DOI:
10.1039/D3AN01096A
(Communication)
Analyst, 2023,
148, 4982-4986
Comparing MS imaging of lipids by WALDI and MALDI: two technologies for evaluating a common ground truth in MS imaging†
Received
30th June 2023
, Accepted 15th September 2023
First published on 15th September 2023
Abstract
In this study, we conducted a direct comparison of water-assisted laser desorption ionization (WALDI) and matrix-assisted laser desorption ionization (MALDI) mass spectrometry imaging, with MALDI serving as the benchmark for label-free molecular tissue analysis in biomedical research. Specifically, we investigated the lipidomic profiles of several biological samples and calculated the similarity of detected peaks and Pearson's correlation of spectral profile intensities between the two techniques. We show that, overall, MALDI MS and WALDI MS present very close lipidomic analyses and that the highest similarity is obtained for the norharmane MALDI matrix. Indeed, for norharmane in negative ion mode, the lipidomic spectra revealed 100% similarity of detected peaks and over 0.90 intensity correlation between both technologies for five samples. The MALDI-MSI positive ion lipid spectra displayed more than 83% similarity of detected peaks compared to those of WALDI-MSI. However, we observed a lower percentage (77%) of detected peaks when comparing WALDI-MSI with MALDI-MSI due to the rich WALDI-MSI lipid spectra. Despite this difference, the global lipidomic spectra showed high consistency between the two technologies, indicating that they are governed by similar processes. Thanks to this similarity, we can increase datasets by including data from both modalities to either co-train classification models or obtain cross-interrogation.
Mass spectrometry imaging (MSI) is a robust label-free molecular technique that enables the distribution of a diverse range of endogenous and exogenous molecules in biological tissues without compromising sample integrity or morphology.1 Due to years of instrumentation, sample preparation and bioinformatics advances, MSI has been developed widely and its range of applications broadened in the biomedical and pharmaceutical fields.2 In particular, due to a wide range of accessible analytes, matrix-assisted laser desorption ionization mass spectrometry imaging (MALDI-MSI) has demonstrated its vast potential for clinical applications including biomarker discovery, diagnostics, prognosis and patient stratification.3 While MALDI-MSI remains the most popular and democratized MSI technique, it requires sample preparation that is not trivial and time-consuming especially for proteins, peptides and glycans. Analysis of lipids, particularly glycerophospholipids (GPLs), present in tissues, has gradually gained importance in the course of the last 15 years and lipids are now recognized as important mediators in pathophysiological mechanisms.4 In this context, MALDI-MSI has quickly emerged as one of the universal tools for studying lipid biochemistry across tissue sections.5,6 Many lipids are readily detected due to their higher abundance in cells. The variations of lipid composition within tissues was already being studied in oncology7,8 and neurodegenerative diseases,9,10 among others. As the distribution and abundance of metabolites and lipids follow the changes of cell phenotypes, lipid MSI has gradually emerged as an alternative to histopathology.11,12 Several ambient ionization mass spectrometry (AIMS) technologies have become widespread for imaging samples under their near-native conditions since they require minimal sample preparation for analysis, unlike traditional vacuum-based MS approaches. The most commonly used techniques include desorption electrospray ionization (DESI),13 atmospheric pressure matrix-assisted laser desorption ionization (AP-MALDI),14,15 laser-ablation electrospray ionization (LAESI)16–18 or matrix-assisted laser desorption electrospray ionization (MALDESI).19
SpiderMass AIMS employs contactless micro-invasive analysis, using a laser desorption/ionization process in the mid-infrared range at 2.94 μm to excite the strongest vibrational bond of water molecules (O–H stretching).20 This enables a MALDI-like process, utilizing endogenous water as the MALDI matrix; hence the name water-assisted laser desorption ionization (WALDI).20,21 Additionally, the system is designed to enable remote analysis of desorbed materials, which is achieved through aspiration using a tubing line connected directly to the MS instrument that creates differential pressure. Like other AIMS technologies, SpiderMass provides direct analysis of metabolites and lipids, and has also been demonstrated on protein standards.22 The system has already been applied in different fields, including ex vivo analysis of glioblastoma3 and oral squamous cell carcinoma,23 and in vivo analysis of human skin20 as well as cultured cells without preparation.24 To enable MSI, the system was recently coupled to a robotic arm equipped with a distance sensor.25 This way, the WALDI-MSI can provide 2D as well as 3D molecular topographic images. Interestingly, because the laser microprobe is moved above the sample surface, both ex vivo imaging of flat tissue sections and in vivo imaging of human tissues are possible.25The molecular profiles from WALDI-MS reveal undeniable resemblance and similar characteristics to those observed during MALDI-MS processes. Similar collective behaviour to MALDI was previously observed in WALDI while tuning the laser wavelength to achieve resonant excitation.22 In this study, we aimed to compare WALDI-MSI and MALDI-MSI by calculating the similarity of detected peaks and correlation indexes of lipidomic profiles from several biological samples, including those from rat brain and human gastric cancer.
