DOI:
10.1039/D4GC01877J
(Paper)
Green Chem., 2024,
26, 9142-9155
Molecular origins of enhanced bioproduct properties by pretreatment of agricultural residues with deep eutectic solvents†
Received
16th April 2024
, Accepted 28th June 2024
First published on 11th July 2024
Abstract
Pretreatment facilitates cost-effective operations on lignocellulosic biomass ranging from densification to deconstruction and bioproduct development. However, determining molecular-level mechanisms behind pretreatment and their effects has remained elusive. Here, we combine computational simulation and experiment to investigate the effects on wheat straw agricultural residue densification of an emerging pretreatment solvent, namely, a deep eutectic solvent (DES) consisting of choline chloride (ChCl) and oxalic acid (OA). Ab initio molecular dynamics indicates that dissociation of lignin from cellulose in lignin–carbohydrate complexes, which does not occur to a significant extent in aqueous solution, is favorable in the DES and occurs via cleavage of the guaiacyl
:
xylose ether bond linkage by OA. The ensuing hemicellulose removal exposes lignin to the DES which, molecular dynamics simulation indicates, leads to lignin expansion. The resulting changes in wheat straw fiber structure, lignin distribution, and functional group modifications upon DES treatment by scanning electron and fluorescence microscopy along with Fourier-transform infrared spectroscopy. The molecular expansion of lignin enhances inter-particle binding in wheat straw, leading to denser structures under pelletization. The resulting high mechanical stability and combustion properties make the wheat straw a suitable precursor of high-quality densified solids (e.g., solid biofuel). Overall, we shed light on the molecular-level mechanisms involved in DES pretreatment for biomass densification, demonstrated here in the development of a solid biofuel. The approach here illuminates the rational design from first chemical principles of methods to convert lignocellulosic resources into advanced materials.
1. Introduction
Developing workable routes for migrating from contemporary bioproduct and fuel production to sustainable practices using green resources is challenging. Lignocellulosic biomass, the most plentiful source of organic carbon on Earth, is a potentially ideal alternative resource for isolating bio-based green chemicals and energy precursors so as to curtail dependence on fossil carbon and serve future bioindustries.1–3 However, the extraction of valuable compounds from biomass is hampered by the robust heterogeneous plant cell wall structure. Lignin, among the three most abundant biopolymers in the secondary cell wall of lignocellulosic biomass, consists of a complex network of cross-linked aromatic molecules and is bonded to plant cell wall polysaccharides to form lignin–carbohydrate complexes (LCCs).4 LCCs are a primary reason for the recalcitrance of lignocellulosic biomass,5,6 forming a physical barrier that blocks contact with cellulose, negatively impacting its processing such as, for example, saccharification during bioconversion.7 Increasing cellulose accessibility along with creating value-added products produced from lignin has yet to result in complete usage of carbon from lignocellulosic feedstocks. Also, agricultural residues such as wheat straw usually contain a high hemicellulose content, ∼40%,8 which again hinders the accessibility of cellulose and the efficiency for the utilization of this resource.7
As the most plentiful sustainable supply of aromatic chemicals,9,10 lignin has long been acknowledged for its potential as a feedstock for the production of renewable chemicals, materials, and fuels.11,12 Accordingly, the quest for developing effective, environmentally friendly methods that can increase lignin extraction and transformation while improving the efficiency of carbohydrate access has become most critical. Pretreatment techniques utilizing multifunctional cosolvents have shown promising prospects. For example, it has been reported that tetrahydrofuran–water cosolvents promote lignin depolymerization, liberation from cellulose, and cellulose reactivity, all of which contribute to improved biomass use.13 Other work has shown that deep eutectic solvents (DESs) are green and recyclable alternatives that can be easily modularized from a wide variety of hydrogen bond donors (such as amides, amines, alcohols, sugar alcohols and acids) and acceptors (such as choline chloride, acetylcholine chloride, and benzyl dimethyl ammonium chloride).14,15 Traditional pretreatment methods, such as steam explosion and hydrothermal carbonization, require high quality pressure vessels and high temperature steam (180–260 °C).16,17 These methods are more energy intensive than DES pretreatments, which require temperatures between 60–120 °C. Moreover, DESs are easily prepared, inexpensive, nontoxic, and biodegradable.18 Numerous binary and ternary DES formulations have been proposed for lignocellulose fractionation with encouraging results in their capacity to deconstruct robust interactions between lignin and cellulose.18–21
An important challenge is to understand the effects of cosolvents such as DESs on 3D biomass structure and the chemical reactions involved in biomass deconstruction. Computational chemistry can play an important role in a molecular level understanding of these processes. Some computational studies have been performed on bond cleavage in related systems. For example, an MD study investigated bond cleavage of a phenyl glycoside lignin–carbohydrate complex in ChCl-based DES but used the highly approximate ReaxFF method.22 Lignin has both C–O and C–C bonds. β-O-4 linkages consist of C–O bonds, whereas β-5 linkages consist of C–C bonds. Another MD study showed that both β-O-4 (i.e., C–O) and β-5 (i.e., C–C) linkages within lignin bonds are stretched under electric fields imparted during microwave irradiation and proposed that this effect increases the probability of bond breaking.23
Chemical reactions cannot easily be explicitly modeled with classical molecular mechanics force fields and quantum chemical studies are required. In this regard recent work using density functional theory (DFT) and classical molecular dynamics (MD) simulations has explored the mechanism of dissociation of hemicelluloses using lactic acid-based DESs.24 However, the DFT calculations were applied only to optimize the structure of the DES and to estimate noncovalent interactions; chemical reactions of hemicelluloses or lignin during DES treatment were not examined. Therefore, taken together the above studies point to the existence of conformational changes and chemical reactions during DES treatment, but that an understanding of these multiscale structural effects and reaction mechanisms is lacking.
Our previous work investigated the potential of DES treated biomass for solid biofuel production. The mixture of raw wheat straw and DES-treated wheat straw exhibited improvements in hydrophobicity and mechanical strength.25 However, the mechanism of action at molecular detail was not determined in this paper. In this study, we applied extensive computations with large scale classical all-atom MD to calculate both lignin configurational changes and smaller scale ab initio MD simulation to determine chemical reaction mechanisms and free energies. Classical all-atom molecular dynamics (MD) simulations are used here to examine the physical properties of the biomass polymers upon DES treatment. We also examine the cleavage of LCCs, which plays an essential role in hemicellulose removal, using ab initio molecular dynamics (AIMD) simulations based on quantum mechanics with density functional theory (DFT) that provide accurate descriptions of bond cleavage and formation in biomass DES systems. In addition, we carried out experiments on wheat straw to examine how these molecular effects translate to the macroscopic level. For this purpose, we noted that acid-based DESs are generally more efficient for xylan removal than other DES types.26,27 Moreover, a DES consisting of choline chloride (ChCl) and oxalic acid (OA) has been shown to exhibit excellent hemicellulose removal (xylan dropped from 20.6% to 1.5%) from rice straw at 120 °C for 4 h while largely keeping the lignin content of 22.8%.28 This DES was used here to obtain upgraded feedstock for downstream conversion. The experiments, including scanning electron microscopy, fluorescence microscopy, and Fourier-transform infrared spectroscopy, determine the fibril structure, lignin distribution, and functional group modifications after DES treatment.
We show that DES-treated wheat straw is a promising route to solid biofuel and bioproduct precursors, encouraging durability, hardness, and water resistance, all of which are relevant to transport, processing, and manufacturing. The results provide a rationale linking the molecular-level changes to macroscopic wheat straw densification in solid fuels and bioproduct precursors and provide a pathway for rational design based on molecular-level processes for the circular bioeconomy.
