DOI:
10.1039/D4RA05972G
(Review Article)
RSC Adv., 2024,
14, 37580-37597
Comprehensive analysis of electrochemical biosensors for early ovarian cancer detection
Received
17th August 2024
, Accepted 14th November 2024
First published on 25th November 2024
Abstract
Ovarian cancer is one of the leading causes of mortality among women worldwide. However, early detection can significantly reduce mortality rates and mitigate subsequent complications related to both economic burden and mental well-being. Despite the development in the field of medical diagnosis, the death rates due to ovarian cancer have sharply increased. Among the recent technologies suggested as suitable diagnostic techniques for the early detection of ovarian cancer, biosensor technology has emerged as a cutting-edge technology, with electrochemical biosensors providing one of the most efficient types of biosensors. Therefore, this review discusses the application of electrochemical biosensors as a viable alternative to conventional diagnostic techniques for the timely identification of ovarian cancer, its advantages over other types of biosensors and conventional diagnostic techniques, and the types of electrochemical biosensors.
1. Introduction
Cancer is characterized by the uncontrolled proliferation of cells surpassing their normal limits, causing their expansion, invasion, and spreading into nearby tissues. It is the leading cause of mortality globally, accounting for approximately 10 million deaths and 19.3 million new cases in 2020. One of the common types of cancer in women is ovarian cancer, which is a gynecological cancer. In the case of ovarian cancer, specifically, the stage of the tumor at the diagnosis time is a crucial factor in determining the patients' survival, where women detected at Stage I have a three-fold greater survival rate compared to those found at Stages III–IV. Regrettably, most women with ovarian cancer get their diagnosis at Stage III or Stage IV, indicating that the cancer has spread to other areas of the body.1 According to the most recent data, the current mortality rate for ovarian cancer remains at a high level of 65.9%, while early-stage ovarian cancer patients have a 5 years survival rate that can reach up to 90%. The effective diagnosis rate for such cases is only 34%,2 which highlights the urgent need for improving the current diagnostic techniques.
The current detection methods include non-invasive methods such as X-ray computed tomography (CT), positron emission tomography (PET), ultrasonography (US), magnetic resonance spectroscopy (MRS), magnetic resonance imaging (MRI), and molecular diagnostic techniques such as the enzyme-linked immunosorbent assay (ELISA) test. While these non-invasive diagnostic methods offer benefits such as accessibility and minimal invasiveness in clinical settings, they have some drawbacks, including having challenges in accurately diagnosing certain types of tumors, especially tumors located deep within the body, being unaccessible, having high cost, low prediction ability, low detection limit and sensitivity.3,4
After that, either minimally invasive biopsy procedures like needle aspirations or more invasive surgical biopsies are done. These are then linked with histopathological evaluation to find out what kind of cancer it is and what stage it is in. Enhancing the rate of cancer detection facilitates prompt commencement of therapy, resulting in a decrease in the prevalence of late-stage cancer and improved long-term results. Therefore, early detection techniques are necessary to minimize the risk. However, the early detection of ovarian cancer presents several challenges that can be summarized in Fig. 1, including:
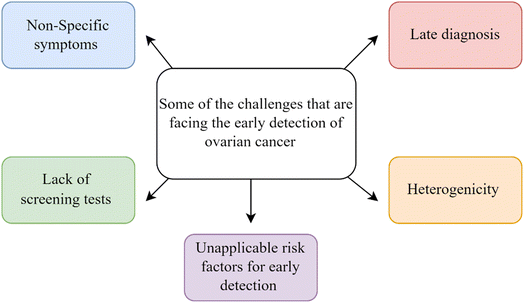 |
| Fig. 1 Challenges facing early detection of ovarian cancer. | |
(1) Non-specific symptoms: ovarian cancer often presents with vague symptoms such as bloating, abdominal or pelvic pain, fullness,5 and urinary urgency,6 which are easily overlooked or mistaken for other conditions.
(2) Lack of screening tests: unlike some other cancers, there are no highly effective screening tests for ovarian cancer available for routine use in the general population.7 Furthermore, it is currently not advised to conduct screening in the general population due to the lack of accurate and precise biomarkers for detecting early-stage disease.8
(3) Late presentation: OC is frequently detected at an advanced stage because it lacks identifiable symptoms and good screening tests. By the time it is detected, the disease has typically progressed beyond the ovaries, making treatment more challenging.9
(4) Heterogeneity: OC is a heterogeneous disease with different subgroups, each having unique molecular features and clinical behaviors. This heterogeneity complicates the development of effective screening strategies.10
(5) Risk factors: while certain risk factors, such as family history and BRCA gene mutations, are associated with an increased risk of OC, they are not universally applicable for early detection efforts.11
In order to tackle these issues, it is necessary to employ innovative methods such as creating biomarkers that are more sensitive and specific, using new imaging techniques, and utilizing advanced screening technologies. Therefore, this review will highlight the new findings in the ovarian cancer detection by elechemical biosensors as a new alternative for the conventional techniques, discuss the different kinds of biosensors, the advantages and challenges of utilizing the elechemical biosensors, and how this technology is possible to be a solution for many drawbacks of the current detection techniques of ovarian cancer, which may save the lives of many patients and improve their quality of life.
2. Biosensors
The biosensor is defined as an analytical device that is self-contained and integrated by the International Union of Pure and Applied Chemistry (IUPAC). The system of the biosensor is composed of a biological recognition element, such as DNA, aptamers, enzymes, peptides, antibodies, antigens, or living cells, which are directly contacted with a transduction element. The transduction element can have an electrochemical, optical, or mechanical nature. Biosensors have been utilized in many fields, including industrial, clinical, environmental, and agricultural analyses, since the amperometric glucose enzyme electrode introduction by Leland Clark Jr. in 1962.12 Originally, scientists created biosensors to test biomolecular targets, aiming to expand clinical analysis beyond specialized laboratories to public environments like non-hospital nursing settings, hospitals, or homes.13
2.1 Types of biosensors
Biosensors are divided into electrochemical, physical, or optical categories based on their detecting method and transducer system (Fig. 2).
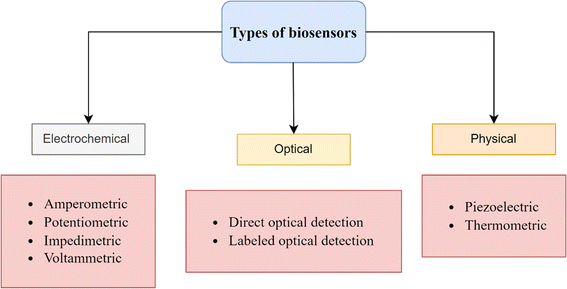 |
| Fig. 2 Types of biosensors based on transducer operation. | |
This review will focus specifically on electrochemical biosensors due to their numerous advantages over optical and physical biosensors. These advantages include simple instrumentation, high sensitivity, and the potential for miniaturization. Furthermore, electrochemical biosensors are among the most prevalent and accessible options on the market, as they are generally more portable, user-friendly, and cost-effective compared to their counterparts.14
2.2 Electrochemical biosensors
According to recent updates in the literature, electrochemical biosensors have been proven to be a potential solution for early detection of diseases, including breast cancer15 Alzheimer's disease,16 acute and chronic leukemias,17 and infectious diseases, such as COVID-19 (ref. 18) epresents a promising advancement in medical diagnostics. For instance, the glucose biosensor is a type of electrochemical biosensor that has played a significant role in advancing the field of biosensors in medical diagnosis.19
2.2.1 Definition and principles of electrochemical biosensors. Electrochemical biosensors leverage the principles of electrochemistry to detect and quantify biological molecules or biomarkers that are indicative of various health conditions. They typically consist of a biorecognition element, like enzymes, antibodies, or DNA probes, adhered to a transducer surface. When the specific biomarker attaches to the biorecognition element, it causes a detectable alteration in the electrical signal. This change is accurately linked to the concentration of the biomarker in the sample, as demonstrated in Fig. 3.
