DOI:
10.1039/D0NA00543F
(Minireview)
Nanoscale Adv., 2020,
2, 4295-4304
Microneedle-based bioassays
Received
29th June 2020
, Accepted 23rd August 2020
First published on 18th September 2020
Abstract
Disease diagnosis and therapeutic efficacy can be monitored via a number of established bioassays that sample body fluids to assess and monitor health conditions. Traditional bioassays generally include several steps and start with invasive body fluid extraction procedures. These steps are painful and often require specialized techniques and tailored equipment, as well as the supervision of professional medical personnel. Innovations in engineering alternative bioassays to address these shortcomings are thus desired. Microneedles (MNs) represent promising tools to sample body fluids, in view of their minimal invasiveness, painlessness, and uncomplicated implementation. Recent progress in microfabrication and materials engineering, including the development of hollow and solid MNs with uniquely optimized architectures and multi-functional materials, has positioned MN-based platforms as prime candidates for bioassay solutions. In this minireview, we summarize the studies of MN-based platforms for detection and diagnosis. We categorize the platforms based on three different mechanisms: MNs as body fluid reservoirs, MNs integrated with electrochemical assays, and MNs engineered with colorimetric analyses. A discussion of design principles for MN-based bioassay platforms is presented. We also discuss the challenges and opportunities associated with MN-based bioassays in future clinical applications.
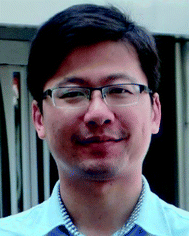 Jixiang Zhu | Jixiang Zhu is an Associate Professor working in the Department of Biomedical Engineering, School of Basic Medical Sciences, Guangzhou Medical University. He earned his Ph.D. in Materials Physics and Chemistry from Sun Yat-Sen University (China). He is interested in the development of new biomaterials, scaffolds, and devices for regenerative medicine applications. |
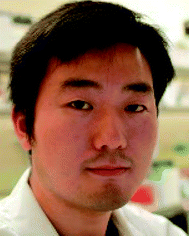 Wujin Sun | Wujin Sun is currently a faculty member at the Terasaki Institute for Biomedical Innovation. He did his postdoctoral training at the University of California, Los Angeles, and completed his Ph.D. in the Joint Department of Biomedical Engineering at the University of North Carolina at Chapel Hill and North Carolina State University. He is interested in integrating biomaterial engineering and cell engineering for healthcare applications. |
1. Introduction
Bioassays employ quantitative or qualitative analytical methods to detect biologically relevant target molecules in a living body. They play an essential role in disease diagnosis and disease management, especially in the clinic, where diverse biomarkers, metabolites, and therapeutic drugs are readily analysed from patient-derived samples.1,2 Body fluids, such as sweat, saliva, interstitial skin fluid (ISF), and blood, are some of the target samples that can be investigated.3 Of these, sweat, saliva, and ISF are composed of aqueous fluids rich in electrolytes and macromolecules, and contain an overall lower protein content. This latter property allows us to identify drugs or biomarkers in their free form, which is a much easier process, especially when compared to blood.4 Depending on the nature of the various body fluids, distinct sampling and detection strategies can be engineered. Blood sampling is a ubiquitous diagnostic approach with which a pathophysiological status is assessed.5 However, this approach is inextricably associated with invasive techniques, including hypodermic needling or finger pricking. These processes are considered undesirable and painful by many patients and pose further limitations, such as an increased risk of both localized and systemic infection. Consequently, researchers have resorted to high accuracy but less invasive alternatives to blood sampling, by targeting the skin. The skin is the largest organ of the human body, providing an abundant source of ISF, a targetable body fluid. Skin tissue undergoes constant exchange of substances with blood vessels, making ISF an alternative source for biomarker detection, albeit with a slight delay in the dynamics of analytes.6 For instance, glucose level fluctuations in ISF are generally delayed by 4–10 min compared to those in blood.7 Furthermore, from a sampling perspective, sourcing ISF is a more complicated and time-consuming technique in the clinic, though several techniques have been developed for ISF sampling, including using suction blisters,8,9 reverse iontophoresis,10 and microdialysis.11,12 Unlike blood, ISF can also be continuously monitored as it does not clot. These strategies require specialized equipment that need to be operated by trained medical professionals, making a convenient and minimally invasive bioassay technique that is capable of readily sampling target fluids highly desired.
The skin's outer epidermis structure is the major barrier to extracting body fluid albeit a soft and flexible organ. One of the skin's primary biological functions is to act as the body's physical barrier to the external world, as well as providing homeostatic regulation and sensory perception.13–15 The skin's structure can be generally divided into three layers: the epidermis, dermis, and hypodermis – from the outermost to the innermost layer. Within the epidermis, the lower portion is an avascular environment with keratinocytes and ISF perfusion.16 Cells residing in the epidermis layer are nourished by the nutrients diffused from blood microcirculation within the dermis. The dermis – or dermal layer, provides a major source for ISF sampling, and both ISF and blood in the dermis can be sampled for diagnostic applications.17 In order to reach ISF-rich tissue, sampling techniques require the disruption of the epidermal barrier and its connective tissue with the dermis. Microneedles (MNs) are known to efficiently penetrate the skin barrier with minimal invasiveness and pain, and have been used in many biomedical fields ranging from transdermal drug delivery to biosensing.18,19 Moreover, owing to their easy-to-use nature, MNs can pave the way to patient self-administering procedures, helping alleviate the demand for medical personnel and improve bioassay accessibility.
MN fabrication comprises a plethora of options, providing a versatile platform to engineer discrete geometries and architectures that can be specifically tailored to the fluid sampling requirement. For instance, MNs can be designed with a certain length, aspect ratio, and stiffness to pierce the stratum corneum (the outermost of the epidermis) and form microscale ISF extraction channels. Moreover, by tuning the geometric scale of MNs, they can be engineered to reach the blood-perfused dermis and enable direct blood extraction and detection.20,21 Furthermore, MNs can be integrated with other detection strategies. In this minireview, we summarize the designs of MN platforms for bioassay use, including hollow MNs and solid MNs with different shapes and structures (Fig. 1A). Various strategies that have been utilized for MN fabrication are discussed (Fig. 1B). We also summarize detection and diagnosis mechanisms into three distinct MN platforms: MNs as body fluid reservoirs, MN-based electrochemical assays, and MNs integrated with colorimetric analyses (Fig. 1C). Finally, we discuss the challenges and opportunities for future clinical applications of MN-based bioassay platforms.
