DOI:
10.1039/D2AN02057B
(Paper)
Analyst, 2023,
148, 2073-2080
Prediction of gastric cancer by machine learning integrated with mass spectrometry-based N-glycomics†
Received
19th December 2022
, Accepted 29th March 2023
First published on 30th March 2023
Abstract
Early and accurate diagnosis of gastric cancer is vital for effective and targeted treatment. It is known that glycosylation profiles differ in the cancer tissue development process. This study aimed to profile the N-glycans in gastric cancer tissues to predict gastric cancer using machine learning algorithms. The (glyco-) proteins of formalin-fixed parafilm embedded (FFPE) gastric cancer and adjacent control tissues were extracted by chloroform/methanol extraction after the conventional deparaffinization step. The N-glycans were released and labeled with a 2-amino benzoic (2-AA) tag. The MALDI-MS analysis of the 2-AA labeled N-glycans was performed in negative ionization mode, and fifty-nine N-glycan structures were determined. The relative and analyte areas of the detected N-glycans were extracted from the obtained data. Statistical analyses identified significant expression levels of 14 different N-glycans in gastric cancer tissues. The data were separated based on the physical characteristics of N-glycans and used to test in machine-learning models. It was determined that the multilayer perceptron (MLP) was the most appropriate model with the highest sensitivity, specificity, accuracy, Matthews correlation coefficient, and f1 scores for each dataset. The highest accuracy score (96.0 ± 1.3) was obtained from the whole N-glycans relative area dataset, and the AUC value was determined as 0.98. It was concluded that gastric cancer tissues could be distinguished from adjacent control tissues with high accuracy using mass spectrometry-based N-glycomic data.
Introduction
Cancer is the abnormal proliferation of cells due to both genetic problems and environmental influences. Because of metastasis, it spreads to other tissues and organs and multiplies.1 Gastric cancer is the third most common cause of cancer death worldwide.2 It is mainly seen in the elderly and diagnosed histologically. Scientists have been trying to reduce the deadly effects of all types of cancer including gastric cancer.3 Detecting cancer accurately in the early stages is vital in terms of starting treatments in the earliest period. Many researchers are focusing on this issue and trying to develop new methods for cancer diagnosis.4 Efforts to find new biomarkers for cancer diagnosis are increased in the literature.5
Glycosylation, which is the attachment of glycan structures to proteins, is a post-translational modification that causes wider proteomic variety compared to other post-translational modifications.6,7 It is critical for various cellular processes, such as cell adhesion to the extracellular matrix and protein–ligand interactions within the cell.6 Therefore, it is necessary to detect the changes in diseases that occur during glycosylation.8 It has been reported that abnormal glycosylation in protein structures is associated with cancer, genetic disorders, and immune system diseases.9–11 These variations in glycosylation can be identified by specific changes in O- and N-glycan structures.12 Furthermore, differences in glycosyl-transferase expression, which is described as the production of transcripts and enzyme activity, dramatically affect the output of different glycans and the degree of branching of core glycans that can alter the glycan structures.13,14 Glycomics is a field that focuses on the analysis of N- or O-linked glycans found in the cellular glycoproteome. Thanks to the technological advances in mass spectrometry and sample preparation methods, glycans belonging to any biological sample can be profiled accurately and quickly.15–17 Although many studies have demonstrated the alteration of glycosylation in cancer processes, its performance in cancer-control distinction in many biomedical applications has not been adequately examined yet. Regarding glycosylation analysis, most of the studies conducted with machine learning have focused on data analysis such as predictions of glycosylation sites or glycoforms.18–22 In very few studies, clinical glycomics have been integrated with machine learning. Recently, in a study conducted by Chocholova et al., a machine learning application was combined with glycomics to identify seropositive and seronegative rheumatoid arthritis patients.23 Mészáros et al. developed another application profiling the human serum N-glycome for lung tumor surgery by using machine learning-based analysis.24 In addition, Pan et al. used machine learning to screen and diagnose colorectal cancer and advanced adenoma based on human serum N-glycome profiles.25
The aberrant glycan profiles play a vital role in the pathophysiological steps of gastric cancer. It has been determined that glycosylation is a modulator for gastric cancer cell behavior and can be used for the clinical management of cancer patients.26 The significant targets of clinical cancer management are to enhance early diagnosis, improve medicinal consequences, and ease the suffering of patients. Novel approaches for early diagnosis are required, and glycans can be a valuable source for such applications. Most of the traditional cancer markers, such as CEA125 (for ovarian cancer) and PSA (for prostate cancer), are glycoproteins used for diagnosis.27 However, the specificity and sensitivity of these markers to detect several cancer types are poor.28 Therefore, research is needed for new biomarker candidates. On the other hand, profiling the glycans of a particular glycoproteome may contribute to creating a biomarker with higher specificity for early cancer detection. Indeed, specific glycosylation profile-based biomarker candidate has improved the early diagnosis of cancers, such as prostate cancer.29 Therefore, considering the potential change of N-glycan profiles during gastric cancer cell proliferation, we tried to distinguish gastric cancer tissues from control tissues by using a machine learning model to reduce the time and cost of the diagnosis.
