DOI:
10.1039/D1RA02233D
(Paper)
RSC Adv., 2021,
11, 19323-19332
Exploration of stilbenoid trimers as potential inhibitors of sirtuin1 enzyme using a molecular docking and molecular dynamics simulation approach†
Received
20th March 2021
, Accepted 21st May 2021
First published on 27th May 2021
Abstract
A combination of molecular docking and molecular dynamics simulation (250 ns) has been carried out to study the interaction of stilbenoid trimer compounds with the SIRT1 enzyme as the target protein. SIRT1 expression regulates cellular stress responses that lead to the development of cancer. Redocking showed a good native ligand pose with an RMSD value of 1.40 Å at the receptor active site's coordinates. The molecular docking score uses a grid score functional (kcal mol−1), which shows results of 1NS: 79.56, TS1: −26.83, TS2: −87.77, and TS3: −83.67. The TS2 and TS3 candidates were chosen for further analysis because they had a lower grid score than the native ligand (1NS). Furthermore, prediction of binding free energy (kcal mol−1) using the Quantum Mechanics/generalized Born Surface Area (QM/MM-GBSA) method shows the results of 1NS: −31.52 ± 0.39, TS2: −58.99 ± 0.34, and TS3: −43.38 ± 0.35. These results indicate that the TS2 and TS3 compounds have good potential as inhibitors of the SIRT1 enzyme. Additionally, the amino acid residues were responsible for the inhibition mechanism through hydrogen bond interactions at the molecular level, including ASP22, PHE91, PRO11, ILE165, ASP166, and VAL230. The observations made in this study provide theoretical information for exploring the stilbenoid trimers as anticancer agents by targeting the SIRT1 enzyme.
Introduction
The enzyme sirtuin1 is present in the nucleus, and belongs to class III histone deacetylases (HDACs) which regulate multiple biological processes.1,2 The enzyme sirtuin1 (SIRT1) is one of the isoforms of the sirtuin enzyme family (SIRT1–SIRT7), which has become the center of attention due to its implications for cancer.3–5 It is known that the overexpression of the SIRT1 enzyme affects cancer development, such as breast cancer,6,7 colon cancer,7 and prostate cancer.7,8 The nicotinamide adenine dinucleotide (NAD) deacetylase dependent plays an important role in regulating cellular stress responses.9 SIRT1 enzyme can deacetylate other proteins to facilitate cell growth through cell cycle pathways, such as FOXO3a, RB1, KU70, and E2F1.10 Besides, the activity of the SIRT1 enzyme can mediate cancer growth through the apoptotic pathway by inhibiting p53 activity.11 Therefore, studies regarding the inhibition of the SIRT1 enzyme are promising as an inhibitory target in suppressing cancer growth.
Studies on the inhibition of the SIRT1 enzyme had reported by several previous studies using in vitro approach.12–16 One of them was 4-(4-(2-[(methylsulfonyl)amino]ethyl)piperidin-1-yl)thieno [3,2-d]pyrimidine-6-carboxamide had known can inhibit the SIRT1 enzyme with IC50 value 4 nM.16 Additionally, stilbenoid derivatives showed SIRT1 (IC50 μM) enzyme inhibitory activity, such as stilbenoid dimer ((−)-ampelopsin F: 24.4 μM) and stilbenoid tetramer ((+)-vitisin A: 22.0 μM, (−)-vitisin B: 21.1 μM, (+)-hopeaphenol: 3.45 μM, (−)-hopeaphenol: 18.1 μM, and (−)-isohopeaphenol: 21.6 μM).17
Stilbenoid derivatives obtained from natural products are one of the solutions to get potential inhibitors against cancer.18 Stilbenoid derivatives are a class of polyphenol compounds have known to inhibit the SIRT1 enzyme.17,19 Stilbenoid derivatives such as stilbenoid trimers isolated in the Dryobalanops20,21 and Vitis labrusca22 plants, widely distributed in Indonesia and Malaysia. Structurally, the stilbenoid trimers consist of three resveratrol monomers, which are linked by cyclic 2,3-dihydrofuran. Besides, the stilbenoid trimers have three hydroxy (–OH) groups in each monomer.23 The presence of this hydroxy group is expected to have a promising interaction with the amino acid residues at the active site of the SIRT1 enzyme in the form of a hydrogen bond donor (HBD).
The combination and integration of molecular docking and molecular dynamic (MD) simulation is the main focus to study the potential of stilbenoid trimers as inhibitors of the SIRT1 enzyme. The structure-based approach has the advantage of exploring the inhibitory mechanism through the interaction between ligand and receptor at the molecular level.24 Evaluation of the interaction energy between ligand and receptor through a combination of molecular docking and MD simulation are the main parameters that are focused on this research. The grid score functional has been reported to have the advantage of efficient calculation in finding the coordinates of the receptor active site.25 Meanwhile, further evaluation about binding affinity through the calculation of binding free energy using the Quantum Mechanics/Molecular Mechanics-generalized Born Surface Area (QM/MM-GBSA) method. The binding free energy calculation based on QM/MM requires a high cost and a long calculation time. However, calculation results can improve the prediction accuracy compared to conventional calculation (MM).26,27 It is expected that a structure-based approach by considering the important parameters presented in this study will help in understanding the inhibition mechanism of the stilbenoid trimers against the SIRT1 enzyme.
