DOI:
10.1039/D3TA03675H
(Perspective)
J. Mater. Chem. A, 2023,
11, 18626-18645
Towards sustainable electrochemical ammonia synthesis
Received
22nd June 2023
, Accepted 21st August 2023
First published on 22nd August 2023
Abstract
Ammonia plays a pivotal role in various sectors such as global agriculture, chemical industries, and clean energy systems. To align with the development of a low-carbon economy, it is crucial to adopt sustainable and environmentally friendly production methods for ammonia. In this regard, electrochemical nitrogen reduction reaction (eNRR) powered by renewable energies has emerged as a promising alternative for green ammonia synthesis, effectively mitigating the detrimental impacts of fossil fuels in industrial processes. Despite significant progress in eNRR research, challenges persist due to the inherent stability of nitrogen molecules and the competition from hydrogen evolution reactions in aqueous solutions. This perspective explores the mechanisms and current research surrounding eNRR, encompassing a wide range of catalysts, electrolytes, and cell configurations, along with various ammonia test methods. Feasible strategies are proposed for enhancing ammonia yield and synthesis efficiency through electrocatalyst design and device optimization. Furthermore, the challenges and prospects are evaluated for future eNRR development, offering insights that can drive advancements in electrochemical ammonia synthesis. By addressing the current status, challenges, and perspectives of this field, this perspective aims to contribute to the application research of sustainable and efficient ammonia production processes, supporting the transition towards a carbon-neutral future.
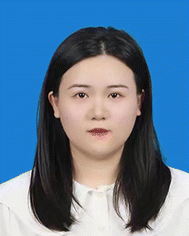 Ruonan Li | Ruonan Li attained her B.S. degree in 2019, followed by her Master's degree in 2022, both from Guangxi University, China. Presently, she is pursuing her PhD studies at the School of Chemical Engineering, Zhengzhou University. Her current research focuses on the development of highly efficient catalysts and systems for electrochemical ammonia synthesis. |
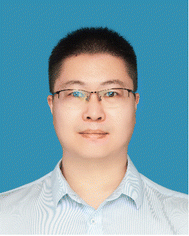 Wei Ma | Wei Ma received his B.S. degree in Inorganic Nonmetal Materials Engineering from Central South University, China in 2009, followed by his PhD in Mineral Materials Science from the same university in 2015. During his doctoral studies, he spent two years (2013 to 2015) at the National Institute for Materials Science (NIMS), Japan, as a joint PhD student. Since 2016, he has been a faculty member at Zhengzhou University, China, where he is now an associate professor at the School of Chemical Engineering. His research focuses on the development of advanced nanomaterials for electrocatalysis. |
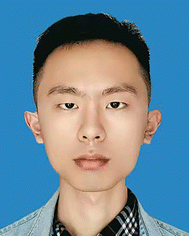 Yujie Liu | Yujie Liu received his B.S. degree from Tianjin University in 2021, and is currently pursuing his M.S. degree under the supervision of Prof. Wei Ma at Zhengzhou University. His research interest is the design and preparation of highly efficient catalysts for electrochemical ammonia synthesis. |
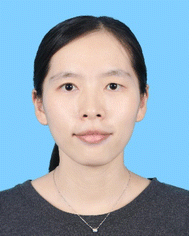 Lili Zhang | Lili Zhang received her PhD degree in 2019 from South China University of Technology, under the supervision of Prof. Haihui Wang. Since 2019, she has been working at Zhengzhou University. Her current research interest focuses on electrocatalytic water splitting, as well as nitrogen and oxygen reduction reactions. |
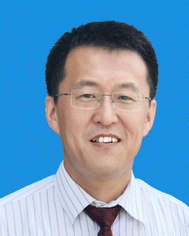 Zhen Zhou | Zhen Zhou completed his B.S. degree in Applied Chemistry in 1994, followed by his PhD in Inorganic Chemistry in 1999, both from Nankai University, China. He commenced his academic career at Nankai University as a lecturer in 1999. After two years, he embarked on a postdoctoral fellowship at Nagoya University, Japan. In 2005, he returned to Nankai University, assuming the role of associate professor, and later attained the position of full professor in 2011. In 2021, he transitioned to Zhengzhou University, China, where he holds the position of Distinguished Professor and currently serves as the Dean of the School of Chemical Engineering. His primary research focus on the integration of high-throughput computations, experiments, and machine learning for energy storage and conversion. |
1. Introduction
Ammonia (NH3), as a crucial chemical raw material and energy carrier, finds wide application in fertilizer synthesis, chemical production, fuel cells, and more.1,2 The predominant method for industrial NH3 synthesis is the renowned Haber–Bosch process, developed into a commercial technology by Nobel Prize laureates Fritz Haber and Carl Bosch. This process involves nitrogen reacting with hydrogen at high temperatures and pressures in the presence of Fe-based catalysts, relying on coal or natural gas for energy input.3,4 However, this method consumes approximately 1–2% of global annual fossil energy and results in a substantial increase of approximately 300 million metric tons of CO2 emissions.5,6 With the growing energy scarcity and environmental concerns, the need for green and sustainable ammonia synthesis processes has become urgent. Various routes for ammonia synthesis have been explored, including the Haber–Bosch process, biocatalysis, photocatalysis, and electrocatalysis. Although the natural nitrogenase-driven NH3 formation is time-consuming, it serves as a significant pathway. Photocatalytic nitrogen fixation offers a carbon-free approach to ammonia synthesis, but its efficiency and NH3 yield are limited by lighting conditions. In contrast, the electrochemical nitrogen reduction reaction (eNRR) powered by renewable energy sources (such as solar, tidal, and wind) holds promise for maximizing the utilization of clean and volatile energy, with advancements in power grid technology. Moreover, electrocatalysis has the advantage of surpassing thermodynamic limits through potential adjustments, making it appealing for achieving distributed, on-demand ammonia synthesis under ambient conditions with simple and flexible electrochemical devices. Thus, eNRR emerges as a research frontier in the pursuit of green ammonia synthesis, offering potential advantages and broad prospects.7
The eNRR involves the electrochemical conversion of N2 and H2O molecules (N2 + 3H2O → 2NH3 + 1.5O2) at the solid–liquid–gas three-phase interface (catalyst-electrolyte-nitrogen) in aqueous solutions. However, achieving the activation and conversion of inert N2 to NH3 under ambient conditions presents a significant challenge due to the high cleavage energy of N
N triple bonds (940 kJ mol−1), low water solubility of N2 molecules (20 mg L−1), and the presence of competitive hydrogen evolution reaction (HER). In recent years, extensive research has focused on screening and developing nano-catalysts to enhance N2 activation. Concurrently, the faradaic efficiency (FE) of electrochemical nitrogen fixation for ammonia synthesis has been improved by investigating the microstructure and electronic properties of nanomaterials, as well as the influence of electrolytes. Furthermore, advancements in electrode assembly technology and the structural design of electrolyzers have been pursued to increase the yield of electrochemical NH3 synthesis, moving closer to practical goals. In this perspective, we provide a brief introduction to the mechanism of nitrogen reduction and comprehensively explore the progress made in the electrochemical synthesis of ammonia. This includes the rational design of catalysts, electrolyte selection, optimization of electrode assembly and electrolyzer structures, efficient anode reaction compatibility, and mediator development. Finally, we present a prospective outlook on future research areas to drive the rapid development of electrochemical nitrogen fixation for sustainable ammonia synthesis.
2. Reaction mechanisms for eNRR
At present, the widely accepted pathways of N2 reduction to ammonia include dissociative mechanism, associative mechanism, and Mars–van Krevelen (MVK) mechanism.8 As shown in Fig. 1, N2 is first dissociated into two N atoms and adsorbed on the active sites, and then the N atoms are gradually hydrogenated to form NH3 molecules in the process of dissociative mechanism (Fig. 1a). The Haber–Bosch process generally follows this dissociative mechanism, which consumes a large amount of energy to break the N
N triple bond of the adsorbed N2.9 Whereas, the breaking of N–N bond occurs after the hydrogenation in the associative mechanism. Depending on the adsorption configuration of N2 and hydrogenation order of two N atoms, the associative mechanism can proceed by distal, alternating or enzymatic pathways. In the mode that N2 is fixed at the active site via end-on adsorption, hydrogenation preferentially occurs on the N atom far from the surface in the distal pathway, and then the N–N bond breaks, with one NH3 molecule forming and the remaining N atom progressively hydrogenated to yield another NH3 (Fig. 1b). By contrast, the two N atoms in the alternating pathway are hydrogenated alternately, forming two NH3 molecules in succession after the breaking of the N–N bond (Fig. 1c). In the enzymatic pathway, N2 tends to adsorb on the surface through a side-on model, and its hydrogenation process is similar to that of the alternating pathway (Fig. 1d). Besides that, transition metal nitrides, such as ZrN, NbN, CrN, and VN, can trigger eNRR by MVK mechanism, where the surface N atom can be directly hydrogenated into ammonia, leaving a nitrogen vacancy on the surface that is subsequently occupied by dissolved N2 molecules to achieve a catalytic cycle (Fig. 1e).10 Generally, in the progress of hydrogenation of the association mechanism, the weak chemical bond between the two N atoms leads to facile breakage. Therefore, the associative mechanism and MVK mechanism may be more energy-saving and thermodynamically favorable than dissociative mechanisms during electrochemical N2 reduction, but possibly resulting in different products (ammonia or hydrazine) based on the adsorption properties of intermediates.
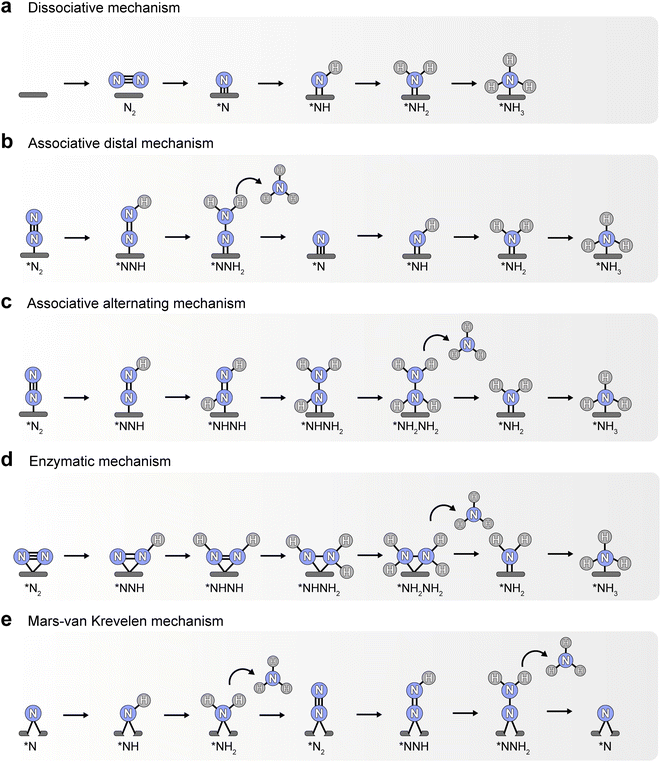 |
| Fig. 1 Common reaction mechanisms for eNRR. (a) Dissociative mechanism. (b) Associative distal mechanism. (c) Associative alternating mechanism. (d) Enzymatic mechanism. (e) Mars–van Krevelen mechanism. | |
3. Current status of eNRR systems
3.1. Catalysts
To overcome the slow kinetics of N2 activation and the competitive side reactions, four types of nanomaterials, i.e., noble-metal-based, non-noble-metal-based, atomically dispersed metal, and metal-free electrocatalysts, have been developed for eNRR. Fig. 2 and Table 1 show the eNRR performances of these catalysts in terms of FE, NH3 yield and current density.
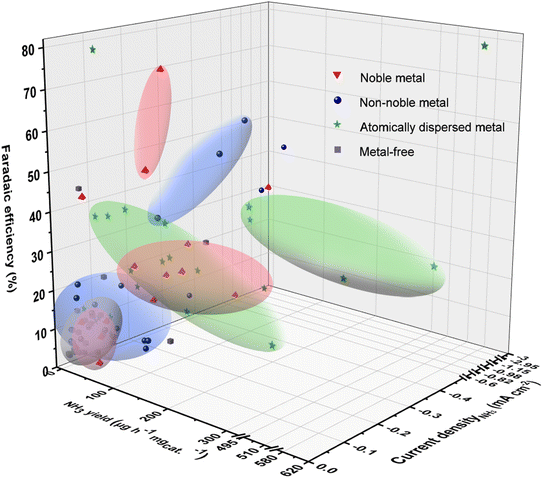 |
| Fig. 2 Summary about the experimental performances (NH3 yield, FE, and current density ascribed to NH3) of reported eNRR catalysts. The specific performances are shown in Table 1. | |
Table 1 Detailed experimental performances of reported eNRR catalystsa
|
Catalysts |
NH3 yield (μg h−1 mgcat.−1) |
FE (%) |
Potential (V vs. RHE) |
J
total (mA cm−2) |
J
NH3 (mA cm−2) |
Stability (h) |
Ref. |
FE represents faradaic efficiency of eNRR, Jtotal represents the total current density of work electrodes, and JNH3 represents the current density of eNRR to ammonia synthesis.