Machine learning is a branch of artificial intelligence that uses various statistical and optimization techniques that allow computers to learn from past examples and detect distinctive patterns, which are difficult to distinguish, from large and complex data sets.30 Machine learning algorithms are frequently used in biomedicine applications, such as the calculation of risk factors of individuals in many diseases, cancer diagnosis, image processing, and drug discovery.31–34 The need for accuracy in the histopathological diagnosis of cancer is increasing since there is a need for accurate biomarker assessment for personalized cancer therapy.35 Therefore, to be able to diagnose diseases rapidly, it is necessary to develop new applications similar to the histopathological method of cancer tissues.
In this current study, an approach was developed to predict gastric cancer tissues with the help of machine learning by using mass spectrometry-based N-glycan datasets. The analysis of N-glycans derived from FFPE cancer and control tissues was achieved with a fast approach by MALDI-MS. The datasets were created using the detected N-glycans’ relative and analyte areas. Machine learning models were tested to distinguish gastric cancer tissues from adjacent control tissues based on their N-glycome profiles. Based on our knowledge related to the literature, it can be said that this is the first study focusing on distinguishing gastric cancer tissues from adjacent control tissues by using a machine learning approach integrated with mass spectrometry-based N-glycomics.
Materials and methods
Some of the materials used in the study, such as methanol, acetonitrile, acetic acid (CH3COOH), ethanol, xylene, 2-amino benzoic acid (2-AA), dimethyl sulfoxide (DMSO), trifluoroacetic acid (TFA), 1,4-dithiothreitol (DTT), sodium dodecyl sulfate (SDS), sodium cyanoborohydride (NaBH3CN), and sodium chloride (NaCl), were obtained from Sigma-Aldrich Company (St Louis, MO, USA). Peptide-N-glycosidase F (PNGase F) enzyme was obtained from Promega (Madison, WI, USA). 2,5-Dihydroxy benzoic acid (DHB) was obtained from Bruker Daltonics (Bremen, Germany). Deionized water (dH2O) was taken from an Expe–Ultrapure Water System (Mirae St, Korea).
Sample collection
This study was approved by Karabük University Clinical Research Ethics Committee (Ethics Committee Decision no: 2019/117). FFPE gastric cancer tissues used in the study were obtained from Karabük University, Faculty of Medicine, Medical Pathology Department (Karabük-Turkey). The tissue samples were collected retrospectively. The pathologist distinguished cancer and adjacent control tissues by histopathological evaluation. Histochemically confirmed gastric specimens of 33 cancer and 31 adjacent control FFPE tissues were separately cut from tissue blocks using a microtome. FFPE blocks were 10 μm thick. The tissue sections were transferred into the microcentrifuge tubes and stored at room temperature before the analysis.
Protein extraction and N-glycan release from FFPE tissues
The previously applied protocol was followed with minor modifications.36 The FFPE tissues underwent the following process: first, they were subjected to incubation at 60 °C for half an hour, then treated with 1 mL of xylene for 5 minutes and rinsed twice with 1 mL of 100% ethanol. The liquids were removed and the tissues were dried using a SpeedVac concentrator at 45 °C for 5 minutes. Next, the tissues were incubated with 150 μL of 0.1 M DTT, followed by sonication (1.5 pulses with 3-second intervals for 30 seconds). Finally, 60 μL of 16% SDS was added to the tissues and they were incubated at 99 °C for 1 hour while being agitated at 600 rpm. After centrifugation at 2000 rcf for 20 minutes, 150 μL of each sample was taken from the upper phase and transferred to new microcentrifuge tubes. Then, 600 μL of methanol, 150 μL of chloroform, and 450 μL of deionized water were added to the samples, which were mixed for 10 seconds and then centrifuged at 14
000 rcf for 5 minutes. The upper phase was carefully removed without disturbing the middle pellet. The samples were then treated with 450 μL of methanol and centrifuged at 14
000 rcf for 10 minutes. The proteins at the bottom were dried using a speed vacuum concentrator at 45 °C for 30 minutes, and finally stored at −20 °C.