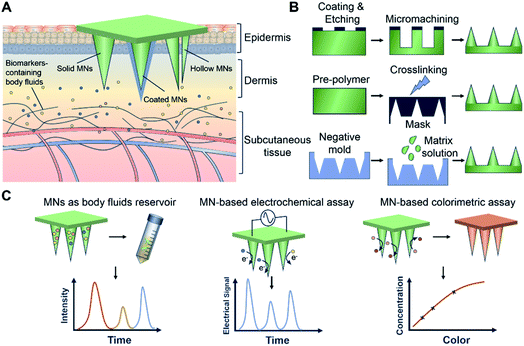 |
| Fig. 1 Summary of MN-based bioassays. (A) Structure designs of MNs, including solid MNs, hollow MNs, and coated MNs. (B) Examples of MN fabrication methods, including etching, lithography, and molding. (C) Analyte detection using MN platforms, including MNs as body fluid reservoirs, MN-based electrochemical assays, and MNs integrated with colorimetric analyses. | |
2. Design and fabrication strategies of MNs
MN-based bioassays establish a connective interface between body fluid and the MN matrix by a minimally invasive penetration of a defined area of the skin. The design and fabrication of MNs determine their specific bioassay features. Many strategies (e.g., etching,22,23 lithography,24–26 micromachining,27–29 and three-dimensional (3D) printing30–32) have been used for these purposes. Etching is one of the traditional methods to directly fabricate MNs through chemical reagents or physical ions, and is especially used to produce metal-based MNs.33 Jin et al. fabricated solid steel MN patches by laser micro-etching techniques, that were subsequently coated with reduced graphene oxide (rGO) and Pt nanohybrids. The MNs functioned as an electrochemical system for minimally invasive transdermal detection of H2O2. To avoid mechanical destruction of the Pt/rGO layer during the skin insertion process, the authors also engineered a water-soluble polymer (polyvinylpyrrolidone) that was assembled outside the nanohybrid layer. Real-time sensing of H2O2 in living mice was achieved by using the MN electrodes, with the nanohybrid coating shown to significantly improve detection sensitivity.34 In spite of their ability to detect subdermal biomarkers in situ, solid metal MN-based electrodes were unable to extract body fluid and thus presented limited use in multi-biomarker detection. Alternative designs are required to potentiate extraction capabilities for MNs, such as using hollow MNs. Through a deep-reactive ion etching method, Li et al. fabricated silicon hollow MNs with the aim of monitoring biomarkers in ISF. The MNs presented circular holes with diameters of 30 μm, and a depth of >300 μm was achieved via the efficient elimination of fluorocarbon passivation polymers by ion bombardment.35 In contrast, advances in additive manufacturing, such as 3D printing, have provided an alternative strategy to design and fabricat MNs with bespoke structures.36–38 Yeung et al. utilized stereolithography-based 3D printing to create hollow MNs interfaced with microfluidic structures. In contrast to previous work showing only 3D printed MN devices, the authors integrated microfluidics and MNs in one step. These 3D printed and microfluidic-integrated hollow MNs can be adopted in future biomedical devices targeted at transdermal drug delivery and biofluid extraction, the latter being an essential element in bioassays.39
Aside from fabricating MNs directly, the most commonly used approach for MN fabrication is molding, which can realize accurate duplicates of designed structures and allow the use of various materials otherwise unsuitable for etching and 3D printing.40–42 The designed master template can initially be produced via etching, lithography, micromachining, or 3D printing.43,44 Complementary negative molds can be produced with curable materials such as polydimethylsiloxane (PDMS). Some negative molds are commercially available with customizable designs, which can enhance the productivity, reduce the batch-to-batch variability, and facilitate access to MN fabrication techniques. To improve aspect ratios and tip sharpness of MNs, Krieger et al. developed a simple MN mold-fabrication technique using a low-cost desktop stereolithography (SLA) 3D printer. The authors printed MNs and subsequently carried out silicone casting. The female silicone mold was obtained after degassing and heating. MNs composed of carboxymethyl cellulose and polylactic acid (PLA) were successfully manufactured via this silicone mold.45 Chang et al. used stainless-steel master molds of MNs to fabricate a negative PDMS template. Hyaluronic acid (HA)-based MNs were efficiently produced through this mold, with the swellable MNs shown to be capable of rapid extraction of skin ISF for timely metabolic analysis.46
Overall, different fabrication strategies can enable the manufacture of MNs from different materials. Direct fabrication approaches (etching, lithography, micromachining, and 3D printing) are compatible with metals, silicon ceramics, and polymers. In contrast, the indirect approach based on molding is mostly for making polymer-based MNs.
Based on the aforementioned manufacturing techniques, diverse raw materials (such as metals,47,48 silicon,49,50 ceramics,51,52 and polymers53–55) have been utilized to fabricate MN patches containing arrays of solid,56,57 porous,58,59 or hollow60,61 needles. Samant et al. investigated the effects of the size of MNs on pressure-driven extraction of ISF, and the results showed a decreased volume of collected ISF with the increase of MN length between 250 μm and 750 μm. However, these effects were shown to be negligible (no statistically significant difference).62 To improve the extraction and detection efficiencies, MNs with novel microstructures or materials can be prepared by a multi-step process.63–67 For example, Mandal et al. designed a MN patch with a core–shell structure for longitudinal sampling of cells and skin ISF. The group first fabricated polylactic acid (PLA) MN arrays through PDMS molds and subsequently coated them with polylysine, alginate, and sucrose mixtures. By incorporating molecular adjuvants and specific antigen nanocapsules into the alginate hydrogel coating, antigen-specific lymphocytes could be enriched in the extracted cell populations, allowing for subsequent phenotypic and functional analysis.68 Moreover, Sulaiman et al. coated an alginate-peptide nucleic acid hybrid material into the PLA MN arrays to obtain sequence-specific sampling, isolation, and detection of nucleic acid-based biomarkers from dermal ISF.69 This core–shell MN-based technology was efficient as a diagnostic platform for biomarker extraction through hydrogel swelling and achieved the monitoring of patient-specific disease progression. Zhang et al. engineered photonic crystal (PhC) barcode-integrated MNs to enable multiplexed detection of dermal ISF biomarkers (Fig. 2). The biomarkers could be read out by fluorescence intensity and the species of biomarkers were distinguished through the reflection peaks of PhC barcodes. Compared to existing MNs used for ISF analysis, the advantages of these encoded MNs are their equivalent detection efficacies, largely simplified operational process, and simultaneous detection of multiple biomarkers.70
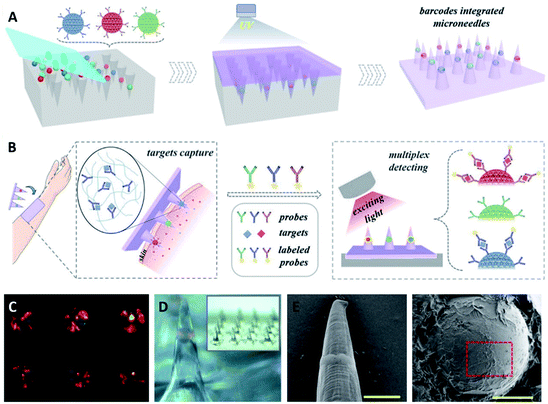 |
| Fig. 2 Fabrication and application of the encoded MNs. (A) Schematic illustration of the fabrication of the encoded MNs. (B) Schematic illustration of the application of the MNs in ISF detection. (C) Barcodes with color in negative molds. (D) Optical image of MNs with one barcode loaded in a single tip. (E) Scanning electron microscope (SEM) images of a one-barcode-loaded MN. (F) The whole view of the barcode with inverse opal structure (reproduced from ref. 70 with the permission of John Wiley & Sons, Inc, Copyright 2019). | |
3. Detection and diagnosis using MNs
