Development of a rapid detection protocol for microplastics using reflectance-FTIR spectroscopic imaging and multivariate classification†
Received
12th December 2022
, Accepted 20th February 2023
First published on 6th March 2023
Abstract
Microplastics present a serious and worsening environmental threat to marine and aquatic ecosystems. Continued monitoring is crucial in assessing the hazards and provenance of plastics. Unfortunately, analytical methods that are currently available suffer limitations that prevent widespread uptake of microplastic monitoring across the environmental sector. Reflectance-FTIR spectroscopy may present an alternative to current microplastic analytical methods, as it provides spatially resolved chemical specificity (allowing determination of particle size, abundance and polymer identity); is a non-contact method (allowing high throughput of samples); eliminates the problems of complete infrared absorption by larger particles; and does not require expensive infrared-transparent substrates. This study has used reflectance-FTIR spectroscopic imaging to build a spectral library, which was then coupled with multivariate statistics (chemometrics) to develop a protocol for microplastic detection and a semi-automated data processing pipeline. The workflow was used to detect microplastics from marine salt samples that were concentrated via filtration onto cost-effective and routinely available Whatman cellulose filter papers. Specifically, polyethylene (PE) and polypropylene (PP) microplastics from marine salt samples could be identified with 100% specificity (PE and PP) and sensitivities of 78% (PE) and 82% (PP), highlighting the immense potential for future use of this method as a cost-effective option for high-throughput and semi-automatable microplastic analyses.
Introduction
Microplastics, defined as synthetic organic polymers less than 5 mm in size,1 have emerged as an environmental pollutant of concern, particularly in aquatic ecosystems. Microplastics can block the gastrointestinal tract of smaller marine organisms, often resulting in starvation.2 In addition to the direct adverse health effects associated with ingestion, microplastics can have secondary detrimental effects that result from pollutants adsorbed to the plastic surface. Marine microplastics contain various adsorbed or added organic contaminants such as polychlorinated biphenyls (PCBs), polycyclic aromatic hydrocarbons, organochlorine pesticides and petroleum hydrocarbons.3,4 The ingestion of microplastics by aquatic organisms can provide a direct route of exposure to toxic substances, disrupting the endocrine system and harming the organism.4,5 Despite knowledge of multiple pathways through which microplastics may be harmful, the extent to which microplastic contamination has, or is currently impacting ecosystems is not well understood.2,6 In part, this uncertainty derives from the difficulty in quantifying and characterising microplastics in environmental samples in a robust, rapid, and cost-effective manner. High volume and routine methods to assess microplastic abundance in environmental samples are needed to monitor the impact of this high profile and topical pollutant and inform environmental management.6
Numerous methods have been used to identify microplastics to date. Optical microscopy has been used extensively to assess microplastic abundance, and it is a relatively cost-effective and high throughput method.6,7 However, optical microscopy cannot confirm the chemical composition of identified particles, with plastic classification based on examination of colour and morphology alone reported to have error rates as high as 70%.7 Analytical methods that are capable of confirming both microplastic abundance and chemical composition include vibrational spectroscopic methods such as transmission- or attenuated total reflectance- (ATR) Fourier transform infrared (FTIR) spectroscopy, and Raman spectroscopy.6–13 When used in a microscopy or imaging configuration, both are very well suited to determine plastic identity, in addition to the relative abundance and size of particles. Unfortunately, to-date, widespread use of Raman microscopy has been limited by long data collection times and sample autofluorescence.12,14 Similarly, ATR-FTIR measurements require contact between the ATR crystal and the individual plastic particle resulting in long data collection times; and transmission-FTIR requires the use of relatively costly infrared-transparent substrates. Further, collection of FTIR spectra in transmission mode limits analysis to particles small enough to transmit infrared light, or requires time consuming manual pressing of particles (e.g., with a diamond anvil) to produce a film that is sufficiently thin to transmit infrared light (typically <50 μm).8,12,15
Reflectance-FTIR spectroscopy is a promising analytical technique to detect microplastics and has numerous advantages over more costly transmission-FTIR or labour intensive ATR-FTIR.16,17 Reflectance-FTIR is a non-contact technique, making it non-destructive, automatable and time efficient. Unlike transmission-FTIR, reflectance-FTIR does not require infrared-transparent substrates and allows the analysis of thicker or more opaque samples which would otherwise completely absorb incident infrared radiation. Thus, when used in an imaging configuration (e.g. multi-pixel array detector) reflectance-FTIR spectroscopic imaging can screen microplastics across a large area of filter paper, eliminating the requirement for preliminary visual examination of particles. To date, however, there has not been a high uptake of reflectance-FTIR spectroscopy for microplastic analyses, most likely because it is more impacted by spectral distortion resulting from irregularly shaped particles and non-uniform refractive indices in heterogenous samples.8,12,18–20 The difficulty in interpreting reflectance spectra is further compounded by a lack of extensive spectral libraries for polymers collected in reflectance modes.21
Despite this, a proof-of-concept for the applicability of reflectance-FTIR spectroscopic imaging for microplastic detection has been demonstrated,17 which highlighted capability to identify polyethylene (PE) microplastics spiked in marine sediments. An additional study compared transmission vs. reflectance FTIR imaging with a focal plane array (FPA) detector.8 These methods, however, were focused on screening for a limited number of plastic types, as identification was based on a limited number of absorption bands. Confirmation of identity still required manual examination of spectra. Lastly, a method was developed using FPA-based reflectance-FTIR spectroscopic imaging for microplastic analysis in organic matter-rich samples from a wastewater treatment plant, which was subsequently validated for multiple polymers using spiked samples.16 The method was successfully applied to “real” (i.e., not spiked) wastewater samples in a subsequent study,22 though identification was still manual, and based on the presence of selected absorbance bands. While these studies suggest that reflectance-FTIR spectroscopic imaging has promise for analysis of microplastics concentrated on filter papers, it requires further validation and development of an automated data processing pipeline. It is well established that multivariate statistics allows for objective evaluation and classification of chemical data for a range of applications,23,24 including application to microplastic libraries.6,9,25 To drastically reduce the analysis time requirements associated with identification of plastic type, analysis of reflectance-FTIR spectra needs to shift away from manual univariate classification and instead incorporate multivariate classification, as has been achieved with transmission-FTIR.15,26
In this study, reflectance-FTIR spectroscopic imaging was used to build a spectral library of common plastic polymers, which was then combined in an analytical workflow with partial least squares-discriminant analysis (PLS-DA) for the semi-automated detection and polymer classification of microplastics. The workflow was used to detect microplastics from marine salt samples that were concentrated via filtration onto cost-effective and routinely available Whatman cellulose filter papers. The results highlight immense potential of micro-reflectance-FTIR imaging for further development as a high throughput and cost-effective method of microplastic identification in environmental samples.