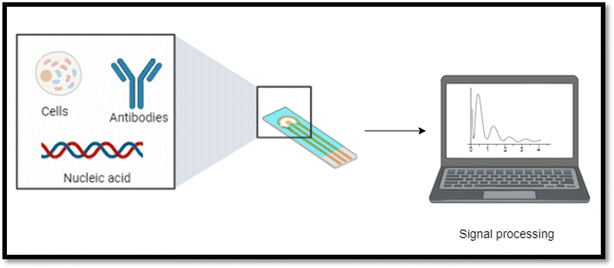 |
| Fig. 3 The diagram illustrates the structure and components of an electrochemical biosensor. Biological sensing elements connect to electrodes. These devices convert the signal in order to produce a legible output. | |
2.2.2 Electrochemical techniques as a sensing mechanism. Electroanalytical devices such as electrochemical biosensors are considered a sub-discipline of analytical chemistry that includes both oxidation–reduction reactions and charge transfers.20 Biorecognition elements play a crucial role in analyte detection. In order to generate a signal based on the concentration of the analyte, it is necessary to employ this component in conjunction with a converter.21 Within an electrochemical transducer system, measurable signals such as current, conductivity, impedance, and potential are acquired due to the interaction between samples and a bioreceptor. Electrochemical biosensors are categorized into many classes based on the signals they generate, including conductometric, amperometric, impedimetric, and potentiometric22–24
(a) Potentiometric. Potentiometric biosensors measure the potential difference between the reference and the working electrodes without the presence of an electric current. They use ion-selective electrodes (ISEs) that respond to ion concentration changes, which can indicate the presence of specific biomarkers. These biosensors are commonly used for detecting charged species such as ions, pH, and gases. However, they can also be adapted for biomarker detection by incorporating selective membranes or recognition elements.25
(b) Amperometric. Amperometric biosensors measure the electrical current generated by a redox reaction at the working electrode under a constant applied voltage. Typically, they consist of a functional electrode modified with a recognition component, such as an enzyme, antibody, or DNA that is specific to the target biomarker. When the target biomarker binds with the recognition element, it initiates a biological response that produces an electrical signal directly proportional to the biomarker's concentration. Amperometric biosensors offer high sensitivity and are widely used for the detection of biomolecules such as glucose, neurotransmitters, and proteins, including those relevant to ovarian cancer.25
(c) Impedimetric. Impedimetric biosensors measure the impedance (i.e., resistance to alternating current) of the system, typically at frequencies ranging from a few Hz to several megahertz. They detect changes in impedance caused by the binding of target biomolecules to the surface of the electrode or changes in the electrical properties of the surrounding medium. Impedimetric biosensors eliminate the need for labels and offer benefits such as exceptional specificity, continuous monitoring, and suitability for downsizing in point-of-care applications. These biosensors can detect a wide range of biomarkers, including nucleic acids, proteins, and tiny molecules that are related to ovarian cancer.25
(d) Conductimetric. Conductimetric biosensors measure the change in conductivity (inverse of resistance) in the solution or medium surrounding the electrodes. The function of these biosensors is to detect changes in conductivity that arise from the interaction between the targeted biomarker and the electrode's surface-fixed recognition element. These biosensors are sensitive to changes in ion concentration, viscosity, and dielectric properties, making them suitable for detecting biomolecules with different physicochemical properties. Conductimetric biosensors have been employed for the detection of various analytes, including proteins, DNA, and ions relevant to ovarian cancer biomarkers.26
(e) Capacitive. Capacitive biosensors measure changes in capacitance, which occur when the dielectric properties of the medium between the electrodes change upon biomarker binding. They typically consist of interdigitated electrode structures with a high surface area, allowing for enhanced sensitivity and detection limits. Capacitive biosensors have advantages such as the ability to detect without the need for labels, the ability to monitor in real-time, and compatibility with making devices smaller for portability and wearability. These biosensors have been utilized to identify a diverse array of biomolecules, such as proteins, nucleic acids, and tiny molecules linked to the advancement of ovarian cancer.27
2.2.3 Advantages of electrochemical biosensors over traditional detection methods. As mentioned previously, the current diagnostic techniques for ovarian cancer have some drawbacks, such as challenges in accuracy, being unaccessible, high cost and low sensitivity. Enzyme-linked immunosorbent assay (ELISA) is an example of the conventional diagnosis technique for ovarian cancer. It is an immunological assay widely utilized in diagnostics, basic science research and clinical application studies. It depends on the interaction between the primary antibody that is specific to the antigen of interest (i.e., the target protein), where the antigen presence is confirmed through the enzyme-linked antibody catalysis of the added substrate. The products of this process can be qualitatively detected by visual inspection or quantitatively detected using readouts from either a spectrophotometer or a luminometer.28 It is one of the most straightforward and specific assays that are used for detecting biomolecules.29 It has been demonstrated to be a practical method for the early diagnosis of ovarian cancer. However, in comparison to electrochemical biosensors, ELISA has many drawbacks, including that it is commonly performed in laboratory settings and is not suitable for on-site clinical consultations, whereas electrochemical biosensors can be designed for point-of-care testing.30 Additionaly, ELISA results require several hours to obtain, while electrochemical biosensors can provide results in minutes. Furthermore, electrochemical biosensors can enhance specificity by employing multi-target measurements, allowing for the simultaneous detection of multiple biomarkers.In addition, from an economic perspective, althoughthe production of electrochemical biosensors may involve high initial costs primarily during the research and design phase, large-scale production is generally affordable. Moreover, electrochemical biosensors achieve limits of detection in the picogram and fimtogram range, which are often unattainable with ELISA and other conventional diagnostic methods. In addition, ELISA has several limitations, including being a tedious and labo-intensive assay procedure, insufficient sensitivity in bio-recognition of challenging biomolecular entities such as microRNAs, and the requirement for relatively high sample volumes. Furthermore, the limit of detection of ELISA is less than nanomolar concentration level, which is inadequate to reach the clinical threshold of many protein biomarkers, particularly in the early stages of diseases.31 Other challenges include the high cost associated with antibody preparation, a significant possibility of false positives and negatives, antibody instability, and the need for refrigerated transport and storage due to the protein nature of antibodies.32 Table 1 includes a comparison between elecrochemical biosensors and the ELISA technique:
Table 1 A comparison between elecrochemical biosensors and the ELISA technique
Point of comparison |
ELISA |
Electrochemical biosensors |
Princible |
Measures the binding of antibodies to antigens with enzyme-linked detection |
Measures electrical changes resulting from analyte interaction |
Detection time |
Takes hours |
Provides real-time results within minutes |
Sensitivity |
Highly sensitive, with limits of detection in the nanogram range |
Extremely sensitive, achieving limits of detection in the femtogram and picogram range |
Accessability |
Requires complex laboratory equipment and cannot be used for on-site clinical consultations |
More portable and suitable for point-of-care testing |
Stability |
Less stable as it mainly depends on antibodies that are protein in nature |
Generally more stable |
Sample volume |
Requires larger sample volume |
Required lower sample volume |
Multiplexing capability |
Can be designed for multiplexing but often requires more steps |
Can detect multiple targets simultaneously |
Cost |
High cost of reagents, antibodies design and equibment |
Lower cost of production |
Interference |
Can be affected by sample matrix and interference and higher chance of false positive or negative results |
Less affected by turbidity and color |
Therefore, in comparison to traditional detection methods, electrochemical biosensors provide quick identification, easy transportability, cost efficiency, portability, accessibility, and exceptional sensitivity due to the use of nanoparticles with very small sizes to enhance their sensitivity and specificity, where they can sense and react with the molecule of interest at very small concentrations, making them appropriate for on-site testing in areas with limited resources and enabling early detection of the disease, facilitating timely intervention, and improving patient outcomes. Fig. 4 illustrates some of these advantages.
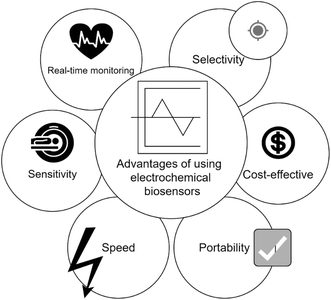 |
| Fig. 4 Advantages of using electrochemical biosensors. | |
3. Biomarkers for ovarian cancer detection
3.1 Biomarkers principle
Molecular cancer biomarkers encompass measurable molecular indicators that provide information about cancer risk, occurrence, or outcome in patients. Tumor biopsies or less invasive samples such as blood, saliva, buccal swabs, stool, or urine are commonly used to detect biomolecular changes in proteins, DNA, RNA, and other molecules. These changes provide valuable information for cancer diagnosis at an early stage, prognosis, precision medicine, predicting drug responses, guiding cancer treatment, and monitoring cancer. Advancements in detection methods, including next-generation sequencing, nanotechnology, and the analysis of circulating tumor DNA, RNA, or exosomes, have greatly enhanced our capacity to detect these biomarkers. These biomolecules, generated from either cancer cells or normal cells in response to malignancy, can be found in tissues or body fluids.33
Certain inherited or germline variants increase an individual's susceptibility to cancer. For example, the BRCA1 and BRCA2 variations exhibit a robust association with breast and ovarian cancer. Considering the high heterogeneity of tumors is crucial, necessitating a multi-gene approach when developing reliable cancer biomarkers. Moreover, personalized treatment approaches based on individual tumor profiling are increasingly being employed to account for the specific mutation profiles of cancer patients.33 Some ovarian cancer biomakers are depicted in Fig. 5.
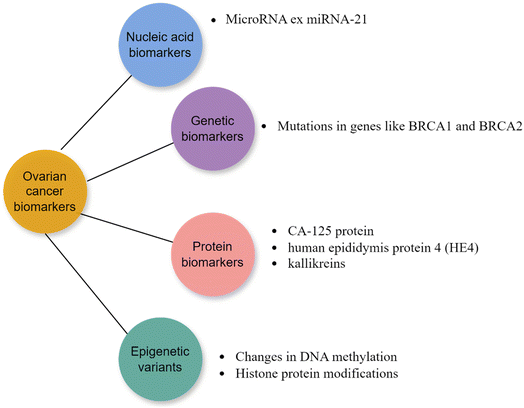 |
| Fig. 5 Some ovarian cancer biomakers. | |
3.2 Ovarian cancer biomarkers
3.2.1 Ovarian cancer biomarkers include.
(a) Nucleic acid biomarkers.
(i) miRNAs.
MiRNAs are short RNA molecules that are naturally present in eukaryotes. They do not code for proteins and are approximately 20 to 25 nucleotides long. They have a role in regulating several biological processes, such as regulating gene expression by either degrading or blocking the translation of target mRNAs that have been discovered to be disrupted in different types of cancer.33 They can be isolated from cells, tissues, and different body fluids, such as urine, blood, etc.34 A single miRNA has the ability to target and regulate numerous mRNAs by influencing the expression of various genes through their interaction with the targeted mRNAs. Research has demonstrated that miRNAs play a crucial role in the development of numerous diseases.35 Furthermore, miRNAs have significant functions in multiple cancer-related biological processes, including tumor development, cell growth, specialization, programmed cell death, angiogenesis, cancer cell infiltration and spread, resistance to treatment, cell type transformation, and disease outcome prediction. During the development of malignancies, miRNAs can function as either oncogenic factors or tumor suppressors.36,37 Some microRNA biomarkers are mentioned in Table 2.
Table 2 Some of the microRNA biomarkers associated with ovarian cancer
Elevated microrna |
Decreased microrna |
Reference |
miRNA-21 |
— |
38 and 39 |
miR-26b, miR-26a, miR-182, miR-103 |
Let-7d, miR-127, miR-34b, miR-15a, and miR-34a |
36 and 40 |
miR-214 |
— |
41 and 42 |
miR-200 |
— |
40 and 43 |
miR-141 |
— |
44 and 45 |
miR-155 |
miR-214, miR-199a, let-7b, miR-31 |
37 and 46 |
hsa-miR-106a-5p, hsa-miR-93-5p, hsa-let-7d-5p |
hsa-miR-122-5p, hsa-miR-99b-5p, and hsa-miR-185-5p |
47 |
miR-23a, miR-27a, miR-30d, miR-125b, miR-126, miR-141, miR-182, miR-200c-3p, miR-603, miR-1307, miR-200b, miR-205, miR-221, miR-31, miR-375, miR-429, miR-509-3p, miR-9, let-7a, let-7d, let-7c, and let-7f, miR-203, miR-155, miR-200a, miR-25, miR-200c, miR-506, miR-130b, miR-133a, miR-137, miR-193b, miR-21, miR-22, miR-29b, miR-335 and let-7b, miR-106a, miR-182, miR-25, miR-200a, miR-200c-3p, miR-205, miR-221, miR-30d, and miR-603 |
miR-22, miR23b, miR-27a, miR-106a, miR-139, miR-149, miR-199a-3p, miR-200b, miR363, miR-409-3p, miR-494, miR-145, miR-148a, miR-182, miR-141, miR-23b, miR-29b, miR-30a, miR-335, and miR-497, miR-149, miR-20a, miR-21 miR-23a, miR-25, miR-203, miR-221, miR-30d, and miR-363 |
48 |
(b) Genes and proteins biomarkers. Cancer typically develops as a result of the accumulation of somatic mutations, which can either be unique to a specific type of cancer or common across other types. Protein biomarkers were among the initial substances employed in cancer diagnoses. The majority of tests rely on cancer enzymes, antigens, and hormones, as well as alterations in the protein glycosylation profile that is a distinctive attribute of cancer. Glycans are monosaccharides chains that can bind to proteins to create glycoproteins. Differently expressed glycans or glycoproteins, serve as valuable cancer biomarkers in both tumor tissue and blood. The modifications in protein glycosylation might arise from the modified manifestation of glycoproteins or variations in the glycans or glycotransferases. Some glycans and glycoproteins, like AFP, β-hCG, CEACAMS HER2, sTn antigen, CA19-9, CA27.29, CA125, CA549, CEA, onfFN, PLAP, TG, TAG-72, PSA, and Tn antigen, can be used to detect cancer.49 Table 3 shows some protein biomarkers for ovarian cancer.
