Trends in atmospheric pollution in the Third Pole: analyses of tropospheric NO2 for the period 2005–2020†
Received
23rd June 2022
, Accepted 19th March 2023
First published on 20th March 2023
Abstract
The Third Pole, encompassing Hindu Kush Himalaya (HKH) and Tien Shan mountains, has been closely monitored for the past few decades because of its deteriorating environmental conditions. Here, we analyse the spatio-temporal changes in tropospheric NO2 over the TP using satellite observations from 2005 to 2020. The highest NO2 concentrations (i.e. ≥ 1 × 1015 molec. cm−2) are found at the boundaries close to the Indo-Gangetic Plain (IGP) and Yellow and Yangtze River basins (YYRB). The analysis of the Emissions Database for Global Atmospheric Research (EDGAR v6.1) shows that the main contribution to NO2 in the region is from road transport (81%) and then the power sector (7%). Hybrid Single Particle Lagrangian Integrated Trajectory (HYSPLIT) analyses illustrate that the major regions from which air mass reaches the TP are the IGP, Southeast Asia, YYRB, Central Asia and Middle East. Our analysis reveals a positive trend in NO2 over most regions of the TP (up to 0.05 ± 0.01 × 1015 molec. cm−2 year−1) in the yearly averaged data for the period 2005–2020, which suggest that pollution is spreading even to the inner regions of the TP. Therefore, this study reveals that the inner TP, one of the most pristine regions on Earth, is getting polluted because of high anthropogenic activities within and nearby areas/cities, indicating the impact of regional development activities and socioeconomic changes in recent years.
Environmental significance
The Third Pole (TP) is one of the remote and pristine regions on Earth. Therefore, any change in the environment would be readily visible, signalling changes in the region. Here, we examine the tropospheric NO2 column to assess the evolution of atmospheric pollution during the period 2005–2020 in the TP. We find an increasing trend of NO2 in the period, suggesting a declining air quality owing to recent development activities in and around the TP. Henceforth, this study unveils the atmospheric composition change in one of the pristine regions on Earth, which is a serious concern.
|
1. Introduction
The oxides of nitrogen (i.e. NOx = NO2 + NO) in the atmosphere affect human health, the ecosystem and climate.1,2 Combustion, lightning and microbial processes are the major sources of NOx,3–5 but the emission rate of microbial processes is mostly temperature dependent.6 Wet deposition and photolysis are the processes that act as sinks of NO2 in the atmosphere.3,7 NOx plays an important role in the formation of secondary aerosols, particulate matter (PM) and different trace gases including surface ozone and indirectly affect the regional and global climate.8,9
The Third Pole (TP) is one of the remote and pristine regions on Earth that encompasses the Hindukush Himalaya (HKH) and Tien Shan Mountains.10,11 This region has complex mountainous topography and has sources of freshwater for almost 2 billion people in the downstream of the TP.12 Past studies show that air quality is continuously declining in the TP due to pollution from within and boundary regions of the TP, including the inflow from the Yellow and Yangtze River basins (YYRB) in the east and the Indo-Gangetic Plain (IGP) in the south.9,13 Transboundary transport from other regions of Asia also contributes significantly to air pollution in the TP.14,15 The economic growth of neighbouring nations has impacted the air quality in the TP through atmospheric transport and thus, it is particularly sensitive to regional socioeconomic changes.16 In addition, the TP houses thousands of lakes and glaciers with a total area of more than 10
000 sq. km.12 The majority of the lakes and glaciers in the TP are found in isolated areas without inhabitants, making them particularly vulnerable to climate change.
Tropospheric NO2 over the TP is dependent on local emissions, meteorological conditions and seasonal cycles. The strong increase in NO2 over the eastern boundary of the TP is likely to be associated with an increase in industrial development, followed by traffic and residential sources there.17 A positive trend in NO2 is found in the densely populated regions such as Urumqi (0.15 ± 0.04 × 1015 molec. cm−2 year−1) from 1996 to 2005.18 Ghude et al.19 found a positive trend of 1.76 ± 1.1% year−1 in south Asia for the period 1996–2006, which includes India, Pakistan, Bangladesh and Nepal. Lu et al.20 pointed out that the increase of NO2 in India is mainly associated with coal-fired power plants, followed by industries and traffic emissions, as assessed from the measurements made between 2004 and 2015. Ramachandran et al.21 found a significantly positive trend in India based on NO2 measurements from SCIAMACHY on-board Envisat in 2002–2012. For instance, Delhi and the eastern mining regions of India show a linear trend of 3.24 ± 1.22 molec. cm−2 year−1 and 7.27 ± 1.27 molec. cm−2 year−1, respectively, from 2002 to 2012. Positive trends in NO2 with 44.1, 23.4, 31.4 and 32.3% year−1 are also found in the densely populated cities of Islamabad, Lahore, Dera Ghazi Khan and Karachi, respectively.22 However, there is evidence of decrease in NO2 in some regions of China after 2012.23 One major goal of the 12th Five-Year Plan of China during the period 2011–2015 was to reduce NOx emissions24 and the associated positive change is also reflected in recent assessments.25
Due to the importance of the TP environment, it is essential to continuously observe NO2 over this region. However, because of the complex topography, a wide ground-based monitoring network is difficult in this region. Conversely, the spectral absorption of NO2 in the visible and near-ultraviolet range (300–700 nm) allows its global measurements from satellites. There are several space-based observations for tropospheric NO2. The first space-based NO2 observation started in the mid-1990s with the Global Ozone Monitoring Experiment (GOME: 1995–2011).26 Similar measurements with a better spatial resolution are continued with the Scanning Imaging Absorption Spectrometer for Atmospheric Cartography (SCIAMACHY: 2002–2012),27 Ozone Monitoring Instrument (OMI: 2004–2015),28 Global Ozone Monitoring Experiment-2 (GOME-2: 2006–to date)29 and Tropospheric and Monitoring Instrument (TROPOMI: 2017–to date).30 Among them, TROPOMI has better spatial resolution and OMI has the longest measurement record.31 However, previous studies on NO2 were limited to the urban regions of the TP and its adjacent boundary. Therefore, to assess the changing environment of the TP, we examine the spatio-temporal features of NO2 over the entire HKH and TSM regions from 2005 to 2020, using the merged observations of OMI and GOME-2B. Furthermore, to know the direct impact of anthropogenic sources of NO2 over the region, the trend analysis of NO2 is carried out over 20 cities in and around the region. The cities are Kabul, Peshawar, Khuzdar, Srinagar, Dehradun, Shillong, Silchar, Agartala, Kathmandu, Chittagong, Thimphu, Naypyitaw, Xining, Lhasa, Kunming, Urumqi, Dushanbe, Almaty, Tashkent, and Bishkek from 12 different countries: Afghanistan, Pakistan, India, Nepal, Bangladesh, Bhutan, Myanmar, China, Kazakhstan, Kyrgyzstan, Uzbekistan, and Tajikistan (see Fig. 1b). Therefore, this is the first comprehensive study that deals with NO2 pollution for the entire TP using measurements made in the past two decades. This type of long-term study is expected to help policymakers and scientists to better comprehend pollution sources and their linkage with regional climate change, which would first appear in remote and extreme places like the TP.