Individual assessment of peak similarity and intensity correlations of MALDI-MSI and WALDI-MSI lipidomic profiles
First, we evaluated the effectiveness of our newly developed correlation and similarity calculations (ESI†) by examining triplicates of MALDI and WALDI MSI analyses of rat brain (RB) tissues in both positive and negative ion modes. In both ion modes, 100% of the same peaks were detected in each replicate for MALDI (M) and WALDI (W) (ESI†). The intensity correlations were well above 94% with a coefficient of variance no higher than 3% between the MALDI data sets in both modes (ESI†). A slightly higher intensity correlation, above 97%, was obtained for WALDI-MSI triplicates with a coefficient of variance no higher than 1%. Regardless of different anatomical regions of the rat brain tissue sections, the techniques display high analytical and molecular reproducibility. The obtained results, therefore, highlight the robustness and the precision of our methods for further evaluation of the correlation and similarity between the two technologies.
Similarity of peak detection and correlation of intensities and variances between MALDI-MSI and WALDI-MSI
In WALDI, endogenous water plays the role of the matrix, but in MALDI, it is well known that a different family or subfamily of molecules are better detected by changing the matrix. Therefore, the experiments were carried out using the four most commonly utilized matrices for lipid MALDI MSI analysis, namely norharmane, 9-AA, 2,5-DHB, and DAN. The glycerophospholipid species are typically observed in the 500–1000 mass range with exceptions of lyso-glycerophospholipids. 2,5-DHB analysis was only performed in the positive ion mode and conversely 9-AA only in the negative ion mode, since these two matrixes are known to be more specific to one of the two modes.26,27 The norharmane matrix negative ion mode spectrum displayed high overlap with WALDI-MSI in the lipid 600–1000 m/z range (Fig. 1). This is further supported by the zoom and selected ion images at m/z 790.6 [PE (18:0_22:6)-H]−, m/z 885.6 [PI (18:0_20:4)-H]− and m/z 890.7 [SHexCer (d18:1/24:0)-H]− (Fig. 1a). pLSA was used to further elucidate the spectral signals resulting from a particular spatial feature within the two brain sections (Fig. 1b). The pLSA components 2, 3 and 5 outline only the major contributors to the grey and white matter by both modalities. The same 3 clusters are observed with bisecting k-means segmentation (ESI†). The ions at m/z 885.6, 888.6 [SHexCer (d18:1/24:1)-H]− and m/z 906.7 [SHexCer (42:1;3)-H]− display the highest contribution to each component. There was no dissimilarity of peaks detected and the intensity correlations were well above 91% and above 88% for variance (Fig. 1c and ESI†) with only 2 and 6% coefficient of variance between the triplicates. The 9-AA matrix revealed 84% peak similarity for MALDI vs. WALDI while only 48% peak similarity of WALDI compared to MALDI (ESI†). This is in line with the 9-AA matrix being conventionally used for metabolite imaging in the lower mass range. The DAN matrix showed 77% peak similarity and only 7% intensity correlation (ESI†).
