DOI:
10.1039/C7LC00815E
(Critical Review)
Lab Chip, 2018,
18, 249-270
Recent advances in microfluidic technologies for cell-to-cell interaction studies
Received
2nd August 2017
, Accepted 30th October 2017
First published on 31st October 2017
Abstract
Microfluidic cell cultures are ideally positioned to become the next generation of in vitro diagnostic tools for biomedical research, where key biological processes such as cell signalling and dynamic cell-to-cell interactions can be reliably analysed under reproducible physiological cell culture conditions. In the last decade, a large number of microfluidic cell analysis systems have been developed for a variety of applications including drug target optimization, drug screening and toxicological testing. More recently, advanced in vitro microfluidic cell culture systems have emerged that are capable of replicating the complex three-dimensional architectures of tissues and organs and thus represent valid biological models for investigating the mechanism and function of human tissue structures, as well as studying the onset and progression of diseases such as cancer. In this review, we present the most important developments in single-cell, 2D and 3D microfluidic cell culture systems for studying cell-to-cell interactions published over the last 6 years, with a focus on cancer research and immunotherapy, vascular models and neuroscience. In addition, the current technological development of microdevices with more advanced physiological cell microenvironments that integrate multiple organ models, namely, the so-called body-, human- and multi-organ-on-a-chip, is reviewed.
Introduction
Interactions between cells are an essential feature of multicellular organisms and are crucial for development and physiological functioning on the tissue level. Cell-to-cell interactions occur directly, such as at stable cell–cell junctions that organize cell layers in tissue, or indirectly, as when cells communicate by secreting signalling molecules. Interactions take place between cells of the same phenotype as well as between cells of different phenotypes. It is of great importance to understand the phenomenon of cellular interactions in order to obtain information about several biological functions including the development and migration of cancer, wound healing and stem cell development.1,2 This information can be translated into applications such as drug screening and tissue engineering. To establish assays for studies of cell-to-cell interactions, co-culture of two or more cell types is conducted. The standard method of co-culture comprises the direct addition of different cell types to the same culture well or, for example, the culture of cells in Transwell® systems, which consist of two compartments separated by a permeable membrane within each well. Although the culture of cells on flat surfaces is favoured owing to the simplicity of the approach, the models are based on 2D monolayers of cells that differ from cells in vivo in qualities such as cell morphology, physiology, and gene expression. The microenvironment of most cells in living tissue is the extracellular matrix (ECM), which is an environment with a complex molecular composition and fibrous nature that provides structural support and thereby allows cells to grow three-dimensionally.3,4 In approaches used to mimic the ECM in vitro, cells are cultured in 3D gels or matrices. However, it is challenging to create a well-controlled microenvironment with dimensions that correspond to tissue structures in vivo, and thus the cellular morphogenesis will still differ from that in native tissues.5 To develop more physiologically relevant co-culture cell models, microfluidic and organ-on-a-chip systems are used as advanced tools for studies of cell–cell interactions.
For over two decades, the application of micromachining technologies for biomedical research has led to the development of miniaturized assays for advanced in vitro cell analysis in so-called cell-based microfluidic platforms. To date, a variety of fabrication methods have been utilized for microfluidic systems, including etching techniques, photo- and e-beam lithography, embossing, replica moulding, and laser photoablation, as well as 3D printing as an additive manufacturing technique, owing to its recent affordability.6,7 The selection of the correct fabrication method is mostly determined by the existing infrastructure (technology and equipment), fabrication speed, desired resolution, and fabrication material. With fabrication techniques that originated from the microelectronics industry, early devices consisted of glass-, silicon- and photopolymer-based microfluidics. In particular, glass was a favoured material owing to its compatibility with biomedical applications. However, the micromachining of glass and silicon wafers involves the use of cost-intensive techniques and requires clean room infrastructure.8,9 Therefore, a diverse set of rapid and cost-efficient fabrication techniques for microfluidics that are applicable to various materials, such as hydrogels, thermosets and thermoplastics, are increasing in popularity.10–15 With the emergence of inexpensive soft lithography as a technique for molding microchannels, the cost, as well as the availability, of microfabricated devices has improved.16 Since then, microfluidic devices have provided powerful tools for biological and chemical studies and are spreading rapidly.17–19 Integrated systems that combine microchannels with pumps, valves, filters and sensors are referred to as ‘lab-on-a-chip’ (LOC) or ‘micro total analysis systems’ (μTAS).20 The introduction of microfluidics into the life sciences has enabled crucial limitations of standard assay formats to be addressed, including temperature control, gas control, precise control over geometry, nutrient supply, waste removal, chemical administration, and assay parallelization, as well as cell culture automation. Initially, cell-based microfluidics were developed for counting and analysing cells in miniaturized flow cytometers, which are systems that are commercially available today.21 Microfluidic devices for cellular studies have also been devised to investigate the transport and cultivation of cells in the absence and presence of concentration and temperature gradients or shear force conditions.22 In summary, microfluidic systems have been used to perform cell sampling, cell trapping, sorting, patterning, capture, drug administration and multi-parameter cell analysis.23–26 Microfluidic systems can provide defined and reproducible simulation scenarios that enable the reliable investigation of cell behaviour in an environment that mimics mechanical forces within living tissues. In particular, mechanical strain, which represents an important factor that can trigger stem cell differentiation in vitro,27–31 can be effectively achieved by using elaborate microscale systems with various techniques.32–35 However, in the human body individual cell types are spatially arranged in three dimensions with high precision and constantly interact with and respond to adjacent cells. Because dynamic, yet controlled, cell-to-cell interactions play a key role in the maintenance of tissue function, regeneration and repair, co-culture systems have been established as an indispensable tool for investigating the dynamic interplay between homotypic, as well as heterotypic, cell populations. Therefore, microfluidics underwent a transition from 2D monolayer cell culture to 3D cell culture and thereby advanced the technology to provide more physiologically relevant in vitro models, including bioengineering methods such as cell-laden scaffolds and tissue spheroids on-chip. A further trend comprises the integration of complex co-cultures rather than single cell populations. For instance, co-culture systems can foster cell-to-cell interactions to improve the function and regeneration and differentiation capacity of cells and the activation of immune cells.36–40
In this review, we report on the latest progress in microfluidic devices developed to study interactions between heterotypic cell populations, with the exception of neurobiology, where homotypic interactions between single neurons are also described. A more comprehensive review that describes homo- and heterotypic cell–cell interactions on-chip can be found elsewhere.41 We begin by introducing 2D models for the investigation of the development and progression of cancer and proceed to more complex 3D models for the study of cancer biology and drug testing, neurobiology, and engineering of vascular models. Furthermore, we briefly review recent devices for analysing cell–cell interactions at the single-cell level. In the last section, we examine recent advances in multi-organ-on-a-chip and body-/human-on-a-chip systems with respect to the interactions of cell co-cultures. The reviewed literature was strictly selected using the criteria of recency (since 2010) and significance with respect to cell-to-cell interaction and cellular crosstalk (>1 cell type).
1. Microfluidic models for cancer biology, cancer immunology and cancer therapy
The immune system functions as a regulatory authority and is responsible for the maintenance of stable human physiology. The study of immunology using in vitro models has so far provided the opportunity to obtain more insight into the complex processes of immune response and many immune-related diseases. Microfluidic systems have been used as miniaturized in vivo-like physiological models that mimic cell-to-cell interactions and simulate the human metabolism in health as well as disease. In particular, two main fields for the application of such microsystems are immunology and cancer research. Even though the immune response during inflammation and allergic reactions is of great interest and therefore has been extensively analysed using microfluidic devices, the application of microfluidics in this field mainly focuses on the responses of immune cell populations to soluble pro- and anti-inflammatory factors rather than actual cell-to-cell interaction studies. Therefore, more detailed information on such microfluidic models for studies of immune-mediated cell motility and allergy-on-a-chip can be found elsewhere and is not reviewed here.42 However, cancer biology encompasses a broad range of research disciplines that share a common goal in the establishment of tumour tissue models with greater in vivo relevance for improvements in drug development, lead optimization studies and screening efforts. An important aspect of microfluidic tumour research is concerned with understanding cell-to-cell interactions between tumour cells and various types of target cell, including stromal, endothelial and immune cells, under physiologically relevant conditions.2,38,43 Over the years, a variety of advanced microfluidic in vitro tumour models have been established for (i) the two-dimensional cultivation of tumour cells and (ii) the formation, as well as cultivation, of three-dimensional tumour structures (e.g., cell-laden hydrogels or spheroids).5,44–47 More recently, the immunology of cancer has gained momentum owing to the emergence of cell-based immunotherapy as a promising complementary strategy for anticancer treatment. In the following subsections, the bioengineering of cancer microenvironments on-chip, as well as recent applications of novel microfluidic cancer models for the study of immune and cancer cell crosstalk, as well as chemotherapy and immunotherapy, is discussed in more detail.
1.1 Recent advances in two-dimensional models of cell migration for cancer research
The application of two-dimensional microfluidic cell culture systems that are capable of monitoring the interactions of stromal cells with tumour cells is of particular importance in understanding the development and progression of cancer. To enable microfluidics to act as a promising tool for such studies, much research has focused on the compartmentalization of co-cultures in the form of separate microfluidic chambers, as well as cellular patterns. To gain a deeper understanding of the site-directed migration of cancer cells, Ma et al. developed a microfluidic device that promotes indirect cell-to-cell interactions.48 As shown in Fig. 1A, an interconnected network of microfluidic channels enables the cultivation of various co-culture systems for the study of, for example, the interactions of human embryonic lung fibroblasts (HFL-1) with either carcinoma cells (HepG2, ACC-M and ACC-2) or normal epithelial cells (GES-1). The results of this study revealed that the site-directed migration and transdifferentiation of embryonic fibroblasts exclusively takes place in the presence of carcinoma cells. Similar microdevices based on interconnected cell culture chambers have also successfully been employed in a variety of cancer cell migration assays and motility studies, as well as chemotherapy screening applications; however, the basic functions of the biochip remain similar.49–51 To produce spatially resolved cell culture compartments that separate cancer cells from stromal cells in a more refined approach, Menon et al. integrated anisotropic cell culture surfaces on the basis of the tuning of surface wettability to study the induction of reactive oxygen species (ROS) in stromal cells (Fig. 1B).2 With this technology, it is possible to control the time point at which cell co-culture is initiated by simply injecting media through the hydrophobic central compartment that interconnects the lateral chambers and commencing both direct and indirect cell-to-cell interactions and cell migration. The authors demonstrated that during the co-culture of HS5 bone marrow stromal cells and HuH7 liver tumour cells within the microfluidic device the generation of ROS was increased fourfold, which led to the ROS-induced death of stromal cells. At day 9 of on-chip co-culture, the transformation of highly aggressive metastatic HuH7 tumour cells was observed, which indicated that the ROS concentration influenced the rate of tumour invasion and progression.52 Another two-dimensional co-culture system was recently established to investigate the interactions of tumour cells with immune cells (Fig. 1C).43,53,54 In this study, protein micropatterning based on a laminar flow patterning technique was used to establish an anisotropic nanobiointerface that enabled guided cell adhesion and direct cell-to-cell contact between adherent anchorage-dependent cell types and surface-activated immune cells. As an application scenario, Liu et al. established a microfluidic co-culture model for anticancer drug screening of different chemotherapeutic agents by mimicking a bladder cancer microenvironment.55 This microfluidic device consisted of four cell culture chambers separated by hydrogel barriers to allow the diffusion of nutrients and soluble factors (Fig. 1D). The authors showed that the interaction of cancer cells with stromal and immune cells displayed a close analogy to the in vivo pathology of bladder cancer, including the migration of macrophages towards cancer cells and phenotypic alterations of stromal cells, as well as the formation of vascular-like tubes of tumour cells. The expansion, invasion and metastasis of tumours depend on complex direct and indirect cell–cell interactions between cancer cells and the immune system of the host. To study immune surveillance, which comprises complex crosstalk between cancer cells and the immune system, Businaro et al. (Fig. 1E) employed a device similar to that devised by Ma et al. (see Fig. 1A) to analyse the influence of interferon regulatory factor 8 (IRF-8), which plays an important role in the induction of competent immune responses and is also a tumour suppressor.56,57 The authors showed that the knockout of IRF-8 in spleen cells inhibited the motility of cells and their interactions with B16 melanoma cells, as well as the suppression of tumours, which thus increased the melanoma extravasation rate, whereas wild-type (WT) cells retained their tumour-suppressing character.