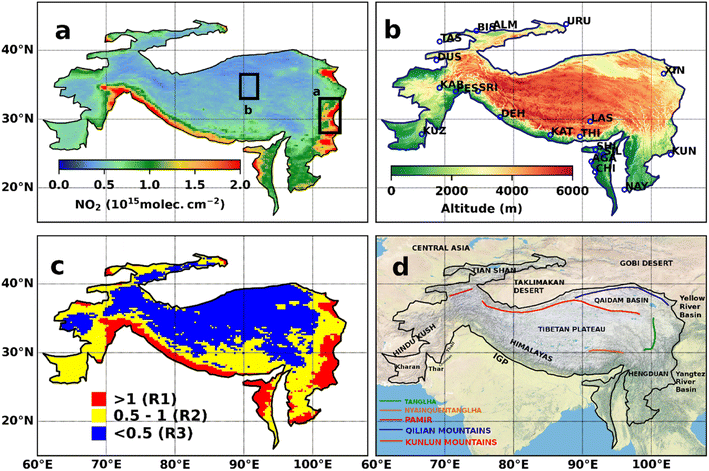 |
| Fig. 1 (a) Mean (2005–2020) tropospheric NO2 column (in 1015 molec. cm−2) in the TP. Box a represents the most polluted region and box b represents a pristine region in the TP. (b) Topography of the study region with selected cities marked in circles. (c) Mean (2005–2020) tropospheric NO2 over the TP with three distinct ranges of NO2: regions with NO2 less than 0.5 × 1015 molec. cm−2 (blue colour), between 0.5 and 1 × 1015 molec. cm−2 (yellow colour), and greater than 1 × 1015 molec. cm−2 (red colour). (d) The geographic map of the TP and adjacent regions (no country borders marked). | |
2. Data and methods
We have used NO2 observations of OMI on-board Aura and GOME-2B on-board Metop-B from November 2004 to December 2014 and from January 2013 to March 2021, respectively. Both OMI and GOME-2B are nadir viewing instruments, which measure backscattered radiation at a spatial resolution of 13 × 24 km2 and 80 × 40 km2, respectively.32 OMI provides daily global measurements whereas GOME-2B has a default swath width of 1920 km, allowing for global coverage within 1.5–3 days at the Equator.33 The OMI NO2 measurements are made in 3 channels between 264 and 504 nm and GOME-2B in 4 bands between 240 and 790 nm.34 We have merged OMI (2004–2014) and GOME-2B (2013–2021) data using a modified bias correction method by finding the cumulative distribution of data for the overlapping period (Fig. S1†) to minimize bias in the older instrument using measurements from the new instrument, as described by Bai et al.,35 to get long-term continuous data from 2004 to 2021. The difference in the overlapping period of OMI and GOME-2B measurements is within ±0.5 × 1015 molec. cm−2. Also, there are regions with larger differences (0.5 × 1015 molec. cm−2 in the east of 100° E and west of 75° E (Fig. S1,† bottom). OMI is affected by a loss of spatial coverage after 2014, commonly referred to as row-anomaly, which is about 5–35% of the total pixels captured by the instrument.36,37 Therefore, we consider GOME-2B observations after 2014 for better accuracy.
To understand the seasonal distribution of biomass and agricultural waste burning, we use fire count data from MODIS Terra at a spatial resolution of 1 km. Furthermore, we use ERA5 reanalysis for 10 m winds for the illustration of seasonal winds over the TP.38 Here, the seasons are defined as winter (December–January–February, DJF), spring (March–April–May, MAM), summer (June–July–August, JJA) and autumn (September–October–November, SON).
We have used the Emissions Database from Global Atmospheric Research (EDGAR v6.1), a bottom-up inventory that estimates emissions for a number of trace gases and aerosols, including NO2. It has a spatial resolution of 0.1° × 0.1° and covers a period from 1970 to 2018. The activity data and emission factors are used to determine emissions from each source and country per annum.39
We have used the HYSPLIT Lagrangian transport model for making air mass trajectories. The backward trajectories provide a path of air parcels in the chosen period to identify the potential source regions and transport pathways of pollutants. Studies show that the average lifetime of NOx estimated for Asia, including India, China and Indonesia, is between 15 and 47 hours, and is highly seasonal and temperature dependent.40 Therefore, we have computed three dimensional 3 day backward trajectories at 500 m above the ground at three distinct receptor sites, each of which represents a distinct region: 27° N, 102° E (S1, highly polluted region), 33.5° N, 88° E (S2, less polluted region) and 36.2° N, 67° E (S3, moderately polluted region) from the east, central and west TP, respectively, to analyse the seasonal variation of air mass transport to the TP by using meteorological input from the Global Data Assimilation System (GDAS) at 1° × 1° resolution. The GDAS meteorological data have been widely used for air mass trajectory calculations.41 The height 500 m is chosen to diminish the effect of friction and to represent the winds in the lower troposphere.42 Trajectories were then clustered using the Total Spatial Variance method.43 Furthermore, we have also estimated the integration error by computing forward and backward trajectories from each receptor site and found an uncertainty of about 171.4, 200.2 and 159 km, respectively, which are half the distance between end and starting points at each receptor site.
3. Results and discussion
3.1 Spatial distribution of tropospheric NO2 over the TP
Fig. 1a shows the long-term mean (2005–2020) of tropospheric NO2 over the TP (10°–45° N and 60°–105° E) derived from the merged OMI and GOME-2B data. Fig. 1b shows the topography of the TP, including 20 selected cities in and around the region. The boundaries of the TP within and close to the IGP in the south and the YYRB in the eastern TP have low-lying (i.e. elevation below 2000 m from the mean sea level) densely populated and economically dynamic regions.22,44 In comparison to other regions of the TP, these city regions are highly populated and therefore, are characterised by higher concentrations of NO2 (>1 × 1015 molec. cm−2). On the other hand, the inland TP is surrounded by high mountains: the Pamir and Hindukush in the west, the Himalaya in the south (stretching across the countries of Pakistan, India, Nepal, Bhutan and China), the Nyainquentanglha and Handgun mountains in the southeast, the Tengla mountain in the east, and the Kunlun and Qilian Mountains in the north (Fig. 1d), which act as natural barriers that block air mass inflow to the TP from the surrounding regions of the IGP, YYRB, Middle East, Central Asia, and Taklimakan and Gobi deserts. This complex geographical structure of boundary regions of the TP and long dry season are favourable for the accumulation of air pollutants in the lower basins. However, these pollutants can reach the inland TP under the influence of atmospheric transport.
The vertical transport of NO2 is highly dependent on the lifetime and chemistry of NO2 in the atmosphere. However, these high-altitude regions (i.e. >4000 m from the mean sea level) are the most pristine regions with near-zero tropospheric NO2 (<0.5 × 1015 molec. cm−2) and no anthropogenic activity. Therefore, the sparsely populated hilly regions (with altitude <4000 m), including the northwest TP around the Taklimakan desert, have NO2 less than 0.5 × 1015 molec. cm−2. Also, the less-populated low-lying regions close to Thar, Cholistan, Thal and Kharan in the southwest TP have moderate levels of NO2, within 0.5–1 × 1015 molec. cm−2. Lower pollution in this region could be due to less anthropogenic activities together with small population owing to the complex terrain. Henceforth, for the assessment of spatial and temporal changes in tropospheric NO2, the study area is divided into three regions based on the mean distribution of NO2 over the TP, specifically high (>1 × 1015 molec. cm−2), moderate (0.5–1 × 1015 molec. cm−2) and low (<0.5 × 1015 molec. cm−2) concentrations referred to as R1, R2 and R3, respectively, as shown in Fig. 1c.
3.2 Seasonal variation in NO2
Fig. 2 shows the seasonal distribution of tropospheric NO2 over the TP for the period 2005–2020, which are overlaid with the winds. The seasonal variation of tropospheric NO2 is primarily associated with the changes in meteorological conditions, including winds, humidity and temperature. Therefore, these meteorological parameters can highly impact the emissions, evolution and long-range transport of pollutants. The climate of the TP is primarily affected by the Asian monsoon in summer and westerlies in other seasons, and thus the transport of pollutants. The eastern boundary of the TP close to the vicinity of the YYRB shows comparatively higher tropospheric NO2 (>2 × 1015 molec. cm−2) in DJF, but lower during JJA (1.25–2 × 1015 molec. cm−2). In contrast, the highest concentrations of NO2 are observed during MAM (1.25–2 × 1015 molec. cm−2) and the lowest during SON (1–1.75 × 1015 molec. cm−2) over the boundary regions of the TP close to the IGP. Higher NO2 near the IGP and YYRB is primarily due to anthropogenic activities throughout the year.45 However, the increase in NO2 during MAM over the southern boundaries of the TP near the IGP is due to the enhanced biomass burning associated with agriculture. The stubble burning during the wheat-rice rotation period is among the prominent reasons for a seasonal increase in NO2 in this region.4,46,47 Major stubble burning occurs during January–May in Lahore, Faisalabad, Dera Ghazi Khan, Narowal and Hafizabad in Pakistan, Punjab, Haryana, Himachal Pradesh, Uttarakhand, Uttar Pradesh and Bihar in India, Nepal and Myanmar.44,48 The fire count data also reveal an increase in the number of fires, particularly during MAM when compared to other seasons (Fig. S3†). Furthermore, the meteorological conditions such as low humidity, low wind speed, low temperature and geographical features of the IGP support the concentration of air pollutants in this region.49
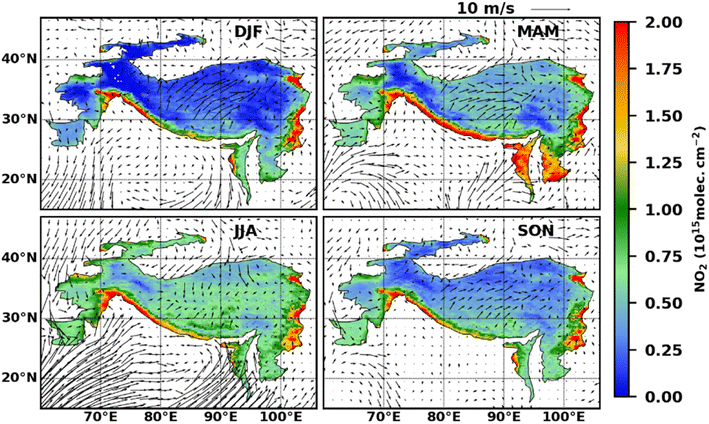 |
| Fig. 2 Seasonal changes in the tropospheric NO2 column in the TP. The seasons are defined as winter (December–January–February, DJF), spring (March–April–May, MAM), summer (June–July–August, JJA) and autumn (September–October–November, SON). The corresponding seasonal wind vectors are overlaid. | |
In addition, higher NO2 during MAM over the south-east regions of the TP, including northeast India, Myanmar, Bhutan and Bangladesh, is primarily due to the wildfires in this dry season.48 The YYRB region is one of the densely populated and industrialised regions in the world,13 and thus, anthropogenic emissions are relatively higher throughout the year, with very small seasonal variation (>1.5 × 1015 molec. cm−2). However, coal-fired heating contributes significantly to NO2 in this region during DJF.45 Additionally, seasonal analysis shows high variation throughout the year (increases from 0.2–1 × 1015 molec. cm−2 from winter to summer). This difference in the seasonal cycle is related to various sources of NOx in different regions. Van der A et al.18 suggested that the major sources of NOx over the high altitude TP are natural (soil and lightning) emissions and are highly dependent on changes in temperature, soil and precipitation.
