DOI:
10.1039/D3RA03518B
(Paper)
RSC Adv., 2023,
13, 24909-24917
Multi-resistant diarrheagenic Escherichia coli identified by FTIR and machine learning: a feasible strategy to improve the group classification
Received
25th May 2023
, Accepted 14th August 2023
First published on 21st August 2023
Abstract
The identification of multidrug-resistant strains from E. coli species responsible for diarrhea in calves still faces many laboratory limitations and is necessary for adequately monitoring the microorganism spread and control. Then, there is a need to develop a screening tool for bacterial strain identification in microbiology laboratories, which must show easy implementation, fast response, and accurate results. The use of FTIR spectroscopy to identify microorganisms has been successfully demonstrated in the literature, including many bacterial strains; here, we explored the FTIR potential for multi-resistant E. coli identification. First, we applied principal component analysis to observe the group formation tendency; the first results showed no clustering tendency with a messy sample score distribution; then, we improved these results by adequately selecting the main principal components which most contribute to group separation. Finally, using machine learning algorithms, a predicting model showed 75% overall accuracy, demonstrating the method's viability as a screaming test for microorganism identification.
Introduction
The introduction of antibiotics into clinical use was the most significant medical advance of the 20th century. The rapid discovery of several classes of antibiotics in a relatively short period has led to the overuse of these drugs, which promotes increasing antimicrobial resistance and antibiotics effectiveness loss.1 The O'Neill report2 predicted that without urgent action, ten million people a year will die from drug-resistant infections by 2050. The WHO World Health Organization3 lists bacterial agents and their respective resistance to antimicrobials to warn the world about the growing global resistance to antimicrobials, divided into critical, high, and medium priority.
Essential strategies have been adopted in this scenario for world public health maintenance, between them the identification of multidrug-resistant bacteria in herds of animals,4 since we have observed the migration of this microorganism to a human–animal–environment interface, such as Acinetobacter baumannii,5,6 Pseudomonas aeruginosa,7,8 Staphylococcus aureus;9 Salmonella spp.,10,11 Campylobacter spp.,12 and Escherichia coli.13–15
Besides, diarrhea in calves is one of the leading causes of economic losses in Brazilian and worldwide livestock. Since the beginning of the 20th century, the worldwide scientific community has pointed to the bacteria E. coli as one of the leading agents involved in diarrhea of infectious origin; therefore, this bacterial genus and its pathogenic strains began to be studied and identified.16–19
The E. coli bacteria is considered a commensal of the microbiota; however, a small part of the strains has pathogenicity responsible for diseases, and the differentiation of pathogenic (diarrheagenic) from non-pathogenic strains is based on the production of virulence factors.17 Once E. coli bacteria with virulence factors spread in the cattle herd, serious concerns arise due to its capability of expressing enzymes that confer resistance to multiple antimicrobials.17,20,21 Animal feces, which have multiresistant strains, serve as constant reservoirs for bacteria dissemination to humans,22,23 the environment, and other animals,24,25 which hinders adequate therapy, increased morbidity, mortality, and causes economic losses to producers and industry.26,27
Phenotypic and molecular methods have been used in bacterial culture to analyze the nucleic acid sequences and identify microorganisms with multidrug resistance characteristics. Still, their variable discriminatory capacity, high cost, and time-consuming experimental routine are difficult the implementation as a standard laboratory procedure.28,29
To overcome such limitations, several studies have been developed by using Fourier Transform Infrared Spectroscopy (FTIR) as a screening test,30,31 which can obtain information about the sample chemical compounds through their vibrational molecular modes, enabling the identification of sample molecular composition (nucleic acids, proteins, lipids, and carbohydrates) of these bacterial cells.32–34
The potential use of FTIR spectroscopy to discriminate, classify and identify microorganisms has been successfully demonstrated in the literature, including many bacterial strains from Gram-positive and Gram-negative species.32,35–41 The specific spectral patterns observed for each molecular group can be revealed with multivariate analysis and/or machine learning algorithms aid, which enable FTIR spectroscopy as an alternative for rapid bacterial identification at the subspecies level.42,43 Despite its great applicability for microorganism identification in recent years, this technique's potential in microbiology routines is still underestimated due to the microbiological community's difficult acceptance and comprehension of the methodology.31
Therefore, we explore using FTIR spectroscopy associated with machine learning algorithms for data analysis to identify and classify multidrug-resistant strains from E. coli species responsible for diarrhea in calves, which may add value to the microbiology laboratory toolbox and society.26,27,31 Our study is focused on developing a simple laboratory routine for sample preparation and data acquisition. Our data analysis aims to improve the method's accuracy for future implementation as a screening tool in bacterial strain identification in microbiology laboratories.
Methods
Sample preparation and FTIR spectra acquisition
A total of 80 Escherichia coli samples were analyzed. The sample set was divided into 40 samples obtained by repetitions from standard E. coli ATCC®. And 40 samples were identified as multidrug-resistant (MR) E. coli isolates. These isolates came from stool and rectal swabs of beef calves aged from one to 60 days. Fecal samples were collected from Cerrado and Pantanal biomes farms in Mato Grosso do Sul, Brazil. The study was conducted at the Veterinary Bacteriology Laboratory at the Universidade Federal de Mato Grosso do Sul (UFMS), under ethical committee approval – from March 2021 to December 2022 – CEUA 1.134/2020. Relevant guidelines and regulations are carried out in all methods.
