DOI:
10.1039/D2YA00204C
(Paper)
Energy Adv., 2022,
1, 984-998
Can vehicle-to-grid facilitate the transition to low carbon energy systems?†
Received
3rd August 2022
, Accepted 14th October 2022
First published on 18th October 2022
Abstract
Vehicle-to-grid (V2G) is when electric vehicles (EVs) provide services to the power grid, such as shifting when they charge or discharging to serve peaks loads. At scale, an aggregator can coordinate and optimize the charge and discharge of individual vehicles to function as a synergistic, bulk energy resource and load. Here we generate new insights into V2G's long run value for deep decarbonization, assessing its potential to displace stationary storage and other generators at scale. As a case study, V2G impact is measured via the buildout and operation of a New England-sized power system subject to high EV penetration and tight emissions constraints. We find V2G's effect on system capacity and value to be substantial, with participation from 13.9% of the New England light-duty vehicle fleet displacing 14.7 GW of stationary storage (over $700 million in capital savings). On the whole, total system savings span 2.2% to 20.3% ($183–1326 million) between participation rates of 5% and 80%, respectively. Savings are driven first by displacement of stationary storage, and second by reductions in firm generation capacity and shifts in renewable generation portfolios. When compared to traditional demand response schemes, even at modest participation rates (5–10%), V2G yields over 337% more savings and tenfold the storage displacement. V2G's marginal benefit is greatest under aggressive emission caps (10 gCO2 per kW per h per load), as it decreases the need for excess renewables capacity and costly CCS technologies. Further, the nature of optimal V2G dispatch is shown to change non-monotonically with participation rate. Below 50% participation, V2G power injection is called on at a higher rate to shave evening charging loads, with 21.3–32.8% of charge reinjected to the grid, whereas greater participation rates require significantly less reinjection (<15%) via charge load shifting. Finally, our destination-based system topology quantifies locational V2G contributions and reveals that V2G capabilities are as much as 2.5 times more valued in residential areas than at workplaces alone.
Introduction
Electric vehicle (EV) adoption is accelerating. In the United States (US), EV sales doubled from 2020 to 2021, rising to 4% of passenger car sales.1 In Europe, EVs were 4% of sales in 2019, 20% in 2021, and 30% in December 2021.2 Given such growth, and large investments in EV and battery manufacturing, EVs will plausibly displace internal combustion vehicles (ICEVs) as the world's most common passenger cars over the next 30 years, particularly in the US, Europe, and China.3 Like EVs, renewable electricity sources have grown dramatically in recent years. From 2012 to 2021 in the US, wind grew from ∼3% to >9% of power generation (∼10% to >40% in Kansas), and solar grew from ∼0% to >4% (1% to >20% in California).4,5 Many analyses have identified further dramatic growth of solar and wind as key to reducing GHG emissions and global warming.6–8 As a result, multiple US regional governments have adopted renewable portfolio standards (RPSs) that require utilities to source over 50% of power from solar and wind (also called variable renewable energy, VRE) by 2050.9 However, VRE growth faces physical constraints that EV sales do not, namely intermittency. As VRE exceeds 50% of generation, matching power supply and demand becomes impossible without significant energy storage or over-building of VRE generators (leading to increased curtailment‡) or both.10,11 For example, in the US Northeast, a hypothetical 2050 power grid with a generation mix of 40/40/20 solar/wind/natural gas would require at least 15% of renewable generation to be curtailed, and at least 15% of costs to be spent on energy storage, assuming plausible declines in storage costs and no V2G.12 Furthermore, vehicle charging in futures with high EV fleet penetration, if left unmanaged, is anticipated to add significant strain to the grid during evening hours.13–15 Using California as another example, a 23% BEV fleet share in 2050 increases evening demand peaks by as much as 32% when charging is unsupervised, requiring increased thermal generation and additional generation capacity.12
In light of these challenges, vehicle-to-grid (V2G) has been proposed as a form of flexible load and decentralized energy storage. Within a V2G framework, grid-connected electric vehicles provide services to power grids, for example by shifting when they charge (demand response), providing frequency regulation and operating reserves, or discharging their batteries to the grid when power demand is high. In practice, an aggregator coordinates the charge and discharge decisions of individual vehicles in order to function as a synergistic, bulk energy resource and load. The independent system operator (ISO), which monitors and controls bulk power systems, interfaces with the aggregator at the transmission and distribution levels and makes decisions regarding resource dispatch. For example, an ISO may opt to use EVs to store excess renewables generation during low-price overnight or midday hours and, then, inject the stored energy back into the grid during evening hours when dispatchable generation costs are higher.
EVs are well equipped to provide grid services because average passenger vehicles sit parked for over 90% of their operating lives16 and EV battery packs can provide the same power and ancillary services as stationary grid energy storage.15,17 Moreover, EV batteries have been shown to degrade more via “calendar” aging than via cycling18 and EV charging has already proven to be an exceptionally elastic source of power demand.14 Thus, by harnessing the flexibility of these under-utilized and degrading capital assets, V2G stands to recoup EV battery investments via reduced intermittency costs and peak load shaving and thus facilitate renewables growth as the world progresses toward a “net-zero” future. Accordingly, this study specifically assesses V2G's value in the context of future, low-carbon regional power systems with high EV market penetration.
While prior studies have analyzed V2G's potential for supporting VRE systems19–21 and reducing emissions,22,23 several efforts have been limited in their scope and applicability to future low-carbon systems. Schuller et al. formulate an optimization model to maximize EV's VRE utilization under different power generation and charging infrastructure portfolios.19 The authors demonstrate that coordinated charging can more than double VRE utilization, but that efficacy is limited by the length of the lookahead period. A study by Mehrjerdi and Rakhshani uses stochastic programming to optimize EV charging and discharging in a 33-bus distribution grid such that VRE intermittency is damped and battery cycling is reduced.20 A third study assumes the risk-management perspective of ISOs, considering both uncertainties of VRE output, load and parking patterns, and transmission line reliability in optimizing V2G dispatch.21 Limitations of all three works, however, are their relatively small fleet and system sizes. Thus, results do not necessarily extend to behaviors in the broader power system, for which large EV fleets can, in theory, influence system-wide generation mixes and long-run VRE investment decisions. As for emissions reductions, Sioshansi and Denholm use a power system model to demonstrate that V2G (including spinning reserves) from plug-in hybrid electric vehicles (PHEVs) can reduce CO2 and non-ozone NOx charging emissions up to ∼31% and 77%, respectively, for the ERCOT grid.22 The analysis, though, is limited to the 2005 ERCOT grid with low PHEV penetration (15%), and so results do not assess future V2G potential. A more recent study by Zhao and Baker assesses V2G's environmental impacts in a 2050 United Kingdom system using consequential LCA methodology.23 While the approach neglects time-dependent dispatch and other system dynamics, results indicate that V2G, when employed under the right conditions, can indeed contribute to mitigating electricity generation's environmental footprint in high renewables scenarios. Among studies that do assess V2G dispatch and outcomes on a regional or country-wide basis, few fully capture the characteristics of future fleets, power systems, and their interdependence, nor does analysis segment and quantify the specific sources and mechanisms of V2G value. Table 1 provides an overview of select regional V2G studies and their select features of note.
Table 1 Attributes of select regional V2G studies
Study |
Study comprises… |
Results capture… |
EV fleet size |
High VRE share |
Varied grid mix |
Service/EV bat. degrad. costs |
EV-storage interactions |
Infrastr./service sensitivities |
Cap. expan. implications |
Spatial V2G differences |
System costs dynamics/segments |
24
|
4 MM |
✓ |
✓ |
✓ |
|
✓ |
|
|
✓ |
25
|
1 MM |
|
|
|
|
✓ |
|
|
✓ |
26
|
3.8 K |
|
|
✓ |
|
✓ |
|
|
✓ |
27
|
8 MM |
✓ |
|
|
|
|
|
✓ |
|
28
|
2.6 MM |
✓ |
|
|
|
|
|
|
|
29
|
28 MM |
✓ |
✓ |
|
✓ |
✓ |
✓ |
|
|
30
|
14.7 MM |
✓ |
|
|
✓ |
✓ |
|
✓ |
|
31
|
30 K |
|
|
|
|
✓ |
|
|
|
32
|
50 K |
|
|
✓ |
|
|
|
|
|
33
|
9 MM |
✓ |
✓ |
|
|
✓ |
|
|
|
34
|
3.6 K |
|
|
|
|
|
|
|
|
35
|
5 K |
|
|
|
|
✓ |
|
|
|
36
|
45 K |
✓ |
✓ |
|
|
✓ |
|
|
|
37
|
500 |
|
|
✓ |
|
✓ |
|
✓ |
|
This study |
8.6 MM |
✓ |
✓ |
✓ |
✓ |
✓ |
✓ |
✓ |
✓ |
For instance, several of the listed works do not sufficiently survey future VRE scenarios and constraints, namely not evaluating V2G value across different generation mixes/emissions constraints or neglecting V2G operating costs at scale (i.e. service or degradation charges). Wang and Craig provide insightful analysis of EV-grid price interactions in a 2030 California system, using a co-optimization of the EV fleet and the power system to how V2G revenues change with scale and renewables deployment.24 Their results indicate that while more valuable than smart charging alone, future V2G revenues can quickly decline as a function of fleet-wide participation and declining electricity prices. One limitation of the study is that it does not consider V2G's interactions (operational or system planning) with non-hydro, stationary storage technologies like Li-ion batteries, which are speculated to play a key role in the energy transition.38–40 Thus, it is possible that stationary storage could further influence market outcomes via energy arbitrage or that V2G has unaccounted value in deferring storage or firm capacity investments. Along these lines, a study by Tarroja et al. provides a head-to-head comparison of V2G and stationary storage performance.30 Investigating operational outcomes as opposed to cost implications, the authors found that V2G outperforms stationary storage in VRE utilization but underperforms when it comes to balancing the power plant fleet. Their analysis goes onto to show that V2G's benefits heavily depend on sufficient infrastructure being available at workplaces so that EVs can charge when solar generation peaks.