Transdermal monitoring and detection of biomarkers using the MN platform are highly desirable for personalized diagnostics as well as point-of-care testing.71 MNs have the potential to meet the need of different detection strategies, including being used as body fluid sampling tools and being coupled with traditional analytical methods. In addition, MNs can be combined with transdermal electrodes for electrochemical detection, and can be embedded with chemical agents to initiate analytically relevant colorimetric reactions. Various coupled MN-detection mechanisms have been developed. Swellable hydrogels have gained significant attention for MN development in view of their biocompatibility and versatility.72,73 Their native swelling feature is also desirable to extract body fluids when applied to the skin. The hydrogel matrix can potentially trap and protect extracted cargos to enable subsequent analytical measurements. In this manner the MNs can become a reservoir of collected analytes, allowing them to be detected in later steps. Indeed, body fluid collection by absorption into hydrogel MNs requires the materials to have outstanding swelling abilities. An early study from Prausnitz's group fabricated hydrogel MN patches with crosslinked synthetic polymers poly(methyl vinyl ether-alt-maleic acid) (PMVE/MA) and poly(ethylene glycol) (PEG).74 The hydrogel MN patch demonstrated a swelling capacity of up to 50-fold in volume and was capable of extracting considerable amount of dermal ISF (about 0.84 μL per MN patch).75 Caffarel-Salvador et al. utilized the same hydrogel-forming MNs to detect caffeine and glucose in human volunteers. The MNs were able to collect sufficient dermal ISF, with the extracted caffeine- and glucose-profused ISF easily recovered through elution steps. With the support of analytical instruments, the concentrations of caffeine or glucose were found to be of comparable levels to those from patients' plasma.76 Compared to the synthetic polymer, nature-derived biomaterials have good biocompatibility. MNs based on natural polymer hydrogels can also extract body fluids efficiently and function as reservoirs. We have fabricated gelatin methacryloyl (GelMA) MNs with tuneable swelling and mechanical properties, which were achieved by altering the prepolymer concentration and crosslinking time. The optimized MNs efficiently extracted dermal ISF (about 2.5 μL per MN patch) via the excellent swelling properties of gelatin-based hydrogel. We demonstrated the detection of commonly monitored biomarkers, including glucose and vancomycin, showing comparable results between MN-based ISF detection and traditional plasma tests (Fig. 3). The use of GelMA MNs for dermal ISF extraction was therefore capable of monitoring the dynamic fluctuation of biomarker concentration.77 To collect more ISF for rapid analysis, Zheng et al. designed an osmolyte-powered hydrogel MN patch composed of maltose (osmolytes) and methacrylated HA. The osmolytes were dissolved in extracted body fluids and provided osmotic pressure during the sampling process, which extracted 7.90 μL of ISF form pigskin ex vivo, and 3.82 μL of ISF from mouse skin in vivo within 3 min.78 Another approach to make MNs a body fluid reservoir utilized a multi-component design of non-swelling MN devices. Kolluru et al. designed a MN patch comprising a two-component system that included a strip of paper (which serves as a reservoir) arranged on the back of a stainless-steel MN array.79 Upon application of the MN patch, ISF flowed out of the skin through micropores created by the MNs and got collected (about 4 μL per MN patch) in the paper reservoir. The group studied the pharmacokinetic profile of vancomycin, identifying similar trends in ISF as compared to serum. In rats immunized with the polio vaccine, serum was tested to find polio-specific neutralizing antibodies, and anti-polio IgG content in ISF was similar to that from serum.80 The applications of MNs have also been expanded to blood testing, which is believed to be a more reliable body fluid source for diagnostic purposes. Blicharz et al. developed a MN-based device for a one-step painless collection of capillary blood samples. As shown in Fig. 4, the authors integrated an array of solid MNs, a high-velocity MN insertion mechanism, stored vacuum, and a microfluidic system containing a lithium heparin anticoagulant into a single compact device. Around 100 μL of blood samples could be collected each time by the single push of a button. The use of the device was shown to be simple and straightforward, making it suitable for self-collection of blood samples at home.81
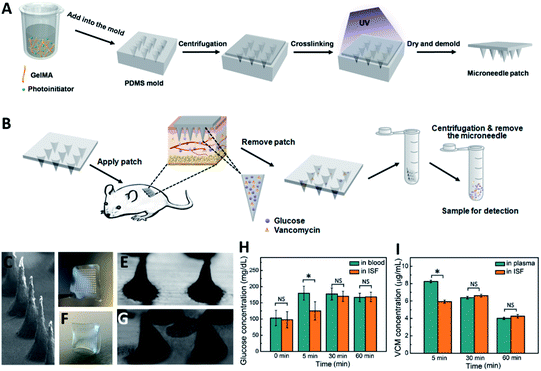 |
| Fig. 3 (A) Fabrication of the GelMA MN patch. The patch was prepared by a molding approach, in which the GelMA prepolymer was cast into a predesigned mold by centrifugation. (B) Workflow of ISF extraction and target molecule recovery using the GelMA MN patch. (C) SEM images showing the side view of the GelMA MN array. Appearance of the GelMA MN patch (D and E) before and (F and G) after pressing against the agarose hydrogel. (H) Detected glucose concentrations in ISF compared to glucose concentrations in blood. (I) Detected vancomycin (VCM) concentrations in ISF compared to VCM concentrations in plasma (reproduced from ref. 77 with the permission of John wiley & Sons, Inc, copyright 2020). | |
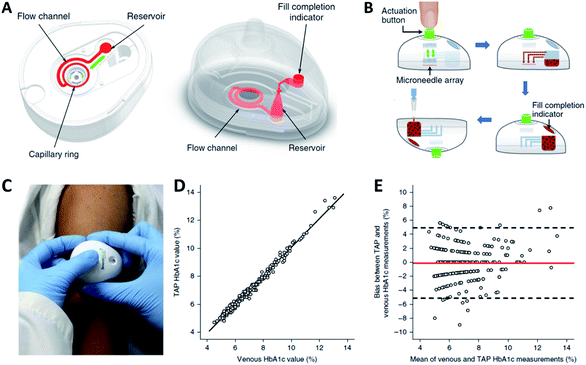 |
| Fig. 4 (A) Illustration of the blood-collection device. (B) Collection of capillary blood samples with the device. (C) Photo of the device being used. Comparison of HbA1c measurements obtained from the commercialized “TAP” device and venepuncture (n = 243) using (D) Linear regression analysis and (E) Bland-Altman analysis (reproduced from ref. 81 with the permission of Nature, Copyright 2018). | |
When the MN platform serves as a body fluid reservoir, a recovery step is normally necessary, which results in the need for further off-line analysis using laboratory equipment. To enable real-time analysis of body fluid during extraction, MNs ought to be integrated with electrochemical sensing technology.82,83 Electrodes can be inserted into MNs or MNs themselves can be engineered as electrodes by fabricating them with electroconductive materials. The detecting agents such as enzymes and chemical catalysts can be immobilized on MNs via coating, blending, or covalent bonding. A detection-relevant redox reaction can be initiated after pushing the engineered MNs into the skin, where they interact with the biomarkers in the target fluid. The sustained chemical reaction can be used to trigger an electrical signal, which can be interpreted by a detection instrument.84–86 Wang's group developed an attractive skin-worn MN sensing device for the continuous detection of organophosphate (OP) nerve agents (Fig. 5). The sensor relied on the coupling of the biocatalytic action of organophosphorus hydrolase (OPH) with hollow pyramidal MNs. The MNs were modified with carbon paste array electrode transducers, which allowed the rapid voltammetric measurement of p-nitrophenol (a product from the OPH enzymatic reaction). The MN device presented a highly linear response for methyl paraoxon in the 20–180 μM range, with high selectivity and stability when exposed to ISF.87 Given the pressing need to distinguish opioid overdoses from nerve agent poisoning, wearable MN devices can be engineered to provide a solution. A smart MN patch developed by Mishra et al. was the first sensor to accomplish continuous monitoring of fentanyl concentration down to the nanomolar level through a nanomaterial-based multi-layered architecture.88 Wang's group also designed hollow MNs integrated with Pt and Ag wires for interference-free ethanol detection in artificial ISF, which showed good sensitivity, stability, and response rates. In their case, the MN aperture was modified by electropolymerizing o-phenylene diamine onto the Pt wire micro-transducer, followed by the immobilization of alcohol oxidase in an intermediate chitosan layer along with an outer Nafion layer.89 To improve the capture efficiency for tumor-related biomarkers, Yang et al. designed a wearable platform that can capture cell-free