Experimental methods
A flow diagram of the analytical protocol developed is shown in Fig. 1, with the specific methods of each step in the protocol described in detail below.
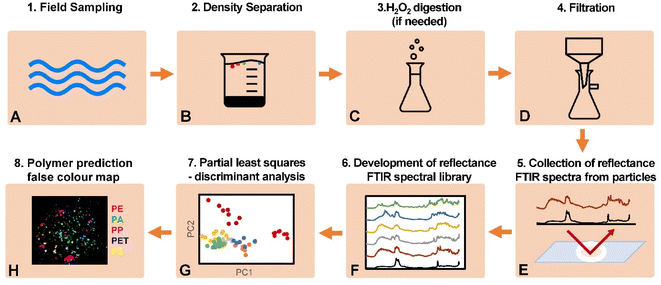 |
| Fig. 1 Flow diagram of the protocol for reflectance-FTIR detection of microplastics in environmental samples. | |
Development of plastic reference library
A library of reference plastic particles were sourced from commercial suppliers and from marine salt samples (provided by the analytical industry, Intertek). Commercially sourced reference plastics are listed in Table 1 and included polyethylene (PE), polypropylene (PP), polystyrene (PS), polyethylene terephthalate (PET), polyamide (PA), and poly(methyl methacrylate) (PMMA). Most were supplied as powders or microspheres and used as supplied, except for PP for which particles were self-generated via abrasion using a metal file. Stock aqueous suspensions of microplastic particles were generated from the addition of 1–5 mg of plastic to a water/ethanol mixture. The exact mass of plastic added was influenced by electrostatic forces between particles and spatula. To prepare the water/ethanol mixture, particles were first transferred to water, and then ethanol subsequently added to lower solution density until particles became suspended (the approximate water/ethanol ratio was 5
:
3 parts). For subsequent analysis, 2 μL aliquots of the stock solution were dispensed onto filter paper (Whatman, Grade 1) at known locations (i.e., the filter paper was divided into six sections, marked with graphite pencil, with one plastic type deposited into each section). To prepare samples containing a heterogenous mixture of different particle types, aliquots from each suspension were deposited onto the same location on the filter paper. Filter papers were adhered to glass slides with double-sided tape to maintain a flat surface for FTIR imaging.
Table 1 Characteristics and sources of polymer particle standards
Polymer |
Particle type |
Particle size |
Origin |
PE (ultra-high molecular weight) |
Powder |
40–48 μm |
Sigma Aldrich |
PE (ultra-high molecular weight) |
Powder |
125 μm (average) |
Sigma Aldrich |
PE (red) |
Microspheres |
10–27 μm |
Cospheric |
PE (red) |
Microspheres |
53–63 μm |
Cospheric |
PS |
Microspheres |
14–20 μm |
Cospheric |
PS |
Microspheres |
85–105 μm |
Cospheric |
PMMA |
Microspheres |
1–75 μm |
Cospheric |
PET (semi-crystalline) |
Powder |
300 μm (maximum) |
Goodfellow |
PA (nylon 6) |
Powder |
5–50 μm |
Goodfellow |
PP (black) |
Abrasion |
Unknown, not characterised |
Bunnings, retailer |
Analysis of microplastics in environmental samples (marine salt)
Marine salt samples were sourced from industry (Intertek), from a previous commercial consultancy project in which 3 commercial salt samples were analysed. For each salt sample, 180 g of sample was added to 500 mL deionized water to make a saturated brine solution with a target density of 1.2 g cm−3 (ZnCl2 was added to brine solutions if density adjustment was required). The brine was heated and sonicated for 15 min until all salt crystals were fully dissolved. The brine was then transferred to separation funnel and left overnight. The upper layer of the brine containing less dense material (e.g., plastics) was decanted and filtered through a 0.45 μm pore size, 44 mm diameter mixed cellulose ester (MCE) filter paper. Filter papers were then adhered to glass slides with double-sided tape to maintain a flat surface for FTIR imaging.
Analysis of particle size distribution with optical microscopy
The particles on the MCE filter paper were initially assessed using optical microscopy (Nikon Inverted Eclipse Ti2-U microscope, with NiS Elements software). Optical microscopy was used to assess the size distribution of particles on the filter, which revealed most particles were ∼25–75 μm in size, with an average particle size and standard deviation of 82 ± 135 μm, and median particle size of 44 μm (ESI Fig. S1†). Evidence of smaller particles (<10 μm) was observed with subsequent synchrotron radiation ATR-FTIR mapping (ESI Fig. S2†).
Fourier transform infrared spectroscopic data collection
All FTIR spectroscopic data collection was performed using a Nicolet iN10 MX Infrared Imaging Microscope (Thermo Scientific Inc. USA) with a liquid nitrogen-cooled single point detector or a liquid nitrogen-cooled linear array mercury cadmium telluride (MCT) detector. The pixel size on the array detector was 25 μm. FTIR analysis was undertaken in several modes: single point micro-ATR-FTIR with a MCT detector for confirmation of polymer identity; or micro-reflectance-FTIR imaging using a 8 × 2 pixel linear array detector, for development of the spectral library and analysis of unknowns. For all single point micro-ATR-FTIR the infrared beam aperture was adjusted from 10–150 μm2 as necessary to minimise interference from the surrounding filter paper. A background was collected every 300 minutes using the same parameters. Instrumental parameters used for each mode are summarised in Table 2. For the marine salt samples, a total of 65 particles were analysed (20 particles for calibration library, and 45 for testing/validation).
Table 2 Instrumental parameters used for FTIR spectroscopy in different modes
|
Micro-ATR-FTIR |
Micro-reflectance FTIR imaging |
Detector |
Single point, MCT |
Linear array |
Pixel size (μm) |
Variable aperture |
25 |
ATR crystal |
Germanium, single bounce |
— |
Spectral resolution (cm−1) |
4 |
4, 8 or 16 |
Number of co-added scans |
128 |
2, 16 or 128 |
Beam aperture (μm2) |
10–150 |
— |
Background location |
Clean ATR crystal |
Aluminium |
Mode |
Absorbance |
Absorbance |
FTIR spectroscopic data analysis
Reflectance-FTIR images were viewed in CytoSpec 2.00.03, where univariate imaging was performed and individual spectra were exported. Opus 8.0 (Bruker) was used to view and manipulate spectra. Data analysis was performed using RStudio Version 1.4.1106. For multivariate data analysis, raw spectra exported from CytoSpec were imported into RStudio using the package ‘readJDX’ and pre-processed (vector normalisation and Savitzky–Golay second derivative, as required) using a combination of custom scripts and the package ‘prospectr’. The package ‘mdatools’ was used for all multivariate data analysis. Other packages used include ‘dplyr’, ‘tidyr’, and ‘readxl’. False colour maps were constructed in ImageJ 1.53e.