|
Noble metal |
Au NPs |
17.49 |
5.79 |
−0.14 |
∼−0.3 |
−0.01737 |
6 |
18
|
np-PdH0.43 |
20.4 |
43.6 |
−0.15 |
∼−0.1 |
−0.0436 |
10 |
13
|
IrTe |
34.6 |
11.2 |
−0.15 |
∼−0.70 |
−0.0784 |
12 |
19
|
Rh-SeNCs |
35.1 |
13.3 |
−0.1 |
∼−0.092 |
−0.01224 |
10 |
20
|
Rh2SbRNRs/C |
45.77 |
6.32 |
−0.45 |
∼−7 |
−0.4424 |
10 |
21
|
Ru88Pt12 |
47.1 |
8.9 |
−0.2 |
∼−0.52 |
−0.04628 |
15 |
22
|
Pt–Sc |
48.5 |
19 |
−0.2 |
∼−1.2 |
−0.228 |
12 |
23
|
AgNNs |
52.5 |
72.3 |
−0.3 |
∼−0.3 |
−0.2169 |
12 |
14
|
Rh0.6Ru0.4NAs/CP |
57.75 |
3.39 |
−0.2 |
∼−0.75 |
−0.02543 |
12 |
24
|
AgTPs |
58.5 |
25 |
−0.25 |
∼−0.5 |
−0.125 |
4 |
25
|
Pt–Y |
65.2 |
26.7 |
−0.2 |
∼−1 |
−0.267 |
12 |
23
|
Pt–La |
71.4 |
35.6 |
−0.2 |
∼−1.8 |
−0.6408 |
12 |
23
|
IrP2@PNPC-NF |
94 |
17.8 |
−0.2 |
∼−0.7 |
−0.1246 |
50 |
26
|
Au1Cu1/GCE |
154.91 |
54.96 |
−0.2 |
∼−0.04 |
−0.02198 |
12 |
15
|
Ru(III)-PEI@MWCNTs |
188.9 |
30.93 |
−0.1 |
∼−0.2 |
−0.06186 |
18 |
27
|
Non-noble metal |
Fe3C@C |
8.53 |
9.15 |
−0.2 |
∼−0.7 |
−0.06405 |
10 |
28
|
Mo2C/C nanosheets |
11.3 |
7.8 |
−0.3 |
−4.5 |
−0.351 |
12 |
29
|
Mo thin films |
3.09 × 10−11 mol s−1 cm−2 |
0.72 |
−0.49 |
−2.75 |
−0.0198 |
9 |
30
|
Mo-FeP/CP |
13.1 |
7.49 |
−0.3 |
−0.15 |
−0.01124 |
10 |
31
|
BiNS |
13.23 |
10.46 |
−0.8 |
∼−0.12 |
−0.01255 |
12 |
32
|
MoS2/C3N4 |
18.5 |
17.8 |
−0.3 |
∼−0.8 |
−0.1424 |
14 |
33
|
FePc-Py-CNT |
21.7 |
22.2 |
−0.5 |
∼−0.1 |
−0.0222 |
50 |
34
|
2H-MoS2 |
21.8 |
31.6 |
−0.4 |
∼−3.2 |
−1.0112 |
16 |
35
|
ZnS2 |
23.7 |
15.7 |
−0.3 |
∼−0.4 |
−0.0628 |
9 |
36
|
Fe3O4@rGO |
28.01 |
19.42 |
−0.3 |
∼−0.05 |
−0.00971 |
10 |
37
|
Cr2O3 nanofiber |
28.13 |
8.56 |
−0.75 |
∼−0.2 |
−0.01712 |
12 |
38
|
Vr-ReSe2@CBC |
28.3 |
42.5 |
−0.25 |
∼−7 |
−2.975 |
20 |
39
|
450-NiMnO3 |
31.44 |
14.5 |
−0.3 |
∼−0.3 |
−0.0435 |
20 |
40
|
MXene@NiCoB |
38.7 |
6.92 |
−0.4 |
∼−1.3 |
−0.08996 |
16 |
41
|
Nb2O5 |
43.6 |
9.26 |
−0.55 |
∼−1 |
−0.0926 |
27 |
42
|
Fe2Mo6S8 |
70 |
12.5 |
−0.2 |
∼−0.2 |
−0.025 |
50 |
43
|
Fe3S4 nanosheets |
75.4 |
6.45 |
−0.4 |
∼−1.9 |
−0.12255 |
10 |
44
|
Mo2N |
78.4 |
4.5 |
−0.2 |
∼−2.7 |
−0.1215 |
30 |
45
|
NiFe@MoS2NCs |
128.17 |
11.34 |
−0.3 |
∼−0.5 |
−0.0567 |
15 |
46
|
CoPPi |
159.6 |
43.37 |
−0.2 |
∼−0.1 |
−0.04337 |
10 |
47
|
WSe2−x |
181.3 |
62.5 |
−0.5 |
∼−0.4 |
−0.25 |
30 |
48
|
GDY/Co2N |
219.72 |
58.6 |
0.101 |
∼−0.2 |
−0.1172 |
10 |
49
|
Atomically dispersed metal |
CNT@C3N4-Fe & Cu |
10.27 |
15.3 |
−1.2 |
∼−1.3 |
−0.1989 |
10 |
50
|
Ag4Ni2 |
23.32 |
78.97 |
−0.2 |
∼−0.1 |
−0.07897 |
10 |
51
|
D-Fe4-C |
24.8 |
15.8 |
−0.4 |
∼−0.2 |
−0.0316 |
20 |
52
|
FeMo/NC |
26.8 |
11.8 |
−0.6 |
∼−0.5 |
−0.059 |
10 |
53
|
FeSA-NSC |
30.4 |
21.9 |
−0.4 |
∼−0.7 |
−0.1533 |
30 |
54
|
AuSA/np-MoSe2 |
30.83 |
37.82 |
−0.3 |
∼−0.25 |
−0.09455 |
32 |
55
|
Mo/BCN |
37.67 |
13.27 |
−0.4 |
∼−0.4 |
−0.05308 |
16 |
56
|
U-SAs/TiO2 |
40.57 |
25.77 |
−0.55 |
∼−2.4 |
−0.61848 |
10 |
57
|
MnSA/GDY |
46.78 |
39.83 |
−0.045 |
∼−0.1 |
−0.03983 |
8 |
58
|
NC-Cu |
53.3 |
13.8 |
−0.35 |
∼−0.45 |
−0.0621 |
16 |
59
|
Cd/In2O3(VO) |
57.5 |
9.6 |
−0.43 |
∼−0.6 |
−0.0576 |
44 |
60
|
ISAS-Fe/NC |
62.9 |
18.6 |
−0.4 |
∼−1.6 |
−0.2976 |
22 |
61
|
Mn–O3N1/PC |
66.41 |
8.91 |
−0.35 |
∼−2.9 |
−0.25839 |
60 |
62
|
PdCu/NC |
69.2 |
24.8 |
−0.45 |
∼−0.75 |
−0.186 |
30 |
63
|
d-AuNCs/MXene |
88.3 |
9.3 |
−0.35 |
∼5 |
−0.465 |
54 |
64
|
Pd/HsGDY |
115.93 |
44.45 |
−0.25 |
∼−0.05 |
−0.02223 |
20 |
65
|
PdFe1 |
111.9 |
37.8 |
−0.2 |
∼−0.35 |
−0.1323 |
20 |
66
|
RuSAs/N-C |
120.9 |
29.6 |
−0.2 |
∼−0.44 |
−0.13 |
12 |
67
|
SA-Ag/NC |
270.9 |
21.9 |
−0.65 |
∼−1.8 |
−0.3942 |
40 |
68
|
Fe-(O-C2)4 |
307.7 |
51 |
−0.15 |
∼−0.1 |
−0.051 |
40 |
69
|
Ru@ZrO2/NC |
504 |
21 |
−0.21 |
∼−0.21 |
−0.0441 |
60 |
70
|
Fe–Co |
579.2 |
79 |
−0.3 |
∼−1.5 |
−1.185 |
10 |
71
|
Fe–MoS2 |
613.7 |
31.6 |
−0.2 |
∼−1.2 |
−0.3792 |
50 |
72
|
Metal-free |
NSG |
7.7 |
5.8 |
−0.6 |
∼−1.8 |
−0.1044 |
12 |
73
|
PCN |
8.09 |
11.59 |
−0.2 |
∼−0.65 |
−0.07533 |
16 |
74
|
d-FG/CP |
9.3 |
4.2 |
−0.7 |
∼−0.2 |
−0.0084 |
12 |
75
|
Eex-COF/NC |
12.53 |
45.43 |
−0.2 |
∼−0.1 |
−0.04543 |
20 |
76
|
BNS/CP |
13.22 |
4.04 |
−0.8 |
∼−0.15 |
−0.00606 |
12 |
77
|
N, B-PC |
16.4 |
10.58 |
−0.2 |
∼−0.1 |
−0.01058 |
12 |
78
|
MBN |
18.2 |
5.5 |
−0.7 |
∼−0.2 |
−0.011 |
12 |
79
|
S-CNS |
19.07 |
7.47 |
−0.7 |
∼−0.1 |
−0.00747 |
12 |
80
|
O-CN |
20.15 |
4.97 |
−0.6 |
∼−0.2 |
−0.00994 |
10 |
81
|
BCN-900 |
21.62 |
9.88 |
−0.3 |
∼−0.2 |
−0.01976 |
16 |
82
|
h-BNNS |
22.4 |
4.7 |
−0.75 |
∼−0.4 |
−0.0188 |
12 |
83
|
P-CNTs |
24.4 |
12.5 |
−0.3 |
∼−0.61 |
−0.07625 |
30 |
84
|
BP/CP |
26.42 |
12.7 |
−0.6 |
∼−0.25 |
−0.03175 |
16 |
85
|
B4C |
26.57 |
15.95 |
−0.75 |
∼−0.08 |
−0.01276 |
14 |
86
|
S-G |
27.3 |
11.5 |
−0.6 |
∼−0.4 |
−0.046 |
12 |
87
|
SDG |
28.56 |
7.07 |
−0.85 |
∼−0.4 |
−0.02828 |
10 |
88
|
FL-BPNSs |
31.37 |
5.07 |
−0.7 |
∼−0.4 |
−0.02028 |
10 |
89
|
BG-1 |
54.87 |
10.2 |
−0.5 |
∼−0.4 |
−0.0248 |
10 |
90
|
F-D-BPene |
70 |
26 |
−0.5 |
∼−0.1 |
−0.026 |
16 |
91
|
C-ZIF |
87.51 |
10.2 |
−0.3 |
∼−0.2 |
−0.0204 |
10 |
92
|
B/N-C NS |
153.4 |
33.1 |
−0.4 |
∼−0.55 |
−0.18205 |
48 |
93
|
C-BN@600 |
204 |
16.7 |
−0.2 |
∼−0.028 |
−0.00468 |
10 |
94
|
It is commonly considered that the empty d orbital of transition metals can receive the lone pair in N2, while d-orbital electrons feed back to the antibonding orbital of N2. This “push and pull” action results in the catalytic activation of N2 by hundreds of transition metal compounds.11,12 The noble-metal electrocatalysts (red regions) exhibited good nitrogen activation ability with most of NH3 yield higher than 40 μg h−1 mgcat.−1, but their FEs were normally lower than 20% and current densities assigned to NH3 are not high due to the severe HER. Pd-based catalysts have the higher FE of 40% but mild current density resulting in low NH3 yield.13 For some precious metals with poor HER performance, such as Ag and Au,14,15 the FE of eNRR is up to 50–80%. Consequently, inhibiting HER on noble metal surfaces is one of the major challenges for eNRR. Additionally, the scant resources and high price increase the economic cost; therefore, noble metals are prepared into advanced nanostructures or atomically dispersed forms to improve their utilization. In particular, carbon-supported Ru active centers with K as the auxiliary agent showed high potential in industrial ammonia synthesis, sparking the interest of scientists in studying Ru-based catalysts.16 Subsequently, the new carbon-supported Ru-based catalyst with Ce and Ba as the accelerator was successfully applied to industrial ammonia synthesis operating at a lower reaction pressure by increasing the electron density of Ru nanoparticles.17 Moreover, considering the stability of carbon materials under continuous industrial ammonia production, researchers further focus on anchoring Ru on highly stable oxides such as Al2O3, CeO2, MgO, perovskites and composite oxides to synthesize catalysts with long-term stability and high activity. These industrial advances provide the inspiration for the development of eNRR electrocatalysts with improved economy.
Non-noble-metal-catalysts have the advantages of low costs and abundant resources, and are expected to be substitutes for precious metals in eNRR.95 The studies on non-noble-metal catalysts mainly focus on metal elements, oxides, chalcogenides, nitrides, carbides, phosphates, and their composites. Particularly, inspired by natural nitrogen-fixing enzymes, Mo-based materials are highly anticipated eNRR catalysts. Additionally, the development of Mo-based catalysts used in industrial ammonia synthesis, such as Co3Mo3N with Ce as the accelerator with long-term activity,96 has also promoted the research of Mo-based electrocatalysts. It has been reported that Mo thin films,30 MoS2,97 Mo2C,29etc. can electrocatalyze N2 reduction to ammonia under ambient conditions. Besides, Fe-based catalysts have been widely used in the Haber–Bosch process for more than a century, mainly classified as Fe–Al–K, Fe–Co, Fe–Co–Re and FeO catalysts. These catalysts are made from Fe3O4 and Fe as raw materials by adding different auxiliaries. Inspired by the above Fe-based catalysts, Fe is one of the earliest elements used in electrocatalytic nitrogen reduction processes. A variety of Fe-based catalysts, including Fe3O4,37 Mo-doped FeP,31 Fe3S4,44 Fe3C@C,28etc. are also popular catalysts for eNRR. The blue regions in Fig. 2 show the eNRR performance of non-noble metal-based catalysts. It can be observed that some of them (such as MoS2/C3N4, FePc-Py-CNT, Fe3O4@rGO) have higher FE than noble metals, indicating the enhanced inhibition of competitive HER. However, their current densities are low because of the limited intrinsic activation ability for N2, resulting in low ammonia yield rates. For some catalysts designed with specific active sites, such as CoPPi with microflower structures offered superior charge transfer during NH3 synthesis with good stability, WSe2−x with rich Se-vacancies suppressed H2 evolution and enhanced π-back-donation ability, and GDY/Co2N with unique p-electronic characters to optimally modify the Co–N surface bonding, possess both high FEs (40–70%) and ammonia yield rates (>150 μg h−1 mgcat.−1). It follows that the intrinsic activity of non-noble metal-based catalysts for eNRR is expected to be further enhanced on the basis of inhibiting HER, that is, maintaining the high FE value at large current density.