Methodology
Ligand and receptor preparation
The target protein used the NAD-dependent protein deacetylase sirtuin-1 (SIRT1) enzyme obtained from the Protein Data Bank (PDB code: 4ZZ1). The crystal structure of SIRT1 (PDB code: 4ZZ1) has native ligands, namely 4-(4-(2-[(methylsulfonyl)amino]ethyl) piperidin-1-yl)thieno[3,2-d]pyrimidine-6-carboxamide (PDB code: 1NS) at the active site's.28 The native ligand (1NS) was a reference in this study. Because it has known the 1NS ligand can inhibit the SIRT1 enzyme from previous research.16 Meanwhile, the ligand candidates used in this study were stilbenoid trimer compounds obtained from the tree bark of Dryobalanops oblongifolia and Vitis labrusca (Fig. 1). The stilbenoid trimer compounds that were successfully isolated and characterized are (−)-α-viniferin (TS1),22 cis-diptoindonesin B (TS2),29 and trans-diptoindonesin B (TS3).29 The TS2 and TS3 compounds are new compounds that were successfully reported by previous research.29
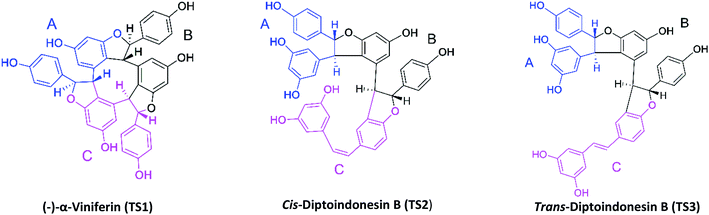 |
| Fig. 1 Chemical structure of stilbenoid trimers isolated from the tree bark of Dryobalanops oblongifolia and Vitis labrusca, where the atomic label and ring region are marked. | |
Geometry optimization of the stilbenoid trimers as a ligand using the Semiempirical Quantum Method-Austin Model 1 (SQM-AM1) to calculate the electrostatic potential (ESP) charges using the Gaussian 16 package.30 The coordinates of the native ligand (PDB code: 1NS) and standard residue (receptor) were extracted from the crystal structure of SIRT1 (PDB code: 4ZZI) using Chimera version 1.13 package. Besides, missing residues and loop segments rebuilt using Modeller 9.21 package. The AMBER FF14SB force field and Austin Model 1-Bond Charge Correction (AM1-BCC) had used to calculate ligand and receptor parameters such as bonded, non bonded, and charge.
Molecular docking
Molecular docking uses the performance of the Dock6 package. The cluster sphere selection used a radius of 10.0 Å from the coordinates of the native ligand. The grid-box determination conducted using grid spacing 0.5 Å with a box size based on the selected cluster sphere. The minimization process at the molecular docking stage needs to minimize the energy when the ligand docking process to the receptors. The type of conformation used in observing ligand–receptor interactions is flexible conformation with energy calculations using a grid score functional. The validation process aims to find out the best pose using the RMSD criterion ≤ 2.0 Å.31 This step aims to obtain the best possible native ligand coordinates as a reference with RMSD close to 0 Å. Furthermore, footprint analysis had done to see the residues responsible for the ligand–receptor interactions on the active site by comparing van der Waals energy (EvdW) and electrostatic energy (Eele). This comparison aims to see the difference between the EvdW and Eele of native ligand between the crystal structure (reference) and the redocking result (pose).
Molecular dynamics simulation
Integration of the score function aims to obtain ligand coordinates from the docking results using the General AMBER Force Field (GAFF). Several AMBER18 tools such as antechamber and parmchk had used to generate ESP charges and missing ligand parameters. Topology preparation (ligand, receptor, complex, and solvated complex) using tleap. The solvent model used is the TIP3P water solvent model with a minimum distance of 12 Å. Then, the sodium ions (Na+) were randomly added to neutralize the simulated system. The process of system minimization goes through three stages of minimization: (i) minimization of water molecules, (ii) minimization of complexes, and (iii) minimization of the entire system. The added hydrogen atoms and water molecules were minimized by 500 steps of steepest descent and 3000 steps of conjugated gradient, while the rest of the atoms were restrained. This step aims to remove poor atomic contacts. Then, the receptor and ligand were minimized by 500 steps of steepest descent and 3000 steps of the conjugated gradient with the restrained solvent. Finally, the entire system was fully minimized by the same procedure. The heating stage of each system was gradually heated from 100 K to 310 K for 200 ps. The density (300 ps) stage and equilibrium (1000 ps) stage were performed with harmonic restrain of 30, 20, 10, and 5 kcal mol−1 Å−2. The entire system was simulated under the NPT (310 K and 1 atm) ensemble until reaching 250 ns to produce trajectories for further analysis purposes. Trajectory analysis uses tools available in AMBER18, such as process_mdout.perl and cpptraj. Analysis of system stability, such as total energy, temperature, density, pressure, and root-mean-square displacement (RMSD), was carried out for the entire trajectory with a simulation time of 250 ns. Meanwhile, further analysis needs such as root-mean-square fluctuation (RMSF), B-factor, atom contacts, the radius of gyration (RoG), solvent-accessible surface area (SASA), hydrogen bond, and binding affinity (ΔGbind) performed at the last 50 ns of the trajectory. The calculation of binding free energy (ΔG) using the MMPBSA.py tools available in the AMBER18 package.32 The Quantum Mechanics/Molecular Mechanics-generalized Born Surface Area (QM/MM-GBSA) method with quantum mechanics theory used is the Austin Model 1 (AM1) method. Mathematically, binding free energy (ΔGbind) can be defined using eqn (1) and (2). The determination of binding free energy using the quantum mechanics (QM) method with the theoretical level of AM1 Hamiltonian generate the calculation parameter of self consistent energy (ΔGSCF).33 Specifically, the energy components that affect binding free energy can be detailed in the gas phase (eqn (3)) and the solvation free energy (eqn (4)). The energy component in the gas phase shows the energy component consisting of bonded energy (ΔEbonded), van der Waals energy (ΔEvdW), and electrostatic energy (ΔEele).34 Meanwhile, the solvation free energy is the total of generalized-Born models (ΔGelesolv) and solvent-accessible surface area energy (ΔGnonpolarsolv).35 |
ΔGbind = ΔGcomplex − (ΔGreceptor + ΔGligand)
| (1) |
|
ΔGbind = ΔGGas + ΔGSCF + ΔGsolv
| (2) |
|
ΔGGas = ΔEbonded + ΔEvdW + ΔEele
| (3) |
|
ΔGSolv = ΔGelesolv + ΔGnonpolarsolv
| (4) |
Results and discussion
Redocking: active site analysis
The active site determination of the SIRT1 enzyme plays a crucial role in the success of molecular docking. The active site determination used the redocking method, which is the native ligand (1NS) against the receptor (SIRT1 enzyme) as a reference. The coordinates obtained from the redocking process of the 1NS native ligand against the receptor can be used as a coordinate reference for the docking process of candidate stilbenoid trimers. The initial coordinates of the native ligand on the active site selected based on the cluster sphere within a radius of 10.0 Å (Fig. 2A). The native ligand that has docked in the sphere cluster is expected to provide good coordinates according to the crystal structure's ligand reference coordinates. The RMSD value expresses the native ligand coordinate at the redocking stage. This value shows in two types of conformation, which are minimization conformation and flexible conformation. The results show that the two conformation types' poses have good coordinates with the reference ligands (Fig. 2B). The RMSD value between the reference ligand and the ligand pose shows the difference in the initial coordinates where the native ligand is located.36 Overall, the initial coordinate determination showed a good 1NS native ligand pose by meeting the criteria for an RMSD value ≤ 2.0 Å (Table 1). Furthermore, the binding energy analysis between the native ligand (pose) and the receptor is calculated based on the grid score, which is the total energy of the van der Waals (EvdW) and electrostatic (Eele) energy. The results show that the native ligand (pose) has a grid score of −79.56 kcal mol−1 (flexible conformation). It will be used as comparison data with the candidate ligand.