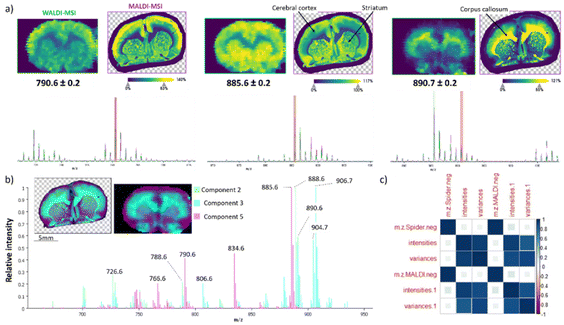 |
| Fig. 1 WALDI-MSI and MALDI-MSI (norharmane matrix) of M1 and W1 brain tissue in the negative ion mode. (a) Selected ion images and spectral zoom at m/z 790.6, 885.6 and 890.7. (b) Probabilistic latent semantic analysis (pLSA) loading plots and spectra of the resulting components in the negative ion mode (components 2, 3 and 5) for WALDI-MSI and MALDI-MSI. The loading spectra are annotated with the ions that show the highest contribution to each component. (c) Pearson's intensity correlation matrix for intensities and variances of the WALDI-MSI and MALDI-MSI data. | |
In positive ion mode, the WALDI-MSI spectrum shows strong visual differences compared to other MALDI matrices. It exhibits more diverse lipid content particularly in the 600–700 m/z range (ESI†). This is also confirmed by the similarity calculations with a low of 77% for norharmane, 51% for DHB and 75% for DAN (ESI†). The dissimilarity could be due to several additional diglycerides found in the WALDI-MSI spectrum. DGs are natively present in the tissue; however, the specific mass range can also indicate fragments from fatty acid neutral losses of PCs or TGs. To refute this assumption, a PC standard (18
:
1/18
:
1) was analysed by MALDI and by WALDI. The spectrum showed no fragmentation with both technologies (ESI†). It has already been demonstrated that DHB displays lower lipid coverage than norharmane28 and consequently has lower similarity index in rat brain samples. The sensitivity and the molecular coverage achievable by MALDI-MSI are ultimately governed by the ionization efficiencies (fraction of ions compared to the desorbed neutrals), which is relatively low and differs with each matrix. Despite this fact, the data clearly demonstrate that over 84.52% of all lipid peaks detected by MALDI-MSI are also detected by WALDI-MSI (ESI†) with norharmane being the most comparable matrix in both positive and negative ion modes.28,29
Effects of the laser spot size and tissue thickness on molecular profiles
So far, the two technologies cannot be performed with the same laser spot size with WALDI-MSI resolution limited to the incident laser beam diameter (∼400–500 μm). Thus, it could be speculated that the comparison between MALDI-MSI and WALDI-MSI may be affected by differences in the amount of analysed materials. To assess the impact of laser spot size on similarity and correlation calculations, MALDI-MSI analysis was conducted at a reduced spatial resolution of 150 μm. While there is 100% peak similarity, there is only a 2% difference in the intensity correlations between MALDI- and WALDI-MSI when MALDI data are collected at either 150 μm or 50 μm spatial resolution (ESI†). Similar findings are observed when comparing different thicknesses of the tissue. The peak similarity is equal and intensity correlations are above 92% between 12 μm and 20 μm tissue thicknesses (ESI†). According to this, the spatial resolution and tissue thickness have only a limited influence on the peak similarity and the correlation of intensities and variances between the two imaging modalities.