First, the isolates were screened for analysis and placed in Brain Heart Infusion (BHI) broth for activation, subsequently spread on MacConkey Agar to verify purity and isolate selected colonies. Then, the colonies of MR E. coli and ATCC E. coli were suspended in 1 mL of BHI broth, and a resulting turbid inoculum was adjusted to the 0.5 McFarland scale (108 cfu mL−1) before the measurements.
A small aliquot (30 μL) of the bacterial isolate was carefully deposited onto a flat silicon substrate (SiO2) by casting, followed by drying at 50 °C per 30 minutes. This procedure was repeated three times until the obtention of a thick film. Each sample was produced in duplicate. The samples were analyzed in two different spots from the center to the border to avoid inhomogeneity in the sample composition due to the drying process.44 Finally, the mid-infrared spectra were obtained using an attenuated total reflectance accessory (ATR) at Fourier Transform Infrared Spectrophotometer (Spectrum 100, PerkinElmer). The spectra were collected from 4000 to 700 cm−1, with 4 cm−1 resolution and 10 scans. This entire experimental roadmap is illustrated in Fig. 1.
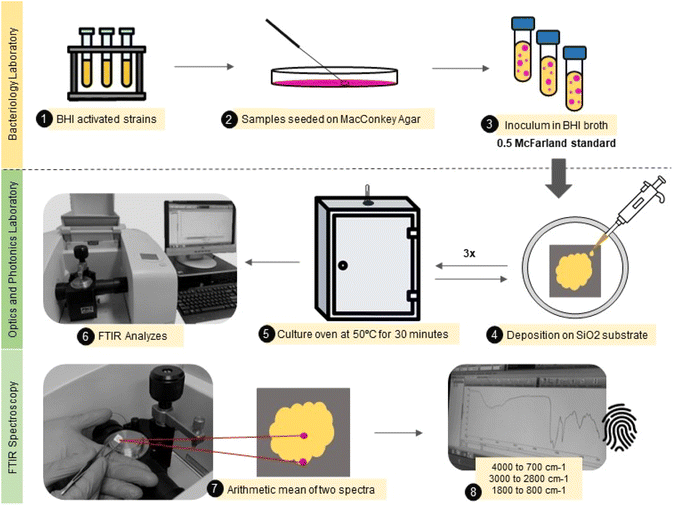 |
| Fig. 1 Illustrative flowchart describing the main steps from the sample (E. coli) preparation to FTIR spectra acquisition. | |
Data analysis and sample classification
The data analysis was performed in Python (version 3.9.12) using the Scikit-learn package (version 1.1.2).45 First, the FTIR spectra from two different spots of duplicate samples were averaged and then subjected to the Standard Normal Variate (SNV) pre-processing method, which removes the variation from the baseline and rescales the spectral intensity to prevent interference in the data analysis due to random experimental variations.46
The average FTIR-SNV spectra for MR E. coli and ATCC E. coli group, in the 4000 to 800 cm−1 range, were submitted to principal component analysis (PCA).47 PCA is an unsupervised method that will project our pre-processed data set into a new dimension (PCs – principal components) which aims to maximize the data variance; this dimensionally reduced data set retains most of the information from the original variables and shows how each data sample is distributed in this new dimension (score plot), allowing cluster the similar samples and distinguish groups. Each PC represents a percentage of the data variance and will enable us to analyze the main spectral range that most contribute to the data variance percentage through the loading plot. PCA is an essential step in visualizing the group classification tendency. Then, the Hotelling T2 test was performed to remove outliers. Here we analyzed three different ranges: (i) 4000 to 800 cm−1; (ii) 3000 to 2800 cm−1, and (iii) 1800 to 800 cm−1, to use only those vibrational modes that improve the group clustering and classification and eliminate highly correlated data.48
The sample classification is performed by prediction models built by machine learning (ML) algorithms using PCs output data from 70% sample set. Before sample classification tests, we must determine the ideal number of PCs used by ML algorithms to avoid overfitting and underfitting.49,50 Here we used the feature selection Recursive Feature Elimination (RFE), which selects the main PCs that most contribute to achieving high accuracy and remove other PCs with the weakest contribution to correct sample classification in ML tests.51 The use or removal of a determined PC was made based on the accuracy achieved using Linear Discriminant Analysis (LDA) to classify the samples in a Leave One Out Cross-Validation (LOOCV) test.