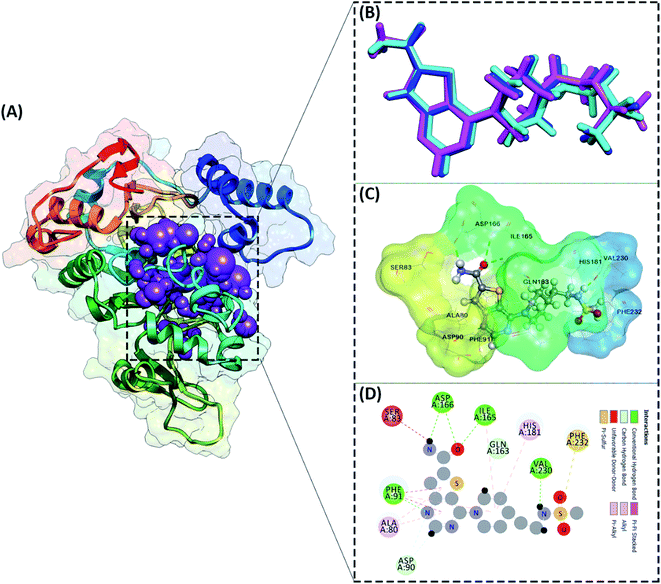 |
| Fig. 2 Active site determination: (A) cluster sphere selected within radius 10 Å from the native ligand, (B) the ligand native is represented by a crystal conformation (cyan), a minimized conformation (blue), and a flexible conformation (magenta), (C) the active site interaction between 1NS (flexible conformation) and amino acid residues, (D) the interaction types shown in 2D-diagram. | |
Table 1 Molecular docking validation of native ligand (1NS-4ZZI) using flexible conformation
Parameters |
Minimization |
Flexible |
RMSD (Å) |
0.55 |
1.40 |
Grid score (kcal mol−1) |
−75.72 |
−79.56 |
EvdW (kcal mol−1) |
−70.72 |
−71.92 |
Eele (kcal mol−1) |
−5.00 |
−7.63 |
Eint (kcal mol−1) |
13.37 |
9.16 |
Other parameters measured in the redocking process are the interaction of amino acid residues with the native ligand. The number of amino acid residues responsible for the interaction with the native ligand is ten amino acid residues (Fig. 2C). In more detail, the amino acid residues PHE91, ILE165, ASP166, and VAL230 are the amino acids that have significant contributions to their interactions as hydrogen bonds (Fig. 2D). The footprint analysis aimed to see each amino acid residue's energy contribution in the interaction with the native ligands.37 The footprint analysis plays an important role in the docking validation process for the involvement of amino acid residues on the active site of the receptor (Fig. S1†). Overall, the footprint analysis on the native pose ligands of each amino acid residue on the active site showed the energy value of the footprint (EvdW + Eele) ≤ 0 kcal mol−1. The analysis of amino acid residues having hydrogen bond interactions identified the EvdW + Eele values, such as PHE91: −8.45 kcal mol−1, ILE165: −4.42 kcal mol−1, ASP166: −1.56 kcal mol−1, and VAL230: −0.53 kcal mol−1, respectively. The coordinates of the receptor active sites have been successfully validated. It then meets the criteria for the docking analysis stage of the stilbenoid trimers as candidate ligand.
Molecular docking: stilbenoid trimers-SIRT1 enzyme
ADMET predictions (absorption, distribution, metabolism, excretion, and toxicity) were performed using the pkCSM website service.38 ADMET analysis provides an important image in predicting the activity of the candidate as a drug before using molecular docking against the SIRT1 enzyme. Overall, the results indicated that the stilbenoid trimers met the criteria for a good ADMET as a drug (Table S1†). Especially the toxicity parameter, each candidate shows good suitability as a drug because it is non-toxic.
Molecular docking has been successfully implemented to study the interaction between the ligand candidate stilbenoid trimers and the SIRT1 enzyme as the target protein. The docking algorithms were constructed to fast calculate the possible ligand conformation within the receptor active site.39 The process of docking the candidate ligand with SIRT1 enzyme was carried out based on the initial coordinates obtained from the redocking process using flexible conformations. The results of the docking of candidate ligands with the receptor showed that the TS2 and TS3 ligands had good potential to bind to the SIRT1 enzyme because they had a lower grid score than the native ligand (Fig. 3). On the other hand, the TS1 candidate shows less good interaction because it has a larger grid score than the native ligand. The van der Waals energy (EvdW) and electrostatic energy (Eele) to the grid score of each ligand in the gas phase. Especially EvdW has a significant contribution. In detail, the results of the docking energy components (kcal mol−1), such as TS1 (EvdW: −20.03, Eele: −6.80), TS2 (EvdW: −76.44, Eele: −11.32), and TS3 (EvdW: −74.51, Eele: −9.16). Thus, overall the molecular docking results have good potential in interacting with the SIRT1 enzyme as an inhibitor: TS2> TS3> 1NS > TS1. Based on these considerations, candidate ligands with a grid score > 1NS (pose) grid score were selected for further analysis at the molecular dynamics simulation.