DNA from ISF within 10 min of application, by exploiting a dual-extraction effect created by reverse iontophoresis and MNs.90 Another application of electrochemistry-based MN devices is continuous glucose monitoring, which could greatly help improve diabetes management.91,92 Bollella et al. developed a porous gold MN-based biosensor for minimally invasive monitoring of glucose. The MN electrode was prepared by gold electrodeposition, and the porous gold surface was immobilized with 6-(ferrocenyl) hexanethiol (a redox mediator) and flavin adenine dinucleotide glucose dehydrogenase. The designed MN-based biosensor showed remarkable performance for glucose detection in artificial ISF with an extended linear range, high sensitivity, stability, selectivity, and short response time.93 Kim et al. fabricated MN electrodes based on glucose oxidase, and the integrated device was made wireless to allow remote glucose monitoring via a Bluetooth-enabled mobile phone.94 Since enzyme-based MN devices have demonstrated instability in systems with unsuitable temperature or pH (due enzymatic denaturing), non-enzymatic MN sensors can provide significant advantages in glucose detection.95 Chinnadayyala et al. fabricated a stainless-steel MN patch by coating the tips with gold, Nafion, and platinum black for non-enzymatic (direct) sensing of glucose. The sensor presented good reproducibility and stability even in an acidic environment.96 In addition, electrochemical technology-based MN devices also showed various applications in ion monitoring (e.g., lithium,97 potassium98) and metabolite detection (e.g., lactate,99 urea100) in vivo.
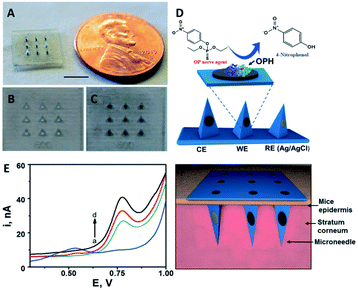 |
| Fig. 5 Schematic of transdermal OPH sensing MN. (A) Actual picture of hollow MN arrays (scale bar 10 mm). Optical images of the hollow (unpacked) (B) and carbon-paste packed (C) MNs. (D) Schematic representation of electrochemical detection of OP nerve agents using the OPH MN sensor based on the carbon- and Ag/AgCl-based MNs. (E) Ex vivo voltammetric detection of methyl paraoxon in contaminated mice skin samples using 0 (blue curve), 50 (green curve), 100 (red curve) and 150 (black curve) μM methyl paraoxon. (F) Schematic of the MN sensor array piercing the skin (reproduced from ref. 87 with the permission of Royal Society of Chemistry, copyright 2017). | |
Recently, MN platforms for bio-fluid extraction and biomarker detection via colorimetric reactions attracted significant interest, especially as they bypass the need for complex in-lab apparatus, and pave the way for future use in self-diagnosis at home.101–103 He et al. developed a hydrogel MN patch made of polyvinyl alcohol (PVA) and chitosan (CS) for point-of-care testing. After the extraction process, the target biomarkers from the MN patch were rapidly recovered by gentle heating because the PVA/CS hydrogel is water-soluble at higher temperatures. The recovered biomarkers (glucose, chlorine, lactate, or BSA) were successfully detected using a colorimetric method without the need for any bulky instrument.104 Furthermore, to simplify the detection process and improve the operability of the device for end-users, an all-in-one fluid sampling and result displaying transdermal colorimetric MN patch was developed. Wang et al. designed a double-layered MN patch with dual enzyme systems for simultaneous glucose sensing and color conversion. The coloration of 3,3′,5,5′-tetramethylbenzidine was triggered by the cascade enzymatic reactions of glucose oxidase and horseradish peroxidase at high glucose levels in dermal ISF. The color could be visualized by the naked eyes and further quantified by imaging (Fig. 6).105 Li et al. developed an all-in-one multi-diagnostic system for blood collection, serum separation, and biomarker detection. They integrated a hollow MN with a paper-based sensor to detect glucose and cholesterol in rabbits.106 Besides the aforementioned approaches, novel detection methods are emerging. A new MN platform was integrated with surface-enhanced Raman spectroscopy (SERS) for sensing chemicals in ISF. The polymeric MNs were coated with gold nanorods or silver. With the immobilization of different functional molecules (4-mercaptobenzoic acid or 1-decanethiol) in the coating layer, the platform was capable of measuring pH65 or quantifying glucose.67 In another study, Yuan et al. designed a theranostic MN probe combining optical coherence tomography imaging with laser ablation. The MN achieved real-time imaging as well as ablation of tumors deep in a mouse brain.107
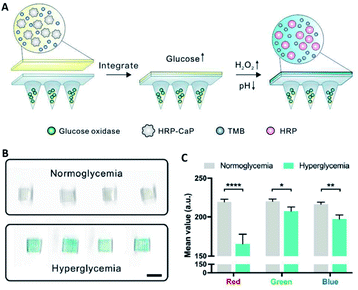 |
| Fig. 6 (A) Schematic of the glucose-responsive colorimetric MN-sensing platform triggered by both pH decrease and H2O2 generation. (B) Scanned images of the colorimetric microneedle patches after ISF extraction from four mice with normoglycemia (100 mg dL−1) and hyperglycemia (400 mg dL−1). Scale bar, 4 mm. (C) Red Green Blue (RGB) mean – value of scanned images in (B) (reproduced from ref. 105 with the permission of Elsevier Inc, copyright 2020). | |
4. Summary and outlook
MN-based bioassays are minimally invasive strategies compared to traditional sampling strategies that generally involve syringes and needles. This feature could potentially relieve the burden on medical resources, improve the efficiency of healthcare services, as well as improve patient compliance. In addition, integrative or all-in-one designs, can be achieved in MN platforms, which could further improve the user-friendly feature of these solutions, especially if test accuracy is maintained and user-dependent variability is controlled. Here, we reviewed the design and fabrication strategies of MNs with a focus on their applications for detection and diagnosis. The MN-based bioassays covered in this review are summarized in Table 1. As increasing research efforts have been devoted to MN-based body fluid extraction and bioassays, there is an emerging trend for developing better MN-based real-time assays for personalized or point-of-care devices. However, challenges remain for MN-based bioassays, and additional design strategies ought to be considered. For instance, adhesiveness could be added to current designs since sufficient extraction of body fluids requires extended time. Unstable physical adherence of MN patches may cause the MN tips to shed off and decrease the extraction efficiency. Many studies pertaining to the use of MNs in drug delivery demonstrate that MNs with barbs are able to prevent their tips from falling off the skin;108 however, this structure may not be suitable for MN-based extraction and detection since the locked MN tips may be hard to remove after body fluid extraction. Indeed, the balance between fixation and separation of MNs during body fluid extraction is critical. Furthermore, continuous efforts are needed for material innovations to improve liquid absorption capabilities of MNs. The development of MNs capable of capturing and detecting molecules from body fluids (such as antibodies, metabolites, and drug intermediates) will be an important step forward. In addition, most of the MN-based bioassay studies are tested on small animals (mice, rats, and rabbits). In order to translate their application for human use, the scale and design of MNs may need to be tailored to fit the physiological features of the human skin. A novel MN-based device capable of blood extraction has been approved by the US Food and Drug Administration (FDA).81 It paves the way for future commercialization of MN-based bioassay platforms. With continuous progress made in the development of MN devices, MN-based bioassays will serve as a minimally invasive alternative to traditional detection methods in clinics and function as a crucial intermediate step for the future of personalized healthcare.