In a brief description, Discriminant Analysis (DA) classifies the sample based on the distance between the sample data and the contour built by using a linear (L) or quadratic (Q) function to separate the classes (group).52 In LOOCV, one sample is taken from the data set, and the others are used to build the prediction model (training). Then, the prediction model accuracy is tested using the sample data withdrawal from the data set. The procedure is repeated until all sample data have been tested.53
After determining the ideal number of PCs and which PCs most contribute to sample classification in each spectral range analyzed, a LOOCV test was performed – by using the respective RFE-PCs data for each range – based on three different methods: (i) DA (described above); (ii) k-Nearest Neighbor (KNN), which uses the Euclidean distance between k closest neighbors to classify the sample;54 and (iii) Support Vector Machine (SVM), which organizes each sample class through the optimization of a hyperplane – the hyperplane can be linear or nonlinear, being optimized to reach high performance – between the classes.55 Finally, we determined the best spectral range, ML algorithm, and PCs to build a predicting model, whose ability for generalization was tested in an external validation test using 30% of the sample set.
Results and discussion
The average FTIR-SNV spectra for E. coli ATCC and MR E. coli samples, Fig. 2, exhibit remarkable similarity between them, with a small standard deviation into the data set. It suggests that direct identification of the isolate is impossible, and the data set shows great coherence. There is a remarkable presence of the three most pronounced bands in the spectra; the first, around 1600 cm−1 is the result of overlapped bands 1639 and 1583 cm−1, assigned to amides I and II from proteins, which is relatively wide, suggesting the presence of a third band, mainly due to the small shoulder observed around 1500 cm−1. The vibrational bands assigned to amides I (N–H and C
O) and II (N–H and C–N) have shown significant contributions to biological sample classification in the literature.56,57 The second band, around 1400 cm−1, can be assigned to C–H and C
O vibrational modes from lipids and proteins, while the third band, around 1080 cm−1, is usually assigned to nucleic acid and phospholipids. Also, weak bands assigned to C–H and C–H–O vibrational modes from carbohydrate below 1000 cm−1 was identified in the spectra.58,59
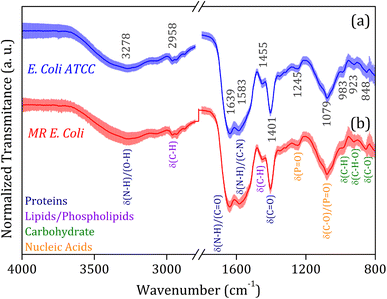 |
| Fig. 2 Average FTIR-SNV spectra for (a) E. coli ATCC (blue line) and (b) multidrug-resistant (MR) E. coli (red line) isolates. The main vibrational assignments are indicated in detail, and their related molecular groups (proteins, lipids, carbohydrates, and fatty acids) are identified by colors. The symbol δ indicates the deformation vibrations. | |
Fig. 3 shows the principal component analysis results for the FTIR-SNV spectral data from E. coli ATCC and MR E. coli sample groups. On the left side, we can observe the score plot, and on the right side, the loading plot for (i) 4000 to 800 cm−1; (ii) 3000 to 2800 cm−1, and (iii) 1800 to 800 cm−1 range. For all cases analyzed, two main characteristics stand out, the score plot exhibits a single cluster for both groups, which hinders the future group classification, and the loading shows that the main contribution for the data variance comes from the respective vibrational bands identified in the FTIR spectra. We found PC1, PC2, and PC3 responsible for 79.3%, 89.7%, and 81.7% of data variance at 4000 to 800 cm−1, 3000 to 2800 cm−1, and 1800 to 800 cm−1 ranges, respectively; besides this great contribution for data variance, previous studies have shown that the first PCs can be ignored, and high order PCs can be used to improve the group classification for ML algorithms.60
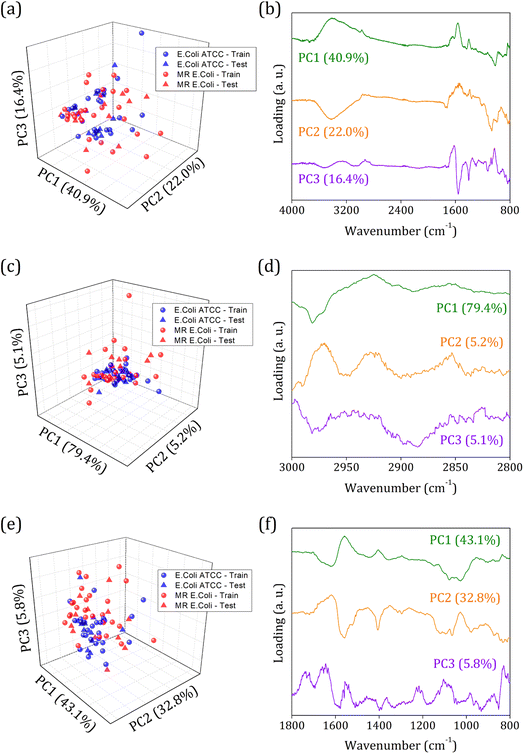 |
| Fig. 3 Principal component analysis for E. coli ATCC (blue circles/stars) and multidrug-resistant (MR) E. coli (red circles/stars) isolates average FTIR-SNV spectra. The score plot and respective loading for the three different ranges were analyzed: (a and b) 4000 to 800 cm−1; (c and d) 3000 to 2800 cm−1; and (e and f) 1800 to 800 cm−1. The circles represent 70% of the sample data set used to build the predicting model, and the stars represent 30% of the sample data set used for external validation. | |
Usually, the proper choice of spectral range helps to improve group classification and clustering formation61 since we use only spectral information that most contributes to clustering instead of those with highly correlated data, which hinders cluster formation. But here, we couldn't succeed with this strategy probably because of the high similarities between the groups involved and the highly correlated data present. The presence of antibiotic resistance genes in the MR E. coli group was mainly identified as resulting of the ESBL enzyme – previous study – which is responsible for amine beta-lactam ring hydrolysis, resulting in antibiotics inactivation on the bacterial cell walls. Since it represents a break in the C–N bond (amide group) and the appearance of N–H and O–H bonding, almost no difference in the IR spectra will be observed. Because the C–N and N–H vibrational modes are superimposed around 1580 cm−1, and the O–H vibrational mode, around 3278 cm−1, is too wide to provide enough information for group differentiation.