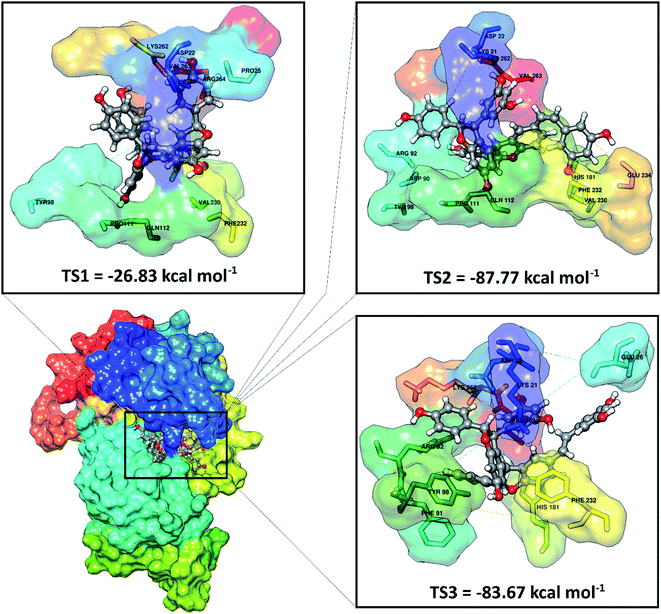 |
| Fig. 3 Molecular docking result of the candidate-receptor shows closer on the pocket area of the active site. | |
Further analysis of the interaction between amino acid residues and candidate ligand on the active site aims to study the candidate ligand's inhibition mechanism against the SIRT1 enzyme at the molecular level. The interactions between ligand and receptor affect the conformation changes of the complex structure.40 These conformational changes can lead to target protein inhibition. The candidate ligands' molecular docking results with the SIRT1 enzyme showed several types of interactions with the amino acid residues that were on the receptor active site (Fig. S2†). The TS1 ligand shows that five amino acid residues are responsible for hydrogen bonding, including ASP22 (region A: O48 and region B: O46), PRO111 (region C: O51), GLN112 (region A: O50), VAL230 (region B: O49), LYS262 (region B: O46). Meanwhile, the TS2 compound shows that eight amino acid residues are involved in the hydrogen bond interactions, such as LYS21 (region B: O12), ASP22 (region A: O24), ASP90 (region B: O26), TYR98 (region B: O1), PRO111 (region B: O1), VAL320 (region C: O50), GLU234 (region C: O51), and LYS262 (region A: O24). The TS3 compound shows that four amino acid residues are involved in the hydrogen bond interaction, such as LYS21 (region B: O12), ASP22 (region A: O25), GLU26 (region C: O50), and LYS262 (region A: O25). It should be noted that there are some differences in the C region conformation for the cis (TS2) and trans (TS3) positions of the stilbenoid trimers, which cause different interactions with the amino acid residues. It can be seen that the cis position tends to bind with the VAL230 and GLU234 residues as hydrogen bond donors. Conversely, the trans-position avoids both residues and is more likely to interact with the GLU26 residue as a hydrogen bond donor. There is a slight difference at the atomic level due to differences in conformation in region A on the ASP22 and LYS262 residues. The interaction occurs at atoms O24 for the cis position and O25 for the trans position with the ASP22 and LYS262 residues as hydrogen bond donors. This interaction is expected to provide an overview of the inhibition mechanism of the SIRT1 enzyme at the molecular level. For further analysis is to understand the interaction between the stilbenoid trimers and the SIRT1 enzyme. The deeper study should be carried out using molecular dynamics simulation.
System stability
The stability analysis of each system through several stages, such as minimization, heating, density, equilibrium, and production, was carried out for 250 ns simulation time. Several parameters need to be validated to see the system stability, such as total energy, temperature, density, pressure, and RMSD. Overall, some of the parameters analyzed, such as total energy (kcal mol−1), temperature (K), density (g mL−1), and pressure (bar), showed good stability with no significant fluctuation (Fig. S3†). It should be noted, the average value of each system is 1NS-SIRT1 (total energy: −147,170 ± 799.27, temperature: 310.00 ± 1.58, density: 1.00 ± 0.00, and pressure: 0.36 ± 110.17), TS2-SIRT1 (total energy: −146,966 ± 803.55, temperature: 310.01 ± 1.57, density: 1.00 ± 0.00, and pressure: 0.65 ± 111.34), and TS3-SIRT1 (total energy: −146,936 ± 801.78, temperature: 310.00 ± 1.58, density: 1.00 ± 0.00, and pressure: 1.96 ± 112.14). Meanwhile, the root-mean-square displacement (RMSD) value of each system is the main parameter in assessing the system stability for further analysis.41–43 The RMSD value (nm) shows that each system has fluctuation with its average value of Apo protein: 0.27 ± 0.04, 1NS-SIRT1: 0.31 ± 0.04, TS2-SIRT1: 0.35 ± 0.05, and TS3-SIRT1: 0.35 ± 0.05. The TS2-SIRT1 system showed excellent stability compared to the Apo protein, 1NS-SIRT1, and TS2-SIRT1 systems (Fig. 4). It shows that the fluctuation occurred in the TS2-SIRT1 at the beginning of 70 ns and did not experience significant fluctuation until 250 ns. Significant fluctuation occurs in the Apo protein (90–105 ns), 1NS-SIRT1 (90–105 ns), and TS3-SIRT1 (180–190 ns). The fluctuation occurs because there is a conformational change in the complex structure during the simulation time. These conformational changes occur in the protein and ligand structure. But, there were no significant conformational changes in the ligands. Additionally, the ligand coordinates of each complex are still on the active side (pocket) of the receptor. The analysis process is carried out on the last 50 ns (200–250 ns) trajectory of each system for further analysis, considering that each system has achieved good stability.
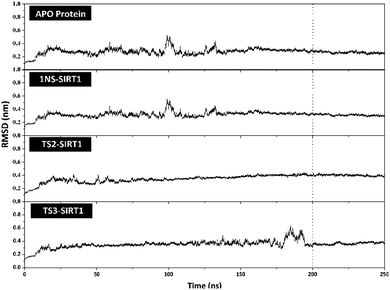 |
| Fig. 4 Trajectories analysis: the root-mean-square displacement of each system plotted along the 250 ns of MD simulation. | |
MD simulation analysis: flexibility, compactness, atom contacts, and solvent accessibility
Trajectories analysis in the last 50 ns used to calculate some crucial parameters for each system. The root-mean-square fluctuation (RMSF) and B-factor analysis aimed at looking for the complex flexibility.44 The TS2-SIRT1 showed lower fluctuation than the Apo protein, 1NS-SIRT1, and TS3-SIRT1 (Fig. 5). It indicates that the TS2 complex is more rigid because it has smaller mean RMSF (nm) and B-factor (nm−2) values compared to the Apo protein, 1NS-SIRT1, and TS3-SIRT1 (Table S2†). These results show a good correlation with the RMSD of each complex, as indicated by the dominance of the TS2-SIRT1 complex stability compared to other (Fig. 4). Specifically, the fluctuation occurs in the entire complex during the simulation time (200–250 ns). In particular, the loop region for APO protein, 1NS-SIRT1, and TS3-SIRT1 have fluctuation above RMSF: ∼1.0 nm and B-factor: ∼30 nm−2 (residues: 190–192, 212–223, and 323–328).
