019, 12, 1282 CrossRef CAS PubMed.
- S. Chaurasia, U. Rao, A. K. Mishra, C. Sijoy and V. Mishra, J. Raman Spectrosc., 2020, 51, 860–870 CrossRef CAS.
- F. Güell, P. R. Martínez-Alanis, S. Khachadorian, J. Rubio-García, A. Franke, A. Hoffmann and G. Santana, Phys. Status Solidi B, 2016, 253, 883–888 CrossRef.
- K. Michaelian, S. Oladepo, J. Shaw, X. Liu, D. Bégué and I. Baraille, Vib. Spectrosc., 2014, 74, 33–46 CrossRef CAS.
- J. Lee and W. D. Lu, Adv. Mater., 2018, 30, 1702770 CrossRef PubMed.
- S. Goswami, A. J. Matula, S. P. Rath, S. Hedström, S. Saha, M. Annamalai, D. Sengupta, A. Patra, S. Ghosh and H. Jani, Nat. Mater., 2017, 16, 1216–1224 CrossRef CAS PubMed.
- S. Slesazeck and T. Mikolajick, Nanotechnology, 2019, 30, 352003 CrossRef CAS.
- J. J. Yang, M. D. Pickett, X. Li, D. A. Ohlberg, D. R. Stewart and R. S. Williams, Nat. Nanotechnol., 2008, 3, 429–433 CrossRef CAS PubMed.
- C. Hao, F. Wen, J. Xiang, S. Yuan, B. Yang, L. Li, W. Wang, Z. Zeng, L. Wang and Z. Liu, Adv. Funct. Mater., 2016, 26, 2016–2024 CrossRef CAS.
- Y. Li, Z. Wang, C. Zhang, P. Gu, W. Chen, H. Li, J. Lu and Q. Zhang, ACS Appl. Mater. Interfaces, 2018, 10, 15971–15979 CrossRef CAS PubMed.
- B. Cho, S. Song, Y. Ji, T. W. Kim and T. Lee, Adv. Funct. Mater., 2011, 21, 2806–2829 CrossRef CAS.
- W. P. Lin, S. J. Liu, T. Gong, Q. Zhao and W. Huang, Adv. Mater., 2014, 26, 570–606 CrossRef CAS PubMed.
- Y. Wang, Z. Lv, Q. Liao, H. Shan, J. Chen, Y. Zhou, L. Zhou, X. Chen, V. A. Roy and Z. Wang, Adv. Mater., 2018, 30, 1800327 CrossRef.
- G. Terán-Escobar, J. Pampel, J. M. Caicedo and M. Lira-Cantú, Energy Environ. Sci., 2013, 6, 3088–3098 RSC.
- U. I. Bature, I. M. Nawi, M. H. M. Khir, F. Zahoor, A. S. Algamili, S. S. B. Hashwan and M. A. Zakariya, Materials, 2022, 15, 1205 CrossRef CAS PubMed.
- S. Dai, Y. Zhao, Y. Wang, J. Zhang, L. Fang, S. Jin, Y. Shao and J. Huang, Adv. Funct. Mater., 2019, 29, 1903700 CrossRef CAS.
- N. Duan, Y. Li, H.-C. Chiang, J. Chen, W.-Q. Pan, Y.-X. Zhou, Y.-C. Chien, Y.-H. He, K.-H. Xue and G. Liu, Nanoscale, 2019, 11, 17590–17599 RSC.
- L. Yin, C. Han, Q. Zhang, Z. Ni, S. Zhao, K. Wang, D. Li, M. Xu, H. Wu and X. Pi, Nano Energy, 2019, 63, 103859 CrossRef CAS.
- C. S. Yang, D. S. Shang, N. Liu, G. Shi, X. Shen, R. C. Yu, Y. Q. Li and Y. Sun, Adv. Mater., 2017, 29, 1700906 CrossRef.
- J. T. Yang, C. Ge, J. Y. Du, H. Y. Huang, M. He, C. Wang, H. B. Lu, G. Z. Yang and K. J. Jin, Adv. Mater., 2018, 30, 1801548 CrossRef PubMed.