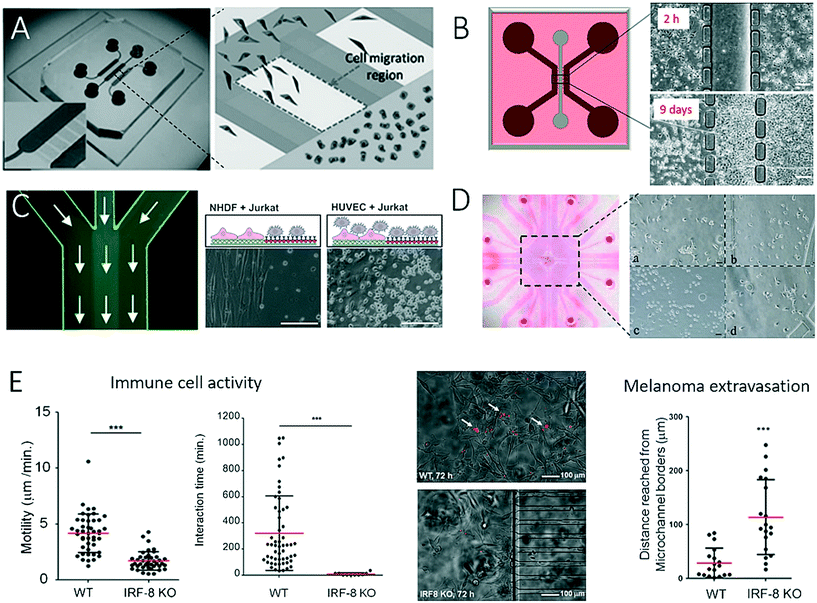 |
| Fig. 1 Examples of microfluidic devices with inter-connected cell culture chambers for studying of cell-to-cell interaction between 2-dimensional layers of tumour cells and various types of mammalian cells. In (A) Ma et al. observed interactions between fibroblasts and tumour cells. Adapted from ref. 48 with permission of John Wiley and Sons. In (B), Menon et al. studied cell migration and cellular interaction between bone marrow stromal cells and a liver tumour cells, scale bar 20 μm. Adapted from ref. 2 with permission of AIP Publishing LLC. (C) By patterning a microfluidic channel with different anisotropic crystalline protein nanolayers, Rothbauer et al. could co-culture and study the interaction between cancer cells and immune cells, scale bar 200 μm. Adapted from ref. 43. In (D) Liu et al. could mimic the microenvironment in a bladder by co-culturing four different cell types in cell culture chambers separated by hydrogel barriers. Reproduced from ref. 55 with permission of Impact Journals, LLC. (E) Businaro et al. studied the role of interferon regulatory factor 8 (IRF-8) in cancer progression using a two-dimensional microfluidic co-culture as immunodeficiency model. Reproduced from ref. 56 with permission from The Royal Society of Chemistry. | |
The integration of microvalves, as well as non-invasive biosensors, has proven suitable for increasing throughput and facilitating the automation of such two-dimensional microfluidic cell migration systems. For instance, Gao et al. introduced an enhanced in vitro co-culture platform that consisted of a pneumatically activated microvalve system for physically separating different cell types within microfluidic channels (Fig. 2A).58 In this PDMS-based microdevice, direct cell-to-cell contact was initiated by opening the valves between two cell culture chambers. Cross-migration of 4T1 murine mammary tumour cells and human dermal microvascular endothelial cells (HDVECs) was analysed using live-cell imaging. In normoxic conditions, tumour cells and endothelial cells migrated towards each other, whereas under hypoxic conditions induced by cobalt chloride (CoCl2) cell migration was predominantly hindered for tumour cells. In a similar approach, Zheng et al. developed a microfluidic microarray that contained integrated central separation barriers to create a device for parallel cell migration (Fig. 2B).59 Using a 4 × 4 interconnected microchamber array, controlled cell seeding, co-culture, and medium exchange, as well as multiplexed analysis of migration, were simultaneously achieved. In addition to the integration of microvalves and separation barriers into microdevices, another reported technological advance comprises the combination of complementary and orthogonal sensing strategies to monitor cell-to-cell interactions.38Fig. 2C shows a microfluidic biochip that contains embedded interdigitated arrays of impedance sensors and organic photodetectors for optical light scattering measurements to assess the interactions of a small number of DU-145 prostate cancer cells with an endothelial cell barrier and a stromal cell culture.38 The results of the study showed that DU-145 prostate cancer cells were not able to invade a functional endothelial cell barrier under physiological flow conditions but could freely pass through stromal cells, which indicated their low metastatic ability. The authors demonstrated how non-invasive biosensors can be employed for the automation of assays of cell migration and tumour cell invasion on-chip, as they are highly sensitive to cell population responses without the need for cell staining or fluorescent transfection reagents.
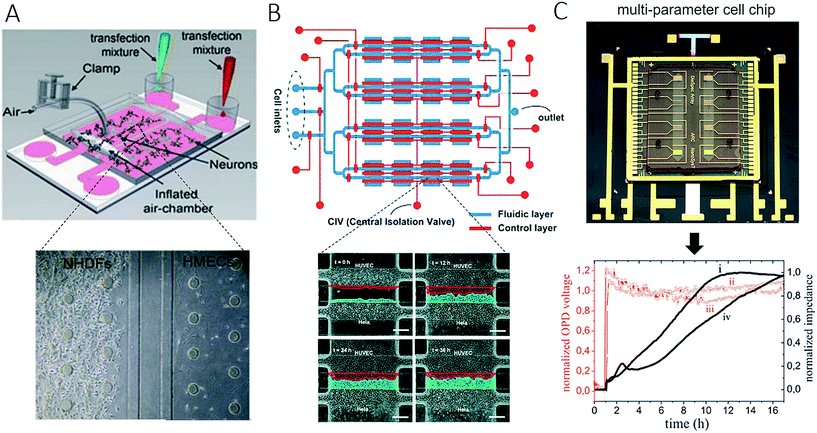 |
| Fig. 2 Examples of complex microfluidic devices for studying of cell-to-cell interactions. (A) Gao et al. controlled cell-to-cell contact between tumour cells and endothelial cells with integrated pneumatic valves. Figure was adapted from ref. 58 with permission from Springer Science+Business Media, LLC. In (B) Zheng et al. could monitor cell-migration in 4 × 4 interconnected microchambers simultaneously by using pneumatic-controlled valves. Adapted from ref. 59. Copyright 2017 American Chemical Society. (C) Charwat et al. studied the invasive capability of DU-145 cells towards vascular cells in a microfluidic chip with integrated impedance sensor arrays and organic photodetectors. Adapted from ref. 38. | |
1.2 On-chip cell migration and cancer in the third dimension
Because human physiology is complex in its architecture, in the last two decades research efforts have been invested in bioengineering mimics of in vivo physiology on a three-dimensional level, and some methods were even translated into microfluidic models. Three-dimensional systems that are in vivo-like with respect to not only cellular function but also tissue and organ geometry can shed more light on the structural and functional relationships between vasculature, the immune system and cancer. Although most microfluidic studies of cancer are conducted using two-dimensional monolayer cell cultures, these planar models of cancer are known to have limited practicality in understanding the complex physiology of tumours in vivo. The main reason for this is that in vivo both the structure and the geometry of tissue significantly influence the growth rates of tumour cells. To address this limitation, a number of microfluidic devices and methods for three-dimensional cell culture (so-called spheroids) have been devised in recent years.5,44–47 Microdevices that feature the three-dimensional co-culture of cancer spheroids have been developed to mimic the three-dimensional cancer environment for a variety of types of cancer including cancer of the salivary glands and lung cancer, as well as for studies of intra- and extravasation. For instance, to increase the relevance of assays of tumour invasion and cell migration to the biology of breast cancer Sung et al. proposed the sequential loading of cell types at different time points using pumping driven by surface tension to establish a three-dimensional model of breast cancer invasion (Fig. 3A).60 Using the microfluidic device for modelling breast cancer, the authors confirmed the relevance of their model by monitoring the transition from ductal carcinomas in situ (DCIS) to invasive ductal carcinoma (IDC) in vitro, which showed good agreement with in vivo xenograft models used for tumour invasion studies. Also employing spheroids, Liu et al. proposed a three-dimensional microfluidic network that consisted of intersecting cell culture chambers to determine the influence of carcinoma-associated fibroblasts (CAFs) on the progression of malignant cancer (Fig. 3B).61 The invasion of spheroids of adenoid cystic carcinoma (ACC) of the salivary gland was exclusively observed in the presence of CAFs that expressed α-SMA, which thus indicated the mechanism of invasion involved. An important aspect of cancer research comprises cell migration, which can lead to intravasation and extravasation events during the formation of metastases. As an alternative strategy to spheroid technology, cell-laden hydrogels have also been integrated on-chip for cancer research. For instance, a three-dimensional microfluidic model of breast cancer metastasis introduced by Bersini et al. that consisted of vascular and hydrogel tissue compartments was used to study the motility of MDA-MB-231 cancer cells in a microenvironment conditioned by osteo-cells (Fig. 3C).62 Extravasated and active cancer cells proliferated and formed micrometastases with increased sizes of up to 132 cells after 5 days of culture. Because the precise control of biochemical factors is a key parameter in understanding the tumour–vasculature interface, Zervantonakis et al. established a three-dimensional microfluidic model to study the intravasation of cancer cells that lead to the formation of secondary tumours (Fig. 3D).63 Using a three-dimensional microfluidic model of the vascular barrier based on triple co-culture of endothelial cells, macrophages and cancer cells, the authors observed similar percentages of tumour cells in various vascular barriers and found that tumour necrosis factor-alpha (TNF-α) secreted by macrophages was the main driving force that compromised the tightness of the endothelial barrier and allowed tumour cells to circulate in the vascular channel compartment, which thus indicated the key roles of immune cells and soluble factors in the progression of cancer via metastasis.
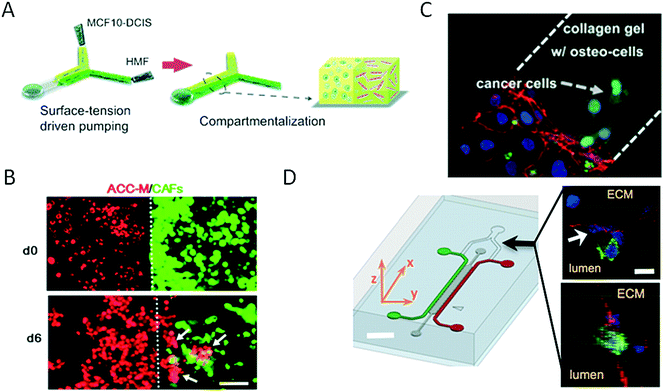 |
| Fig. 3 Three-dimensional strategies for on-chip bioengineering of the cancer microenvironment. (A) Sung et al. established a 3D breast cancer invasion model by sequential loading of cells with surface-tension driven pumping. Reproduced from ref. 60 with permission from The Royal Society of Chemistry. In (B) Liu et al. co-cultured carcinoma-associated fibroblasts (CAFs) and salivary gland adenoid cystic carcinoma (ACC) cells in a 3D matrix, demonstrating that CAFs was promoting ACC cell invasion and thereby indicating its critical role in cancer invasion. Scale bar 100 μm. Reproduced from ref. 61 with permission from The Royal Society of Chemistry. (C) Bersini et al. studied extravasation and micrometastasis generation of breast cancer cells within a bone-like microenvironment. Reproduced from ref. 62 with permission from Elsevier. (D) Zervantonakis et al. demonstrated the impact of macrophages on tumor cell intravasation with a three-dimensional microfluidic barrier model. Scale bar 30 μm. Reproduced from ref. 63. Copyright 2012 National Academy of Sciences. | |
The importance of vascularization in cancer biology and the connection between invading tumour cells and vascular barrier cells further led to the development of various microsystems that more closely resemble the architecture of blood vessels (round channel morphology like a cellular lumen). For instance, Wong and Searson established a live-cell analysis platform that was capable of investigating the behaviour of metastatic cancer cells within a functional artificial ECM-based microvessel.64 More recently, the same team used another vascularized microdevice with similar lumen morphology to demonstrate that brain microvascular endothelial cells resist elongation in response to curvature and shear stress, and form tighter barriers in contrast to vascular endothelial cells (see Fig. 4A).65 A refined bioengineering approach was also established by George et al., who exploited the self-organizing nature of human microvessels to perfuse cancer cell spheroids (Fig. 4B).47,66 Another demonstration of the usefulness of complex bioengineered systems containing cancer and vascular microenvironments for the establishment of meaningful in vitro models of tumours was provided by Buchanan et al., who showed that all tumour-expressed pro-angiogenic genes were significantly upregulated during co-culture with endothelial cells and when exposed to shear stress.68 In addition, angiopoietin-2 (ANG2) and platelet-derived growth factor-B (PDGFB), which are both factors that are involved in angiogenesis in breast cancer,69 were upregulated upon cellular stimulation by shear stress.
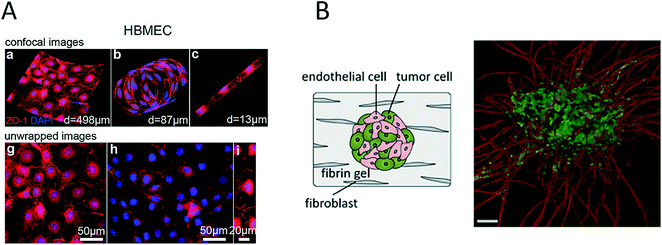 |
| Fig. 4 Microsystems mimicking complex vasculature structures. (A) Ye et al. investigated the shear-dependent elongation behaviour of brain microvascular endothelial cells. Adapted from ref. 65. (B) Ehsan et al. investigated early events of solid tumour progression with a prevascularized tumour (PVT) model composed of spheroids of endothelial and tumour cells, embedded in a fibrin matrix containing fibroblasts. Bar size 100 μm. Adapted from ref. 66 with permission of The Royal Society of Chemistry. | |
1.3 Microfluidic cell co-culture systems for anticancer drug screening
As discussed in the last section, a variety of bioengineering approaches are capable of the generation of more relevant in vitro models of cancer. Within this section, we intend to focus on the application of such models and highlight recent advances in drug screening that aim for better therapeutic outcomes in cancer. The main limitation of most on-chip models used for drug screening is that these microdevices in most cases do not have sufficient throughput to be an effective screening tool. For instance, Choi et al. developed a microfluidic device for drug screening that was capable of co-culturing breast tumour spheroids and human mammary duct epithelial cells, as well as mammary fibroblasts, using a compartmentalized 3D microfluidic device (Fig. 5A).67 Using this “breast cancer-on-a-chip” device, the impact of treatment with the clinical anticancer drug paclitaxel on the size of DCIS spheroids was investigated to demonstrate the efficacy of the drug in arresting the proliferation of tumour cells and thus preventing the growth of DCIS lesions in a microenvironment that closely resembled that of breast ductal carcinoma. Even though the microsystem employed exceptional bioengineering to create a complex tumour model, each device consisted of only one cell culture and thus failed to provide adequate throughput for drug screening. To create a system that was capable of higher throughput, Xu et al. established a system comprising 4 × 3 hydrogel culture chambers in parallel for anticancer drug screening using co-culture of a human non-small cell lung cancer cell line (SPCA-1), a human lung fibroblast cell line (HFL-1) and patient-derived lung cancer cells (Fig. 5B).70 For chemotherapeutic screening of different anticancer drugs, the authors employed on-chip concentration gradient generators (CGG) to dispense three different concentrations automatically to each functional screening unit. The microdevice enabled accurate screening of the sensitivities of eight samples of patient-derived lung cancer cells to different anticancer drugs in parallel, which resulted in appropriate doses and single- as well as multi-drug chemotherapy schemes. A different three-dimensional microfluidic cell culture system containing a concentration gradient generator, which was also termed the SpheroChip system, enabled the on-chip formation of spheroids of liver and colon cancer for drug sensitivity testing.71 In the work of Bauer et al., a platform that contained an array of 96 single microfluidic channels was used for the analysis of paracrine signalling using a 3D co-culture of human mammary fibroblasts and T47D breast carcinoma cells (Fig. 5C).72 With this system, the authors aimed to replace the conventional microwell format for three-dimensional cell culture and enable automated high-throughput cancer screening for individual patients, as well as for drug discovery. A disadvantage of the system may be that intensive automation technology is needed for medium exchange or the injection of drugs owing to the lack of nutrient administration via microchannels and pumps, which are both major advantages of microfluidic systems. Another very promising pharmacological approach was reported by Imura et al., who established a bioassay system that could assess the intestinal absorption, hepatic metabolism and bioactivity of ingested substances, including anticancer therapeutic agents (Fig. 5D).73,74 This idea is similar to the micro cell culture analogue (μCCA) concept, which had already been introduced by Sung and Shuler in 2009, which highlights the importance not only of cellular but also of organ-level function.75 The authors evaluated the microsystem via the inclusion or exclusion of digestion processes during the administration of anticancer drugs. The anticancer activity of TGF was lost in all cases, which indicates that the drug was degraded by synthetic gastric juices and was in accordance with the known properties of these drugs. These results emphasize that pharmacological aspects, in particular the oral uptake of anticancer medications, are very important parameters to be considered for new therapeutic agents, because enzymes can degrade bioactive substances and thus render them ineffective.