To improve the interfacial catalytic efficiency, it is desirable to downsize the particles to clusters or single atoms and increase the low-coordinated or unsaturated atoms for functional active sites.98 By embedding isolated atoms in substrates with higher specific surface area, such as two-dimensional materials (graphene, molybdenum disulfide, etc.) and three-dimensional porous carbon, the metal active sites possess maximized atomic utilization efficiency and lower coordination number, presenting excellent electrocatalytic eNRR activity.63,68,70 According to the number of active sites, atomically dispersed catalysts can be subdivided into single-atom catalysts (SACs), dual single-atom catalysts (DSACs), and atomic clusters. Most SACs have only a single active site, leading to difficulty in breaking the linear scaling relationships of intermediates.99 In addition, in order to maintain the dispersion of single atoms, most reported catalysts have very low metal loading (generally less than 1.5%), resulting in unsatisfactory nitrogen activation capacity.100 As shown in the green regions in Fig. 2, the eNRR FE and ammonia yield rate of SACs generally below 40% and 100 μg h−1 mgcat.−1, respectively. It is noteworthy that DSACs constructed with controllable coupling of different compositions have strong synergistic effect between metal atoms, and increased metal loadings, exhibiting outstanding eNRR activity.101 The NH3 yield rate of Fe–Co71 and Fe–MoS2
72 bimetallic active site catalysts can even reach up to about 600 μg h−1 mgcat.−1. For some noble-metal atomic clusters, such as d-AuNCs/MXene and Pd/HsGDY can induce high NH3 yield (80–120 μg h−1 mgcat.−1).
Nonetheless, with increasing the surface free energy, the atomically dispersed metals are easily aggregated during electrocatalysis, which poses a challenge to the long-term durability of these catalysts for eNRR. Metal-free catalysts with poor HER activity also have been applied to eNRR, and heteroatom-doped carbon materials are the hotspot. However, their nitrogen fixing ability is weak with low FE and small current density due to the outermost electrons of the nonmetallic elements are in the s or p orbitals. Interestingly, B with the semi-metallic properties exhibits reactive lone pair electrons and empty orbitals, enabling it to generate sp3 hybridization and form a B–N back-donation p-bond, which is similar to the interaction of N2 with the d orbital electrons of metals.102 Black phosphorous with abundant unpaired electrons shows outstanding nitrogen reduction performance. Hence, it is also necessary to regulate the orbital and electronic properties of the catalyst in space and energy to facilitate the activation of N2.
Overall, the currently developed nitrogen reduction catalysts are rich and cost-effective materials. However, note that most stability tests of the catalysts reported above were maintained in a few hours (<60 h), which is far from the commercial goal. Learning from the boosting strategy in industrial ammonia synthesis catalysts, the suitable additives and carriers may further improve the long-term operation and reduce the economic cost of the eNRR electrodes.
3.2. Electrolytes
At present, the electrolytes used for electrocatalytic nitrogen reduction are usually aqueous solutions and non-aqueous protic solvents. In aqueous solutions, acidic (such as 0.1 M/0.01 M HCl solution), neutral (such as 0.1 M Na2SO4 solution, 0.1 M phosphate buffer saline solution), or alkaline (such as 0.1 M KOH solution) electrolytes are selected for eNRR according to various catalysts and application purpose. Specifically, acidic electrolytes are suitable for precious metals catalysts and non-metal catalysts that can maintain structural stability without corrosion during electrocatalysis. For alkaline and neutral electrolytes, the FE of eNRR in these two systems may be higher than that in acidic systems due to the lack of direct proton sources for competitive HER. However, it should be emphasized that the buffer solution is recommended to keep the pH value stable in the neutral electrocatalysis, and the current density is generally low in the neutral electrolyte because of the large resistance of the solution. In non-aqueous protic solvents, it can promote nitrogen solubility and inhibit hydrogen evolution. Some ionic liquids,103–105 tetrahydrofuran (THF),106–109 dimethyl sulfoxide (DMSO), etc., have been developed to provide a more favorable reaction environment to suppress HER and achieve high selectivity for eNRR. However, the poor ion conductivity and insufficient proton supply limit the ammonia yield. In addition, most of these non-aqueous protic solvents are organic products, leading to the inevitable introduction of NH3 or nitrogenous substances in their production process and generating trace residues in these solvent products. Therefore, pretreatment is required to eliminate contamination. In the process of laboratory research, the appropriate reference electrode and ammonia collection method should be selected to match the pH value of the electrolyte to ensure the accuracy of the experimental results in either electrolyte.
3.3. Electrolytic cells
Fig. 3 shows five types of electrolytic cells used in the research of electrochemical eNRR, which are single-chamber cells, H-type cells, back-to-back cells, polymer electrolyte membrane (PEM)-type cells, and electrochemical flow cells.68,110 The single-chamber and H-type cells are filled with liquid electrolytes, and the direct contact between the catalyst and electrolyte leads to the formation of gas–liquid–solid three-phase interface, resulting in serious competitive HER and insufficient supply of N2 in the cathode. They are widely applied in current laboratory research due to the simple device and easy operation, which focus on designing advanced catalysts with excellent inhibition of HER, and screening suitable electrolytes. Back-to-back cells and PEM cells are structurally conducive to inhibiting hydrogen evolution because their cathodes are both solid–gas electrochemical reactions. In a back-to-back cell, the two porous electrodes, which are composed of gas diffusion layer (GDL) and catalysts, respectively, are often separated by proton or cation exchange membrane. In this electrocatalytic process, the N2 is supplied to the cathode as feedstock, but the proton source is limited. In contrast to the back-to-back cell, PEM-type cells have better ion conductivity because the electrolyte is filled in the anode. During the PEM cell operation, protons can be generated by hydrolysis in the liquid chamber, and transferred to the solid cathode to catalyze the conversion of N2 to NH3. In particular, Fig. 3e reveals a flow cell similar in structure to the single-chamber cell, containing a flowing electrolyte filled in two chambers, the cathodic GDL, the proton exchange membrane, and the anode either with a GDL or not.110 Introduction of the flowing electrolyte is beneficial to promote mass transfer and control reaction environment.
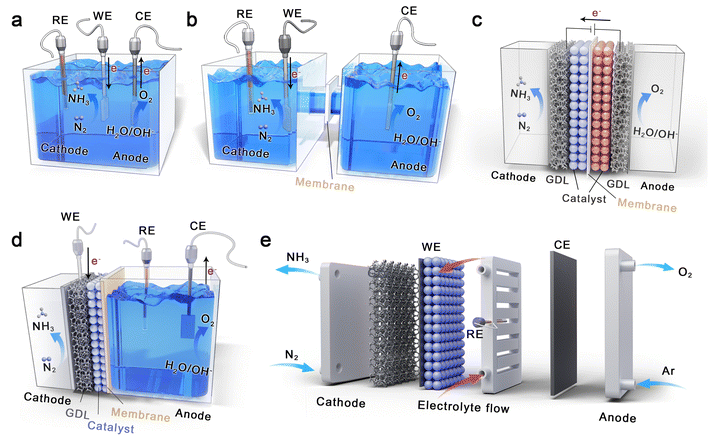 |
| Fig. 3 Schematic illustrations of different electrochemical reaction cells for eNRR under ambient conditions. (a) A single-chamber cell. (b) An H-type cell. (c) A back-to-back cell. (d) A PEM-type cell. (e) An electrochemical flow cell (GDL = gas diffusion layer, WE = working electrode, RE = reference electrode, and CE = counter electrode). | |
3.4. Determination methods
The quantitative detection method of electro-synthetic ammonia should be characterized by high sensitivity, high selectivity, and high precision due to the low ammonia output of eNRR. There are several quantitative methods used in current ammonia detection, including ion chromatography, spectrophotometry, fluorescence, ammonia gas-sensing electrodes, and nuclear magnetic resonance spectroscopy (NMR). Ion chromatography has the advantages of time saving, good reproducibility, and high sensitivity, but it is expensive and requires complex instruments. Spectrophotometry is widely used in the detection of ammonia because of its facile operation and high sensitivity of 10−1 μg mL−1 which includes Nessler's reagent method, indophenol blue method, salicylate method.111 In the former two methods, the potassium mercuric iodide, and hypochlorite and phenol react with ammonia under alkaline conditions to form colored compounds, which are strongly absorbed at the wavelength of 420 nm and 660 nm, respectively. The salicylate method is a modification of the indophenol blue method in which the safer sodium salicylate is used instead of phenol to react with ammonia, and the product is usually performed colorimetrically at a wavelength of 640 nm. Unlike spectrophotometry based on the absorption spectrum generated by the electron transition within a molecule, the fluorescence is the luminescence phenomenon when molecules absorb light into excited states and return to the ground state. Specifically, ammonia with o-phthaldialdehyde and sulfite results in a strongly fluorescent compound with maximum excitation and emission wavelengths at 362.5 and 423.0 nm, respectively. Compared with the former, the fluorescence method has higher sensitivity, better selectivity, but limited application scope.
In ammonia gas-sensing electrode method, aqueous ammonium chloride solution is usually used as an intra-electrode solution, which is separated from the sample solution by a hydrophobic gas permeable membrane. The ammonium salt is converted to NH3 by adding a strong alkali solution to the sample solution. The generated NH3 then diffuses across the membrane, changing the pH of the inner solution. The pH value is proportional to the ammonia concentration in the sample solution. The ammonia gas-sensing electrode is convenient and efficient, but it is sensitive to high concentration of dissolved metal ions in the electrolyte.112 NMR is another effective mean to quantitatively test the concentration of ammonia products. More importantly, it can be combined with isotope tracer experiments to qualitatively determine the nitrogen source in the products. It is strongly recommended to explore the source of N by NMR detection of isotopically labeled 15N2 products when the yield is below hundreds of orders of magnitude (μg h−1 mgcat.−1).
Additionally, various rigorous control experiments should be performed to eliminating the interference of potential ammonia nitrogen pollution sources, such as gas feedstocks, reaction devices, and electrode materials. For the reliability and accuracy of the results, at least two detection means should be achieved to take their average value, and the main experimental results should be repeated for more than three times.110 In addition, it is recommended that the NH3 yield is normalized both by the mass of the electrocatalyst and the geometric area of the electrode as descriptors for the eNRR performance.113
4. Designing efficient catalysts
Computations can be used to design catalysts reasonably and predict their catalytic performance theoretically because modifying the microstructure of the surface/interface of catalysts is a sensible way to realize the high-efficiency activation of N2. It has proven that the catalytic performance can be adjusted by the introduction of defect engineering, morphological engineering, and interface engineering, as well as the synergistic effect among several strategies. Compared with time-consuming trial-and-error experiments, theoretical calculation based on the Density Functional Theory (DFT) can rapidly predict the properties and guide the structural optimization of catalysts. In addition, machine learning (ML) methods enable high-throughput catalyst screening, providing a very convenient path for the design and construction of efficient eNRR catalysts. In this section, we will discuss the synergic development of theoretical calculation, machine learning and structural regulation strategies to screen and construct highly efficient eNRR electrodes (Fig. 4).
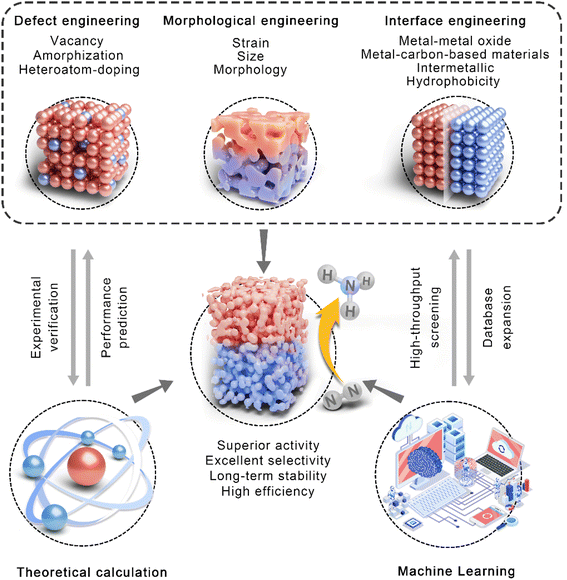 |
| Fig. 4 Schematic overview of theoretical calculation, machine learning, and defect, morphology, and interface engineering for efficient eNRR electrocatalysts. | |
4.1. High-throughput computations
The traditional method of trial and error was often used in the selection of catalysts, resulting in many disadvantages, such as low efficiency, high cost, long cycle, and low return. In recent years, high-throughput computations based on a large amount of data come lucrative in order to overcome the shortcomings of traditional trial and error methods. Through the high throughput calculation of the electronic structure, structural defects, and reactant interface of the catalyst, researchers can identify most promising candidates for experimental validation, reducing the time and cost needed for catalyst development. Furthermore, high-throughput computations can provide insights into the underlying mechanisms of catalytic processes, facilitating the design of novel catalysts with improved performance. For example, computational simulations can provide information on the reaction pathway, reaction kinetics, and the selectivity of the catalyst, promoting the optimization of the catalytic performance of the material. ML techniques can accelerate the discovery and screening of electrocatalysts, which could efficiently navigate the huge materials' space of electrocatalysts.114,115 Key reaction data, such as the adsorption and reaction energy, can be rapidly predicted with ML models trained by big data, which greatly facilitate the high-throughput screening and rational design of advanced catalysts.116
Nørskov group have theoretically calculated the free energies of the adsorption and further reduction of N and H atoms on transition metal surfaces in acidic electrolytes.117 It could be seen that most metals preferred to bind H atoms compared to N atoms. Mo, Fe, Rh, W, Ru, and Ir metals were located at the top of the volcano plot by dissociative mechanism, indicating higher eNRR activity. However, the adsorption capacity of these metals to H atoms was higher than that of N atoms, resulting in a lower selectivity of eNRR. Only the early transition metal surfaces including Ti, Zr, Y, and Sc had a stronger adsorption ability to N atoms than to H atoms, indicating their intrinsic inhibition of the HER process. The volcano plot provides a useful tool for predicting the activity of a catalyst based on its binding energies of adsorption and further reduction of N and H atoms.
Similarly, it was calculated that the initial potential of eNRR generated by associative mechanism on nanoclusters was higher than that HER.118 The optimal catalysts were Mo and Fe nanoclusters, which located at the top of the volcano plot. For transition metal single-atom catalysts, catalysts containing Mo single atoms had the highest activity because of the moderate adsorption energy for *N2H.119 Further, NiN, RhN, PdN and PtN had low initial potentials in nitrides that have been proved by most theoretical calculations for nitrogen reduction by the MVK mechanism.120 Additionally, the nitrogen reduction capacity of the oxide (110) crystal planes of 11 rutile structures was assessed, and IrO2 was the best catalyst for eNRR but the strong hydrogen adsorption capacity may poison the surface, while ReO2 and TaO2 had stronger adsorption energy for *NNH than *H.121 These studies demonstrate the potential for optimizing eNRR by selecting appropriate materials and structures, and the efficiency and selectivity of eNRR can be increased, contributing to the development of sustainable methods for nitrogen fixation and fertilizer production.