In analyzing system outcomes, several studies quantify sensitivities to V2G infrastructure but few capture the locational value of V2G or account for network topologies. In addition to the work of Tarroja et al., Staudt et al. employ a transmission system model of Germany to simulate outcomes of a local V2G flexibility market heuristic for alleviating transmission congestion.27 Since flexibility bids are made locally, the optimal flexibility, and thus revenues, of V2G participation differs spatially across the grid. While the heuristic is shown to aid in grid stabilization, results do not elaborate on implications for deferral of additional transmission and/or generation capacity. Likewise, relatively little work has been done in assessing V2G's long run value in the context of system planning (i.e. capacity expansion modeling). In a study of utility-controlled charging (UCC), the authors combine empirical vehicle adoption and charging models with an electricity system model to simulate power system evolution in Canada through 2050.41 Their results show that UCC reduces future generation capacity by 1.5% and 7.5% and lowers electricity prices by 0.6% and 0.7% for British Columbia and Alberta, respectively. However, V2G services are not considered. Forrest et al. show that V2G and smart charging can potentially eliminate the need for stationary storage, but do not consider other generation capacity.29 Finally, while most works quantify V2G's monetary benefits in some form, few provide thorough breakdowns of system savings and/or demonstrate how they change with system context. For instance, Brinkel et al. decouple V2G's operational and investment savings, and Huda et al. segments V2G costs and revenues across different tariff structure and EV travel cases.25,26 Neither study, though, quantifies V2G's marginal value of adoption, which can be defined in terms of participation or infrastructure (among other variable) and in turn generate insights into when and where V2G is most valuable.
Building upon the methods and findings of prior works, this study analyzes V2G in the context of: (1) large EV fleets with variable participation, (2) different low carbon scenarios, and (3) varying service capabilities. Likewise, the results and analysis provide new insights into (4) the dynamics of optimal V2G dispatch behavior and associated emissions outcomes at scale, (5) the mechanisms by which large-scale V2G can augment VRE growth, and (6) where, and to what extent, V2G infrastructure investments are most valuable. As a case study, we quantify V2G value via the greenfield buildout and operation of a New England-sized power system subject to high EV penetration and tight emissions constraints.
This study's contribution is unique in its assessment of V2G's potential for offsetting stationary storage and renewables expansion requirements in achieving low carbon futures. We do not ask how V2G interacts with a pre-defined power system, but how significant V2G activity may define that system, by augmenting capacity expansion requirements and associated costs. More specifically, our analysis segments and quantifies the “why” and “how” of V2G value and its margin and employs a destination-based topology to uncover the locational value of V2G. The ultimate goal of this work is to provide actionable information to help system operators and society to maximize benefits and minimize damages from EV growth.
Modeling approach
We model V2G impact on grid investments and operations from the perspective of an independent system operator (ISO) using a modified version of GenX, an open-source capacity expansion model (CEM).42 The CEM makes system-wide investment and operational decisions for least-cost optimization of meeting forecasted electricity demand over the course of one year at an hourly resolution. While an ISO has the ability to commit thermal resources at the generator level, its knowledge and control of V2G resources are typically limited to aggregate-level resolutions provided by a vehicle aggregator. Thus, the theoretical ISO makes decisions according to knowledge of large vehicle clusters (e.g. available power and energy capacities), and an aggregator optimizes the utilization of individual vehicles. Though this approach does not fully capture real-time market conditions, the CEM's high temporal resolution is key to studying the dynamic behavior of decentralized V2G resources and their sensitivities to varying market conditions.
The system load spatially segments total load into residential, commercial, and “other” demand centers (Fig. 1) to which power flows from a single generation node. Synthetic load profiles (detailed further in the ESI†) are derived from ISO New England (ISONE) load reports, EIA data, and NREL's ResStock and ComStock datasets corresponding to the year 2018 and unsupervised EV charging profiles are constructed using empirical data from Idaho National Laboratory's “The EV Project”.
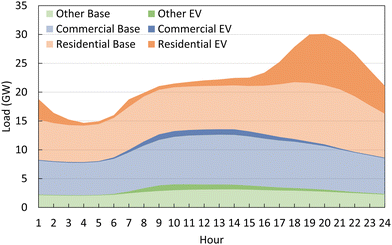 |
| Fig. 1 Average daily load profiles in baseline case with unsupervised charging and no V2G participation. Both residential base load and vehicle charging exhibit peaks during evening hours, whereas commercial and other load centers experience more subtle base and EV charging peaks around midday. | |
The constraints on accessible vehicle energy and power capacity are informed by an EV travel, storage, and charging model that we developed using the data from the 2017 National Household Travel Survey and EV manufacturer data. Unlike many stationary storage technologies, we constrain minimum charge to both satisfy future travel demand and adhere to minimum charge heuristics for good EV battery health.43 Temporally, we do not model real-time capacity degradation or dynamics for either stationary and EV storage within the CEM, as it introduces significant computational complexity and long-run aggregate degradation is less pronounced due to stock retirements and replenishment. In fact, among several works44–48 that address V2G-induced battery degradation, Wang et al. shows degradation to be minimal when vehicles are selectively utilized for peak shaving and regulation service on “high demand” days, rather than used around the clock.46 Further, Hoke et al. predict that managed EV charging can actually prolong EV battery lifetime relative to uncontrolled charging.48 However, we still expect increased cycling to shorten EV battery lifetime and so we synthesize publicly available battery degradation data and price projections to assign a variable degradation system cost to EV utilization and to quantify annual degradation a posteriori.
V2G module
Because we are most interested in generation technology tradeoffs and dispatch trends, our base case is effectively a single node network analysis, in which we assume that adequate transmission and distribution capacity is installed and do not model line losses. Vehicles can service all load segments equally. In this study we limit V2G participation to homes and workplaces, as travel surveys show the significant majority of vehicles (70–80% being the minimum) to be parked at either location when not driving.49,50 The following variables and constraints describe cases in which an EV aggregate contributes toward general load balancing as well as provides regulation and reserve services to the grid.