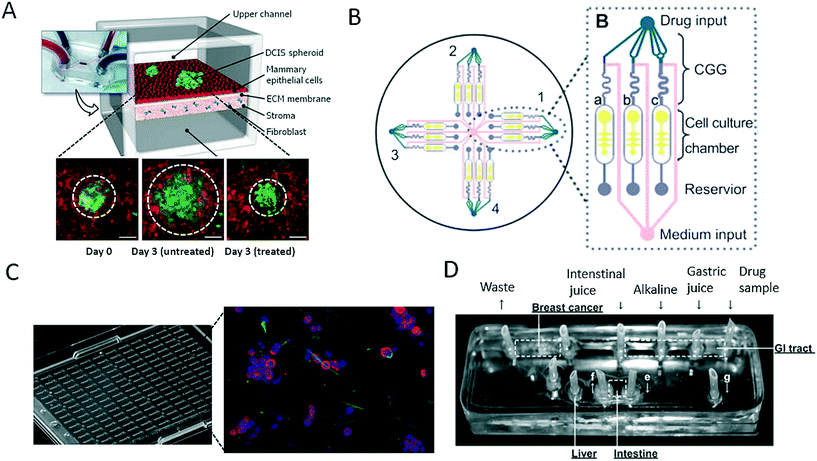 |
| Fig. 5 Microfluidic models for screening of anticancer drugs. (A) Choi et al. developed a breast cancer-on-a-chip for drug screening purposes. The efficacy of the anti-cancer drug paclitaxel was demonstrated by treating a DCIS spheroid and monitoring the size reduction. Scale bars 100 μm. Reproduced from ref. 67 with permission from The Royal Society of Chemistry. (B) Xu et al. established a microfluidic three-dimensional co-culture system for drug sensitivity testing of a lung cancer model. Reproduced from ref. 70 with permission from Elsevier. (C) Bauer et al. performed high-throughput screening on 3D co-culture of mammary fibroblasts and breast carcinoma cells. Reproduced from ref. 72 with permission from The Royal Society of Chemistry. (D) Imura et al. established a micro total bioassay system mimicking physiological processes with the aim of evaluating orally administered cancer drugs. Reprinted and adapted from ref. 73. Copyright 2017 American Chemical Society. | |
1.4 Recent advances in immunotherapy-on-a-chip
Immunotherapy in general is the clinical application of therapeutic agents that can enhance immune effector mechanisms. More recently, cell-based therapeutic agents have gained momentum as the rationale behind personalized patient-derived anticancer treatment. Among the variety of blood cell populations that are available, antigen-presenting dendritic cells, as well as CD8+ T cells, are two promising cell types that can increase the potency of current anticancer therapies. A more comprehensive review of the state of the art in adoptive cell-mediated cancer immunotherapy based on T cells, as well as dendritic cell vaccines, can be found elsewhere.76,77 Dendritic cells can act as an adjuvant owing to their role in identifying and presenting antigens to T cells to create an antitumour immune response. Therefore, microsystems have recently emerged that aim to shed light on the complex cellular mechanisms that guide antitumour responses to enable the creation of more effective cell-based immunotherapies. For instance, Parlato et al. presented a microfluidic system (Fig. 6A) for analysing the tumour-suppressing ability of dendritic cells conditioned by interferon-alpha (IFN).78 The device enabled the analysis of cell migration, as well as the infiltration of dendritic cells into the tumour compartment. The authors showed that combinatorial treatment with interferon-alpha and romidepsin (RI) resulted in high levels of cell apoptosis and increases in the phagocytosis of tumour cells by IFN-dendritic cells. In summary, this microdevice enabled the analysis of dendritic cell-cancer cell interactions within a three-dimensional tumour microenvironment and the identification of major underlying factors (e.g., CXCR4) and thus proved the potential of such microfluidic devices as innovative tools for the effective testing of novel immunotherapeutic strategies. Our group has reported a lab-on-a-chip system (Fig. 6B) for the non-invasive multiparametric dynamic monitoring of T cells and cancer cells.38 Initially, we demonstrated how such a system can be used for the label-free automation of a conventional T cell proliferation assay, where primary T cells were stimulated using CD3/CD28-labelled Dynabeads nanoparticles for the expansion and proliferation of T cells. Furthermore, biparametric analysis was employed in the microsystem to identify how the priming of T cells with Dynabeads leads to tumour-suppressing responses in OCM-1 melanoma cells with the formation of two-dimensional, irregular aggregates of cancer cells. These results indicate that label-free biosensing methods can prove their worth for quality control, as well as non-invasive readout, in such delicately bioengineered co-culture systems. In a three-dimensional approach, Pavesi et al. incorporated human cancer hepatocytes (single cells or tumour cell aggregates) in a 3D collagen hydrogel in a microfluidic device to assess the tumour-suppressing ability of engineered, T cell-based anticancer vaccines.79 The authors demonstrated how human T cells that were engineered to express tumour-specific T cell receptors (TCR–T cells) migrated and killed the target tumour cells. In addition, they analysed soluble factors under conditions of varying oxygen levels and in the presence of pro-inflammatory cytokines. Interestingly, cancer aggregates, but not conventional cultures, were affected by environmental changes. In a final set of experiments, the authors demonstrated that the 3D microdevice could be used to analyse the efficacy of TCR–T cells in an immunosuppressive cell-cancer cell co-culture scenario. In general, the main advantage of employing microfluidic strategies lies in the inherent ability of such systems to geometrically confine cells, which therefore enables the analysis of cell–cell interactions using microscopy and label-free biosensors.
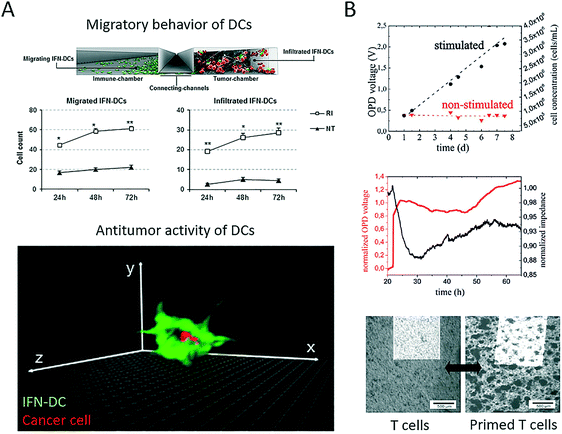 |
| Fig. 6 Microfluidic systems for cell-based immune therapy applications. (A) Parlato et al. demonstrated with their multi-compartment microsystem how IFN alpha preconditioning on dendritic cells (IFN-DC) in combination with romidepsin (RI treatment) results in increased migration, infiltration and cancer engulfment of dendritic cells. Adapted from ref. 78. (B) Charwat et al. studied both tumour invasion and suppression in a microfluidic chip with integrated impedance sensor arrays and organic photodetectors. Reproduce from ref. 38. | |
2. Microfluidics and neurobiology
The nervous system of the human body can be structurally divided into the central nervous system (CNS), which comprises the brain and spinal cord, and the peripheral nervous system (PNS), which includes all other nerve structures throughout the body. Along these lines, established lab-on-a-chip and organ-on-a-chip systems mimic the physiology of these different tissue structures to gain a deeper understanding of various aspects of brain development and dysfunctions, including the onset and progression of neurodegenerative diseases. The premise of these advanced microdevices is to overcome existing limitations of conventional cell-based technologies that mainly record neuronal data based on the activity of cellular clusters and thus only provide information on subpopulations of neurons.80 Consequently, the following section on microfluidic technology mainly highlights more recent developments that provided deeper insights into neurobiology. A basic overview of on-chip neurobiology and a report on progress prior to 2010 can be found elsewhere.81,82
One recent technological advance involves the generation and manipulation of synapses on-chip, where rat hippocampal neurons were used to form synapses in a microgroove array to study the effect of chemical injuries between two spatially resolved compartments used for neural cell culture.83 The system that was presented enables spatial and temporal control of the neuronal microenvironment, which is not possible to achieve in conventional culture systems (Fig. 7A). Similarly, Shin et al. developed a microfluidic microgroove system to reliably generate neurons derived from stem cells on-chip as a replacement for primary neuron cultures.84,85 Higashimori and Yang used a similar design for application as a microfluidic co-culture platform to study the interactions of neurons with glial cells. They demonstrated how microfabricated systems enable the use of delicate imaging instrumentation and therefore allow the more detailed analysis of cell-to-cell interactions in the central nervous system (Fig. 7B).86
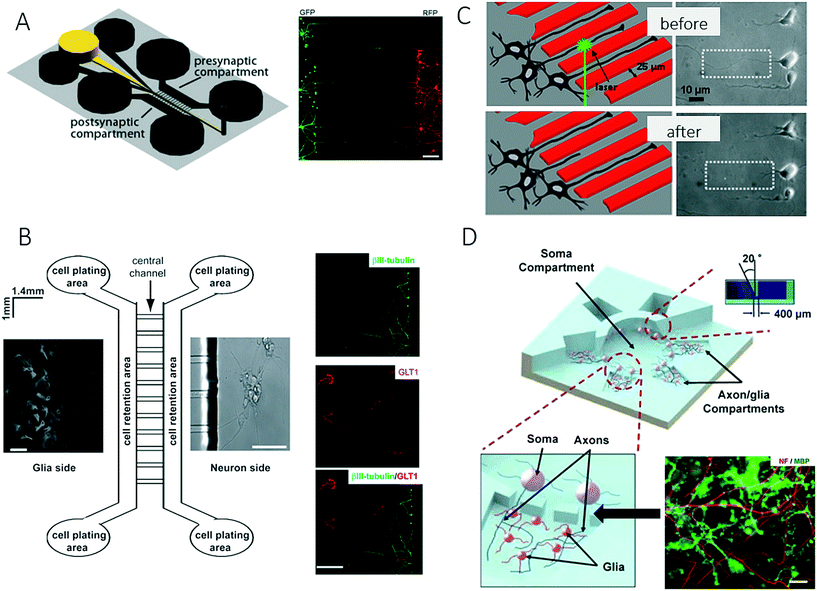 |
| Fig. 7 Microfluidic neuronal models for studying interactions between cells in the central nervous system. (A) Taylor et al. studied the effect of chemical injuries between two spatially resolved neural cell culture compartment. Scale bar: 150 μm. Reproduced from ref. 85 with permission from Elsevier. (B) Higashimori et al. combined a microfluidic co-culture system with delicate imaging instrumentation to examine cell-to-cell interactions between axonal/dendritic and glial cells. Right: Axon bundles (green) and astrocytes (red). Scale bar: 50 μm. Reproduced from ref. 86. Copyright 2012, Journal of Visualized Experiments (C) Kim et al. developed a platform to quantify the regeneration of injured CNS. By generating precise lesions sited in neurons with laser induces axotomy regeneration of single axons could be precisely studied. Reproduced from ref. 87 with permission from Springer US. (D) Park et al. established a complex microfluidic 3D platform for studying axon-glia interactions during drug and biomolecule treatment on multiple co-cultures. Reproduced from ref. 89 with permission from The Royal Society of Chemistry. | |
For precise control over CNS injuries and lesions, Kim et al. introduced a microfluidic platform that was capable of controlling axonal growth by surface modification, as well as controlling soluble factors (Fig. 7C).87 In addition, the authors demonstrated how an optical setup can be used for the precise generation of lesion sites (laser-induced axotomy), as well as the analysis of regeneration at a single-axon resolution. A deeper analysis of the central nervous system was further accomplished by introducing on-chip high-resolution imaging to study cell-to-cell interactions between neurons and glial cells and also to account for the complex bidirectional signalling processes that take place in specific neuronal structures such as axons or dendrites. In a related study, Zahavi et al. used a similar compartmentalized microfluidic system to establish a neuromuscular co-culture model for investigating cell-to-cell interactions between motor neurons and muscle at neuromuscular junctions.88 The authors showed that glial-derived neurotrophic factor (GDNF) modulates growth and muscle innervation at axons, in contrast to survival pathways in the soma. In an attempt to increase the number of compartments and to integrate a larger, central soma compartment, Park et al. combined various microfabrication methods including micromilling, hot embossing and soft lithography to fabricate a complex three-dimensional microfluidic culture system (Fig. 7D).89 This technological advance enabled for the first time multiple experimental conditions for heterotypic cell culture and different localized biomolecular treatments on a single device to study cell-to-cell interactions and the development of oligodendrocyte progenitor cells (OPC), as well as the investigation of axonal responses to various chemical stimuli.