Comparative analysis and the interrogation model for gastric cancer
While rat brain tissue sections serve effectively as the standard for MALDI and AIMS MSI, we also wanted to confirm our hypothesis for other biological tissues, such as human gastric cancer. Building on the above, we exclusively used the norharmane matrix since it displayed the highest similarity to the WALDI-MSI analysis. Two heterogeneous tissue sections of poorly cohesive esogastric carcinoma (PCC) were used for analysis. The first tissue section is composed of healthy tissue with 10% tumor as indicated in the optical image (Fig. 2a). Visual comparison of the selected ion images (Fig. 2a) and spectra (Fig. 2c) shows high similarity between the WALDI- and MALDI-MSI. Specifically, ions at m/z 857.5 [PI (36:4)-H]−, m/z 863.6 [PI (18:1_18:0)-H]− and m/z 883.5 [PI (18:1_20:4)-H]− reveal not only the same distribution in the two subzones but also localized sub-features in the tissue. Indeed, the ion at m/z 863.6 is specific to the epithelium, in contrast to the ion at m/z 857.5, which is preferentially observed in mucosal tissue. The extracted overview spectra revealed a 100% peak similarity, 91% correlation of intensities and 75% correlation of variances (ESI†). Furthermore, exploration of the imaging data by ROC analysis (AUC above 0.85 thresholds) showed 2 discriminatory peaks in MALDI-MSI and 5 discriminatory peaks in WALDI-MSI. The peaks at m/z 766.5 [PE (18:0_20:4)-H]− and m/z 788.6 [PS (18:1_18:0)-H]− were only found with lower intensities in the WALDI data (ESI†). On the other hand, peaks at m/z 642.5 [HexCer 30:1;2-H]− and m/z 687.6 [PA (P-20:0_16:0) or (O-18:0_18:1)-H]− were not found in the MALDI-MSI data while those at m/z 701.5 [PA (18:1_18:0)-H]−, m/z 750.5 [PE (16:0_20:4)-H]− and m/z 853.6 [PI (36:6)-H]− were found but only with lower intensities (ESI†). The second-analysed gastric cancer tissue section shows important tumoral and peritumoral areas (Fig. 2b). Similarly, in the other tissue section, the selected ion distributions are uniform in both the WALDI and MALDI images (Fig. 2b). Indeed, ions at m/z 857.5 and m/z 863.6 are specific to the healthy tissue, in contrast to the ion at m/z 797.7, which is attributed to [PG (18:1_20:3)-H]− or [PG (18:0_20:4)-H]− and is only present in the cancerous tissue. The overview spectra, however, do reveal more pronounced differences in intensities (Fig. 2d). Although there is 100% similarity of the detected peaks, there is only 77% correlation of intensities and 67% correlation of variances (ESI†).
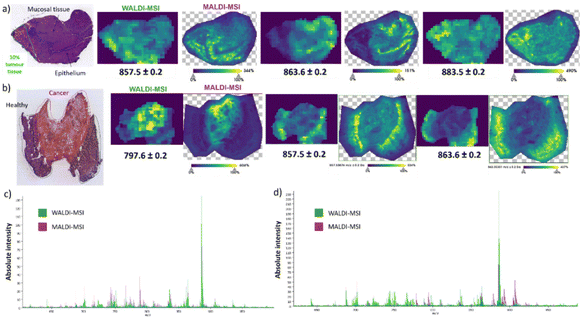 |
| Fig. 2 WALDI-MSI and MALDI-MSI (norharmane matrix) gastric cancer imaging data in the negative ion mode. (a) Optical image of the HPS-stained gastric tissue section A with the annotated cancer region, mucosal tissue and epithelium with the selected ion images at m/z 857.5, 863.6 and 883.5. (b) Optical image of the HPS-stained gastric tissue section B with the annotated cancer region and two healthy regions, accompanied to the right by the selected ion and spectral zoom images at m/z 797.7, 857.5 and 863.5. (c and d) WALDI-MSI (green) and MALDI-MSI (purple) overview spectra of (c) gastric tissue section A and (d) gastric tissue section B. | |
Finally, we assessed whether the high similarity enables one to train with MALDI data and then interrogate the WALDI data against the MALDI training. For this purpose, a two-class CNN model was built with MALDI-MSI data that is able to distinguish the cancerous tissue and the healthy tissue of gastric tissue section B (ESI†). Then, thanks to this, the label of 30 random samples from WALDI-MSI data was predicted. As shown in Fig. 3, we obtained good classification with 93% prediction of cancerous tissue with 81% mean accuracy and 80% prediction of healthy tissue with a mean accuracy of 80%. This confirmed that MALDI-MSI and WALDI-MSI data show the same ground truth in MS imaging and that WALDI can be trained from MALDI data.