2. Materials and methods
2.1. Sample preparation
Wheat straw was obtained from a farm in Alberta, Canada. Straw was hammer milled (Model 10HMBL, Glen Mills Inc.) to below 3.18 mm particle size in the Biomass and Bioenergy Research Group at the University of British Columbia. The ground sample was oven dried at 50 °C for 48 h to ensure the mass was consistent. The dried sample was then sealed in a glass container until further processing. DES solutions were prepared by mixing choline chloride (ChCl) and oxalic acid (OA) at the molar ratios of 2
:
1, 1
:
1, and 1
:
2. Mixtures were heated in an oil bath at 80 °C under stirring until a clear solution formed. 10 g wheat straw was mixed in a glass flask with 100 g of each DES solution, and the suspensions were stirred at 80 °C for 1 h. The whole experimental process is shown in Fig. S1.† The solids were weighed after drying at 50 °C for 48 h. The treated samples were stored at room temperature in glass jars until further analysis. The treatment conditions of DESs with molar ratios of ChCl to OA of 2
:
1, 1
:
1, and 1
:
2 are denoted as DES2-1, DES1-1, and DES1-2.
2.2. MD simulations
All classical MD simulations were conducted using GROMACS version 2020.629 with the CHARMM36 force field for carbohydrates.30,31 The stable cellulose Iβ crystalline structure exists in wheat straw.32 and was therefore used to investigate the cellulose–lignin interfaces in the corresponding DES solution environment. The systems included oxalic acid, choline chloride, cellulose Iβ complex, and lignin models. The initial systems were constructed in a rectangular box with dimensions 200 Å × 85 Å × 98 Å using Packmol.33 There were ∼18k atoms in each of the three different molar ratio (ChCl to OA 2
:
1, 1
:
1, and 1
:
2) DES systems. A detailed explanation of the construction process for the cellulose crystal and lignin models is provided in ESI sections S1 and S2.† The configurations underwent energy minimization using both steepest descent and conjugate gradient methods, ensuring convergence when the maximum force was less than 100 kJ mol−1 nm−1.
Following the initial energy minimization, a pre-equilibration procedure was performed in the NPT ensemble at 353.15 K using the velocity-rescaling method for temperature coupling with a time constant of 1.0 ps.34 A pressure of 1 bar was maintained using the Berendsen algorithm for pressure coupling with a time constant of 0.5 ps.35 This pre-equilibration phase lasted for 1 ns. Subsequently, the production simulation was carried out in the same NPT ensemble, maintaining a temperature of 353.15 K and a pressure of 1 bar. This phase was characterized by a longer time constant of 2.0 ps and spanned a duration of 100 ns. The pressure coupling method was switched to the Parrinello-Rahman algorithm during the production simulation,36 while temperature coupling using the velocity-rescaling method34 remained consistent throughout the process. During all simulations, the particle mesh Ewald (PME) approach was used to evaluate long-range electrostatic interactions.37 Short-range coulombic and van der Waals (vdW) interactions were cut off at 12 Å. The LINCS algorithm was applied to constrain all covalent bonds to hydrogen.38 Lignin was constructed using the LigninBuilder tool.39 The oxalic acid and choline chloride were constructed by CHARMM-GUI.40 Visualization and analysis of MD trajectories were carried out with VMD version 1.9.3.41
2.4.
Ab initio molecular dynamics (AIMD) simulations
AIMD simulations were performed using the QUICKSTEP module of the CP2K package (version 9.1).42 The lignin carbohydrate complex (LCC) was built with Avogadro.43 The system (LCC and DES) was modeled using an orthorhombic periodic cell of dimensions 20.0 Å × 15.0 Å × 15.0 Å. Additional details on the lignin/hemicellulose complex configurations are found in ESI section S3.† The geometries of all structures were first optimized to remove steric clashes. Atomic forces and energies were calculated using density functional theory (DFT) with the Perdew–Burke–Ernzerhof (PBE) exchange–correlation functional with D3 dispersion corrections and Becke–Johnson damping.44,45 The DZVP-MOLOPT-SR-GTH basis set was used for all atoms.46 The plane wave cutoff energy of the finest grid level was selected as 600 Ry after testing a range of values from 400–800 Ry. The total AIMD simulation was 50 ps with a time step of 1 fs. The temperature of the simulation was maintained at 353.15 K using the Canonical Sampling through Velocity Rescaling (CSVR) thermostat coupled to the system with a time constant of 200 fs.
2.5. Metadynamics
Molecular dynamics simulations may exhibit metastability in which the system is trapped in local energy minima.47 To accelerate conformational transitions between metastable states, well-tempered metadynamics was used to obtain a free energy profile for decomposition of the lignin–carbohydrate complex LCC in DES.48 The ability of metadynamics to accelerate sampling and to reconstruct free energy landscapes depends on the choice of collective variables (CVs), which are often interatomic distances. The ideal collective variable should take values in all the relevant metastable states and in the transition states between them. In a chemical reaction in which a specific bond in a molecule is broken, an appropriate CV might be the distance between the two atoms forming the bond.49 In this work, two different scenarios were tested, assuming that oxalic acid transfers a proton to the ether oxygen of the LCC, which then leads to the departure of either the lignin monomer (coniferyl alcohol) or the hemicellulose monomer (xylose) leaving groups and the formation of the corresponding OA adducts (Fig. 1a and b). Thus, we used the two distances between the carbons (two carbons in the ether bond) and the oxygen of the C–O–C ether bonds in LCC as collective variable (CV) 1 in the two scenarios (Fig. 3). The distance between the proton that is transferred from oxalic acid (HOA) and the oxygen of the ether bond in LCC (OLCC) was used as the other collective variable, CV2. Specifically, in the former scenario the first collective variable, CV1 is defined as the distance between OLCC and the carbon of the lignin monomer (CL), denoted as OLCC–CL. The second collective variable, CV2, follows the distance between OLCC and HOA, denoted as OLCC–HOA. For the latter scenario, CV1 is defined as the distance between OLCC and the carbon of the hemicellulose monomer (CH), which is denoted as OLCC–CH. CV2 is the distance between OLCC and HOA. Also, to compare with the ability of the DES to cleave the β-O-4 ether linkage with lignin, another scenario was designed in which CV1 is defined as the distance between the CL and OLCC, while CV2 is the distance between OLCC and HOA. Repulsive Gaussian potential barriers with a height of 2.5 kJ mol−1 and a width of 0.1 Å were added to the potential every 5 MD steps for all collective variables. All systems ran for 50 ps to ensure sufficient sampling (Fig. S2†).
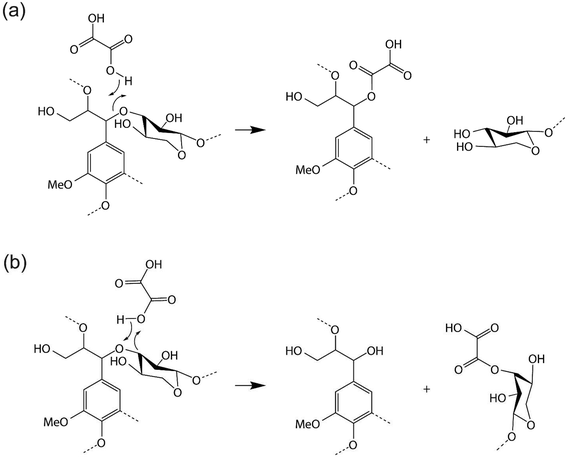 |
| Fig. 1 Proposed mechanisms: (a) Scenario 1: cleavage of the linkage between the ether oxygen (OLCC) and the α-carbon of the lignin monomer in which OA transfers a proton to OLCC, leading to the departure of the hemicellulose monomer (xylose) and formation of an adduct between OA and lignin (guaiacyl). (b) Scenario 2: cleavage of the ether linkage between OLCC and C3 of the hemicellulose monomer in which coniferyl alcohol is the leaving group and OA forms an adduct with hemicellulose (xylose). | |
2.6. Fukui functions
The Fukui function, f(r), is a fundamental regioselectivity indicator in the DFT approach to chemical reactivity50 that measures the differential change in the electron density induced by a change in the number of electrons at fixed molecular geometry. Larger values of f(r) indicate greater reactivity. The Fukui function is defined as, | 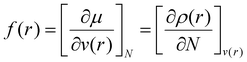 | (1) |
where N is the number of electrons in the system, μ is the chemical potential of the system, ρ(r) is the electron density, and v(r) is the external potential. The partial derivative of the electron density with respect to N is discontinuous when N is an integer, so eqn (1) cannot be calculated directly. Using finite difference approximations to calculate the left and right derivatives and their averages, the Fukui function can be associated with three types of reaction: | f0 = [f+(r) + f−(r)]/2 | (4) |
where ρN, ρN+1, and ρN−1 represent the electron densities of the system in the original state (N electrons), in the state after adding one electron (N + 1), and after removing one electron (N − 1). The function f+ measures the response of the electron density following the addition of an electron. Larger the f+ values at site r (on an atom in a molecule) indicate that the site is more reactive during a nucleophilic attack. Therefore, an atom in a molecule with a large f+ value is susceptible to nucleophilic attack.51
All Fukui function parameters and Hirshfeld charges were calculated after optimizing the geometry of the LCC model with Orca (version 5.03),52 followed by a vibrational frequency calculation to ensure that the final structure had no imaginary frequencies. All steps were calculated with the B3LYP functional53 and def2-TZVP basis set.54 The Multiwfn program version 3.8 (dev) was used to calculate the Fukui indices and Hirshfeld charges.55
3. Results and discussion
3.1. Chemical reaction sites and pathways
To provide insight into the reaction chemistry of hemicellulose removal during DES treatment, quantum chemical calculations were carried out. As visualized in Fig. 1a and b, two possibilities exist for cleaving the C–O–C ether linkages: on the lignin side or the hemicellulose side. Specifically, OA transfers a proton to an ether oxygen of LCC, which can lead to the departure of either a lignin (coniferyl alcohol) or hemicellulose (xylose) monomer. Fukui functions were calculated to obtain insights into the reactivity of the system. Subsequent AIMD simulations elucidated the potential reaction pathways based on the Fukui functions as well as the free energy profiles for the reactions.