Table 3 Some proteomic biomarkers for ovarian cancer
Gene/protein name |
References |
Tumor protein 53 (TP53) protein |
50 |
CA-125 protein |
39 and 50 |
Human epididymis protein 4 (HE4) |
39 |
Mesothelin protein |
Kallikreins |
Aldehyde dehydrogenase 1 (ALDH1) |
α1-antitrypsin (AAT) |
51 |
Nuclear factor-κB (NFKB) |
Phosphomevalonate kinase (PMVK) |
Vascular adhesion protein 1 (VAP1) |
Fatty acid-binding protein 4 (FABP4) |
Platelet factor 4 (PF4) |
Apolipoprotein A1 (APOA1) |
51 |
α1-Acid glycoprotein (AGP) |
51 |
(c) Epigenetic variants. Epigenetic variations, such as changes and modifications in histone protein or DNA methylation, do not modify the DNA coding sequence but can have a substantial effect on the structure and stability of DNA. Epigenetic alterations significantly contribute to the progression of cancer and serve as crucial biomarkers for diagnosis and prognosis. DNA methylation biomarkers can also be utilized to accurately predict the response to cancer treatment. Increased DNA methylation of promoter regions is an early event during development of cancer. This methylation can be detected in ctDNA, accentuating the promise of methylated ctDNA as a biomarker for OC diagnosis.52 Global DNA methylation loss is a prevalent occurrence in various cancers and is associated with DNA damage, genomic instability and transposons and retroviruses reactivation. In addition, certain alterations in DNA methylation at CpG-rich gene promoter regions can result in the deactivation of tumor suppressor genes. An example of this is the CpG island methylator phenotype, which is defined by the excessive methylation of numerous sites.52 One instance is when the MGMT promoter, which is a gene responsible for DNA repair, undergoes hypermethylation. This hypermethylation is linked to a positive reaction to alkylating medications. As a result, the evaluation of MGMT promoter hypermethylation is utilized in clinical testing for glioblastomas.53 The food and drug administration (FDA) has approved the use of certain DNA methylation-based biomarkers for colorectal cancer screening. These biomarkers include SEPT9 from plasma (Epi ProColon) and a combination of NDRG4 and BMP3 from stool samples.33
3.3 Challenges in identifying reliable biomarkers
As previously mentioned, due to the complexity and heterogeneity of the ovarian cancer and its various subtypes, each subtype has its own distinct molecular profile. This complexity makes it difficult to find biomarkers that can accurately detect all types of ovarian cancer. In addition, as most of the cases are diagnosed in late stages, identifying biomarkers for early detection becomes more challenging.54 Furthermore, any biomarker should provide the characteristic of being specific only for the ovarian cancer disease, which is rare. Many biomarkers are elevated in many medical cases. For example, CA-125 is one of the main biomarkers that are associated with ovarian cancer; however, its levels are elevated in many non-ovarian malignancies, including lung, colorectal, cervical and endometrialcancers. In addition, it increases in states of inflammation. Recent studies have demonstrated that COVID-19 patients had a temporary rise in CA-125 levels and other cancer biomarkers.55,56 Which highlights the need for more valid and specific biomarkers for ovarian cancer to minimize the risk of misdiagnosis and unnecessary interventions.57 In addition, other factors, such as age, hormonal status, the dynamic changes in biomarker levels over time and stages of the disease and comorbidities, can influence biomarker levels, potentially leading to false interpretations of their diagnostic value. Controlling of these factors and evaluating the stability and predictive value of biomarkers across different disease stages and treatment responses is crucial in assessing the true utility of biomarkers.58
4. Nanomaterials used in electrochemical biosensor manufacturing
To meet the increasing need for biosensors in different scientific and technological fields, researchers have been motivated to explore new nanoscale materials for use in sensor technologies to get the best results. In the last 20 to 30 years, nanotechnology has made substantial strides in its development and real-world implementation.59 Usually, these materials have a small size between 1 and 100 nanometers in at least one dimension. Nanomaterials have become essential elements in diverse scientific disciplines, with applications spanning from biotechnology to energy storage due to their unique physical and chemical features, including high surface-to-volume ratio, high reactivity, variations in electrical conductivity and optical qualities as compared to larger materials.60 In addition, all these qualities can be customized for individual applications by altering the dimensions, form, surface area, chemical makeup, and porosity, making them highly advantageous in many applications, including biomedical applications. For example, the importance of nanomaterials in biology has greatly escalated in relation to the advancement of biochips for purposes such as stem cell treatment and drug administration.61 Nanomaterials provide numerous benefits for biological applications. Their nanoscale size enables them to easily pass through cellular membranes. Additionally, several nanomaterials demonstrate biocompatibility with cells, rendering them suitable as templates for delivering drugs and inducing differentiation directly into cells and tissues. Furthermore, the utilization of magnetic nanoparticles (MNPs) that are coated with biocompatible metal NPs allows for accurate manipulation in order to deliver molecules to specific areas.
Many nanomaterials were planned and utilized to improve the overall effectiveness of biosensors especially after the recent breakthroughs in nanoengineering. Biosensors typically comprise three fundamental components: a target recognition element that interacts with the biological analyte, a transducer that turns this contact into a quantifiable signal, and a signal processing element that receives and interprets the signal. Various nanostructure designs with dimensions ranging from zero to three dimensions have been utilized to improve biosensor design to primarily enhance sensitivity (i.e., accurately detecting the target analyte), specificity (i.e., distinguishing the target analyte from other sample components), decreasing response time and reducing the limit of detection (LOD). The limit of detection of a biosensor is the lowest quantity of material that can be reliably detected, even when the analyte is not present in the sample.62 Therefore, diagnostics is one of the fields that is significantly impacted by nanostructures specifically after the wide application in the creation of biosensors for in vitro diagnostics.63 In vitro diagnostics refers to the performance of analytical tests on samples derived from the human body, including tissue, blood and saliva for detecting diseases and monitoring an individual's health condition.
Therefore, nanomaterials have expanded the possibilities for developing sensitive detection systems in the field of biosensors. The combination of biomolecules and nanomaterials can effectively use the distinct benefits of both. Novel nanomaterials have the ability to increase the strength of the signal in biosensors that rely on surface-enhanced Raman scattering (SERS). On the other hand, the weak signal of electron transfer that is often observed with enzymes can be amplified by including metal nanoparticles, leading to the development of highly responsive electrochemical biosensors based on enzymes.64 Below are many frequently utilized nanomaterials in electrochemical biosensors for the detection of diverse biomarkers, including those associated with ovarian cancer.
4.1 Metal-based nanoparticles
Metal oxide nanoparticles have distinctive physicochemical characteristics at the nanoscale, rendering them exceptionally favorable for augmenting the biosensors' sensitivity and reactivity. Nanowires and nanorods, due to their 1D structure, enhance the effectiveness of charge transfer and signal transduction. Furthermore, these nanomaterials possess remarkable electrical, mechanical, and thermal characteristics that can be utilized to enhance the performance of biosensors. Quantum dots, renowned for their adjustable optical characteristics, provide accurate signal amplification and multiplexing, hence enhancing the complexity of biosensor designs. Incorporating nanocomposite dendrimers offers a flexible method for modifying biosensor surfaces, improving stability and boosting binding interactions. This ultimately contributes to the overall biosensor performance's strength and reliability.
Metal oxides such as copper oxide (CuO), iron oxide (Fe2O3), manganese oxide (MnO2), nickel oxide (NiO), cobalt oxide (Co3O4), zinc oxide (ZnO), tin oxide (SnO2), titanium oxide (TiO2), and cadmium oxide (CdO) have been widely used in different industries over the past two decades due to their diverse electrical, chemical, and physical characteristics. Out of these metal oxides, manganese oxides, zinc, copper and iron are widely acknowledged as exceptional magnetic nanomaterials due to their great electron mobility. Consequently, they have been utilized in the electrochemical biosensors development.65
In recent decades, there has been significant interest in noble metal nanoparticles because of their distinctive plasmonic capabilities, which can be utilized to manipulate the optical signal of molecules located nearby. Plasmonic nanoparticles can improve the fluorescence intensity of a fluorophore and the Raman signal of a Raman reporter. The two phenomena that are amplified by plasmons are referred to as metal-enhanced fluorescence (MEF) and surface-enhanced Raman scattering, respectively. Fluorescence and Raman spectroscopy are very effective analytical techniques that have been extensively utilized to create sensitive biosensors for a wide range of applications. These applications include environmental sensing, food safety, and biomedical purposes such as early disease detection and prognosis.66 Due to the dependence of these biosensors on their optical signals, the use of MEF and SERS techniques to improve fluorescence and Raman signal intensity provides a distinct benefit in achieving extremely low detection limits, even at the level of a single molecule.
4.1.1 Silver. Among the noble metals, gold and silver have gained significant attention due to their tunable plasmonic properties, which make them highly suitable for downstream applications. Silver (Ag) possesses several advantageous characteristics compared to gold. It exhibits higher thermal and electrical conductivity and stability, catalytic activity, and more efficient electron transfer, resulting in sharper extinction bands and improved detection limits for target molecules. Additionally, modified Ag nanoparticles have demonstrated enhanced stability in water and air. As a result, Ag has gained appeal in diverse domains such as diagnosis, medicinal delivery, environmental research, electronics, and as an antibacterial agent. Ag nanoparticles have gained significant use in biosensors and bioimaging. Multiple studies have shown that Ag nanoparticles have the capability to improve the precise identification of clinical indicators.67Previous studies have established the stability of Ag nanoparticles, and their ability to remain stable has been confirmed through stability analyses.68 When attaching Ag nanoparticles to sensing surfaces, electrostatic attraction can be utilized, although modifying the surface to ensure a strong chemical attachment is generally recommended. Depending on the desired molecular attachment, Ag nanoparticles are suitable for various surface chemical modifications. For instance, the silane reaction can chemically functionalize Ag nanoparticles using (3-aminopropyl) triethoxysilane, taking advantage of the oxide groups formed on the Ag nanoparticle when exposed to atmospheric moisture.