3.2.1 Air mass transport to the TP.
The TP region is surrounded by high mountains, which are natural barriers that can impede pollutants reaching the main body of the TP. Nevertheless, the valleys in these mountainous regions serve as channels that drive pollutants towards the inner regions of the TP. To identify the air mass transport towards the TP from different regions, we run three-day backward trajectories at three receptor sites S1, S2 and S3 for the year 2018, representing three regions in the east, central and west TP from R1, R2 and R3, respectively (Fig. 3). The trajectories are computed for each season and calculated every 6 hours at 500 m above each site using the HYSPLIT model. The air mass transport is further analysed by clustering the trajectories to four groups based on the percentage of transport towards these sites.
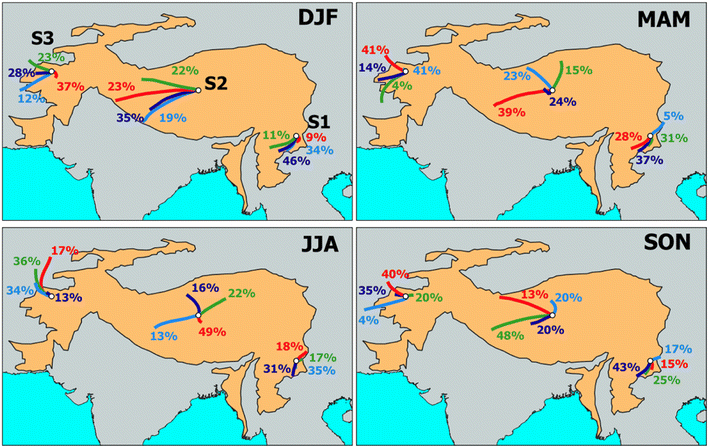 |
| Fig. 3 Three-day backward air-mass trajectory clusters at three receptor sites S1 (27° N, 102° E), S2 (33.5° N, 88° E) and S3 (36.2° N, 67° E) for the year 2018 in the TP. The seasons are defined as winter (December–January–February, DJF), spring (March–April–May, MAM), summer (June–July–August, JJA) and autumn (September–October–November, SON). | |
We observe that about 75–80% trajectories arrive at S3 are from west in all seasons, reflecting that the region is westerly dominated and the major air mass transport towards the TP is mainly from Central Asia and Middle East. However, local source contribution is also significant, about 35–40% in DJF and MAM and about 10–20% in JJA and SON. Likewise, about 75–80% trajectories at S2 in the central TP are from the west and northwest during SON, DJF and MAM, which suggests that the westerly effect is dominant there. However, during JJA the trajectories arrive at S2 are mostly from South, Southwest and Southeast (about 83%) and have their origin within the TP. This points out that the South Asian Summer Monsoon (SASM) dominates the air flow during JJA in this region. Conversely, about 55–60% trajectories reach S1 from the west and southwest during SON, DJF and MAM. The trajectories that reach S1 are mostly influenced by the southern branch of westerlies in these seasons, indicating the impact of westerlies in the southeast TP. However, in JJA, all trajectories reaching S1 are from south and southeast, which clearly shows that SASM dominates the air flow in the southeast TP, but the trajectories are relatively short and not clearly distinct due to higher humidity in the atmosphere.
Our results from the cluster analysis and the seasonal distribution of NO2 show that pollutants reaching the TP are mostly from its boundary regions. For example, the western TP is affected by the pollution from central Asia, the eastern TP from Southeast Asia and the southern TP from the IGP. In addition to this, the winds transport NO2 from the east, particularly from the YYRB to the inner TP and a significant amount of NO2 (0.75–1.25 × 1015 molec. cm−2) gets concentrated in the northern foothills of the Himalaya during JJA, which is more than 1.5–2 times the amount of NO2 (0.5–0.75 × 1015 molec. cm−2) present in other seasons. Similarly, the pass in mountain chains also channels the air mass towards the mainland of the TP during JJA, which is not prominent in other seasons.
3.3 Temporal variation of NO2
Fig. 4 (top) shows the time series of monthly averaged NO2 over the three regions (R1, R2 and R3) in the TP. At R1, the peak concentration of NO2 slowly decreased from 1.9 to 1.6 × 1015 molec. cm−2 during 2005–2009, which can be linked to the global economic recession and in the countries neighbouring the TP. The recession significantly reduced the amount of traffic and goods transport, which further decreased the anthropogenic activities and thus, the emissions.50 However, there are no significant changes during this period over R2 and R3. Subsequently, we notice a steady increase in NO2 from 2010 to 2012, with a peak of about 2.2–2.4 × 1015 molec. cm−2 in R1. Following this, there is a gradual decline in NO2 (2.4–1.6 × 1015 molec. cm−2) during 2012–2018, which may be due to the implementation of several policies for regulating air pollution in the neighbouring countries (China, India, Bhutan, Pakistan and Nepal) of the TP. These policies include the State council air pollution prevention and control action plan (CAAC)51 in China, National Environment Protection Act of Bhutan (NEPAB);52 Pakistan Environmental Protection Act (PEPA),53 and Pollution Control Acts, Rules and Notifications54 in India. Thenceforth, we also find an abrupt increase in NO2 in R1 during 2018–2020, which is about 2 times (1.6–3 × 1015 molec. cm−2) the amount of NO2 in 2018. The dramatic change in NO2 may be closely linked to the rapid development in the transportation sector in the Tibetan region. Over the past five years, an average of about 7500 km new highways were built every year there, which connects this region with other major cities of China (TARGWR).55 Additionally, there is a continuous expansion of the railway network in the TP region.56 Recently, the road transport and railway tracks were extended to more regions, e.g. Lhasa–Xigaze (completed in 2015) and Lhasa–Nyingchi (completed in 2020), and are opened for public and commercial services.57 However, these development activities dramatically changed the TP environment, as also reported in Cui and Graf.58 In R2, a consistent annual variation in NO2 is found from 2004 to 2012, but a slight decline in 2014, from 0.8 to 0.6 × 1015 molec. cm−2. Nevertheless, an abrupt change in NO2 from 0.6 to 2 × 1015 molec. cm−2 is found during 2018–2020. A similar interannual variability with a sudden rise from 2018 is also observed in R3. A rapid rise in NO2 from 2018 onwards shows the impact of anthropogenic emissions reaching the inner regions of the TP.