3.1.1. Reactive site characterization with Fukui functions.
Three structures, including the whole LCC and two (xylose and coniferyl alcohol) monomers were analyzed using Fukui functions to identify favorable reaction positions for electrophilic and nucleophilic attack. The electron density isosurface of f− (Fig. 2b) suggests that OLCC is the most likely site of electrophilic attack. This result is consistent with an electrostatic potential (ESP) analysis (Fig. S3†) in which OLCC shows the most negative potential minimum.
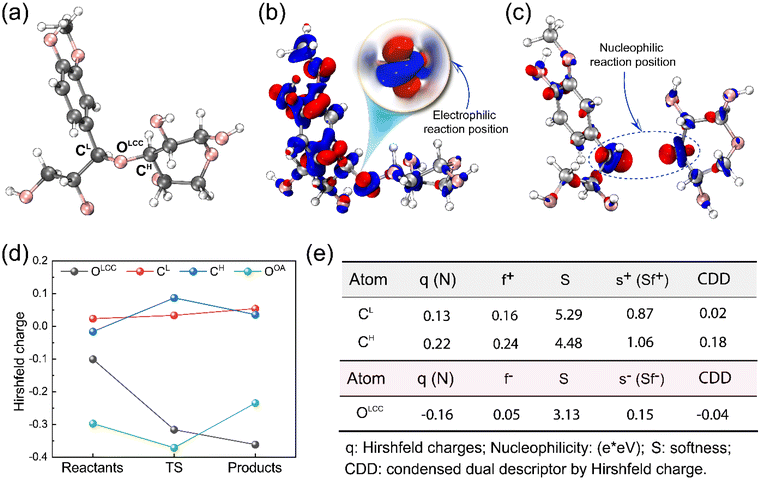 |
| Fig. 2 (a) Simplified LCC model consisting of xylose, representing a hemicellulose monomer, bonded via an ether bond to a guaiacyl unit, representing a lignin monomer; (b) f− of the LCC isosurface; (c) f+ of coniferyl alcohol and xylose isosurfaces. Red and blue correspond to the regions of positive and negative spin density, respectively. The isosurface cutoff values are ±0.005 a.u.; (d) Hirshfeld charges of selected atoms in the LCC model; (e) Fukui indices for selected atoms in LCC. | |
The Fukui function parameters for xylose and coniferyl alcohol were calculated to explore the potential nucleophilic attack site (Fig. 2c). The atom having the largest values of f+ and local softness, s+, (equal to softness S multiplied by f) is predicted to be the most favorable attack site. As shown in Fig. 2e, CH has a larger f+ value of 0.24 compared to CL of 0.16, and a larger s+ of 1.06 compared to CL of 0.87, which indicates that CH is the preferred nucleophilic attack site. To obtain further insight into the proposed reaction, we calculated the Laplacian of the electron density, ∇2ρ, a key quantity of the atoms in molecules (AIM) theory, which describes the electron density distribution within a molecule.56 Fig. S4† shows that the Laplacian of the electron density of CH in the marked region is positive, which indicates that nucleophilic attack is favorable.
The dual descriptor (DD)57 is a real-space function used to analyze the reactivity of molecular systems. DD is derived from conceptual density functional theory (CDFT), which is a theoretical approach for understanding chemical reactivity based on DFT. Condensed DD (CDD) is used to compare the DD of specific sites. The negative CDD value found for OLCC indicates that this atom is susceptible to electrophilic attack, while the positive values for CH and CL mean these atoms are subject to nucleophilic attack. The larger CDD of CH also verifies the result of local softness. Additional details of the Fukui indices are shown in Tables S1–S3.†
Monitoring changes in charge distribution along the reaction pathways provides additional insight into electrophilic and nucleophilic reactions. The calculated Hirshfeld charges show that CH is more positively charged in TS than in the reactant and the product states (Fig. 2d), indicating a greater attraction to nucleophilic reagents that can stabilize the partial positive charge. After forming a new bond, the computed charge of CH decreased in the product. Simultaneously, the Hirshfeld charge of OOA was the most negative at TS and increased after combining with CH in the product state.
3.1.2. Assessing reaction pathways via AIMD simulation.
The Fukui functions provided a clear prediction of the electrophilic and nucleophilic attack sites. Next, then, we investigated the potential reaction pathways for the cleavage of the C–O–C ether bond between hemicellulose and lignin via free energy simulation. Free energy surfaces were generated with AIMD as a function of the collective variables (CVs, see Methods). Fig. 3d and e show that there is a deep free energy minimum at the CV1 value of 3.0 Å, indicating the occurrence of ether bond cleavage and adduct formation. Fig. 3d shows the free energy profile for Scenario 1 (Fig. 1a), i.e., transfer of a proton from OA to the ether oxygen of LCC, which leads to the departure of the xylose leaving group and concurrent formation of an OA–lignin adduct. The change in free energy along the pathway is shown in Fig. 3d. The free energy barrier is 33.9 kJ mol−1 and the reaction free energy is −6.7 kJ mol−1 relative to the reactant state.