Ag nanoparticles have gained recognition for their antibacterial capabilities. However, current research has emphasized their importance and influence in the fields of biosensors and bioimaging applications. Ag nanoparticles exhibit a lower refractive index in comparison to the majority of molecules. Attaching proteins to Ag nanoparticles causes an increase in the local refractive index, which leads to a noticeable change in the extinction of Ag that can be seen. These variations in optical characteristics have been extensively harnessed by various sensors to efficiently detect target molecules. Moreover, the enhancement of biomolecular detection has been achieved by adding a protective covering, such as silica, to Ag nanoparticles.69
4.1.2 Magnetic nanomaterials. Superparamagnetic nanoparticles are commonly used in biosensor applications because they can be magnetized when exposed to a magnetic field. Lately, the use of these nanoparticles for biosensing has become increasingly common. The aggregation of magnetic nanoparticles has demonstrated significant efficacy in biosensing. The magnetic nanoparticles' high surface-to-volume ratio confers advantages for biosensing. Nanoscale magnetic materials possess magnetic properties that make them well-suited for use as biosensing labels. Furthermore, their composition, size, and morphology can be modified to align with the specific needs of the biosensing application. Magnetic nanoparticles have garnered significant interest for their distinctive physico-chemical properties, straightforward synthesis, magnetic resonance imaging contrast capabilities, simple surface modifications, excellent biodegradability and minimal toxicity. These qualities make them excellent candidates for use as delivery vehicles and imaging agents in cancer theranostics.70 Magnetic nanoparticles exhibit enhanced magnetization when subjected to an external magnetic field, making them effective agents for magnetic resonance imaging. Additionally, MNPs possess excellent T2/T2* relaxation capabilities. Therefore, MNPs are widely used in diverse applications of cancer theranostics, such as biosensors, MRI imaging, theranostics, delivery, photodynamic therapy, magnetic hyperthermia, and photothermal ablation therapy. In addition, magnetic particle imaging (MPI) has been garnering significant interest as an imaging technique utilizing magnetic nanoparticles. Several research groups intensively investigated the condition of the applied field, functionalization of magnetic nanoparticles, and particle shape for MPI.71
4.2 Carbon nanostructures
Carbon-based materials are widely researched and utilized in the field of nanotechnology because of their exceptional features. Carbonaceous structures possess a multitude of advantages compared to typically utilized materials, particularly due to their remarkable physical and chemical capabilities. In addition, carbon-based materials can be used as a cost-effective substitute for expensive electronic compounds. These materials exhibit exceptional performance and are also regarded as environmentally sustainable. As a result, there has been significant research conducted on carbon nanostructures due to their potential application in advanced sensor systems. These nanostructures possess exceptional physical and chemical characteristics that enhance their sensing capabilities.
Several carbon-based structures that were found many decades ago continue to be researched and utilized in modern technological gadgets. Nanodiamond (ND) structures, which originated in the 1960s, are carbon structures with nanoscale dimensions (5–100
nm) that are largely generated through sp3 hybridization. The distinctive electrical and optical properties of these materials arise from the presence of dopants inside their structure, while their exceptional surface reactivity can be attributed to structural flaws and unsaturated chemical bonds originating from carbon atoms.72–76 The identification of fullerene (FLN) was made in 1985 by Kroto et al. FLNs are a type of carbon allotrope that have a 3D closed-cage structure made up of five- and six-membered rings. The structure has 12 pentagons and a variable hexagons number, depending on the FLN size.77 A carbon nanotube (CNT) is a type of carbon-based structure that belongs to the fullerene family and has a quasi-one-dimensional structure. It can exist as a single-walled or multi-walled CNT. The publication of Iijima in 1991, which examined and showed the tubular structure of carbon nanotubes (CNTs), sparked a significant increase in interest. Graphene (GPN) is the initial two-dimensional atomic crystal to be discovered. It is composed of a single carbon atoms sheet that are organized in a honeycomb lattice pattern.78
4.2.1 Carbon nanotubes. Carbon nanotubes (CNTs) are highly investigated nanomaterials with a one-dimensional structure that have been intensively studied in many applications, such as biosensors, cell labeling and trackin, diagnostics, tissue engineering and drug delivery. CNTs are cylindrical tubes that can be classified as single-, double-, or multi-walled, depending on the number of concentric graphite layers they contain. These layers are capped with fullerene hemispheres. These structures possess distinctive attributes, such as exceptional mechanical and electrical properties, notable electrocatalytic activity, elevated thermal conductivity, chemical stability, minimum surface fouling, reduced over-voltage, and a high aspect ratio (surface-to-volume).79 The electronic conductance of these nanostructures is extremely sensitive to small surface changes, such as those generated by the binding of macromolecules, due to their high surface-to-volume ratio and unique electron transport capabilities.Carbon nanotube-based biosensors and diagnostics have been utilized for sensitive detection in various fields, including healthcare, food quality analysis, environmental monitoring and industries. They are commonly employed in electrochemical sensing, particularly for the monitoring of glucose, as well as for galactose, fructose, neurochemicals, neurotransmitters, albumin, amino acids, insulin, streptavidin, immunoglobulin, human chorionic gonadotropin (hCG), C-reactive protein (CRP), microorganisms, cancer biomarkers, DNA, cells, and other biomolecules detection. Multi-walled carbon nanotubes (MWCNTs) are widely used in all nanotube biosensing applications. These nanomaterials, which are one-dimensional in structure, provide accurate and sensitive bioelectronic detection without the need for labels. Additionally, they demonstrate significant redundancy in arrays of nanosensors.
4.2.2 Graphene. Graphene has made important contributions to several study areas as a result of its unique physical and chemical properties.14,20,80 The benefits of graphene-based sensors for sensing applications are as follows:(1) High specific surface area: single-layer graphene has a theoretical specific surface area of 2630 m2 g−1, which allows for the possible attachment of many recognition components or analyte molecules. This feature improves the ability to detect small amounts of something and makes it easier to make the device smaller.
(2) Graphene possesses exceptional electronic properties and exhibits efficient electron transport capabilities. This is due to the sp2 hybridization of carbon atoms, which results in the formation of a massive π–π conjugate system that allows electrons to flow unrestrictedly. Graphene's inherent features make it highly suitable for electrochemical sensing applications.
(3) Single-layer graphene, which is around 0.335 nm thick, possesses exceptional mechanical strength and pliability. This is attributed to the strong C
C bonding in the atomic plane, resulting in a hardness greater than that of diamond. Graphene is a soft substance due to its interlayer bonding by van der Waals forces, which is different from the strong bonding found in diamond. This characteristic is beneficial for the advancement of wearable sensor devices.
Graphene and its derivatives possess notable attributes such as a substantial specific surface area, rapid electron transport rate, and resilience to elevated temperatures. Consequently, they can function as signaling devices or carriers for biometric components, facilitating the precise measurement of biomolecules.81
4.2.3 Reduced graphene oxide. Graphene oxide (GO) is composed of hydrophobic carbon atoms bound in both sp2 and sp3 configurations, along with various oxygen-containing functional groups such as carbonyl, epoxy, hydroxyl, and carboxyl groups. These functional groups are present on the basal plane as well as at the margins of the GO nanosheet. GO shares similar characteristics to graphene, including distinctive electrical, electrochemical, mechanical and thermal properties. In addition, due to its hydrophilic nature, it can be transformed into transparent, flexible and biocompatible nanosheets. The presence of functional groups on GO nanosheets enables their interaction with a diverse array of biomolecules, which is extremely beneficial for the advancement of biosensor technology. Nanosheets of GO have been synthesized using the Staudenmaier, Brodie and Hummers processes, as well as their modified versions. Additionally, the conversion of GO into rGO can be achieved using several processes, such as high-temperature thermal annealing, chemical reduction, electrochemical reduction and ultra-violet irradiation. The reduced graphene oxide (rGO) also demonstrates exceptional characteristics, such as its ability to disperse in solvents, as well as its impressive electrical, optical, and mechanical capabilities. Additionally, it possesses thermal stability that is on par with graphene and graphene oxide (GO) nanosheets. Graphene quantum dots (GQDs) are a new type of zero-dimensional (0D) graphene derivatives that exhibit distinct features due to quantum confinement and edge effects. These properties make them suitable for use in biosensor applications.80,82The incorporation of graphene-based materials (GBMs) with various metal nanoparticles, organic polymers, and surface functions, together with numerous proteins and enzymes, is highly advantageous for the advancement of novel biosensors. GO and rGO have been widely employed in the development of nanocomposite-based biosensors due to their reactive surface defects and oxygen functional group. These features enable the regulated nucleation and growth of metal, metal oxides, and semiconductor nanoparticles. Graphene and its oxidized derivatives, including graphene oxide, which possess diverse oxygen functional groups (such as hydroxyl, carboxyl, and epoxy groups), have been identified as promising candidates for application in biosensors. GO sheets possess functional groups that confer strong hydrophilicity and enable the incorporation of diverse inorganic nanoparticles, including metal oxides, noble metals, semiconducting nanoparticles, nanoclusters (NCs) and quantum dots (QDs). This integration enhances the sensors performance utilizing GO sheets. In addition, the conversion of graphene oxide into reduced graphene oxide creates a significant number of defects, resulting in enhanced electrochemical activity as compared to graphene generated using chemical vapor deposition (CVD). This characteristic is particularly advantageous for the development of electrochemical biosensors. Graphene-based nanocomposites possess distinct morphological features and characteristics that are advantageous for sensing applications. The graphene nanocomposites possess 3D interconnected hierarchical structures that enhance the diffusion of various biomolecules and maintain their biocatalytic functionalities, hence optimizing the biosensing capability. Graphene-based hybrids incorporating polymers and surface-decorated metal nanoparticles have been investigated for biosensing applications because of their exceptional biocompatibility, large surface area, and ability to selectively attach biomolecules.
5. Electrochemical biosensors for detecting ovarian cancer biomarkers
5.1 Detection of CA125 in ovarian cancer
In the study of Samadi et al.,83 the authors documented the creation and advancement of a unique electrochemical immunosensor for identifying the CA125 oncomarker. The utilization of polyamidoamine/gold nanoparticles aimed to augment the conductivity and amplify the quantity of antibodies that were bound to the electrode surface. The glassy carbon electrode was enhanced by using 3D reduced graphene oxide-multiwall carbon nanotubes to increase the specific surface area and conductivity of the electrode. The tracers used were toluidine blue and antibody, which were linked to O-succinyl-chitosan-magnetic nanoparticles. A unique modification strategy was employed to enhance the solubility of chitosan by utilizing succinic anhydride. The immunosensor that was created showed an exceptional limit of detection of around 6 μU mL−1 and a broad linear range of 0.0005–75 U mL−1. The immunosensor reliability in detecting CA125 was confirmed using the standard addition recovery method, which was then compared to an enzyme-linked immunosorbent assay. The immunosensor that was suggested demonstrated exceptional stability, notable sensitivity, selectivity and commendable reproducibility. The illustration of the immunosensor preparation steps is mentioned in Fig. 6.