Another technological advance in chip-based neurobiology included the integration of microvalves for opening and closing connecting conduits between neighbouring cell culture compartments to study interactions between spatially resolved neurons and glial cells.58,90 In a follow-up paper, the same research group further demonstrated that the valve-integrated microfluidic platform for neuron–glial cell co-culture dramatically increased synaptic stability, with elevated levels of soluble factors, and thus provided a deeper insight into how synapses may be modulated (Fig. 8A).91 For instance, on-chip co-culture in a vertically layered configuration with neurons cultured on the bottom of a microchannel and glial cells cultured on the ceiling of the channel led to an increase in dendritic protrusions, as well as synaptic contacts, which indicated that soluble factors alone within a conditioned medium were insufficient to enhance the stability of synaptic contacts. In contrast to microfluidic strategies that confine and guide neuronal cell-to-cell interactions, a microcontact printing (μCP) technique has been employed by Marconi et al. to control the functional properties of neurons on the basis of adjusting the surface topography (Fig. 8B).92 The authors concluded that cellular micropatterning, which is a technique frequently employed in tissue engineering, does not influence the electrophysiological behaviour or the connectivity of neurites. In a similar fashion, nanovolume patterning has been used to establish microarrays of live neuronal cells within biochips.93 Using such a microfluidic live-cell microarray platform, the authors demonstrated the sensitivity and reliability of patterning approaches for screening defects in connectivity in neuropathophysiology (Fig. 8C).93 Strategies that are less often used in microfluidics include the integration of intact tissue slices in so-called brain slice on-a-chip devices for studying tissue responses within a functioning in vivo 3D cellular organization. Because the majority of these systems rely on rodent organ donors, the results may not be transferable to human neuronal (patho)physiology.94 It is, however, important to note that, as in cancer biology, recent trends in this very promising research field have increasingly shifted from two-dimensional co-cultures to complex three-dimensional cultures and artificial microtissues.95
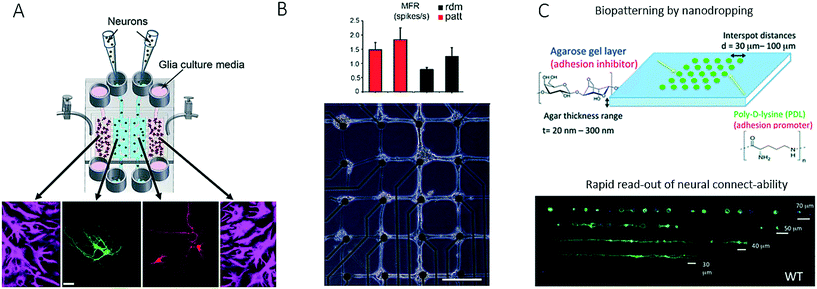 |
| Fig. 8 Further examples of microfluidic systems for investigating cell-to-cell interactions in the central nervous system utilizing different methods for controlling cell growth into complex networks. (A) Shi et al. studied synapses with a microfluidic neuron-glia co-culture platform. Scale bar: 25 μm. Adapted from ref. 91 with permission of The Royal Society of Chemistry. (B) Marconi et al. used micropatterning for guiding neurons into forming a neural network with a defined topology as well as for investigating the functional properties of the network. Scale bar: 200 μm. Adapted from ref. 92. (C) Petrelli et al. established a micro-array for screening of neural connectability by using nano-volume patterning in a biochip. Adapted from ref. 93 with permission of The Royal Society of Chemistry. | |
3. Bioengineering of vascular models on-chip
Blood vessels, as principal transporters of oxygen and nutrients, play a key role in maintaining organ health and have been incorporated or reengineered into microfluidic devices in the last decade to study the effects of increasing shear forces, platelet adhesion and nutrient supply in biomimetic tissue structures, including the diffusion and uptake of soluble factors and nanomaterials. Because the vascular system, in particular, the endothelial wall, represents a natural barrier that needs to be breached by migrating tumour cells during intra- and extravasation processes in cancer metastasis, many vascular models have been established to gain deeper insights into cell-to-cell interactions of malignant cells in the circulatory system.47 Recent efforts in advancing the microfabrication of artificial vessels using different techniques and biochips are described in the following section.
One major improvement over 2D microfluidic endothelial monolayers96,97 comprises the creation of perfusable capillaries and lumen-like structures based on either cellular self-organization or microfabrication techniques employing hydrogels. For instance, a co-culture microdevice based on a versatile polymeric two-component microfabrication system was recently established for spatially separating vein endothelial cells from cultures of adipose-derived mesenchymal stem cells that had been embedded in a 3D fibrin matrix.97 In addition, Yeon et al. employed microfluidic techniques to form in vitro three-dimensional tubular capillary networks on-chip (Fig. 9A) by facilitating the invasion of human umbilical vein endothelial cells (HUVECs) into microchannels embedded in a fibrin hydrogel, which formed perfusable, lumen-like structures over a period of 5 days.98 Similarly, Kim et al. presented a microdevice that contained microstructures for promoting on-chip vasculogenesis and angiogenesis after the addition of pro-angiogenic factors and the formation of lumenised microvessels in the presence of endothelial cell-fibroblast interactions (Fig. 9B).99 The authors demonstrated that not only could angiogenesis be fully reproduced, but also the monitoring of diverse types of cell–cell interactions was enabled, including tumour-induced angiogenesis (endothelial cells and U87MG cells), as well as endothelial cell–pericyte interactions (endothelial cells, fibroblasts and pericytes). A similar, but more complex, microfluidic design consisted of seven interconnected microfluidic compartments, which enabled the controlled spatial distribution of stromal cells in the vicinity of the endothelium for studying the impact of scenarios involving multiple soluble factors on the diameter of the vessel that was formed.101 A higher degree of parallelization was also achieved using a high-throughput microvessel platform that contained interconnected networks of human capillaries with perfusable lumens (Fig. 9C).100,102 The same device was subsequently used for screening applications of homo- and heterotypic cell-to-cell communication based on multiple stimulation scenarios with different soluble factors. To analyse the pro-angiogenic influence of the proximity of stem cells and/or secretomas on the formation of vascular networks, a microfluidic platform containing mono- and co-cultures of human umbilical vein endothelial cells and adipose-derived embryonic stem cells embedded in fibrin was established in a single device (Fig. 9D).103 Moreover, a co-culture system embedded in fibrin was employed for the dynamic tuning of soluble factors within a hydrogel tissue construct, which resulted in an inhomogeneous vascular network that exhibited different tube dimensions. These and other examples show how microfluidic techniques can be used not only for the supply of nutrients but also as an innovative tool to control the morphology of a vascular network inside a three-dimensional tissue construct, including the size and length of tubes.
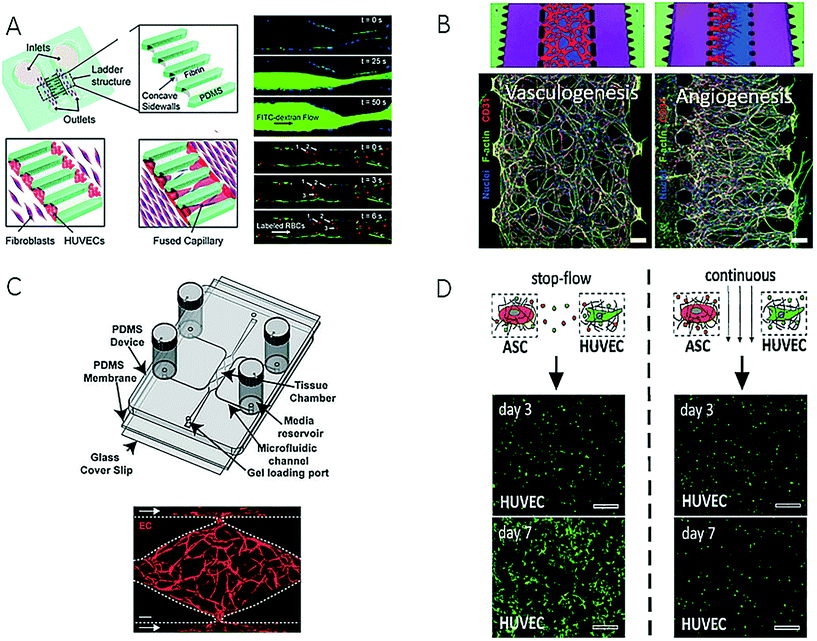 |
| Fig. 9 Examples of microfluidic devices for creation of artificial vascular system. (A) Sobrino et al. could form a capillary network by growing human umbilical vein cells (HUVECs) in microfabricated channels filled with fibrin gel. Reproduced from ref. 100 with permission from The Royal Society of Chemistry. (B) Kim et al. were able to reproduce vasculogenesis and angiogenesis and could monitor different cell-to-cell interactions. Reproduced from ref. 99 with permission from The Royal Society of Chemistry. (C) Moya et al. created an interconnected capillary network with perfusable lumen inside a multi-plexed microvessel platform. Scale bar: 50 μm (left) and 200 μm (right). Adapted from ref. 102. (D) Purtscher et al. presented a microfluidic chip capable of tuning secretoma-related aspects of vascular network formation on a mesenchymal stem cell/HUVEC co-culture using stop-flow and perfused culture protocols. Reproduced from ref. 103. | |
Although microsystems based on fibrin hydrogels are most commonly used to support the growth and invasion of vascular sprouts over several days, alternative 3D matrices have been used. For instance, van der Meer et al. presented a novel approach for the on-chip formation of a microfabricated vessel that was based on the precisely controlled co-culture of human umbilical vein endothelial cells and pericytes derived from human embryonic stem cells co-injected with rat tail collagen-I (Fig. 10A).104 The authors showed that a mature endothelial layer with characteristic cell-to-cell interactions was formed within 12 hours of culture for the study of defective vasculogenesis and angiogenesis related to errors in the transforming growth factor-beta (TGF-β) pathway in human disease. Another interesting approach comprises a PDMS–glass–hydrogel microfluidic device containing an integrated micromolded collagen microchannel (Fig. 10B) for identifying the invasion of distinct types of endothelial cells upon the stimulation or depletion of VEGF.105 A human vascular microsystem for angiogenesis was developed to study barrier permeability during cancer cell migration using a collagen-I matrix (Fig. 10C).106 After the formation of a mature microvessel, human umbilical vein endothelial cells induced sprouting through the collagen-I matrix using an established gradient of a growth factor cocktail. In a similar manner, Nguyen established a microdevice that comprised two micromolded collagen microchannels for the morphogenetic analysis of endothelial sprouting and the impact of angiogenesis inhibitors on the morphogenesis of sprouting (Fig. 10D).107 In summary, even though different microengineering strategies were used and hydrogels also varied between the proposed microdevices, the common feature comprises the formation of a perfusable bioengineered microvessel for future research into angiogenesis and vascularization.
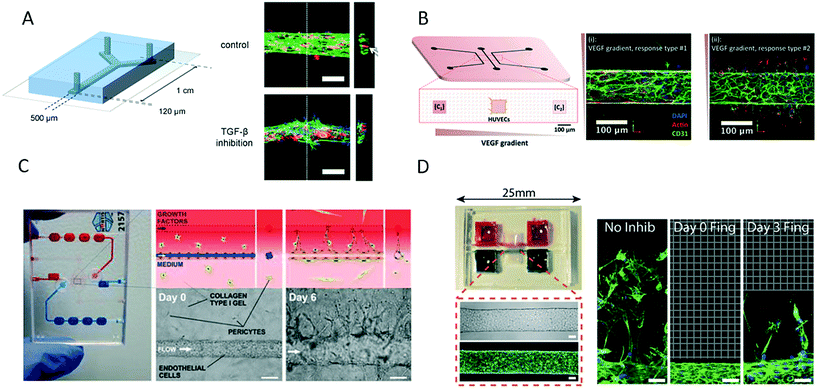 |
| Fig. 10 Microfluidic vascular models based on different 3D matrices. (A) van der Meer et al. used rat tail collagen to support growth of an endothelial layer inside a microfluidic channel to study the impact of errors in the transforming growth factor-beta (TGF-β) pathway in vasculo- and angiogenesis. Scale bars: 100 μm. Adapted from ref. 104 with permission from The Royal Society of Chemistry. (B) Verbridge et al. studied endothelial sprouting in response to vascular endothelial growth factor (VEGF) gradients in type I collagen hydrogel-embedded channels. Adapted from ref. 105 with permission from John Wiley and Sons. (C) Tourovskaia et al. could form a mature microvessel in a collagen I matrix for investigating angiogenesis, barrier permeability and cancer cell migration. Scale bars: 125 μm. Adapted from ref. 106. (D) Nguyen et al. studied multicellular endothelial sprouting as an effect of angiogenic factors in a vascular model formed in collagen. Scale bars: 50 μm. Adapted from ref. 107. | |
4. Cell–cell interaction on the single-cell level
In the first sections of this paper, we reviewed microfluidic systems where in vivo-like models were created to study cell–cell interactions between a variety of cells. The different cell populations in these devices are most commonly seeded individually and initially separated with a barrier, and cell–cell interactions are observed dynamically owing to cell motility and changes in cell proliferation.108 Cells that are physically distant communicate by releasing signalling molecules, which diffuse through the extracellular fluid to the target cell, where they are detected by membrane proteins.1 Besides indirect communication, cells that are in direct contact also communicate by sending signalling molecules across gap junctions.1 To investigate the molecular mechanisms that underlie cell–cell interactions between cells in direct contact, cell populations must be decomposed and isolated to the single-cell level. In this manner, the variety in phenotype and genotype within the same cell population, which is referred to as cellular heterogeneity, can be studied.109 Because microfluidic technology enables the spatial control of cells, microsystems offer the unique possibility of positioning two cells next to each other.110 Cell pairing can be achieved either horizontally or vertically, and cell trapping in microfluidics can be conducted via several different approaches, such as microwells,111 dielectrophoresis,112 surface acoustic waves,113 encapsulation in microdroplets,114 and hydrodynamic trapping.115 In the following section, microfluidic platforms for studies of cell–cell interactions at the single-cell level are briefly reviewed to the best of our knowledge.