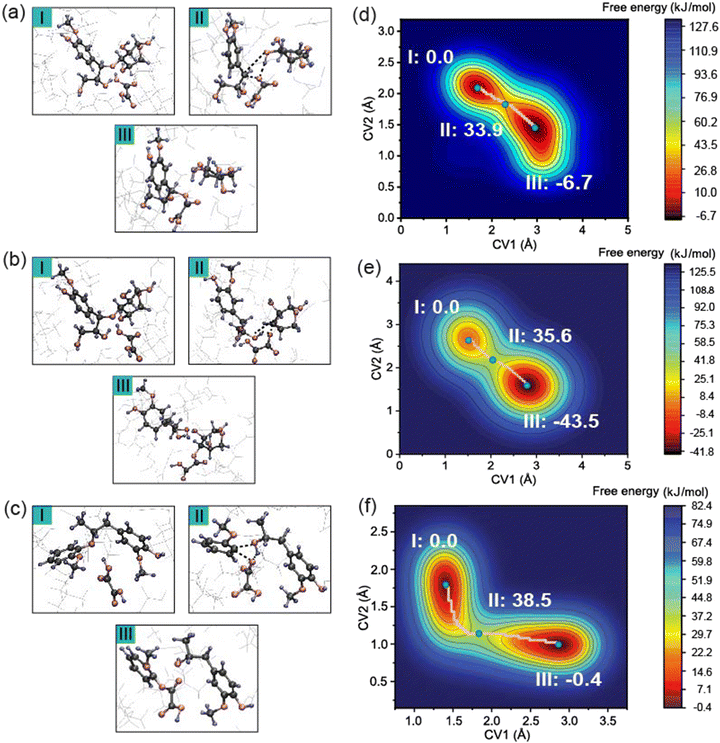 |
| Fig. 3 Selected snapshots of (I) reactants, (II) transition states, and (III) products for (a) Scenario 1; (b) Scenario 2; and (c) β-O-4 ether cleavage of lignin; 2D free energy surfaces for the reaction processes of (d) Scenario 1 and (e) Scenario 2; (f) β-O-4 ether cleavage of lignin in DES (ChCl/OA). | |
Fig. 3b presents another possible reaction pathway during the pretreatment process. Like Scenario 1, the free energy landscape in Fig. 3e exhibits deep energy minima for the reactants and products. Upon proton transfer to the ether bond oxygen, cleavage of the ether bond occurs, leading to a change in CV1 from 1.5 Å to 3.0 Å. The departure of the coniferyl alcohol leaving group and concurrent formation of an OA–xylose adduct proceeds with an activation free energy of 35.6 kJ mol−1. Thus, the free energy barriers for Scenarios 1 and 2 are nearly indistinguishable at this level of theory. However, more favorable reaction free energy of −43.5 kJ mol−1 in Scenario 2 suggests that this pathway may be the primary reaction during the pretreatment process. This pathway is also consistent with the results of the Fukui function in that CH with a larger f+ value is calculated to be the preferred nucleophilic attack site such that the cleavage of the CH–OLCC bond is more favorable.
Fig. 3c shows the typical covalent β-O-4 ether linkage between lignin monomers. The collective variables in this system were defined as the distance between the OLCC and CL and the distance between the OLCC and HOA. The free energy surface along the pathway is shown in Fig. 3f. The free energy barrier is 38.5 kJ mol−1 and the reaction free energy is negligible at −0.4 kJ mol−1. The data presented suggest that the cleavage of the ether bonds in LCC may be slightly more favorable than these in lignin, although the differences in calculated free energy barriers between these competing processes are very small.
3.2. Characteristics of the treated material
To examine the consistency between the computational and experimental results, we determined the physicochemical effects of DES treatment on wheat straw. Scanning electron microscopy (SEM) images show that the untreated wheat straw exhibits an intact fiber structure (Fig. 4a). Wheat straw treated with DES (ChCl/OA of 1
:
1 and 1
:
2, denoted DES1-1 and DES1-2) reveals that an increased oxalic acid content in DES leads to more pores, disruption, and cracks on the surface of the fibers (Fig. 4b, c and d). These features are quantified by the specific surface area (SSA) and volume of the pores (Vp), Fig. 4f. The number and volume of the pores increases as the OA content increases in the DES, increasing the microporosity of the treated samples. Additional physicochemical properties of untreated and treated wheat straw samples are provided in ESI section S4.†
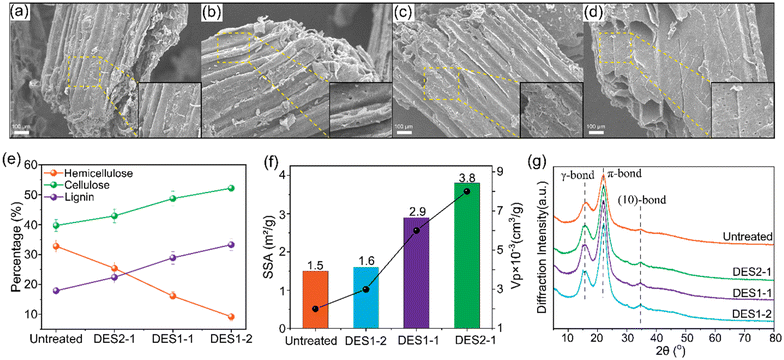 |
| Fig. 4 SEM image of (a) untreated wheat straw; (b) wheat straw treated with DES2-1; (c) wheat straw treated with DES1-1; (d) wheat straw treated with DES1-2; (e) chemical composition of untreated, DES2-1, DES1-1, and DES1-2-treated wheat straw; the yellow dashed circles highlight the specific areas with higher resolution (inset); (f) specific surface area (SSA) and volume of the pores (Vp) of untreated, DES2-1, DES1-1, and DES1-2-treated wheat straw; (g) FTIR spectra of untreated, DES2-1, DES1-1, and DES1-2-treated samples. | |
In the DES1-2 sample a significant reduction in hemicellulose content is observed, from 33% to 9%, when compared to untreated wheat straw (Fig. 4e). As a consequence, the fractional lignin content increases from 18% to 33%. In previous studies, acidic ChCl/OA DES has been shown to be effective in promoting the dissolution of hemicelluloses by cleaving lignin and lignin–carbohydrate complex linkages.58,59 Hemicelluloses have a lower crystallinity than cellulose and are easier to deconstruct.60,61 Lignin, by contrast, is resistant to degradation, often requiring a base catalyst to facilitate hydrolysis.61 Thus, the lower proportions of cellulose and lignin being decomposed compared to hemicelluloses explain the increases in their relative concentrations (from 18 to 31% for lignin and from 40% to 52% for cellulose) after DES1-2 treatment. These results are consistent with our quantum chemical studies.
Fig. 4g shows FTIR spectra for untreated and DES-treated wheat straw. The peak at 1632 cm−1 is the C
C stretching of the phenyl ring in lignin, the intensity of which increases after DES treatment, consistent with proportionally higher lignin content in the samples treated with DES. In contrast, the peaks at 1735 cm−1 and 1250 cm−1, which we attribute to the C
O stretching band of the carboxylate groups in hemicelluloses, are weaker after DES treatment. Also, the intensity of these two peaks decreases as the OA ratio increases, signifying a decrease in the amount of carboxylate groups in the hemicellulose. The vibrational peak at 893 cm−1 is attributed to the C–O–C stretching vibration of the amorphous band of cellulose. Likewise, the enhanced peak intensity coincides with the increased cellulose content.
3.3. Lignin spatial configurations
To examine the influence of DES on the modification of the spatial configurations of lignin at 80 °C, classical molecular dynamics simulations are next performed with the well-tempered metadynamics, extended adaptive biasing force (WTM-eABF) method, which is particularly cost-effective for exploring rugged free energy landscapes. Traditional eABF uses an adaptive biasing force to enhance sampling by gradually reducing energy barriers. It is less efficient in exploring complex landscapes with high-energy barriers and convergence is slower and less smooth.62 In contrast, WTM-eABF uses the metadynamics approach to deposit history-dependent biases in the form of Gaussian hills, which progressively fill up free energy basins and flatten the free energy landscape. Well-tempered metadynamics ensures that the added bias diminishes over time, preventing oversampling and leading to smoother convergence of the free energy profile. The interaction energy density was calculated to monitor non-covalent interactions. These computational techniques are herein used to determine free energy profiles, providing insights into the structural changes and modifications in lignin induced by different ChCl/OA ratios in DES.
3.3.1. Free energy of lignin expansion.
The changes of the lignin structure treated with DES2-1 and DES1-2 based on the MD simulations are illustrated in Fig. 5a and b, respectively. Lignin exhibits a more pronounced extension after DES1-2 treatment compared to that with DES2-1, suggesting a role for OA. The in-plane extension of lignin in DES1-2 can be quantitively visualized: the lignin areal footprint is approximately 20 Å longer (increased by ∼14%) in the DES1-2 simulations than in DES2-1. DES-treated lignin expands also in Y and Z directions. In Fig. S5,† a clear length increase trend in Y direction was observed (the expansion of red area). Same in Fig. S6,† the length in Z direction increased from 3.3 Å to 4.5 Å. For a given system, the radius of gyration is defined as the root mean square distance of the particles from their center of mass. The formula to calculate the radius of gyration for a set of N points (e.g., atoms or molecules) with positions ri and masses mi is defined as
, where rcm is the center of mass of the system. A low Rg indicates that the system is compact. By comparing the Rg of lignin in different DES, we can quantify the effect of different OA in DES on the compactness of lignin. The largest radius of gyration of DES1-2 among all DESs in Fig. 5c further indicates that the higher concentration of OA in DES promotes lignin extension. The calculated free energy decreases from 663 kJ mol−1 in DES2-1 to 243 kJ mol−1 in DES1-2 following the molecular shift from “globular” relative to the corresponding reactant states (Fig. 5d). This decrease in free energy suggests that lignin samples much more extended conformations in DES1-2. Thus, the higher concentration of OA facilitates the formation of lignin structures with longer end-to-end distances.
