DOI:
10.1039/D1SC00512J
(Edge Article)
Chem. Sci., 2021,
12, 9022-9030
Translating daily COVID-19 screening into a simple glucose test: a proof of concept study†
Received
27th January 2021
, Accepted 25th May 2021
First published on 25th May 2021
Abstract
Home testing is an attractive emerging strategy to combat the COVID-19 pandemic and prevent overloading of healthcare resources through at-home isolation, screening and monitoring of symptoms. However, current diagnostic technologies of SARS-CoV-2 still suffer from some drawbacks because of the tradeoffs between sensitivity, usability and costs, making the test unaffordable to most users at home. To address these limitations, taking advantage of clustered regularly interspaced short palindromic repeats (CRISPRs) and a portable glucose meter (PGM), we present a proof-of-concept demonstration of a target-responsive CRISPR-PGM system for translating SARS-CoV-2 detection into a glucose test. Using this system, a specific N gene, N protein, and pseudo-viruses of SARS-CoV-2 have been detected quantitatively with a PGM. Given the facile integration of various bioreceptors into the CRISPR-PGM system, the proposed method provides a starting point to provide patients with a single-device solution that can quantitatively monitor multiple COVID-19 biomarkers at home.
Introduction
The recent COVID-19 pandemic has highlighted the significance of accurate and reliable diagnostic technologies for control and prevention of virus spread.1–4 Current diagnostic testing of SARS-CoV-2 mainly includes the detection of nucleic acids, antigens, and antibodies using different approaches, such as real-time quantitative polymerase chain reaction (RT-qPCR),5 optical immunoassay,6,7 lateral flow assays,8–10 automated microfluidic devices,11,12 and clustered regularly interspaced short palindromic repeat (CRISPR)-associated methods.13–18 Despite the progress, most of these systems still suffer from some drawbacks because of the tradeoffs between sensitivity, usability and costs, making the test unaffordable to most users at home.19,20 Moreover, a most recent investigation demonstrated that effective COVID-19 screening is mainly dominated by the test frequency and speed and is only marginally improved by high test sensitivity.21 Thus, in addition to sensitivity and specificity, the focus on developing new COVID-19 detection methods has shifted to cost, turnaround time and ease of use by unskilled operators in a resource-limited setting.
Home testing is an attractive strategy to combat the COVID-19 pandemic and prevent overloading of healthcare resources through at-home isolation, COVID-19 screening, and monitoring of symptoms.22–24 In addition, once mass vaccination has been initiated, routine monitoring of SARS-CoV-2 will be necessary but facing significant challenges as the virus can mutate and may render the vaccine less effective.25 In this context, bringing the SARS-CoV-2 detection from centralized laboratories to point-of-care (POC) or even the patients themselves for self-monitoring is essential to increase prevention and treatment effectiveness.26,27 To achieve this purpose, some portable devices have been developed and applied for rapid and sensitive diagnosis of SARS-CoV-2 at the POC.28,29 Although promising, the user-friendliness and cost of these devices cannot fulfill the critical requirements of home self-testing, limiting their marketability.30 Therefore, developing and commercializing a single device with the required sensitivity, specificity, versatility and adaptability for diverse targets, low-cost, portability, and more importantly amenability for self-testing remains an unmet need that currently available diagnostic approaches cannot provide.31,32
The portable glucose meter (PGM) is arguably the most widely available POC device on the market with several features of good portability and usability, low cost, and reliable quantitative results with connectivity to mHealth networks.33 In this regard, the PGM would be an ideal alternative to laboratory-based devices for the self-monitoring of SARS-CoV-2 and related biomarkers in the field or at home, but a link between the glucose concentration and target concentrations must be established in advance. A major challenge in using a PGM for SARS-CoV-2 diagnosis is the inherent poor sensitivity (∼0.6–33 mM glucose),34 and therefore it is not suitable for the detection of COVID-19 biomarkers with low abundance (∼pM). To address this issue, we and others have incorporated many signal amplification strategies into the glucose meter for POC quantification of numerous targets.34–39 Despite these successes, these new strategies come with tradeoffs in terms of sensitivity, specificity and turnaround time. Therefore, there is still a need for the discovery of new strategies to improve the sensitivity and specificity of PGM-based sensors, especially for the detection of trace amounts of nucleic acids or protein targets of SARS-CoV-2.