Indices and sets
Notation |
Description |
t ∈ T |
t is a time step and T is the set of time steps over which grid operations are modeled |
T
start ∈ T |
1 |
T
interior ∈ T |
T
interior is the set of interior series time steps |
EV |
The single EV aggregate |
Decision variables
Notation |
Description |
Γ
EV,t ∈ + |
Stored energy level of the EV aggregate at time step t |
Γ
grid
t
∈ + |
Stored energy level of grid-connected vehicles at time step t, derived from ΓEV,t and fraction of grid-connected vehicles available for V2G |
Π
EV,t ∈ + |
Energy withdrawn from grid by EV aggregate at time step t |
Θ
EV,t ∈ + |
Energy injected into grid by EV aggregate at time step t |
f
chargeEV,t ∈ + |
EV aggregate contribution to frequency regulation for up and down reserves from charging at time step t |
f
dischargeEV,t ∈ + |
EV aggregate contribution to frequency regulation for up and down reserves from discharging at time step t |
r
chargeEV,t ∈ + |
EV aggregate contribution to upward spinning reserves from charging at time step t |
r
dischargeEV,t ∈ + |
EV aggregate contribution to upward spinning reserves from discharging at time step t |
Parameters
Notation |
Description |
D
wheel,t
|
Energy consumed by EV aggregate for driving purposes at time step t |
τ
period
|
Number of time steps being modeled |
Γ
min
t
|
Minimum energy level constraint for EV aggregate at time step t |
Γ
max
|
Maximum energy level constraint for EV aggregate |
Δ
powerEV,t
|
Total grid-connected power capacity of EV aggregate at time step t |
Δ
energyEV,t
|
Total grid-connected energy capacity of EV aggregate at time step t |
Δ
powerserv_limit,t
|
Grid-connected power capacity available for injection and ancillary services when locational service constraints are active. |
η
chargeEV
|
Single-trip efficiency of EV charging |
η
dischargeEV
|
Single-trip efficiency of EV discharging |
η
lossEV
|
Self-discharge rate per time step per unit of installed capacity, 0 |
υ
regEV
|
Maximum fraction of connected capacity that EV aggregate can contribute to frequency regulation reserve requirements |
υ
rsvEV
|
Maximum fraction of connected capacity that EV aggregate can contribute to upward spinning reserve requirements |
First, because the EV aggregate represents a large population of decentralized vehicles and vehicle clusters, we permit it to simultaneously charge and discharge. For a given time step, t, the aggregate EV state-of-charge, ΓEV,t, must remain between a prescribed minimum and the physical maximum. Similarly, the net change in aggregate state of charge must not exceed the uncharged/available grid-connected energy capacity at the start of t. | Γmint ≤ ΓEV,t ≤ Γmax, t ∈ T | (1) |
| ΠEV,t − ΘEV,t ≤ ΔenergyEV,t − Γgridt, t ∈ T | (2) |
The inter-temporal constraints (3) and (4) relate EV aggregate state-of-charge at the beginning and end of time step t to charge/discharge decisions and driving and self-discharge processes. Modeling operations over a single contiguous period, the constraint links the storage inventories of the first and last time steps. | 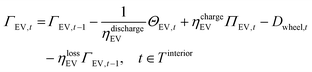 | (3) |
| 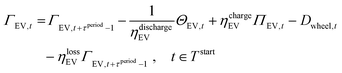 | (4) |
The EV aggregate can contribute to regulation and reserves while both charging and discharging. Here, pairs of proxy variables sum to the total contributions of the EV aggregate to each ancillary service. We impose no limit on the fraction of EV power capacity that can go toward reserves. | fEV,t = fchargeEV,t + fdischargeEV,t, t ∈ T | (5) |
| rEV,t = rchargeEV,t + rdischargeEV,t, t ∈ T | (6) |
The sum of charge rate, discharge rate, and contribution to ancillary services is constrained by the total connected power capacity at time t. | ΠEV,t + fchargeEV,t ≤ ΔpowerEV,t, t ∈ T | (7) |
| ΘEV,t + fdischargeEV,t + rdischargeEV,t ≤ ΔpowerEV,t, t ∈ T | (8) |
| ΠEV,t + fchargeEV,t + ΘEV,t + fdischargeEV,t + rdischargeEV,t ≤ ΔpowerEV,t, t ∈ T | (9) |
As well as connected energy capacity and availability: | ΠEV,t + fchargeEV,t ≤ ΔenergyEV,t − Γgridt, t ∈ T | (10) |
| ΘEV,t + fdischargeEV,t + rdischargeEV,t ≤ Γgridt, t ∈ T | (11) |
Because reduced charging rates decrease the net demand on the system, the EVs contribution to upward regulation and reserves increases. Similarly, reduced discharge rates lower net supply and contributes to downward regulation. Thus, the sum of these rates and ancillary contributions must be greater than zero. | ΠEV,t − fchargeEV,t−rchargeEV,t ≥ 0, t ∈ T | (12) |
| ΘEV,t − fdischargeEV,t ≥ 0, t ∈ T | (13) |
When modeling cases with no reserves or vehicle demand response (VDR), the state of charge (SOC) balances (1)–(4) are unchanged and constraints (7)–(9) and (12), (13) reduce to: | ΠEV,t ≤ ΔpowerEV,t, t ∈ T | (14) |
We also, in select cases, constrain V2G capabilities (i.e. VDR and the ability to inject power and to provide ancillary services, or only VDR) at homes and workplaces. This enables us to enumerate the locational value of V2G across locations. In these cases, when eliminating power injection and ancillary services, for instance at workplaces, we introduce an additional constraint that reflects the reduced V2G service capability of the aggregate fleet (while still allowing for unrestricted demand response for participating vehicles across all locations): | ΘEV,t + fEV,t + rEV,t ≤ Δpowerserv_limit,t, t ∈ T | (16) |
We also formulate and test a case to evaluate Vehicle-to-Building (V2B) technology in the ESI,† in which vehicles only serve local loads and not the grid. Overall, these destination-based constraint formulations and topologies (Fig. 2) enables us to independently quantify the system value and storage displacement specifically derived from (1) location-specific charging infrastructure investments, (2) overall and destination-based V2G participation, (3) and specific services offered to the grid.
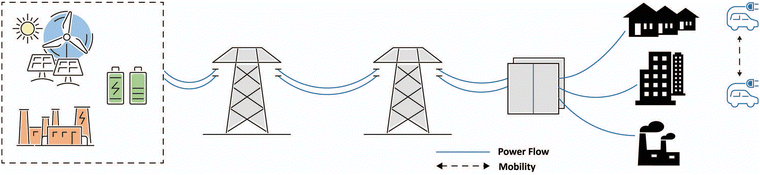 |
| Fig. 2 V2G-power system topology. All generation capacity and stationary storage exists at a single, zero-demand node, from which power is transmitted to demand centers (residential, commercial, and other) with no line losses or constraints. EVs participate in the V2G market from residential and commercial locations, and collectively serve all three loads. The network can also be constrained such that vehicles only serve local loads (vehicle-to-building). In all cases EV power is constrained from flowing to stationary storage. (Figure credit: Heather Hodgkins). | |
Constraint parameterization: travel, storage, and charging model
A fleet travel and charging model is used to parametrize the EV aggregate's power and energy constraints. Based on raw vehicle trip data and statistics from the 2017 National Household Travel Survey (NHTS), individual vehicle travel and location profiles are constructed via trip chaining then combined to determine a representative vehicle mile traveled (VMT) profile and the share of the LDV fleet at different locations (classified as either driving, home, work, or other) throughout both weekdays and weekends. The daily profiles are linked to construct continuous, yearlong parameter profiles. Further details and results of this step are provided in the ESI.†
The number of EVs participating in the V2G program, NEVs, is a function of fleet size, penetration rate, and participation rate. Independent on charging/discharging decision variables, the EV aggregate discharges energy at each time step to satisfy travel demand (“wheel demand”), Dwheel,t, which is a function of aggregate VMT for the hour, VMTt, and vehicle fuel economy, FEV.
The maximum state of charge for the EV aggregate,
Γmax, is fixed in time, depending only on the number participating EVs and the average battery capacity of a single EV,
ΔenergycapSingleEV. The minimum state of charge,
Γmint, varies in time and is a function of both the remaining daily wheel demand,
δwheel,t, and a fractional contingency factor,
βmin, which reflects heuristics intended to avoid rapid degradation from too deeply discharging the battery.
δwheel,t is at its maximum at the start of the travel day, which we define as 4 AM to maintain consistency with NHTS data.
Γmax = NEVs × ΔenergycapSingleEV |
Γmint = NEVs× [(βmin × ΔenergycapSingleEV) +δwheel,t] |
In addition to the
Γmint constraint for charge management throughout the day, we require that the average vehicle have a minimum 70-mile range at the start of the travel day (4 AM), over the course of which the average vehicle drives approximately 30 miles. This requirement is both described more completely and derived in the ESI,
† in which we also describe why this value remains highly uncertain.
As for charger access in the base case, it is assumed that V2G participation only takes place at home or work, so grid-connected energy capacity, ΔenergyEV,t, is a function of fractional vehicle locations, xhome,t and xwork,t and their respective charger accessibilities, αhome and αwork (the fraction of home or work locations with charger access). Available power capacity is similarly computed, with effective home and work power capacities as a function of charger shares, xL1, and xL2, at each location.
ΔenergyEV,t = NEVs × (αhomexhome,t + αworkxwork,t) × ΔenergycapSingleEV |
ΔpowerEV,t = NEVs × [(αhomexhome,t × Δpowerhome) × (αworkxwork,t × Δpowerwork)] |
Δpowerhome = [ΔpowerL1,ΔpowerL2]·[xL1,home,xL2,home] |
Δpowerwork = [ΔpowerL1,ΔpowerL2]·[xL1,work,xL2,work] |
when constraint
(16) is active,
Δpowerserv_limit,t is simply scaled accordingly from
ΔpowerEV,t by looking at the fraction of overall power capacity being contributed at each location. Constraint parameters are computed on a minute basis and converted to average hourly values, some of which are shown in the ESI.