The dried protein pellet was dissolved by 25 μL of 1% SDS, followed by vortexing for 20 seconds and incubation at 60 °C for 5 minutes. Then, 12.5 μL of 2% Igepal-CA360, 12.5 μL of 5X PBS, and 1 μL of PNGase F were added to each sample, which were further incubated overnight at 37 °C.
2-AA labeling and purifications of N-glycans
For 2-AA labeling of released N-glycans, 25 μL of the 2-AA (48 mg mL−1), 25 μL of sodium cyanoborohydride (63 mg mL−1) prepared in DMSO, and glacial acetic acid at a ratio of 10
:
3 were inserted to each sample. The samples were then incubated at 65 °C for 2 h. As described previously,37 the labeled N-glycans were purified with cellulose and porous graphitized carbon-containing SPE cartridges.
MALDI-MS analysis
MALDI-MS analyses of 2-AA-labeled N-glycans were performed using a RapiFlex MALDI-TOF/TOF-MS/MS (Bruker Daltonik GmbH, Bremen, Germany) incorporating SmartBeam 3D laser technology. 1 μL of the purified sample solutions was dropped directly onto the MALDI target plate and allowed to dry. 1 μL of 5 mg mL−1 DHB matrix prepared using 50% dH2O and 50% ACN was added to the dried samples. The MALDI-MS spectra were recorded at 20 kV acceleration potential in negative ionization mode with a mass range of 1000–4000 Da using reflectron mode. Spectra were recorded by collecting 8000 laser pulses at 2000 Hz frequency. Four different spectra of each sample were obtained from 4 different spots and used in the analyses.
Data analysis for MALDI-MS experiments
The obtained MS and MS/MS spectra were transferred to Protein Scape V4 (Bruker Daltonik GmbH, Bremen, Germany) software to identify their composition and structures using the GlycoQuest algorithm. Based on the previous descriptions,38 the N-glycan structures were confirmed with MS/MS spectra matches by using GlycoQuest algorithms. The analyte areas of the detected N-glycans were extracted using the MassyTools software.39 The relative area of each detected N-glycans was calculated using the total area normalization approach. The total area normalization approach was applied for neutral and whole N-glycan compositions separately.
Statistical analysis
GraphPad Prism software (9.0) was used for statistical analysis in the study. Mann–Whitney test was used to determine any differences in expression levels of N-glycans between gastric cancer and control tissues. In addition, ROC (Receiver Operating Characteristics) analysis was performed with a 95% confidence interval (CI) by the Wilson/Brown method. The statistical analyses were performed on relative area values.
Machine learning
The data were manually evaluated based on the quality criteria by checking their S/N ratio. Then, a total of 250 MALDI-MS spectra were obtained from 33 cancerous and 31 control tissues and used in the machine learning analysis (ESI Tables S2–S5†). Data from 4 technical replicates of each cancer and control sample were included in the study. First, four datasets were created, including relative and absolute analyte area values of total and neutral N-glycans obtained from cancerous and adjacent control tissues. The five-fold cross-validation approach was used for the training process, and the classification accuracy was calculated to illustrate the performance of each model for the four created different datasets. The confusion matrix and area under the curve (AUC) of the model were developed to detect the most appropriate method for distinguishing gastric cancer tissues from adjacent control tissues. The confusion matrix includes four parameters as follows: True Positive (TP), False Positive (FP), False Negative (FN), and True Negative (TN). Whereas TP refers to correctly determining conditions, FP refers to incorrectly determining conditions. Whereas FN refers to incorrectly rejected conditions, TN refers to correctly rejected conditions. Mathematical calculations of sensitivity, specificity, accuracy, MCC, and F1 score are displayed in eqn (1)–(5). | 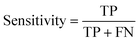 | (1) |
| 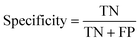 | (2) |
| 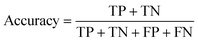 | (3) |
|  | (4) |
| 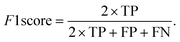 | (5) |
Results and discussion
Profiling of FFPE gastric tissue N-glycans by MALDI-MS
In the study, 33 cancerous and 31 adjacent control tissues belonging to gastric cancer patients were analyzed. Demographics of the individuals from the cohort are presented in Table S1.† In the analysis, proteins were extracted from the tissues using the method described in the Method section. Afterward, glycoproteins were treated with the PNGase F enzyme to release the N-glycans and labeled with a 2-AA fluorescence label. Their analysis was performed by MALDI-MS in negative ionization mode, and an overview of the applied methodology is given in Fig. 1. Fig. 2 displays the MALDI-MS spectra of gastric cancerous tissue and an adjacent control tissue. 59 N-glycans, including 45 neutral glycans and 14 acidic glycans, were detected (ESI Table S2†). MS/MS spectra of 37 N-glycans were obtained, and the resulting MS/MS fragment products were searched using the GlycoQuest tool to confirm the structure of the glycans. The created datasets were then used for statistical and machine-learning analysis.