Then, an alternative to improve group clustering and separation is using feature selection Recursive Feature Elimination (RFE) to find the best PCs (data projection) that most contribute to data separation. The RFE can select PCs that most contribute to achieving high accuracy and remove those with a small contribution. Here, the main PCs were determined by the overall accuracy achieved in a Leave One Out Cross-Validation (LOOCV) test using Linear Discriminant Analysis (LDA).60 The main PCs found for our data were: PC1 and PC2 for the 4000 to 800 cm−1 range, responsible for 62.83% of data variance; 49 first PCs for the 3000 to 2800 cm−1 range, responsible for 99.9% of data variance; and PC3, PC4, PC9, PC18, PC23, and PC36 for the 1800 to 800 cm−1 range responsible for 11.30% of data variance.
Fig. 4 shows the violin plot for the six most relevant PCs, in the 1800 to 800 cm−1 range, with a prevalence of a monomodal distribution for the score data project over its respective PC for both sample groups. A bimodal distribution can also be observed for the ATCC group at PC3 and MR group at PC18 and PC23. But the more important characteristic to be observed is the median value (dashed thick line around the peak center), which assumes a better distinct position projection over the axis for these PCs compared to the others and improves the clustering and group classification of our data in the LOOCV test with LDA.
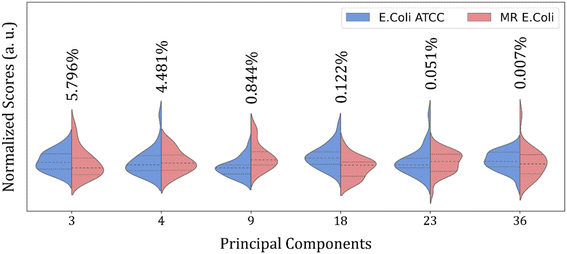 |
| Fig. 4 Violin plot for nine main PCs normalized scores selected by RFE in 1800 to 800 cm−1 range from E. coli ATCC® 25922 (blue) and multidrug-resistant (MR) E. coli (red) isolates. The thick dashed line represents the data distribution median. The data variance percentage of each PC is described above each plot. | |
Fig. 5 shows the score plot and loading for RFE-PCs: PC3, PC4, and PC9, which did not improve the clustering formation and were responsible for only 11.1% of the data variance. Besides that, the loading plot for each PC, Fig. 5 (right column), shows a contribution for data variance in the 1800 to 800 cm−1 range in accordance with the main bands identified in the FTIR-SNV spectra (Fig. 2) assigned to protein and phospholipids molecules. In this case, with remarkable similarity among the samples, then between the spectra – high data correlation – the first PCs can be responsible for a significant percentage of data variance, with a small contribution to group separation. However, low data variance PCs can still be helpful for group classification and better influence the algorithm performance, as demonstrated in the literature.60
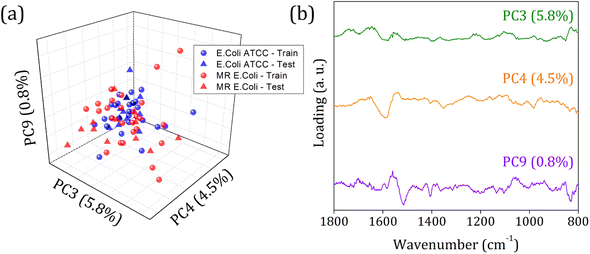 |
| Fig. 5 (a) Score plot clustering improvement due to PCs selection by RFE, and (b) loading for PC4, PC17, and PC28 from E. coli ATCC® 25922 (blue square), and (b) multidrug-resistant (MR) E. coli (red circles) isolates. | |
The RFE-PCs were submitted to machine learning algorithms (DA, SVM, and KNN) to build a prediction model for sample classification; the overall accuracy achieved for each model by using different functions for classification in the LOOCV test is summarized in Fig. 6(a). The maximum overall accuracy achieved by the predicting models was 88.5% for linear SVM using 6 PCs – responsible for 11.30% of data variance – within the 1800 to 800 cm−1 range. An external validation test was performed using 30% of the sample set, Fig. 6(c), achieving an overall accuracy of 75%, demonstrating a good generalization capacity of the prediction model. A slight deviation in the accuracy percentage between LOOCV and the external validation test is expected.