†
Model scenarios
We model the greenfield buildout and operation of a low-carbon power system with high EV penetration in the year 2050. The hourly load profiles, VRE availability, and vehicle fleet size are characteristic of future projections for the ISONE region, and are generated using the Sustainable Energy System Analysis Modelling Environment (SESAME).51 We specifically use SESAME's power grid and LDV fleet modeling capabilities to project LDV stock growth, base load growth, and uncontrolled EV charging load as a function of charger access. This analysis does not consider the loads and V2G activity of commercial EV fleets.
To understand the potential for V2G to displace firm dispatch and storage in low-carbon futures, we make available combined cycle natural gas turbines (with and without carbon capture and storage), onshore wind and solar installations, and utility-scale storage for the GenX CEM to build and dispatch. The objective function of the CEM for each scenario includes both the fixed capital and operating and variable costs for these technologies to serve the prescribed load. Fig. 3 provides a conceptual framework of how SESAME, GenX, the V2G module, and constraint models interact with one another and exogenous inputs.
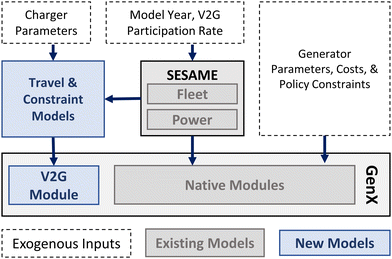 |
| Fig. 3 Simplified input flows among SESAME, GenX, and V2G constraint models. SESAME computes the electricity demand scaling of the base load profiles and EV fleet size and charger access projections characteristic of New England in 2050. Base loads are directly input to GenX, while fleet details are inputs to the travel and constraint models, which also use travel charger parameters and travel data to compute power and energy limits for the V2G module. | |
We assume that EVs comprise 60% of the LDV vehicle stock (via BNEF projections) and impose CO2 emissions caps informed by long run decarbonization goals of the New England states (detailed in ESI†). The base case asserts 100% Level 2 charger share, a carbon cap of 50 g CO2 kW−1 h−1 load, and that 2050 driver behaviors will be comparable to those of ICEG passengers today (i.e. similar travel demand and diminished “range anxiety” relative to today). All vehicles participating in V2G are able to provide peak shaving, VDR, and frequency regulation and operating reserve services. Key scenario parameters are listed in Table 2, with additional parameters and sources detailed in both the methods section and ESI.†
Table 2 Key base case parameters
LDV stock and load characteristics |
|
LDV stock (million) |
14.4 |
EV share of LDV stock (%) |
60 |
Peak base load without V2G (GW) |
41.4 |
EV share of electricity consumption (%) |
15.4 |
V2G parameters |
|
V2G VOM (2019 $ per MW per h) |
9.5 |
Level 1 charge capacity (kW) |
1.9 |
Level 2 charge capacity (kW) |
10 |
Homes with charger access (%) |
90 |
Workplaces with charger access (%) |
20 |
Available V2G services |
Peak shaving, frequency regulation, operating reserves |
Emissions constraints |
|
2050 goal – base (gCO2 per kW per h per load) |
50 |
Low carbon (gCO2 per kW per h per load) |
10 |
Our study scenarios are formulated to uncover the opportunity space of V2G and its primary value to drivers. Using a two-pronged approach, we first probe generation capacity and dispatch dynamics by enabling unconstrained V2G capabilities, allowing vehicles to provide power injection and ancillary services to the grid from any location (base case). Then, in latter scenarios, we impose constraints on charger levels and locational V2G capabilities that elucidate the coupling and value of V2G infrastructure and location.
Results
Drivers of V2G value in low-carbon futures
We find that V2G significantly lowers total system costs across all emissions scenarios. Our first analysis assumes no transmission or distribution constraints, and varies power grid emissions limit and share of EVs participating in V2G (participation rate). In the base case, 40% and 80% participation rates produce relative savings of 15% and 21%, respectively (Fig. 4a). Savings are driven first by displacement of stationary storage, and second by reductions in firm generation capacity and shifts in renewable generation portfolios. Fig. 4b shows installed generator and storage capacities under different emissions constraints and V2G participation rates. With just 23.1% EV participation,§ corresponding to 13.9% of the LDV fleet, 14.7 GW of 6 h stationary storage is completely displaced. The 14.7 GW of power capacity corresponds to 1438% of installed U.S. large-scale storage capacity in 2019¶ (∼490× New England capacity) and alone yields fixed cost savings of $729 million.52
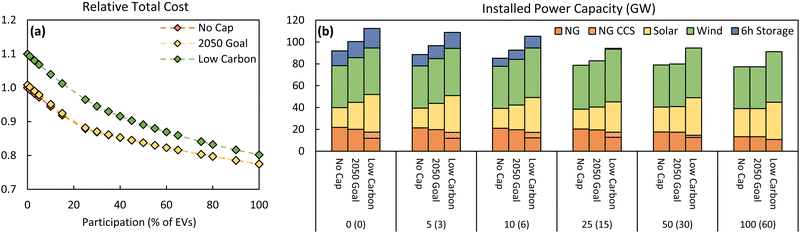 |
| Fig. 4 System values relative to no emissions cap, zero-V2G participation baseline (left) and installed generator and storage capacities (right) under varying emissions constraints and V2G participation rates. The 2050 Goal and Low Carbon emissions constraints correspond to caps of 50 gCO2 per kW per h per load and 10 gCO2 per kW per h per load, respectively. | |
In addition to displacing storage, V2G increases VRE generation shares and decreases dependence on natural gas (NG) power plants across all emissions scenarios (Fig. S4, ESI†). As V2G increases, so does the share of solar within the VRE portfolio. This is driven by the fact that as more EV storage is added, the lower availability of solar relative to wind becomes less important and the lower cost of solar more important. These dynamics of wind and solar capacity, however, are expected to differ across the US. In California and the US Southwest, for example, solar is both cheaper and has a higher annual capacity factor than wind. That said, the overall observed trends of reduced stationary storage and increased VRE penetration with V2G participation broadly extend to other regions of the US, which have similar EV sales projections and per capita vehicle counts and energy demand. The overall implications of results, however, are highly sensitive to anticipated EV market penetration in the US. The US today has one of the largest light duty vehicle fleets in the world and is anticipated to undergo significant vehicle electrification (∼60% of light duty fleet) by 2050. This is quite different from countries like India, which have far fewer vehicles but still relatively high energy demands and slower EV adoption (∼2% of sales in 2021, compared to ∼6% in the US53,54). Thus, higher shares of VRE capacity, stationary storage, and dispatchable resources will be required to achieve low-carbon emissions goals, even with V2G.
Fig. 5 demonstrates V2G dispatch behavior for a representative week with 50% V2G participation. The aggregator-controlled V2G fleet charges during low demand, peak VRE hours and discharges back to the grid during evening hours when baseload and non-participating EV charging both peak. The V2G demand shift reduces evening loads and associated natural gas generation. Similar results are presented by Forrest et al., who show V2G load shifting and peak shaving service to completely displace stationary storage in pursuit of an 80% RPS. Different from our model, which sizes storage and VRE investments simultaneously for least cost, the authors parametrically size storage with respect to a fixed VRE portfolio. A benefit of this approach is that it isolates the direct tradeoffs between EV charging strategies and storage requirements. The relative strength of our framework, however, is that we capture the full spectrum of system flexibility and savings conferred by V2G in a planning context and under different levels of participation.
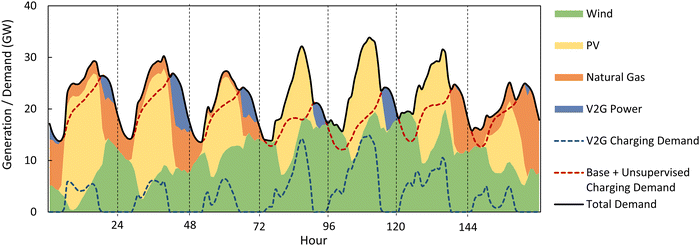 |
| Fig. 5 Generation and demand profiles in a representative week with 50% V2G participation. Hourly base load and EV charging demand profiles are indicated by dashed lines and sum to equal total generation. | |
We also demonstrate that the magnitude and nature of V2G savings changes with system context. For instance, the marginal value of V2G is highest under aggressive emissions caps (Fig. 6). This is indicative of higher carbon prices brought on by high cost carbon capture and storage (CCS) technology and excessive VRE capacity needed to satisfy tight carbon constraints. For all emissions scenarios, marginal V2G value is highest at low participation rates, when stationary storage is still being displaced and vehicle utilization is maximized. While this result is consistent with Wang and Craig's finding of value (in their case, revenues) declining with participation, their analysis does not consider the value of deferred investment despite electricity prices declining with decarbonization. Our work goes a step further in quantifying investment deferral savings and by showing its value to increase with deeper decarbonization (i.e. higher marginal value in lower carbon scenarios).