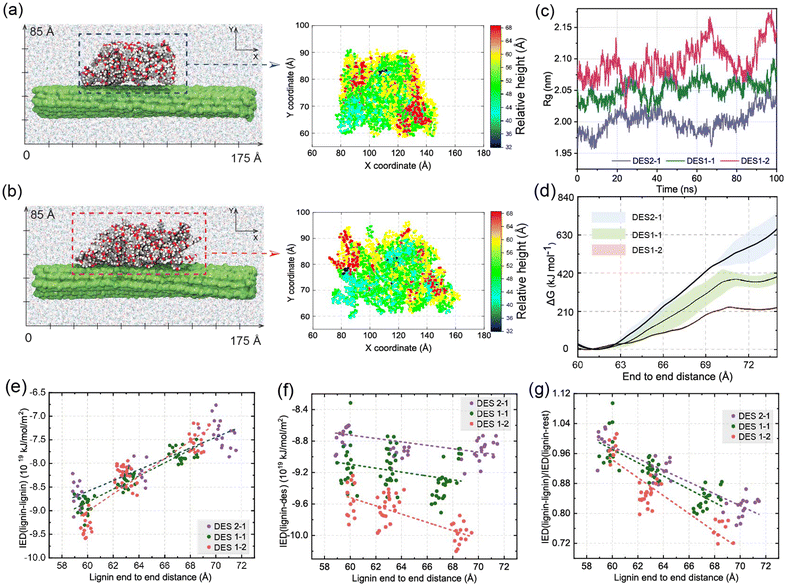 |
| Fig. 5 Lignin–cellulose systems in (a) DES2-1 and (b) DES1-2 from MD simulations. (c) Radius of gyration of lignin in DESs; (d) free energy profiles for three DES systems. Interaction energy density of (e) lignin–lignin; (f) lignin–DES; (g) normalized ratio of interaction energy density for intra-lignin interactions and of lignin with the rest of the system. | |
3.3.2. Interaction energy densities.
To investigate the non-covalent interactions between the DES solvent and lignin, interaction energy densities (IED) between lignin–lignin and lignin–DES were calculated. The interaction energy E between two components is defined as the difference in potential energy of the structure in which the components interact and when they are separated by an infinite distance (in this case lignin and the DES), In a molecular mechanics representation, the interaction energy consists of Lennard-Jones (i.e., van der Waals) and coulombic (i.e., electrostatic) terms. The interaction energy E was divided by the surface area, S, of lignin to obtain the interaction energy density. Fig. 5e and f demonstrate a linear relationship between the end-to-end distances and the IEDs of lignin–lignin and lignin–DES. The IED of lignin–lignin displays a positive correlation with the end-to-end distance of lignin. As the OA ratio increases, the slope of the lignin–lignin interaction energy density increases. This result suggests the higher OA ratio in the DES promotes weaker contacts within the lignin structure and leads to is extension. This finding also explains the inverse relationship between the end-to-end distance of lignin and the lignin–DES interaction energy density. The decreased lignin–lignin interaction can be attributed to the higher lignin–DES interaction energy as the lignin extends. Furthermore, the interaction energy density of lignin–DES increases more rapidly with the OA ratio in the DES (i.e., the interaction between lignin and the DES becomes stronger). Fig. 5g integrates the IEDs to exhibit the interactions within lignin and lignin with the rest of the system. The dramatically falling slope of DES1-2 indicates weak interaction within lignin and strong interaction between lignin and the rest of the system.
3.4. Fluorescence microscopy
An increase in relative lignin content is accompanied by a decrease in hemicellulose content after DES treatment compared to the initial wheat straw (Fig. 4d). The efficient removal of hemicelluloses in acidic DES leads to a higher proportion of lignin being exposed in the lignin–carbohydrate matrix. This concept is further supported by comparing fluorescence microscopy images of untreated (Fig. 6a) and DES-treated (ChCl/OA = 1
:
2) wheat straw samples (Fig. 6b). The concept of the “lignin glass shift” suggests that temperature plays a crucial role in facilitating the expansion of lignin. When the temperature surpasses the glass transition temperature of lignin (60–90 °C), the lignin molecules dissolve and migrate.63 The proportion of lignin in the untreated wheat straw increased from 6.6% to 11.1% after DES1-2 treatment.
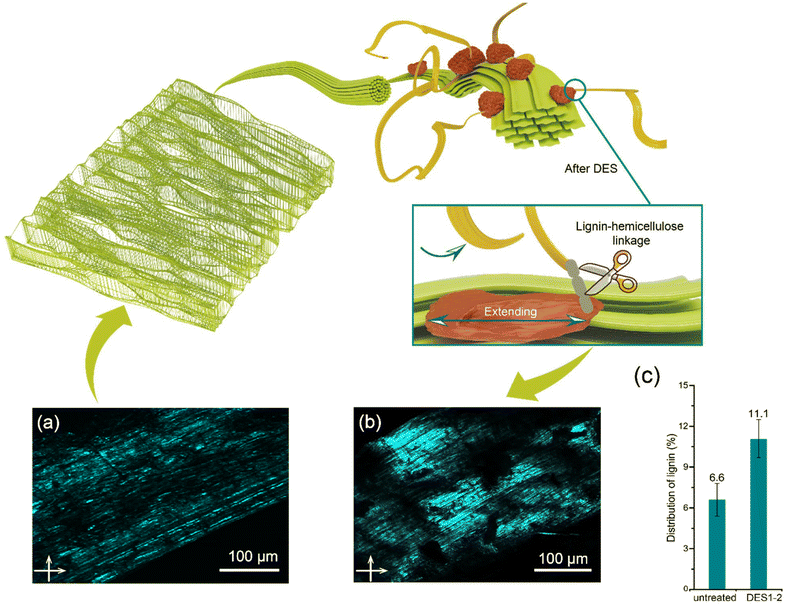 |
| Fig. 6 Fluorescence microscopy image of (a) untreated and (b) DES1-2-treated wheat straw material (lignin in cyan); (c) distribution of lignin fluorescence percentage of untreated and DES1-2-treated wheat straw. | |
3.5. Material densification
Lignin is a recognized binding macromolecule that helps improving the mechanical strength of densified products. This characteristic is relevant to the development of solid fuels but also, more universally, in designing adhesives, structural materials, and other applications that benefit from densification. The biobased adhesives and structural materials are areas that have resurfaced in the literature of advanced materials. In this study, we restrict our experimental inquiries to the classical process of pelletization, used here as a reference. Indeed, high-quality agricultural pellets can potentially be converted to valuable solid fuels using DES treatment after removing hemicellulose and changing lignin configuration. Lignin is commonly accepted as the binding agent for making pellets, and higher lignin content can enhance pellet durability.8 After DES treatment, hemicellulose removal helps increase the relative lignin content, which enhances the mechanical strength of the pellets. As the heating value of hemicellulose is 16–18 MJ kg−1 while the heating value of lignin is 23–27 MJ kg−1, the decreased hemicellulose content and increased relative lignin content therefore contribute to the increase in the calorific value.64 The characteristics of pellets produced from related materials are thus evaluated to determine how DES-treated wheat straw affects pellet quality.