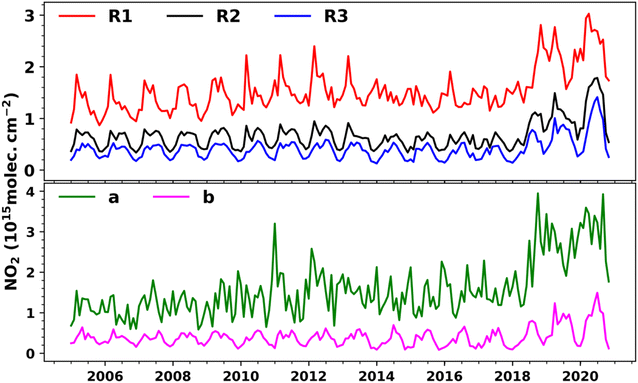 |
| Fig. 4 (Top) Temporal evolution of tropospheric NO2 over three distinct NO2 regions. (Bottom) Tropospheric NO2 in two selected regions a and b for the period from January 2005 to November 2020. R1, R2, and R3 are the regions of different NO2 concentration levels, and a and b are the sub-regions in the TP with high pollution and the pristine environment, respectively (see the text and Fig. 1 for details). | |
We have also examined the changes in monthly mean NO2 over two sub-regions a and b (see Fig. 1a). Fig. 4 (bottom) shows the monthly averaged tropospheric NO2 over these sub-regions from 2005 to 2020. Sub-region ‘a’ is highly polluted, whereas ‘b’ is less polluted. In region-b, a consistent temporal variation in NO2 is found from 2004 to 2017. After 2018, we find a sharp increase of about 0.5–1.2 × 1015 molec. cm−2 in NO2, which indicates the spread of pollution even to the inner TP. On the other hand, in region-a, NO2 gradually increased from 2004 to 2010 with a sharp peak of about 3.5 × 1015 molec. cm−2 in 2011, but gradually decreased to 2 × 1015 molec. cm−2 by 2018. The NO2 concentrations show a significant increase thereafter to 1.5–4 × 1015 molec. cm−2 in 2020. Liu et al.59 found that NO2 concentration in China peaked to 5.8 × 1015 molec. cm−2 in 2011 and then decreased by 32% from 2011 to 2015, though the Chinese economy kept growing after 2012.60 The change in NO2 distribution over the TP shows the positive effect of national pollution control and the industrial transformation policy of China. For instance, the industrial transformation processes in China evolved in two phases. The first phase was during 1970–1980, when international industries were transferred from the western developed countries to the eastern coastal areas of China. Since 2000, in the second phase, industries were relocated from coastal to Central and Western regions of China.61 Apart from these, the relocation of industries within provinces and inter-provinces has also occurred during the second phase.62,63
3.4 Trends in the tropospheric NO2 column
Fig. 5 shows the trend (2005–2020) of NO2 in the TP. Small positive trends (up to 0.1 × 1015 molec. cm−2 year−1) are observed in most regions. Comparatively higher trends (i.e. ≥0.1 × 1015 molec. cm−2 year−1) are found over the eastern boundaries of the TP around Qinghai and Sichuan provinces that connect the east, central, west and south Asia through roadways. We also find higher trends in NO2 (i.e., >0.05 × 1015 molec. cm−2 year−1) near the IGP around Kabul, Peshawar, Srinagar, Punjab, Haryana, Himachal Pradesh and Uttarakhand. In contrast, the highest region-wise annual trend is found over R1 (0.07 ± 0.01 × 1015 molec. cm−2 year−1) and smallest over R3 (0.01 ± 0.01 × 1015 molec. cm−2 year−1). We also find positive trends in all regions of the TP in the annual and seasonal data. In regions a and b, the highest positive trend is found in MAM (0.24 ± 0.01 × 1015 molec. cm−2 year−1) and SON (0.1 ± 0.01 × 1015 molec. cm−2 year−1). In a similar study, Rupakheti et al.47 found high positive trends of NO2 in the IGP (0.052 × 1015 molec. cm−2 year−1 in winter) and Himalaya (0.035 × 1015 molec. cm−2 year−1) in the pre-monsoon season, and the Tibetan Plateau (0.016 × 1015 molec. cm−2 year−1) in summer using the OMI measurements for the period 2004–2015.
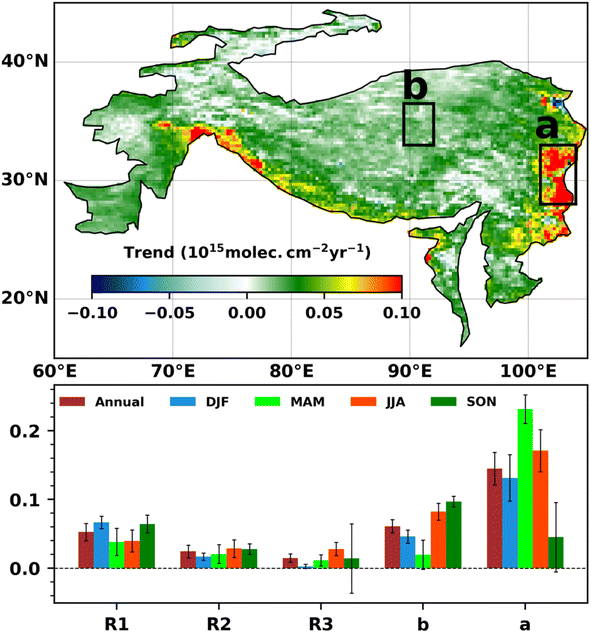 |
| Fig. 5 (Top) Annual trends (2005–2020) in tropospheric NO2 in the TP. Box a represents the most polluted regions and box b represents a pristine region in the TP. (Bottom) Annual and seasonal trends (2005–2020) of the tropospheric NO2 column (in 1015 molecule cm−2 year−1) in three distinct NO2 column regions R1, R2, and R3 and two selected sub-regions a and b (see Fig. 1). The seasons are defined as winter (December–January–February, DJF), spring (March–April–May, MAM), summer (June–July–August, JJA) and autumn (September–October–November, SON). | |
Furthermore, we have also analysed the NO2 trends for 20 selected cities with respect to the annual and seasonal data, as shown in Fig. 6 (top). The bottom panel of the figure shows an approximate area of the selected cities and average population based on the last census (year may be different for each country). The estimated trends are positive for all cities, except Urumqi and Almaty, and they show a decreasing annual trend of −0.42 ± 0.08 × 1015 molec. cm−2 year−1 and −0.01 ± 0.03 × 1015 molec. cm−2 year−1, respectively. However, most cities within the TP show a clear positive trend. The highest increasing annual trend of 0.32 ± 0.07 × 1015 molec. cm−2 year−1 is found at Kunming and the lowest of 0.02 ± 0.01 × 1015 molec. cm−2 year−1 at Khuzdar. Therefore, the analysis for the cities in the region further confirms that the pollution is rising and is also transferred to the inner TP.
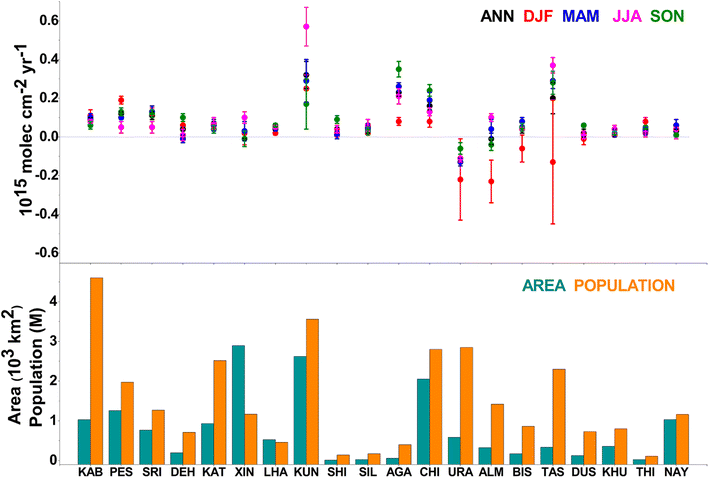 |
| Fig. 6 (Top) Annual and seasonal trend (2005–2020) of the tropospheric NO2 column (in 1015 molec. cm−2 year−1) in 20 selected cities for the same period. (Bottom) Approximate city area (km2) and population (in million, M) based on the last census (different for each country, e.g. 2011 for India, 2011 for China and 2017 for Pakistan). The seasons are defined as winter (December–January–February, DJF), spring (March–April–May, MAM), summer (June–July–August, JJA) and autumn (September–October–November, SON). The city names are shown in brackets for their abbreviated form, i.e. KAB (Kabul), PES (Peshawar), SRI (Srinagar), DEH (Dehradun), KAT (Kathmandu), XIN (Xining), KUN (Kunming), SHI (Shillong), SIL (Silchar), AGA (Agartala), CHI (Chittagong), URA (Urumqi), ALM (Almaty), BIS (Bishkek), TAS (Tashkent), LHA (Lhasa), THI (Thimphu), DUS (Dushanbe), KUZ (Khuzdar), and NAY (Naypyitaw). | |
3.5 Sources of NO2 emissions from EDGAR v6.1
To examine the impact of anthropogenic sources of NO2 on the TP, we have analysed the emission inventory EDGARv6.1 available from 1970 to 2018. It shows that the contribution of emissions from road transport, power and refinery sectors increased by 0.8, 0.4 and 0.4%, respectively, whereas the contribution from agricultural activities is reduced by 2% during 2005–2018 compared to that in 1970–2018. This decline might be due to change in climate and associated reduction in certain agricultural activities. For instance, Bai et al.64 observed the loss in agricultural production due to climate change in the TP for the period 2000–2019; consistent with our analysis.