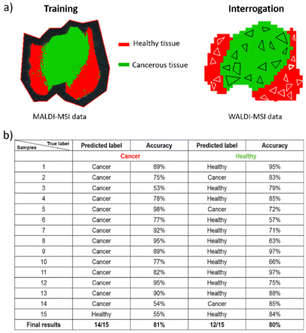 |
| Fig. 3 CNN model based on MALDI-MSI data with WALDI-MSI data for the prediction of cancerous and healthy tissues from gastric tissue section B. (a) Regions of interest based on segmentation used for the training (MALDI-MSI data) and the interrogation (WALDI-MSI data) of the model. (b) Predicted labels and their accuracies obtained for 30 random samples from WALDI-MSI data (black and white triangles). | |
In summary, we have demonstrated high resemblance between the newly developed WALDI-MSI and MALDI-MSI for lipidomic analysis. Our findings show not only the detection of the same species but also a strong correlation between the spectral intensities obtained through both modalities. This correlation is particularly notable in the negative ion mode and when using norharmane25,26 as the MALDI matrix. Despite differences in resolution between the two techniques, we were able to identify the same tissue heterogeneity. In gastric cancer, it was possible to differentiate not only the tumoral and peritumoral regions but also the dense cancer cells, epithelium, and mucosal tissue. In the future, we intend to further increase the spatial resolution to 100 μm through mechanical improvements. Our data clearly demonstrate that the WALDI-MSI technique is a promising new ambient ionization imaging method. Moving forward, we intend to leverage MALDI-MSI data to expedite and enhance WALDI-MSI training, particularly in the context of data intensive supervised machine learning.
Author contributions
L.L., I.F., N.O. and Y.Z. wrote the manuscript; I.F. and N.O. designed the experiments. L.L. and Y.Z. performed the experiments. L.L. and Y.Z. analyzed the data. Y.Z. built the similarity and correlation code. F.R. provided the gastric cancer samples and HPS annotations. L.L., Y.Z., I.F., N.O., M.S. and L.D. corrected the manuscript. I.F. and N.O. supervised the project. I.F. and M.S. provided the funding.
Conflicts of interest
There are no conflicts to declare.
Acknowledgements
The project was supported by the Ministère de l'Enseignement Supérieur et de la Recherche (I.F.), Inserm (I.F.) and Excellence Initiative ISite ERC Generator (I.F.). The authors would like to thank the Centre for Studies and Research on Lasers and Applications (CERLA) for support.
References
- A. R. Buchberger, K. DeLaney, J. Johnson and L. Li, Anal. Chem., 2018, 90, 240–265 CrossRef CAS PubMed.
- P.-M. Vaysse, R. M. A. Heeren, T. Porta and B. Balluff, Analyst, 2017, 142, 2690–2712 RSC.
- M. Duhamel, L. Drelich, M. Wisztorski, S. Aboulouard, J.-P. Gimeno, N. Ogrinc, P. Devos, T. Cardon, M. Weller, F. Escande, F. Zairi, C.-A. Maurage, É. Le Rhun, I. Fournier and M. Salzet, Nat. Commun., 2022, 13, 6665 CrossRef CAS PubMed.
- X. Han, Nat. Rev. Endocrinol., 2016, 12, 668–679 CrossRef CAS PubMed.
- R. C. Murphy, J. A. Hankin and R. M. Barkley, J. Lipid Res., 2009, 50(Suppl), S317–S322 CrossRef PubMed.
- A. P. Bowman, R. M. A. Heeren and S. R. Ellis, TrAC, Trends Anal. Chem., 2019, 120, 115197 CrossRef.
- S. Guo, Y. Wang, D. Zhou and Z. Li, Sci. Rep., 2014, 4, 5959 CrossRef CAS PubMed.
- M. K. Andersen, T. S. Høiem, B. S. R. Claes, B. Balluff, M. Martin-Lorenzo, E. Richardsen, S. Krossa, H. Bertilsson, R. M. A. Heeren, M. B. Rye, G. F. Giskeødegård, T. F. Bathen and M.-B. Tessem, Cancer Metab., 2021, 9, 9 CrossRef PubMed.
- K. Mallah, J. Quanico, A. Raffo-Romero, T. Cardon, S. Aboulouard, D. Devos, F. Kobeissy, K. Zibara, M. Salzet and I. Fournier, Anal. Chem., 2019, 91, 11879–11887 CrossRef CAS PubMed.
- I. A. Mulder, N. O. Potočnik, L. a. M. Broos, A. Prop, M. J. H. Wermer, R. M. A. Heeren and van den A. M. J. M. Maagdenberg, Sci. Rep., 2019, 9, 1–10 CrossRef CAS PubMed.