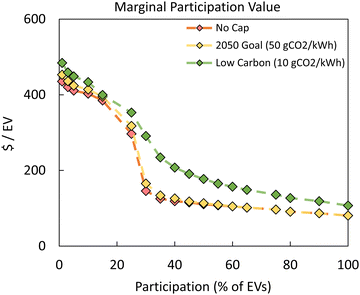 |
| Fig. 6 Marginal service value of V2G as a function of participation under varying carbon constraints. Marginal service value is defined as the rate of increase in overall savings per additional participating EV. At low participation rates, marginal service value remains high as incremental EV participation displaces fixed cost storage investments. If all storage has been displaced, marginal service value is significantly lower because the incremental benefits of remaining generator substitutions and associated re-optimizations are comparatively smaller. Across both domains, marginal value simultaneously decreases due to diminishing vehicle utilization, as uncontrolled EV loads are smaller and V2G power injection is required less frequently (Fig. S7, ESI†). | |
To better understand V2G impact on costs, Fig. 7 segments savings by type and technology. For the base case (middle panel, 2050 Goal), in addition to stationary storage substitution, improved VRE utilization reduces not only VRE capacity but also natural gas capacity. At 50% V2G participation, 13.2% and 4.8% GW of thermal and VRE capacity are displaced, respectively. The no emissions cap (left) and low carbon (right) scenarios also show a decrease in thermal capacity and resulting savings. In contrast to the 2050 Goal case, they show an increase in VRE capacity, but the cost of this increase (solid green) is more than offset by the thermal cost savings. As more EV resources become available, additional VRE capacity can be efficiently utilized, meaning that the thermal generators and CCS technologies used to support peak loads and lower carbon output at low V2G are no longer necessary.
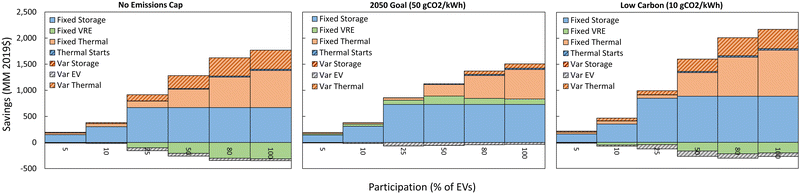 |
| Fig. 7 Breakdown of contributions to overall system cost change (relative to zero V2G participation base) at different participation rates. Positive values indicate a savings (e.g. reduction in storage capacity), and negative values indicate a new expense (e.g. increased VRE capacity). Fixed costs include both overnight investment and annual maintenance costs. Variable (Var) costs include fuel for thermal resources. | |
The savings detailed above majorly outweigh the EV battery degradation costs (‘Var EV’) from V2G, which are only ∼5% to ∼8% of V2G's net value. In other words, the pool of cost savings for potential V2G stakeholders, such as utilities, is more than large enough to both incentivize EV owners to participate and to compensate aggregators. As a sensitivity, we demonstrate that increasing V2G's degradation cost (via increased battery cost and/or increased degradation per cycle), and thus the utilization fee required to compensate EV owners for that cost, by as much as five-fold from our default value has little impact on the rate of storage displacement and V2G use (Fig. S5, ESI†). Small degradation costs are explained partly by moderate increases in cycling from V2G. Across all participation rates and load centers, the V2G fleet provides power back to the grid for just ∼7% to ∼21% of hours. Table S5 (ESI†) shows that 25% V2G participation increases cycling by ∼39%, while 75% participation increases cycling by ∼14%, relative to the no V2G case (0% participation). To put this increased cycling in terms of increased degradation, consider a typical EV after 5 years of use (∼70 thousand miles): 25% more cycling means that battery capacity and EV range after 5 years are ∼94% of their day 1 values, vs. ∼95% in the no V2G case.
Analysis of optimal V2G dispatch
Fig. 8 presents the day-averaged net service profiles of participating EV aggregate and the overall, combined EV fleet as it compares to the uncontrolled charging baseline. Results indicate that the vehicle aggregate strictly charges during midday hours, which coincides with solar generation profiles, and injects power back into the grid when base and uncontrolled EV loads peak in the evening. Results also reveal that optimal V2G power injection is a nonlinear function of participation. At low V2G participation rates, substantial uncontrolled charging peaks occupy the evenings (baseline uncontrolled EV demand is denoted by the dashed line) and are partially offset by V2G power injection. As participation increases, more of the EV demand is controlled, allowing it to be dispersed to periods with smaller baseloads and higher VRE availability, which in turn reduces both peak natural gas generation and V2G peak shaving service events. Note, however, that evening charging is not entirely eliminated due to daytime charging constraints (gridded capacity peaks in the evenings) and variation among daily load profiles. Nonetheless, this load shifting is a critical aspect of V2G servicing and its realized value, as uncontrolled EV demand, on average, constitutes 32% of peak evening loads. This behavior is also driven, in part, by the nature of EV travel demand, which continually consumes stored energy and requires minimum levels of charge for future trips. As is also found by Tarroja et al., EVs’ peak shaving capacity is thus limited relative to stationary storage.30 In Fig. S6 (ESI†), we capture this difference in flexibility by comparing the EV aggregate's and stationary storage's average state-of-charge throughout the day.
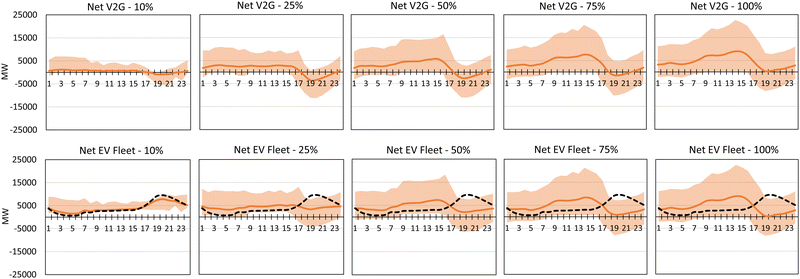 |
| Fig. 8 Daily averaged net service profiles of participating EV aggregate (top row) and overall, combined EV fleet (bottom row) at different participation rates. Positive values indicate net charging and negative values indicate net injection to the grid. The shaded region is the middle 90th percentile of service values and the dashed line is net load of uncontrolled EV charging in the base case. | |
We also note that optimal fleet utilization is determined with respect to sufficient transmission and distribution corridors for unconstrained V2G charging and injection during all hours. While the assumption is reasonable for a system like ISONE, which can trade with other systems and is historically stable, it does not reflect the transmission challenges within systems like ERCOT. The majority of Texas’ wind and solar generators are installed in the northwest part of the state, away from large load centers in the east, and there is shortfall of transmission capacity in between. As a result, VRE generation is often extremely curtailed when the system faces peak demand strains (during hot summer months) and thus poses an obstacle to achieving full V2G benefits, as well as accurately modeling them.
Charging level and participation dynamics
We also examine the sensitivities of V2G value as a function of charging levels, charging access, V2G service capabilities. To start, Fig. 9 explores the relative and synergistic effects of charger level and participation rate, showing how savings, marginal value, and storage displacement vary with each. In the base scenario, high V2G participation rates realize net savings and stationary storage displacements exceeding 20% and 90%, respectively, across all charging levels. However, the stark differences in marginal service value across ∼0–40% participation tells an interesting story.
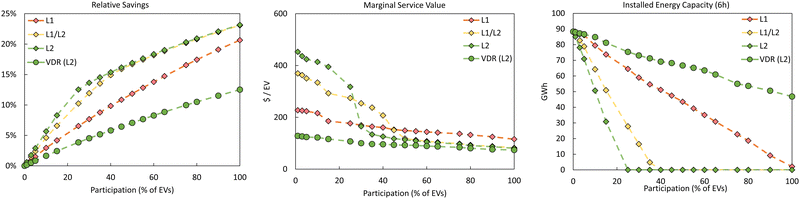 |
| Fig. 9 Net savings (left), marginal vehicle service value (center), and installed stationary storage energy capacity (right) as a function of EV fleet participation rate and charging infrastructure. L1, L1/L2, and L2 indicate 100 : 0, 50 : 50, and 0 : 100 Level 1/Level 2 charger shares, respectively. VDR (L2) indicates demand response only with all Level 2 charging. | |
It indicates that at low participation rates, V2G's utility is most often constrained by available power capacity rather than available energy capacity (also see Fig. S7, ESI†). Likewise, increased power capacity, either from increased participation or charger level, improves both aggregate discharge and demand response flexibility and thus service utility. As more power capacity comes online, there is a point at which the marginal value of a higher charger level diminishes,|| which is observed in the parity of outcomes for 50
:
50 Level 1/Level 2 charging and 100% Level 2 charging at high participation. Instead, aggregate V2G utility is predominantly constrained by grid-connected energy capacity and state-of-charge requirements. Therefore, it is prudent for initial V2G efforts to balance investments in charger capabilities with overall accessibility and enrollment incentives. In scenarios where only VDR infrastructure is available (no bi-directional capabilities), value is significantly diminished, with both overall savings and storage displacement less than half of what is achieved in the V2G base case. VDR cannot contribute to VRE generation shifting nor ancillary services, and so even at high participation rates stationary storage and natural gas dispatch are necessary to balance peak demand periods, yielding investment savings that lag relative to V2G.