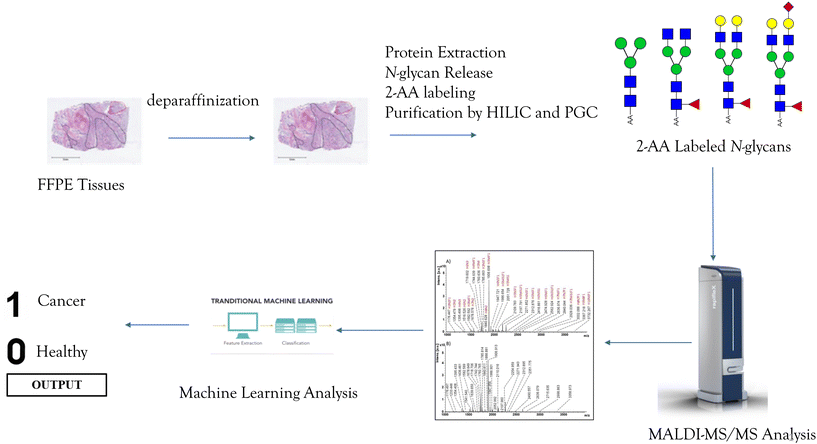 |
| Fig. 1 An overview of the methodology applied in the study. | |
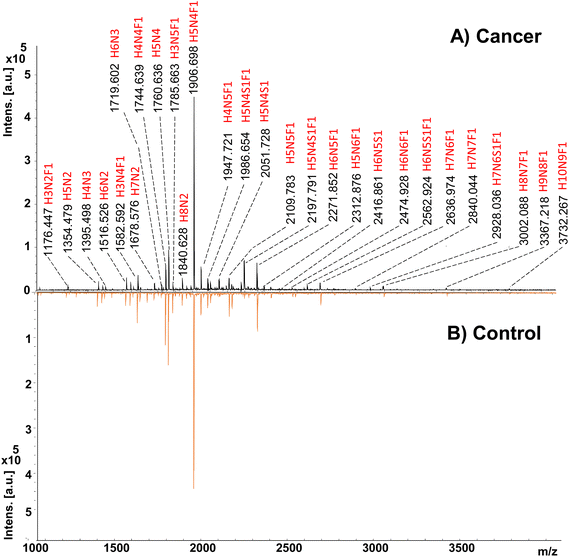 |
| Fig. 2 MALDI-MS spectra of 2-AA labeled N-glycans belonging to (A) gastric cancer tissue, and (B) control cancer tissue. | |
Investigation of N-glycan changes between gastric cancer and adjacent control tissues
ESI Table S3† shows that there were 14 N-glycans with statistically significant differences between cancer cases and controls, as evidenced by a p-value less than 0.001. The relative areas of N-glycans were compared in a box plot, as shown in ESI Fig. S1.† In gastric cancer tissues, N-glycans such as H3N2F1, H4N3F1, H6N5F1, H6N6F1, H7N6F1, H6N5S3, H8N7F1, and H10N9F1 were increased, while N-glycans including H3N4F1, H4N4F1, H3N5F1, H4N5F1, and H3N6S1F1 were decreased. ROC analysis was performed to identify potential biomarker candidates with the Wilson/Brown method at a 95% confidence interval, and the results including ROC curve graphs and AUC values are shown in ESI Fig. S3 and ESI Table S3,† respectively. The best N-glycan conformations for distinguishing gastric cancer tissues from controls were found to be H3N5F1 with an AUC of 0.76 and H7N6F1 with an AUC of 0.75.
Classification of gastric cancer tissues by machine learning models
In order to differentiate between gastric cancer tissues and adjacent control tissues, machine learning models were tested using the relative and analyte areas of the neutral and acidic N-glycan datasets. The study included 250 MALDI-MS spectra obtained from technical replicates of 33 cancerous and 31 adjacent control tissue samples. The relative and analyte areas of N-glycans utilized in the classification analysis are given in ESI Tables S4–S7.† These datasets were separated as neutral N-glycans and whole N-glycans, including acidic types (Tables S4–5† for whole N-glycan datasets, Tables S6–7† for neutral N-glycan datasets). The study was employed normalized and non-normalized datasets to evaluate the classification accuracy of machine learning models in both types of data.