Spectra were pre-processed using vector normalisation over the whole spectral range, followed by derivation (Savitzky–Golay 21 smoothing point 2nd derivative function). PLS-DA models were initially generated for discrimination of the six reference polymer types using PLS-2 using the SIMPLS algorithm. All PLS-DA models built from commercially sourced plastics used eight replicate reflectance-FTIR spectra (n = 8 per polymer). The ideal number of components to use for prediction was determined using the correct classification rate of leave-one-out cross-validation. To determine predictions of polymer type, each spectrum in a micro-reflectance-FTIR image was classified by the PLS-DA model, and the classifications reconstructed into a false colour image. To evaluate the model, particle identity was confirmed by using single-point ATR-FTIR spectroscopy and then compared with the predicted classification for 96 particles (n = 16 per polymer) for a sample prepared on a filter on which individual microplastic types had been deposited at unknown locations.
A second PLS-DA model was constructed from representative examples of plastics within the marine salt samples (e.g., “matrix matched”), using replicate reflectance-FTIR spectra (n = 4) from 10 particles per group (PE, PP, filter paper). The identity of each reflectance spectrum (PE, PP, filter paper) was confirmed with ATR-FTIR. The validation library for the marine salt samples consisted of micro-reflectance-FTIR spectra from n = 45 microplastic particles (n = 23 PE particles, n = 22 PP particles). The model was evaluated using the sensitivity (true positive rate) and specificity (true negative rate) of the model in classifying the validation subset, and by confirming the predicted classification of 45 particles (n = 23 PE particles, n = 22 PP particles) in the marine salt sample using micro-ATR-FTIR.
Scanning electron microscopy (SEM) analysis of particle morphology
To aid interpretation of the impact of particle morphology on distortion in reflectance-FTIR spectra, four PE particles [53–63 μm microsphere, 40–48 μm granule, supermarket freezer bag (Coles, Perth, Australia), weathered particle from the marine salt sample] were imaged using a benchtop SEM (Joel JCM-6000 plus) following gold-coating. The samples were fixed onto a SEM stub using carbon tape. The stub was coated with gold for 2 min using smart coater (DII-29030SCTR) prior to SEM imaging.
Results and discussion
Optical microscopy characterisation of particles from marine salt samples
Optical microscopy was used to assess the visual appearance and size of particles from marine salt that were deposited onto the filter paper. In general, particles were observed to be approximately 25–75 μm in diameter, although occasional smaller particles (5–10 μm) were also observed (ESI Fig. S1†). An important consideration when developing FTIR spectroscopic imaging methods is the difference in diffraction limit (and therefore spatial resolution) between optical microscopy utilising light in the visible wavelength range (400–700 nm) and infrared microscopy (2–10 μm wavelength range). The diffraction limit on the infrared microscope utilised for this study was determined to be 3 μm (at best), using eqn (1).27,28 | Diffraction limited resolution = 0.61 × λ/N.A. | (1) |
[λ = 3.5 μm (2852 cm−1, νsCH2), numerical aperture (N.A.) = 0.7].
However, as the FTIR imaging was undertaken with an array detector with pixel size of 25 μm, the FTIR imaging spatial resolution was limited to 25 μm. Therefore, the methods presented herein can be viewed has having a minimum particle size detection limit of 25 μm. FTIR microscopy can detect particles smaller than thus, by using smaller aperture sizes in combination with smaller pixel sizes (and with a brighter light source, such as a synchrotron source, to maintain adequate S/N), as presented in ESI Fig. S1.† However, there is a substantial time requirement to collect diffraction-limited FTIR data (e.g. >2 hours was required to image the particles in ESI Fig. S1†). In practice therefore, high throughput plastic analysis is likely to be limited to the pixel size of available array detectors (e.g. 25 μm).
Development of a reflectance-FTIR spectral library (with plastic identity validated using ATR-FTIR)
The ATR-FTIR and reflectance-FTIR spectra of the six reference virgin plastic polymers (PE, PMMA, PP, PA, PS and PET), plus filters (Whatman Grade 1) are shown in Fig. 2. As expected, the ATR-FTIR spectra display a relatively “simple” spectral profile of characteristic absorbance bands, on a uniform baseline. The ATR-FTIR spectra presented in Fig. 2 show excellent agreement with those already presented in the literature.10 In contrast, the corresponding reflectance spectra display a highly varied baseline attributed to light scattering, and distorted band shapes (associated with Christensen effects). It is clear from the spectra in Fig. 2 that based purely on spectral quality, ATR-FTIR spectra are superior to reflectance-FTIR spectra for polymer identification, owing to the numerous spectral distortions present in reflectance-FTIR spectra.
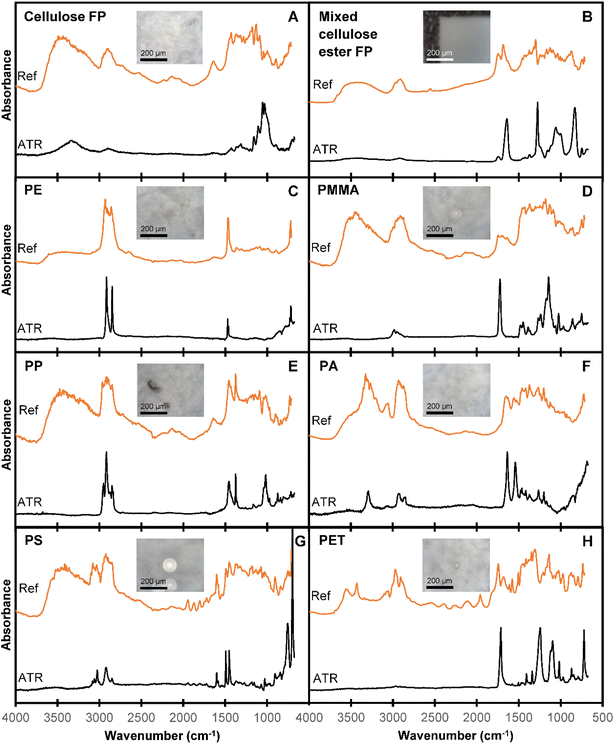 |
| Fig. 2 Comparison of single point micro-ATR-FTIR (bottom trace, black) and micro-reflectance-FTIR imaging spectra (top trace, orange) of (A) cellulose filter paper (FP), (B) mixed cellulose ester FP, (C) PE, (D) PMMA, (E) PP, (F) PA, (G) PS, and (H) PET. Spectra have been offset for clarity. Single point micro-ATR-FTIR spectra were collected at 4 cm−1 spectral resolution with co-added 128 scans, while micro-reflectance-FTIR imaging spectra were collected at 8 cm−1 spectral resolution and 2 co-added scans. The optical images of each particle are shown in the inset, highlight the ease at which some microplastics can be visualised with optical microscopy (e.g., panel (D), (E), (G), (H)), while also highlighting the difficulty associated with visualization other types of plastic particles with optical microscopy (e.g., panel (C), (F)). | |
Due to the spectral distortions in reflectance-FTIR spectra, the resulting reflectance spectrum is a product of both chemical and physical properties of the sample. Distortions in reflectance spectra may present as shifted absorbance bands, derivative-like line shapes, altered relative intensities of absorbance bands, additional features, split absorbance bands, and uneven or undulating baselines,29–31 and occur as a result of inhomogeneous refractive indices and irregular particle morphologies.8,12,18,19,29,31 Despite these limitations of reflectance-FTIR spectra, the data collection time of reflectance-FTIR spectroscopy is substantially reduced compared to ATR-FTIR spectroscopy, as physical contact between the ATR crystal and sample does not need to be made and optimised (as is required for ATR-FTIR spectroscopy). Therefore, although reflectance-FTIR spectra contain numerous distortions, as long as sufficient chemical information is retained to enable polymer identification, reflectance-FTIR offers opportunities for substantially reduced data collection time (and therefore higher throughput in environmental testing).