We previously determined that the optimal moisture content range for pelleting cellulosic materials is 8–12%.65 Also, at optimal moistures of 10–15% in corn stover, the glass transition temperature decreased and resulted in enhanced binding at lower temperatures.66 Based on these findings, we used a 10% wet basis moisture content in this study. Fig. 7b displays the durability and change in pellet density over a two-week period. When compared to untreated pellets, the density of DES2-1 pellets rose from 1.32 g cm−3 to 1.34 g cm−3. In comparison, the density of the DES1-1 and DES1-2 particles rose sharply to 1.37 g cm−3 and 1.40 g cm−3, respectively. We note that after two weeks of storage the pellet densities dropped as a result of volumetric expansion, also known as the “spring back effect”67 (for untreated pellets this changed corresponded to a shift from 1.32 to 1.29 g cm−1 while the pellets produced from DES-treated samples underwent limited changes, from 1.33 to 1.32 g cm−3 and from 1.40 to 1.39 g cm−3 for DES2-1 and DES1-2 pellets, respectively). Moreover, Fig. S8† shows that the Mayer hardness doubled from 1.9 N mm−2 to 3.8 N mm−2 and was accompanied by an increase in the energy density by 20% from 21.3 GJ m−3 for untreated pellets to 25.6 GJ m−3 for DES1-2 pellets.
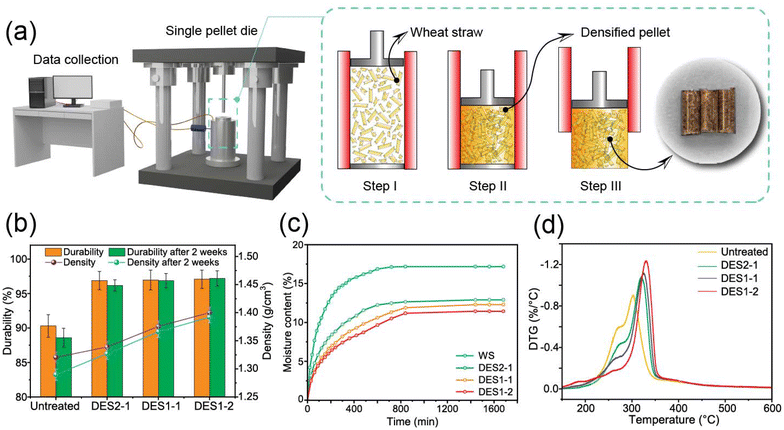 |
| Fig. 7 (a) Pelletization procedure; (b) pellet durability, pellet density, and their changes after two weeks; (c) water uptake in the humidity chamber; (d) DTG curves of untreated, DES1-1, and DES1-2 samples. | |
The DES treatment led to smaller particle sizes, which encourages densification and promotes stronger binding during high-pressure pelletization. Simultaneously, the relative increase in lignin content in DES-treated samples and the extension of lignin are contributing effects that increase the calorific value.
For the shipping and handling economy of the pellets, high pellet density and durability are essential. The durability of pellets created from DES-treated samples improve from 90.2% to 96.9–97.1% after two weeks for untreated pellets (Fig. 7b). Although there is no significant difference between the three pellets made from DES-treated samples (p > 0.05), the improvement in the durability of the DES-treated pellet (up 7.3% to 7.5% compared to untreated pellets) is significant (p < 0.05).
Fig. 7c shows the water absorption of both untreated and all DES-treated pellets. After DES treatment, the equilibrium moisture content (EMC) decreased. The untreated pellets show the most hygroscopic characteristics, for which the equilibrium moisture content reached 17% after 800 min. In contrast, the EMCs of DES2-1, DES1-1, and DES1-2 pellets dropped to 13%, 12%, and 11%. After 11 hours, the moisture content of the treated particles did not reach equilibrium. The water sorption rate slowed down as the amount of OA in DES increases. The hydrophobicity of the DES-treated pellets was further quantified by measuring the water contact angle for each sample. We found that the initial contact angle of DES1-2 pellets decreased from 106° to 91°, which indicates a modest wettability reduction due to containing more hydrophobic lignin (Fig. S9†). These findings imply that DES-treated sample pellets are less hydrophilic than corresponding untreated pellets and require more time to reach equilibrium moisture. The chemical composition has an impact on the water adsorption capacity of biomass. Among the major polymeric components of biomass, hemicelluloses are the most hygroscopic.68 Therefore, the lower hydrophilicity of pellets produced from samples that have undergone DES treatment results from the reduced hemicellulose content. Pellets with improved mechanical characteristics, energy density, and hydrophobicity are more competitive and reliable during storage and transportation.
The derivative thermogravimetric (DTG) curves for all pellets are shown in Fig. 7d. For untreated pellets, there is an obvious shoulder at around 270 °C, representing the decomposition of hemicelluloses. The intensity of the hemicellulose peak decreased as the OA ratio increased in the DES, with more hemicellulose decomposed. The second largest peak, in the range of 280–360 °C, was assigned to cellulose. The intensity of this peak for the DES pellets increased as the OA ratio increased, due mainly to the decomposition of hemicelluloses, leading to an increase in the fractional cellulose content. The cellulose peak shifted to a higher temperature range after DES treatment. The crystallinity index (CrI) increased from 45.8% of raw wheat straw pellets to 59.2% of DES1-2 pellets because crystalline cellulose is more thermally stable. The CrI and pyrolysis parameters are listed in Table S4.†
Finally, in an effort to examine the role of densification on solid fuel value, we report on the ignition index Di, which is used to quantify volatile matter release. Higher Di values correspond to easier release of volatile matter. The decreased ignition temperature Ti indicates that the DES pellets decompose at lower temperatures: the Di value increased from 3.3 to 6.0–7.6 after DES treatment. The Di value increased the most for DES1-2 pellets at 7.6, more than twice that of the pellets with untreated WS. The increased thermal reactivity indicates that the DES pellets decompose at lower temperatures than untreated pellets. As mentioned above, the modification of the structure of the treated fibers and the porous surface with enhanced SSA promote mass and heat transfer and therefore enhance pyrolysis.
4. Conclusions
DES, as a green solvent, shows promise in fractionation of biomass components, densification and in high-quality solid fuel production. The present work leads to a multiscale picture of the effect of a promising DES on biomass pretreatment (Fig. 8). By combining computational and experimental approaches, we offer insights into the phenomena involved in the decomposition of lignin–carbohydrate complexes under the effects of DES pretreatment, impacting its promise for biomass conversion, densification, and other activities.
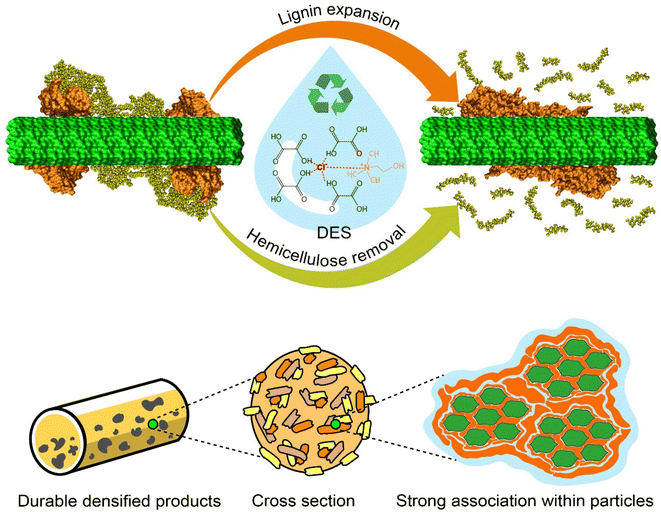 |
| Fig. 8 Schematic illustration of the multiscale effects of DES pretreatment in densification. | |
Our simulations suggest that the ether bonds between lignin and hemicellulose are cleaved through an acidolysis reaction with concurrent covalent modification of xylose by oxalic acid. Lignin then extends on the cellulose surface in a manner similar to that seen upon organosolv pretreatment,69,70 and thus acts a binding agent during densification.71 The strong association within particles due to the adhesion of lignin leads to durable densified materials with enhanced mechanical properties. In this study we have comprehensively investigated the chemical reactions that occur during DES pretreatment using quantum chemistry calculations. In addition, we have shown the modifications to lignin through classical molecular dynamics simulations and revealed improvements in the mechanical properties of DES-treated wheat straw pellets via experimental characterization. Based on these findings, DES-assisted pretreatment appears to be an effective method for biomass fractionation and valorization. More generally, the present work shows how processes important to chemical engineering for the circular bioeconomy can be rationalized by multiscale studies down to the molecular level.