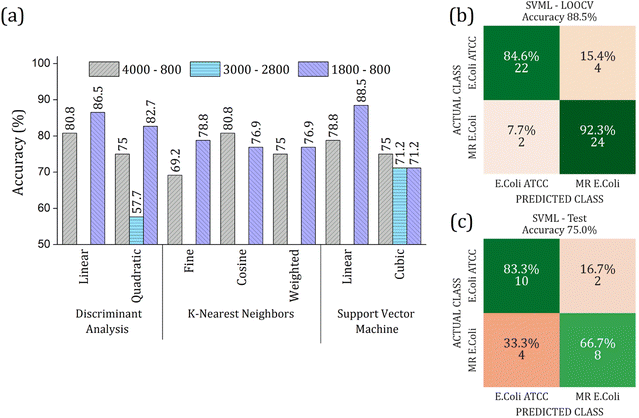 |
| Fig. 6 (a) Overall accuracy obtained in the LOOCV tests for Discriminant Analysis (DA), K-Nearest Neighbor (KNN), and Support Vector Machine (SVM) algorithms. Different ranges were analyzed with respective RFE-PCs: (i) 4000 to 800 cm−1 (gray bar color with right inclined line pattern) using 2 PCs; (ii) 3000 to 2800 cm−1 (light blue color with horizontal line pattern) by using 49 PCs; (iii) 1800 to 800 cm−1 (purple color with left inclined line pattern) by using 6 PCs. The highest overall accuracy was 88.5% for linear SVM at 1800 to 800 cm−1 range. Confusion matrix for the performance of linear SVM algorithm in 1800 to 800 cm−1 range using 6 PCs (b) LOOCV test with 70% data set, and (c) external validation test with 30% data set. The input PCA data from average FTIR-SNV spectra of E. coli ATCC® 25922 and multidrug-resistant (MR) E. coli isolates. | |
The real contribution of the RFE-PCs in the improvement of the overall accuracy in the classification test was demonstrated by using the PC1, PC2, and PC3, Fig. 7. Here, Fig. 7(a), we obtained a maximum overall accuracy of 80.8% in the LOOCV test for linear DA and linear SVM at 4000–800 cm−1 range, Fig. 7(b). The drop in the overall accuracy value was in the same order as before, exhibiting 66.7%. As can be observed in Fig. 6 and 7, the prediction model fails more to identify E. coli ATCC samples, which is less problematic for a trial method considering the need for large-scale tests to make a fast decision in livestock management.
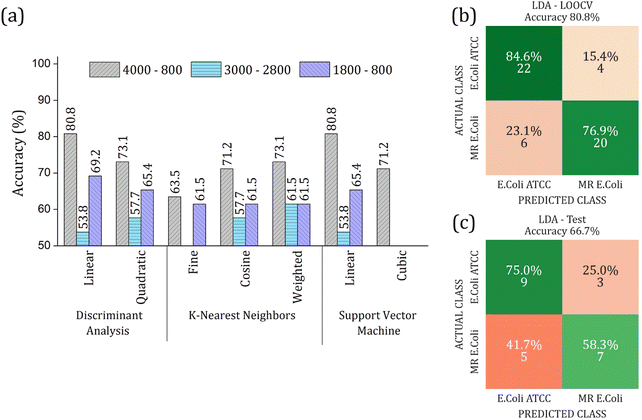 |
| Fig. 7 (a) Overall accuracy obtained in the LOOCV tests for Discriminant Analysis (DA), K-Nearest Neighbor (KNN), and Support Vector Machine (SVM) algorithms. Different ranges were analyzed by using PC1, PC2, and PC3: (i) 4000 to 800 cm−1 (gray bar color with right inclined line pattern); (ii) 3000 to 2800 cm−1 (light blue color with horizontal line pattern); (iii) 1800 to 800 cm−1 (purple color with left inclined line pattern). The highest overall accuracy was 80.8% for linear DA and linear SVM at 4000 to 800 cm−1 range. Confusion matrix for the performance of linear DA algorithm in 4000 to 800 cm−1 range using 3 PCs (b) LOOCV test with 70% data set, and (c) external validation test with 30% data set. The input PCA data from average FTIR-SNV spectra of E. coli ATCC® 25922 and multidrug-resistant (MR) E. coli isolates. | |
The current study presented an easy and suitable methodology for identifying MR-E. Coli bacteria by FTIR spectroscopy and machine learning algorithms, but we must remember that the methods and results can still be improved. Hence, the possibility for new studies is open. Here, we discussed the FTIR limitations to evidenced spectral changes due role played by ESBL enzyme to create MR bacteria, so new photonic techniques must be explored for better data acquisition. Our study explores a promising route to build a prediction model with good performance in the external validation test. However, we can still search for the best hyperparameters for the algorithm or even explore new pre-processing data methods and algorithms to build the prediction model and improve its generalization capacity in the external validation test.