Table 1 Summary of MN-based bioassays
Detection approach |
Structure |
Fabrication method |
Target molecule |
Reference |
Reservoir and recovery |
Hydrogel MNs |
Molding |
Glucose, cholesterol |
40,46 and 78
|
|
Hydrogel MNs |
Molding |
Vancomycin |
72
|
|
Hydrogel MNs |
Molding |
Theophylline, caffeine, glucose |
76
|
|
Hydrogel MNs |
Molding |
Glucose, vancomycin |
77
|
|
Solid MNs |
Laser etching |
Vancomycin, anti-polio IgG, |
80
|
|
Hydrogel MNs |
Molding |
Lithium |
97
|
Electrochemical assay |
Coated MNs |
Micro-etching |
H2O2 |
34 and 86
|
|
Coated MNs |
Molding |
β-Lactam antibiotic |
57
|
|
Coated MNs |
Molding and assembly |
Cell-free DNA |
66, 90
|
|
Hollow MNs |
Molding |
Organophosphate, opioid, alcohol, β-hydroxybutyrate, glucose |
87–89 and 92
|
|
Coated MNs |
Molding |
Glucose |
93–96
|
|
Solid MNs |
Assembly |
Potassium |
98
|
|
Coated MNs |
Molding |
Lactate, urea |
99 and 100
|
Colorimetric assay |
Coated MNs |
Etching |
Antigen-specific IgG |
50
|
|
Coated MNs |
Molding |
S100B (biomarker) |
54
|
|
Encoded MNs |
Molding |
TNF-α, IL-1β, IL-6 |
70
|
|
Hydrogel MNs |
Molding |
Glucose |
104 and 105
|
|
Hollow MNs |
Assembly |
Glucose, cholesterol |
106
|
Conflicts of interest
There are no conflicts to declare.
Notes and references
- M. Yang, Y. Liu and X. Jiang, Chem. Soc. Rev., 2019, 48, 850–884 Search PubMed
.
- W. Sun, J. Lee, S. Zhang, C. Benyshek, M. R. Dokmeci and A. Khademhosseini, Adv. Sci., 2019, 6, 1801039 Search PubMed
.
- T. K. L. Kiang, S. A. Ranamukhaarachchi and M. H. H. Ensom, Pharmaceutics, 2017, 9, 43 Search PubMed
.
- Q. Cheng, J. Zhang, H. Wang, D. Wang, X. Feng and L. Jiang, Adv. Mater. Interfaces, 2020, 7, 1902172 Search PubMed
.
- D.-S. Lee, C. G. Li, C. Ihm and H. Jung, Sens. Actuators, B, 2018, 255, 384–390 Search PubMed
.
- A. Than, P. Zan and P. Chen, View, 2020, 1, e21 Search PubMed
.
- M. S. Boyne, D. M. Silver, J. Kaplan and C. D. Saudek, Diabetes, 2003, 52, 2790–2794 Search PubMed
.
- J. Kool, L. Reubsaet, F. Wesseldijk, R. T. Maravilha, M. W. Pinkse, C. S. D'Santos, J. J. van Hilten, F. J. Zijlstra and A. J. Heck, Proteomics, 2007, 7, 3638–3650 Search PubMed
.
- A. C. Muller, F. P. Breitwieser, H. Fischer, C. Schuster, O. Brandt, J. Colinge, G. Superti-Furga, G. Stingl, A. Elbe-Burger and K. L. Bennett, J. Proteome Res., 2012, 11, 3715–3727 Search PubMed
.
- A. Sieg, R. H. Guy and M. B. Delgado-Charro, Clin. Chem., 2004, 50, 1383–1390 Search PubMed
.
- S. Schmidt, R. Banks, V. Kumar, K. H. Rand and H. Derendorf, J. Clin. Psychopharmacol., 2008, 48, 351–364 Search PubMed
.
- N. M. F. Voelkner, A. Voelkner, J. Costa, S. K. B. Sy, J. Hermes, J. Weitzel, S. Morales and H. Derendorf, Int. J. Antimicrob. Agents, 2018, 51, 190–196 Search PubMed
.
- A. Korosec, S. Frech, B. Gesslbauer, M. Vierhapper, C. Radtke, P. Petzelbauer and B. M. Lichtenberger, J. Invest. Dermatol., 2019, 139, 342–351 Search PubMed
.
- Y. Xia, Y. Wu, T. Yu, S. Xue, M. Guo, J. Li and Z. Li, ACS Appl. Mater. Interfaces, 2019, 11, 21117–21125 Search PubMed
.
- M. Qu, X. Jiang, X. Zhou, C. Wang, Q. Wu, L. Ren, J. Zhu, S. Zhu, P. Tebon, W. Sun and A. Khademhosseini, Adv. Healthcare Mater., 2020, 9, e1901714 Search PubMed
.
- P. Xue, L. Zhang, Z. Xu, J. Yan, Z. Gu and Y. Kang, Appl. Mater. Today, 2018, 13, 144–157 Search PubMed
.
- W. Villena Gonzales, A. T. Mobashsher and A. Abbosh, Sensors, 2019, 19, 800 Search PubMed
.