Here, we report the development of a target-responsive CRISPR-PGM system for translating SARS-CoV-2 detection into a glucose test. The system consists of two programmable modular components that can be triggered by viral RNA of SARS-CoV-2: a padlock probe-based rolling circle amplification (RCA) and subsequent CRISPR-Cas12a-based signal amplification. To link the CRISPR-RCA reaction with a glucose test, poly-invertase-DNA immobilized magnetic nanoparticles (MNPs-poly-invertase) were further introduced as the substrate for Cas12a with collateral cleavage activity. In this way, a number of poly-invertases were cleaved and released into the solution, which subsequently catalyzed the hydrolysis of sucrose to glucose, thereby producing an amplified glucose signal that is detectable using a PGM. Such a construct combines key features of RCA and CRISPR technologies to improve sensitivity and specificity40 and directly quantified viral nucleic acids using a glucose readout, facilitating the development of promising alternative methods for low-cost POC screening of SARS-CoV-2. The assay achieved a high sensitivity of 30.3 fM for N gene within 3 hours and high specificity to accurately discriminate N gene mutations. We further validated the performance and reliability of the CRISPR-PGM system with clinical throat swab samples. Finally, we applied this system to quantitatively detect the N protein of SARS-CoV-2 by incorporating an additional antibody-assisted proximity ligation module with the CRISPR-PGM. Although the CRISPR-PGM system is developed in the context of COVID-19, we anticipate that this new method can be expanded to diagnose other pathogens and build preparedness for future potential infectious disease outbreaks.
Results and discussion
Detection strategy and workflow of the CRISPR-PGM system for COVID-19 screening
The detection strategy and workflow of the CRISPR-PGM system that incorporates RCA and CRISPR-mediated amplification with a PGM-based glucose test is shown in Scheme 1. The whole workflow is composed of three components: the input of viral RNA by extraction from SARS-CoV-2 infected patients, the CRISPR-based signal transduction, and the output glucose test using a PGM. Among them, the CRISPR-based signal transduction is the key process, in which the specific gene region of interest is amplified using RCA. Briefly, a padlock probe N (PPN) is designed to be capable of hybridizing with the target N gene, facilitating its cyclization by T4 DNA ligase. After addition of phi29 DNA polymerase, the RCA reaction occurs, producing a long single stranded DNA product with a large amount of tandem sequence repeats, each of which contains one recognition site of restriction endonuclease EcoRI. Then, in the presence of EcoRI endonuclease, the RCA product is cut into many small DNA fragments (activator), each of which is complementary to 3′ and 5′ terminals of crRNA. Then, the resulting DNA fragment is recognized by a pre-assembled Cas12a/crRNA ribonucleoprotein complex, activating the collateral activity of Cas12a. The active Cas12a then cleaves the MNPs-poly-invertase to release a number of DNA–invertase conjugates and subsequently catalyzes the hydrolysis of sucrose to glucose. Finally, the glucose produced was measured using a PGM. Since the output glucose signal is directly related to the presence and concentration of the released poly-invertase in solution, which in turn depends on the collateral cleavage activity of Cas12a triggered by the target viral RNA, the presence and concentration of the target can be determined by monitoring the glucose signal in the system. Using this strategy, we can transform the viral RNA detection into a simple glucose test for SARS-CoV-2 diagnosis. In addition, the combination of RCA and CRISPR-Cas12a with a glucose test takes advantage of the isothermal amplification ability of RCA, the high specificity to single-base variation of CRISPR technology, the significant turnover enzyme activity of both Cas12a and the poly-invertase system, and more importantly the user-friendly, portability, and cost-effective properties of the PGM. Therefore, we anticipate that our CRISPR-PGM system will be useful for rapid COVID-19 screening with high sensitivity and specificity in a POC format.
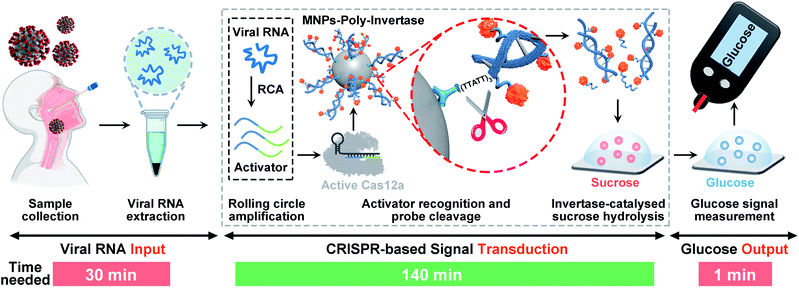 |
| Scheme 1 Detection strategy and workflow of our CRISPR-PGM system for COVID-19 screening. SARS-CoV-2 RNA is first extracted, and a specific gene region of interest is amplified using rolling circle amplification (RCA). The RCA products then bind to Cas12a/crRNA, activating the collateral activity of Cas12a. The active Cas12a cleaves the poly-invertase-DNA immobilized magnetic nanoparticles to release a number of DNA–invertase conjugates and subsequently catalyzes the hydrolysis of sucrose to glucose, thereby producing an amplified glucose signal that is detectable using a PGM. The output glucose signal is correlated with the concentration of the input SARS-CoV-2 RNA. | |
Design and validation of the CRISPR-RCA assay for SARS-CoV-2 N gene
As a proof of concept for detecting SARS-CoV-2, we initially designed a padlock probe to target an already validated N (nucleoprotein) gene in the SARS-CoV-2 genome (Fig. 1A). All oligo sequences are specified in Table S1.† The padlock probe N (PPN) consists of two recognition regions with complementary DNA sequences to the N gene target; one, a region with complementary DNA sequences to the phi29 primer and two, specific EcoRI recognition sites for subsequent endonuclease cleavage. Such a construct could efficiently prevent the nonspecific RCA reaction in the absence of N gene. Specifically, when N gene was present, it hybridizes with the padlock probe and induces the circularization of PPN with the assistance of DNA ligase. The N gene-mediated ligation process was confirmed by denatured polyacrylamide gel electrophoresis (PAGE), which is shown in Fig. S2.† The subsequent RCA reaction is then triggered upon the addition of phi29 DNA polymerase (phi29 DP), resulting in multiple repeated hairpin DNA regions that could be recognized and cleaved by EcoRI. This enzymatic cleavage reaction produces thousands of ssDNA activators for subsequent CRISPR-Cas12a activation. Thus, a small amount of the N gene target could be converted to a large number of Cas12a activators to initiate the collateral cleavage amplification reaction, achieving CRISPR-RCA-mediated signal amplification.