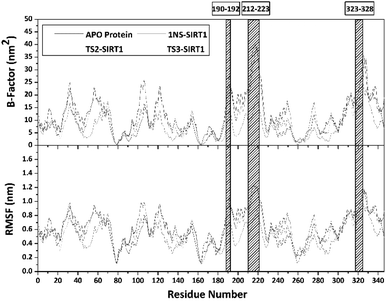 |
| Fig. 5 Flexibility analysis: the B-factor (top) and the root-mean-square fluctuation (bottom). | |
Atom contacts identified that the interaction between ligand and receptor occurred during the simulation time in the last 50 ns trajectory (Fig. 6). The results showed that the 1NS ligand had more contact with the amino acid residues on the receptor's active site than the TS2 and TS3 ligands (Table S2†). Additionally, the radius of gyration (RoG) analysis is one of the parameters for studying complex structure's compactness. The RoG value can provide a good image of a stably folded structure or unfolded structure.45 It shows by looking at the RoG value of the Apo protein (without ligand) and 1NS-SIRT1 (with ligand) as the standard of comparison (Fig. 6). The results show that the mean RoG values of the TS2-SIRT1 and TS3-SIRT1 complexes are relatively identical and consistent with the mean RoG values of 1NS-SIRT1 (Table S2†). Thus, these results identify the folded TS2-SIRT1 and TS3-SIRT1 structures stably.
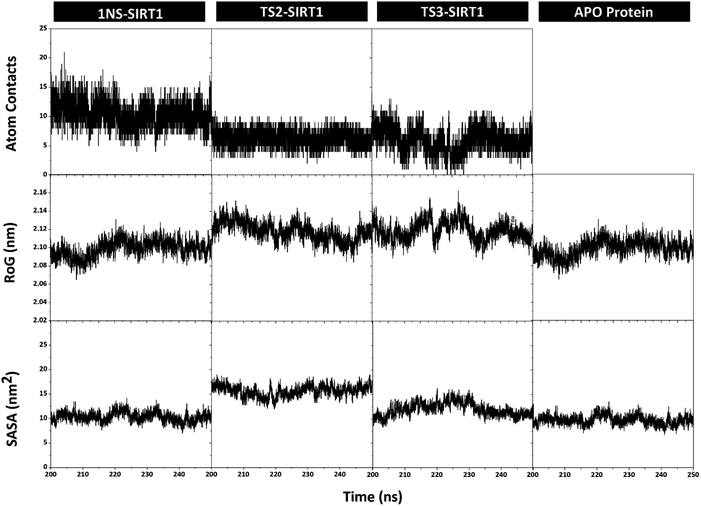 |
| Fig. 6 Trajectories analysis during the simulation over the last 50 ns: atom contacts within radius 3.5 Å (top), the radius of gyration (middle), and the solvent-accessible surface area (bottom). | |
The solvent-accessible surface area (SASA) parameter aims to determine how many water molecules (solvent) can access the active site surface of the complex during the simulation.42 The results showed no significant difference in the mean value of SASA (nm−2) between the Apo protein, 1NS-SIRT1, TS2-SIRT1, and TS3-SIRT1 (Table S2†). Furthermore, the TS2-SIRT1 shows that the surface-active site (radius 3.5 Å of the ligand) is more likely to be accessed by water molecules as a solvent than others. However, the overall analysis of the SASA values showed good stability for each complex. It shows by low fluctuation for the SASA value (Fig. 6).
Prediction of binding affinity
The stilbenoid trimers' susceptibility to the SIRT1 enzyme was performed using the QM/MM-GBSA method at 100 frames extracted from the last 50 ns trajectories. The binding affinity prediction was calculated based on the contribution of ligands, receptors, and complexes in the gas and solvated phases.46 The calculation energy parameters generated using the MMPBSA.py tool contained in the AMBER18 package produce energy components in detail (Table 2). The energy interaction in the gas phase (ΔGgas) shows that the main contribution to the interaction between the receptor (SIRT1 enzyme) and the ligand is the van der Waals energy (ΔEvdW). It is indicated by a suitable contribution of the van der Waals energy, which is greater than the contribution of electrostatic energy (ΔEele). Meanwhile, the solvated phase (ΔGsolv) modeled using GB models shows the contribution of good binding energy in the solvent's polar free energy (ΔGelesolv). On the other hand, unsatisfactory results were noted on the solvent's nonpolar free energy (ΔGnonpolarsolv) for each complex. The calculation of QM/MM-GBSA electrostatic term (ΔGSCF) using the semiempirical theory of Hamiltonian AM1 shows an excellent contribution to the binding free energy. It can be indicated by a more negative value in thermodynamic aspects.
Table 2 Binding free energy prediction calculated with the Quantum Mechanics/Molecular Mechanics-generalized Born Surface Area (QM/MM-GBSA) method. Data are shown as mean ± standard error of mean (SEM)
Energy (kcal mol−1) |
1NS-SIRT1 |
TS2-SIRT1 |
TS3-SIRT1 |
ΔEvdW |
−56.88 ± 0.26 |
−69.96 ± 0.30 |
−68.61 ± 0.35 |
ΔEele |
0.25 ± 0.00 |
0.14 ± 0.00 |
0.15 ± 0.00 |
ΔGgas |
−56.63 ± 0.26 |
−69.82 ± 0.30 |
−68.46 ± 0.35 |
ΔGSCF |
−46.08 ± 0.48 |
−49.62 ± 0.45 |
−42.69 ± 0.44 |
ΔGelesolv |
77.42 ± 0.49 |
70.10 ± 0.41 |
76.83 ± 0.31 |
ΔGnonpolarsolv |
−6.23 ± 0.01 |
−9.65 ± 0.02 |
−9.05 ± 0.02 |
ΔGsolv |
71.19 ± 0.49 |
60.44 ± 0.41 |
67.77 ± 0.30 |
ΔGbind |
−31.52 ± 0.39 |
−58.99 ± 0.34 |
−43.38 ± 0.35 |
Each energy contribution provides the ligand-receptor binding affinity information expressed in terms of binding free energy (ΔGbind). The TS2-SIRT1 and TS2-SIRT1 complexes show good binding affinity compared to INS-SIRT1 as the comparison standard. These results indicate that the stilbenoid trimers have an excellent potential in inhibiting the SIRT1 enzyme through a strong binding on the active site of its inhibition. To be more specific, the TS2 ligand has a lower ΔGbind than the TS3, which allows the TS2 ligand as a SIRT1 inhibitor to have a better potency than the TS3 ligand. Overall, the binding free energy calculation results show the binding affinity (kcal mol−1) of each complex: TS2 > TS3> 1NS. The results show a good correlation with the calculation of the grid score using molecular docking. Ligands that have low binding free energy are expected to bind with amino acid residues on the receptor active site, responsible for the activity of the SIRT1 enzyme. The strong binding affinity between the ligand-receptors is expected to change the complex structure's conformation and block the active site of the SIRT1 enzyme. Thus, the SIRT1 enzyme becomes inactive in providing regulation of cellular stress responses through NAD-dependent protein deacetylase, which plays an important role in cancer development.47
The binding energy decomposition analysis aims to identify the contribution of residues that have good bonds with ligands. Its calculation performed using the MM-GBSA method (Fig. S4†). Residues that have good contribution energy criteria are residues that have ΔGresiduebind ≤ −1.0 kcal mol−1.48 The results show overall that 20 residues have good contribution energy criteria, such as HIE9, ILE12, ALA80, PHE91, ARG92, TYR98, PRO111, GLN112, PHE115, ASN164, ILE165, ASP166, HIE181, VAL230, PHE231, PHE232, LEU236, LYS262, VAL263, and ARG264. Additionally, the 1NS-SIRT1 complex as a reference shows a good correlation between the energy decomposition and redocking result. Residues that correlate with bond contribution (MD simulation and docking) on the active site of the SIRT1 enzyme are PHE91, ILE165, ASP166, HIE181, VAL230, and PHE232 residues.