In general, high-throughput screening involves both theoretical computations and ML. DFT calculations are widely regarded as a powerful tool for screening catalysts with superb activity and selectivity for eNRR by estimating the ability of the catalyst to adsorb nitrogen and bind key reaction intermediates.8,122 While the ML based on the neural network potential of various key intermediates adsorbed by materials can be used to study the catalytic reaction mechanism in detail, screen relevant catalysts from a large number of candidate materials, and guide the progress of experimental synthesis.116 The two methods provide theoretical guidance for target catalysts as well as guiding each other. At the same time, the regulated catalyst expands back to the database of theoretical calculation and ML. Combining experimental data with computational simulations allows for the validation and refinement of theoretical models and provides more comprehensive understanding of the reaction mechanism and the catalyst's properties. Therefore, the synergy between experimental and theoretical research is crucial for advancing the field of eNRR catalysis.112,123 Understanding the underlying concepts from above aspects can optimize the design of catalysts and ultimately enhance their efficiency in ammonia production.
4.2. Defect engineering
Defects engineering refers to the introduction of defects on the surface of catalysts to adjust the electronic structure and enhance their catalytic activity. Defects can increase the number of active sites in the catalyst surface and improve the adsorption and activation of nitrogen. To date, a large number of defective nanomaterials (vacancies, amorphous phases, doping, etc.) have been prepared, showing great potential for eNRR.
Among many vacancy defects, oxygen vacancies (OVs) are prevalent due to their relatively low formation energy, which boost the catalytic activity of eNRR by exposing unsaturated metal sites and increasing nitrogen capture for electrocatalytic reaction.110 CeO2 has been widely used as an electrocatalytic nitrogen reduction catalyst due to its excellent properties, such as its large number of OVs, easy Ce3+/Ce4+ redox cycles, and good chemical stability.124,125 In addition to OVs, nitrogen vacancies (NVs) can also be used effectively for eNRR due to their unique N2 adsorption properties and HER suppression activity.126,127 As shown in Fig. 1e, VN, ZrN, NbN and CrN can be employed to produce ammonia via MVK mechanism. The N atoms on the surface of the metal nitride are hydrogenated into NH3 molecules and desorbed on the surface of the catalyst to form a NV, adsorbing the nitrogen molecules dissolved in the electrolyte. The surface NV may be replenished by nitrogen atoms in the bulk phase of the nitride or prone to inactivation upon the adsorption of an N2 molecule, resulting in poor stability. Based on the results of electrochemical experiments and theoretical calculations, it has been proved that NVs on 2D metal nitrides are stable by virtue of the high valence state of metal atoms and 2D confinement effect.126,127 Additionally, it is potentially achievable that favorable N2 adsorption and activation on NV-enriched metal-free catalysts.52,128–130 Similarly, S vacancies, Se vacancies, etc. are also expected to afford remarkable catalytic properties for eNRR by exposing extra unsaturated metal sites for N2 adsorption and activation.48,131 Therefore, the vacancies can effectively activate N2 and facilitate electron transfer during eNRR, enhancing NH3 yield and FE.
Structural optimization of catalysts through amorphous engineering will expose rich active sites and induce new defective sites to enhance electrocatalytic activity.132–134 The amorphous Bi4V2O11/CeO2 contains high-valence Bi defects and enriched OVs, reducing the reaction energy barrier, with a 3-fold increase in NH3 yield compared with crystalline Bi4V2O11/CeO2.133 Heteroatomic doping usually leads to local charge redistribution, which can not only activate the dopant or neighboring atoms to become active sites, but also promote the charge transfer from the active site to the adsorbed reactant, thereby accelerating the ammonia electrosynthesis even at very low doping levels.95 It has been certified that introducing metal elements into the materials can effectively adjust the electronic structure and generate additional unsaturated metal sites to improve eNRR performance, especially the formation of a heterojunction between carbon materials and metal catalysts can significantly enhance the catalytic performance of eNRR electrocatalysts.95,135 In addition, nonmetallic doped carbon materials have good catalytic activity for nitrogen fixation by promoting the N2 adsorption and N
N bond elongation, boosting the electron transfer and creating more defective/active sites.73,92 Particularly, high-efficiency ammonia electrosynthesis is achieved via a two-step method, that is, N2 is oxidized to NOx− assisted by plasma-assisted defects, and then NOx− is highly selective converted to NH3 by electrocatalytic reduction.136–139
4.3. Morphological engineering
Optimization of catalyst morphology, size, strain engineering, etc. can also dramatically enhance the electrochemical nitrogen fixation. Generally, the morphology regulation makes a large specific surface area for the catalyst, which remarkably increases the density of catalytic active sites and thus facilitates the catalytic reactions. Hollow Au nanocages had a 3-fold improvement in FE compared with solid Au nano-cubs, indicating that the improved reaction rates due to increased surface area and confinement effects.140 By regulating the size of the eNRR electrocatalyst, low coordination sites can be generated in the originally densely packed sites, which affects the bond formation strength of the reaction intermediate and thus the selectivity and activity of the catalyst.134,141 Under the condition of the same quality of Au anchored on a bisubstrate of CeOx and RGO (namely Au/CeOx–RGO), Au/CeOx–RGO with good dispersion and small particle size showed that the NH3 yield was 4 times higher than that of Au/CeOx with larger Au NPs particles and less dispersion.142 Moreover, this strategy can lessen the use of reactive metals, thereby reducing the production cost. Particularly, it is believed that the evolution from nanoparticles and clusters to single atoms maximizes the electrocatalytic efficiency and utilization of noble metals, resulting in higher NH3 yield and FE.143
In recent years, strain engineering techniques have been used to adjust the atomic distance and electron interaction of catalysts and then improve the intrinsic activity of catalysts. The delocalization and band broadening of 6p electrons in a Bi lattice matrix caused by strain compression engineering, promote the chemical affinity of intermediates and the activity and selectivity of NO3− conversion to NH3.144 Oppositely, the oxygen-induced tension field designed in Rh nanoclusters could inhibit HER and effectively reduce the energy barrier of hydrogenation of intermediates during the transition from nitrate to ammonia.145 Similarly, controlling the strain to modulate the electronic structure of transition metal oxides will enhance binding strength with the reaction intermediate and facilitate the electrocatalytic eNRR.
4.4. Interface engineering
Interface engineering is also a feasible approach to improve the catalytic activity of electrocatalysts by exposing more active sites at the interface and accelerating electron transport at the interface.146 The strong modulation ability for the chemical adsorption of reaction intermediates on the surface of the catalyst effectively accelerates the kinetic process of nitrogen reduction through the synergistic mechanism.147
The metal (derivative)-metal oxide interface has a strong interface interaction and plays a crucial role in improving catalytic activity. Regulating metal oxide interface is also a feasible and effective method to solve dynamic problems in eNRR. The electronic interactions of metal and metal oxide elevate the d-state electron center, enabling strong back-bonding for N2 molecules and improving the selectivity and activity of eNRR.148,149 Combining of metal (derivative) catalysts and carbon-based materials to form fine interface engineering has been demonstrated as an effective method to improve the performance of eNRR. Especially, combining graphitic materials and transition metal nanoparticles or other compounds has received more attention in the field of catalysis because of the unique d–π/d–p interactions.49,147,150
Through reasonable design of intermetallic compounds, the active sites with strong stability were implanted in the lattice frame to modify the N2 activated charge states.151 Recently, intermetallic compounds have been widely used in the field of electrocatalytic nitrogen reduction.152–156 Binary alloys with lower formation energies for the *N–N transition state generated a higher turnover frequency, i.e., the activity of Mo2/Ag was even higher than that of the Ru catalyst under industrial conditions by using DFT calculations and microkinetic modeling.157 Further, both experiments and calculations showed that the apparent activation energy of N2 on the ternary intermetallic compounds La-TM-Si (TM = Co, Fe, and Mn) was significantly lower than that of conventional Ru catalysts, indicating that the specific electronic structure and atomic configuration of intermetallic compound catalysts greatly promoted the dissociation of N2.158
However, HER is dominant with the high concentration of electrons or protons near the active site.159 Thus, reducing the concentration of electrons or protons near the catalyst will theoretically significantly improve the selectivity of the reaction. Considering that electron concentration is difficult to control, the catalyst surface will be coated with a layer of a hydrophobic protective layer, which can dynamically slow down the diffusion process of protons to the catalyst surface and improve the selectivity of the reaction, such as bilayer hydrophobic carbonized bacterial cellulose layer39 and self-assembly of hexyl mercaptan hydrophobic monolayer.160 Homoplastically, an N2-microextractor (consisting of polymer framework and THF extractant)161 has the high solubility for N2 and continuously feeds N2 into the catalyst, greatly restraining the occurrence of HER and increasing the FE (80.1%) of eNRR.
5. Optimization of the cell system
It remains a great challenge to achieve applicable ammonia yield by electrochemical synthesis only relying on the modification of eNRR catalysts. The overall optimization from the perspective of electrochemical reaction engineering can further improve the efficiency of ammonia synthesis, in which several aspects need to be considered comprehensively (Fig. 5).
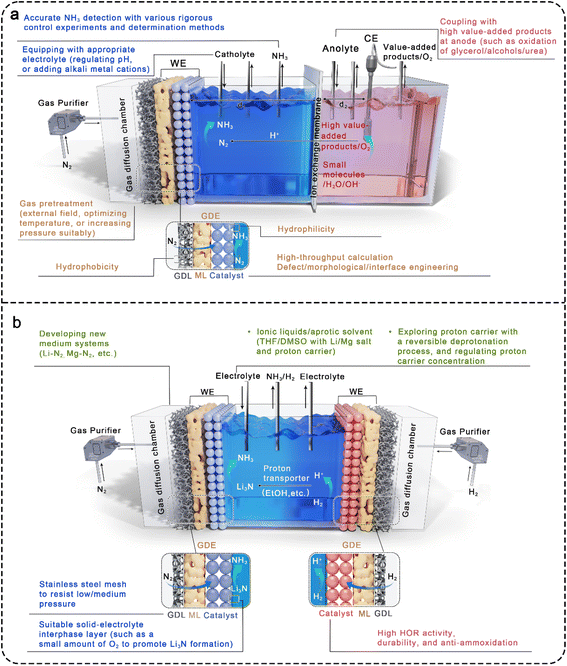 |
| Fig. 5 Schematic diagram of optimization strategies for the reaction cell in (a) aqueous and (b) non-aqueous solutions to improve electrochemical eNRR. | |
5.1. Improvement of cell structures
The electrocatalytic reaction cells provide a stable working environment for eNRR, and their structure directly determines the connection mode of the anode/cathode chambers and the arrangement of electrodes. Therefore, it is necessary to reasonably design the structure of electrolytic cells in the practical application environment. According to the five types of electrolytic cells in Fig. 3, the PEM cell has improved proton supply compared with the back-to-back type, but the proton transfer rate is limited by the lack of electrolytes in the cathode chamber. For reference to the characteristics of H-type electrolytic cells, adding a gas diffusion chamber and regulating the volume of the cathode chamber filled with electrolytes, that is, fine-tuned PEM-type cells with separated gas chamber and liquid cathode chamber, is a promising device. Theoretically, both nitrogen and proton supply can be taken into account in the designed cell, with the advantages of N2 adsorption and activation at the gas–solid interface maintained. Herein, it is necessary to emphasize the influence of distance regulation between electrode and membrane (d1 and d2 in Fig. 5a) on proton transport and input voltage, as well as the different requirements of hydrophilicity on both sides of the gas diffused electrode (GDE).
The GDE is structurally composed of a GDL, a microporous layer (ML), and a catalyst layer (CL). The gas diffusion side is required to meet high hydrophobicity to promote nitrogen diffusion at the gas–solid interface and maintain the separation of the gas chamber and the liquid cathode chamber. However, the catalyst side that contacts the electrolyte needs a certain hydrophilicity to ensure proton transport, and it can be considered to introduce proton transfer groups (such as –SO3H) into the catalyst composition. To strengthen the interface process at the electrode, a bionic strategy has been used to construct “Janus” electrodes by using gas-philic ultra-thin porous Bi5O7I nanotubes and hydrophilic carbon spheres, which could effectively improve the N2 concentration on the catalyst surface, weaken the hydrogen evolution process, promote the release of reaction products, and accelerate the electrocatalytic N2 fixation process.162 However, the non-aqueous electrolytes can penetrate into the carbon fiber, flooding the GDL and hindering gas diffusion. To address this problem, stainless steel mesh is often used to replace the carbon fiber skeleton, while a certain pressure is applied in the gas chamber to maintain the three-phase interface, promoting efficient electrochemical nitrogen fixation reaction.163 Moreover, increasing pressure suitably or optimizing temperature can significantly enhance the adsorption and activation of N2 at the gas–solid interface.
Actually, an external field (such as plasma,164 electricity, light, magnetism) can be added to activate N2 prior to the inlet, further solving the chemical inertness of N2 and boosting the kinetics of eNRR.