Fig. 7 (top) shows that the agricultural activities are mostly confined to the regions in close proximity to the IGP (both in India and Pakistan), Northeast India, Afghanistan, Nepal, Chittagong hill side of Bangladesh and Myanmar. Wheat, barley and rice are the major agricultural crops produced in Afghanistan, while rice, wheat, sugarcane and groundnuts are the major agricultural products of the IGP.65 One of the main sources of NO2 in these regions is open stubble burning. However, during the wheat-rice rotation period, biomass burning frequency is very high as observed from the fire count analysis (Fig. S2†). Major stubble burning occurs during October–May in the IGP, YYRB, Nepal, Myanmar and East Asia.4,44,46 However, large areas on the southern slope of the TP, including Nepal, Bhutan, Bangladesh, Northeast India, Myanmar, East Asia and some regions of the YYRB, are covered with broadleaf forest, and the fire count frequency is high during December–June there, as illustrated in Fig. S2.†
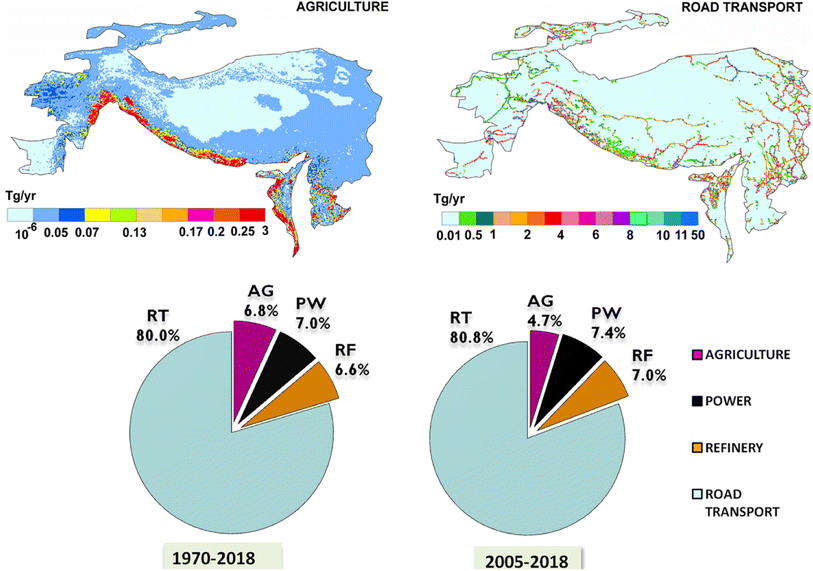 |
| Fig. 7 (Top) Spatial representation of NO2 emissions from the agriculture sector and road transport during the period 1970–2018. (Bottom) Percentage contributions of anthropogenic NO2 emissions from road transport (RT), the agricultural sector (AG), the power generation sector (PW) and a refinery (RF) during the periods 1970–2018 and 2005–2018 using the EDGAR v6.0 inventory. | |
Fig. 7 (bottom) shows the NO2 pollution through road transport in the TP, which are very small (<4 Tg year−1) in Nepal, Bhutan and Myanmar. In the roadways of Diqing, Nujiang and Dali prefectures of Yunnan province, Ganjzi, Aba and Liangshan prefectures of Sichuan Province and the entire Qinghai Province of China, the NO2 emission rate is <8 Tg year−1. However, the emission is comparatively higher on the highways of these regions, such as Qinghai-Tibet, Sichuan – Tibet and Xinjiang – Tibet highways, about 11–50 Tg year−1. On the Sino – Nepal friendship highway and Thimphu – Lhasa highway, the NO2 emission rate is <4 Tg year−1. Similarly, a high emission of 11–50 Tg year−1 is observed along the roadways that connect major cities like Peshawar, Islamabad, Rawalpindi, Lahore, Faisalabad, Multan and Quetta in Pakistan. On the roadways in Uttarakhand, Himachal Pradesh, Jammu and Kashmir, the NO2 emission rate is within 1–8 Tg year−1. This is due to the gradual increase in the electricity demand and industrial production in the adjacent regions. Inventory analysis (Fig. 7) shows that the contribution of the refinery sector is about 7%, and there are no such direct anthropogenic sources of NO2 in the inner TP. Long-distance transport from the YYRB is the main source of NO2 from the refinery sector during summer, but refinery supplies are also located around the IGP, Middle East and Central Asian regions to contribute to the NO2 emissions in other seasons.
3.6 Error assessment
One of the limitations of the analysis presented here is the uncertainties associated with NO2 measurements. These depend mainly on the estimation of the Air Mass Factor (AMF) for converting the Slant Column Density (SCD) to the Vertical Column Density (VCD), which relies on a priori, surface albedo, satellite geometry, cloud fraction and cloud pressure.66 Zhao and Wang67 reported an error of less than 0.5 × 1015 molec. cm−2, and the total error per retrieval from OMI was about 30% over most of Eastern China for the measurements in July 2007. Nonetheless, the DOAS method is widely accepted to have the highest accuracy for different satellite sensors to retrieve atmospheric NO2.68 Previous studies have shown that satellites underestimate the amount of NO2 in regions that are characterised by inhomogeneous distributions, particularly urbanised and industrialised locations, i.e. the gradient smoothing effect.69 For instance, Celarier et al.68 estimated the correlation of NO2 between OMI and DOAS measurements from Cabauw, Netherlands, for which the correlation to ground-based (at Bremen and Heidelberg) and air-borne measurements [Intercontinental Chemical Transport Experiment (INTEX) and Polar Aura Validation Experiment (PAVE)] was greater than 0.6 for the year 2005. Ma et al.70 reported an underestimation of 26–38% in NO2 concentrations by OMI at Beijing, China for the measurements in 2008–2011. Irie et al.71 showed a bias of about 10% for NO2 from the space-borne measurements of SCIAMACHY, OMI and GOME-2 as compared to the ground-based measurements (MAX-DOAS) at Tokyo, Japan for the period 2006–2011. Wenig et al.72 validated the NO2 measurements from OMI with a ground-based Brewer instrument and reported an uncertainty within 25% when compared to the satellite measurements. They also reported 5–10% error in the NO2 retrieval from the satellite, which is mainly due to the hotspot regions with a high spatial gradient. However, the inner TP does not have such hotspots and thus, we assume the bias to be relatively lower when compared to that of other regions. We also compared the satellite measurements to the ground-based measurements at Urumqui, Kunming and Chengguan, and found that the satellite measurements have reasonably reproduced seasonal and interannual variability of NO2 in these regions, although these measurements are not directly comparable because of different measurement units (Fig. S3†).
EDGAR provides the monthly, annual and country specific emission maps and time series data. However, the disaggregation of EDGAR to the real time data has a notable uncertainty, since the temporal evolution of pollutants is difficult to estimate due to their complex and heterogeneous distributions.73 The agricultural emission data from EDGAR, due to the lack of adequate representation, have relatively higher uncertainties.74 Nevertheless, EDGAR assumes constant production of crops in a year, which also adds uncertainties in the emission estimates. Crippa et al.75 validated the EDGAR emission inventory by estimating the uncertainties using a quality score from 1 to 4. Solazzo et al.76 find that the bias in anthropogenic emissions covered by the EDGAR inventory for CO2, CH4, and N2O is between −15% and +20% for the year 2015. Therefore, we have used the EDGAR emission inventory only to examine the key emission or pollution sources in and around the TP.
4. Conclusions
The spatio-temporal variation of tropospheric NO2 in the TP is analysed using OMI and GOME-2B satellite measurements from 2005 to 2020. We observe high levels of NO2 (>1 × 1015 molec. cm−2) in the low-lying regions of the TP, particularly in the regions close to the IGP in the south and the YYRB in the east, and NO2 is comparably lower (<0.5 × 1015 molec. cm−2) in the high-altitude regions of the TP. A major contribution towards tropospheric NO2 in the TP is from road transport (81%), owing to the impact of anthropogenic activities such as infrastructure development in the region during 2010–2020, together with climate change. Seasonal analysis shows a significant increase in the distribution of NO2 from 0.25 to 1 × 1015 molec. cm−2, which invades the pristine regions of the inner TP. We find that the NO2 trend is positive for most regions of the TP and near-by cities and even in the pristine areas of the central TP (up to 0.05 ± 0.01 × 1015 molec. cm−2 year−1), suggesting a gradual decline in air quality in the TP. NO2 over the TP sharply increased after 2018, which is almost double in all regions, and is a signal of environmental change. This increasing pollution is a menace to the life of about 2 billion people living in the downstream of the TP. Henceforth, in the context of current global warming, the observed signature of climate and environment change in one of the most remote and pristine regions on Earth is a serious concern, which reiterates the importance of this study.
Author contributions
BRS: methodology, data analyses, visualization, validation, software, and writing–original draft. JK: conceptualization, methodology, visualization, supervision, and review and editing of the original draft. GSG and MP: data analyses, visualization, and review and editing of the original draft.