4.1 On-chip cancer biology on the single-cell level
Cancer cell–stromal cell interaction is a critical process in tumorigenesis, and its study is important for the understanding of the development and progression of cancer. In a work by Yoon et al.,116 a platform was developed for cell trapping and cell pairing using dual-stream hydrodynamic trapping techniques. The cell pairing ratio and cell–cell interaction time could be precisely controlled by the electrolytic generation of bubbles. Via a cell interaction assay between prostate cancer cells (PC3) and myoblasts (C2C12) at different cell pairing ratios, it was demonstrated how the proliferation rate of the myoblasts was increased at a higher pairing ratio of cancer cells. The enhanced proliferation of C2C12 cells can be explained by growth factors secreted by PC3 cells, which are known to increase the reproduction of C2C12 cells, which in turn has an effect on tumour growth and progression.117 To investigate the angiogenic potential of tumour cells in response to cell–cell interactions at the single-cell level, Tan et al. developed a peel-off cell culture array that was capable of patterning and culturing cells on a large scale. The cell culture array was microfabricated by etching rectangular cavities into parylene and thereafter filling the cavities with fibronectin to enable cell adhesion. After peeling off the parylene template, cells were seeded onto the fibronectin array, and, depending on the size of the fibronectin features, the tumour cells were cultured in clusters or at the single-cell level. With this system, the authors investigated the impact of the proliferation of human oral squamous cell carcinoma (OSCC3) and human prostate carcinoma (DU145) cells at the individual level in comparison with cell clusters and their role in the regulation of tumour progression and angiogenesis. The secretion of the pro-angiogenic factors VEGF, bFGF and IL-8 was analysed as a measure of cell–cell interactions that promote angiogenesis. In the present study, it was seen that the secretion of VEGF was upregulated for the incubation of cell clusters in comparison with single cells, which indicated that cell–cell interactions promoted enhanced proliferation and had pro-angiogenic potential. In summary, single-cell technology is an important strategy for identifying cellular heterogeneity within a single cancer type. However, for studies of cell–cell interactions Boyden chamber-like or hydrogel-based multi-compartment chips seem to be more physiologically relevant and in vivo-like.
4.2 Neurobiology and microfluidics at the single-cell level
As is evident from the preceding section on neuronal on-chip biology, most microdevices that have been developed provide data with single-cell resolution owing to the availability of high-resolution live-cell imaging and high-content biosensing strategies. For the sake of completeness, we mention one intricate approach that is used for cellular alignment at the single-cell level. Qin et al. used a live-cell printing technique, which is termed “block cell printing”, to investigate cellular communication between heterotypic cell pairs.118 This system was employed to analyse the intercellular communication of individual rat primary cortical neurons mediated by gap junctions. Neurons could be printed with high precision and efficiency and were cultured for 14 days with continuous monitoring of their morphology and neurite outgrowth. After 7 days of on-chip culture, single and paired neurons with highly branched dendrites were obtained on such arrays submerged in Petri dishes. Moreover, axons were successfully printed onto the substrate, which would enable the measurement of electrical signals for further analyses in future experiments. Although it is appealing from a technological viewpoint, the application of three-dimensional hydrogel cultures, as well as two-dimensional networks of neurons on MEAAs, as mentioned earlier, looks more promising with respect to high-content cell analysis on the single-cell level. Furthermore, block cell printing still needs to be incorporated into perfused microfluidic systems (on-chip integration).
4.3 Single-cell microdevices for immunotherapy research
Vaccination with dendritic cell–tumour cell fusions is an intricate strategy for cancer immunotherapy. A conventional method for the fusion of differentiated dendritic cells and tumour cells uses electrofusion, but this method is challenging owing to the high operating voltage and high probability of multiple cell fusions. Lu et al. developed a microfluidic device for the precise and rapid fusion of homogeneous or heterogeneous cell types.119 Cells are paired via a combination of hydrodynamic trapping and positive dielectrophoresis (pDEP) and thereafter fused by electrofusion inside cell traps. The functioning of the system was tested by capturing, pairing, and fusing cells of an A549 human lung carcinoma cell line with cells from a human peripheral blood acute monocytic leukaemia cell line (THP-1). Cell fusion could be achieved with an efficiency of 64%. After cell fusion, the cells could be removed from the microfluidic chip by employing negative dielectrophoresis (nDEP), which is a feature that makes this device distinguishable from other microfluidic electrofusion devices. Even though its efficiency may be high, it is questionable how this technology can be used to generate the millions of clinical-grade cell-based anticancer vaccines that are necessary for therapy. To enable cell-based immunotherapy using a microdevice with a higher throughput, Han et al. developed a single-cell high-throughput transfection tool comprising an array of small microstructures.120 Upon biophysical deformation, cells are temporarily perforated and take up molecules. The authors proposed this method for the high-throughput transfection of cell types that are difficult to transfect and demonstrated its use for gene editing of immune cells. In general, both methods represent outstanding techniques for generating novel and effective cell-based anticancer vaccines. However, these methods do not seem to be applicable in microdevices for the co-culture, maintenance and analysis of cells.
5. Organ-on-a-chip technology – a decade of more relevant on-chip biology?
In this section, we briefly give an overview of organ-on-a-chip technology over the last decade, with a focus on microdevices that employ heterotypic cell-to-cell interaction schemes (>2 cell types). In addition, we intend to highlight the current state of the art in organ models and how this interdisciplinary research field evolved. More focused and comprehensive reviews of organ-on-a-chip systems that do not meet the criteria for on-chip cell-to-cell interactions can be found elsewhere.121–128 As was pointed out in the last sections, it is evident that in vitro cell models are increasing in complexity and that a third dimension is a key requirement for the generation of functional microtissues and organoids of meaningful physiology in vivo. Cell- and organ-on-a-chip systems represent a promising and effective technology for in vitro drug screening and the development of novel medicaments. As seen in Table 1, complex organ systems have been integrated into functional microdevices that mimic the physiology of organs such as the brain, heart, intestine, kidney, lung, placenta, vasculature and even teeth. Most of these microdevices employ a combination of primary cells, as well as cancer cell types, to mimic the physiology of specific organs. Recently, a clear trend has been observed in the form of the employment of microdevices to interconnect more organ-on-a-chip modules to form a human-on-a-chip to gain deeper insights into complex human physiology on a completely different scale to standard in vitro assays. Even though the human-on-a-chip concept was already introduced by Albert Li (integrated discrete multiple organ culture – IdMOC129) and Michael Shuler (micro cell culture analogue – μCCA130) in 2004, over a decade of interdisciplinary research was necessary to refine cell biology to enable integration, create sophisticated microfluidic biochips as technical aids, and finally bring these two components together to recreate more than merely a single physiological function at organ level on a single microdevice.
Table 1 Overview of organ-on-a-chip models including single- as well as multi-organ systems
Organ model |
Cells (+/− primary) |
Features |
On-chip sensing |
Ref. |
Single-organ devices |
Blood–brain barrier |
bEnd.3 (+) |
Mechanical modulation (shear stress), barrier integrity and permeability |
Transepithelial resistance (TEER) |
139
|
C8D1A astrocytes (−) |
hCMEC/D3 (+) |
Mechanical modulation (shear stress), inflammation, barrier integrity |
Transepithelial resistance (TEER) |
140
|
Intestine |
Caco-2 (−) |
Mechanical modulation (pneumatic actuation, shear), co-culture with intestinal microbes, tissue functionality (aminopeptidase assay), barrier integrity |
— |
141
|
Lactobacillus (+) |
HCT-116 (−) |
Open microfluidic hanging-drop device, on-chip spheroid formation, real-time lactate monitoring, metabolic activity |
Lactate, glucose |
142, 143
|
Kidney |
Human proximal tubule cells (+) |
Mechanical modulation (shear stress), static vs. flow experiments, inflammation, barrier integrity, cellular transport (fluorescent albumin uptake, glucose transport), pharmacology |
— |
144
|
Rat inner medullary collecting duct cells (+) |
Mechanical modulation (shear), static vs. flow, viability, apical and basolateral markers, cytoskeletal morphology, drug screening |
— |
145
|
Human proximal tubular epithelial cells (+) |
Modeling of renal drug clearance and drug-induced nephrotoxicity |
— |
146
|
Lung |
HPMEC (+) |
Mechanical modulation (pneumatic actuation), double-barrier model, tissue functionality (aminopeptidase assay), barrier integrity (fluorescent albumin transport), inflammation, nanotoxicology (NPs) |
— |
|
A549 (−) |
H441 (−) |
Mechanical modulation (pneumatic actuation), double-barrier model, barrier integrity and permeability, oxygen transport |
— |
137
|
HPMEC (+) |
Tooth innervation |
Embryonic trigeminal ganglia (+) |
Two-compartment microfluidics, neural growth |
— |
147
|
Molars (+) |
Incisors (+) |
Eye |
Human corneal epithelial cells (HCECs) |
Bioengineered 3D tissue, mechanical actuation (blinking) |
— |
138
|
Heart |
Human iPS-derived cardiac cells (+) |
Open microfluidic hanging-drop device, impedance biosensor on-chip, beating analysis |
Impedance |
148
|
Human iPS-derived cardiomyocytes (+) |
Array of hanging posts to confine cell-laden gels, pneumatic actuation system to induce homogeneous uniaxial cyclic strains |
|
149
|
Neonatal rat cardiac cells (+) |
Liver |
Rat hepatocytes (+) |
Microfluidic microplate, oxygen transfer, viability, phenotypic analysis |
Oxygen |
150
|
Sinusoidal endothelial cells (+) |
Rat hepatocytes (+) |
Spheroid live-cell microfluidic microarray, mono- vs. co-culture, spheroid morphology, viability, albumin and urea synthesis |
— |
151
|
Hepatic stellate cells (+) |
Rat liver cells (+) |
Open microfluidic hanging-drop device, micropumps, on-chip spheroid formation, substance exposure, on-chip bioactivation loop, prodrugs |
Amperometry (lactate and glucose),134 impedance152 |
132, 133
|
HCT-116 (−) |
132
|
Rat hepatocytes (+) |
Drug screening |
— |
153
|
Human hepatocytes (+) |
Vasculature |
HDMEC (+) |
No other organ models integrated in this paper |
— |
|
Placenta |
Jeg-3 (−) |
Glucose transport (offline) |
— |
154
|
HUVEC (+) |
BeWo (−) |
Vitrified collagen membrane, mechanical modulation (shear stress), glucose transport (offline), microvillus morphology, Ca2+ ion channels |
— |
155, 156
|
HUVEC (+) |
BeWo (−) |
Mechanical modulation (shear stress), microvillus morphology, syncytialization, glucose transport (offline) |
— |
157
|
HPVECs (+) |
BeWo (−) |
Polycarbonate membranes, hydrogel cultures, multilayered microchannels |
— |
|
HUVEC (+) |
adMSC (+) |
Multi-organ devices |
Liver/vasculature |
HeLa (−) |
Coplanar model, control of directional flow of medium |
— |
158
|
HUVEC (+) |
Rat hepatocytes (+) |
3D mechanical modulation (flow conditions), hepatocyte differentiation, urea synthesis, phenotypic markers |
— |
159
|
RAMEC (+) |
BAEC (+) |
Liver/intestine/skin/kidney |
HepaRG (+) |
Organ interconnection (human-on-a-chip), integrated micropumps, air–liquid interface (skin), phenotypic analysis, gene expression patterns |
— |
160
|
Human primary hepatic stellate cells (+) |
Human juvenile prepuce cells (+) |
Human proximal tubule RPTEC/TERT-1 cells (+) |
Liver/skin |
HepaRG (+) |
Organ interconnection, micropumps, fluid flow, metabolic activity, phenotypic analysis, troglitazone screening |
— |
161
|
Human primary hepatic stellate cells (+) |
Human juvenile prepuce cells (+) |
Blood/blood–brain barrier |
MVBEC (+) |
Cell-to-cell adhesion, parasite infection of erythrocytes |
— |
162
|
Erythrocytes (+) |
Liver/bone marrow/tumour |
HepG2 (−) |
Multi-organ chip, pharmacokinetic–pharmacodynamic model (PK–PD) |
— |
163
|
Kasumi-1 (−) |
HCT-116 (−) |
Intestine/liver/tumour |
Caco-2 (−) |
Pharmacokinetic model, cytotoxicity |
— |
164
|
HepG2 (−) |
U 251 (−) |
Vasculature/heart |
HUVEC (+) |
Membrane integration, barrier integrity, cardiac beating, drug screening, barrier alterations |
Transepithelial resistance (TEER), multielectrode arrays (MEAs) |
136
|
Human induced pluripotent stem cell-derived cardiomyocytes (hiPSC-CMs) (+) |
|
Human umbilical cord vascular endothelial cells (+) |
Endothelialized myocardium, real-time and simultaneous assessment of cell barrier function and electrical activity |
Transepithelial resistance (TEER), multielectrode arrays (MEAs) |
165
|
Human induced pluripotent stem cell-derived cardiomyocytes (hiPSC-CMs) (+) |
Initially, the concept of interplay between organ cultures was introduced by Li et al. in devices referred to as integrated discrete multiple organ culture (IdMOC) systems, which were based on a “wells within wells” approach.107 The rationale of IdMOCs is the dynamic interplay between multiple cell types that are cultured in a single well while allowing biochemical communication via the supernatant. To allow more control over the cellular microenvironment and the scaling down of cell cultures, μCCAs advanced IdMOCs by combining multiple cell culture compartments with a microengineered network of fluidic channels that enables precise control over the cellular microenvironment with respect to pharmacology. After a decade of refinement, μCCAs currently enable multi-organ toxicity testing in a four-organ system under continuous-flow conditions in serum for pumpless long-term cell maintenance.131 As a further three-dimensional approach, a reconfigurable microfluidic hanging-drop system has been established for the multiplexed fabrication and analysis of multi-cell organoids.132 This intricate system based on PDMS enables a high degree of flexibility with respect to the size distribution of organoids and the arrangement and interconnection of organs. In summary, an open microfluidic network is combined with a hanging-drop microarray to allow optimal gas exchange during handling of the cell culture while tuning the tissue size and maintaining the functionality and integrity of tissue. Tissue can be formed as hanging-drop cultures in a static fashion, where important parameters, such as the diameter of spheroids, can be controlled as well as monitored in a time-resolved manner. For on-chip experiments, multiple organoid chambers are interconnected and bioassays include drug administration and pharmacology (e.g., bioactivation of prodrugs). Currently, this approach has been upgraded by the integration of peristaltic micropumps133 and electrochemical biosensors (amperometry134 and electrical impedance135) for automated and online multiparametric cell analysis. This device constitutes a promising example of the combination of organ-on-a-chip with lab-on-a-chip concepts to automate the majority of steps in cell culture procedures, including the generation of biomass and tissue and culture maintenance, as well as the time-resolved high-throughput analysis of multiple tissue samples in microarrays. In addition, a further combinatorial approach was established that comprised a multilayered organ-on-a-chip system with integrated electrochemical biosensors, both multielectrode arrays (MEAs) and transepithelial resistance electrodes, on a single chip.136 As a proof of principle for this approach, simultaneous measurements of cellular electrical activity and tissue barrier integrity were carried out in a two-organ system that combined endothelial and beating heart cultures (human cardiomyocytes and primary human endothelial cells). These studies highlighted the simultaneous detection of dynamic alterations in vascular permeability and cardiac function on the same chip. For instance, when the system was challenged with tumour necrosis factor-alpha (TNF-α) and cardiac targeting drugs, the barrier permeability was increased, which thus allowed drug-related changes in cardiac beating rates. In general, such microsystems enable the interactions and thus the retention times of chemicals and drugs with a specific cell type to be tuned by changes in microchannel geometry and can simulate the physiological situation in vivo. In contrast, organ-on-a-chip devices have also been engineered to act as biomimics that simulate the actual mechanical movement and actuation of cell cultures on-chip. For instance, the lung-on-a-chip from the Ingber group is a biomimetic microsystem capable of the mechanical actuation of cultures of lung and vascular cells on-chip via cyclic mechanical strain, which recreates the critical functional alveolar-capillary interface of the human lung.32,137 As a proof of principle, this device has initially been tested as a model of organ-level responses to bacteria and pro-inflammatory cytokines (e.g., asthma), as well as nanomaterials introduced into the alveolar space. The most recent development and advance of this biomimetic device comprised the establishment of a smoker lung-on-a-chip that can recreate the cellular microenvironment of a lung exposed to cigarette smoke via programmable smoking behaviour and hence dosage scenarios. A more recent development is the blinking eye-on-a-chip from the group around Dan Huh.138 This microdevice looks like an actual eye and simulates blinking movements of the eyelid by applying strain at an air–liquid interface. In general, organs-on-a-chip technology appears a promising strategy for the creation of more relevant in vitro models that are applicable in a broad range of scientific fields. Furthermore, more research groups have recently started to work not only on bioengineering but also on biosensing strategies on-chip to gain more control over and insights into these complex models in addition to conventional live-cell microscopy. The only downside to date is that organ-on-a-chip systems mostly comprise a single model per functional chip, which is insufficient and laborious with respect to screening applications.