5.2. Mediator development
In fact, it is a great challenge to electrochemical reduce nitrogen to ammonia by providing H+/e− pairs at sufficient overpotentials without generating the by-product of H2. A possible approach to avoid the hydrogen evolution is to decouple nitrogen fixation and protonation by producing mediators (such as transition-metal compounds, metal-free substances, and enzymes) as a bridge between the two separate steps. In particular, some alkali metal-mediated systems, such as Li–N2,107,165 Al–N2,166 and Mg–N2
167 systems have also been reported. Among them, lithium has been the widely investigated mediator for the electroreduction of N2 to NH3, where the highly active Li metal reacts with N2 to form lithium nitride (Li3N) intermedia, and the subsequent facile protonation of Li3N produces NH3 in protonic solvents. In this approach, Li metal can be obtained by electroplating in the Li+ electrolyte, and the Li salts are regenerated with the formation of Li-mediated NH3 molecules, forming a cyclic process. One hand, it is found that the FE of NH3 is proportional to the pressure of N2via dynamic transport model. On the other hand, the performance of Li-mediated N2 reduction (LiNR) can be regulated by the proton carrier concentration. Taking EtOH as an example, when the concentration is lower than 0.1 M, the FE of NH3 decreases due to the insufficient supply of H+ by EtOH. However, the reaction between metal Li and EtOH becomes intense at higher concentrations, resulting in a weaker selectivity of LiNR. Currently, the LiNR to NH3 has been developed to continuous-flow electrosynthesis in non-aqueous electrolytes containing Li salts and proton donors. As shown in Fig. 5b, the Li-mediated flow cell is typically operated in a single electrolytic chamber because of the poor ion conduction in non-aqueous electrolytes. During electrocatalysis, the Li salts are reduced to Li metal without the formation of H2 under super negative potential, and Li metal reacts with interfacial N2 to form Li3N that is protonated instantaneously by the proton carrier. An important factor affecting the FE in the LiNR system is the solid electrolyte interface (SEI) that forms during Li electrodeposition.168 It has been proven that a small amount of O2 can act as accelerators to improve both the FE and stability of the LiNR system by slowing the Li+ diffusion in the SEI layer and reducing the electron consumption caused by Li deposition.169 Besides that, in some LiNR studies, a sacrificial solvent (such as ethanol) was used as a proton source, facing challenges in scaling up production in batch reactors. By contrast, LiNR paired with hydrogen oxidation reaction (HOR) on the anode can convert a sacrificial solvent to a proton shuttle and lower the input voltage to boost the energy efficiency of ammonia production.109 Under this operational mode, stringent demands are placed on the electrocatalytic selectivity of the anode, requiring materials with high HOR activity while maintaining resistance to ammonia oxidation in organic electrolytes. Following the example of the LiNR system, other metal mediators, such as low-electronegativity Zn, are currently being extensively investigated.
5.3. Electrolyte optimization
Electrolytes are the main place for ion transfer in electrocatalytic reactions. In the eNRR electrocatalysis based on aqueous electrolytes, the pH value, composition, viscosity, and other parameters of the electrolytes have great influence on the FE and yield of ammonia production.
The effect of pH value on the eNRR performance of catalysts has been investigated in recent studies.170–172 The change of pH has little influence on ammonia production rate, but the FE increases significantly with increasing the pH value. The main reason is that HER is blocked by low proton concentration in an alkaline environment, resulting in higher FE of N2 reduction than that in an acidic medium. In the Haber–Bosch process, potassium oxide as an auxiliary agent can donate electrons to weaken the N
N bond and promote the desorption of ammonia from the catalyst surface.173 Inspired by this, alkali metal ions, i.e., lithium174 and potassium175,176 were added into the aqueous solution to restrain the HER, stabilize the reaction intermediate, and improve the reaction selectivity. Studies have shown that a high FE of 66% and an order of magnitude improvement of ammonia yield of 3400 μg h−1 mgcat.−1 could be achieved by stabilizing key intermediates (*NNH) with potassium ions and regulating proton transfer to increase selectivity in an aqueous electrolyte under ambient conditions.175 In addition, the proton transfer process can be affected by adjusting the concentration of electrolytes. It has been proved that a high concentration of LiCl solute ions exhibited a strong affinity for surrounding water molecules, limiting the role of water as a proton source and solvent.174 This result effectively inhibits the hydrogen evolution and promotes the N2 enrichment at the reaction interface, which increases the selectivity (FE of 71 ± 1.9%) and the activity (a yield of 58.14 μg h−1 mgcat.−1) of eNRR.
For the LiNR system, the moderate polar aprotic solvents, such as THF with high N2 solubility (6 mmol L−1, 25 °C), are widely used as electrolytes.177 The hydrogen evolution is inhibited in the organic electrolytes, but additional proton donors are essential for the formation of ammonia. However, the commonly used small molecular solvents are oxidized at the anode, which as sacrificial agents cannot sustainably provide protons. In addition to the mentioned strategy that couples HOR in the anode, some studies have been performed to optimize the composition of electrolytes. Notably, a proton carrier [P6,6,6,14]+ with higher chemical stability, electrochemical stability and thermal stability has been introduced in the LiNR system, which could operate as a reversible deprotonation process, so as to sustainably transport protons between the cathode and anode.165 Meanwhile, the electrolytes containing [P6,6,6,14]+ have increased N2 solubility, and the LiNR system with [P6,6,6,14]+ can achieve an ammonia production rate of 53 ± 1 nmol s−1 cm−2 and a FE of 69% ± 1% under 0.5 bar hydrogen and 19.5 bar nitrogen. Inspired by the inherent differences in the proton-supplying capacity of alcohol and water, an alcohol–water electrolyte system was developed to regulate local proton concentrations and microenvironments at the electrode–electrolyte interface, where water availability and dissociation processes were greatly limited, inhibiting HER within a wide electrochemical window.178 Particularly, in the methanol-containing electrolyte system, FE reached a record of 75.9 ± 4.1% and NH3 yield was 262.5 ± 7.3 μg h−1 mgcat.−1 for eNRR.
5.4. High value-added products at anode
Similar to industrial electrolytic hydrogen production, the efficiency of eNRR to ammonia synthesis is also affected by the reaction kinetics of the counter electrode (the anode), which is a common feature of electrosynthesis. In aqueous electrolytes, the anodic reactions of electrochemical ammonia synthesis and water splitting are slow-kinetic oxygen evolution reactions with four-electron transfer process. The electrolytic voltage of the electrolytic cell is mainly determined by the rate-limiting oxygen evolution reaction (OER), and the value of oxidation products (O2) is of little significance.179 Recently, several effective measures have been developed to replace oxidation of water with more thermodynamically favorable electrooxidation reactions of small molecules (hydrazine, alcohol, urea, primary amine, etc.) to improve the efficiency of water splitting for hydrogen production.180–183 Similarly, the energy saving strategy of eNRR to NH3 can also be realized by coupling more energetically favorable oxidation reactions and obtaining high value-added products at the anode, which is expected to improve the energy efficiency of ammonia synthesis and increase economic benefits. Some of these reactions, such as sodium gluconate oxidation reaction,184 5-hydroxymethylfurfural oxidation,27 and glycerol oxidation reaction185 have been successfully demonstrated to reduce the overall electrolytic voltage with high ammonia yield. Moreover, the multifunctional catalytic activity of the electrocatalyst will also reduce the cost and time. A ruthenium(III) polyethyleneimine (Ru(III)-PEI) difunctional catalyst supported on carboxyl-modified carbon nanotubes (Ru(III)-PEI@MWCNTs) could induce both high FE of 30.93% for eNRR in the cathode and excellent FE of 94% for 2,5-furandicarboxylic acid production in the anode, with the cell voltage of 220 mV lower than that coupling with OER.27 Also, OER was replaced with the reaction of oxidizing sodium gluconate to gluconic acid, the voltage required by the system was reduced to 400 mV without affecting on the eNRR activity.184 These proposals provide a promising pathway to obtain both NH3 and valuable organic chemicals with high efficiency and economic benefit.
6. Conclusion and perspective
In conclusion, the unremitting efforts of academic researchers, from quantification of ammonia products and precise characterization of nitrogen sources to design and preparation of catalysts, optimization of electrolytic cell structure, and development of new nitrogen reduction systems, are briefly summarized and discussed in this perspective. Despite the considerable progress of eNRR, challenges remain to reach the practical targets for ammonia yield and electrosynthesis efficiency. It is far away from the U.S. Department of Energy targets that electrochemical synthesis techniques should achieve the current density >300 mA cm−2, faradaic efficiency >90%, and the full cell energy efficiency >60%.186 To achieve practical application of electrochemical ammonia synthesis, it is necessary to optimize the device on the basis of in-depth study on the catalytic mechanism and structure–activity relationship.
6.1. Energy efficiency needs to be improved
Most current studies in the field primarily focus on enhancing the FE of nitrogen reduction and the yield of ammonia through catalyst design, electrolyte selection, and electrode optimization. However, these studies often overlook the power cost and energy efficiency aspects. Although alternative anode reactions can generate high-value chemicals and offer attractive economic advantages, electro-oxidation processes of organic compounds are typically more complex, involving multi-electron processes compared with the four-electron OER. These oxidation reactions generally exhibit low thermodynamic potential but suffer from sluggish kinetic processes. Consequently, improving the ammonia yield at low overpotential is limited in this system, and the high resistance of the organic matter at the anode becomes a significant concern. Furthermore, only a few estimations have been made regarding the energy efficiency of ammonia synthesis.
For the Li-mediated system, the FE can be markedly increased, but the reduction of Li+ requires additional energy, resulting in more energy consumption in the whole process. It has been reported that the power consumption of eNRR systems coupled with OER was about 10.5 MW h per tonne with at least 2.0 V of the overall cell.5 Though the Li-mediated system coupled with a continuous flow electrolytic cell and anodic hydrogen oxidation achieved a high FE of up to 61 ± 1%, its energy efficiency was only 13%.109 Therefore, it is encouraged to estimate the energy efficiency in various eNRR systems in future studies to stimulate rapid development of green ammonia synthesis.
6.2. Screening electrocatalysts with high selectivity and stability
Electrocatalysts play a crucial role in achieving efficient nitrogen fixation. Currently, the development of catalysts heavily relies on chemical experience and intuition, which involves a time-consuming trial-and-error process within a vast materials space. This poses a significant challenge for the efficient screening of advanced catalysts. To address this, key descriptors based on adsorptive free energy of intermediates, calculated on basis of DFT, have been widely used to rapidly predict the nitrogen reduction activity of catalysts. However, certain approximate calculations are limited to specific surfaces, and the scaling relationships often restrict catalyst performance, leading to inconsistent predictions when compared with experimental studies. This limitation hampers their broad application, highlighting the need to break the scaling relationships for the discovery of promising electrocatalysts.
Additionally, machine learning holds promise in optimizing the collection and analysis of high-dimensional data, uncovering hidden statistical patterns that can aid in the selection of efficient electrocatalysts from a large pool of candidate materials. This, in turn, can guide advancements in catalyst design, experimental synthesis, and more. However, constructing a comprehensive “big data” framework through high-performance computing and conducting thorough analyses of experimental results present attractive yet challenging engineering goals. Moreover, establishing universal machine learning models applicable to different eNRR systems is an inevitable requirement for future development.
6.3. Combining in situ techniques with advanced calculations to clarify electrocatalytic mechanisms
Understanding the electrocatalytic mechanism is crucial for guiding the design of electrolytic cells, electrolytes, and catalysts. In recent years, with advancements in science and technology, various in situ characterization techniques have emerged. These techniques, such as in situ infrared spectroscopy, in situ Raman, differential electrochemical mass spectrometry (DEMS), in situ XRD, in situ synchrotron radiation, quasi-in situ XPS, and Operando XAS, have been utilized to monitor reaction intermediates and dynamic changes in catalysts. They provide valuable insights into reaction mechanisms and help identify active sites. However, several challenges remain.
For instance, in situ infrared monitoring of nitrogen-containing intermediates is not ideal due to the influence of electrolytes. Additionally, current theoretical calculations primarily focus on the thermodynamic aspects of eNRR mechanism, neglecting kinetic factors. Moreover, theoretical calculations often serve the purpose of validating field monitoring analyses or eNRR performance testing, rather than making predictions for macroscopic experiments that may not be conducted. It is essential to develop computational models that incorporate time-dependent changes, employing molecular dynamics simulations to accurately capture the dynamic catalytic mechanism. Furthermore, advanced computational methods, such as the solvation model, should be developed to better mimic real experimental conditions, surpassing the limitations of traditional computational hydrogen electrode models. To enhance prediction accuracy, the optimized model must consider the three-phase interface of gas, catalyst, and electrolyte.
The design of theoretical models should be integrated with experimental conditions to bridge the gap between theoretical predictions and experimental monitoring in real electrochemical environments. This will lead to a more comprehensive understanding of electrocatalytic processes.
6.4. Advanced membrane technology
Currently, Nafion membrane with perfluorosulfonic acid as the main component is the usually used proton exchange membrane in eNRR cells.187 The hydrated protons in the solvent can move between the sulfonic roots of the branch chain to achieve proton conduction. Unfortunately, it has demonstrated that ammonia, like hydrating protons, could pass through Nafion membranes, transfer between the anode chamber and cathode chamber, and cause corrosion to the membrane, resulting in measurement errors in ammonia quantization and short operating cycles of the device in practical applications.188 Meanwhile, there are also some common characteristics with the current commercial water splitting. The ionic conductivity of the Nafion membrane is lower in an alkaline environment than that in an acidic medium, and also the price of proton exchange membrane is generally high. The development of low-cost, controllable structure and simple preparation process of non-fluoride anion exchange membranes is expected to solve the above problems. However, in the alkaline system, quaternary amine groups on the traditional quaternary amine anionic conduction membrane will bring Hoffmann elimination and nucleophilic substitution, resulting in poor stability of the membrane.189 If a new type of high-stability anion exchange membrane with appropriate proton and electron transport is exploited to replace the existing commercial membrane, it is believed to be of great significance for the continuous production of high-yield ammonia. In addition, with the development of nitrogen reduction research, the operation time of electrolytic devices tends to be extended under the condition of high current, and a lot of waste heat is generated. With water electrolysis devices, the efficient use of waste heat to boost energy efficiency is a challenging but attractive study. In this regard, nitrogen reduction devices driven by heat and electricity, such membrane reactor,190,191 may be a promising but less focused technology for ammonia synthesis.
In conclusion, electrochemical nitrogen reduction holds great potential for clean and environmentally friendly ammonia production with renewable electric energy. This approach offers the prospect of reducing global energy consumption and addressing impending food and environmental crises. Though the electrocatalytic synthesis of ammonia still faces challenges compared with the well-established Haber–Bosch process, significant progress has been made through ongoing research efforts. Moving forward, it is crucial to delve deeper into the reaction mechanism, develop catalysts with high performance and energy efficiency, optimize electrocatalytic cells, and explore innovative membrane technologies. These areas of exploration are key to realizing the industrial application of electrochemical ammonia synthesis under ambient conditions. By addressing these challenges and making advancements in these areas, we can pave the way for a sustainable and efficient method of ammonia production, contributing to a cleaner and more sustainable future.