The locational value of V2G
Building upon the results in Fig. 9, we investigate how the value of V2G varies across locations and levels of infrastructure access. Results from our travel and charging models in Fig. 10 demonstrate that location-specific power capacities vary widely throughout the day as a function of aggregate travel patterns and are sensitive to both charging infrastructure (level) and V2G participation rate. These results are generalized to the US at large and assume sufficient system planning. Thus, effective V2G capacity is also expected to vary across US regions and countries depending on upgrades to distribution grids for supporting EV loads as well as policy to increase charger access. Likewise, not all V2G infrastructure upgrades will be of equal benefit to the grid. For workplace capacity in particular, even with level two charging and high participation, available capacity remains small relative to residential areas because it is bounded by low vehicle number and charger accessibility (installations).
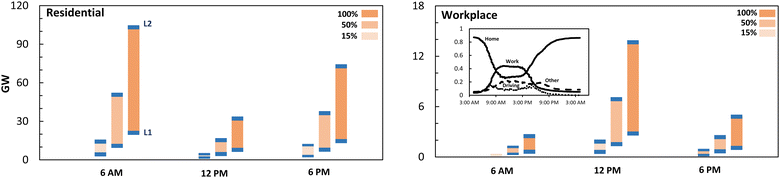 |
| Fig. 10 Contribution of hourly V2G power capacity as a function of location, time, participation level, and charger mix for ISONE. Fleet participation is a fraction of EV. Scenarios assumes 14.3 MM LDVs in 2050 with 60% EV fleet penetration and 90% and 20% home and workplace charger availability, respectively. Inset: The locational distribution of the LDV fleet during a typical weekday. | |
To examine this further, we pose scenarios in which all V2G injection capabilities (power injection and frequency regulation and operating reserve services) are restricted to particular locations. In this case we look at “injection at home only”, “injection at work only”, and “VDR only” and other sensitivities to decouple the value that each location provides. Unidirectional VDR is still available and unrestricted across all charging locations for participating vehicles.
In Fig. 11, we examine participating vehicle utilization patterns to understand optimal dispatch under each scenario. When V2G injection is limited to residential areas (home only), the aforementioned non-monotonic shift in power injection is again observed, with power injection declining with increased participation (also see Table S5, ESI†). Table S5 (ESI†) also shows home only power injection to nearly mirror that of the base cases, suggesting that power injection value is derived primarily from shaving evening load peaks, when residential areas contribute over ∼94% of grid-connected power capacity after 6 PM. Thus, it is unsurprising that Fig. 12 shows virtually all value and storage displacement to be achieved compared to the base case. Limiting V2G injection to workplaces, on the other hand, offers substantially lower value to the grid – from 29% to 61% less. Workplace EV power capacity tends to peak alongside midday VRE solar generation and troughs in the evenings when vehicles depart for home but peak shaving is most needed, and so there is negligible increase in storage displacement relative to VDR alone. Still, value is derived at higher participation (up to 15% relative system savings) from peak shaving events and ancillary services that defer dispatchable capacity investment that would otherwise be needed.
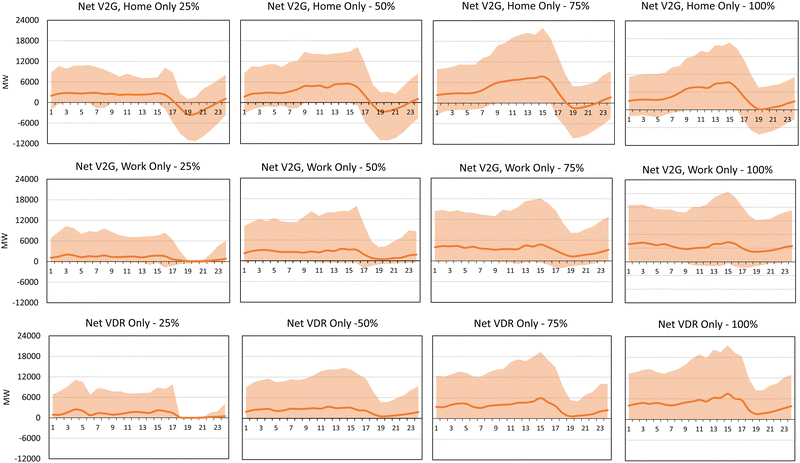 |
| Fig. 11 Daily averaged net service profiles of the participating V2G EV aggregate when constrained to V2G injection at “home only” (top row), V2G injection at “work only” (middle row), as well as no V2G injection/“VDR-only” (bottom row). Positive values indicate net charging and negative values indicate net injection to the grid. The shaded region is the middle 90th percentile of service values. When restricted to VDR only, similar daytime charging patterns are observed, but with less overall energy throughput since the charged energy only serves EV travel demand. | |
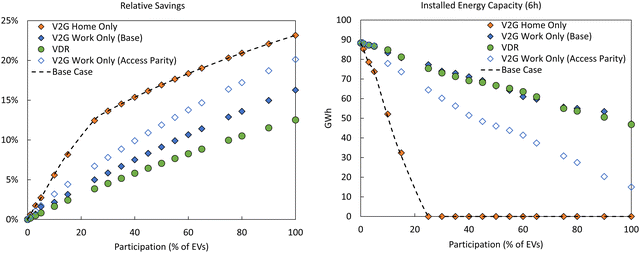 |
| Fig. 12 Net savings (left) and installed stationary storage energy capacity (right) as a function of EV fleet participation rate and V2G restrictions. The dashed black line coincides with unrestricted V2G in the base case (green diamonds in Fig. 9). All cases assume a 100% Level 2 charger share. “Access Parity” indicates a 90% charger accessibility for both home and workplace charging. All other cases assume 90% and 20% home and workplace charger availability, respectively. | |
Of course, these results are a function of case study assumptions and parameters, namely a significant difference in home and workplace charger access, which are 90% and 20%, respectively. When there is parity in charger access (90%), we see that work only V2G offers significantly more value and storage displacement. Interestingly, increasing charger access to 90% across all locations in the unconstrained base case offers limited marginal benefit in terms of savings and storage displacement, and only at low participation (see Fig. S8, ESI†). However, this is consistent with the results presented in Fig. 10 and 11, as the optimal load shifting profile is not constrained by the grid connected capacity. So, while we have confirmed that increased charger access and capacity serves to benefit the power system via increased flexibility, we also demonstrate that the marginal benefits of such upgrades are highly sensitive to system context – the specific combination of V2G availability, charger level, and participation. Elements of market saturation come into play. This is particularly evident in Fig. 9, as upgraded charging capacity (L1/L2 vs. L2) does not result in additional savings beyond ∼40% participation. Thus, one should carefully account for all three of accessibility, service capabilities, and anticipated market size when evaluating the ROI of V2G, VDR, or any form bidirectional infrastructure. For instance, take a hypothetical scenario in which there is low participation but all participating vehicles have access to Level 2 chargers at both home and work. In this case, upgrading to DC fast chargers will only do so much to effective capacity since the vehicles would be limited by relatively small battery capacities. Instead, it may be appropriate to invest in bringing V2G capabilities to new locations altogether, like shopping malls, to increase the number grid-connected EVs and effective capacity. As an extension of these results and discussion, we also include results and analysis for a “Vehicle-to-building” (V2B) arrangement, where vehicles only serve the loads of the locations/buildings at which they are parked, in the ESI.†
Conclusions and discussion
In this study we assessed V2G potential within the context of the greenfield buildout and operation of a low-carbon, New England power system in 2050. Across a range of carbon constraints and participation rates, the V2G aggregate is able to shift load via demand response and also shift excess renewables generation to periods of low availability and high net loads. Participating in both power and ancillary markers, the V2G aggregate provided substantial value, primarily deriving savings via stationary storage displacement. With relatively little participation, just 13.9% of the New England LDV fleet, 14.7 GW of 6 h stationary storage is completely displaced and amounts to over $700 million in savings. Additional savings come in the form of reductions in firm generation capacity and more efficient VRE utilization (i.e. reduced curtailment). Not only does this analysis demonstrate V2G's utility, but also the importance of how one chooses to measure its value (i.e. counting investment deferral), particularly in the context of future systems.