While the reflectance-FTIR spectra presented in Fig. 2 are more difficult to interpret than the corresponding ATR-FTIR spectra, the relevant chemical information for polymer identification is still present. Indeed, based on comparison of the reflectance-FTIR and ATR-FTIR spectra one can still identify general regions of interest for polymer absorption bands including the CH stretching (3000–2800 cm−1) and bending regions (1470–1365 cm−1), the carbonyl stretching region (1820–1680 cm−1) for polymers such as PET and PMMA, and the NH stretching region (3500–3300 cm−1) for PA.10 These results highlight that while visual interpretation and/or univariate analysis of reflectance-FTIR spectra is complicated by the presence of dispersion artefacts, sufficient chemical information can likely be extracted using multivariate statistics, to enable classification and polymer identification. In addition, reflectance-FTIR spectra are less prone (relative to transmission spectra) to total absorption of infrared light, which causes the appearance of “flat-topped” peaks (ESI Fig. S3†).
Optimisation of spectral collection parameters for microplastic analysis
A major limitation of current spectroscopic imaging techniques for microplastic analyses is the length of time required to obtain a spectral map (or image) of the whole area of a filter paper, which can be many hours for a single filter paper. The time required will ultimately depend on the analytical technique, instrumental parameters, detector type, and total filter paper area. While particle counts are sometimes extrapolated from a subsampled area of filter paper, this is problematic due to the heterogeneous distribution of particles across the filter paper.8,12 The large quantity of data produced in spectroscopic imaging can also be challenging to process and store. Therefore, we investigated whether the spectral resolution and the number of co-added scans could be optimised to minimise data collection time and file storage space without compromising the discrimination ability of a chemometric model.
A dataset of reflectance-FTIR imaging spectra was collected from 33 PE and 32 PP particles separated from the marine salt using varying spectral resolution (4, 8 and 16 cm−1) and number of co-added scans (2, 16 and 128), for a total of nine different combinations. Representative spectra for each combination of parameters are shown in ESI Fig. S4 (PE) and S5 (PP).† The polymer identity of each tested particle was also confirmed with ATR-FTIR analysis.
The dataset was split into subsets to calibrate (n = 20) and validate (n = 45) a PLS-DA model for polymer prediction (Fig. 3). A separate PLS-DA model was generated for each set of experimental parameters. The optimal parameters were chosen based on the sensitivity of the classification model at each combination of parameters. An 8 cm−1 spectral resolution and two co-added scans were found to be sufficient for polymer discrimination for both PE and PP, while also reducing data collection time and minimising file sizes for data storage.
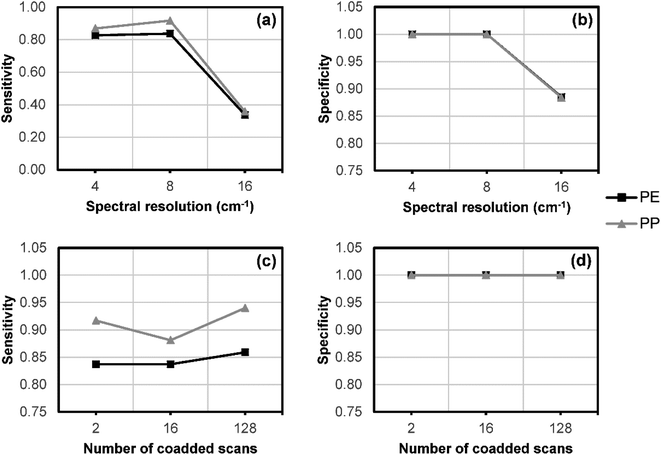 |
| Fig. 3 Change in (a) sensitivity* and (b) specificity** of PLS-DA polymer prediction with decreasing spectral resolution at 2 co-added scans. Change in (c) sensitivity and (d) specificity with increasing number of co-added scans at an 8 cm−1 spectral resolution. Data points overlap in (b) and (d). The data collection time for a spectrum collected with 2 co-added scans at 4, 8, and 16 cm−1 is 1.8, 1.4, 0.7 seconds, respectively. The data collection time for spectra collected at 8 cm−1 spectral resolution with 2, 16, and 128 co-added scans is 1.4, 7.5, 56.4 seconds, respectively. *Sensitivity defined as the true positive rate (TP = true positive, FN = false negative): **Specificity defined as the true negative rate (TN = true negative, FP = false positive):  | |
Use of multivariate analysis to enable semi-automated data processing for microplastic identification
Previous investigations of reflectance-FTIR microscopy for microplastic analysis have used univariate imaging methods for particle detection.8,17,18,32 Since many polymers have absorbance bands in similar regions, it is difficult or sometimes impossible to select a region that differentiates a single polymer type from a diverse set of polymers. This is particularly true of reflectance-FTIR spectra, where refractive index and particle morphology can change the position, shape or intensity of key absorbance bands.29–31
Herein, we report the capability of multivariate statistics to enable semi-automated data processing, and more rapid and objective identification of microplastics from reflectance-FTIR. Initially, our model was calibrated with commercially sourced microplastics, before being validated on both a different set of commercial microplastics, and a “real-world” industry sourced marine salt sample. The PLS-DA model was calibrated with reflectance-FTIR spectra (n = 8 spectra for each polymer) recorded from a filter paper spiked with polymers in known locations (Fig. 4A–D). Principle components 1 and 3 provided the best visual discrimination between polymer types in the calibration model (Fig. 4A and B, loadings shown in Fig. 4C). To determine predictions of polymer type, each spectrum in a reflectance-FTIR image was classified by the PLS-DA model, and the classifications reconstructed into a false colour image (Fig. 4D and E). Although the best visual separation between polymer types in a 2D PCA scatter plot was observed between component 1 and 3, the PLS-DA model was built from the first 10 principal components (PCs). Overall, the false colour image (Fig. 4E) indicates that the PLS-DA combined with reflectance-FTIR imaging results in excellent identification of particle abundance, distribution and polymer classification. The model was evaluated by confirming the predicted classification of 96 particles (n = 16 per polymer) on a filter on which reference polymers were at unknown locations (Fig. 4F and G). Correct classification rates were 100% for PE, PP, PS, and PMMA, 94% for PET, and 88% for PA (Fig. 4H).