Hydrogen bonding analysis
The hydrogen bond is a significant interaction that contributes to a strong bond between ligand and receptor.48,49 It was recorded that the last 50 ns simulation time showed the number of hydrogen bond (Fig. S5†) and the percentage of hydrogen bond occupation (Fig. 7) of each ligand with amino acid residues on the active site of the SIRT1 enzyme. However, the amount of hydrogen having good bonding criteria is expressed by the presentation of hydrogen occupation during the simulation time. In detail, the hydrogen bond of each complex is well recorded through the interactions at the atomic level (Fig. 8).
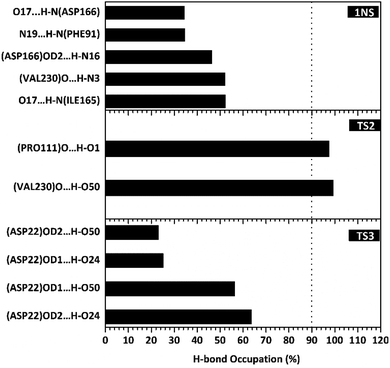 |
| Fig. 7 Percentage of hydrogen bond over the last 50 ns of MD simulation. | |
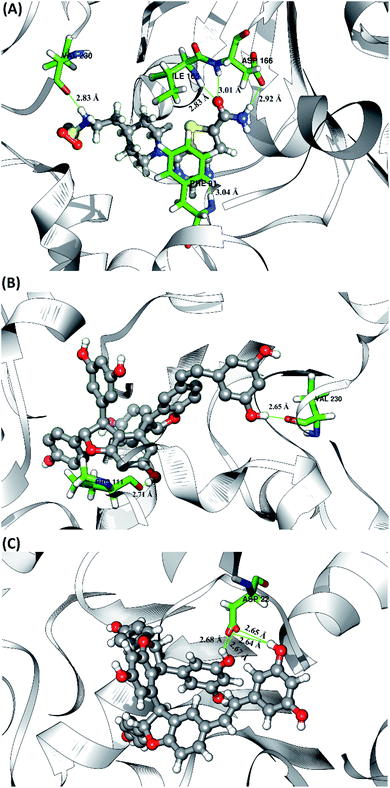 |
| Fig. 8 Visualization of hydrogen bond interaction between candidate and receptor on the active site. Hydrogen bond data are represented by a green line with the average value of distance in the angstroms unit (Å). | |
The 1NS ligand shows that there are four amino acid residues involving five hydrogen bonds: (i) O17⋯H–N (ILE165) at 52.32%, 2.83 Å, (ii) O (VAL230)⋯H–N3 at 52.28%, 2.84 Å, (iii) OD2 (ASP166)⋯H–N16 at 46.44%, 2.92 Å, (iv) N19⋯H–N (PHE91) at 34.6%, 3.04 Å, and (v) O17⋯H–N (ASP166) at 34.42%, 3.01 Å. The hydrogen bond identification of the 1NS ligand using the MD simulation showed a good correlation with the molecular docking results as an initial reference (Fig. 2D). Meanwhile, for each ligand candidate shows the hydrogen bond of the ligand TS2: (i) O (VAL230)⋯H–O50 (region C) at 99.26%, 2.65 Å and (ii) O (PRO111)⋯H–O1 (region B) at 97.38%, 2.71 Å. Hydrogen bond TS3: (i) OD2 (ASP22)⋯H–O24 (region A) at 63.60%, 2.68 Å, (ii) OD1 (ASP22)⋯H–O50 (region C) at 56.32%, 2.65 Å, (iii) OD1(ASP22)⋯H–O24 (region A) at 25.16%, 2.67 Å, and (iv) OD2 (ASP22)⋯H–O50 (region C) at 23.04%, 2.64 Å. These results indicate that the TS2 ligand has a strong interaction with two amino acid residues (POR111 and VAL230) on the active site of the SIRT1 enzyme. The TS2 ligand has a hydrogen bond occupation ≥90%, which indicates the criteria for a strong hydrogen bond.42 The TS3 ligand has four hydrogen bond interactions with one amino acid residue of ASP22 with a variation of occupation hydrogen bonds at different atoms. Hydrogen bond analysis shows a good evaluation of the TS2 candidate ligands that have strong bond criteria. This result shows a good correlation with the RMSD value of the TS2 complex (Fig. 4), which has good stability compared to the 1NS and TS3 complexes. It is possible due to the influence of the strong hydrogen bond possessed by the TS2 complex. Besides, the hydrogen bond can be an important factor in inhibiting the SIRT1 enzyme.