To distinguish between gastric cancer and adjacent control tissues, machine learning models were tested using four different datasets. The dataset was divided into folds to evaluate the model's performance on new data using a five-fold cross-validation approach. The data sets were divided into training sets (80%) and validation sets (20%) and various machine learning models were tested. It was determined that the multilayer perceptron (MLP) algorithm was the most accurate model for distinguishing gastric cancer tissues based on N-glycan profiles. The results of the MLP algorithm in terms of sensitivity, specificity, accuracy, MCC, and F1 score are provided in Table 1. The best results in terms of predicting gastric cancer were obtained from the relative area datasets of neutral and whole N-glycans with accuracy scores of 96.0% and 94.0%, respectively. The prediction accuracy for the analyte area datasets of neutral and whole N-glycans was 92.2% and 94.0%, respectively. Confusion matrices were also created to validate the results and determine the most appropriate approach for classifying gastric cancer tissues. The confusion matrices obtained from the four datasets based on N-glycan profiles are shown in Fig. 3. The true positive ratios, representing the prediction accuracy of gastric cancer cases, were 93.1% and 96.3% for the relative area datasets of neutral and whole N-glycans, respectively. The true negative ratios, representing the prediction accuracy of control tissues, were 100% and 95.6% for the relative areas of neutral and whole N-glycan datasets, respectively. On the other hand, the true positive ratio for the analyte area datasets of neutral and whole N-glycans was 92.6% and 96.8%, respectively, while the true negative ratio was 91.7% and 92.3%, respectively.
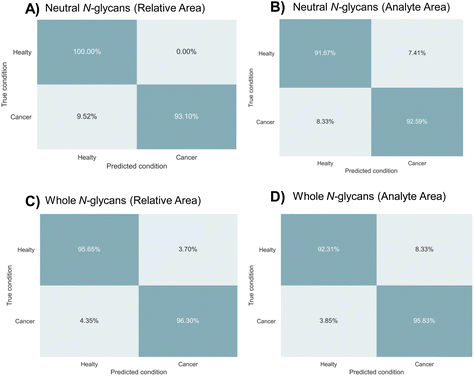 |
| Fig. 3 Confusion matrixes of MLP model from different datasets (A) relative area of neutral N-glycans, (B) analyte area of neutral N-glycans, (C) relative area of whole N-glycans, and (D) analyte area of whole N-glycans. | |
Table 1 Sensitivity, specificity, accuracy, f1, and MCC results of machine learning model (multilayer perceptron, MLP) to discriminate gastric cancer tissues based on their N-glycan profiles
Parameters |
Dataset |
Neutral N-glycans (relative area) |
Neutral N-glycans (analyte area) |
Whole N-glycans (relative area) |
Whole N-glycans (analyte area) |
Sensitivity % |
0.93 |
0.93 |
0.96 |
0.96 |
Specificity % |
0.87 |
0.92 |
0.96 |
0.92 |
MCC |
0.92 |
0.84 |
0.92 |
0.88 |
F1 score |
0.96 |
0.93 |
0.96 |
0.94 |
Accuracy % |
96.0% |
92.16% |
96.0% |
94.0% |
Split (5) average accuracy score |
95.6 ± 3.44 |
91.37 ± 2.0 |
96.0 ± 1.26 |
93.2 ± 3.71 |
In machine learning analysis, the most reasonable performance metric is the ratio of correctly classified samples to the total number of samples.40 In addition to accuracy, sensitivity, and specificity, other parameters, such as the Matthews Correlation Coefficient (MCC) and F1 score, are also used to determine the performance of a machine learning model. The MCC measures the correlation between the binary classification predictions made by the model and the actual classifications, and ranges from −1 to 1, with 1 indicating perfect prediction and −1 indicating complete disagreement between the predictions and actual results. The F1 score, which combines the harmonic mean of precision and recall, provides a balanced evaluation of the model's performance by taking both precision and recall into account. The results of the MLP algorithm showed that the MCC score was 0.92 and the F1 score was 0.96 for the relative area dataset of the all N-glycans, suggesting that the model has the ability to provide accurate results based on the high values of both parameters.
ROC curves were obtained to evaluate the diagnostic ability of each dataset using the MLP model. The performance of the model was assessed through 5-fold cross-validation in the training cohort, using the area under the receiver operating characteristic curves (AUC). Fig. 4 shows the ROC curves and the calculated AUC values for each dataset. For the relative area datasets of neutral and whole N-glycans, the AUC values were found to be 0.97 and 0.98 respectively (Fig. 4A and C). For the analyte area datasets of neutral and whole N-glycans, the AUC values were 0.96 and 0.98, respectively (Fig. 4B and D).