- X. Hou, C. Liu, Y. Ding, L. Liu, S. Wang and P. Zhou, Adv. Sci., 2020, 7, 2002072 CrossRef CAS.
- Y. Wang, Q. Liao, D. She, Z. Lv, Y. Gong, G. Ding, W. Ye, J. Chen, Z. Xiong and G. Wang, ACS Appl. Mater. Interfaces, 2020, 12, 15370–15379 CrossRef CAS PubMed.
- J. Sun, S. Oh, Y. Choi, S. Seo, M. J. Oh, M. Lee, W. B. Lee, P. J. Yoo, J. H. Cho and J. H. Park, Adv. Funct. Mater., 2018, 28, 1804397 CrossRef.
- C. S. Yang, D. S. Shang, N. Liu, E. J. Fuller, S. Agrawal, A. A. Talin, Y. Q. Li, B. G. Shen and Y. Sun, Adv. Funct. Mater., 2018, 28, 1804170 CrossRef.
- J. Yoon, B. You, Y. Kim, J. Bak, M. Yang, J. Park, M. G. Hahm and M. Lee, ACS Appl. Mater. Interfaces, 2023, 15, 18463–18472 CrossRef CAS.
- M.-K. Kim and J.-S. Lee, Nano Lett., 2019, 19, 2044–2050 CrossRef CAS.
- M. T. Sharbati, Y. Du, J. Torres, N. D. Ardolino, M. Yun and F. Xiong, Adv. Mater., 2018, 30, 1802353 CrossRef.
- K. C. Kwon, Y. Zhang, L. Wang, W. Yu, X. Wang, I.-H. Park, H. S. Choi, T. Ma, Z. Zhu and B. Tian, ACS Nano, 2020, 14, 7628–7638 CrossRef CAS PubMed.
- CrossSim Platform (accessed July 2019), https://github.com/sandialabs/cross-sim.
- Y. LeCun, L. Bottou, Y. Bengio and P. Haffner, Proc. IEEE, 1998, 86, 2278–2324 CrossRef.
- A. Asuncion and D. Newman, UCI Machine Learning Repository (Univ. of California, School of Information and Computer Science), 2007 Search PubMed.
- J. A. Cox, C. D. James and J. B. Aimone, Procedia Comput. Sci., 2015, 61, 349–354 CrossRef.
Footnote |
† Electronic supplementary information (ESI) available: XRD patterns of PMMA/ZnO/2IF and pristine (PMMA/ZnO) films with 2θ versus intensity plots; Raman spectra of PMMA, PMMA:ZnO, PMMA/ZnO/2IF and 2IF on a glass substrate; transmission spectra of 2IF materials; UV absorption spectrum of the 2IF material measured by density functional theory; HOMO–LUMO energy gap for 2IF materials; electrical characteristics: I–V characteristics of various PMMA/ZnO device concentrations and the corresponding SET and RESET; electrical characteristics: I–V characteristics of various concentrations of the PMMA/ZnO/2IF device; distributions of set/reset voltages during electrical switching cycles; electrical resistive switching characteristics of the memristor for the PMMA/ZnO device; fitted I–V curve of the set process of the memristor; electrical resistive switching characteristics of the memristor for the PMMA/ZnO/2IF device; endurance test of the memristor for 200 cycles of PMMA/ZnO; electrical reliability test of the memristor for more than 103 s for the PMMA/ZnO device; AFM of the tomographic image of the top surface of a PMMA/ZnO/2IF layer; long-term potentiation and long-term depression of the device with pulse numbers; nonlinear values of potentiation and depression of the device; and electrical and optical pulses applied on the optoelectronic synaptic device for LTP/LTD emulation and optoelectronic artificial synapses. Recognition accuracy evolution with training epochs for small and large handwritten digits and cyber-type images for PMMA/ZnO/2IF. See DOI: https://doi.org/10.1039/d4tc01796j |
|
This journal is © The Royal Society of Chemistry 2025 |
Click here to see how this site uses Cookies. View our privacy policy here.