Conclusions
In this present study, a combination of molecular docking and molecular dynamics simulation was carried out to understand stilbenoid trimers' interaction as an inhibitor of the SIRT1 enzyme. The results of molecular docking using flexible conformation show that the stilbenoid trimers interact well on the receptor's active site. The TS2 and TS3 ligands showed good interaction energy with the SIRT1 enzyme, indicated by the grid score < grid score 1NS as the native ligand. Furthermore, the binding affinity analysis using molecular dynamics simulation with the QM/MM-GBSA approach shows that TS2 and TS3 ligands have ΔGbind < ΔGbind 1NS values. The hydrogen bond interaction has an important role in the interaction of the stilbenoid trimers with the amino acid residues on the active site of the SIRT1 receptor. The evaluation results using molecular docking and molecular dynamics simulation showed that the TS2 ligand has a stronger hydrogen bond (hydrogen bond occupation ≥90%) than the TS3. Additionally, the residues are responsible for the hydrogen bond interaction on the TS2 are PRO111 and VAL230. Overall, the combination of molecular docking and molecular dynamics simulation processes evaluated that the TS2 and TS3 ligands had promising potential as inhibitors of the SIRT1 enzyme.
Conflicts of interest
The authors declare no conflict of interest.
Acknowledgements
This study was supported by the research grant Program of “HIBAH RISET MANDAT KOLABORASI MITRA LUAR NEGERI” from Institute for Research and Community Service, Universitas Airlangga. Contract Number: 800/UN3.15/PT/2021.
References
- T. Liu, P. Y. Liu and G. M. Marshall, Cancer Res., 2009, 69, 1702–1705 CrossRef CAS PubMed.
- F. Yang, N. Zhao, D. Ge and Y. Chen, RSC Adv., 2019, 9, 19571–19583 RSC.
- Z. Lin and D. Fang, Genes Cancer, 2013, 4, 97–104 CrossRef CAS PubMed.
- N. Wössner, Z. Alhalabi, J. González, S. Swyter, J. Gan, K. Schmidtkunz, L. Zhang, A. Vaquero, H. Ovaa, O. Einsle, W. Sippl and M. Jung, Front. Oncol., 2020, 10, 1–15 CrossRef PubMed.
- E. S. Hwang and S. B. Song, Cell. Mol. Life Sci., 2017, 74, 3347–3362 CrossRef CAS PubMed.
- V. Carafa, L. Altucci and A. Nebbioso, Front. Pharmacol., 2019, 9, 1–14 Search PubMed.
- C. X. Deng, Int. J. Biol. Sci., 2009, 5, 147–152 CrossRef CAS PubMed.
- L. Ruan, L. Wang, X. Wang, M. He and X. Yao, Oncotarget, 2018, 9, 2002–2016 CrossRef PubMed.
- M. C. Vasquez and L. Tomanek, Comp. Biochem. Physiol., Part A: Mol. Integr. Physiol., 2019, 236, 1–11 CrossRef PubMed.
- E. Zhao, J. Hou, X. Ke, M. N. Abbas, S. Kausar, L. Zhang and H. Cui, Carbon, 2019, 11, 1–22 CrossRef.
- J. T. Lee and W. Gu, Genes Cancer, 2013, 4, 112–117 CrossRef CAS PubMed.
- V. Heger, J. Tyni, A. Hunyadi, L. Horáková, M. Lahtela-Kakkonen and M. Rahnasto-Rilla, Biomed. Pharmacother., 2019, 111, 1326–1333 CrossRef CAS PubMed.
- M. V. B. Rao, D. SriLaxmi, S. V. Vardhini, V. R. Guttikonda, R. Kapavarapu and M. Pal, ChemistrySelect, 2020, 5, 14239–14246 CrossRef CAS.
- C. Sekhar, N. Kumar, V. Nallanchakravarthula, D. Nayakanti, R. Kapavarapu and M. Pal, J. Mol. Struct., 2021, 1240, 130541 CrossRef.
- D. S. Laxmi, S. V. Vardhini, V. R. Guttikonda, M. V. B. Rao and M. Pal, Anti-Cancer Agents Med. Chem., 2020, 8, 932–940 CrossRef PubMed.
- J. S. Disch, G. Evindar, C. H. Chiu, C. A. Blum, H. Dai, L. Jin, E. Schuman, K. E. Lind, S. L. Belyanskaya, J. Deng, F. Coppo, L. Aquilani, T. L. Graybill, J. W. Cuozzo, S. Lavu, C. Mao, G. P. Vlasuk and R. B. Perni, J. Med. Chem., 2013, 56, 3666–3679 CrossRef CAS PubMed.
- K. Hikita, N. Seto, Y. Takahashi, A. Nishigaki, Y. Suzuki, T. Murata, A. Loisruangsin, N. S. Aminah, Y. Takaya, M. Niwa and N. Kaneda, Nat. Prod. Commun., 2018, 13, 1531–1534 CrossRef.
- M. H. Keylor, B. S. Matsuura and C. R. J. Stephenson, Chem. Rev., 2015, 115, 8976–9027 CrossRef CAS PubMed.
- A. Loisruangsin, K. Hikita, N. Seto, M. Niwa, Y. Takaya and N. Kaneda, BioFactors, 2019, 45, 253–258 CrossRef CAS PubMed.
- Indriani, Y. Takaya, N. N. T. Puspaningsih and N. S. Aminah, Chem. Nat. Compd., 2017, 53, 559–561 CrossRef CAS.
- A. Wibowo, N. Ahmat, A. S. Hamzah, A. S. Sufian, N. H. Ismail, R. Ahmad, F. M. Jaafar and H. Takayama, Fitoterapia, 2011, 82, 676–681 CrossRef CAS PubMed.
- M. A. Saputra, H. M. Sirat and N. S. Aminah, Chem. Nat. Compd., 2013, 49, 924–926 CrossRef CAS.
- T. Shen, X. N. Wang and H. X. Lou, Nat. Prod. Rep., 2009, 26, 916–935 RSC.
- V. Salmaso and S. Moro, Front. Pharmacol., 2018, 9, 1–16 CrossRef PubMed.
- H. S. Muniz and A. S. Nascimento, PLoS One, 2017, 12, 1–19 Search PubMed.
- B. Nutho, S. Pengthaisong, A. Tankrathok, V. S. Lee, J. R. K. Cairns, T. Rungrotmongkol and S. Hannongbua, Biomolecules, 2020, 10, 1–19 CrossRef PubMed.
- C. Peng, J. Wang, Y. Yu, G. Wang, Z. Chen, Z. Xu, T. Cai, Q. Shao, J. Shi and W. Zhu, Future Med. Chem., 2019, 11, 303–321 CrossRef CAS PubMed.