Author contributions
Y. Y. designed the study, performed experiments, performed MD and DFT simulations and the analysis, analyzed the data, and wrote the manuscript. Z. M. W. performed MD and DFT simulations and analyzed the results. J. M. P. analyzed the simulation data and helped write the manuscript. S. S. provided experimental support and analyzed the data. O. J. R. and J. C. S. analyzed the data, edited the manuscript, and supervised the research.
Data availability
The data supporting this article have been included as part of the ESI.†
Conflicts of interest
The authors declare no competing interests.
Acknowledgements
Support for this scientific research was provided through the Biomass Canada Cluster (BMC) funded by Agriculture and Agri-Food Canada's AgriScience program and industry partners. Natural Sciences and Engineering Research Council of Canada supported the core research. Orlando J. Rojas and Zhangmin Wan are grateful for funding support from the Canada Excellence Research Chair Program (CERC-2018-00006) and the Canada Foundation for Innovation (project number 38623). This research was also supported the through computational resources and services provided by Advanced Research Computing at the University of British Columbia and Compute Canada. This research used resources of the National Energy Research Scientific Computing Center (NERSC), a U.S. Department of Energy Office of Science User Facility located at Lawrence Berkeley National Laboratory, operated under Contract No. DE-AC02-05CH11231 using NERSC awards ALCC-ERCAP-m4196 and BER-ERCAP-m906. This work was supported and provided by the U. S. Department of Energy (DOE), Office of Science, Office of Biological and Environmental Research, through the Genomic Science Program (contract no. FWP ERKP752). This manuscript has been authored by UT-Battelle, LLC under Contract No. DE-AC05-00OR22725 with the US Department of Energy (DOE). The United States Government retains and the publisher, by accepting the article for publication, acknowledges that the United States Government retains a non-exclusive, paid-up, irrevocable, world-wide license to publish or reproduce the published form of this manuscript, or allow others to do so, for United States Government purposes. The Department of Energy will provide public access to these results of federally sponsored research in accordance with the DOE Public Access Plan (https://energy.gov/downloads/doe-public-access-plan).
References
- H. Dong, L. Zheng, P. Yu, Q. Jiang, Y. Wu, C. Huang and B. Yin, ACS Sustainable Chem. Eng., 2019, 8, 256–266 CrossRef
.
- C. Huang, W. Lin, C. Lai, X. Li, Y. Jin and Q. Yong, Bioresour. Technol., 2019, 285, 121355 CrossRef CAS PubMed
.
- Y. H. Ci, F. Yu, C. X. Zhou, H. E. Mo, Z. Y. Li, Y. Q. Ma and L. H. Zang, Green Chem., 2020, 22, 8713–8720 RSC
.
- N. Giummarella, Y. Pu, A. J. Ragauskas and M. Lawoko, Green Chem., 2019, 21, 1573–1595 RSC
.
- A. K. Mohanty, S. Vivekanandhan, J. M. Pin and M. Misra, Science, 2018, 362, 536–542 CrossRef CAS PubMed
.
- Z. H. Liu, N. Hao, S. Shinde, Y. Pu, X. Kang, A. J. Ragauskas and J. S. Yuan, Green Chem., 2019, 21, 245–260 RSC
.
- Y. Yu, J. Wu, X. Ren, A. Lau, H. Rezaei, M. Takada, X. Bi and S. Sokhansanj, Renewable Sustainable Energy Rev., 2022, 154, 111871 CrossRef CAS
.
- Y. Yu, A. Lau and S. Sokhansanj, Renewable Energy, 2022, 190, 1018–1028 CrossRef CAS
.
- S. Xie, S. Sun, F. Lin, M. Li, Y. Pu, Y. Cheng, B. Xu, Z. Liu, L. da Costa Sousa, B. E. Dale, A. J. Ragauskas, S. Y. Dai and J. S. Yuan, Adv. Sci., 2019, 6, 1801980 CrossRef PubMed
.
- Z. H. Liu, N. Hao, Y. Y. Wang, C. Dou, F. Lin, R. Shen, R. Bura, D. B. Hodge, B. E. Dale, A. J. Ragauskas, B. Yang and J. S. Yuan, Nat. Commun., 2021, 12, 1–13 CrossRef
.
- N. A. Nguyen, S. H. Barnes, C. C. Bowland, K. M. Meek, K. C. Littrell, J. K. Keum and A. K. Naskar, Sci. Adv., 2018, 4, eaat4967 CrossRef CAS PubMed
.
- Y. Cao, M. He, S. Dutta, G. Luo, S. Zhang and D. C. W. Tsang, Renewable Sustainable Energy Rev., 2021, 152, 111722 CrossRef CAS
.
- A. S. Patri, B. Mostofian, Y. Pu, N. Ciaffone, M. Soliman, M. D. Smith, R. Kumar, X. Cheng, C. E. Wyman, L. Tetard, A. J. Ragauskas, J. C. Smith, L. Petridis and C. M. Cai, J. Am. Chem. Soc., 2019, 141, 12545–12557 CrossRef CAS PubMed
.
- Y. Liu, N. Deak, Z. Wang, H. Yu, L. Hameleers, E. Jurak, P. J. Deuss and K. Barta, Nat. Commun., 2021, 12, 1–15 CrossRef PubMed
.
- Y. Liu, N. Deak, Z. Wang, H. Yu, L. Hameleers, E. Jurak, P. J. Deuss and K. Barta, Nat. Commun., 2021, 12, 1–15 CrossRef PubMed
.
- Y. Yu, A. Lau and S. Sokhansanj, Ind. Crops Prod., 2021, 169, 113654 CrossRef CAS
.
- Y. Yu, Y. Guo, G. Wang, Y. A. El-Kassaby and S. Sokhansanj, Fuel, 2022, 324, 124341 CrossRef CAS
.
- X. J. Shen, J. L. Wen, Q. Q. Mei, X. Chen, D. Sun, T. Q. Yuan and R. C. Sun, Green Chem., 2019, 21, 275–283 RSC
.
- Z. Guo, Q. Zhang, T. You, X. Zhang, F. Xu and Y. Wu, Green Chem., 2019, 21, 3099–3108 RSC
.
- Y. Liu, W. Chen, Q. Xia, B. Guo, Q. Wang, S. Liu, Y. Liu, J. Li and H. Yu, ChemSusChem, 2017, 10, 1692–1700 CrossRef CAS PubMed
.
- Z. Chen, W. A. Jacoby and C. Wan, Bioresour. Technol., 2019, 279, 281–286 CrossRef CAS PubMed
.
- H. Xu, Y. Kong, J. Peng, W. Wang and B. Li, ACS Sustainable Chem. Eng., 2021, 9, 7101–7111 CrossRef CAS
.
- P. D. Muley, J. K. Mobley, X. Tong, B. Novak, J. Stevens, D. Moldovan, J. Shi and D. Boldor, Energy Convers. Manage., 2019, 196, 1080–1088 CrossRef CAS
.
- B. Zhu, Y. Xu, H. Ge, S. Wang, W. Wang, B. Li and H. Xu, Int. J. Biol. Macromol., 2023, 125342 CrossRef CAS PubMed
.
- T. Guo, Y. Yu, Z. Wan, S. Zargar, J. Wu, R. Bi, S. Sokhansanj, Q. Tu and O. J. Rojas, Renewable Energy, 2022, 194, 902–911 CrossRef CAS
.
- Q. Yu, A. Zhang, W. Wang, L. Chen, R. Bai, X. Zhuang, Q. Wang, Z. Wang and Z. Yuan, Bioresour. Technol., 2018, 247, 705–710 CrossRef CAS PubMed
.