Conflicts of interest
There are no conflicts to declare.
Acknowledgements
This work was supported by the National Natural Science Foundation of China (U21A20281).
Notes and references
- J. N. Galloway, A. R. Townsend, J. W. Erisman, M. Bekunda, Z. Cai, J. R. Freney, L. A. Martinelli, S. P. Seitzinger and M. A. Sutton, Science, 2008, 320, 889–892 CrossRef CAS PubMed.
- W. Liao, K. Xie, L. Liu, X. Wang, Y. Luo, S. Liang, F. Liu and L. Jiang, J. Energy Chem., 2021, 62, 359–366 CrossRef CAS.
- X. Wang, X. Peng, W. Chen, G. Liu, A. Zheng, L. Zheng, J. Ni, C.-T. Au and L. Jiang, Nat. Commun., 2020, 11, 653 CrossRef CAS PubMed.
- L. Li, Y.-F. Jiang, T. Zhang, H. Cai, Y. Zhou, B. Lin, X. Lin, Y. Zheng, L. Zheng, X. Wang, C.-Q. Xu, C.-T. Au, L. Jiang and J. Li, Chem, 2022, 8, 749–768 CAS.
- D. R. MacFarlane, P. V. Cherepanov, J. Choi, B. H. R. Suryanto, R. Y. Hodgetts, J. M. Bakker, F. M. Ferrero Vallana and A. N. Simonov, Joule, 2020, 4, 1186–1205 CrossRef CAS.
- R. F. Service, Science, 2014, 345, 610 CrossRef CAS PubMed.
- X. Peng, Y. Mi, H. Bao, Y. Liu, D. Qi, Y. Qiu, L. Zhuo, S. Zhao, J. Sun, X. Tang, J. Luo and X. Liu, Nano Energy, 2020, 78, 105321 CrossRef CAS.
- Z. Chen, C. Liu, L. Sun and T. Wang, ACS Catal., 2022, 12, 8936–8975 CrossRef CAS.
- M. A. Shipman and M. D. Symes, Catal. Today, 2017, 286, 57–68 CrossRef CAS.
- Y. Abghoui and E. Skúlason, Catal. Today, 2017, 286, 78–84 CrossRef CAS.
- M.-A. Légaré, G. Bélanger-Chabot, R. D. Dewhurst, E. Welz, I. Krummenacher, B. Engels and H. Braunschweig, Science, 2018, 359, 896–900 CrossRef PubMed.
- X. Rao, M. Liu, M. Chien, C. Inoue, J. Zhang and Y. Liu, Renewable Sustainable Energy Rev., 2022, 168, 112845 CrossRef CAS.
- W. Xu, G. Fan, J. Chen, J. Li, L. Zhang, S. Zhu, X. Su, F. Cheng and J. Chen, Angew. Chem., Int. Ed., 2020, 59, 3511–3516 CrossRef CAS PubMed.
- W. Liao, K. Liu, J. Wang, A. Stefancu, Q. Chen, K. Wu, Y. Zhou, H. Li, L. Mei, M. Li, J. Fu, M. Miyauchi, E. Cortes and M. Liu, ACS Nano, 2022, 17, 411–420 CrossRef PubMed.
- Y. Liu, L. Huang, X. Zhu, Y. Fang and S. Dong, Nanoscale, 2020, 12, 1811–1816 RSC.
- K.-I. Aika, H. Hori and A. Ozaki, J. Catal., 1972, 27, 424–431 CrossRef CAS.
- F. Rosowski, A. Hornung, O. Hinrichsen, D. Herein, M. Muhler and G. Ertl, Appl. Catal., A, 1997, 151, 443–460 CrossRef CAS.
- C. Chen, C. Liang, J. Xu, J. Wei, X. Li, Y. Zheng, J. Li, H. Tang and J. Li, Electrochim. Acta, 2020, 335, 135708 CrossRef CAS.
- B. Jiang, H. Xue, P. Wang, H. Du, Y. Kang, J. Zhao, S. Wang, W. Zhou, Z. Bian, H. Li, J. Henzie and Y. Yamauchi, J. Am. Chem. Soc., 2023, 145, 6079–6086 CrossRef CAS PubMed.
- C. Yang, B. Huang, S. Bai, Y. Feng, Q. Shao and X. Huang, Adv. Mater., 2020, 32, e2001267 CrossRef PubMed.
- N. Zhang, L. Li, J. Wang, Z. Hu, Q. Shao, X. Xiao and X. Huang, Angew. Chem., Int. Ed., 2020, 59, 8066–8071 CrossRef CAS PubMed.
- W. Zhang, L. Yang, C. An, J. Zhang, J. Zhu and P. Chen, J. Mater. Chem. A, 2020, 8, 25142–25147 RSC.
- Y.-J. Mao, F. Liu, Y.-H. Chen, X. Jiang, X.-S. Zhao, T. Sheng, J.-Y. Ye, H.-G. Liao, L. Wei and S.-G. Sun, J. Mater. Chem. A, 2021, 9, 26277–26285 RSC.
- L. Zhao, X. Liu, S. Zhang, J. Zhao, X. Xu, Y. Du, X. Sun, N. Zhang, Y. Zhang, X. Ren and Q. Wei, J. Mater. Chem. A, 2021, 9, 259–263 RSC.
- W.-Y. Gao, Y.-C. Hao, X. Su, L.-W. Chen, T.-A. Bu, N. Zhang, Z.-L. Yu, Z. Zhu and A.-X. Yin, Chem. Commun., 2019, 55, 10705–10708 RSC.
- X. Yang, F. Ling, X. Zi, Y. Wang, H. Zhang, H. Zhang, M. Zhou, Z. Guo and Y. Wang, Small, 2020, 16, e2000421 CrossRef PubMed.
- G.-R. Xu, M. Batmunkh, S. Donne, H. Jin, J.-X. Jiang, Y. Chen and T. Ma, J. Mater. Chem. A, 2019, 7, 25433–25440 RSC.
- M. Peng, Y. Qiao, M. Luo, M. Wang, S. Chu, Y. Zhao, P. Liu, J. Liu and Y. Tan, ACS Appl. Mater. Interfaces, 2019, 11, 40062–40068 CrossRef CAS PubMed.
- H. Cheng, L.-X. Ding, G.-F. Chen, L. Zhang, J. Xue and H. Wang, Adv. Mater., 2018, 30, 1803694 CrossRef PubMed.
- D. Yang, T. Chen and Z. Wang, J. Mater. Chem. A, 2017, 5, 18967–18971 RSC.
- Y.-X. Luo, W.-B. Qiu, R.-P. Liang, X.-H. Xia and J.-D. Qiu, ACS Appl. Mater. Interfaces, 2020, 12, 17452–17458 CrossRef CAS PubMed.
- L. Li, C. Tang, B. Xia, H. Jin, Y. Zheng and S.-Z. Qiao, ACS Catal., 2019, 9, 2902–2908 CrossRef CAS.
- K. Chu, Y.-P. Liu, Y.-B. Li, Y.-L. Guo and Y. Tian, ACS Appl. Mater.
Interfaces, 2020, 12, 7081–7090 CrossRef CAS PubMed.
- S. Xu, Y. Ding, J. Du, Y. Zhu, G. Liu, Z. Wen, X. Liu, Y. Shi, H. Gao, L. Sun and F. Li, ACS Catal., 2022, 12, 5502–5509 CrossRef CAS.
- P. E. P. Win, D. Yu, W. Song, X. Huang, P. Zhu, G. Liu and J. Wang, Small Methods, 2023, 7, 2201463 CrossRef CAS PubMed.
- R. Zhang, X.-H. Liu, D.-Y. Wu, H.-B. Wang, C.-Q. Cheng, Q.-J. Guo, J. Mao, T. Ling, C.-K. Dong, H. Liu, B.-Q. Zhang, X.-L. Zheng, L.-L. Han, J.-M. Zhang, A. Head, X. Tong, Z. Liang, J. Luo, H. L. Xin and X.-W. Du, ACS Catal., 2022, 12, 6809–6815 CrossRef CAS.
- F. Wang, L. Xia, X. Li, W. Yang, Y. Zhao and J. Mao, Energy Environ. Mater., 2021, 4, 88–94 CrossRef CAS.
- H. Du, X. Guo, R. M. Kong and F. Qu, Chem. Commun., 2018, 54, 12848–12851 RSC.
- F. Lai, W. Zong, G. He, Y. Xu, H. Huang, B. Weng, D. Rao, J. A. Martens, J. Hofkens, I. P. Parkin and T. Liu, Angew. Chem., Int. Ed., 2020, 59, 13320–13327 CrossRef CAS PubMed.
- H. Li, X. Xu, X. Lin, J. Chen, K. Zhu, F. Peng and F. Gao, Nanoscale, 2023, 15, 4071–4079 RSC.
- C. Wang, Q.-C. Wang, K.-X. Wang, M. De Ras, K. Chu, L.-L. Gu, F. Lai, S.-Y. Qiu, H. Guo, P.-J. Zuo, J. Hofkens and X.-D. Zhu, J. Energy Chem., 2022, 77, 469–478 CrossRef.
- J. Han, Z. Liu, Y. Ma, G. Cui, F. Xie, F. Wang, Y. Wu, S. Gao, Y. Xu and X. Sun, Nano Energy, 2018, 52, 264–270 CrossRef CAS.
- K. Lu, F. Xia, B. Li, Y. Liu, I. B. Abdul Razak, S. Gao, J. Kaelin, D. E. Brown and Y. Cheng, ACS Nano, 2021, 15, 16887–16895 CrossRef CAS PubMed.
- X. Zhao, X. Lan, D. Yu, H. Fu, Z. Liu and T. Mu, Chem. Commun., 2018, 54, 13010–13013 RSC.
- X. Ren, G. Cui, L. Chen, F. Xie, Q. Wei, Z. Tian and X. Sun, Chem. Commun., 2018, 54, 8474–8477 RSC.
- L. Zeng, X. Li, S. Chen, J. Wen, W. Huang and A. Chen, J. Mater. Chem. A, 2020, 8, 7339–7349 RSC.
- D. Gupta, A. Kafle and T. C. Nagaiah, Small, 2023, 19, 2208272 CrossRef CAS PubMed.
- P. Shen, X. Li, Y. Luo, Y. Guo, X. Zhao and K. Chu, ACS Nano, 2022, 16, 7915–7925 CrossRef CAS PubMed.
- Y. Fang, Y. Xue, Y. Li, H. Yu, L. Hui, Y. Liu, C. Xing, C. Zhang, D. Zhang, Z. Wang, X. Chen, Y. Gao, B. Huang and Y. Li, Angew. Chem., Int. Ed., 2020, 59, 13021–13027 CrossRef CAS PubMed.
- X. Wang, S. Qiu, J. Feng, Y. Tong, F. Zhou, Q. Li, L. Song, S. Chen, K.-H. Wu, P. Su, S. Ye, F. Hou, S. X. Dou, H. K. Liu, G. Q. Lu, C. Sun, J. Liu and J. Liang, Adv. Mater., 2020, 32, 2004382 CrossRef CAS PubMed.
- M. Han, M. Guo, Y. Yun, Y. Xu, H. Sheng, Y. Chen, Y. Du, K. Ni, Y. Zhu and M. Zhu, Adv. Funct. Mater., 2022, 32, 2202820 CrossRef CAS.
- Y. Kong, L. Wu, X. Yang, Y. Li, S. Zheng, B. Yang, Z. Li, Q. Zhang, S. Zhou, L. Lei, G. Wu and Y. Hou, Adv. Funct. Mater., 2022, 32, 2205409 CrossRef CAS.
- W. Cui, B. Geng, X. Chu, J. He, L. Jia, X. Han, X. Wang, S. Song and H. Zhang, Nano Res., 2022, 16, 5743–5749 CrossRef.
- Y. Li, Y. Ji, Y. Zhao, J. Chen, S. Zheng, X. Sang, B. Yang, Z. Li, L. Lei, Z. Wen, X. Feng and Y. Hou, Adv. Mater., 2022, 34, 2202240 CrossRef CAS PubMed.
- D. Chen, M. Luo, S. Ning, J. Lan, W. Peng, Y.-R. Lu, T.-S. Chan and Y. Tan, Small, 2022, 18, 2104043 CrossRef CAS PubMed.
- L. Shi, S. Bi, Y. Qi, R. He, K. Ren, L. Zheng, J. Wang, G. Ning and J. Ye, ACS Catal., 2022, 12, 7655–7663 CrossRef CAS.
- T. Chen, T. Liu, B. Pang, T. Ding, W. Zhang, X. Shen, D. Wu, L. Wang, X. Liu, Q. Luo, W. Zhu and T. Yao, Sci. Bull., 2022, 67, 2001–2012 CrossRef CAS PubMed.
- Y. Fang, Y. Xue, L. Hui, X. Chen and Y. Li, J. Mater. Chem. A, 2022, 10, 6073–6077 RSC.
- W. Zang, T. Yang, H. Zou, S. Xi, H. Zhang, X. Liu, Z. Kou, Y. Du, Y. P. Feng, L. Shen, L. Duan, J. Wang and S. J. Pennycook, ACS Catal., 2019, 9, 10166–10173 CrossRef CAS.
- Z. Yao, S. Liu, H. Liu, Y. Ruan, S. Hong, T. S. Wu, L. Hao, Y. L. Soo, P. Xiong, M. M. J. Li, A. W. Robertson, Q. Xia, L. X. Ding and Z. Sun, Adv. Funct. Mater., 2022, 33, 2209843 CrossRef.
- F. Lü, S. Zhao, R. Guo, J. He, X. Peng, H. Bao, J. Fu, L. Han, G. Qi, J. Luo, X. Tang and X. Liu, Nano Energy, 2019, 61, 420–427 CrossRef.
- L. Han, M. Hou, P. Ou, H. Cheng, Z. Ren, Z. Liang, J. A. Boscoboinik, A. Hunt, I. Waluyo, S. Zhang, L. Zhuo, J. Song, X. Liu, J. Luo and H. L. Xin, ACS Catal., 2020, 11, 509–516 CrossRef.