Conclusions
In 1800 to 800 cm−1 range, the FTIR spectra of multi-resistant diarrheagenic Escherichia coli isolates obtained from calves' feces showed great potential for microorganism identification. The usual principal component analysis could not provide a promising clustering formation for future sample classification. Then, we applied the feature selection Recursive Feature Elimination (RFE) algorithm, from which the most important PCs were chosen before being used in machine learning algorithms. The score plot of PC3 × PC4 × PC9 clearly demonstrated the improvement of clustering tendency and group separation; here, 6 PCs were selected by RFE and used to build a prediction model using linear SVM. The prediction model showed an 88.5% overall accuracy in the LOOCV test and achieved 75% overall accuracy, with 66% sensitivity and 83% specificity in the external validation test.
Data availability
All authors agree to share the supplementary files on the reasonable request received by the corresponding author. The data sets used and analyzed during the current study are also available from the corresponding author upon reasonable request.
Conflicts of interest
Thera are no conflicts to declare.
Acknowledgements
The present study was carried out with the support of the Universidade Federal de Mato Grosso do Sul – UFMS/MEC, Brazil and Fundação Oswaldo Cruz – FIOCRUZ-RJ. Coordenação de Aperfeiçoamento de Pessoal de Nível Superior (CAPES), code 001. Conselho Nacional de Desenvolvimento Científico e Tecnológico (CNPQ), code 403651/2020-5; 302525/2022-0; 440214/2021-1. Fundação de Apoio ao Desenvolvimento do Ensino, Ciência e Tecnologia do Estado de Mato Grosso do Sul (FUNDECT), code 007/2019; 360/2022.
References
- L. Katz and R. H. Baltz, Natural product discovery: past, present, and future, J. Ind. Microbiol. Biotechnol., 2016, 43, 155–176 CrossRef CAS PubMed.
- J. O’Neill, Tackling drug-resistant infections globally: final report and recommendations, Review on Antimicrobial Resistance, Wellcome Trust and HM Government, disponível em, https://amrreview.org/sites/default/files/160525_Final_paper_with_cover.pdf, 2016 Search PubMed.
- WHO World Health Organization, Global Action Plan on Antimicrobial Resistance, World Health Organization, Geneva, Switzerland, 2017 Search PubMed.
- R. Han, et al., Elimination kinetics of ceftiofur hydrochloride in milk after an 8-day extended intramammary administration in healthy and infected cows, PLoS One, 2017, 12(11), e0187261 CrossRef PubMed.
- C. Pomba, et al., First Report of OXA-23-Mediated Carbapenem Resistance in Sequence Type 2 Multidrug-Resistant Acinetobacter baumannii Associated with Urinary Tract Infection in a Cat, Antimicrob. Agents Chemother., 2015, 58(2), 1267–1268 CrossRef PubMed.
- C. Ewers, et al., OXA-23 and ISAba1–OXA-66 class D β-lactamases in Acinetobacter baumannii isolates from companion animals, Int. J. Antimicrob. Agents, 2017, 49(1), 37–44 CrossRef CAS PubMed.
- M. R. Fernandes, et al., Zooanthroponotic Transmission of Drug-Resistant Pseudomonas aeruginosa, Brazil, Emerg. Infect. Dis., 2018, 24(6), 1160–1162 CrossRef CAS PubMed.
- E. A. Elshafiee, et al., Carbapenem-resistent Pseudomonas aeruginosa originating from farm animals and people in Egypt, J. Vet. Res., 2019, 63, 333–337 CrossRef CAS PubMed.
- C. J. B. Oliveira, et al., Methicillin-resistant Staphylococcus aureus from Brazilian dairy farms and identification of novel sequence types, Zoonoses Public Health, 2016, 63, 97–105 CrossRef CAS PubMed.
- L. Colobatiu, et al., First description of plasmid-mediated quinolone resistance determinants and beta-lactamase encoding genes in non-typhoidal Salmonella isolated from humans, one companion animal and food in Romania, Gut Pathog., 2015, 16(7), 2–11 Search PubMed.
- B. R. Pribul, et al., Characteristics of quinolone resistance in Salmonella spp. Isolates from the food chain in Brazil, Front. Microbiol., 2017, 8, 299 Search PubMed.
- B. Frasao and S. Da, et al., Detection of fluoroquinolone resistance by mutation in gyrA gene of Campylobacter spp. Isolates from broiler and laying (Gallus gallus domesticus) hens, from Rio de Janeiro State, Brazil, Cienc. Rural, 2015, 45(11), 2013–2018 CrossRef.
- L. C. Melo, et al., Prevalence and molecular features of ESBL/pAmpC-producing Enterobacteriaceae in healthy and disease companion animals in Brazil, Vet. Microbiol., 2018, 221, 59–66 CrossRef CAS PubMed.
- M. C. Brisola, et al., Escherichia coli used as a biomarker of antimicrobial resistance in pig farms of Southern Brazil, Sci. Total Environ., 2019, 647, 362–368 CrossRef CAS PubMed.
- J. D. Palmeira, et al., Epidemic spread of Incl1/Pst113 plasmid carrying the Extended-Spectrum Beta-Lactamase (ESBL) blaCTX-M-8 gene in Escherichia coli of Brazilian catte, Vet. Microbiol., 2020, 243, 108629 CrossRef CAS PubMed.