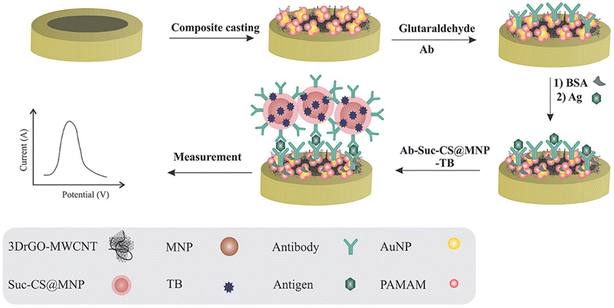 |
| Fig. 6 A schematic illustration of the immunosensor preparation steps. The modification of GCE with 3DrGO-MWCNT-PAMAM/AuNP, sandwich method formation by Ab2-Suc-CS@MNP-TB, and detection of CA125 using SWV. “This figure has been adapted from ref. 83 with permission from Elsevier, copyright 2020”. | |
In another study by Fatima et al.,84 the study introduces a novel biosensor, Cat@AMQDs-GCE, which is a cost-effective and selective device for hydrogen peroxide detection. This biosensor is based on the immobilization of catalase on an antimonene quantum dot-modified glassy carbon electrode. Antimonene quantum dots were manufactured for the electrochemical detection of hydrogen peroxide using a simplified process and analyzed using multiple analytical techniques. The catalase enzyme, which is specialized in reducing H2O2, is mounted onto AMQDs (amino-modified quantum dots) to enhance its detection using cyclic voltammetry and amperometry techniques. The linearity of Cat@AMQDs-GCE was established to be 0.989 in addition, the limit of detection was 4.4 μM. Amperometric experiments demonstrated a recovery rate ranging from 95 to 103.4% for hydrogen peroxide in human serum samples. The electrochemical stability of the Cat@AMQDs-GCE was observed for up to 30 cycles, resulting in a reduction in the cost of analysis. The Cat@AMQDs-GCE exhibited excellent selectivity in the presence of dopamine, ascorbic acid, leucine and glucose. The prepared electrode was also utilized to quantitatively determine the H2O2 concentration in serum samples from patients with ovarian cancer. In addition, Cotchim et al. created a straightforward electrochemical immunosensor for detecting ovarian cancer without the need for labels. This immunosensor utilized a hierarchical microporous carbon material made from waste coffee grounds. The analysis technique included near-field communication (NFC) and a potentiostat based on a smartphone. The coffee grinds were subjected to pyrolysis in the presence of potassium hydroxide and then employed to alter a screen-printed electrode. The screen-printed electrode was enhanced with the addition of gold nanoparticles in order to selectively capture a particular antibody. The characterization of the modification and immobilization processes was conducted using cyclic voltammetry and electrochemical impedance spectroscopy. The sensor exhibited a dynamic range of 0.5 to 50.0 U mL−1 for the cancer antigen 125 tumor marker, with a highly accurate correlation coefficient of 0.9995. The detection limit was 0.4 U mL−1.85 On the other hand, Mu et al.86 developed a very sensitive electrochemical immunosensor that does not require a label and is specifically designed for detecting CA125. This sensor utilizes nanocomposites made of nanogold-functionalized copper-cobalt oxide nanosheets (CuCo-ONSs@AuNPs). The immunosensor demonstrated a linear detection range of 1 × 10−7 U mL−1 to 1 × 10−3 U mL−1 and a detection limit of 3.9 × 10−8 U mL−1 under ideal conditions. The signal-to-noise ratio for the detection limit was 3. The suggested label-free electrochemical immunosensor provides a direct, reliable, and highly sensitive method for quantifying CA125.
Moreover, Rebelo et al.87 work focuses on creating molecular imprint polymers (MIPs) on a gold electrode surface to detect and identify the CA-125 biomarker. The CA-125 imprinting was prepared using the electropolymerization of pyrrole (Py) monomer on a gold electrode using cyclic voltammetry (CV). This method was employed to create materials that are extremely selective and possess excellent molecular recognition capabilities. The CA-125 biomarker was quantified by comparing two methods: electrochemical (square wave voltammetry – SWV) and optical transduction (surface plasmon resonance – SPR). SWV has gained significant popularity in the field of biological molecule analysis because of its rapidity and high sensitivity. Surface Plasmon Resonance (SPR) is an optical technology that allows for the analysis of interactions between the CA-125 biomarker and Molecularly Imprinted Polymers (MIP) without causing any damage. This technique gives precise and reliable analytical data. To evaluate the performance of the CA-125 biosensor, various analytical parameters, including sensitivity, linear response interval, and detection limit, were evaluated for both electrochemical and optical transduction methods. The biosensor utilizing electrochemical transduction demonstrated superior analytical properties, exhibiting excellent selectivity and a detection limit (LOD) of 0.01 U mL−1. Moreover, it offered a linear concentration range spanning from 0.01 to 500 U mL−1. The researchers used an electrochemical biosensor for the investigation, and it was effectively used to analyze CA-125 in artificial serum samples. The recovery rates ranged from 91 to 105%, with an average relative error of 5.8%.
In the study of Hu et al.,88 as illustrated in Fig. 7, sensitive detection of CA125 was achieved by constructing an electrochemical biosensor based on aptamers. The stable layered substrate, molybdenum disulfide (MoS2), was utilized in combination with the irregular branched structure of gold nanoflowers (AuNFs) to create a sensing interface with a very large specific surface area. This was achieved using a one-step process of electrodeposition, resulting in the formation of AuNFs@MoS2. The electrode modification phase was streamlined, resulting in better electrode stability, superior electrochemical performance, and the creation of many sulfhydryl binding sites. Subsequently, a sensor including AuNFs@MoS2/CA125 aptamer/MCH was developed specifically for the purpose of detecting CA125. The CA125 aptamer with sulfhydryl was immobilized onto the AuNFs@MoS2 electrode through gold sulfur bonds. The compound 6-mercapto-1-hexanol (MCH) was employed to obstruct the electrode and diminish the occurrence of non-specific adsorption. DPV analysis was used to detect CA125, with a detection range of 0.0001 U mL−1 to 500 U mL−1. The aptamer sensor that was designed exhibited satisfactory specificity, repeatability, and stability.
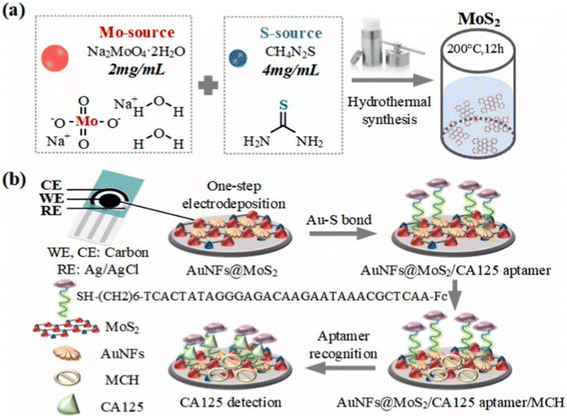 |
| Fig. 7 Schematic diagrams of the sensing platform. (a) Synthesis of MoS2. (b) Modification of AuNFs@MoS2/CA125 aptamer/MCH for CA125 detection. “This figure has been adapted from ref. 88 with permission from Elsevier, copyright 2023”. | |
In the work of Amirabadizadeh et al.,89 a sensitive detection of CA125 was achieved by constructing an electrochemical biosensor based on aptamers. The stable layered substrate, molybdenum disulfide (MoS2), was utilized in combination with the irregular branched structure of gold nanoflowers (AuNFs) to create a sensing interface with a very large specific surface area. This was achieved using a one-step process of electrodeposition, resulting in the formation of AuNFs@MoS2. The electrode modification phase was streamlined, resulting in better electrode stability, superior electrochemical performance, and the creation of many sulfhydryl binding sites. Subsequently, a sensor including AuNFs@MoS2/CA125 aptamer/MCH was developed specifically for the purpose of detecting CA125. The CA125 aptamer with sulfhydryl was immobilized onto the AuNFs@MoS2 electrode through gold sulfur bonds. The compound 6-mercapto-1-hexanol (MCH) was employed to obstruct the electrode and diminish the occurrence of non-specific adsorption. DPV analysis was used to detect CA125, with a detection range of 0.0001 U mL−1 to 500 U mL−1. The aptamer sensor that was designed exhibited satisfactory specificity, repeatability, and stability. In addition, Runprapan et al.90 developed label-free immunosensors to detect ovarian cancer by cancer antigen (CA125). Acids were used to treat four types of carbon nanomaterials, including multi-wall carbon nanotubes, graphite KS4, vapor-grown carbon fiber (VGCFs) and carbon black super P (SP), in order to create a carbon nanomaterial/gold (Au) nanocomposite. An AuNPs@carbon nanocomposite was produced on a glassy carbon electrode (GCE) using an electrochemical method. This composite was used as a substrate to create a label-free immunosensor for detecting CA125. Out of the four AuNPs@carbon composites, the sensor based on AuNPs@MWCNTs showed a remarkable sensitivity of 0.001 μg mL−1 for the biomarker CA125 using the square wave voltammetry (SWV) approach. The excellent conductivity and large surface area of multi-walled carbon nanotubes (MWCNTs) facilitated the immobilization of gold nanoparticles (AuNPs). Furthermore, the acid treatment resulted in an increased abundance of carboxylic (COO–) functional groups in MWCNT, making it an ideal substrate for the production of electrochemical biosensors. This study demonstrated a sustainable approach to synthesizing a layer-by-layer (LBL) assembly of AuNPs@carbon nanomaterials for an electrochemical immunoassay targeting CA125 in clinical diagnostics. The method is cost-effective and suitable for point-of-care diagnosis. In addition, as illustrated in Fig. 8, Krathumkhet et al.91 presented a new method for detecting a cancer antigen 125 (CA125) biomarker using an electrochemical immunosensor The researchers utilized a biomarker on conductive composite materials consisting of carbon ink, carbon dot, and zinc oxide (C-ink/CD/ZnO) as an electrode platform. They employed an ITO substrate to enhance the interaction between antibodies (Ab) and the catalytic performance of ZnO, which serves as a labeling signal molecule. Furthermore, the nanocomposite consisting of silver and polypyrrole (Ag@PPy) was employed as a promising redox mediator. The accuracy of the construction labeled with Ag@PPy was higher compared to the building without labeling. The immunosensor that was developed exhibited a broad linear range from 1 ag mL−1 to 100 ng mL−1, together with a low limit of detection of 0.1 fg mL−1 under the ideal conditions. This indicates that the immunosensor is regarded as a precise and effective diagnostic technique for CA125.
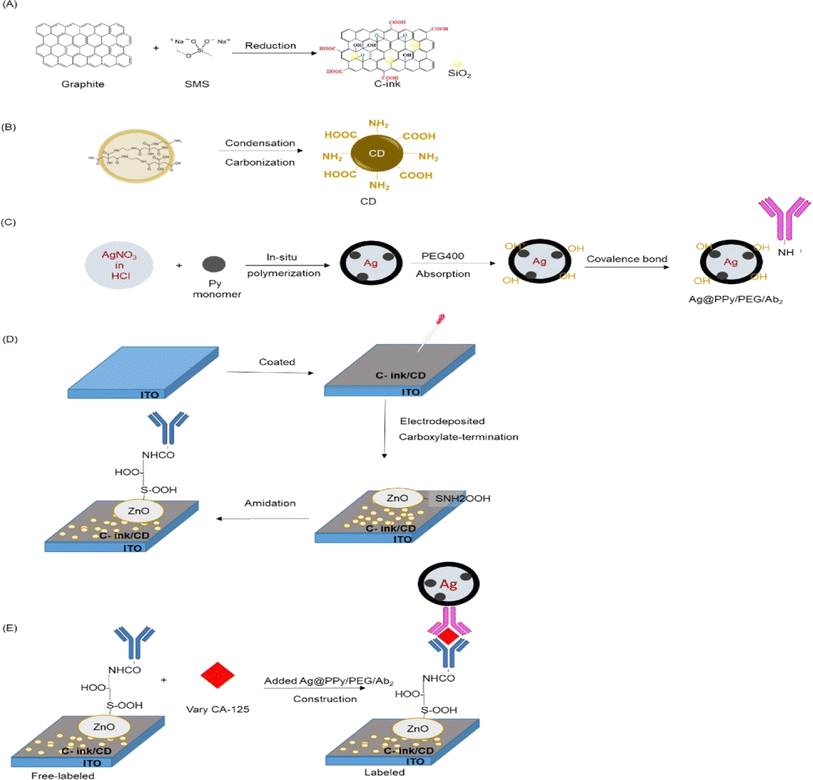 |
| Fig. 8 Preparation steps of materials for CA-125 immunosensor. (A) Synthesis of C-ink and (B) CD. (C) Combined of Ag@PPy/PEG and Ab2 with covalence bond. (D) Electrode of ITO/C-ink/CD/ZnO/Ab1 as free-labeled. (E) Construction of ITO/C-ink/CD/ZnO/CA-125 labeled with Ag@PPy/PEG/Ab2. “This figure has been adapted from ref. 91 with permission from Elsevier, copyright 2023”. | |
In the study of Wang et al.92 a new ultrasensitive electrochemical immunosensor was developed, which was composed of two antibodies, gold nanoparticles, reduced graphene oxide, thionine chloride and bovine serum albumin. The immunosensor had a detection limit of 4.10 pg mL−1 (127 nU mL−1), which shows that the sensor can be used for the early detection of ovarian cancer. Furthermore, it demonstrated high selectivity after comparing the results of a clinical test in healthy individuals and patients and the obtained high-regression-curve rate from human blood serum analysis can be used to differentiate ovarian cancer from other gynecological diseases, such as the inflammation of pelvic cavity, endometriosis and other gynecological diseases that may cause the elevation of CA125 levels.