- L. Han, Z. Ren, P. Ou, H. Cheng, N. Rui, L. Lin, X. Liu, L. Zhuo, J. Song, J. Sun, J. Luo and H. L. Xin, Angew. Chem., Int. Ed., 2021, 60, 345–350 CrossRef CAS PubMed.
- X. Peng, R. Zhang, Y. Mi, H. T. Wang, Y. C. Huang, L. Han, A. R. Head, C. W. Pao, X. Liu, C. L. Dong, Q. Liu, S. Zhang, W. F. Pong, J. Luo and H. L. Xin, ChemSusChem, 2023, 16, e202201385 CrossRef CAS PubMed.
- Y. Guo, J. Liu, Q. Yang, P. Khemthong, Z. Huang, Y. Zhao, Z. Chen, B. Dong, X.-Z. Fu, J.-L. Luo and C. Zhi, Nano Energy, 2021, 86, 106099 CrossRef CAS.
- X. Li, P. Shen, Y. Luo, Y. Li, Y. Guo, H. Zhang and K. Chu, Angew. Chem., Int. Ed., 2022, 61, e202205923 CrossRef CAS PubMed.
- Z. Geng, Y. Liu, X. Kong, P. Li, K. Li, Z. Liu, J. Du, M. Shu, R. Si and J. Zeng, Adv. Mater., 2018, 30, e1803498 CrossRef PubMed.
- Y. Chen, R. Guo, X. Peng, X. Wang, X. Liu, J. Ren, J. He, L. Zhuo, J. Sun, Y. Liu, Y. Wu and J. Luo, ACS Nano, 2020, 14, 6938–6946 CrossRef CAS PubMed.
- S. Zhang, M. Jin, T. Shi, M. Han, Q. Sun, Y. Lin, Z. Ding, L. R. Zheng, G. Wang, Y. Zhang, H. Zhang and H. Zhao, Angew. Chem., Int. Ed., 2020, 59, 13423–13429 CrossRef CAS PubMed.
- H. Tao, C. Choi, L.-X. Ding, Z. Jiang, Z. Han, M. Jia, Q. Fan, Y. Gao, H. Wang, A. W. Robertson, S. Hong, Y. Jung, S. Liu and Z. Sun, Chem, 2019, 5, 204–214 CAS.
- S. Zhang, M. Han, T. Shi, H. Zhang, Y. Lin, X. Zheng, L. R. Zheng, H. Zhou, C. Chen, Y. Zhang, G. Wang, H. Yin and H. Zhao, Nat. Sustain., 2022, 6, 169–179 CrossRef.
- J. Li, S. Chen, F. Quan, G. Zhan, F. Jia, Z. Ai and L. Zhang, Chem, 2020, 6, 885–901 CAS.
- Y. Tian, D. Xu, K. Chu, Z. Wei and W. Liu, J. Mater. Sci., 2019, 54, 9088–9097 CrossRef CAS.
- C. Lv, Y. Qian, C. Yan, Y. Ding, Y. Liu, G. Chen and G. Yu, Angew. Chem., Int. Ed., 2018, 57, 10246–10250 CrossRef CAS PubMed.
- J. Zhao, J. Yang, L. Ji, H. Wang, H. Chen, Z. Niu, Q. Liu, T. Li, G. Cui and X. Sun, Chem. Commun., 2019, 55, 4266–4269 RSC.
- S. Liu, M. Wang, T. Qian, H. Ji, J. Liu and C. Yan, Nat. Commun., 2019, 10, 3898 CrossRef PubMed.
- X. Zhang, T. Wu, H. Wang, R. Zhao, H. Chen, T. Wang, P. Wei, Y. Luo, Y. Zhang and X. Sun, ACS Catal., 2019, 9, 4609–4615 CrossRef CAS.
- J.-T. Ren, C.-Y. Wan, T.-Y. Pei, X.-W. Lv and Z.-Y. Yuan, Appl. Catal., B, 2020, 266, 118633 CrossRef CAS.
- J. Zhao, X. Ren, X. Li, D. Fan, X. Sun, H. Ma, Q. Wei and D. Wu, Nanoscale, 2019, 11, 4231–4235 RSC.
- L. Xia, X. Wu, Y. Wang, Z. Niu, Q. Liu, T. Li, X. Shi, A. M. Asiri and X. Sun, Small Methods, 2019, 3, 1800251 CrossRef.
- H. Huang, L. Xia, R. Cao, Z. Niu, H. Chen, Q. Liu, T. Li, X. Shi, A. M. Asiri and X. Sun, Chem.–Eur. J., 2019, 25, 1914–1917 CrossRef CAS PubMed.
- L. Shi, S. Bi, Y. Qi, G. Ning and J. Ye, J. Colloid Interface Sci., 2023, 641, 577–584 CrossRef CAS PubMed.
- Y. Zhang, H. Du, Y. Ma, L. Ji, H. Guo, Z. Tian, H. Chen, H. Huang, G. Cui, A. M. Asiri, F. Qu, L. Chen and X. Sun, Nano Res., 2019, 12, 919–924 CrossRef CAS.
- L.-P. Yuan, Z.-Y. Wu, W.-J. Jiang, T. Tang, S. Niu and J.-S. Hu, Nano Res., 2020, 13, 1376–1382 CrossRef CAS.
- X. Zhu, T. Wu, L. Ji, C. Li, T. Wang, S. Wen, S. Gao, X. Shi, Y. Luo, Q. Peng and X. Sun, J. Mater. Chem. A, 2019, 7, 16117–16121 RSC.
- W. Qiu, X. Y. Xie, J. Qiu, W. H. Fang, R. Liang, X. Ren, X. Ji, G. Cui, A. M. Asiri, G. Cui, B. Tang and X. Sun, Nat. Commun., 2018, 9, 3485 CrossRef PubMed.
- L. Xia, J. Yang, H. Wang, R. Zhao, H. Chen, W. Fang, A. M. Asiri, F. Xie, G. Cui and X. Sun, Chem. Commun., 2019, 55, 3371–3374 RSC.
- H. Chen, X. Zhu, H. Huang, H. Wang, T. Wang, R. Zhao, H. Zheng, A. M. Asiri, Y. Luo and X. Sun, Chem. Commun., 2019, 55, 3152–3155 RSC.
- L. Zhang, L. X. Ding, G. F. Chen, X. Yang and H. Wang, Angew. Chem., Int. Ed., 2019, 58, 2612–2616 CrossRef CAS PubMed.
- X. Yu, P. Han, Z. Wei, L. Huang, Z. Gu, S. Peng, J. Ma and G. Zheng, Joule, 2018, 2, 1610–1622 CrossRef CAS.
- H. Liu, G. Hai, L. X. Ding and H. Wang, Angew. Chem., Int. Ed., 2023, 135, e202302124 CrossRef.
- S. Mukherjee, D. A. Cullen, S. Karakalos, K. Liu, H. Zhang, S. Zhao, H. Xu, K. L. More, G. Wang and G. Wu, Nano Energy, 2018, 48, 217–226 CrossRef CAS.
- L. Yan, Y. Zhao, S. Zhang, E. Guo, C. Han, H. Jiang, Q. Fu, L. Yang, W. Niu, Y. Xing, Q. Zheng and X. Zhao, Small, 2023, 19, e2300239 CrossRef PubMed.
- D. Gupta, A. Kafle, S. Kaur, T. S. Thomas, D. Mandal and T. C. Nagaiah, ACS Appl. Mater. Interfaces, 2023, 15, 4033–4043 CrossRef CAS PubMed.
- X.-W. Lv, C.-C. Weng and Z.-Y. Yuan, ChemSusChem, 2020, 13, 3061–3078 CrossRef CAS PubMed.
- D. McKay, D. H. Gregory, J. S. J. Hargreaves, S. M. Hunter and X. Sun, Chem. Commun., 2007, 29, 3051–3053 RSC.
- L. Zhang, X. Ji, X. Ren, Y. Ma, X. Shi, Z. Tian, A. M. Asiri, L. Chen, B. Tang and X. Sun, Adv. Mater., 2018, 30, 1800191 CrossRef PubMed.
- W. Ma, Z. Deng, X. Zhang, Z. Zhang and Z. Zhou, J. Mater. Chem. A, 2023, 11, 12643–12658 RSC.
- W. Zhang, Y. Chao, W. Zhang, J. Zhou, F. Lv, K. Wang, F. Lin, H. Luo, J. Li, M. Tong, E. Wang and S. Guo, Adv. Mater., 2021, 33, 2102576 CrossRef CAS PubMed.
- Z. Li, S. Ji, Y. Liu, X. Cao, S. Tian, Y. Chen, Z. Niu and Y. Li, Chem. Rev., 2020, 120, 623–682 CrossRef CAS PubMed.
- S. Wang, M. Luo and S. Guo, Nat. Synth., 2022, 1, 676–677 CrossRef.
- J. Wang, B. Huang, Y. Ji, M. Sun, T. Wu, R. Yin, X. Zhu, Y. Li, Q. Shao and X. Huang, Adv. Mater., 2020, 32, 1907112 CrossRef CAS PubMed.
- B. H. R. Suryanto, C. S. M. Kang, D. Wang, C. Xiao, F. Zhou, L. M. Azofra, L. Cavallo, X. Zhang and D. R. MacFarlane, ACS Energy Lett., 2018, 3, 1219–1224 CrossRef CAS.
- F. Zhou, L. M. Azofra, M. Ali, M. Kar, A. N. Simonov, C. McDonnell-Worth, C. Sun, X. Zhang and D. R. MacFarlane, Energy Environ. Sci., 2017, 10, 2516–2520 RSC.
- C. S. M. Kang, X. Zhang and D. R. MacFarlane, J. Phys. Chem. C, 2018, 122, 24550–24558 CrossRef CAS.
- R. Tort, O. Westhead, M. Spry, B. J. V. Davies, M. P. Ryan, M.-M. Titirici and I. E. L. Stephens, ACS Energy Lett., 2023, 8, 1003–1009 CrossRef CAS PubMed.
- N. Lazouski, Z. J. Schiffer, K. Williams and K. Manthiram, Joule, 2019, 3, 1127–1139 CrossRef CAS.
- R. Y. Hodgetts, H.-L. Du, T. D. Nguyen, D. MacFarlane and A. N. Simonov, ACS Catal., 2022, 12, 5231–5246 CrossRef CAS.
- X. Fu, J. B. Pedersen, Y. Zhou, M. Saccoccio, S. Li, R. Sažinas, K. Li, S. Z. Andersen, A. Xu, N. H. Deissler, J. B. V. Mygind, C. Wei, J. Kibsgaard, P. C. K. Vesborg, J. K. Nørskov and I. Chorkendorff, Science, 2023, 379, 707–712 CrossRef CAS PubMed.
- X. Cui, C. Tang and Q. Zhang, Adv. Energy Mater., 2018, 8, 1800369 CrossRef.
- Y. Zhao, R. Shi, X. Bian, C. Zhou, Y. Zhao, S. Zhang, F. Wu, G. I. N. Waterhouse, L.-Z. Wu, C.-H. Tung and T. Zhang, Adv. Sci., 2019, 6, 1802109 CrossRef PubMed.
- G. Qing, R. Ghazfar, S. T. Jackowski, F. Habibzadeh, M. M. Ashtiani, C. P. Chen, M. R. Smith and T. W. Hamann, Chem. Rev., 2020, 120, 5437–5516 CrossRef CAS PubMed.
- Y. Ren, C. Yu, X. Tan, H. Huang, Q. Wei and J. Qiu, Energy Environ. Sci., 2021, 14, 1176–1193 RSC.
- L. Chen, X. Zhang, A. Chen, S. Yao, X. Hu and Z. Zhou, Chin. J. Catal., 2022, 43, 11–32 CrossRef CAS.
- N. Zhang, B. Yang, K. Liu, H. Li, G. Chen, X. Qiu, W. Li, J. Hu, J. Fu, Y. Jiang, M. Liu and J. Ye, Small Methods, 2021, 5, 2100987 CrossRef CAS PubMed.
- X. Zhang, Y. Tian, L. Chen, X. Hu and Z. Zhou, J. Phys. Chem. Lett., 2022, 13, 7920–7930 CrossRef CAS PubMed.
- E. Skúlason, T. Bligaard, S. Gudmundsdóttir, F. Studt, J. Rossmeisl, F. Abild-Pedersen, T. Vegge, H. Jónsson and J. K. Nørskov, Phys. Chem. Chem. Phys., 2012, 14, 1235–1245 RSC.
- J. G. Howalt, T. Bligaard, J. Rossmeisl and T. Vegge, Phys. Chem. Chem. Phys., 2013, 15, 7785–7795 RSC.
- J. Zhao, J. Zhao and Q. Cai, Phys. Chem. Chem. Phys., 2018, 20, 9248–9255 RSC.
- Y. Abghoui and E. Skúlason, J. Phys. Chem. C, 2017, 121, 6141–6151 CrossRef CAS.
- Á. B. Höskuldsson, Y. Abghoui, A. B. Gunnarsdóttir and E. Skúlason, ACS Sustain. Chem. Eng., 2017, 5, 10327–10333 CrossRef.
- H. Shen, C. Choi, J. Masa, X. Li, J. Qiu, Y. Jung and Z. Sun, Chem, 2021, 7, 1708–1754 CAS.
- X. Hu, S. Yao, L. Chen, X. Zhang, M. Jiao, Z. Lu and Z. Zhou, J. Mater. Chem. A, 2021, 9, 23515–23521 RSC.
- Y. Yang, Y. Tang, H. Jiang, Y. Chen, P. Wan, M. Fan, R. Zhang, S. Ullah, L. Pan, J.-J. Zou, M. Lao, W. Sun, C. Yang, G. Zheng, Q. Peng, T. Wang, Y. Luo, X. Sun, A. S. Konev, O. V. Levin, P. Lianos, H. Zhuofeng, Z. Shen, Q. Zhao, Y. Wang, N. Todorova, C. Trapalis, M. V. Sheridan, H. Wang, L. Zhang, S. Sun, W. Wang and J. Ma, Chin. Chem. Lett., 2019, 30, 2089–2109 CrossRef CAS.