- R. Longuespée, R. Casadonte, M. Kriegsmann, C. Pottier, de G. P. Muller, P. Delvenne, J. Kriegsmann and E. D. Pauw, Proteomics: Clin. Appl., 2016, 10, 701–719 Search PubMed.
- M. Holzlechner, E. Eugenin and B. Prideaux, Cancer Rep., 2019, 2, e1229 CrossRef PubMed.
- Z. Takáts, J. M. Wiseman, B. Gologan and R. G. Cooks, Science, 2004, 306, 471–473 CrossRef PubMed.
- V. V. Laiko, N. I. Taranenko, V. D. Berkout, M. A. Yakshin, C. R. Prasad, H. S. Lee and V. M. Doroshenko, J. Am. Soc. Mass Spectrom., 2002, 13, 354–361 CrossRef CAS PubMed.
- V. V. Laiko, M. A. Baldwin and A. L. Burlingame, Anal. Chem., 2000, 72, 652–657 CrossRef CAS PubMed.
- P. Nemes and A. Vertes, J. Visualized Exp., 2010, e2097 Search PubMed.
- P. Nemes and A. Vertes, Methods Mol. Biol., 2010, 656, 159–171 CrossRef CAS PubMed.
- P. Nemes, A. S. Woods and A. Vertes, Anal. Chem., 2010, 82, 982–988 CrossRef CAS PubMed.
- J. S. Sampson, A. M. Hawkridge and D. C. Muddiman, J. Am. Soc. Mass Spectrom., 2006, 17, 1712–1716 CrossRef CAS PubMed.
- B. Fatou, P. Saudemont, E. Leblanc, D. Vinatier, V. Mesdag, M. Wisztorski, C. Focsa, M. Salzet, M. Ziskind and I. Fournier, Sci. Rep., 2016, 6, 25919 CrossRef CAS PubMed.
- N. Ogrinc, P. Saudemont, J. Balog, Y.-M. Robin, J.-P. Gimeno, Q. Pascal, D. Tierny, Z. Takats, M. Salzet and I. Fournier, Nat. Protoc., 2019, 14, 3162–3182 CrossRef CAS PubMed.
- B. Fatou, M. Ziskind, P. Saudemont, J. Quanico, C. Focsa, M. Salzet and I. Fournier, Mol. Cell. Proteomics, 2018, 17, 1637–1649 CrossRef CAS PubMed.
- N. Ogrinc, C. Attencourt, E. Colin, A. Boudahi, R. Tebbakha, M. Salzet, S. Testelin, S. Dakpé and I. Fournier, Front. Oral Health, 2022, 3, 827360 CrossRef PubMed.
- P. Saudemont, J. Quanico, Y.-M. Robin, A. Baud, J. Balog, B. Fatou, D. Tierny, Q. Pascal, K. Minier, M. Pottier, C. Focsa, M. Ziskind, Z. Takats, M. Salzet and I. Fournier, Cancer Cell, 2018, 34, 840–851 CrossRef CAS PubMed.
- N. Ogrinc, A. Kruszewski, P. Chaillou, P. Saudemont, C. Lagadec, M. Salzet, C. Duriez and I. Fournier, Anal. Chem., 2021, 93, 14383–14391 CrossRef CAS PubMed.
- W. J. Perry, N. H. Patterson, B. M. Prentice, E. K. Neumann, R. M. Caprioli and J. M. Spraggins, J. Mass Spectrom., 2020, 55, e4491 CrossRef CAS PubMed.
- M. R. Eveque-Mourroux, P. J. Emans, R. R. M. Zautsen, A. Boonen, R. M. A. Heeren and B. Cillero-Pastor, Analyst, 2019, 144, 5953–5958 RSC.
- T. B. Angerer, J. Bour, J.-L. Biagi, E. Moskovets and G. Frache, J. Am. Soc. Mass Spectrom., 2022, 33, 760–771 CrossRef CAS PubMed.
- S. R. Ellis, J. Cappell, N. O. Potočnik, B. Balluff, J. Hamaide, A. Van der Linden and R. M. A. Heeren, Analyst, 2016, 141, 3832–3841 RSC.
|
This journal is © The Royal Society of Chemistry 2023 |
Click here to see how this site uses Cookies. View our privacy policy here.