5.2 Detection of squamous cell carcinoma antigen (SCC-Ag)
SCC-Ag is a highly effective cancer biomarker, and its increased levels have been utilized for ovarian cancer detection. In the study of Liang et al.,93 the amine-modified MWCNT dielectric sensing surface was utilized to immobilize the anti-SCC-Ag antibody for the purpose of detecting SCC-Ag. The homogeneity of the surface morphology was quantified using a 3D nanoprofiler, and the findings validated the identification of SCC-Ag at around 80 picomolar concentration. The presence of SCC-Ag was specifically validated using two control proteins, human serum albumin and factor IX. Additionally, the system showed no signs of biofouling. The utilization of MWCNTs as a dielectric sensing surface in this experimental configuration has the potential to enable the early identification of ovarian cancer.
5.3 Detection of HE4
In the study of Chen et al.,94 a novel biosensor that does not require labeling was created for the ultrasensitive immunoassay of HE4. The biosensor utilized K3[Fe(CN)6] as the electrochemical probe and PtNi nanocubes assemblies (NCAs) as highly effective biosensing surfaces. The PtNi NCAs were produced using a straightforward solvothermal method, in which two co-structuring directors were used, which are 2,2′-bis(4,5-dimethylimidazole) (BDMM) and N-hexadecyltrimethylammonium chloride (HTAC). The HE4 immunosensor achieved a broad detection range of 0.001 to 100 ng mL−1 and a low detection limit of 0.11 pg mL−1, with a signal-to-noise ratio of 3 under ideal conditions. On the other hand, in the study of Nawaz et al.,95 a novel electrochemical immunosensor was created to specifically detect the ovarian cancer biomarker HE4 using a composite of poly(allylamine hydrochloride) (PAH) and black phosphorus nanosheets (BPNS) over a glassy carbon electrode. PAH has been utilized to preserve the BPNS in its initial honeycomb configuration and to securely attach biomolecules by electrostatic forces on the transducer surface. The electrochemical immunosensor was able to detect HE4 within a linear range of 0.1–300 ng mL−1, with a detection limit of 0.01 ng mL−1. The sensor that was created demonstrated excellent selectivity and sensitivity towards HE4, with high specifity and minimal interference from common biomolecules such as lysozyme, bovine serum albumin, hemoglobin, fetal bovine serum, protamine, glucose and fructose. Furthermore, Bianchi et al.96 demonstrated an electrochemical device that is specifically engineered to have the ability to independently calibrate and analyze data, seamlessly transitioning between calibration and measurement modes. The analytical device was tested and proven effective in human serum. It showed excellent sensing capabilities for analyzing HE4, with detection and quantitation limits of 3.5 and 29.2 pM, respectively, in human serum. These results meet the diagnostic sensitivity requirements and indicate a high potential for using the device as a portable and intelligent diagnostic tool for point-of-care testing.
5.4 Detection of miR-200a biomarker
For the detection of the ovarian cancer biomarker miR-200a, Moazampour et al.97 developed an electrochemical biosensor that is mentioned in Fig. 9, a genosensor utilizing ZnS quantum dots functionalized with L-cysteine was developed, without the need for labeling. The Cys-ZnS-QD genosensor was analyzed using UV-vis absorption, transmission electron microscopy (TEM) and fluorescence techniques. Cysteine-zinc sulfide quantum dots are formed onto the surface of a glassy carbon electrode using electrodeposition. These quantum dots serve as an appropriate substrate for immobilizing the DNA probe. The linear range of miR-200a were determined to be from 1.0 × 10−14 to 1.0 × 10−6 M and the detection limit was 8.4 fM under ideal conditions. In Table 4, we summarize the previously reported examples of electrochemical biosensors for detecting ovarian cancer biomarkers.
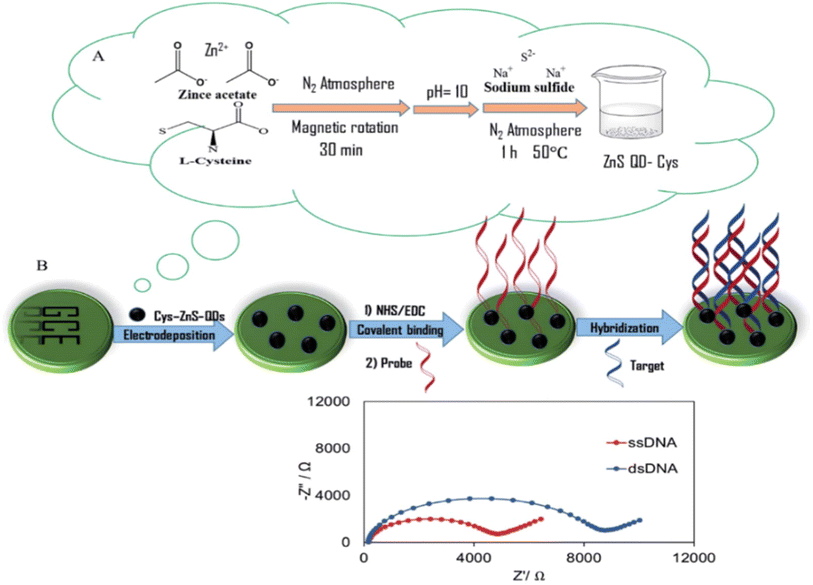 |
| Fig. 9 Schematic representation of (A) the synthesis of L-cysteine functionalized ZnS-QDs and (B) the label free electrochemical genosensor. “This figure has been adapted from ref. 97 with permission from Royal Society of Chemistry”. | |
Table 4 Summary of the previously mentioned examples of electrochemical biosensors for detecting ovarian cancer biomarkers
The detected ovarian cancer biomarker |
The used nanoparticles |
Linear range |
The detection limit |
References |
CA 125 |
Polyamidoamine/gold nanoparticles (PAMAM/AuNPs) used to enhance the number of antibodies (Abs) immobilized on the electrode surface and increase the conductivity. To improve the electrode specific surface area and conductivity, 3D reduced graphene oxide-multiwall carbon nanotubes (3DrGO-MWCNTs) used to modify the glassy carbon electrode |
0.0005–75 U mL−1 |
6 μU mL−1 |
83 |
Ab and toluidine blue attached to O-succinyl-chitosan-magnetic nanoparticles (Suc-CS@MNPs) as a tracer |
Gold nanostructures (GNs) |
10 to 800 U mL−1 |
2.6 U mL−1 |
89 |
Catalase immobilized antimonene quantum dots modified glassy carbon electrode (Cat@AMQDs-GCE) |
0.989 |
4.4 μM |
84 |
Gold nanoparticles (AuNPs) |
0.5 to 50.0 U mL−1 |
0.4 U mL−1 |
85 |
Molybdenum disulfide with the irregular branched structure of gold nanoflowers AuNFs@MoS2 |
0.0001 U mL−1 to 500 U mL−1 |
|
88 |
Carbon ink/carbon dot/zine oxide (C-ink/CD/ZnO) and silver@polypyrrole (Ag@PPy) |
1 ag mL−1 to 100 ng mL−1 |
0.1 fg mL−1 |
91 |
Nanogold-functionalized copper-cobalt oxide nanosheets (CuCo-ONSs@AuNPs) |
1 × 10−7 U mL−1 to 1 × 10−3 U mL−1 |
3.9 × 10−8 U mL−1 |
86 |
AuNPs@MWCNTs |
|
0.001 μg mL−1 |
90 |
Squamous cell carcinoma antigen (SCC-Ag) |
MWCNT |
|
80 pM |
93 |
HE4 |
PtNi nanocubes assemblies (PtNi NCAs) |
0.001–100 ng mL−1 |
0.11 pg mL-1 |
94 |
Black phosphorus nanosheets (BPNS)/poly(allylamine hydrochloride) (PAH) nanocomposite |
0.1–300 ng mL−1 |
0.01 ng mL−1 |
95 |
miR-200a |
Cys-ZnS-QDs |
1.0 × 10−14 to 1.0 × 10−6 M |
8.4 fM |
97 |
6. Conclusion and future remarks
Electrochemical biosensors are a cutting-edge technology that can be a future hope in increasing the chance and the numbers of patients that are early diagnosed with ovarian cancer, which will help in having bigger chances to save their lives and having better responses for medications. However, there are still numerous hurdles and challenges that remain to be resolved as future research directions. One difficulty in the field of cancer biomarkers is the need for improvement through the discovery of new biomarkers that have better specificity, sensitivity, and positive predictive value. Furthermore, the majority of the electrochemical biosensors addressed in the literature are considered prototypes that have only been assessed in controlled laboratory settings. In addition, a limited number of actual samples were used, which were insufficient to study the storage, optimal stability, or provide a good validation of these electrochemical biosensors. Another obstacle lies in the creation of electrochemical biosensors that rely on identifying several indicators of ovarian cancer in bodily fluids like saliva and sweat. These systems encounter difficulties, such as the limited correlation between the levels of biomarkers in blood and other fluids, as well as their much lower concentrations in certain fluids.