Data availability
The data used in this study are publicly available: tropospheric NO2: https://www.temis.nl, topography: https://www.shadedrelief.com/, ERA5 wind data: https://www.ecmwf.int/en/forecasts/datasets/reanalysis-datasets/era5, Hysplit model: https://www.ready.noaa.gov/HYSPLIT.php, EDGAR inventory: https://edgar.jrc.ec.europa.eu/, and population data for respective countries are available on: https://nepalindata.com/media/resources/items/20/bStatistical-Year-Book-2017.pdf, https://www.nsb.gov.bt/publications/census-report/, https://censusindia.gov.in/census.website/data/census-tables, https://www.dop.gov.mm/en/publication-category/2014-reports, https://populationstat.com/kazakhstan/almaty, https://populationstat.com/search/, https://knoema.com/atlas/Tajikistan/Dushanbe#Demography.
Conflicts of interest
There are no conflicts of interest to declare.
Acknowledgements
We thank the Director, Indian Institute of Technology Kharagpur (IIT Kgp), Chairman of CORAL IIT Kgp and the Ministry of Education (MoE) for facilitating the study. BRS acknowledges his IIT Kgp Doctoral Scholarship for Foreign Students. GSG acknowledges the Department of Science and Technology, Ministry of Science and Technology, India for the INSPIRE fellowship for his Doctoral study at IIT KGP. MP acknowledges her doctoral fellowship from MoE through IIT Kgp. We acknowledge the free use of tropospheric NO2 column data from the OMI and GOME-2 (METOP-B) sensor from https://www.temis.nl. We also acknowledge the Copernicus Atmospheric Data Store for the ERA5 reanalysis data. The authors acknowledge the NOAA Air Resource Laboratory (ARL) scientific team for the HYSPLIT model.
References
- D. J. Jacob, E. G. Heikes, S. M. Fan, J. A. Logan, D. L. Mauzerall, J. D. Bradshaw, H. B. Singh, G. L. Gregory, R. W. Talbot, D. R. Blake and G. W. Sachse, Origin of ozone and NOx in the tropical troposphere: A photochemical analysis of aircraft observations over the South Atlantic basin, J. Geophys. Res.: Atmos., 1996, 101, 24235–24250, DOI:10.1029/96JD00336
.
-
World Health Organization, WHO Air Quality Guidelines Global Update 2005: Report on a Working Group Meeting, Bonn, Germany, 18–20 October 2005 (No. WHO/EURO: 2005-4244-44003-62046), 2005, http://www.euro.who.int/pubrequest Search PubMed
.
- O. Badr and S. D. Probert, Oxides of nitrogen in the earth's atmosphere: trends, sources, sinks and environmental impacts, Appl. Energy, 1993, 46, 1–67, DOI:10.1016/0306-2619(93)90076-2
.
- J. Kuttippurath, A. Singh, S. P. Dash, N. Mallick, C. Clerbaux, M. Van Damme, L. Clarisse, P. F. Coheur, S. Raj, K. Abbhishek and H. Varikoden, Record high levels of atmospheric ammonia over India: Spatial and temporal analyses, Sci. Total Environ., 2020, 740, 139986, DOI:10.1016/j.scitotenv.2020.139986
.
- G. S. Gopikrishnan, J. Kuttippurath, S. Raj, A. Singh and K. Abbhishek, Air Quality during the COVID–19 Lockdown and Unlock Periods in India Analyzed Using Satellite and Ground-based Measurements, Environ. Processes, 2022, 1–21, DOI:10.1007/s40710-022-00585-9
.
-
M. K. Firestone and E. A. Davidson, Microbiological Basis of NO and N2O Production and Consumption in Soil, ed. M. O. Andreae and D. S. Schimel, 1989, vol. 47, pp. 7–21, https://www.webofscience.com/wos/WOSCC/full-record/A1989BU45V00002 Search PubMed
.
- M. Pathak and J. Kuttippurath, Air quality trends in rural India: analysis of NO2 pollution using satellite measurements, Environ. Sci.: Processes Impacts, 2022, 24, 2437–2449, 10.1039/D2EM00293K
.
- S. Solomon, R. W. Portmann, R. W. Sanders, J. S. Daniel, W. Madsen, B. Bartram and E. G. Dutton, On the role of nitrogen dioxide in the absorption of solar radiation, J. Geophys. Res.: Atmos., 1999, 104, 12047–12058, DOI:10.1029/1999JD900035
.
- S. Kang, Q. Zhang, Y. Qian, Z. Ji, C. Li, Z. Cong, Y. Zhang, J. Guo, W. Du, J. Huang and Q. You, Linking atmospheric pollution to cryospheric change in the Third Pole region: current progress and future prospects, Natl. Sci. Rev., 2019, 6, 796–809, DOI:10.1093/nsr/nwz031
.
- J. Qiu, China: The third pole, Nature, 2008, 454, 393–396, DOI:10.1038/454393a
.
- J. Kuttippurath, B. R. Sharma and G. S. Gopikrishnan, Trends and variability of Total Column Ozone in the Third Pole, Front. Clim., 2023, 5, 139986, DOI:10.3389/fclim.2023.1129660
.
- T. Yao, L. G. Thompson, V. Mosbrugger, F. Zhang, Y. Ma, T. Luo, B. Xu, X. Yang, D. R. Joswiak, W. Wang and M. E. Joswiak, Third pole environment (TPE), Environ. Dev., 2012, 3, 52–64, DOI:10.1016/j.envdev.2012.04.002
.
-
P. Wester, A. Mishra, A. Mukherji and A. B. Shrestha, The Hindu Kush Himalaya Assessment: Mountains, Climate Change, Sustainability and People, 2019, DOI:10.1007/978-3-319-92288-1
.
- Z. Cong, K. Kawamura, S. Kang and P. Fu, Penetration of biomass-burning emissions from South Asia through the Himalayas: new insights from atmospheric organic acids, Sci. Rep., 2015, 5, 1–7, DOI:10.1038/srep09580
.
- D. Rupakheti, M. Rupakheti, M. Rai, X. Yu, X. Yin, S. Kang, M. D. Orozaliev, V. P. Abdullaev, S. F. Abdullaev, I. D. Sulaymon and J. Hu, Characterization of columnar aerosol over a background site in Central Asia, Environ. Pollut., 2023, 316, 120501, DOI:10.1016/j.envpol.2022.120501
.
- J. W. Sun and E. Kuntsi, Environmental impact of energy use in Bangladesh, India, Pakistan and Thailand, Glob. Environ. Change, 2004, 14, 161–169, DOI:10.1016/j.gloenvcha.2003.12.001
.
- D. G. Streets, K. F. Yarber, J. H. Woo and G. R. Carmichael, Biomass burning in Asia: Annual and seasonal estimates and atmospheric emissions, Global Biogeochem. Cycles, 2003, 17, 1099, DOI:10.1029/2003GB002040
.
- R. J. Van der A, D. H. M. U. Peters, H. Eskes, K. F. Boersma, M. Van Roozendael, I. De Smedt and H. M. Kelder, Detection of the trend and seasonal variation in tropospheric NO2 over China, J. Geophys. Res.: Atmos., 2006, 111, D12317, DOI:10.1029/2005JD006594
.
- S. D. Ghude, S. Fadnavis, G. Beig, S. D. Polade and R. J. Van Der A, Detection of surface emission hot spots, trends, and seasonal cycle from satellite retrieved NO2 over India, J. Geophys. Res.: Atmos., 2008, 113, D20, DOI:10.1029/2007JD009615
.
- Z. Lu, D. G. Streets, B. De Foy and N. A. Krotkov, Ozone Monitoring Instrument observations of interannual increases in SO2 emissions from Indian coal-fired power plants during 2005–2012, Environ. Sci. Technol., 2013, 47, 13993–14000, DOI:10.1021/es4039648
.
- A. Ramachandran, N. K. Jain, S. A. Sharma and J. Pallipad, Recent trends in tropospheric NO2 over India observed by SCIAMACHY: Identification of hot spots, Atmos. Pollut. Res., 2013, 4, 354–361, DOI:10.5094/APR.2013.040
.
- Z. Ul-haq, S. Tariq, M. Ali, K. Mahmood, S. A. Batool and A. D. Rana, A study of tropospheric NO2 variability over Pakistan using OMI data, Atmos. Pollut. Res., 2014, 5, 709–720, DOI:10.5094/APR.2014.080
.
- D. Gu, Y. Wang, C. Smeltzer and Z. Liu, Reduction in NOx emission trends over China: Regional and seasonal variations, Environ. Sci. Technol., 2013, 47, 12912–12919, DOI:10.1021/es401727e
.