- S. Dharadhar, A. Majumdar, S. Dhoble and V. Patravale, Drug Dev. Ind. Pharm., 2019, 45, 188–201 Search PubMed
.
- F. Tasca, C. Tortolini, P. Bollella and R. Antiochia, Curr. Opin. Electrochem., 2019, 16, 42–49 Search PubMed
.
- B. Pamornpathomkul, A. Wongkajornsilp, W. Laiwattanapaisal, T. Rojanarata, P. Opanasopit and T. Ngawhirunpat, Int. J. Nanomed., 2017, 12, 885–898 Search PubMed
.
- G. S. Liu, Y. Kong, Y. Wang, Y. Luo, X. Fan, X. Xie, B. R. Yang and M. X. Wu, Biomaterials, 2020, 232, 119740 Search PubMed
.
- Y. Li, H. Zhang, R. Yang, F. Tazrin, C. Zhu, M. Kaddoura, E. J. M. Blondeel and B. Cui, Sens. Actuators, A, 2019, 292, 149–157 Search PubMed
.
- Y. Xing, J. Qian, M. A. Gosálvez, J. Zhang and Y. Zhang, Microelectron. Eng., 2020, 231, 111375 Search PubMed
.
- T.-H. Lin and J.-M. Jiang, Microsyst. Technol., 2019, 25, 4637–4643 Search PubMed
.
- S. J. Jang, T. Doshi, J. Nerayo, A. Caprio, S. Alaie, J. Auge, J. K. Min, B. Mosadegh and S. Dunham, Micromachines, 2019, 10, 705 Search PubMed
.
- Z. Chen, R. Ye, J. Yang, Y. Lin, W. Lee, J. Li, L. Ren, B. Liu and L. Jiang, ACS Biomater. Sci. Eng., 2019, 5, 5506–5513 Search PubMed
.
-
C. A. Berry, Z. R. Smith, S. D. Collins and R. L. Smith, IEEE 33rd International Conference on Micro Electro Mechanical Systems (MEMS), 2020, pp. 365–368 Search PubMed
.
- J. Chen, P. Cheng, Y. Sun, Y. Wang, X. Zhang, Z. Yang and G. Ding, IEEE Trans. Biomed. Eng., 2019, 66, 3480–3485 Search PubMed
.
-
S. Shaikh, N. Bhan, F. C. Rodrigues, E. Dathathri, S. De and G. Thakur, in Bioelectronics and Medical Devices, 2019, pp. 421–441, DOI:10.1016/b978-0-08-102420-1.00023-6
.
- W. Yao, D. Li, Y. Zhao, Z. Zhan, G. Jin, H. Liang and R. Yang, Micromachines, 2019, 11, 17 Search PubMed
.
- K. Moussi, A. Bukhamsin, T. Hidalgo and J. Kosel, Adv. Eng. Mater., 2019, 22, 1901358 Search PubMed
.
- R. M. Taylor, D. Maharjan, F. Moreu and J. T. Baca, Microsyst. Technol., 2020, 26, 2067–2073 Search PubMed
.
- K. Lee, M. J. Goudie, P. Tebon, W. Sun, Z. Luo, J. Lee, S. Zhang, K. Fetah, H. J. Kim, Y. Xue, M. A. Darabi, S. Ahadian, E. Sarikhani, W. Ryu, Z. Gu, P. S. Weiss, M. R. Dokmeci, N. Ashammakhi and A. Khademhosseini, Adv. Drug Delivery Rev., 2019 DOI:10.1016/j.addr.2019.11.010
.
- Q. Jin, H. J. Chen, X. Li, X. Huang, Q. Wu, G. He, T. Hang, C. Yang, Z. Jiang, E. Li, A. Zhang, Z. Lin, F. Liu and X. Xie, Small, 2019, 15, e1804298 Search PubMed
.
- Y. Li, H. Zhang, R. Yang, Y. Laffitte, U. Schmill, W. Hu, M. Kaddoura, E. J. M. Blondeel and B. Cui, Microsyst. Nanoeng., 2019, 5, 41 Search PubMed
.
- M. Yin, L. Xiao, Q. Liu, S. Y. Kwon, Y. Zhang, P. R. Sharma, L. Jin, X. Li and B. Xu, Adv. Healthcare Mater., 2019, 8, e1901170 Search PubMed
.
- M. J. Uddin, N. Scoutaris, S. N. Economidou, C. Giraud, B. Z. Chowdhry, R. F. Donnelly and D. Douroumis, Mater. Sci. Eng., C, 2020, 107, 110248 Search PubMed
.
- I. Xenikakis, M. Tzimtzimis, K. Tsongas, D. Andreadis, E. Demiri, D. Tzetzis and D. G. Fatouros, Eur. J. Pharm. Sci., 2019, 137, 104976 Search PubMed
.
- C. Yeung, S. Chen, B. King, H. Lin, K. King, F. Akhtar, G. Diaz, B. Wang, J. Zhu, W. Sun, A. Khademhosseini and S. Emaminejad, Biomicrofluidics, 2019, 13, 064125 Search PubMed
.
- J. Chen, M. Wang, Y. Ye, Z. Yang, Z. Ruan and N. Jin, Biomed. Microdevices, 2019, 21, 63 Search PubMed
.
- Z. Luo, W. Sun, J. Fang, K. Lee, S. Li, Z. Gu, M. R. Dokmeci and A. Khademhosseini, Adv. Healthcare Mater., 2019, 8, e1801054 Search PubMed
.
- X. Zhou, Z. Luo, A. Baidya, H. J. Kim, C. Wang, X. Jiang, M. Qu, J. Zhu, L. Ren, F. Vajhadin, P. Tebon, N. Zhang, Y. Xue, Y. Feng, C. Xue, Y. Chen, K. Lee, J. Lee, S. Zhang, C. Xu, N. Ashammakhi, S. Ahadian, M. R. Dokmeci, Z. Gu, W. Sun and A. Khademhosseini, Adv. Healthcare Mater., 2020, 9, e2000527 Search PubMed
.
- K. Badnikar, S. N. Jayadevi, S. Pahal, S. Sripada, M. M. Nayak, P. K. Vemula and D. N. Subrahmanyam, Macromol. Mater. Eng., 2020, 305, 2000072 Search PubMed
.
- K. J. McHugh, L. Jing, S. Y. Severt, M. Cruz, M. Sarmadi, H. S. N. Jayawardena, C. F. Perkinson, F. Larusson, S. Rose, S. Tomasic, T. Graf, S. Y. Tzeng, J. L. Sugarman, D. Vlasic, M. Peters, N. Peterson, L. Wood, W. Tang, J. Yeom, J. Collins, P. A. Welkhoff, A. Karchin, M. Tse, M. Gao, M. G. Bawendi, R. Langer and A. Jaklenec, Sci. Transl. Med., 2019, 11, eaay7162 Search PubMed
.
- K. J. Krieger, N. Bertollo, M. Dangol, J. T. Sheridan, M. M. Lowery and E. D. O'Cearbhaill, Microsyst. Nanoeng., 2019, 5, 42 Search PubMed
.