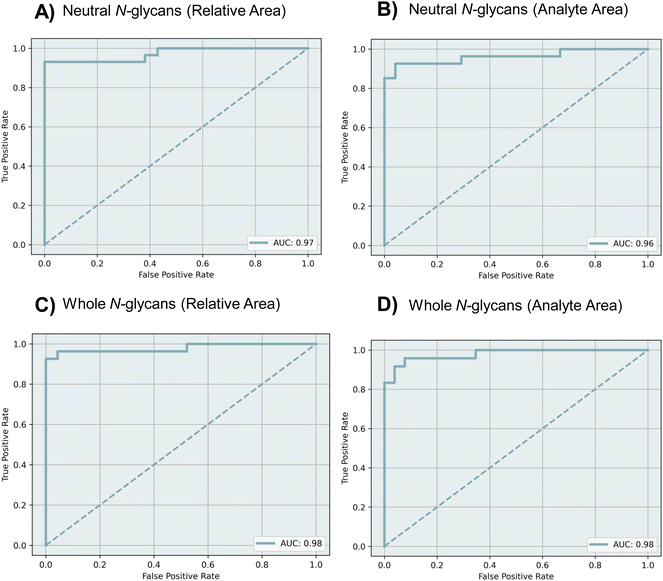 |
| Fig. 4 ROC curves including AUC values of MLP model from different datasets (A) relative area of neutral N-glycans, (B) analyte area of neutral N-glycans, (C) relative area of whole N-glycans, and (D) analyte area of whole N-glycans. | |
In order to determine which glycan structures played important roles in discriminating cancer cases from controls, the Fischer score analysis was applied. Various N-glycan structures played significant roles in the discrimination of cancer cases from controls (Fig. S3–6†). It was determined that some structures with a high Fischer score were the glycans that significantly changed between cancer cases and controls (for the relative area dataset of whole N-glycans). However, the other glycan structures that were not expressed considerably also contributed to the model's performance.
Machine learning is of great interest for computer-aided diagnostics due to its fast and cost-effective analytical solutions. In this study, machine learning was integrated with mass spectrometry-based N-glycomics to accurately diagnose gastric cancer. Four novel datasets were created and analyzed, including the relative and analyte areas of N-glycan profiles of gastric cancer and control tissues. After testing various machine learning models, it was found that MLP was the best for diagnosing gastric cancer. All datasets showed good sensitivity, specificity, and accuracy (as seen in Table 1). The whole N-glycan dataset had the highest accuracy (96.0%) and AUC value (0.98). This demonstrates that machine learning analysis of glycomic datasets can effectively diagnose gastric cancer in tissues. Additionally, the normalized datasets (relative areas of N-glycans) showed more robust results according to the machine learning performance criteria (as seen in Table 1).
Statistical analysis showed that 14 N-glycan types were differently expressed in gastric cancer tissues. The best discriminator N-glycan types for gastric cancer were H3N5F1 with an AUC of 0.76 and H7N6F1 with an AUC of 0.75. However, when all N-glycan types were analyzed together using machine learning, gastric cancer tissues were discriminated from controls with high accuracy (96.0%). Additionally, the performance scores of the machine learning models, including sensitivity, specificity, MCC, and f1, were found to be high. These results are stronger because machine learning considers the dataset as a whole compared to statistical methods.
N-Glycan analysis using MALDI-MS is a common method in the field of glycomics. In this study, an appropriate method was chosen for N-glycan profiling of FFPE tissues. A previously described protocol was employed to extract proteins from FFPE tissues for N- and O-glycan analysis with PGC-MS.36 This extraction method yields an adequate amount of proteins (10–50 μg), as measured by the BCA assay method using a spectrophotometer. On the other hand, the 2-AA labeling approach improves the ionization efficiency of N-glycans, allowing for the efficient analysis of even small amounts.41 The labeling strategy is simple and cost-effective. The use of a two-step purification process involving HILIC and PGC materials results in a cleaner sample compared to using a single HILIC protocol. Furthermore, the deglycosylation process can be completed in just one hour, rather than requiring overnight incubation. To minimize variations between samples, all samples were subjected to identical analysis conditions, such as constant voltage and laser energy power. This method can be applied in any laboratory for quick N-glycan profiling of tissue and may be used to accurately diagnose gastric cancer.
Conclusion
In a study, it was discovered that the 14 N-glycan composition was expressed differently in gastric cancer tissues compared to adjacent control tissues. The gastric cancer tissues were differentiated from the control tissues using a machine learning model based on glycomic data. The highest results in accuracy, specificity, sensitivity, MCC, and F1 score were achieved using an MLP machine learning model. It was found that the relative area N-glycan dataset covering all glycans was the best choice for diagnosing gastric cancer tissue. The N-glycomic method applied to FFPE tissue and the machine learning model may be used for an accurate and fast diagnosis of gastric cancer.