We also demonstrate that the magnitude and nature of V2G savings changes with system context. Under more aggressive emissions caps, V2G decreases the need for excessive VRE buildout or CCS technologies and its marginal value is thus greater. Further, the nature of optimal V2G dispatch is shown to change non-monotonically with participation rate. At low participation, V2G power injection is called on at a higher rate in order to shave uncontrolled evening charging loads, while higher participations rates rely on less injection through charge load shifting. Similarly, the value of V2G charging infrastructure changes with participation and location. Using our destination-based system topology, we demonstrate how charger level impacts system flexibility, that V2G injection capabilities are most valuable in residential areas, and that VDR alone will still require significant storage to support VRE generators under tight emissions constraints. We also demonstrate that the marginal benefits of such upgrades are highly sensitive to system context, providing examples of diminishing investment returns and highlighting the need for decision makers to balance investments in charger capabilities with overall accessibility and enrollment incentives.
Note that while the trend of V2G reducing stationary storage requirements holds independent of chemistry and size, our analysis does not consider V2G tradeoffs and interactions with future classes of long duration energy storage (LDES) that have been shown to support deep decarbonization.55 Including pumped hydroelectric and hydrogen storage, LDES can be synchronized with EV activity to further extend V2G benefits like load shifting or ancillary services. In the case of VRE utilization, for example, EV travel demand must be satisfied regardless of solar and wind output. Shorter duration storage, such as the 6-hour Li-ion batteries in this study, are not sufficient to remedy extended periods of low VRE generation, and so firm generators are required to satisfy EV loads. However, LDES (with durations on the order of days) synchronized with VDR confers a synergistic combination of significant supply side and demand side flexibility that stands to smooth over intermittencies, reduce grid congestion, and enable “dispatchable” VRE utilization across several days. Another possibility is that V2G and LDES can complement and add flexibility to each other's ancillary service offerings, with EV batteries providing rapid frequency regulation while LDES can in turn increase its operating reserve contributions. Thus, these areas stand to be investigated further in future works.
It is also important to note that these results are specific to a low-carbon case study characteristic of New England with high EV penetration. For instance, wind availabilities tend to be higher across the Midwest and EV market share may be less or more in other regions, and so V2G may displace storage and firm generators at different rates in other areas. This is particularly relevant in other countries with fewer vehicles and less infrastructure, and so results should not be generalized to all areas of the globe. Another regional difference of note, but not explored in this work, is the capacity and influence of existing pumped hydro assets. The implications of pairing V2G and pumped hydroelectric storage, which is expected to provide significant utility throughout the US northwest, are discussed above.
Further, there are certain limitations to our stylized analysis that impact results. For one, the greenfield analysis and assumption of no transmission and distribution constraints enables one-to-one sensitivity analysis of the tradeoffs between V2G service characteristics and other generation or storage investment, but simultaneously overestimates the true V2G system flexibility. Likewise, by using a single aggregate vehicle representation of all V2G participants, the locational value of vehicle clusters and their unique service capabilities have not been fully evaluated. Introducing multiple generation and load nodes (beyond the vehicle destinations) and vehicle clusters with their associated constraints can offer a more realistic view and reveal the value of vehicle co-location with distributed VRE installations. We also assume that future charging accessibility is similar to that of today, with V2G being possible at all participant locations with charger access. This neglects a key infrastructure investment cost and leaves untouched the possibility of direct current fast chargers, for example. Seeing the system sensitivity to charger level in this study, a more granular analysis of different infrastructure schemes and their costs are in order.
Looking ahead, this study stands to be built upon by including analysis of additional V2G locations and a wider breadth of EV fleets. For one, locations like shopping malls and restaurants are anticipated to install considerable charging capacity as the EV market grows. Given the long residence times of vehicles at these locations, it poses further opportunities for grid servicing beyond the home and/or workplace. This is particularly interesting to examine for scenarios in which participation is low and V2G services are not fully available (i.e. VDR only) or access is significantly limited (i.e. only available at workplace or commercial charging stations). By unlocking the connection capacity of additional locations, participating EV can better utilize VRE resources and in turn reduce storage in cases this work has shown to have lagging displacement. Likewise, V2G is not limited to light-duty passenger vehicles. Commercial EV fleets, such as school buses and delivery trucks, are poised to electrify and have unique characteristics – namely large batteries, predictable operating schedules, and overnight co-location – that make them prime V2G candidates able to offer significant quantities of demand response and ancillary services. The revenues earned from providing these services can be put toward offsetting costs of fleet electrification.
Author contributions
James Owens: conceptualization, methodology, software, investigation, formal analysis, writing – original draft, visualization. Ian Miller: conceptualization, methodology, writing – original draft. Emre Gençer: conceptualization, writing – review and editing, supervision, funding acquisition.
Conflicts of interest
There are no conflicts to declare.
Acknowledgements
The authors would like to thank Dr Dharik Mallapragada for providing his GenX model expertise and input on V2G model integration within GenX; and Prof. Robert C. Armstrong and Prof. Christopher Knittel and Amanda Farnsworth for their support and input over the course of this work. The authors also acknowledge the MIT SuperCloud and Lincoln Laboratory Supercomputing Center for providing computing resources that have contributed to the research results reported within this paper. This research was supported by MIT Energy Initiative Future Energy Systems Center.
References
- New Plug-in Electric Vehicle Sales in the United States Nearly Doubled from 2020 to 2021|Department of Energy, https://www.energy.gov/energysaver/articles/new-plug-electric-vehicle-sales-united-states-nearly-doubled-2020-2021.
- Electric Car Market Grows to 29% in Europe as EV Sales Explode! https://cleantechnica.com/2022/01/30/29-of-cars-sold-in-europe-were-plugin-electric-vehicles-in-december/.
- EVO Report 2021|BloombergNEF|Bloomberg Finance LP, https://about.bnef.com/electric-vehicle-outlook/.
- Electricity data browser – Net generation for all sectors, https://www.eia.gov/electricity/data/browser/.
- Frequently Asked Questions (FAQs) – U.S. Energy Information Administration (EIA), https://www.eia.gov/tools/faqs/faq.php?id=427&t=3.
- B. Obama, The irreversible momentum of clean energy: Private-sector efforts help drive decoupling of emissions and economic growth, Science, 2017, 355, 126–129 CrossRef CAS PubMed
.
- L. E. Erickson, Reducing greenhouse gas emissions and improving air quality: Two global challenges, Environ. Prog. Sustainable Energy, 2017, 36, 982–988 CrossRef CAS
.
- Climate Change 2022: Impacts, Adaptation and Vulnerability|Climate Change 2022: Impacts, Adaptation and Vulnerability, https://www.ipcc.ch/report/ar6/wg2/.
- State Renewable Portfolio Standards and Goals, https://www.ncsl.org/research/energy/renewable-portfolio-standards.aspx.
- O. J. Guerra, J. Eichman and P. Denholm, Optimal energy storage portfolio for high and ultrahigh carbon-free and renewable power systems, Energy Environ. Sci., 2021, 14, 5132–5146 RSC
.
- N. Armaroli and V. Balzani, Towards an electricity-powered world, Energy Environ. Sci., 2011, 4, 3193–3222 RSC
.
- SESAME, https://sesame.mit.edu/.
- M. B. Anwar,
et al., Assessing the value of electric vehicle managed charging: a review of methodologies and results, Energy Environ. Sci., 2022, 15, 466–498 RSC
.
- I. Miller, M. Arbabzadeh and E. Genç, Hourly Power Grid Variations, Electric Vehicle Charging Patterns, and Operating Emissions, Environ. Sci. Technol., 2020, 54, 16071–16085 CrossRef CAS
.
- B. K. Sovacool, L. Noel, J. Axsen and W. Kempton, The neglected social dimensions to a vehicle-to-grid (V2G) transition: A critical and systematic review, Environ. Res. Lett., 2018, 13, 013001 CrossRef
.
-
P. Barter, Cars are parked 95% of the time, 2013, https://www.reinventingparking.org/2013/02/cars-are-parked-95-of-time-lets-check.html.
-
K. Knezović, M. Marinelli, P. Codani and Y. Perez, Distribution grid services and flexibility provision by electric vehicles: A review of options, Proc. Univ. Power Eng. Conf., 2015-November, 2015.
- F. Yang, Y. Xie, Y. Deng and C. Yuan, Predictive modeling of battery degradation and greenhouse gas emissions from U.S. state-level electric vehicle operation, Nat. Commun., 2018, 9, 1–10 CrossRef PubMed
.