- H. Chang, M. Zheng, X. Yu, A. Than, R. Z. Seeni, R. Kang, J. Tian, D. P. Khanh, L. Liu, P. Chen and C. Xu, Adv. Mater., 2017, 29, 1702243 Search PubMed
.
- X. X. Yang, P. Feng, J. Cao, W. Liu and Y. Tang, ACS Appl. Mater. Interfaces, 2020, 12, 13613–13621 Search PubMed
.
- E. M. Cahill, S. Keaveney, V. Stuettgen, P. Eberts, P. Ramos-Luna, N. Zhang, M. Dangol and E. D. O'Cearbhaill, Acta Biomater., 2018, 80, 401–411 Search PubMed
.
- S. Pradeep Narayanan and S. Raghavan, Int. J. Adv. Manuf. Tech., 2018, 104, 3327–3333 Search PubMed
.
- J. W. Coffey, S. R. Corrie and M. A. F. Kendall, Biomaterials, 2018, 170, 49–57 Search PubMed
.
- B. Schepens, P. J. Vos, X. Saelens and K. van der Maaden, Eur. J. Pharm. Biopharm., 2019, 136, 259–266 Search PubMed
.
- S. Gholami, M. M. Mohebi, E. Hajizadeh-Saffar, M. H. Ghanian, I. Zarkesh and H. Baharvand, Int. J. Pharm., 2019, 558, 299–310 Search PubMed
.
- H. Juster, B. Aar and H. Brouwer, Polym. Eng. Sci., 2019, 59, 877–890 Search PubMed
.
- S. Totti, K. W. Ng, L. Dale, G. Lian, T. Chen and E. G. Velliou, Sens. Actuators, B, 2019, 296, 126652 Search PubMed
.
- P. Liu, H. Du, Y. Chen, H. Wang, J. Mao, L. Zhang, J. Tao and J. Zhu, J. Mater. Chem. B, 2020, 8, 2032–2039 Search PubMed
.
- J. Madden, C. O'Mahony, M. Thompson, A. O'Riordan and P. Galvin, Sens. Biosensing Res., 2020, 29, 100348 Search PubMed
.
- S. A. N. Gowers, D. M. E. Freeman, T. M. Rawson, M. L. Rogers, R. C. Wilson, A. H. Holmes, A. E. Cass and D. O'Hare, ACS Sens., 2019, 4, 1072–1080 Search PubMed
.
- K. Takeuchi, N. Takama, B. Kim, K. Sharma, O. Paul and P. Ruther, Biomed. Microdevices, 2019, 21, 28 Search PubMed
.
- H. Lee, K. Takeuchi, Y. Sasaki, N. Takama, T. Minami and B. Kim, IEEE CPMT Symposium Japan, 2019, 39–42 Search PubMed
.
- F. S. Iliescu, J. C. M. Teo, D. Vrtacnik, H. Taylor and C. Iliescu, Microsyst. Technol., 2017, 24, 2905–2912 Search PubMed
.
- C. Liao, W. Anderson, F. Antaw and M. Trau, ACS Omega, 2019, 4, 1401–1409 Search PubMed
.
- P. P. Samant and M. R. Prausnitz, Proc. Natl. Acad. Sci. U. S. A., 2018, 115, 4583–4588 Search PubMed
.
- X. Ning, C. Wiraja, D. C. S. Lio and C. Xu, Adv. Healthcare Mater., 2020, 9, e2000147 Search PubMed
.
- C. Kolluru, R. Gupta, Q. Jiang, M. Williams, H. Gholami Derami, S. Cao, R. K. Noel, S. Singamaneni and M. R. Prausnitz, ACS Sens., 2019, 4, 1569–1576 Search PubMed
.
- J. E. Park, N. Yonet-Tanyeri, E. Vander Ende, A. I. Henry, B. E. Perez White, M. Mrksich and R. P. Van Duyne, Nano Lett., 2019, 19, 6862–6868 Search PubMed
.
- B. Yang, X. Fang and J. Kong, ACS Appl. Mater. Interfaces, 2019, 11, 38448–38458 Search PubMed
.
- J. Ju, C. M. Hsieh, Y. Tian, J. Kang, R. Chia, H. Chang, Y. Bai, C. Xu, X. Wang and Q. Liu, ACS Sens., 2020, 5, 1777–1785 Search PubMed
.
- A. Mandal, A. V. Boopathy, L. K. Lam, K. D. Moynihan, M. E. Welch, N. R. Bennett, M. E. Turvey, N. Thai, J. H. Van, J. C. Love, P. T. Hammond and D. J. Irvine, Sci. Transl. Med., 2018, 10, eaar2227 Search PubMed
.
- D. Al Sulaiman, J. Y. H. Chang, N. R. Bennett, H. Topouzi, C. A. Higgins, D. J. Irvine and S. Ladame, ACS Nano, 2019, 13, 9620–9628 Search PubMed
.
- X. Zhang, G. Chen, F. Bian, L. Cai and Y. Zhao, Adv. Mater., 2019, 31, e1902825 Search PubMed
.
- O. Hosu, S. Mirel, R. Săndulescu and C. Cristea, Anal. Lett., 2018, 52, 78–92 Search PubMed
.
- Y. Ito, Y. Inagaki, S. Kobuchi, K. Takada and T. Sakaeda, Int. J. Med. Sci., 2016, 13, 271–276 Search PubMed
.
- H. Chang, M. Zheng, S. W. T. Chew and C. Xu, Adv. Mater. Technol., 2020, 5, 1900552 Search PubMed
.
- J. W. Lee, J.-H. Park and M. R. J. B. Prausnitz, Biomaterials, 2008, 29, 2113–2124 Search PubMed
.
- A. V. Romanyuk, V. N. Zvezdin, P. Samant, M. I. Grenader, M. Zemlyanova and M. R. Prausnitz, Anal. Chem., 2014, 86, 10520–10523 Search PubMed
.
- E. Caffarel-Salvador, A. J. Brady, E. Eltayib, T. Meng, A. Alonso-Vicente, P. Gonzalez-Vazquez, B. M. Torrisi, E. M. Vicente-Perez, K. Mooney, D. S. Jones, S. E. Bell, C. P. McCoy, H. O. McCarthy, J. C. McElnay and R. F. Donnelly, PLoS One, 2015, 10, e0145644 Search PubMed
.
- J. Zhu, X. Zhou, H. J. Kim, M. Qu, X. Jiang, K. Lee, L. Ren, Q. Wu, C. Wang, X. Zhu, P. Tebon, S. Zhang, J. Lee, N. Ashammakhi, S. Ahadian, M. R. Dokmeci, Z. Gu, W. Sun and A. Khademhosseini, Small, 2020, 16, e1905910 Search PubMed
.
- M. Zheng, Z. Wang, H. Chang, L. Wang, S. W. T. Chew, D. C. S. Lio, M. Cui, L. Liu, B. C. K. Tee and C. Xu, Adv. Healthcare Mater., 2020, 9, e1901683 Search PubMed
.