6. Conclusions and future prospects
Microfluidic systems provide the technology to develop in vivo-like environments for the study of a broad variety of medical models. In this review, we present the latest developments in microfluidic co-culture systems for studies of cell-to-cell interactions, with a focus on cancer research, vascular models, and neuroscience. Here, we give an overview of both 2-dimensional systems and more complex 3-dimensional models and the current technological development of multi-organ-on-a-chip systems. The integration of 3D matrices into microfluidics has enabled the transition from 2D culture models to 3D and multi-organ systems. In cancer biology, decades of bioengineering have resulted in highly complex three-dimensional systems that mimic a variety of different types of cancer, such as breast, lung, liver or bone cancer. The main application areas are anticancer drug screening and fundamental research into cancer metastasis. Fundamental research aims to understand the biological processes behind cancer, tries to create relevant models by applying 3D cell culture techniques to microfluidic devices, and frequently includes drug testing. However, to create clinical relevance, the greatest challenge will be the establishment of high-throughput and high-content analysis within microsystems for testing new drug candidates. An even greater challenge is the standardization of 3D models, as well as the adaptation of this technology for commercial uses and thus to hospitals to provide personalized anticancer drug screening platforms designed for individual patients. The greatest opportunity for microfluidic technology stems from novel emerging therapeutic strategies that are still difficult to analyse with conventional cell analysis techniques such as immunotherapy, cell-based vaccines and cellular gene editing. In neurobiology, most microdevices are based on a similar design principle, but the combination of multidisciplinary research has provided lab-on-a-chip systems with microstructures for cell separation, surface micropatterns that guide the adhesion and migration of cells, and cell manipulators for studies of trauma and regeneration, as well as biosensors for non-invasive monitoring of cell-to-cell interactions. However, neurobiology-on-a-chip still needs to advance from well-established two-dimensional models to more complex and relevant three-dimensional models. The challenge will be to transfer existing knowledge to organ-on-a-chip-like systems with three-dimensional architectures as well as organ-specific functions on a cellular level. The aim of vascular biochip models is fundamental research into angiogenesis as well as vasculogenesis. On the one hand, the screening of biomolecules that promote the formation of vascular systems as well as their regeneration is interesting for research in developmental biology. On the other hand, the screening of inhibitory compounds and drugs may prove valuable for studies in cancer biology, as well as anticancer drug screening. In addition, cell motility and movement across vascular barriers are of great interest, in particular for research into metastasis. In summary, a variety of different hydrogels and matrices have been employed to successfully create 3-dimensional vessels, most frequently fibrin-based gels. Interestingly, none of the presented systems employ on-chip analysis strategies other than standard optical microscopy. The main challenge for on-chip applications lies in the formation of microvasculatures that allow continuous perfusion. Potentially, these microstructures will enable the integration of engineered anastomoses between different organ-on-a-chip models. The state of the art is still flexible endothelialized PDMS channels that are easy to fabricate rather than complex 3-dimensional bioengineered microvasculatures. The emergence of organ-on-a-chip technology has recently revived studies of cell-to-cell interactions on microdevices. Single organs-on-a-chip comprise up to four different cell types (primary as well as cancer cell lines), which provide the organ functions within the microsystems. Some create organ functions from the chip itself using mechanical stimuli by integrating actuated flexible membranes. Others rely on classical bioengineering, including the self-assembly and organization of different cell types and thus cell-to-cell interactions, to create actual organ functions on a chip. Most recent developments interconnect multiple organs within a single chip to recreate human physiology in so-called human- or body-on-a-chip systems. In summary, only a few of the presented systems can be categorized as lab-on-a-chip systems, which can only be achieved by a combination of complex biology with integrated biosensors for detecting, for example, electrical activity, TEER, impedance, oxygen, lactate, and glucose.
To conclude, microfluidics is a powerful tool and set of techniques for controlling and analysing cell-to-cell interactions on different levels of complexity, such as single cells as well as microtissues. However, most of these efforts are still proofs of principle rather than fully developed and broadly applicable alternatives to existing models. To create relevant microfluidic systems for studies of cell-to-cell interactions, the integration of primary cell models in combination with standardized microdevices is desirable. Both primary cell models and plastic microchips are readily available for research purposes, and a variety of companies are readily commercializing biochips, organ-on-a-chips, and in vitro cell models, as well as a variety of biosensors. Future efforts may lie more in the selection and combination of standardized modules and models and their application within microfluidic chips to generate more human-like biological features.
Conflicts of interest
There are no conflicts to declare.
Acknowledgements
The authors acknowledge the TU Wien University Library for financial support through its Open Access Funding Programme, the European Union's Horizon 2020 research and innovation programme (685817) and the Austrian Research Promotion Agency (FFG; 849791).
Notes and references
-
G. M. Cooper, The Cell: A Molecular Approach, Sinauer Associates, 2nd edn, 2000 Search PubMed.
- N. V. Menon, Y. J. Chuah, B. Cao, M. Lim and Y. Kang, Biomicrofluidics, 2014, 8, 064118 CrossRef PubMed.
- E. Cukierman, R. Pankov and K. M. Yamada, Curr. Opin. Cell Biol., 2002, 14, 633–639 CrossRef CAS PubMed.
- K. M. Yamada and E. Cukierman, Cell, 2007, 130, 601–610 CrossRef CAS PubMed.
- Y. Shin, S. Han, J. S. Jeon, K. Yamamoto, I. K. Zervantonakis, R. Sudo, R. D. Kamm and S. Chung, Nat. Protoc., 2012, 7, 1247–1259 CrossRef CAS PubMed.
- G. S. Fiorini and D. T. Chiu, BioTechniques, 2005, 38, 429–446 CrossRef CAS PubMed.
- V. Dragone, V. Sans, M. H. Rosnes, P. J. Kitson and L. Cronin, Beilstein J. Org. Chem., 2013, 9, 951–959 CrossRef CAS PubMed.
- D. J. Harrison, A. Manz, Z. H. Fan, H. Ludi and H. M. Widmer, Anal. Chem., 1992, 64, 1926–1932 CrossRef CAS.
- A. Manz, D. J. Harrison, E. M. J. Verpoorte, J. C. Fettinger, A. Paulus, H. Ludi and H. M. Widmer, J. Chromatogr., 1992, 593, 253–258 CrossRef CAS.
- G. S. Fiorini, G. D. Jeffries, D. S. Lim, C. L. Kuyper and D. T. Chiu, Lab Chip, 2003, 3, 158–163 RSC.
- G. S. Fiorini, R. M. Lorenz, J. S. Kuo and D. T. Chiu, Anal. Chem., 2004, 76, 4697–4704 CrossRef CAS PubMed.
- D. C. Duffy, J. C. McDonald, O. J. A. Schueller and G. M. Whitesides, Anal. Chem., 1998, 70, 4974–4984 CrossRef CAS PubMed.
-
C. D. Rudd, C. M. Duffy, M. S. Johnson and P. J. Blanchard, Broader Meaning to Thermosets, 1998, pp. 177–177 Search PubMed.
- A. P. Golden and J. Tien, Lab Chip, 2007, 7, 720–725 RSC.
- R. Novak, N. Ranu and R. A. Mathies, Lab Chip, 2013, 13, 1468–1471 RSC.
- Y. N. Xia and G. M. Whitesides, Annu. Rev. Mater. Sci., 1998, 28, 153–184 CrossRef CAS.
- P. Mitchell, Nat. Biotechnol., 2001, 19, 717–721 CrossRef CAS PubMed.
- S. R. Quake and A. Scherer, Science, 2000, 290, 1536–1540 CrossRef CAS PubMed.
- L. Wang, L. Lei, X. F. Ni, J. Shi and Y. Chen, Microelectron. Eng., 2009, 86, 1462–1464 CrossRef CAS.
- M. Reichen, F. S. Veraitch and N. Szita, J. Lab. Autom., 2013, 18, 519–529 CrossRef CAS PubMed.
- M. A. McClain, C. T. Culbertson, S. C. Jacobson and J. M. Ramsey, Anal. Chem., 2001, 73, 5334–5338 CrossRef CAS PubMed.
- H. Andersson and A. van den Berg, Sens. Actuators, B, 2003, 92, 315–325 CrossRef CAS.
- V. Charwat, M. Purtscher, S. F. Tedde, O. Hayden and P. Ertl, Lab Chip, 2013, 13, 785–797 RSC.
- B. Ungerbock, V. Charwat, P. Ertl and T. Mayr, Lab Chip, 2013, 13, 1593–1601 RSC.
- T. Vilkner, D. Janasek and A. Manz, Anal. Chem., 2004, 76, 3373–3385 CrossRef CAS PubMed.
- C. Yi, Q. Zhang, C. W. Li, J. Yang, J. Zhao and M. Yang, Anal. Bioanal. Chem., 2006, 384, 1259–1268 CrossRef CAS PubMed.
- J. Y. Park, S. J. Yoo, C. M. Hwang and S.-H. Lee, Lab Chip, 2009, 9, 2194–2202 RSC.
- C. A. Simmons, S. Matlis, A. J. Thornton, S. Chen, C.-Y. Wang and D. J. Mooney, J. Biomech., 2003, 36, 1087–1096 CrossRef PubMed.
- S. Shojaei, M. Tafazzoli-Shahdpour, M. Shokrgozar and N. Haghighipour, Int. J. Artif. Organs, 2013, 36, 663–673 CrossRef PubMed.
- J. S. Park, J. S. Chu, C. Cheng, F. Chen, D. Chen and S. Li, Biotechnol. Bioeng., 2004, 88, 359–368 CrossRef CAS PubMed.
- L. A. McMahon, A. J. Reid, V. A. Campbell and P. J. Prendergast, Ann. Biomed. Eng., 2008, 36, 185–194 CrossRef PubMed.