Abbreviations
NPs | Nanoparticles |
OC | Ovarian cancer |
CT | X-ray computed tomography |
US | Ultrasonography |
PET | Positron emission tomography |
MRS | Magnetic resonance spectroscopy |
MRI | Magnetic resonance imaging |
BRCA | Breast cancer gene |
ISEs | Ion-selective electrodes |
CA-125 | Cancer antigen 125 |
CRC | Colorectal cancer |
ctDNA | Circulating tumor RNA |
CEA | Carcinoembryonic antigen |
LOD | Limit of detection |
TP53 | Tumor protein 53 |
HE4 | Human epididymis protein 4 |
ALDH1 | Aldehyde dehydrogenase 1 |
AAT | α1-antitrypsin |
NFKB | Nuclear factor-κB |
PMVK | Phosphomevalonate kinase |
VAP1 | Vascular adhesion protein 1 |
FABP4 | Fatty acid-binding protein 4 |
PF4 | Platelet factor 4 |
APOA1 | Apolipoprotein A1 |
AGP | α1-Acid glycoprotein |
MEF | Metal-enhanced fluorescence |
SERS | Surface-enhanced Raman scattering |
MNPs | Magnetic nanoparticles |
ND | Nanodiamond |
FLN | Fullerene |
CNT | Carbon nanotube |
MWCNTs | Multi-walled carbon nanotubes |
GPN | Graphene |
hCG | Human chorionic gonadotropin |
CRP | C-reactive protein |
GO | Graphene oxide |
rGO | Reduced GO |
GBMs | Graphene-based materials |
QDs | Quantum dots |
NCs | Nanoclusters |
CVD | Chemical vapor deposition |
Data availability
No data were used for the research described in the article.
Author contributions
Marwa A. El-Gammal: writing – original draft, review & editing, investigation. Fatma E. Sayed: writing – original draft. Nageh K. Allam: conceptualization, writing – review & editing, funding acquisition, supervision.
Conflicts of interest
The authors declare that they have no known competing financial interests or personal relationships that could have appeared to influence the work reported in this paper.
References
- C. Vela-Vallespín, L. Medina-Perucha, C. Jacques-Aviñó, N. Codern-Bové, M. Harris, J. M. Borras and M. Marzo-Castillejo, Health Expect, 2023, 26, 476 CrossRef PubMed
. - Y. Yang, Q. Huang, Z. Xiao, M. Liu, Y. Zhu, Q. Chen, Y. Li and K. Ai, Mater. Today Bio, 2022, 13, 100218 CrossRef CAS PubMed
. - A. Pulumati, A. Pulumati, B. S. Dwarakanath, A. Verma and R. V. L. Papineni, Cancer Rep., 2023, 6, 1764 CrossRef PubMed
. - Ovarian Cancer, https://pubmed.ncbi.nlm.nih.gov/33620837/, accessed October 2024 Search PubMed.
- J. K. Chan, C. Tian, J. P. Kesterson, B. J. Monk, D. S. Kapp, B. Davidson, S. Robertson, L. J. Copeland, J. L. Walker, R. M. Wenham, Y. Casablanca, N. M. Spirtos, K. S. Tewari and J. G. Bell, Obstet. Gynecol., 2022, 139, 157 CrossRef CAS PubMed
. - J. Dilley, M. Burnell, A. Gentry-Maharaj, A. Ryan, C. Neophytou, S. Apostolidou, C. Karpinskyj, J. Kalsi, T. Mould, R. Woolas, N. Singh, M. Widschwendter, L. Fallowfield, S. Campbell, S. J. Skates, A. McGuire, M. Parmar, I. Jacobs and U. Menon, Gynecol. Oncol., 2020, 158, 316 CrossRef PubMed
. - S. S. Buys, E. Partridge, A. Black, C. C. Johnson, L. Lamerato, C. Isaacs, D. J. Reding, R. T. Greenlee, L. A. Yokochi, B. Kessel, E. D. Crawford, T. R. Church, G. L. Andriole, J. L. Weissfeld, M. N. Fouad, D. Chia, B. O'Brien, L. R. Ragard, J. D. Clapp, J. M. Rathmell, T. L. Riley, P. Hartge, P. F. Pinsky, C. S. Zhu, G. Izmirlian, B. S. Kramer, A. B. Miller, J. L. Xu, P. C. Prorok, J. K. Gohagan and C. D. Berg, JAMA, 2011, 305, 2295 CrossRef CAS PubMed
. - J. M. Liberto, S. Y. Chen, I. M. Shih, T. H. Wang, T. L. Wang and T. R. Pisanic, Cancers (Basel), 2022, 14, 2885 CrossRef CAS PubMed
. - PDQ Cancer Information Summaries, https://www.ncbi.nlm.nih.gov/books/NBK66007/, accessed October 2024 Search PubMed.
- M. Kossaï, A. Leary, J. Y. Scoazec and C. Genestie, Pathobiology, 2018, 85, 41 CrossRef PubMed
. - R. J. Kurman and I. M. Shih, Am. J. Surg. Pathol., 2010, 34, 433 CrossRef PubMed
. - L. C. Clark and C. Lyons, Ann. N. Y. Acad. Sci., 1962, 102, 29 CrossRef CAS PubMed
. - J. Wu, H. Liu, W. Chen, B. Ma and H. Ju, Nat. Rev. Bioeng., 2023, 1, 346 CrossRef PubMed
. - M. A. Mohamed, A. M. Yehia, C. E. Banks and N. K. Allam, Biosens. Bioelectron., 2017, 89, 1034 CrossRef CAS PubMed
. - A. Jarahi Khameneh, S. Rahimi, M. H. Abbas, S. Rahimi, S. Mehmandoust, A. Rastgoo, A. Heydarian and V. Eskandari, Chem. Phys. Impact, 2024, 8, 100425 CrossRef
. - P. Valkova and M. Pohanka, Int. J. Anal. Chem., 2021, 2021, 9984876 Search PubMed
. - A. Allegra, C. Petrarca, M. Di Gioacchino, G. Mirabile and S. Gangemi, Cancers (Basel), 2022, 15, 146 CrossRef PubMed
. - S. Madhurantakam, S. Muthukumar and S. Prasad, ACS Omega, 2022, 7, 12467 CrossRef CAS PubMed
. - S. Narlawar, S. Coudhury and S. Gandhi, in Biosensor Based Advanced Cancer Diagnostics: from Lab to Clinics, ed. R. Khan, A. Parihar and S. K. Sanghi, Elsevier, Cambridge, 2022, vol. 10, p. 165 Search PubMed
. - M. A. Mohamed, D. M. El-Gendy, N. Ahmed, C. E. Banks and N. K. Allam, Biosens. Bioelectron., 2018, 101, 90 CrossRef CAS PubMed
. - I. Sharafeldin, S. Garcia-Rios, N. Ahmed, M. Alvarado, X. Vilanova and N. K. Allam, J. Environ. Chem. Eng, 2021, 9, 104534 CrossRef CAS
. - R. G. Elfarargy, M. A. Saleh, M. M. Abodouh, M. A. Hamza and N. K. Allam, Biosensors, 2023, 13, 51 CrossRef CAS PubMed
. - H. Sharma and R. Mutharasan, Sensor Actuators, B, 2013, 183, 535 CrossRef CAS
. - N. Dükar, S. Tunç, K. Öztürk, S. Demirci, M. Dumangöz, M. S. Çelebi and F. Kuralay, Mater. Chem. Phys., 2019, 228, 357 CrossRef
. - M. S. Sumitha and T. S. Xavier, Hybrid Adv., 2023, 2, 100023 CrossRef
. - A. Khanmohammadi, A. Jalili Ghazizadeh, P. Hashemi, A. Afkhami, F. Arduini and H. Bagheri, Iran. Chem. Soc, 2020, 17, 2429 CrossRef CAS
. - J. H. Park, G. Y. Lee, Z. Song, J. H. Bong, Y. W. Chang, S. Cho, M. J. Kang and J. C. Pyun, Biosens. Bioelectron., 2022, 202, 113975 CrossRef CAS PubMed
. - H. Hayrapetyan, T. Tran, E. Tellez-Corrales and C. Madiraju, Methods Mol. Biol., 2023, 2612, 1–17 CrossRef CAS PubMed
. - M. S. Tabatabaei and M. Ahmed, Methods Mol. Biol., 2022, 2508, 115 CrossRef PubMed
. - M. A. Saleh, M. M. Taha, M. A. Mohamed and N. K. Allam, Microchem. J., 2021, 164, 105946 CrossRef CAS
. - S. Hosseini, P. Vázquez-Villegas, M. Rito-Palomares and S. O. Martinez-Chapa, in Enzyme-linked Immunosorbent Assay (ELISA): from A to Z, SpringerBriefs in Applied Sciences and Technology, Springer, Singapore, 2018, vol. 5, p. 67 Search PubMed
. - S. Sakamoto, W. Putalun, S. Vimolmangkang, W. Phoolcharoen, Y. Shoyama, H. Tanaka and S. Morimoto, J. Nat. Med., 2017, 72, 32 CrossRef PubMed
. - V. K. Sarhadi and G. Armengol, Biomolecules, 2022, 12, 1021 CrossRef CAS PubMed
. - A. Yokoi, J. Matsuzaki, Y. Yamamoto, Y. Yoneoka, K. Takahashi, H. Shimizu, T. Uehara, M. Ishikawa, S. ichi Ikeda, T. Sonoda, J. Kawauchi, S. Takizawa, Y. Aoki, S. Niida, H. Sakamoto, K. Kato, T. Kato and T. Ochiya, Nat. Commun., 2018, 9, 4319 CrossRef PubMed
. - T. X. Lu and M. E. Rothenberg, J. Allergy Clin. Immunol., 2018, 141, 1202 CrossRef CAS PubMed
. - L. Zhang, S. Volinia, T. Bonome, G. A. Calin, J. Greshock, N. Yang, C. G. Liu, A. Giannakakis, P. Alexiou, K. Hasegawa, C. N. Johnstone, M. S. Megraw, S. Adams, H. Lassus, J. Huang, S. Kaur, S. Liang, P. Sethupathy, A. Leminen, V. A. Simossis, R. Sandaltzopoulos, Y. Naomoto, D. Katsaros, P. A. Gimotty, A. DeMichele, Q. Huang, R. Bützow, A. K. Rustgi, B. L. Weber, M. J. Birrer, A. G. Hatzigeorgiou, C. M. Croce and G. Coukos, Proc. Natl. Acad. Sci. U. S. A., 2008, 105, 7004 CrossRef CAS PubMed
. - L. Zhao, X. Liang, L. Wang and X. Zhang, Reprod. Sci., 2022, 29, 2760 CrossRef CAS PubMed
. - G. Sun, C. Chen, X. Li, S. Hong, C. Gu and X. Shi, J Cancer, 2023, 14, 707 CrossRef CAS PubMed
. - A. Talaat, M. A. Helmy and S. F. Saadawy, Egpt. J. Med. Hum. Genet., 2022, 23, 1–7 CrossRef PubMed
. - E. R. Asl, S. Sarabandi, B. Shademan, K. Dalvandi, G. sheikhansari and A. Nourazarian, Biochem. Biophys. Rep., 2023, 35, 101519 CAS
. - P. Magee, L. Shi and M. Garofalo, Ann. Transl. Med., 2015, 3, 332 Search PubMed
. - A. A. Alshamrani, Front. Oncol., 2020, 10, 1084 CrossRef PubMed
. - M. Koutsaki, M. Libra, D. A. Spandidos and A. Zaravinos, Oncotarget, 2017, 8, 66629 CrossRef PubMed
. - M. V. Iorio, R. Visone, G. Di Leva, V. Donati, F. Petrocca, P. Casalini, C. Taccioli, S. Volinia, C. G. Liu, H. Alder, G. A. Calin, S. Ménard and C. M. Croce, Cancer Res., 2007, 67, 8699 CrossRef CAS PubMed
. - Y. Chun Gao and J. Wu, Tumor Biol., 2015, 36, 4843 CrossRef PubMed
. - A. K. Mitra, M. Zillhardt, Y. Hua, P. Tiwari, A. E. Murmann, M. E. Peter and E. Lengyel, Cancer Discov., 2012, 2, 1100 CrossRef CAS PubMed
. - H. Zhang, S. Xu and X. Liu, Oncol. Lett., 2019, 17, 5601 CAS
. - P. Ferreira, R. A. Roela, R. V. M. Lopez, M. D. P. Estevez-Diz, P. Ferreira, R. A. Roela, R. V. M. Lopez and M. Del Pilar Estevez-Diz, Oncotarget, 2020, 11, 1085 CrossRef PubMed
. - V. K. Sarhadi and G. Armengol, Biomolecules, 2022, 12, 1021 CrossRef CAS PubMed
. - M. Christie and M. K. Oehler, J. Br. Menopause Soc., 2006, 12, 57 CrossRef PubMed
. - S. I. Kim, M. Jung, K. Dan, S. Lee, C. Lee, H. S. Kim, H. H. Chung, J. W. Kim, N. H. Park, Y. S. Song, D. Han and M. Lee, Cancers (Basel), 2020, 26, 790 CrossRef PubMed
. - D. Müller and B. Győrffy, Biochim. Biophys. Acta Rev. Cancer, 2022, 1877, 188722 CrossRef PubMed
. - M. T. C. Poon, S. Keni, V. Vimalan, C. Ip, C. Smith, S. Erridge, C. J. Weir and P. M. Brennan, Neurooncol. Adv., 2021, 3, 171 Search PubMed
. - R. Zhang, M. K. Y. Siu, H. Y. S. Ngan and K. K. L. Chan, Int. J. Mol. Sci., 2022, 23, 12041 CrossRef CAS PubMed
. - C. C. Johnson, B. Kessel, T. L. Riley, L. R. Ragard, C. R. Williams, J. L. Xu and S. S. Buys, Gynecol. Oncol., 2008, 110, 383 CrossRef CAS PubMed
. - J. Parkash, V. Bansro, G. S. Chhabra and Z. Mujahid, Cureus, 2023, 15, 34534 Search PubMed
. - G. Funston, L. T. A. Mounce, S. Price, B. Rous, E. J. Crosbie, W. Hamilton and F. M. Walter, Br. J. Gen. Pract., 2021, 71, 465 CrossRef PubMed
. - L. Salminen, N. Nadeem, S. Jain, S. Grènman, O. Carpén, S. Hietanen, S. Oksa, U. Lamminmäki, K. Pettersson, K. Gidwani, K. Huhtinen and J. Hynninen, Gynecol. Oncol., 2020, 156, 689 CrossRef CAS PubMed
. - I. M. Sharafeldin, J. E. Fitzgerald, H. Fenniri, N. K. Allam, Computational modeling for biomimetic sensors, in Biomimetic Sensing. Methods in Molecular Biology, Humana, New York, NY, eds. J. Fitzgerald and H. Fenniri, DOI:10.1007/978-1-4939-9616-2_16.