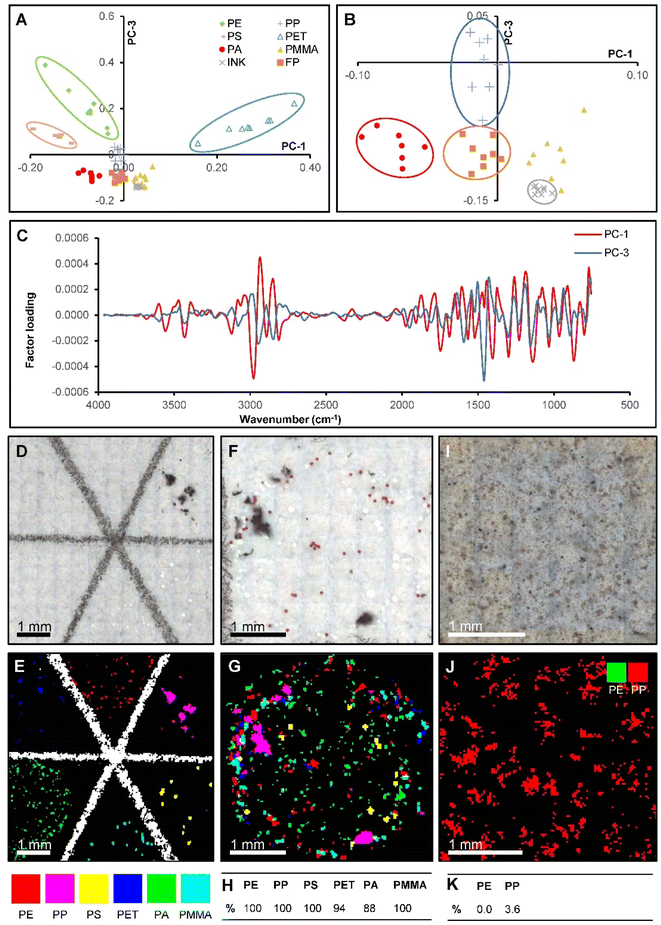 |
| Fig. 4 (A) PLS-DA x-scores plot for the standard polymer particle calibration dataset. Separated clusters have been circled. (B) Enlarged view of tightly grouped clusters in (A) shows all polymers could be separated using only components 1 and 3. (C) PLS-DA x-loadings for components 1 and 3. (D) Optical image and (E) false colour map showing PLS-DA polymer classifications for standard polymer particles spiked in known locations on cellulose filter paper. (F) Optical image and (G) false colour map showing PLS-DA polymer classifications for mixed standard polymer particles spiked on cellulose filter paper. (H) Table showing correct classification rates for the mixed standard sample determined via micro-ATR-FTIR. (I) Optical image and (J) false colour map showing PLS-DA polymer classifications for a marine salt sample known to contain PE and PP on mixed cellulose ester filter paper. Many FTIR spectra in (J) classify as PP even though there was no particle visibly located there, with the mis-classification subsequently confirmed with ATR-FTIR. (K) Table showing correct classification rates for PE and PP in the marine salt sample determined via micro-ATR-FTIR. | |
To investigate the applicability of the PLS-DA model to real environmental samples, the method was then applied to reflectance FTIR images of a filter containing microplastics separated from marine salt (Fig. 4I and J). ATR-FTIR was used to identify whether the filter paper contained PE and PP microplastics. Unfortunately, the PLS-DA model was largely unable to accurately identify PP and PE particles, with correct classification rates of 0% for PE (33 particles) and 3.6% for PP (32 particles), reflected in the poor detection rates in the false colour image (Fig. 4F). A likely cause for the poor performance of the model is spectral variation from factors other than the chemical composition of the sample. These factors include particle morphology and the degree of weathering which may introducing spectral distortions due to differences in scattering artefacts.
Effect of morphology and plastic weathering on micro-reflectance-FTIR spectra and subsequent multivariate analysis
Following the poor performance of the PLS-DA model, built from reflectance-FTIR spectra acquired from commercially sourced microplastics, the effect of sample shape and morphology on reflectance-FTIR spectra was investigated. It is well-known that the irregular particle morphology can lead to the introduction of refractive errors in reflectance-FTIR spectra.8,12,18,19,29,31
The reflectance-FTIR spectra and SEM images of four PE particles with different morphologies were obtained; a commercially sourced sphere (Fig. 5A), a commercially sourced granule (Fig. 5B), a commercially sourced film (Fig. 5C), and a weathered particle obtained from the marine salt samples (Fig. 5D). All had visually discernible variations in their micro-reflectance-FTIR spectra. The sphere exhibited a distinct derivative-like line shape of the symmetric and asymmetric CH stretch absorbance bands, with peak maxima shifted from their normal occurrences at 2915 cm−1 and 2849 cm−1 to 2933 cm−1 and 2860 cm−1, respectively. The granule exhibited a distorted CH stretching region. The film and the weathered particle both showed an additional feature between the two expected CH stretching bands. These are best described as dispersion artefacts, similar to those observed by Bassan, Byrne.29 The film also showed a thin-film interference effect in the undulating baseline, documented as the result of internal reflection of the infrared beam.33,34 Based on these results a PLS-DA model was developed using a sub-set of particles from the marine salt library, which had their identity confirmed as either PE or PP by using micro-ATR-FTIR.