- D. Huh, B. D. Matthews, A. Mammoto, M. Montoya-Zavala, H. Y. Hsin and D. E. Ingber, Science, 2010, 328, 1662–1668 CrossRef CAS PubMed.
- K. Svennersten, M. Berggren, A. Richter-Dahlfors and E. W. H. Jager, Lab Chip, 2011, 11, 3287–3293 RSC.
- T. C. Hausherr, H. Majd, D. Joss, A. Müller, D. P. Pioletti, M. A. M. Gijs and C. Yamahata, Sens. Actuators, B, 2013, 188, 1019–1025 CrossRef CAS.
- C. Y. Bae, M. S. Kim and J.-K. Park, BioChip J., 2011, 5, 106–113 CrossRef CAS.
- C. Guguen-Guillouzo, B. Clement, G. Baffet, C. Beaumont, E. Morel-Chany, D. Glaise and A. Guillouzo, Exp. Cell Res., 1983, 143, 47–54 CrossRef CAS PubMed.
- J. Zheng, Q. Liu, J. Yang, Q. Ren, W. Cao, Z. Yu, F. Yu, Y. Wu, H. Shi and W. Liu, Braz. J. Med. Biol. Res., 2012, 45, 510–515 CrossRef CAS PubMed.
- V. Charwat, M. Rothbauer, S. F. Tedde, O. Hayden, J. J. Bosch, P. Muellner, R. Hainberger and P. Ertl, Anal. Chem., 2013, 85, 11471–11478 CrossRef CAS PubMed.
- D. Talavera-Adame, G. Wu, Y. He, T. T. Ng, A. Gupta, S. Kurtovic, J. Y. Hwang, D. L. Farkas and D. C. Dafoe, Stem Cell Rev. Rep., 2011, 7, 532–543 CrossRef CAS PubMed.
- D. Wartmann, M. Rothbauer, O. Kuten, C. Barresi, C. Visus, T. Felzmann and P. Ertl, Frontiers in Materials, 2015, 2 DOI:10.3389/fmats.2015.00060.
- T. Q. Vu, R. M. B. de Castro and L. D. Qin, Lab Chip, 2017, 17, 1009–1023 RSC.
- Q. Ramadan and M. A. Gijs, Lab Chip, 2015, 15, 614–636 RSC.
- M. Rothbauer, P. Ertl, B. A. Theiler, M. Schlager, U. B. Sleytr and S. Kupcu, Adv. Mater. Interfaces, 2015, 2(1), 1400309 CrossRef.
- F. Chien-Yu, T. Sheng-Yang, Y. Shih-Mo, H. Long, L. Cheng-Hsien and C. Hwan-You, Biofabrication, 2014, 6, 015009 CrossRef PubMed.
- K. Ziółkowska, R. Kwapiszewski, A. Stelmachowska, M. Chudy, A. Dybko and Z. Brzózka, Sens. Actuators, B, 2012, 173, 908–913 CrossRef.
- H.-J. Jin, Y.-H. Cho, J.-M. Gu, J. Kim and Y.-S. Oh, Lab Chip, 2011, 11, 115–119 RSC.
- M. Moya, D. Tran and S. C. George, Stem Cell Res. Ther., 2013, 4(Suppl 1), S15 CrossRef PubMed.
- H. Ma, T. Liu, J. Qin and B. Lin, Electrophoresis, 2010, 31, 1599–1605 CrossRef CAS PubMed.
- E. Agliari, E. Biselli, A. De Ninno, G. Schiavoni, L. Gabriele, A. Gerardino, F. Mattei, A. Barra and L. Businaro, Sci. Rep., 2014, 4, 6639 CrossRef CAS PubMed.
- E. Vacchelli, Y. Ma, E. E. Baracco, A. Sistigu, D. P. Enot, F. Pietrocola, H. Yang, S. Adjemian, K. Chaba, M. Semeraro, M. Signore, A. De Ninno, V. Lucarini, F. Peschiaroli, L. Businaro, A. Gerardino, G. Manic, T. Ulas, P. Günther, J. L. Schultze, O. Kepp, G. Stoll, C. Lefebvre, C. Mulot, F. Castoldi, S. Rusakiewicz, S. Ladoire, L. Apetoh, J. M. Bravo-San Pedro, M. Lucattelli, C. Delarasse, V. Boige, M. Ducreux, S. Delaloge, C. Borg, F. André, G. Schiavoni, I. Vitale, P. Laurent-Puig, F. Mattei, L. Zitvogel and G. Kroemer, Science, 2015, 350, 972–978 CrossRef CAS PubMed.
- Y. Huang, B. Agrawal, P. A. Clark, J. C. Williams and J. S. Kuo, J. Visualized Exp., 2011, 3297, DOI:10.3791/3297.
- S. Reuter, S. C. Gupta, M. M. Chaturvedi and B. B. Aggarwal, Free Radical Biol. Med., 2010, 49, 1603–1616 CrossRef CAS PubMed.
-
M. Rothbauer, S. Kupcu, U. B. Sleytr and P. Ertl, Ifmbe Proc, 2015, vol. 45, pp. 337–340 Search PubMed.
- M. Rothbauer, S. Kupcu, D. Sticker, U. B. Sleytr and P. Ertl, ACS Nano, 2013, 7, 8020–8030 CrossRef CAS PubMed.
- P.-f. Liu, Y.-w. Cao, S.-d. Zhang, Y. Zhao, X.-g. Liu, H.-q. Shi, K.-y. Hu, G.-q. Zhu, B. Ma and H.-t. Niu, Oncotarget, 2015, 6, 37695–37705 Search PubMed.
- L. Businaro, A. De Ninno, G. Schiavoni, V. Lucarini, G. Ciasca, A. Gerardino, F. Belardelli, L. Gabriele and F. Mattei, Lab Chip, 2013, 13, 229–239 RSC.
- F. Mattei, G. Schiavoni, A. De Ninno, V. Lucarini, P. Sestili, A. Sistigu, A. Fragale, M. Sanchez, M. Spada, A. Gerardino, F. Belardelli, L. Businaro and L. Gabriele, J. Immunotoxicol., 2014, 11, 337–346 CrossRef CAS PubMed.
- Y. Gao, D. Majumdar, B. Jovanovic, C. Shaifer, P. C. Lin, A. Zijlstra, D. J. Webb and D. Li, Biomed. Microdevices, 2011, 13, 539–548 CrossRef CAS PubMed.
- C. Zheng, L. Zhao, G. E. Chen, Y. Zhou, Y. Pang and Y. Huang, Anal. Chem., 2012, 84, 2088–2093 CrossRef CAS PubMed.
- K. E. Sung, N. Yang, C. Pehlke, P. J. Keely, K. W. Eliceiri, A. Friedl and D. J. Beebe, Integr. Biol., 2011, 3, 439–450 RSC.
- T. Liu, B. Lin and J. Qin, Lab on a Chip, 2010, 10, 1671–1677 RSC.
- S. Bersini, J. S. Jeon, G. Dubini, C. Arrigoni, S. Chung, J. L. Charest, M. Moretti and R. D. Kamm, Biomaterials, 2014, 35, 2454–2461 CrossRef CAS PubMed.
- I. K. Zervantonakis, S. K. Hughes-Alford, J. L. Charest, J. S. Condeelis, F. B. Gertler and R. D. Kamm, Proc. Natl. Acad. Sci. U. S. A., 2012, 109, 13515–13520 CrossRef PubMed.
- A. D. Wong and P. C. Searson, Cancer Res., 2014, 74, 4937–4945 CrossRef CAS PubMed.
- M. Ye, H. M. Sanchez, M. Hultz, Z. Yang, M. Bogorad, A. D. Wong and P. C. Searson, Sci. Rep., 2014, 4, 4681 CrossRef PubMed.
- S. M. Ehsan, K. M. Welch-Reardon, M. L. Waterman, C. C. W. Hughes and S. C. George, Integr. Biol., 2014, 6, 603–610 RSC.
- Y. Choi, J. Hyun, J. Seo, C. Blundell, H. C. Kim, E. Lee, S. H. Lee, A. Moon, W. K. Moon and D. Huh, Lab Chip, 2015, 15, 3350–3357 RSC.
- C. F. Buchanan, E. E. Voigt, C. S. Szot, J. W. Freeman, P. P. Vlachos and M. N. Rylander, Tissue Eng., Part C, 2014, 20, 64–75 CrossRef CAS PubMed.
- P. Carmeliet and R. K. Jain, Nature, 2000, 407, 249–257 CrossRef CAS PubMed.
- Z. Xu, Y. Gao, Y. Hao, E. Li, Y. Wang, J. Zhang, W. Wang, Z. Gao and Q. Wang, Biomaterials, 2013, 34, 4109–4117 CrossRef CAS PubMed.
- K. Kwapiszewska, A. Michalczuk, M. Rybka, R. Kwapiszewski and Z. Brzozka, Lab Chip, 2014, 14, 2096–2104 RSC.
- M. Bauer, G. Su, D. J. Beebe and A. Friedl, Integr. Biol., 2010, 2, 371–378 RSC.
- Y. Imura, K. Sato and E. Yoshimura, Anal. Chem., 2010, 82, 9983–9988 CrossRef CAS PubMed.
- Y. Imura, E. Yoshimura and K. Sato, Anal. Sci., 2012, 28, 197 CrossRef CAS PubMed.
- J. H. Sung and M. L. Shuler, Lab Chip, 2009, 9, 1385–1394 RSC.
- G. Adriani, A. Pavesi, A. T. Tan, A. Bertoletti, J. P. Thiery and R. D. Kamm, Drug Discovery Today, 2016, 21, 1472–1478 CrossRef CAS PubMed.
- L. Jeanbart and M. A. Swartz, Proc. Natl. Acad. Sci. U. S. A., 2015, 112, 14467–14472 CrossRef CAS PubMed.
- S. Parlato, A. De Ninno, R. Molfetta, E. Toschi, D. Salerno, A. Mencattini, G. Romagnoli, A. Fragale, L. Roccazzello, M. Buoncervello, I. Canini, E. Bentivegna, M. Falchi, F. R. Bertani, A. Gerardino, E. Martinelli, C. Natale, R. Paolini, L. Businaro and L. Gabriele, Sci. Rep., 2017, 7, 1093 CrossRef PubMed.
- A. Pavesi, A. T. Tan, S. Koh, A. Chia, M. Colombo, E. Antonecchia, C. Miccolis, E. Ceccarello, G. Adriani, M. T. Raimondi, R. D. Kamm and A. Bertoletti, JCI Insight, 2017, 2(12), 89762 Search PubMed.
- L. Berdondini, M. Chiappalone, P. D. van der Wal, K. Imfeld, N. F. de Rooij, M. Koudelka-Hep, M. Tedesco, S. Martinoia, J. van Pelt, G. Le Masson and A. Garenne, Sens. Actuators, B, 2006, 114, 530–541 CrossRef CAS.
- L. J. Millet and M. U. Gillette, Yale J. Biol. Med., 2012, 85, 501–521 Search PubMed.
- A. K. Soe, S. Nahavandi and K. Khoshmanesh, Biosens. Bioelectron., 2012, 35, 1–13 CrossRef CAS PubMed.
- A. M. Taylor, D. C. Dieterich, H. T. Ito, S. A. Kim and E. M. Schuman, Neuron, 2010, 66, 57–68 CrossRef CAS PubMed.
- H. S. Shin, H. J. Kim, S. K. Min, S. H. Kim, B. M. Lee and N. L. Jeon, Biotechnol. Lett., 2010, 32, 1063–1070 CrossRef CAS PubMed.
- A. M. Taylor and N. L. Jeon, Curr. Opin. Neurobiol., 2010, 20, 640–647 CrossRef CAS PubMed.
- H. Higashimori and Y. Yang, J. Visualized Exp., 2012, 68 DOI:10.3791/4448.
- H. J. Kim, J. W. Park, J. W. Park, J. H. Byun, B. Vahidi, S. W. Rhee and N. L. Jeon, Ann. Biomed. Eng., 2012, 40, 1268–1276 CrossRef PubMed.
- E. E. Zahavi, A. Ionescu, S. Gluska, T. Gradus, K. Ben-Yaakov and E. Perlson, J. Cell Sci., 2015, 128, 1241–1252 CrossRef CAS PubMed.
- J. Park, H. Koito, J. Li and A. Han, Lab Chip, 2012, 12, 3296–3304 RSC.
- D. Majumdar, Y. Gao, D. Li and D. J. Webb, J. Neurosci. Methods, 2011, 196, 38–44 CrossRef PubMed.
- M. J. Shi, D. Majumdar, Y. D. Gao, B. M. Brewer, C. R. Goodwin, J. A. McLean, D. Lib and D. J. Webb, Lab Chip, 2013, 13, 3008–3021 RSC.
- E. Marconi, T. Nieus, A. Maccione, P. Valente, A. Simi, M. Messa, S. Dante, P. Baldelli, L. Berdondini and F. Benfenati, PLoS One, 2012, 7, e34648 CAS.
- A. Petrelli, E. Marconi, M. Salerno, D. De Pietri Tonelli, L. Berdondini and S. Dante, Lab Chip, 2013, 13, 4419–4429 RSC.
- Y. Huang, J. C. Williams and S. M. Johnson, Lab Chip, 2012, 12, 2103–2117 RSC.
- A. Simi, H. Amin, A. Maccione, T. Nieus and L. Berdondini, Prog. Brain Res., 2014, 214, 415–442 Search PubMed.
- K. Schimek, M. Busek, S. Brincker, B. Groth, S. Hoffmann, R. Lauster, G. Lindner, A. Lorenz, U. Menzel, F. Sonntag, H. Walles, U. Marx and R. Horland, Lab Chip, 2013, 13, 3588–3598 RSC.
- D. Sticker, M. Rothbauer, S. Lechner, M. T. Hehenberger and P. Ertl, Lab Chip, 2015, 15, 4542–4554 RSC.