- C. Alvarez-Vasco, R. Ma, M. Quintero, M. Guo, S. Geleynse, K. K. Ramasamy, M. Wolcott and X. Zhang, Green Chem., 2016, 18, 5133–5141 RSC
.
- X. D. Hou, G. J. Feng, M. Ye, C. M. Huang and Y. Zhang, Bioresour. Technol., 2017, 238, 139–146 CrossRef CAS PubMed
.
- B. Hess, C. Kutzner, D. Van Der Spoel and E. Lindahl, J. Chem. Theory Comput., 2008, 4, 435–447 CrossRef CAS PubMed
.
- O. Guvench, S. S. Mallajosyula, E. P. Raman, E. Hatcher, K. Vanommeslaeghe, T. J. Foster, F. W. Jamison and A. D. Mackerell, J. Chem. Theory Comput., 2011, 7, 3162–3180 CrossRef CAS PubMed
.
- P. Mark and L. Nilsson, J. Phys. Chem. A, 2001, 105, 9954–9960 CrossRef CAS
.
- R. Liu, H. Yu and Y. Huang, Cellular, 2005, 12, 25–34 CrossRef CAS
.
- L. Martinez, R. Andrade, E. G. Birgin and J. M. Martínez, J. Comput. Chem., 2009, 30, 2157–2164 CrossRef CAS PubMed
.
- G. Bussi, D. Donadio and M. Parrinello, J. Chem. Phys., 2007, 126, 14101 CrossRef PubMed
.
- H. J. C. Berendsen, J. P. M. Postma, W. F. Van Gunsteren, A. Dinola and J. R. Haak, J. Chem. Phys., 1984, 81, 3684–3690 CrossRef CAS
.
- M. Parrinello and A. Rahman, J. Appl. Phys., 1981, 52, 7182–7190 CrossRef CAS
.
- T. Darden, D. York and L. Pedersen, J. Chem. Phys., 1993, 98, 10096 CrossRef
.
- B. Hess, C. Kutzner, D. Van Der Spoel and E. Lindahl, J. Chem. Theory Comput., 2008, 4, 435–447 CrossRef CAS PubMed
.
- J. V. Vermaas, L. D. Dellon, L. J. Broadbelt, G. T. Beckham and M. F. Crowley, ACS Sustainable Chem. Eng., 2019, 7, 3443–3453 CrossRef CAS
.
- S. Jo, T. Kim, V. G. Iyer and W. Im, J. Comput. Chem., 2008, 29, 1859–1865 CrossRef CAS PubMed
.
- W. Humphrey, A. Dalke and K. Schulten, J. Mol. Graphics, 1996, 14, 33–38 CrossRef CAS PubMed
.
- M. F. Guest, E. Apra, D. E. Bernholdt, H. A. Früchtl, R. J. Harrison, R. A. Kendall, R. A. Kutteh, X. Long, J. B. Nicholas and J. A. Nichols, Futur. Gener. Comput. Syst., 1996, 12, 273–289 CrossRef
.
- M. D. Hanwell, D. E. Curtis, D. C. Lonie, T. Vandermeersch, E. Zurek and G. R. Hutchison, J. Cheminf., 2012, 4, 1–17 Search PubMed
.
- M. Ernzerhof and G. E. Scuseria, J. Chem. Phys., 1999, 110, 5029–5036 CrossRef CAS
.
- S. Grimme, J. Antony and S. Ehrlich, J. Chem. Phys., 2010, 132, 154104 CrossRef PubMed
.
- J. Vandevondele and J. Hutter, J. Chem. Phys., 2007, 127, 114105 CrossRef PubMed
.
- H. Fu, X. Shao, W. Cai and C. Chipot, Acc. Chem. Res., 2019, 3254–3264 CrossRef CAS PubMed
.
- A. Laio and M. Parrinello, Proc. Natl. Acad. Sci. U. S. A., 2002, 99, 12562–12566 CrossRef CAS PubMed
.
- G. Bussi and A. Laio, Nat. Rev. Phys., 2020, 2, 200–212 CrossRef
.
- R. G. Parr and W. Yang, Annu. Rev. Phys. Chem., 1984, 106, 4050–4051 Search PubMed
.
- B. Wang, C. Rong, P. K. Chattaraj and S. Liu, Theor. Chem. Acc., 2019, 138, 124 Search PubMed
.
- F. Neese, F. Wennmohs, U. Becker and C. Riplinger, J. Chem. Phys., 2020, 152, 224108 CrossRef CAS PubMed
.
- A. D. Becke, J. Chem. Phys., 1993, 98, 1372–1377 CrossRef CAS
.
- F. Weigend and R. Ahlrichs, Phys. Chem. Chem. Phys., 2005, 7, 3297–3305 RSC
.
- T. Lu and F. Chen, J. Comput. Chem., 2012, 33, 580–592 CrossRef CAS PubMed
.
- T. Lu and Q. Chen, Acta Phys.-Chim. Sin., 2018, 34, 503–513 CAS
.
- C. Morell, A. Grand and A. Toro-Labbé, J. Phys. Chem. A, 2005, 109, 205–212 CrossRef CAS PubMed
.
- Y. Liu, B. Guo, Q. Xia, J. Meng, W. Chen, S. Liu, Q. Wang, Y. Liu, J. Li and H. Yu, ACS Sustainable Chem. Eng., 2017, 5, 7623–7631 CrossRef CAS
.
- D. Yongzhuang Liu, W. Chen, Q. Xia, B. Guo, Q. Wang, S. Liu, Y. Liu, J. Li and H. Yu, ChemSusChem, 2017, 10, 1692 CrossRef PubMed
.
- O. Bobleter, Prog. Polym. Sci., 1994, 19, 797–841 CrossRef CAS
.
- D. P. Delmer and Y. Amor, Plant Cell, 1995, 7, 987 CAS
.
- H. Fu, H. Chen, X. Wang, H. Chai, X. Shao, W. Cai and C. Chipot, J. Chem. Inf. Model., 2020, 60, 5366–5374 CrossRef CAS PubMed
.
- N. Kaliyan and R. V. Morey, Bioresour. Technol., 2010, 101, 1082–1090 CrossRef CAS PubMed
.
- H. C. Ong, K. L. Yu, W.-H. Chen, M. K. Pillejera, X. Bi, K.-Q. Tran, A. Pétrissans and M. Petrissans, Renewable Sustainable Energy Rev., 2021, 152, 111698 CrossRef CAS
.
-
S. Sokhansanj, S. Mani, X. Bi, P. Zaini and L. Tabil, in 2005 ASAE Annual Meeting, American Society of Agricultural and Biological Engineers, 2005, p. 1 Search PubMed
.
- J. S. Tumuluru, C. T. Wright, J. R. Hess and K. L. Kenney, Biofuels, Bioprod. Biorefin., 2011, 5, 683–707 CrossRef CAS
.
- S. Ruksathamcharoen, T. Chuenyam, P. Stratong-on, H. Hosoda, L. Ding and K. Yoshikawa, Appl. Energy, 2019, 251, 113385 CrossRef
.
- H. Björk and A. Rasmuson, Fuel, 1995, 74, 1887–1890 CrossRef
.
- P. Langan, L. Petridis, H. M. O'Neill, S. V. Pingali, M. Foston, Y. Nishiyama, R. Schulz, B. Lindner, B. Leif Hanson, S. Harton, W. T. Heller, V. Urban, B. R. Evans, S. Gnanakaran, A. J. Ragauskas, J. C. Smith and B. H. Davison, Green Chem., 2014, 16, 63–68 RSC
.
- M. D. Smith, B. Mostofian, X. Cheng, L. Petridis, C. M. Cai, C. E. Wyman and J. C. Smith, Green Chem., 2016, 18, 1268–1277 RSC
.
- L. Petridis and J. C. Smith, Nat. Rev. Chem., 2018, 2, 382–389 CrossRef CAS
.
|
This journal is © The Royal Society of Chemistry 2024 |
Click here to see how this site uses Cookies. View our privacy policy here.