- T. Smith and M. L. Orcutt, The bacteriology of the intestinal tract of young calves with special reference to the early diarrhea (scours), J. Exp. Med., 1925, 41, 89–106 CrossRef CAS PubMed.
- F. M. Coura, Longitudinal study of Salmonella spp., diarrheagenic Escherichia coli, Rotavirus, and Coronavirus isolated from healthy and diarrheic calves in a Brazilian dairy herd, Trop. Anim. Health Prod., 2015, 47, 3–11 CrossRef PubMed.
- L. B. Cruvinel, et al., Surtos consecutivos ocasionados por Eimeria zuernii em bezerros de corte de uma propriedade do estado de São Paulo, Pesqui. Vet. Bras., 2018, 38(2), 277–284 CrossRef.
- J. F. Tutija, et al., Molecular and phenotypic characterization of Escherichia coli from calves in an important meat-producing region in Brazil, J. Infect. Dev. Countries, 2022, 16(6), 1030–1036 CrossRef CAS PubMed.
- P. C. Blanchard, Diagnostics of dairy and beef cattle diarrhea, Vet. Clin. North Am. Food Anim., 2012, 28, 443–464 CrossRef PubMed.
- L. Poirel, et al., Antimicrobial resistance in Escherichia coli, Microbiol. Spectr., 2018, 6(4), 1–27 CAS.
- A. Carattoli, Animal reservoirs for extended spectrum beta-lactamase producers, Clin. Microbiol. Infect., 2008, 14(Suppl 1), 117–123 CrossRef PubMed.
- A. Schmid, S. Hörmansdorfer and U. Messelhäusser, Prevalence of extended-spectrum β-lactamase-producing Escherichia coli on Bavarian dairy and beef cattle farms, Appl. Environ. Microbiol., 2013, 79(9), 3027–3032 CrossRef CAS PubMed.
- A. Boonyasiri, et al., Prevalence of antibiotic resistant bacteria in healthy adults, foods, food animals,
and the environment in selected areas in Thailand, Pathog. Global Health, 2014, 108, 235–245 CrossRef PubMed.
- L. Tekiner and H. Ozpinar, Occurrence and characteristics of extended spectrum beta-lactamases-producing Enterobacteriaceae from foods of animal origin, Braz. J. Microbiol., 2016, 47, 444–451 CrossRef PubMed.
- S. Santajit and N. Indrawattana, Mechanisms of Antimicrobial Resistance in ESKAPE Pathogens, BioMed Res. Int., 2016, 2016, 1–9 CrossRef PubMed.
- Y. Chong, S. Shimoda and N. Shimono, Current epidemiology, genetic evolution, and clinical impact of extended-spectrum β-lactamase-producing Escherichia coli and Klebsiella pneumoniae, Infect., Genet. Evol., 2018, 61, 185–188 CrossRef PubMed.
- V. Jarlier, et al., Extended broad-spectrum beta-lactamases conferring transferable resistance to newer beta-lactam agents in Enterobacteriaceae: hospital prevalence and susceptibility patterns, Rev. Infect. Dis., 1988, 10, 867–878 CrossRef CAS PubMed.
- A. J. Sabat, A. Budimir and D. Nashev, et al., Overview of molecular typing methods for outbreak detection and epidemiological surveillance, Eurosurveillance, 2013, 18(4), 20380 CrossRef CAS PubMed.
- K. Maquelin, et al., Identification of medically relevant microorganisms by vibrational spectroscopy, J. Microbiol. Methods, 2002, 51, 255–271 CrossRef CAS PubMed.
- A. Novais, A. R. Freitas and C. Rodrigues, et al., Fourier transform infrared spectroscopy: unlocking fundamentals and prospects for bacterial strain typing, Eur. J. Clin. Microbiol. Infect. Dis., 2019, 38(3), 427–448 CrossRef PubMed.
- D. Helm, H. Labischinski and G. Schallehn, et al., Classification and identification of bacteria by Fourier-transform infrared spectroscopy, J. Gen. Microbiol., 1991, 137, 69–79 CAS.
- F. A. Settle, Handbook of Instrumental Techniques for Analytical Chemistry, Prentice Hall, New Jersey, USA, 1997 Search PubMed.
- B. Stuart, Infrared Spectroscopy: Fundamentals and Applications, John Wiley & Sons, West Sussex, England, 2004 Search PubMed.
- L. Mariey, et al., Discrimination, classification, identification of microorganisms using FTIR spectroscopy and chemometrics, Vib. Spectrosc., 2001, 26(2), 151–159 CrossRef CAS.
- L. Beutin, Q. Wang and D. Naumann, et al., Relationship between O-antigen subtypes, bacterial surface structures and O-antigen gene clusters in Escherichia coli O123 strains carrying genes for Shiga toxins and intimin, J. Med. Microbiol., 2007, 56, 177–184 CrossRef CAS PubMed.
- C. Colabella, et al., Merging FTIR and NGS for simultaneous phenotypic and genotypic identification of pathogenic Candida species, PLoS One, 2017, 12(12), e0188104 CrossRef PubMed.