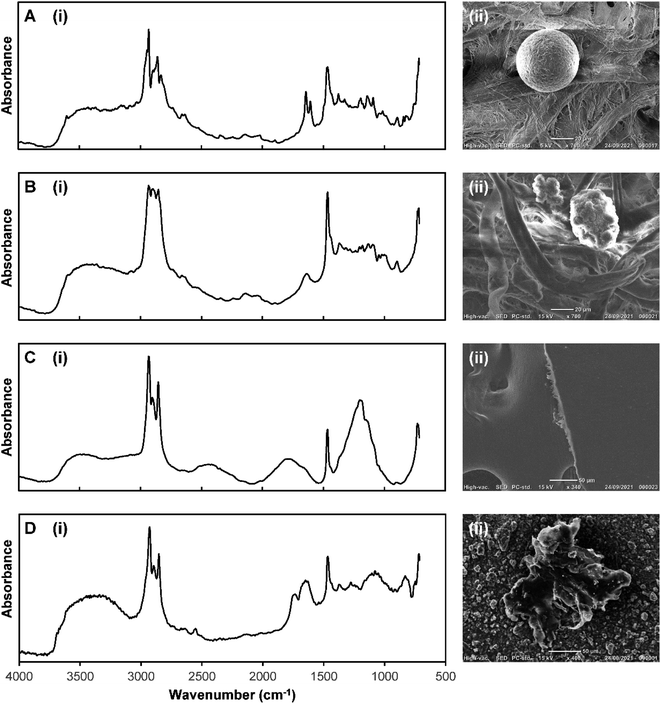 |
| Fig. 5 (i) Micro-reflectance-FTIR spectra and (ii) SEM images of PE particles with different morphologies: (A) sphere, (B) granule, (C) film, and (D) weathered particle (from environmental marine salt sample). Please note, the ATR-FTIR spectrum of virgin PE microplastic is presented in Fig. 2C. Also note that the spectra in panels (A)–(D) are the average of 4 individual spectra (pixels) from each particle, and have been min–max normalised to a range of 0–2, to simplify visual comparison. The raw unprocessed spectra are shown in ESI Fig. S6.† | |
Improved detection of microplastics in environmental marine salt samples
To improve the accuracy of predicting the polymer identity of microplastic particles, we developed a PLS-DA model derived from a subset of plastic particles found in marine salt that have similar morphology and weathering profiles. The feasibility of this approach was tested on PP and PE particles in the marine salt sample. Fig. 6 shows representative examples of the optical images and ATR-FTIR and reflectance-FTIR imaging spectra of a PE (Fig. 6A) and PP (Fig. 6B) particle. Not unexpectedly, the reflectance-FTIR display spectral interference and relatively low S/N (when compared to ATR-FTIR) which prohibits univariate analysis or straight forward visual inspection of the reflectance-FTIR spectra to confirm polymer identity. However, sufficient chemical information is contained within the reflectance-FTIR spectra to enable identification of polymer type through multivariate analysis. Visual discrimination between spectra of the PE and PP particles, in addition to filter paper was evident in a 2D scatter plot of PC1 and PC2 (Fig. 6C), with the corresponding loadings shown in Fig. 6D. On a per-spectrum basis (with n = 4 spectra per particle), this method achieved specificities of 100% for both PE and PP, with a sensitivity of 78% for PE, and 82% for PP, which equates to prediction accuracies of 85% (PE) and 95% (PP) on a per-particle basis (confirmed by ATR-FTIR), as shown in Fig. 6E–G. A representative example of the new PLS-DA model to image microplastics in the marine salt sample is shown in Fig. 6F, which shows the improvement in classification relative to the previous model (Fig. 4J).
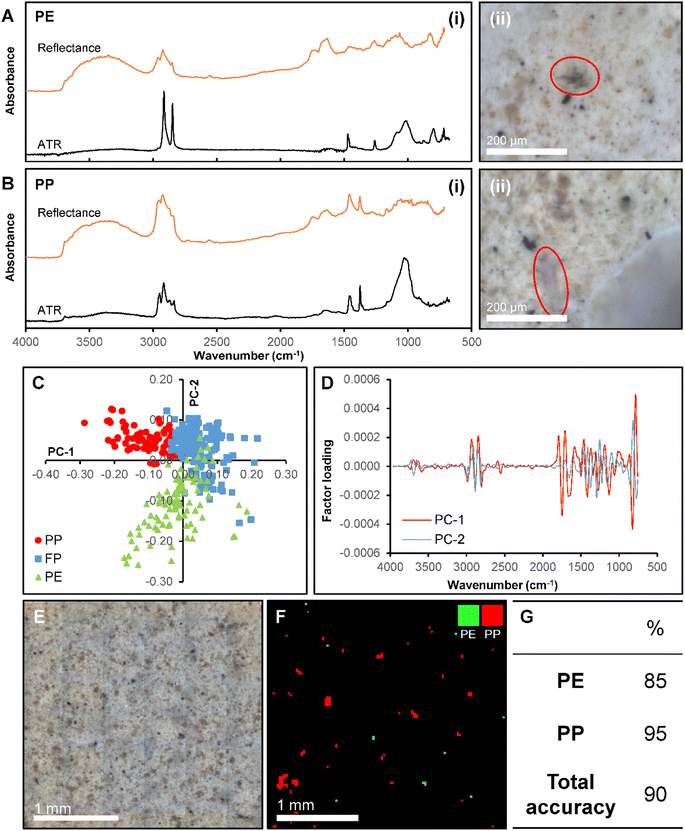 |
| Fig. 6 (i) Comparison of micro-ATR-FTIR (lower) and micro-reflectance-FTIR (upper) spectra and (ii) optical images for representative (A) PE and (B) PP particles in a marine salt sample. (C) x-Scores plot and (D) x-loadings plot for a PLS-DA model calibrated with spectra from PE and PP particles in a marine salt sample. (E) Optical image and (F) false colour map showing PLS-DA polymer classifications for an area of the marine salt sample filter paper. (G) Table showing correct classification rates for the marine salt sample determined via micro-ATR-FTIR. | |
The benefit of our reflectance-FTIR imaging approach is the identification of a larger number of unknown particles while undertaking a relatively limited number of time-consuming ATR-FTIR spectroscopic confirmations. For example, the calibration dataset contained reflectance-FTIR spectra from 10 PE and 10 PP particles, where ATR-FTIR has previously been used to determine the particle polymer. This calibration data was then used to predict the identity of 45 “unknown” particles, which represents a substantial time saving. The average time to collect a single ATR-FTIR spectrum was ∼3 minutes of data collection (single channel background and sample collection), and with an additional 3 minutes per particle to align the micro-ATR crystal and optimise the pressure between the crystal and particle. Therefore, to determine the identity of only one “unknown” particle requires a minimum of 6 minutes of work from the instrument operator. In this the determination of 45 unknown particles equates to 270 minutes of data collection, for which the instrument operator is required to be present. With the use of reflectance-FTIR imaging, a 2.5 × 2.5 mm area of the filter paper can be imaged in 12 minutes and the instrument operator is not required to be present once data collection has begun. The total reflectance-FTIR data collection time for the 45 “unknown” particles in our study was 36 minutes, which is an improvement by a factor of 7.5 with the added advantage of the instrument operator not required to be physically present at the instrument during data collection.