Conflicts of interest
The manuscript was written with the contributions of all authors. All authors have approved the final version of the manuscript. The mass spectrometric data used in machine learning analysis are presented in ESI Tables S4–S7.†
Acknowledgements
This study was supported by Karabük University Scientific Research Unit Program with project number: FYL-2020-2243. This study was also partly funded by the Ministry of Development-Republic of Turkey with project number: 2016 K121230. Bekir Salih would like to thank the Turkish Academy of Science (TUBA) for the partial financial support. This article is dedicated to the people who lost their lives in the earthquake disaster in Turkiye and Syria.
References
- D. Hanahan, Cancer Discovery, 2022, 12, 31–46 CrossRef CAS PubMed.
- E. C. Smyth, M. Nilsson, H. I. Grabsch, N. C. T. van Grieken and F. Lordick, Lancet, 2020, 396, 635–648 CrossRef CAS PubMed.
- E. Van Cutsem, X. Sagaert, B. Topal, K. Haustermans and H. Prenen, Lancet, 2016, 388, 2654–2664 CrossRef CAS PubMed.
- R. Sitarz, M. Skierucha, J. Mielko, G. J. A. Offerhaus, R. Maciejewski and W. P. Polkowski, Cancer Manage. Res., 2018, 10, 239–248 CrossRef CAS PubMed.
- S. S. Pinho and C. A. Reis, Nat. Rev. Cancer, 2015, 15, 540–555 CrossRef CAS PubMed.
- A. Varki, Glycobiology, 2017, 27, 3–49 CrossRef CAS PubMed.
- H. M. Kayili, M. Atakay, A. Hayatu and B. Salih, Adv. Sample Prepar., 2022, 4, 100042, DOI:10.1016/j.sampre.2022.100042.
- L. R. Ruhaak, G. G. Xu, Q. Y. Li, E. Goonatilleke and C. B. Lebrilla, Chem. Rev., 2018, 118, 7886–7930 CrossRef CAS PubMed.
- K. Ohtsubo and J. D. Marth, Cell, 2006, 126, 855–867 CrossRef CAS PubMed.
- M. E. Yaman, E. Aladağ, H. M. Kayili, Y. Kadioğlu and B. Salih, Hacettepe J. Biol. Chem., 2021, 49(2), 147–155, DOI:10.15671/hjbc.717600.
- Ö. F. Koçak, H. M. Kayili, M. Albayrak, M. E. Yaman, Y. Kadıoğlu and B. Salih, Anal. Biochem., 2019, 584, 113389 CrossRef PubMed.
- C. Reily, T. J. Stewart, M. B. Renfrow and J. Novak, Nat. Rev. Nephrol., 2019, 15, 346–366 CrossRef PubMed.
-
N. Taniguchi and Y. Kizuka, in Glycosylation and Cancer, ed. R. R. Drake and L. E. Ball, 2015, vol. 126, pp. 11–51 Search PubMed.
- L. Oliveira-Ferrer, K. Legler and K. Milde-Langosch, Semin. Cancer Biol., 2017, 44, 141–152 CrossRef CAS PubMed.
- I. Trbojevic-Akmaciic, G. S. M. Lageveen-Kammeijer, B. Heijs, T. Petrovic, H. Deris, M. Wuhrer and G. Lauc, Chem. Rev., 2022, 122(20), 15865–15913, DOI:10.1021/acs.chemrev.1c01031.
- N. de Haan, M. Pucic-Bakovic, M. Novokmet, D. Falck, G. Lageveen-Kammeijer, G. Razdorov, F. Vuckovic, I. Trbojevic-Akmacic, O. Gornik, M. Hanic, M. Wuhrer, G. Lauc and P. Human Glycome, Glycobiology, 2022, 32, 651–663 CrossRef CAS PubMed.
- G. S. M. Lageveen-Kammeijer, B. Kuster, D. Reusch and M. Wuhrer, Mass Spectrom. Rev., 2022, 41, 1014–1039 CrossRef CAS PubMed.
- H. Li, A. W. T. Chiang and N. E. Lewis, Biotechnol. Adv., 2022, 60, 108008 CrossRef CAS PubMed.
- D. Bojar and F. Lisacek, Chem. Rev., 2022, 122(20), 15971–15988, DOI:10.1021/acs.chemrev.2c00110.
- A. Antonakoudis, B. Strain, R. Barbosa, I. Jimenez del Val and C. Kontoravdi, Comput. Chem. Eng., 2021, 154 DOI:10.1016/j.compchemeng.2021.107471.