- L. Potocki, et al., FTIR and Raman Spectroscopy-Based Biochemical Profiling Reflects Genomic Diversity of Clinical Candida Isolates That May Be Useful for Diagnosis and Targeted Therapy of Candidiasis, Int. J. Mol. Sci., 2019, 20, 988 CrossRef CAS PubMed.
- R. G. Rustam, et al., Discrimination of Staphylococcus aureus Strains from Coagulase-Negative Staphylococci and Other Pathogens by Fourier Transform Infrared Spectroscopy, Anal. Chem., 2020, 92(7), 4943–4948 CrossRef PubMed.
- S. Pebotuwa, et al., Influence of the Sample Preparation Method in Discriminating Candida spp. Using ATR-FTIR Spectroscopy, Molecules, 2020, 25, 1551 CrossRef CAS PubMed.
- M. Cordovana, et al., Classification of Salmonella enterica of the (Para-)Typhoid Fever Group by Fourier-Transform Infrared (FTIR) Spectroscopy, Microorganisms, 2021, 9, 853 CrossRef PubMed.
- R. A. Meyers, Encyclopedia of analytical chemistry (applications, theory, and instrumentation), Infrared Spectroscopy in Microbiology, 2006, pp. 1–32 Search PubMed.
- H. Shi, et al., The strategy for correcting interference from water in Fourier transform infrared spectrum based bacterial typing, Talanta, 2020, 208, 120347 CrossRef CAS PubMed.
- J. M. Cameron, et al., Biofluid spectroscopic disease diagnostics: a review on the processes and spectral impact of drying, J. Biophot., 2018, 11, e201700299 CrossRef PubMed.
- F. Pedregosa, et al., Scikit-learn: machine learning in Python, J. Mach. Learn. Res., 2011, 12, 2825–2830 Search PubMed.
- Å. Rinnan, F. Van Den Berg and S. B. Engelsen, Review of the most common pre-processing techniques for near-infrared spectra, TrAC, Trends Anal. Chem., 2009, 28(10), 1201–1222 CrossRef.
- I. T. Jolliffe and J. Cadima, Principal component analysis: a review and recent developments, Philos. Trans. R. Soc., A, 2016, 374(2065), 20150202 CrossRef PubMed.
- A. E. Casaril, Intraspecific differentiation of sandflies specimens by optical spectroscopy and multivariate analysis, J. Biophotonics, 2021, 14, e202000412 CrossRef CAS PubMed.
- T. R. Gomes, FTIR spectroscopy with machine learning: a new approach to animal DNA polymorphism screening, Spectrochim. Acta, Part A, 2021, 261, 120036 CrossRef PubMed.
- N. H. Hasbi, et al., Pattern recognition for human diseases classification in spectral analysis, Computation, 2022, 10, 96 CrossRef.
- P. Theerthagiri, Predictive analysis of cardiovascular disease using gradient boosting-based learning and recursive feature elimination technique, Intell. Syst. Appl., 2022, 16, 200121 Search PubMed.
- W. Wu, et al., Comparison of regularized discriminant analysis linear discriminant analysis and quadratic discriminant analysis applied to NIR data, Anal. Chim. Acta, 1996, 329(3), 257–265 CrossRef CAS.
- T. Wong, Performance evaluation of classification algorithms by k-fold and leave-one-out cross-validation, Pattern Recognit., 2015, 48(9), 2839–2846 CrossRef.
- A. Mucherino, P. J. Papajorgji and P. M. Pardalos, K-nearest neighbor classification, in Data mining in Agriculture, Springer, New York, NY, 2009, pp. 83–106 Search PubMed.
- W. S. Noble, What is a support vector machine?, Nat. Biotechnol., 2006, 24(12), 1565–1567 CrossRef CAS PubMed.
- G. Larios, et al., A new strategy for canine visceral leishmaniasis diagnosis based on spectroscopy and machine learning, J. Biophot., 2021, 11, e202100141 Search PubMed.
- E. C. A. Brito, Paracoccidioidomycosis screening diagnosis by FTIR spectroscopy and multivariate analysis, Photodiagn. Photodyn. Ther., 2022, 39, 102921 CrossRef PubMed.
- J. M. Legal, Applications of FTIR spectroscopy in structural studies of cells and bacteria, J. Mol. Struct., 1991, 242, 397–407 CrossRef CAS.
- L. Mariety, Discrimination, classification, identification of microorganisms using FTIR spectroscopy and chemometrics, Vib. Spectrosc., 2021, 26, 151–159 CrossRef.
- M. L. Coelho, et al., Canine visceral leishmaniasis diagnosis by UV spectroscopy of blood serum and machine learning algorithms, Photodiagn. Photodyn. Ther., 2023, 42, 103575 CrossRef CAS PubMed.
- T. Franca, D. Gonçalves and C. Cena, ATR-FTIR spectroscopy combined with machine learning for classification of PVA/PVP blends in low concentration, Vib. Spectrosc., 2022, 120, 103378 CrossRef CAS.
|
This journal is © The Royal Society of Chemistry 2023 |
Click here to see how this site uses Cookies. View our privacy policy here.