- P. N. Navya and H. K. Daima, Nano Convergence, 2016, 3, 1 CrossRef CAS PubMed
. - C. Venkatesh, O. Clear, I. Major, J. G. Lyons and D. M. Devine, Materials, 2019, 12, 1830 CrossRef CAS PubMed
. - M. A Mohamed, M. M Hasan, I. H. Abdullah, A. M. Abdellah, A. M. Yehia, N. Ahmed, W. Abbas and N. K. Allam, Talanta, 2018, 185, 344–351 CrossRef PubMed
. - P. Supraja, V. Singh, S. R. K. Vanjari and S. Govind Singh, Microsyst. Nanoeng., 2020, 6, 3 CrossRef CAS PubMed
. - S. Kurbanoglu, S. A. Ozkan and A. Merkoçi, Biosens. Bioelectron., 2017, 89, 886 CrossRef CAS PubMed
. - S. Malik, J. Singh, R. Goyat, Y. Saharan, V. Chaudhry, A. Umar, A. A. Ibrahim, S. Akbar, S. Ameen and S. Baskoutas, Heliyon, 2023, 9, 19929 CrossRef PubMed
. - X. Wang, S. C. Huang, S. Hu, S. Yan and B. Ren, Nat. Rev. Phys., 2020, 2, 253 CrossRef
. - T. Bruna, F. Maldonado-Bravo, P. Jara and N. Caro, Int. J. Mol. Sci., 2021, 22, 7202 CrossRef CAS PubMed
. - S. Ramanathan, S. C. B. Gopinath, P. Anbu, T. Lakshmipriya, F. H. Kasim and C. G. Lee, J. Mol. Struct., 2018, 1160, 80 CrossRef CAS
. - P. Tan, H. S. Li, J. Wang and S. C. B. Gopinath, Biotechnol. Appl. Biochem., 2021, 68, 1236 CAS
. - K. Zhu, Y. Ju, J. Xu, Z. Yang, S. Gao and Y. Hou, Acc. Chem. Res., 2018, 51, 404 CrossRef CAS PubMed
. - C. Shasha, E. Teeman, K. M. Krishnan, P. Szwargulski, T. Knopp and M. Möddel, Phys. Med. Biol., 2019, 64, 74001 CrossRef CAS PubMed
. - S. Osswald, G. Yushin, V. Mochalin, S. O. Kucheyev and Y. Gogotsi, J. Am. Chem. Soc., 2006, 128, 11635 CrossRef CAS PubMed
. - L. W. Tsai, Y. C. Lin, E. Perevedentseva, A. Lugovtsov, A. Priezzhev and C. L. Cheng, Int. J. Mol. Sci., 2016, 17, 1111 CrossRef PubMed
. - A. M. Schrand, H. Huang, C. Carlson, J. J. Schlager, E. Osawa, S. M. Hussain and L. Dai, J. Phys. Chem. B, 2007, 111, 2 CrossRef CAS PubMed
. - T. Zheng, F. Perona Martínez, I. M. Storm, W. Rombouts, J. Sprakel, R. Schirhagl and R. De Vries, Anal. Chem., 2017, 89, 12812 CrossRef CAS PubMed
. - V. Mochalin, O. Shenderova, D. Ho and Y. Gogotsi, in Nano-Enabled Medical Applications, ed. L. P. Balogh, Jenny Stanford Publishing, Singapore, 2020, vol. 11, p. 313 Search PubMed
. - H. W. Kroto, J. R. Heath, S. C. O'Brien, R. F. Curl and R. E. Smalley, Nature, 1985, 318, 162 CrossRef CAS
. - V. D. N. Bezzon, T. L. A. Montanheiro, B. R. C. De Menezes, R. G. Ribas, V. A. N. Righetti, K. F. Rodrigues and G. P. Thim, Adv. Mater. Sci. Eng., 2019, 2019, 293073 Search PubMed
. - M. M. Hasan, G. E. Khedr and N. K. Allam, ACS Appl. Nano Mater., 2022, 5, 15457 CrossRef CAS
. - A. S. Hassanien, R. A. Shedeed and N. K. Allam, J. Phys. Chem. C, 2016, 120, 21678 CrossRef CAS
. - G. Yildiz, M. Bolton-Warberg and F. Awaja, Acta Biomater., 2021, 131, 62 CrossRef CAS PubMed
. - S. Kazemi, M. Pourmadadi, F. Yazdian and A. Ghadami, Int. J. Biol. Macromol., 2021, 186, 554 CrossRef CAS PubMed
. - P. Samadi Pakchin, M. Fathi, H. Ghanbari, R. Saber and Y. Omidi, Biosens. Bioelectron., 2020, 153, 112029 CrossRef CAS PubMed
. - B. Fatima, D. Hussain, S. Bashir, H. T. Hussain, R. Aslam, R. Nawaz, H. N. Rashid, N. Bashir, S. Majeed, M. N. Ashiq and M. Najam-ul-Haq, Mater. Sci. Eng., C, 2020, 117, 111296 CrossRef CAS PubMed
. - S. Cotchim, P. Thavarungkul, P. Kanatharana, T. Thantipwan, A. Jiraseree-amornkun, R. Wannapob and W. Limbut, Mikrochim. Acta, 2023, 190, 232 CrossRef CAS PubMed
. - W. Mu, C. Wu, F. Wu, H. Gao, X. Ren, J. Feng, M. Miao, H. Zhang, D. Chang and H. Pan, J. Pharm. Biomed. Anal., 2024, 243, 116080 CrossRef CAS PubMed
. - T. S. C. R. Rebelo, R. Costa, A. T. S. C. Brandão, A. F. Silva, M. G. F. Sales and C. M. Pereira, Anal. Chim. Acta, 2019, 1082, 126 CrossRef CAS PubMed
. - C. Hu, Z. Qin, J. Fu, Q. Gao, C. Chen, C. S. Tan and S. Li, Anal. Biochem., 2023, 675, 115213 CrossRef CAS PubMed
. - M. Amirabadizadeh, H. Siampour, S. Abbasian, M. Nikkhah and A. Moshaii, Mikrochim. Acta, 2023, 191, 2 CrossRef PubMed
. - N. Runprapan, F. M. Wang, A. Ramar and C. C. Yuan, Sensors (Basel), 2023, 23, 1131 CrossRef CAS PubMed
. - N. Krathumkhet, T. Imae, F. ming Wang, C. C. Yuan, J. Manidae Lumban Gaol and N. Paradee, Bioelectrochemistry, 2023, 152, 108430 CrossRef CAS PubMed
. - F. M. Wang, S. H. Huang, C. C. Yuan, C. T. Yeh, W. L. Chen, X. C. Wang, N. Runprapan, Y. J. Tsai, Y. L. Chuang and C. H. Su, J. Appl. Electrochem., 2020, 50, 1189 CrossRef CAS
. - T. Liang, Q. Qu, Y. Chang, S. C. B. Gopinath and X. T. Liu, Biotechnol. Appl. Biochem., 2019, 66, 939 CrossRef CAS PubMed
. - D. N. Chen, L. Y. Jiang, J. X. Zhang, C. Tang, A. J. Wang and J. J. Feng, Mikrochim. Acta, 2022, 189, 455 CrossRef CAS PubMed
. - M. A. H. Nawaz, M. H. Akhtar, J. Ren, N. Akhtar, A. Hayat and C. Yu, Nanotechnology, 2022, 33, 485502 CrossRef CAS PubMed
. - V. Bianchi, M. Mattarozzi, M. Giannetto, A. Boni, I. De Munari and M. Careri, Sensors (Basel), 2021, 20, 2016 CrossRef PubMed
. - M. Moazampour, H. R. Zare and Z. Shekari, Anal. Methods, 2021, 13, 2021 RSC
.
|
This journal is © The Royal Society of Chemistry 2024 |
Click here to see how this site uses Cookies. View our privacy policy here.