It should be noted that the exact time advantage offered by reflectance-FTIR imaging relative to ATR-FTIR will vary between different sample types. The greatest time advantage of reflectance-FTIR will occur when collecting data from samples containing a large number of particles, such that each individual filter paper contains many closely spaced particles. Under these conditions, studies aiming to analyse hundreds or thousands of particles are likely to receive a time advantage of one or two orders of magnitude by using reflectance-FTIR relative to ATR-FTIR. In contrast, for studies of samples that are relatively particle free, containing several particles per filter paper, the time advantage of reflectance-FTIR is lost, as most of the time is spent collecting data from the filter paper and not particles. Furthermore, the reflectance-FTIR approach classifies all particles present on the filter paper, rather than identifying and classifying a small sub-sample using the traditional approach which combines optical microscopy and ATR-FTIR, typically cited in the microplastics literature.
Conclusion
This study confirmed the reflectance-FTIR spectra of multiple microplastic types, despite containing numerous spectral distortions, are inherently sensitive to polymer identity. Coupling reflectance-FTIR imaging with a semi-automated data processing pipeline using PLS-DA for spectral classification demonstrates that this approach fulfils the requirements for a rapid, high throughput, cost-effective analytical protocol. Specifically, microplastics from marine salt samples were identified with 100% specificity (PE and PP) and sensitivities of 78% (PE) and 82% (PP). Not surprisingly, this study highlighted that sample size, shape, and morphology had a pronounced effect on the reflectance-FTIR signal, which must be taken into consideration when developing spectral libraries for predictions of unknowns. Future research would benefit from expanding the development of reflectance-FTIR libraries plus integrating this method with multivariate and machine learning pipelines, similar to what has been achieved for transmission-FTIR spectroscopy. The development of integrated methods can be expected to yield microplastic analysis protocols that are more cost effective than those using transmission-FTIR spectroscopy, and which also do not suffer from total-infrared absorption like that occurs in transmission-FTIR spectroscopy. The latter benefit allowing a greater size range of particles to be analysed. Lastly, this study has utilised PLS-DA as a routinely used and easily accessible multivariate tool to build the analytical protocol. However, a range of multivariate strategies exist, including more complex and advanced methods such as machine learning or AI. Future work is now needed to optimise the computational and statistical components of this protocol. Overall, this research demonstrated the immense potential of reflectance-FTIR to provide semi-automated plastic particle identification, at substantially lower cost than current methods. Lowering the cost is essential, if industry and the environmental sector in general is going to adopt routine and regular microplastic monitoring.
Conflicts of interest
There are no conflicts to declare.
Acknowledgements
Thanks to Dr Fatima Naim at the Centre for Crop Disease Management (CCDM) at Curtin University for assistance with collection of SEM data. MW acknowledges support from an Australian Institute of Nuclear Science and Engineering (AINSE) Honours Award. We gratefully acknowledge travel funding provided by ANSTO, funded by the Australian Government. Components of this research were undertaken at the infrared microspectroscopy beamline (IRM) at the Australian Synchrotron, ANSTO, Victoria, Australia.
References
- J. P. Frias and R. Nash, Microplastics: finding a consensus on the definition, Mar. Pollut. Bull., 2019, 138, 145–147 CrossRef CAS PubMed
.
- J. G. Derraik, The pollution of the marine environment by plastic debris: a review, Mar. Pollut. Bull., 2002, 44(9), 842–852 CrossRef CAS PubMed
.
- D. Brennecke, B. Duarte, F. Paiva, I. Caçador and J. Canning-Clode, Microplastics as vector for heavy metal contamination from the marine environment, Estuarine, Coastal Shelf Sci., 2016, 178, 189–195 CrossRef CAS
.
- C. M. Rochman, E. Hoh, B. T. Hentschel and S. Kaye, Long-term field measurement of sorption of organic contaminants to five types of plastic pellets: implications for plastic marine debris, Environ. Sci. Technol., 2013, 47(3), 1646–1654 CAS
.
- E. L. Teuten, J. M. Saquing, D. R. Knappe, M. A. Barlaz, S. Jonsson and A. Björn,
et al., Transport and release of chemicals from plastics to the environment and to wildlife, Philos. Trans. R. Soc., B, 2009, 364(1526), 2027–2045 CrossRef CAS PubMed
.
- J. E. Halstead, J. A. Smith, E. A. Carter, P. A. Lay and E. L. Johnston, Assessment tools for microplastics and natural fibres ingested by fish in an urbanised estuary, Environ. Pollut., 2018, 234, 552–561 CrossRef CAS PubMed
.
- V. Hidalgo-Ruz, L. Gutow, R. C. Thompson and M. Thiel, Microplastics in the marine environment: a review of the methods used for identification and quantification, Environ. Sci. Technol., 2012, 46(6), 3060–3075 CrossRef CAS PubMed
.
- M. G. J. Löder, M. Kuczera, S. Mintenig, C. Lorenz and G. Gerdts, Focal plane array detector-based micro-Fourier-transform infrared imaging for the analysis of microplastics in environmental samples, Environ. Chem., 2015, 12(5), 563–581 CrossRef
.
- S. Primpke, C. Lorenz, R. Rascher-Friesenhausen and G. Gerdts, An automated approach for microplastics analysis using focal plane array (FPA) FTIR microscopy and image analysis, Anal. Methods, 2017, 9(9), 1499–1511 RSC
.
- M. R. Jung, F. D. Horgen, S. V. Orski, V. Rodriguez, K. L. Beers and G. H. Balazs,
et al., Validation of ATR FT-IR to identify polymers of plastic marine debris, including those ingested by marine organisms, Mar. Pollut. Bull., 2018, 127, 704–716 CrossRef CAS PubMed
.
- N. Lutz, J. Fogarty and A. Rate, Accumulation and potential for transport of microplastics in stormwater drains into marine environments, Perth region, Western Australia, Mar. Pollut. Bull., 2021, 168, 112362 CrossRef CAS PubMed
.
- A. Käppler, D. Fischer, S. Oberbeckmann, G. Schernewski, M. Labrenz and K.-J. Eichhorn,
et al., Analysis of environmental microplastics by vibrational microspectroscopy: FTIR, Raman or both?, Anal. Bioanal. Chem., 2016, 408(29), 8377–8391 CrossRef PubMed
.