- J. H. Yeon, H. R. Ryu, M. Chung, Q. P. Hu and N. L. Jeon, Lab Chip, 2012, 12, 2815–2822 RSC.
- S. Kim, H. Lee, M. Chung and N. L. Jeon, Lab Chip, 2013, 13, 1489–1500 RSC.
- A. Sobrino, D. T. Phan, R. Datta, X. Wang, S. J. Hachey, M. Romero-Lopez, E. Gratton, A. P. Lee, S. C. George and C. C. Hughes, Sci. Rep., 2016, 6, 31589 CrossRef CAS PubMed.
- J. A. Whisler, M. B. Chen and R. D. Kamm, Tissue Eng., Part C, 2014, 20, 543–552 CrossRef CAS PubMed.
- M. L. Moya, Y.-H. Hsu, A. P. Lee, C. C. W. Hughes and S. C. George, Tissue Eng., Part C, 2013, 19, 730–737 CrossRef CAS PubMed.
-
M. Purtscher, M. Rothbauer, W. Holnthoner, H. Redl and P. Ertl, Ifmbe Proc, 2015, vol. 45, pp. 313–317 Search PubMed.
- A. D. van der Meer, V. V. Orlova, P. ten Dijke, A. van den Berg and C. L. Mummery, Lab Chip, 2013, 13, 3562–3568 RSC.
- S. S. Verbridge, A. Chakrabarti, P. DelNero, B. Kwee, J. D. Varner, A. D. Stroock and C. Fischbach, J. Biomed. Mater. Res., Part A, 2013, 101, 2948–2956 CrossRef PubMed.
- A. Tourovskaia, M. Fauver, G. Kramer, S. Simonson and T. Neumann, Exp. Biol. Med., 2014, 239, 1264–1271 CrossRef PubMed.
- D. H. Nguyen, S. C. Stapleton, M. T. Yang, S. S. Cha, C. K. Choi, P. A. Galie and C. S. Chen, Proc. Natl. Acad. Sci. U. S. A., 2013, 110, 6712–6717 CrossRef CAS PubMed.
- R. Li, X. Lv, X. Zhang, O. Saeed and Y. Deng, Frontiers of Chemical Science and Engineering, 2015, 10, 90–98 CrossRef.
- S. Hong, Q. Pan and L. P. Lee, Integr. Biol., 2012, 4, 374–380 RSC.
- T. Q. Vu, R. M. de Castro and L. Qin, Lab Chip, 2017, 17, 1009–1023 RSC.
- J. R. Rettig and A. Folch, Anal. Chem., 2005, 77, 5628–5634 CrossRef CAS PubMed.
- Z. Z. Yin, D. Noren, C. J. Wang, R. Hang and A. Levchenko, Mol. Syst. Biol., 2008, 4, 232 CrossRef PubMed.
- F. Guo, Z. M. Mao, Y. C. Chen, Z. W. Xie, J. P. Lata, P. Li, L. Q. Ren, J. Y. Liu, J. Yang, M. Dao, S. Suresh and T. J. Huang, Proc. Natl. Acad. Sci. U. S. A., 2016, 113, 1522–1527 CrossRef CAS PubMed.
- T. P. Lagus and J. F. Edd, RSC Adv., 2013, 3, 20512–20522 RSC.
- A. M. Skelley, O. Kirak, H. Suh, R. Jaenisch and J. Voldman, Nat. Methods, 2009, 6, 147–152 CrossRef CAS PubMed.
- Y. C. Chen, P. Ingram and E. Yoon, Analyst, 2014, 139, 6371–6378 RSC.
- D. R. Hofer, E. R. Sherwood, W. D. Bromberg, J. Mendelsohn, C. Lee and J. M. Kozlowski, Cancer Res., 1991, 51, 2780–2785 CAS.
- K. Zhang, C. K. Chou, X. Xia, M. C. Hung and L. Qin, Proc. Natl. Acad. Sci. U. S. A., 2014, 111, 2948–2953 CrossRef CAS PubMed.
- Y. T. Lu, G. P. Pendharkar, C. H. Lu, C. M. Chang and C. H. Liu, Oncotarget, 2015, 6, 38764–38776 Search PubMed.
- X. Han, Z. Liu, Y. Ma, K. Zhang and L. Qin, Adv. Biosys., 2017, 1 DOI:10.1002/adbi.201600007.
- Š. Selimović, M. R. Dokmeci and A. Khademhosseini, Curr. Opin. Pharmacol., 2013, 13, 829–833 CrossRef PubMed.
- N. S. Bhise, J. Ribas, V. Manoharan, Y. S. Zhang, A. Polini, S. Massa, M. R. Dokmeci and A. Khademhosseini, J. Controlled Release, 2014, 190, 82–93 CrossRef CAS PubMed.
- J. D. Caplin, N. G. Granados, M. R. James, R. Montazami and N. Hashemi, Adv. Healthcare Mater., 2015, 4, 1426–1450 CrossRef CAS PubMed.
- E. W. Esch, A. Bahinski and D. Huh, Nat. Rev. Drug Discovery, 2015, 14, 248–260 CrossRef CAS PubMed.
- M. Rothbauer, D. Wartmann, V. Charwat and P. Ertl, Biotechnol. Adv., 2015, 33, 948–961 CrossRef PubMed.
- M. Baker, Nature, 2011, 471, 661–665 CrossRef CAS PubMed.
- D. Huh, G. A. Hamilton and D. E. Ingber, Trends Cell Biol., 2011, 21, 745–754 CrossRef CAS PubMed.
- D. Huh, Y.-s. Torisawa, G. A. Hamilton, H. J. Kim and D. E. Ingber, Lab Chip, 2012, 12, 2156–2164 RSC.
- A. P. Li, C. Bode and Y. Sakai, Chem.-Biol. Interact., 2004, 150, 129–136 CrossRef CAS PubMed.
- A. Sin, K. C. Chin, M. F. Jamil, Y. Kostov, G. Rao and M. L. Shuler, Biotechnol. Prog., 2004, 20, 338–345 CrossRef CAS PubMed.
- C. Oleaga, C. Bernabini, A. S. T. Smith, B. Srinivasan, M. Jackson, W. McLamb, V. Platt, R. Bridges, Y. Cai, N. Santhanam, B. Berry, S. Najjar, N. Akanda, X. Guo, C. Martin, G. Ekman, M. B. Esch, J. Langer, G. Ouedraogo, J. Cotovio, L. Breton, M. L. Shuler and J. J. Hickman, Sci. Rep., 2016, 6, 20030 CrossRef CAS PubMed.
- O. Frey, P. M. Misun, D. A. Fluri, J. G. Hengstler and A. Hierlemann, Nat. Commun., 2014, 5, 4250 CAS.
- S. R. Yazdi, A. Shadmani, S. C. Burgel, P. M. Misun, A. Hierlemann and O. Frey, Lab Chip, 2015, 15, 4138–4147 RSC.
- P. M. Misun, J. Rothe, Y. R. F. Schmid, A. Hierlemann and O. Frey, Microsyst. Nanoeng., 2016, 2, 16022 CrossRef CAS.
- Y. R. F. Schmid, S. C. Bürgel, P. M. Misun, A. Hierlemann and O. Frey, ACS Sensors, 2016, 1, 1028–1035 CrossRef CAS.
- B. M. Maoz, A. Herland, O. Y. F. Henry, W. D. Leineweber, M. Yadid, J. Doyle, R. Mannix, V. J. Kujala, E. A. FitzGerald, K. K. Parker and D. E. Ingber, Lab Chip, 2017, 17, 2294–2302 RSC.
- D. Huh, D. C. Leslie, B. D. Matthews, J. P. Fraser, S. Jurek, G. A. Hamilton, K. S. Thorneloe, M. A. McAlexander and D. E. Ingber, Sci. Transl. Med., 2012, 4, 159ra147 Search PubMed.
- J. Seo, W. Y. Byun, A. Frank, M. Massaro-Giordano, V. Lee, V. Y. Bunya and D. Huh, Invest. Ophthalmol. Visual Sci., 2016, 57, 3872 Search PubMed.
- R. Booth and H. Kim, Lab Chip, 2012, 12, 1784–1792 RSC.
- L. M. Griep, F. Wolbers, B. de Wagenaar, P. M. ter Braak, B. B. Weksler, I. A. Romero, P. O. Couraud, I. Vermes, A. D. van der Meer and A. van den Berg, Biomed. Microdevices, 2013, 15, 145–150 CrossRef CAS PubMed.
- H. J. Kim, D. Huh, G. Hamilton and D. E. Ingber, Lab Chip, 2012, 12, 2165–2174 RSC.
- O. Frey, P. M. Misun, J. Rothe and A. Hierlemann, Procedia Eng., 2014, 87, 96–99 CrossRef CAS.
-
P. M. Misun, J. Rothe, A. Hierlemann and O. Frey, 2015 Transducers - 2015 18th International Conference on Solid-State Sensors, Actuators and Microsystems, 2015, pp. 1625–1628 Search PubMed.
- K.-J. Jang, A. P. Mehr, G. A. Hamilton, L. A. McPartlin, S. Chung, K.-Y. Suh and D. E. Ingber, Integr. Biol., 2013, 5, 1119–1129 RSC.
- K.-J. Jang and K.-Y. Suh, Lab Chip, 2010, 10, 36–42 RSC.
- E. J. Weber, A. Chapron, B. D. Chapron, J. L. Voellinger, K. A. Lidberg, C. K. Yeung, Z. Wang, Y. Yamaura, D. W. Hailey, T. Neumann, D. D. Shen, K. E. Thummel, K. A. Muczynski, J. Himmelfarb and E. J. Kelly, Kidney Int., 2016, 90, 627–637 CrossRef PubMed.
- P. Pagella, E. Neto, L. Jimenez-Rojo, M. Lamghari and T. A. Mitsiadis, Front. Physiol., 2014, 5, 326 Search PubMed.
-
S. C. Burgel, Y. Schmid, I. Agarkova, D. A. Fluri, J. M. Kelm, A. Hierlemann and O. Frey, 2015 28th Ieee International Conference on Micro Electro Mechanical Systems (Mems 2015), 2015, pp. 226–229 Search PubMed.
- A. Marsano, C. Conficconi, M. Lemme, P. Occhetta, E. Gaudiello, E. Votta, G. Cerino, A. Redaelli and M. Rasponi, Lab Chip, 2016, 16, 599–610 RSC.
- K. Domansky, W. Inman, J. Serdy, A. Dash, M. H. M. Lim and L. G. Griffith, Lab Chip, 2010, 10, 51–58 RSC.
- S.-A. Lee, D. Y. No, E. Kang, J. Ju, D.-S. Kim and S.-H. Lee, Lab Chip, 2013, 13, 3529–3537 RSC.
-
S. R. Yazdi, A. Shadmani, A. Hierlemann and O. Frey, 2015 28th Ieee International Conference on Micro Electro Mechanical Systems (Mems 2015), 2015, pp. 535–538 Search PubMed.
- S. Y. Chang, J. L. Voellinger, K. P. Van Ness, B. Chapron, R. M. Shaffer, T. Neumann, C. C. White, T. J. Kavanagh, E. J. Kelly and D. L. Eaton, Toxicol. In Vitro, 2017, 40, 170–183 CrossRef CAS PubMed.
- J. S. Lee, R. Romero, Y. M. Han, H. C. Kim, C. J. Kim, J. S. Hong and D. Huh, J. Matern.-Fetal Neonat. Med., 2016, 29, 1046–1054 CrossRef CAS PubMed.
-
S. Miura, Y. Morimoto and S. Takeuchi, Proc Ieee Micr Elect, 2013, pp. 257–258 Search PubMed.
- S. Miura, K. Sato, M. Kato-Negishi, T. Teshima and S. Takeuchi, Nat. Commun., 2015, 6 Search PubMed.
- C. Blundell, E. R. Tess, A. S. R. Schanzer, C. Coutifaris, E. J. Su, S. Parry and D. Huh, Lab Chip, 2016, 16, 3065–3073 RSC.
- H. Kaji, T. Yokoi, T. Kawashima and M. Nishizawa, Lab Chip, 2010, 10, 2374–2379 RSC.
- Y. B. Kang, T. R. Sodunke, J. Lamontagne, J. Cirillo, C. Rajiv, M. J. Bouchard and M. Noh, Biotechnol. Bioeng., 2015, 112, 2571–2582 CrossRef CAS PubMed.
- I. Maschmeyer, A. K. Lorenz, K. Schimek, T. Hasenberg, A. P. Ramme, J. Hubner, M. Lindner, C. Drewell, S. Bauer, A. Thomas, N. S. Sambo, F. Sonntag, R. Lauster and U. Marx, Lab Chip, 2015, 15, 2688–2699 RSC.
- I. Wagner, E.-M. Materne, S. Brincker, C. Fradrich Su, M. Busek, F. Sonntag, D. A. Sakharov, E. V. Trushkin, A. G. Tonevitsky, R. Lauster and U. Marx, Lab Chip, 2013, 13, 3538–3547 RSC.
- T. Herricks, K. B. Seydel, G. Turner, M. Molyneux, R. Heyderman, T. Taylor and P. K. Rathod, Lab Chip, 2011, 11, 2994–3000 RSC.
- J. H. Sung, C. Kam and M. L. Shuler, Lab Chip, 2010, 10, 446–455 RSC.
- M. Jie, H.-F. Li, L. Lin, J. Zhang and J.-M. Lin, RSC Adv., 2016, 6, 54564–54572 RSC.
- B. M. Maoz, A. Herland, O. Y. F. Henry, W. D. Leineweber, M. Yadid, J. Doyle, R. Mannix, V. J. Kujala, E. A. FitzGerald, K. K. Parker and D. E. Ingber, Lab Chip, 2017, 17, 2294–2302 RSC.
|
This journal is © The Royal Society of Chemistry 2018 |
Click here to see how this site uses Cookies. View our privacy policy here.