-
J. Delman and O. Odgaard, From ‘Worn’to ‘Green'China Model: Energy in the 12th Five-Year Plan in an Environmental and Climate-Change Perspective, in The Political Economy of Renewable Energy and Energy Security, Palgrave Macmillan, London, 2014, pp. 221–240, DOI:10.1057/978113733887711
.
- R. J. Van der A, B. Mijling, J. Ding, M. E. Koukouli, F. Liu, Q. Li, H. Mao and N. Theys, Cleaning up the air: effectiveness of air quality policy for SO2 and NOx emissions in China, Atmos. Chem. Phys., 2017, 17, 1775–1789, DOI:10.5194/acp-17-1775-2017
.
- J. P. Burrows, M. Weber, M. Buchwitz, V. Rozanov, A. Ladstätter-Weißenmayer, A. Richter, R. DeBeek, R. Hoogen, K. Bramstedt, K. U. Eichmann and M. Eisinger, The global ozone monitoring experiment (GOME): Mission concept and first scientific results, J. Atmos. Sci., 1999, 56, 151–175, DOI:10.1175/1520-0469(1999)056<3C0151:TGOMEG>2.0.CO;2
.
- H. Bovensmann, J. P. Burrows, M. Buchwitz, J. Frerick, S. Noël, V. V. Rozanov, K. V. Chance and A. P. H. Goede, SCIAMACHY: Mission objectives and measurement modes, J. Atmos. Sci., 1999, 56, 127–150, DOI:10.1175/1520-0469(1999)056<3C0127:SMOAMM>2.0.CO;2
.
- P. F. Levelt, G. H. Van Den Oord, M. R. Dobber, A. Malkki, H. Visser, J. De Vries, P. Stammes, J. O. Lundell and H. Saari, The ozone monitoring instrument, IEEE Trans. Geosci. Remote Sens., 2006, 44, 1093–1101, DOI:10.1109/TGRS.2006.872333
.
- R. Munro, R. Lang, D. Klaes, G. Poli, C. Retscher, R. Lindstrot, R. Huckle, A. Lacan, M. Grzegorski, A. Holdak and A. Kokhanovsky, The GOME-2 instrument on the Metop series of satellites: instrument design, calibration, and level 1 data processing — an overview, Atmos. Meas. Tech., 2016, 9, 1279–1301, DOI:10.5194/amt-9-1279-2016
.
- X. Zhao, D. Griffin, V. Fioletov, C. McLinden, A. Cede, M. Tiefengraber, M. Müller, K. Bognar, K. Strong, F. Boersma and H. Eskes, Assessment of the quality of TROPOMI high-spatial-resolution NO2 data products in the Greater Toronto Area, Atmos. Meas. Tech., 2020, 13, 2131–2159, DOI:10.5194/amt-13-2131-2020
.
- V. M. Schenkeveld, G. Jaross, S. Marchenko, D. Haffner, Q. L. Kleipool, N. C. Rozemeijer, J. P. Veefkind and P. F. Levelt, In-flight performance of the Ozone Monitoring Instrument, Atmos. Meas. Tech., 2017, 10, 1957–1986, DOI:10.5194/amt-10-1957-2017
.
- K. F. Boersma, H. J. Eskes, R. J. Dirksen, R. J. Van Der A, J. P. Veefkind, P. Stammes, V. Huijnen, Q. L. Kleipool, M. Sneep, J. Claas and J. Leitao, An improved tropospheric NO2 column retrieval algorithm for the Ozone Monitoring Instrument, Atmos. Meas. Tech., 2011, 4, 1905–1928, DOI:10.5194/amt-4-1905-2011
.
- I. De Smedt, I. T. Stavrakou, F. Hendrick, T. Danckaert, T. Vlemmix, G. Pinardi, N. Theys, C. Lerot, C. Gielen, C. Vigouroux and C. Hermans, Diurnal, seasonal and long-term variations of global formaldehyde columns inferred from combined OMI and GOME-2 observations, Atmos. Chem. Phys., 2015, 15, 12519–12545, DOI:10.5194/acp-15-12519-2015
.
- M. R. Schoeberl, A. R. Douglass, E. Hilsenrath, P. K. Bhartia, R. Beer, J. W. Waters, M. R. Gunson, L. Froidevaux, J. C. Gille, J. J Barnett and P. F. Levelt, Overview of the EOS Aura mission, IEEE Trans. Geosci. Remote Sens., 2006, 44, 1066–1074, DOI:10.1109/TGRS.2005.861950
.
- K. Bai, N. B. Chang, H. Yu and W. Gao, Statistical bias correction for creating coherent total ozone record from OMI and OMPS observations, Remote Sens. Environ., 2016, 182, 150–168, DOI:10.1016/j.rse.2016.05.007
.
- G. S. Gopikrishnan and J. Kuttippurath, A decade of satellite observations reveal significant increase in atmospheric formaldehyde from shipping in Indian Ocean, Atmos. Environ., 2021, 246, 118095, DOI:10.1016/j.atmosenv.2020.118095
.
- O. Torres, P. K. Bhartia, H. Jethva and C. Ahn, Impact of the ozone monitoring instrument row anomaly on the long-term record of aerosol products, Atmos. Meas. Tech., 2018, 11, 2701–2715, DOI:10.5194/amt-11-2701-2018
.
- H. Hersbach, B. Bell, P. Berrisford, S. Hirahara, A. Horányi, J. Muñoz-Sabater, J. Nicolas, C. Peubey, R. Radu, D. Schepers and A. Simmons, The ERA5 global reanalysis, Q. J. R. Meteorol. Soc., 2020, 146, 1999–2049, DOI:10.1002/qj.3803
.
- S. Itahashi, K. Yumimoto, J. I. Kurokawa, Y. Morino, T. Nagashima, K. Miyazaki, T. Maki and T. Ohara, Inverse estimation of NOx emissions over China and India 2005–2016: contrasting recent trends and future perspectives, Environ. Res. Lett., 2019, 14, 124020, DOI:10.1088/1748-9326/ab4d7f
.
- T. Kunhikrishnan, M. G. Lawrence, R. von Kuhlmann, A. Richter, A. Ladstätter-Weißenmayer and J. P. Burrows, Analysis of tropospheric NOx over Asia using the model of atmospheric transport and chemistry (MATCH-MPIC) and GOME-satellite observations, Atmos. Environ., 2003, 38, 581–596, DOI:10.1016/j.atmosenv.2003.09.074
.
- U. Kulshrestha and B. Kumar, Airmass trajectories and long-range transport of pollutants: review of wet deposition scenario in South Asia, Adv. Meteorol., 2014, 14, 596041, DOI:10.1155/2014/596041
.
- A. Fan, P. K. Hopke, T. M. Raunemaa, M. Öblad and J. M. Pacyna, A study on the potential sources of air pollutants observed at Tjörn, Sweden, Environ. Sci. Pollut. Res., 1995, 2, 107–115, DOI:10.1007/BF02986733
.
- A. F. Stein, R. R. Draxler, G. D. Rolph, B. J. B. Stunder, M. D. Cohen and F. Ngan, NOAA’s HYSPLIT atmospheric transport and dispersion modeling system, Bull. Am. Meteorol. Soc., 2015, 96, 2059–2077, DOI:10.1175/BAMS-D-14-00110.1.66
.
- K. V. S. Badarinath, T. K. Chand and V. K. Prasad, Agriculture crop residue burning in the Indo-Gangetic Plains–a study using IRS-P6AWiFS satellite data, Curr. Sci., 2006, 91, 1085–1089 Search PubMed
. https://www.jstor.org/stable/24093988.
- C. Wang, T. Wang and P. Wang, The spatial–temporal variation of tropospheric NO2 over China during 2005 to 2018, Atmosphere, 2019, 10, 444, DOI:10.3390/atmos10080444
.
- J. Kuttippurath, K. Abbhishek, G. S. Gopikrishnan and M. Pathak, Investigation of long–term trends and major sources of atmospheric HCHO over India, Environ. Challenges, 2022, 7, 100477, DOI:10.1016/j.envc.2022.100477
.
- D. Rupakheti, S. Kang, M. Rupakheti, L. Tripathee, Q. Zhang, P. Chen and X. Yin, Long-term trends in the total columns of ozone and its precursor gases derived from satellite measurements during 2004–2015 over three different regions in South Asia: Indo-Gangetic Plain, Himalayas and Tibetan Plateau, Int. J. Remote Sens., 2018, 39, 7384–7404, DOI:10.1080/01431161.2018.1470699
.
- L. K. Sahu, V. Sheel, K. Pandey, R. Yadav, P. Saxena and S. Gunthe, Regional biomass burning trends in India: Analysis of satellite fire data, J. Earth Syst. Sci., 2015, 124, 1377–1387, DOI:10.1007/s12040-015-0616-3
.