- H. Dai, A. W. Case, T. V. Riera, T. Considine, J. E. Lee, Y. Hamuro, H. Zhao, Y. Jiang, S. M. Sweitzer, B. Pietrak, B. Schwartz, C. A. Blum, J. S. Disch, R. Caldwell, B. Szczepankiewicz, C. Oalmann, P. Y. Ng, B. H. White, R. Casaubon, R. Narayan, K. Koppetsch, F. Bourbonais, B. Wu, J. Wang, D. Qian, F. Jiang, C. Mao, M. Wang, E. Hu, J. C. Wu, R. B. Perni, G. P. Vlasuk and J. L. Ellis, Nat. Commun., 2015, 6, 1–10 Search PubMed.
- Y. M. Syah, N. S. Aminah, E. H. Hakim, N. Aimi, M. Kitajima, H. Takayama and S. A. Achmad, Phytochemistry, 2003, 63, 913–917 CrossRef CAS PubMed.
- M. J. Frisch, G. W. Trucks, H. B. Schlegel, G. E. Scuseria, M. A. Robb, J. R. Cheeseman, G. Scalmani, V. Barone, B. Mennucci, G. A. Petersson, H. Nakatsuji, M. Caricato, X. Li, H. P. Hratchian, A. F. Izmaylov, J. Bloino, G. Zheng, J. L. Sonnenberg, M. Hada, M. Ehara, K. Toyota, R. Fukuda, J. Hasegawa, M. Ishida, T. Nakajima, Y. Honda, O. Kitao, H. Nakai, T. Vreven, J. A. Montgomery Jr, J. E. Peralta, F. Ogliaro, M. Bearpark, J. J. Heyd, E. Brothers, K. N. Kudin, V. N. Staroverov, R. Kobayashi, J. Normand, K. Raghavachari, A. Rendell, J. C. Burant, S. S. Iyengar, J. Tomasi, M. Cossi, N. Rega, J. M. Millam, M. Klene, J. E. Knox, J. B. Cross, V. Bakken, C. Adamo, J. Jaramillo, R. Gomperts, R. E. Stratmann, O. Yazyev, A. J. Austin, R. Cammi, C. Pomelli, J. W. Ochterski, R. L. Martin, K. Morokuma, V. G. Zakrzewski, G. A. Voth, P. Salvador, J. J. Dannenberg, S. Dapprich, A. D. Daniels, O. Farkas, J. B. Foresman, J. V. Ortiz, J. Cioslowski, and D. J. Fox, Gaussian 16, Revision C.01, Gaussian Inc., Wallingford CT, 2016 Search PubMed.
- K. Liu and H. Kokubo, J. Comput.-Aided Mol. Des., 2020, 34, 1195–1205 CrossRef CAS PubMed.
- B. R. Miller, T. D. Mcgee, J. M. Swails, N. Homeyer, H. Gohlke and A. E. Roitberg, J. Chem. Theory Comput., 2012, 8, 3314–3321 CrossRef CAS PubMed.
- P. C. Su, C. C. Tsai, S. Mehboob, K. E. Hevener and M. E. Johnson, J. Comput. Chem., 2015, 36, 1859–1873 CrossRef CAS PubMed.
- H. Luo, D. F. Liang, M. Y. Bao, R. Sun, Y. Y. Li, J. Z. Li, X. Wang, K. M. Lu and J. K. Bao, Int. J. Oral Sci., 2017, 9, 53–62 CrossRef CAS PubMed.
- B. Tang, B. Li, B. Li, Z. Li, J. Qin, X. Zhou, Y. Qiu, Z. Wu and M. Fang, RSC Adv., 2017, 7, 39185–39196 RSC.
- D. Ramírez and J. Caballero, Molecules, 2018, 23, 1–17 Search PubMed.
- Y. Zhou, M. W. Elmes, J. M. Sweeney, O. M. Joseph, J. Che, H. C. Hsu, H. Li, D. G. Deutsch, I. Ojima, M. Kaczocha and R. C. Rizzo, Biochemistry, 2019, 58, 4304–4316 CrossRef CAS PubMed.
- Y. Han, J. Zhang, C. Q. Hu, X. Zhang, B. Ma and P. Zhang, Front. Pharmacol., 2019, 10, 1–12 CrossRef PubMed.
- S. Bagheri, H. Behnejad, R. Firouzi and M. H. Karimi-Jafari, Mol. Inf., 2020, 39, 1–11 Search PubMed.
- L. Ivanova, J. Tammiku-Taul, A. T. García-Sosa, Y. Sidorova, M. Saarma and M. Karelson, ACS Omega, 2018, 3, 11407–11414 CrossRef CAS PubMed.
- A. Radwan and G. M. Mahrous, PLoS One, 2020, 15, 1–16 Search PubMed.
- P. Mahalapbutr, N. Darai, W. Panman, A. Opasmahakul, N. Kungwan, S. Hannongbua and T. Rungrotmongkol, Sci. Rep., 2019, 9, 1–11 Search PubMed.
- Y. An, C. Meng, Q. Chen and J. Gao, Med. Chem. Res., 2020, 29, 255–261 CrossRef CAS.
- R. Suno, S. Lee, S. Maeda, S. Yasuda, K. Yamashita, K. Hirata, S. Horita, M. S. Tawaramoto, H. Tsujimoto, T. Murata, M. Kinoshita, M. Yamamoto, B. K. Kobilka, N. Vaidehi, S. Iwata and T. Kobayashi, Nat. Chem. Biol., 2018, 14, 1150–1158 CrossRef CAS PubMed.
- T. Joshi, T. Joshi, P. Sharma, S. Chandra and V. Pande, J. Biomol. Struct. Dyn., 2020, 1–18 Search PubMed.
- U. Ryde and P. Söderhjelm, Chem. Rev., 2016, 116, 5520–5566 CrossRef CAS PubMed.
- R. Raynes, J. Brunquell and S. D. Westerheide, Genes Cancer, 2013, 4, 172–182 CrossRef CAS PubMed.
- B. Nutho, P. Mahalapbutr, K. Hengphasatporn, N. C. Pattaranggoon, N. Simanon, Y. Shigeta, S. Hannongbua and T. Rungrotmongkol, Biochemistry, 2020, 59, 1769–1779 CrossRef CAS PubMed.
- J. R. Daddam, B. Sreenivasulu, K. Peddanna and K. Umamahesh, RSC Adv., 2020, 10, 4745–4754 RSC.
Footnote |
† Electronic supplementary information (ESI) available. See DOI: 10.1039/d1ra02233d |
|
This journal is © The Royal Society of Chemistry 2021 |
Click here to see how this site uses Cookies. View our privacy policy here.