- A. Schuller, C. M. Flath and S. Gottwalt, Quantifying load flexibility of electric vehicles for renewable energy integration, Appl. Energy, 2015, 151, 335–344 CrossRef
.
- H. Mehrjerdi and E. Rakhshani, Vehicle-to-grid technology for cost reduction and uncertainty management integrated with solar power, J. Cleaner Prod., 2019, 229, 463–469 CrossRef
.
- N. Nezamoddini and Y. Wang, Risk management and participation planning of electric vehicles in smart grids for demand response, Energy, 2016, 116, 836–850 CrossRef
.
- R. Sioshansi and P. Denholm, Emissions impacts and benefits of plug-in hybrid electric vehicles and vehicle-to-grid services, Environ. Sci. Technol., 2009, 43, 1199–1204 CrossRef CAS
.
- G. Zhao and J. Baker, Effects on environmental impacts of introducing electric vehicle batteries as storage – A case study of the United Kingdom, Energy Strateg. Rev., 2022, 40, 100819 CrossRef
.
- M. Wang and M. T. Craig, The value of vehicle-to-grid in a decarbonizing California grid, J. Power Sources, 2021, 513, 230472 CrossRef CAS
.
- M. Huda, K. Tokimatsu and M. Aziz, Techno Economic Analysis of Vehicle to Grid (V2G) Integration as Distributed Energy Resources in Indonesia Power System, Energies, 2020, 13, 1162 CrossRef
.
- N. B. G. Brinkel, W. L. Schram, T. A. AlSkaif, I. Lampropoulos and W. G. J. H. M. van Sark, Should we reinforce the grid? Cost and emission optimization of electric vehicle charging under different transformer limits, Appl. Energy, 2020, 276, 115285 CrossRef
.
- P. Staudt, M. Schmidt, J. Gärttner and C. Weinhardt, A decentralized approach towards resolving transmission grid congestion in Germany using vehicle-to-grid technology, Appl. Energy, 2018, 230, 1435–1446 CrossRef
.
- P. Nunes and M. C. Brito, Displacing natural gas with electric vehicles for grid stabilization, Energy, 2017, 141, 87–96 CrossRef
.
- K. E. Forrest, B. Tarroja, L. Zhang, B. Shaffer and S. Samuelsen, Charging a renewable future: The impact of electric vehicle charging intelligence on energy storage requirements to meet renewable portfolio standards, J. Power Sources, 2016, 336, 63–74 CrossRef CAS
.
- B. Tarroja, L. Zhang, V. Wifvat, B. Shaffer and S. Samuelsen, Assessing the stationary energy storage equivalency of vehicle-to-grid charging battery electric vehicles, Energy, 2016, 106, 673–690 CrossRef
.
- B. Zhang and M. Kezunovic, Impact on Power System Flexibility by Electric Vehicle Participation in Ramp Market, IEEE Trans. Smart Grid, 2016, 7, 1285–1294 Search PubMed
.
- U. K. Debnath, I. Ahmad, D. Habibi and A. Y. Saber, Energy storage model with gridable vehicles for economic load dispatch in the smart grid, Int. J. Electr. Power Energy Syst., 2015, 64, 1017–1024 CrossRef
.
- M. Carrión and R. Zárate-Miñano, Operation of renewable-dominated power systems with a significant penetration of plug-in electric vehicles, Energy, 2015, 90, 827–835 CrossRef
.
- Z. Wang and S. Wang, Grid power peak shaving and valley filling using vehicle-to-grid systems, IEEE Trans. Power Delivery, 2013, 28, 1822–1829 Search PubMed
.
- Y. Ma, T. Houghton and A. Cruden, & Infield, D. Modeling the benefits of vehicle-to-grid technology to a power system, IEEE Trans. Power Syst., 2012, 27, 1012–1020 Search PubMed
.
- J. R. Pillai and B. Bak-Jensen, Integration of vehicle-to-grid in the Western Danish power system, IEEE Trans. Sustainable Energy, 2011, 2, 12–19 Search PubMed
.
- S. L. Andersson,
et al., Plug-in hybrid electric vehicles as regulating power providers: Case studies of Sweden and Germany, Energy Policy, 2010, 38, 2751–2762 CrossRef
.
- D. S. Mallapragada, N. A. Sepulveda and J. D. Jenkins, Long-run system value of battery energy storage in future grids with increasing wind and solar generation, Appl. Energy, 2020, 275, 115390 CrossRef
.
- N. A. Sepulveda, J. D. Jenkins, A. Edington, D. S. Mallapragada and R. K. Lester, The design space for long-duration energy storage in decarbonized power systems, Nat. Energy, 2021, 6, 506–515 CrossRef
.
- S. Ericson, K. Anderson, J. Engel-Cox, H. Jayaswal and D. Arent, Power couples: The synergy value of battery-generator hybrids, Electr. J., 2018, 31, 51–56 CrossRef
.
- M. Wolinetz, J. Axsen, J. Peters and C. Crawford, Simulating the value of electric-vehicle–grid integration using a behaviourally realistic model, Nat. Energy, 2018, 3, 132–139 CrossRef
.
- Welcome Page·GenX, https://genxproject.github.io/GenX/dev/.
- M. Woody, M. Arbabzadeh, G. M. Lewis, G. A. Keoleian and A. Stefanopoulou, Strategies to limit degradation and maximize Li-ion battery service lifetime – Critical review and guidance for stakeholders, J. Energy Storage, 2020, 28, 101231 CrossRef
.
- A. W. Thompson, Economic implications of lithium ion battery degradation for Vehicle-to-Grid (V2X) services, J. Power Sources, 2018, 396, 691–709 CrossRef CAS
.
- S. Saxena, C. Le Floch, J. Macdonald and S. Moura, Quantifying EV battery end-of-life through analysis of travel needs with vehicle powertrain models, J. Power Sources, 2015, 282, 265–276 CrossRef CAS
.
- D. Wang, J. Coignard, T. Zeng, C. Zhang and S. Saxena, Quantifying electric vehicle battery degradation from driving vs. vehicle-to-grid services, J. Power Sources, 2016, 332, 193–203 CrossRef CAS
.
- M. Jafari, A. Gauchia, S. Zhao, K. Zhang and L. Gauchia, Electric Vehicle Battery Cycle Aging Evaluation in Real-World Daily Driving and Vehicle-to-Grid Services, IEEE Trans. Transp. Electrif., 2017, 4, 122–134 Search PubMed
.
-
A. Hoke, A. Brissette, D. Maksimović, A. Pratt and K. Smith, Electric vehicle charge optimization including effects of lithium-ion battery degradation, 2011 IEEE Veh. Power Propuls. Conf. VPPC, 2011 DOI:10.1109/VPPC.2011.6043046.
-
A. Langton and N. Crisostomo, Vehicle-Grid Integration A Vision for Zero-Emission Transportation Interconnected throughout California's Electricity System, 2013 Search PubMed
.
- National Household Travel Survey, https://nhts.ornl.gov/documentation.
- E. Gençer, S. Torkamani, I. Miller, T. W. Wu and F. O’Sullivan, Sustainable energy system analysis modeling environment: Analyzing life cycle emissions of the energy transition, Appl. Energy, 2020, 277, 115550 CrossRef
.
- EIA – U.S. Battery Storage Market Trends, https://www.eia.gov/analysis/studies/electricity/batterystorage/.
- Electric Car Expected to Make Up a Third of All Vehicle Sales in India by 2030 – Bloomberg, https://www.bloomberg.com/news/articles/2022-06-17/about-a-third-of-vehicles-sold-in-india-will-be-electric-by-2030.
- Electric Vehicle Sales and Market Share (US – Updated Monthly) – YAA, https://joinyaa.com/guides/electric-vehicle-market-share-and-sales/#EV_Market_Share_in_US.
-
The Future of Energy Storage: An Interdisciplinary MIT Study, 2022, https://energy.mit.edu/research/future-of-energy-storage/.
Footnotes |
† Electronic supplementary information (ESI) available. See DOI: https://doi.org/10.1039/d2ya00204c |
‡ VRE curtailment is the reduction of generator output below what could have otherwise been produced (https://www.caiso.com). Curtailment can arise due to an oversupply of energy and limited storage capacity, or, at other times, due of transmission congestion. |
§ 25% participation of a 60% EV stock corresponds to 15% of total LDV fleet. Likewise, 23.1% EV participation corresponds to 13.9% of the LDV fleet. |
¶ With an average duration of 1.6 hours. |
|| This is true for future cases in which integrated grids enable aggregate level service within residential and commercial clusters. For single-vehicle V2H cases or V2B, the charging infrastructure could remain the limiting component. |
|
This journal is © The Royal Society of Chemistry 2022 |
Click here to see how this site uses Cookies. View our privacy policy here.