- V. Ramanathan, F. Li, M. V. Ramana, P. S. Praveen, D. Kim, C. E. Corrigan, H. Nguyen, E. A. Stone, J. J. Schauer, G. R. Carmichael and B. Adhikary, Atmospheric brown clouds: Hemispherical and regional variations in long-range transport, absorption, and radiative forcing, J. Geophys. Res.: Atmos., 2007, 112, D22S21, DOI:10.1029/2006JD008124
.
- L. Jin-Tai, P. Da and Z. Rui-Xiong, Trend and interannual variability of Chinese air pollution since 2000 in association with socioeconomic development: A brief overview, Atmos. Sci. Lett., 2013, 6, 84–89, DOI:10.1080/16742834.2013.11447061
.
-
Clean Air Alliance of China (CAAC), State Council Air Pollution Prevention and Control Action Plan, 2013, https://policy.asiapacificenergy.org/node/2875 Search PubMed
.
-
National Environment Protection Act of Bhutan (NEPAB), 2007, https://policy.asiapacificenergy.org/node/79, accessed April, 2021.
-
Pakistan Environmental Protection Act (PEPA), 1997, https://www.elaw.org/system/files/Law-PEPA-1997.pdf, accessed May, 2021.
- S. U. Saheb, S. Seshaiah and B. Viswanath, Environment and their legal issues in India, Int. J. Environ. Res., 2012, 1, 44–51 Search PubMed
. https://www.isca.in/.
-
Tibet Autonomous Region Government Work Report (TARGWR), 2021, https://www.xizang.gov.cn/zwgk/xxfb/zfgzbg/202103/t20210324_197174.html, accessed 15 June 2022 Search PubMed.
- D. Gao and S. Li, Spatiotemporal impact of railway network in the Qinghai-Tibet Plateau on accessibility and economic linkages during 1984–2030, J. Transp. Geogr., 2022, 100, 103332, DOI:10.1016/j.jtrangeo.2022.103332
.
-
S. Desai, Civilian and Military Developments in Tibet: Takshashila Discussion Document, The Takshashila Institution, Bengaluru, 2021, https://static1.squarespace.com/static/618a55c4cb03246776b68559/t/61e5f12005a42f2bec475733/1642459437927/Civilian-and-Military-Developments-in-Tibet-1.0-SD.pdf Search PubMed
.
- X. Cui and H. F. Graf, Recent land cover changes
on the Tibetan Plateau: a review, Clim. Change, 2009, 94, 47–61, DOI:10.1007/s10584-009-9556-8
.
- F. Liu, Q. Zhang, B. Zheng, D. Tong, L. Yan, Y. Zheng and K. He, Recent reduction in NOx emissions over China: synthesis of satellite observations and emission inventories, Environ. Res. Lett., 2016, 11, 114002, DOI:10.1088/1748-9326/11/11/114002
.
- F. Liu, S. Beirle, Q. Zhang, R. J. Van Der A, B. Zheng, D. Tong and K. He, NOx emission trends over Chinese cities estimated from OMI observations during 2005 to 2015, Atmos. Chem. Phys., 2017, 17, 9261–9275, DOI:10.5194/acp-2017-369
.
- B. Deng, J. Affolderbach and P. Deutz, Industrial Restructuring through Eco-Transformation: Green Industrial Transfer in Changsha–Zhuzhou–Xiangtan, Hunan Province, Sustainability, 2020, 12, 6945, DOI:10.3390/SU12176945
.
- Y. Xie, W. Wang and Q. Wang, Spatial Distribution and Temporal Trend of Tropospheric NO2 over the Wanjiang City Belt of China, Adv. Meteorol., 2018, 13, 1–13, DOI:10.1155/2018/6597186
.
- H. Zou, X. Duan, L. Ye and L. Wang, Locating sustainability issues: identification of ecological vulnerability in Mainland China's mega-regions, Sustainability, 2017, 9, 1179, DOI:10.3390/su9071179
.
- D. Bai, L. Ye, Z. Yang and G. Wang, Impact of climate change on agricultural productivity: a combination of spatial Durbin model and entropy approaches, Int. J. Clim. Chang. Strateg. Manag., 2022, 1756–8692, DOI:10.1108/IJCCSM-02-2022-0016
.
- V. Tiwari, M. A. Matin, F. M. Qamer, W. L. Ellenburg, B. Bajracharya, K. Vadrevu, B. R. Rushi and W. Yusafi, Wheat area mapping in Afghanistan based on optical and SAR time-series images in google earth engine cloud environment, Front. Environ. Sci., 2020, 8, 77, DOI:10.3389/fenvs.2020.00077
.
- K. F. Boersma, H. J. Eskes, J. P. Veefkind, E. J. Brinksma, R. J. Van Der A, M. V. Sneep, G. H. J. Van Den Oord, P. F. Levelt, P. Stammes, J. F. Gleason and E. J. Bucsela, Near-real time retrieval of tropospheric NO2 from OMI, Atmos. Chem. Phys., 2007, 7, 2103–2118, DOI:10.5194/acp-7-2103-2007
.
- C. Zhao and Y. Wang, Assimilated inversion of NOx emissions over east Asia using OMI NO2 column measurements, Geophys. Res. Lett., 2009, 36, L06805, DOI:10.1029/2008GL037123
.
- E. A. Celarier, E. J. Brinksma, J. F. Gleason, J. P. Veefkind, A. Cede, J. R. Herman, D. Ionov, F. Goutail, J. P. Pommereau, J. C. Lambert and M. Van Roozendael, Validation of Ozone Monitoring Instrument nitrogen dioxide columns, J. Geophys. Res., 2008, 113, D15S15, DOI:10.1029/2007JD008908
.
- D. Chen, B. Zhou, S. Beirle, L. M. Chen and T. Wagner, Tropospheric NO2 column densities deduced from zenith-sky DOAS measurements in Shanghai, China, and their application to satellite validation, Atmos. Chem. Phys., 2009, 9, 3641–3662, DOI:10.5194/acp-9-3641-2009
.
- J. Z. Ma, S. Beirle, J. L. Jin, R. Shaiganfar, P. Yan and T. Wagner, Tropospheric NO2 vertical column densities over Beijing: results of the first three years of ground-based MAX-DOAS measurements (2008–2011) and satellite validation, Atmos. Chem. Phys., 2013, 13, 1547–1567, DOI:10.5194/acp-13-1547-2013
.
- H. Irie, K. F. Boersma, Y. Kanaya, H. Takashima, X. Pan and Z. F. Wang, Quantitative bias estimates for tropospheric NO2 columns retrieved from SCIAMACHY, OMI, and GOME-2 using a common standard for East Asia. Atmospheric Measurement Techniques, Atmos. Meas. Tech., 2012, 5, 2403–2411, DOI:10.5194/amt-5-2403-2012
.
- M. O. Wenig, A. M. Cede, E. J. Bucsela, E. A. Celarier, K. F. Boersma, J. P. Veefkind, E. J. Brinksma, J. F. Gleason and J. R. Herman, Validation of OMI tropospheric NO2 column densities using direct-Sun mode Brewer measurements at NASA Goddard Space Flight Center, J. Geophys. Res.: Atmos., 2008, 113, D16S45, DOI:10.1029/2007JD008988
.
- F. Liu, Q. Zhang, D. Tong, B. Zheng, M. Li, H. Huo and K. B. He, High-resolution inventory of technologies, activities, and emissions of coal-fired power plants in China from 1990 to 2010, Atoms. Chem. Phys., 2015, 15, 13299–13317, DOI:10.5194/acp-15-13299-2015
.
-
T. Pregger, Y. Scholz and R. Friedrich, Documentation of the Anthropogenic GHG Emission Data for Europe provided in the Frame of CarboEurope GHG and CarboEurope IP, Institut für Energiewirtschaft und Rationelle Energieanwendung, Universität Stuttgart, Stuttgart, Germany, 2007. https://carboeurope.org/ceip/products/files/Pregger_IER_Final_Report_Feb2007.pdf Search PubMed
.
- M. Crippa, E. Solazzo, G. Huang, D. Guizzardi, E. Koffi, M. Muntean, C. Schieberle, R. Friedrich and G. Janssens-Maenhout, High resolution temporal profiles in the Emissions Database for Global Atmospheric Research, Sci. Data, 2020, 7, 1–17, DOI:10.1038/s41597-020-0462-2
.
- E. Solazzo, M. Crippa, D. Guizzardi, M. Muntean, M. Choulga and G. Janssens-Maenhout, Uncertainties in the Emissions Database for Global Atmospheric Research (EDGAR) emission inventory of greenhouse gases, Atmos. Chem. Phys., 2021, 21, 5655–5683, DOI:10.5194/acp-21-5655-2021
.
|
This journal is © The Royal Society of Chemistry 2023 |
Click here to see how this site uses Cookies. View our privacy policy here.