- L. Yang, T. Wu, R. Zhang, H. Zhou, L. Xia, X. Shi, H. Zheng, Y. Zhang and X. Sun, Nanoscale, 2019, 11, 1555–1562 RSC.
- H. Jin, L. Li, X. Liu, C. Tang, W. Xu, S. Chen, L. Song, Y. Zheng and S.-Z. Qiao, Adv. Mater., 2019, 31, 1902709 CrossRef PubMed.
- S. Chen, Y. Gao, W. Wang, O. V. Prezhdo and L. Xu, ACS Nano, 2023, 17, 1522–1532 CrossRef CAS PubMed.
- R. M. Lawrence and S. M. Unni, Sustainable Energy Fuels, 2021, 5, 3765–3790 RSC.
- I. E. Khalil, C. Xue, W. Liu, X. Li, Y. Shen, S. Li, W. Zhang and F. Huo, Adv. Funct. Mater., 2021, 31, 2010052 CrossRef CAS.
- C. Lv, Y. Qian, C. Yan, Y. Ding, Y. Liu, G. Chen and G. Yu, Angew. Chem., Int. Ed., 2018, 57, 10246–10250 CrossRef CAS PubMed.
- B. H. R. Suryanto, D. Wang, L. M. Azofra, M. Harb, L. Cavallo, R. Jalili, D. R. G. Mitchell, M. Chatti and D. R. MacFarlane, ACS Energy Lett., 2018, 4, 430–435 CrossRef.
- D. Yang, L. Zhang, X. Yan and X. Yao, Small Methods, 2017, 1, 1700209 CrossRef.
- C. Lv, C. Yan, G. Chen, Y. Ding, J. Sun, Y. Zhou and G. Yu, Angew. Chem., Int. Ed., 2018, 57, 6073–6076 CrossRef CAS PubMed.
- S.-J. Li, D. Bao, M.-M. Shi, B.-R. Wulan, J.-M. Yan and Q. Jiang, Adv. Mater., 2017, 29, 1700001 CrossRef PubMed.
- H.-B. Wang, J.-Q. Wang, R. Zhang, C.-Q. Cheng, K.-W. Qiu, Y.-J. Yang, J. Mao, H. Liu, M. Du, C.-K. Dong and X.-W. Du, ACS Catal., 2020, 10, 4914–4921 CrossRef CAS.
- S. Kelly and A. Bogaerts, Joule, 2021, 5, 3006–3030 CrossRef CAS.
- L. Li, C. Tang, X. Cui, Y. Zheng, X. Wang, H. Xu, S. Zhang, T. Shao, K. Davey and S. Z. Qiao, Angew Chem. Int. Ed. Engl., 2021, 60, 14131–14137 CrossRef CAS PubMed.
- I. Muzammil, Y.-N. Kim, H. Kang, D. K. Dinh, S. Choi, C. Jung, Y.-H. Song, E. Kim, J. M. Kim and D. H. Lee, ACS Energy Lett., 2021, 6, 3004–3010 CrossRef CAS.
- J. Sun, D. Alam, R. Daiyan, H. Masood, T. Zhang, R. Zhou, P. J. Cullen, E. C. Lovell, A. Jalili and R. Amal, Energy Environ. Sci., 2021, 14, 865–872 RSC.
- M. Nazemi, S. R. Panikkanvalappil and M. A. El-Sayed, Nano Energy, 2018, 49, 316–323 CrossRef CAS.
- Y. Wan, J. Xu and R. Lv, Mater. Today, 2019, 27, 69–90 CrossRef CAS.
- S. J. Li, D. Bao, M. M. Shi, B. R. Wulan, J. M. Yan and Q. Jiang, Adv. Mater., 2017, 29, 1700001 CrossRef PubMed.
- T. Lu and H. Wang, Nano Res., 2022, 15, 9764–9778 CrossRef CAS.
- N. Zhang, J. Shang, X. Deng, L. Cai, R. Long, Y. Xiong and Y. Chai, ACS Nano, 2022, 16, 4795–4804 CrossRef CAS PubMed.
- J. Li, G. Zhan, J. Yang, F. Quan, C. Mao, Y. Liu, B. Wang, F. Lei, L. Li, A. W. M. Chan, L. Xu, Y. Shi, Y. Du, W. Hao, P. K. Wong, J. Wang, S.-X. Dou, L. Zhang and J. C. Yu, J. Am. Chem. Soc., 2020, 142, 7036–7046 CrossRef CAS PubMed.
- S. Chen, X. Liu, J. Xiong, L. Mi, X.-Z. Song and Y. Li, J. Mater. Chem. A, 2022, 10, 6927–6949 RSC.
- D. Guo, S. Wang, J. Xu, W. Zheng and D. Wang, J. Energy Chem., 2022, 65, 448–468 CrossRef CAS.
- C. Huang, L. Shang, P. Han, Z. Gu, A. M. Al-Enizi, T. M. Almutairi, N. Cao and G. Zheng, J. Colloid Interface Sci., 2019, 552, 312–318 CrossRef CAS PubMed.
- J. Wei, Y. Jing, Z. Zhao, Z. Fan, Z. Liang, J. Huang, H. Wu, Z. Xie, D. Liu, D. Qu, H. Tang and J. Li, Electrochim. Acta, 2021, 381, 138222 CrossRef CAS.
- J. Wang, Y.-P. Liu, H. Zhang, D.-J. Huang and K. Chu, Catal. Sci. Technol., 2019, 9, 4248–4254 RSC.
- Y. Gong, J. Wu, M. Kitano, J. Wang, T.-N. Ye, J. Li, Y. Kobayashi, K. Kishida, H. Abe, Y. Niwa, H. Yang, T. Tada and H. Hosono, Nat. Catal., 2018, 1, 178–185 CrossRef CAS.
- W. Tong, B. Huang, P. Wang, L. Li, Q. Shao and X. Huang, Angew. Chem., Int. Ed., 2020, 59, 2649–2653 CrossRef CAS PubMed.
- F. Pang, F. Wang, L. Yang, Z. Wang and W. Zhang, Chem. Commun., 2019, 55, 10108–10111 RSC.
- H. Wang, Q. Mao, H. Yu, S. Wang, Y. Xu, X. Li, Z. Wang and L. Wang, Chem. Eng. J., 2021, 418, 129493 CrossRef CAS.
- S. Liu, Y. Xu, S. Jiao, W. Tian, T. Zhou, Z. Wang, X. Li, L. Wang and H. Wang, J. Mater. Chem. A, 2021, 9, 8372–8377 RSC.
- Y. Jin, X. Ding, L. Zhang, M. Cong, F. Xu, Y. Wei, S. Hao and Y. Gao, Chem. Commun., 2020, 56, 11477–11480 RSC.
- Y. Zhang, S. Li, C. Sun, P. Wang, Y. Yang, D. Yi, X. Wang and J. Yao, ACS Catal., 2022, 12, 9201–9212 CrossRef CAS.
- Y. Gong, H. Li, J. Wu, X. Song, X. Yang, X. Bao, X. Han, M. Kitano, J. Wang and H. Hosono, J. Am. Chem. Soc., 2022, 144, 8683–8692 CrossRef CAS PubMed.
- A. R. Singh, B. A. Rohr, J. A. Schwalbe, M. Cargnello, K. Chan, T. F. Jaramillo, I. Chorkendorff and J. K. Nørskov, ACS Catal., 2017, 7, 706–709 CrossRef CAS.
- C. Du, C. Qiu, Z. Fang, P. Li, Y. Gao, J. Wang and W. Chen, Nano Energy, 2022, 92, 106784 CrossRef CAS.
- X. Shen, S. Liu, X. Xia, M. Wang, H. Ji, Z. Wang, J. Liu, X. Zhang, C. Yan and T. Qian, Adv. Funct. Mater., 2022, 32, 2109422 CrossRef CAS.
- Y. Liu, B. Huang, X. Chen, Z. Tian, X. Zhang, P. Tsiakaras and P. K. Shen, Appl. Catal., B, 2020, 271, 118919 CrossRef CAS.
- C. Ampelli, Nat. Catal., 2020, 3, 420–421 CrossRef CAS.
- Y. Ren, C. Yu, L. Wang, X. Tan, Z. Wang, Q. Wei, Y. Zhang and J. Qiu, J. Am. Chem. Soc., 2022, 144, 10193–10200 CrossRef CAS PubMed.
- B. H. R. Suryanto, K. Matuszek, J. Choi, R. Y. Hodgetts, H.-L. Du, J. M. Bakker, C. S. M. Kang, P. V. Cherepanov, A. N. Simonov and D. R. MacFarlane, Science, 2021, 372, 1187–1191 CrossRef CAS PubMed.
- Y. Guo, Q. Yang, D. Wang, H. Li, Z. Huang, X. Li, Y. Zhao, B. Dong and C. Zhi, Energy Environ. Sci., 2020, 13, 2888–2895 RSC.
- Y. Hu, G. Z. Chen, L. Zhuang, Z. Wang and X. Jin, Cell Rep. Phys. Sci., 2021, 2, 100425 CrossRef CAS.
- S. Z. Andersen, M. J. Statt, V. J. Bukas, S. G. Shapel, J. B. Pedersen, K. Krempl, M. Saccoccio, D. Chakraborty, J. Kibsgaard, P. C. K. Vesborg, J. Nørskov and I. Chorkendorff, Energy Environ. Sci., 2020, 13, 4291–4300 RSC.
- K. Li, S. Z. Andersen, M. J. Statt, M. Saccoccio, V. J. Bukas, K. Krempl, R. Sažinas, J. B. Pedersen, V. Shadravan, Y. Zhou, D. Chakraborty, J. Kibsgaard, P. C. K. Vesborg, J. K. Nørskov and I. Chorkendorff, Science, 2021, 374, 1593–1597 CrossRef CAS PubMed.
- S. Sun, Q. An, W. Wang, L. Zhang, J. Liu and W. A. Goddard III, J. Mater. Chem. A, 2017, 5, 201–209 RSC.
- S. Sun, X. Li, W. Wang, L. Zhang and X. Sun, Appl. Catal., B, 2017, 200, 323–329 CrossRef CAS.
- S. Chen, S. Perathoner, C. Ampelli, C. Mebrahtu, D. S. Su and G. Centi, ACS Sustain. Chem. Eng., 2017, 5, 7393–7400 CrossRef CAS.
- T. W. Hansen, J. B. Wagner, P. L. Hansen, S. Dahl, H. Topsøe and C. J. H. Jacobsen, Science, 2001, 294, 1508–1510 CrossRef CAS PubMed.
- M. Wang, S. Liu, H. Ji, T. Yang, T. Qian and C. Yan, Nat. Commun., 2021, 12, 3198 CrossRef CAS PubMed.
- Y.-C. Hao, Y. Guo, L.-W. Chen, M. Shu, X.-Y. Wang, T.-A. Bu, W.-Y. Gao, N. Zhang, X. Su, X. Feng, J.-W. Zhou, B. Wang, C.-W. Hu, A.-X. Yin, R. Si, Y.-W. Zhang and C.-H. Yan, Nat. Catal., 2019, 2, 448–456 CrossRef CAS.
- Q. Zhang, B. Liu, L. Yu, Y. Bei and B. Tang, ChemCatChem, 2019, 12, 334–341 CrossRef.
- R. Battino, T. R. Rettich and T. Tominaga, J. Phys. Chem. Ref. Data, 1984, 13, 563–600 CrossRef CAS.
- Y. Ren, C. Yu, X. Han, X. Tan, Q. Wei, W. Li, Y. Han, L. Yang and J. Qiu, ACS Energy Lett., 2021, 6, 3844–3850 CrossRef CAS.
- S. Niu, W.-J. Jiang, Z. Wei, T. Tang, J. Ma, J.-S. Hu and L.-J. Wan, J. Am. Chem. Soc., 2019, 141, 7005–7013 CrossRef CAS PubMed.
- Z. Li, Y. Yan, S.-M. Xu, H. Zhou, M. Xu, L. Ma, M. Shao, X. Kong, B. Wang, L. Zheng and H. Duan, Nat. Commun., 2022, 13, 147 CrossRef CAS PubMed.
- J.-Y. Zhang, H. Wang, Y. Tian, Y. Yan, Q. Xue, T. He, H. Liu, C. Wang, Y. Chen and B. Y. Xia, Angew. Chem., Int. Ed., 2018, 57, 7649–7653 CrossRef CAS PubMed.
- J. Li, S. Wang, S. Sun, X. Wu, B. Zhang and L. Feng, J. Mater. Chem. A, 2022, 10, 9308–9326 RSC.
- M. Xiang, Z. Xu, Q. Wu, Y. Wang and Z. Yan, J. Power Sources, 2022, 535, 231461 CrossRef CAS.
- L. Zhao, X. Kuang, C. Chen, X. Sun, Z. Wang and Q. Wei, Chem. Commun., 2019, 55, 10170–10173 RSC.
- J. Bai, H. Huang, F.-M. Li, Y. Zhao, P. Chen, P.-J. Jin, S.-N. Li, H.-C. Yao, J.-H. Zeng and Y. Chen, J. Mater. Chem. A, 2019, 7, 21149–21156 RSC.
-
G. Soloveichik, ARPA-E, 2016, https://arpa-e.energy.gov/?q=arpa-e-programs/refuel Search PubMed.
- M. Yang, S. Lu, J. Lu, S. P. Jiang and Y. Xiang, Chem. Commun., 2010, 46, 1434–1436 RSC.
- Y. Ren, C. Yu, X. Tan, X. Han, H. Huang, H. Huang and J. Qiu, Small Methods, 2019, 3, 1900474 CrossRef CAS.
- Z. Yuan, L. Liang, Q. Dai, T. Li, Q. Song, H. Zhang, G. Hou and X. Li, Joule, 2022, 6, 884–905 CrossRef CAS.
- G. Marnellos and M. Stoukides, Science, 1998, 282, 98–100 CrossRef CAS PubMed.
- G. Weng, S. Lei, R. Wang, K. Ouyang, J. Dong, X. Lin, J. Xue, L.-X. Ding and H. Wang, Joule, 2023, 7, 1333–1346 CrossRef CAS.
Footnote |
† These authors contributed equally to this work. |
|
This journal is © The Royal Society of Chemistry 2023 |
Click here to see how this site uses Cookies. View our privacy policy here.