- C. Kolluru, M. Williams, J. Chae and M. R. Prausnitz, Adv. Healthcare Mater., 2019, 8, e1801262 Search PubMed
.
- C. Kolluru, M. Williams, J. S. Yeh, R. K. Noel, J. Knaack and M. R. Prausnitz, Biomed. Microdevices, 2019, 21, 14 Search PubMed
.
- T. M. Blicharz, P. Gong, B. M. Bunner, L. L. Chu, K. M. Leonard, J. A. Wakefield, R. E. Williams, M. Dadgar, C. A. Tagliabue, R. El Khaja, S. L. Marlin, R. Haghgooie, S. P. Davis, D. E. Chickering and H. Bernstein, Nat. Biomed. Eng., 2018, 2, 151–157 Search PubMed
.
- S. Zhang, H. Ling, Y. Chen, Q. Cui, J. Ni, X. Wang, M. C. Hartel, X. Meng, K. Lee, J. Lee, W. Sun, H. Lin, S. Emaminejad, S. Ahadian, N. Ashammakhi, M. R. Dokmeci and A. Khademhosseini, Adv. Funct. Mater., 2019, 30, 1906016 Search PubMed
.
- S. Zhang, Y. Chen, H. Liu, Z. Wang, H. Ling, C. Wang, J. Ni, B. Celebi-Saltik, X. Wang, X. Meng, H. J. Kim, A. Baidya, S. Ahadian, N. Ashammakhi, M. R. Dokmeci, J. Travas-Sejdic and A. Khademhosseini, Adv. Mater., 2020, 32, e1904752 Search PubMed
.
- P. Dardano, I. Rea and L. De Stefano, Curr. Opin. Electrochem., 2019, 17, 121–127 Search PubMed
.
- E. Skaria, B. A. Patel, M. S. Flint and K. W. Ng, Anal. Chem., 2019, 91, 4436–4443 Search PubMed
.
- F. Liu, Z. Lin, Q. Jin, Q. Wu, C. Yang, H. J. Chen, Z. Cao, D. A. Lin, L. Zhou, T. Hang, G. He, Y. Xu, W. Xia, J. Tao and X. Xie, ACS Appl. Mater. Interfaces, 2019, 11, 4809–4819 Search PubMed
.
- R. K. Mishra, A. M. Vinu Mohan, F. Soto, R. Chrostowski and J. Wang, Analyst, 2017, 142, 918–924 Search PubMed
.
- R. K. Mishra, K. Y. Goud, Z. Li, C. Moonla, M. A. Mohamed, F. Tehrani, H. Teymourian and J. Wang, J. Am. Chem. Soc., 2020, 142, 5991–5995 Search PubMed
.
- A. M. V. Mohan, J. R. Windmiller, R. K. Mishra and J. Wang, Biosens. Bioelectron., 2017, 91, 574–579 Search PubMed
.
- B. Yang, X. Fang and J. Kong, Adv. Funct. Mater., 2020, 30, 2000591 Search PubMed
.
- S. R. Chinnadayyala, K. D. Park and S. Cho, ECS J. Solid State Sci. Technol., 2018, 7, Q3159–Q3171 Search PubMed
.
- H. Teymourian, C. Moonla, F. Tehrani, E. Vargas, R. Aghavali, A. Barfidokht, T. Tangkuaram, P. P. Mercier, E. Dassau and J. Wang, Anal. Chem., 2020, 92, 2291–2300 Search PubMed
.
- P. Bollella, S. Sharma, A. E. G. Cass, F. Tasca and R. Antiochia, Catalysts, 2019, 9, 580 Search PubMed
.
- K. B. Kim, W.-C. Lee, C.-H. Cho, D.-S. Park, S. J. Cho and Y.-B. Shim, Sens. Actuators, B, 2019, 281, 14–21 Search PubMed
.
- M. Adeel, M. M. Rahman, I. Caligiuri, V. Canzonieri, F. Rizzolio and S. Daniele, Biosens. Bioelectron., 2020, 165, 112331 Search PubMed
.
- S. R. Chinnadayyala, I. Park and S. Cho, Mikrochim. Acta, 2018, 185, 250 Search PubMed
.
- E. Eltayib, A. J. Brady, E. Caffarel-Salvador, P. Gonzalez-Vazquez, A. Zaid Alkilani, H. O. McCarthy, J. C. McElnay and R. F. Donnelly, Eur. J. Pharm. Biopharm., 2016, 102, 123–131 Search PubMed
.
- M. Parrilla, M. Cuartero, S. Padrell Sanchez, M. Rajabi, N. Roxhed, F. Niklaus and G. A. Crespo, Anal. Chem., 2019, 91, 1578–1586 Search PubMed
.
- P. Bollella, S. Sharma, A. E. G. Cass and R. Antiochia, Biosens. Bioelectron., 2019, 123, 152–159 Search PubMed
.
- M. Senel, M. Dervisevic and N. H. Voelcker, Mater. Lett., 2019, 243, 50–53 Search PubMed
.
- N. Tejavibulya, D. A. M. Colburn, F. A. Marcogliese, K. A. Yang, V. Guo, S. Chowdhury, M. N. Stojanovic and S. K. Sia, iScience, 2019, 21, 328–340 Search PubMed
.
- A. J. Bandodkar, P. Gutruf, J. Choi, K. Lee, Y. Sekine, J. T. Reeder, W. J. Jeang, A. J. Aranyosi, S. P. Lee, J. B. Model, R. Ghaffari, C.-J. Su, J. P. Leshock, T. Ray, A. Verrillo, K. Thomas, V. Krishnamurthi, S. Han, J. Kim, S. Krishnan, T. Hang and J. A. Rogers, Sci. Adv., 2019, 5, eaav3294 Search PubMed
.
- L. Chen, C. Zhang, J. Xiao, J. You, W. Zhang, Y. Liu, L. Xu, A. Liu, H. Xin and X. Wang, Mater. Sci. Eng., C, 2020, 109, 110402 Search PubMed
.
- R. He, Y. Niu, Z. Li, A. Li, H. Yang, F. Xu and F. Li, Adv. Healthcare Mater., 2020, 9, e1901201 Search PubMed
.
- Z. Wang, H. Li, J. Wang, Z. Chen, G. Chen, D. Wen, A. Chan and Z. Gu, Biomaterials, 2020, 237, 119782 Search PubMed
.
- C. G. Li, H. A. Joung, H. Noh, M. B. Song, M. G. Kim and H. Jung, Lab Chip, 2015, 15, 3286–3292 Search PubMed
.
- W. Yuan, D. Chen, R. Sarabia-Estrada, H. Guerrero-Cázares, D. Li, A. Quiñones-Hinojosa and X. Li, Sci. Adv., 2020, 6, eaaz9664 Search PubMed
.
- D. Han, R. S. Morde, S. Mariani, A. A. La Mattina, E. Vignali, C. Yang, G. Barillaro and H. Lee, Adv. Funct. Mater., 2020, 30, 1909197 Search PubMed
.
|
This journal is © The Royal Society of Chemistry 2020 |
Click here to see how this site uses Cookies. View our privacy policy here.