- P. Kotidis and C. Kontoravdi, Metab. Eng. Commun., 2020, 10 DOI:10.1016/j.mec.2020.e00131.
- L. Bezjak, V. E. Zajec, Š. Baebler, T. Stare, K. Gruden, A. Pohar, U. Novak and B. Likozar, Biotechnol. Bioeng., 2021, 118, 1476–1490, DOI:10.1002/bit.27660.
- E. Chocholova, T. Bertok, E. Jane, L. Lorencova, A. Holazova, L. Belicka, S. Belicky, D. Mislovicova, A. Vikartovska, R. Imrich, P. Kasak and J. Tkac, Clin. Chim. Acta, 2018, 481, 49–55 CrossRef CAS PubMed.
- B. Mészáros, G. Járvás, R. Kun, M. Szabó, E. Csánky, J. Abonyi and A. Guttman, Cancers, 2020, 12, 3700 CrossRef PubMed.
- Y. Pan, L. Zhang, R. Zhang, J. Han, W. Qin, Y. Gu, J. Sha, X. Xu, Y. Feng, Z. Ren, J. Dai, B. Huang, S. Ren and J. Gu, Am. J. Cancer Res., 2021, 11, 3002–3020 CAS.
- S. S. Pinho, S. Carvalho, R. Marcos-Pinto, A. Magalhães, C. Oliveira, J. Gu, M. Dinis-Ribeiro, F. Carneiro, R. Seruca and C. A. Reis, Trends Mol. Med., 2013, 19, 664–676 CrossRef CAS PubMed.
- C. A. Reis, H. Osorio, L. Silva, C. Gomes and L. David, J. Clin. Pathol., 2010, 63, 322–329 CrossRef CAS PubMed.
- R. M. Hoffman, F. D. Gilliland, M. Adams-Cameron, W. C. Hunt and C. R. Key, BMC Fam. Pract., 2002, 3, 19 CrossRef PubMed.
- E. Llop, M. Ferrer-Batallé, S. Barrabés, P. E. Guerrero, M. Ramírez, R. Saldova, P. M. Rudd, R. N. Aleixandre, J. Comet, R. de Llorens and R. Peracaula, Theranostics, 2016, 6, 1190–1204 CrossRef CAS PubMed.
- A. Rajkomar, J. Dean and I. Kohane, N. Engl. J. Med., 2019, 380, 1347–1358 CrossRef PubMed.
- J. Vamathevan, D. Clark, P. Czodrowski, I. Dunham, E. Ferran, G. Lee, B. Li, A. Madabhushi, P. Shah, M. Spitzer and S. Zhao, Nat. Rev. Drug Discovery, 2019, 18, 463–477 CrossRef CAS PubMed.
- B. Sahiner, A. Pezeshk, L. M. Hadjiiski, X. S. Wang, K. Drukker, K. H. Cha, R. M. Summers and M. L. Giger, Med. Phys., 2019, 46, e1–e36 CrossRef PubMed.
- M. J. Willemink, W. A. Koszek, C. Hardell, J. Wu, D. Fleischmann, H. Harvey, L. R. Folio, R. M. Summers, D. L. Rubin and M. P. Lungren, Radiology, 2020, 295, 4–15 CrossRef PubMed.
- S. L. Goldenberg, G. Nir and S. E. Salcudean, Nat. Rev. Urol., 2019, 16, 391–403 CrossRef PubMed.
- B. Acs, M. Rantalainen and J. Hartman, J. Intern. Med., 2020, 288, 62–81 CrossRef CAS PubMed.
- H. Hinneburg, F. Schirmeister, P. Korać and D. Kolarich, Methods Mol. Biol., 2017, 1503, 131–145 CrossRef CAS PubMed.
- H. M. Kayili and B. Salih, J. Proteomics, 2022, 267, 104700 CrossRef CAS PubMed.
- H. M. Kayili, Int. J. Mass Spectrom., 2020, 457, 116412 CrossRef CAS.
- B. C. Jansen, K. R. Reiding, A. Bondt, A. L. Hipgrave Ederveen, M. Palmblad, D. Falck and M. Wuhrer, J. Proteome Res., 2015, 14, 5088–5098 CrossRef CAS PubMed.
- D. Chicco and G. Jurman, BMC Genomics, 2020, 21, 6 CrossRef PubMed.
- M. E. Yaman, H. M. Kayili, M. Albayrak, Y. Kadioglu and B. Salih, Mol. Omics, 2021, 17, 394–404 RSC.
|
This journal is © The Royal Society of Chemistry 2023 |
Click here to see how this site uses Cookies. View our privacy policy here.