- R. Lenz, K. Enders, C. A. Stedmon, D. M. Mackenzie and T. G. Nielsen, A critical assessment of visual identification of marine microplastic using Raman spectroscopy for analysis improvement, Mar. Pollut. Bull., 2015, 100(1), 82–91 CrossRef CAS PubMed
.
- C. F. Araujo, M. M. Nolasco, A. M. Ribeiro and P. J. Ribeiro-Claro, Identification of microplastics using Raman spectroscopy: latest developments and future prospects, Water Res., 2018, 142, 426–440 CrossRef CAS PubMed
.
- B. Hufnagl, M. Stibi, H. Martirosyan, U. Wilczek, J. N. Möller and M. G. Löder,
et al., Computer-assisted analysis of microplastics in environmental samples based on μFTIR imaging in combination with machine learning, Environ. Sci. Technol. Lett., 2021, 9(1), 90–95 CrossRef PubMed
.
- A. S. Tagg, M. Sapp, J. P. Harrison and J. J. Ojeda, Identification and quantification of microplastics in wastewater using focal plane array-based reflectance micro-FT-IR imaging, Anal. Chem., 2015, 87(12), 6032–6040 CrossRef CAS PubMed
.
- J. P. Harrison, J. J. Ojeda and M. E. Romero-González, The applicability of reflectance micro-Fourier-transform infrared
spectroscopy for the detection of synthetic microplastics in marine sediments, Sci. Total Environ., 2012, 416, 455–463 CrossRef CAS PubMed
.
- A. S. Tagg, M. Sapp, J. P. Harrison and J. J. Ojeda, Identification and Quantification of Microplastics in Wastewater Using Focal Plane Array-Based Reflectance Micro-FT-IR Imaging, Anal. Chem., 2015, 87(12), 6032–6040 CrossRef CAS PubMed
.
- B. O. Budevska, Minimization of optical non-linearities in Fourier transform-infrared microspectroscopic imaging, Vib. Spectrosc., 2000, 24(1), 37–45 CrossRef CAS
.
- B. J. Davis, P. S. Carney and R. Bhargava, Theory of Mid-infrared Absorption Microspectroscopy: II. Heterogeneous Samples, Anal. Chem., 2010, 82(9), 3487–3499 CrossRef CAS PubMed
.
- M. Picollo, G. Bartolozzi, C. Cucci, M. Galeotti, V. Marchiafava and B. Pizzo, Comparative study of Fourier transform infrared spectroscopy in transmission, attenuated total reflection, and total reflection modes for the analysis of plastics in the cultural heritage field, Appl. Spectrosc., 2014, 68(4), 389–396 CrossRef CAS PubMed
.
- A. S. Tagg, M. Sapp, J. P. Harrison, C. J. Sinclair, E. Bradley and Y. Ju-Nam,
et al., Microplastic monitoring at different stages in a wastewater treatment plant using reflectance micro-FTIR imaging, Front. Environ. Sci., 2020, 8, 145 CrossRef
.
- J. Vongsvivut, P. Heraud, A. Gupta, M. Puri, D. McNaughton and C. J. Barrow, FTIR microspectroscopy for rapid screening and monitoring of polyunsaturated fatty acid production in commercially valuable marine yeasts and protists, Analyst, 2013, 138(20), 6016–6031 RSC
.
- B. R. Wood, M. A. Quinn, F. R. Burden and D. McNaughton, An investigation into FTIR spectroscopy as a biodiagnostic tool for cervical cancer, Biospectroscopy, 1996, 2(3), 143–153 CrossRef CAS
.
- Y. Chen, D. Wen, J. Pei, Y. Fei, D. Ouyang and H. Zhang,
et al., Identification and quantification of microplastics using Fourier-transform infrared spectroscopy: current status and future prospects, Curr. Opin. Environ. Sci. Health, 2020, 18, 14–19 CrossRef
.
- V. H. da Silva, F. Murphy, J. M. Amigo, C. Stedmon and J. Strand, Classification and Quantification of Microplastics (<100 μm) Using a Focal Plane Array–Fourier Transform Infrared Imaging System and Machine Learning, Anal. Chem., 2020, 92(20), 13724–13733 CrossRef CAS PubMed
.
- P. Lasch and D. Naumann, Spatial Resolution in Infrared Microspectroscopic Imaging of Tissues, Biochim. Biophys. Acta, 2006, 1758, 814–829 CrossRef CAS PubMed
.
- E. C. Mattson, M. Unger, B. Manandhar, Z. Alavi and C. J. Hirschmugl, Multi-beam Synchrotron FPA Chemical Imaging: Impact of Schwarzchild Objective on Spatial Resolution, J. Phys.: Conf. Ser., 2013, 142001 CrossRef
.
- P. Bassan, H. J. Byrne, F. Bonnier, J. Lee, P. Dumas and P. Gardner, Resonant Mie scattering in infrared spectroscopy of biological materials – understanding the ‘dispersion artefact’, Analyst, 2009, 134(8), 1586–1593 RSC
.
- E. M. Angelin, S. F. de Sá, I. Soares, M. E. Callapez, J. L. Ferreira and M. J. Melo,
et al., Application of Infrared Reflectance Spectroscopy on Plastics in Cultural Heritage Collections: A Comparative Assessment of Two Portable Mid-Fourier Transform Infrared Reflection Devices, Appl. Spectrosc., 2021, 75(7), 818–833 CrossRef CAS PubMed
.
- P. Bassan, H. J. Byrne, J. Lee, F. Bonnier, C. Clarke and P. Dumas,
et al., Reflection contributions to the dispersion artefact in FTIR spectra of single biological cells, Analyst, 2009, 134(6), 1171–1175 RSC
.
- A. S. Tagg, M. Sapp, J. P. Harrison, C. J. Sinclair, E. Bradley and Y. Ju-Nam,
et al., Microplastic Monitoring at Different Stages in a Wastewater Treatment Plant Using Reflectance Micro-FTIR Imaging, Front. Environ. Sci., 2020, 8, 145 CrossRef
.
-
N. Smyrl, Infrared Interferometric Thickness Measurement of Polymeric Thin Films and Coatings, Oak Ridge
Y-12 Plant, TN, USA, 1979 Search PubMed
.
- T. Konevskikh, A. Ponossov, R. Blümel, R. Lukacs and A. Kohler, Fringes in FTIR spectroscopy revisited: understanding and modelling fringes in infrared spectroscopy of thin films, Analyst, 2015, 140(12), 3969–3980 RSC
.
|
This journal is © The Royal Society of Chemistry 2023 |
Click here to see how this site uses Cookies. View our privacy policy here.