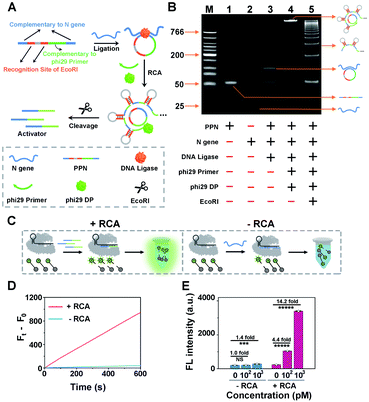 |
| Fig. 1 Design and validation of the CRISPR-RCA assay for SARS-CoV-2 N gene. (A) Schematic of the padlock probe-based rolling circle amplification (RCA) reaction for N gene. (B) Native PAGE (10%) analysis of the RCA reaction for the N gene target. The symbols “+” and “−”, indicate the presence and absence of the reagent, respectively. Graphic illustration (C), fluorescence kinetics measurements (D) and the comparison of fluorescence amplification capability (E) for the detection of N gene by the CRISPR-Cas12a assay with (+RCA) and without (−RCA) amplification using a single-stranded DNA fluorescence reporter (ssDNA-FQ). | |
The N gene-dependent CRISPR-RCA process was first verified by electrophoresis analysis (Fig. 1B). A very bright band in lane 4 with high molecular weight is observed in the presence of N gene, but no band is observed in the same region for PPN (lane 1), N gene (lane 2), or phi29-free control (lane 3). In addition, adding EcoRI results in multiple bright bands with lower molecular weight, indicating the enzymatic digestion of RCA products. To further confirm that the digestion of RCA products could trigger the collateral activity of Cas12a, we performed a fluorescence assay by introducing a 5 nt ssDNA reporter as the substrate for Cas12a–crRNA (Fig. 1C), with a fluorophore (FAM) and quencher (BHQ1) conjugated at the 5′ and 3′ ends, respectively (denoted as ssDNA-FQ). As illustrated in Fig. 1D, a dramatic increase of the fluorescence enhancement rate (FEA) was observed for the RCA-mediated CRISPR-Cas12a assay, as compared to that of the RCA-free CRISPR-Cas12a assay. The FEA value was calculated to be 1.57 s−1 and 0.066 s−1, respectively, indicating that a dramatically amplified fluorescence signal was achieved by the RCA reaction. To better evaluate the capability of signal enhancement through the RCA reaction, we further compared the fluorescence responses of CRISPR-Cas12a assay for different concentrations of the N gene target with and without the RCA reaction (Fig. S3†). Compared with the fluorescence signal of blank samples (F0), no significant difference of the fluorescence signal (Ft) was obtained for 100 pM N gene using the RCA-free CRISPR-Cas12a assay, while 340% of the fluorescence enhancement ratio (FER, defined as (Ft − F0)/F0) was observed for 100 pM N gene using CRISPR-RCA assay (Fig. 1E). In addition, the FER further increases to 13.2 for 1000 pM N gene using the CRISPR-RCA assay. Considering that the FER is only 0.4 for 103 pM N gene using the RCA-free CRISPR-Cas12a assay, an approximately 33-fold increase of signal amplification is therefore obtained by coupling RCA with CRISPR-Cas12a assay. Taken together, these experimental results indicated successful fluorescence amplification for N gene recognition, further demonstrating the feasibility of our CRISPR-RCA system regarding the overall improved sensitivity.
Programmable assembly and characterization of the poly-invertase-DNA immobilized magnetic nanoparticles
To improve the sensitivity and portability of the CRISPR-Cas12a sensor, we further integrated the CRISPR-RCA assay with a PGM and developed a CRISPR-PGM system by introducing MNPs-poly-invertase as the substrate for Cas12a–crRNA. The programmable assembly of the MNPs-poly-invertase by RCA, as illustrated in Fig. 2A (the DNA sequences are shown in Table S1 in the ESI†), includes three steps. First, the RCA primer was immobilized on the streptavidin-coated MNPs through the streptavidin–biotin interaction. Then, a circular padlock probe 2 (cPP2) was prepared (Fig. S4A†) and served as the template to initiate the in situ RCA, producing a large amount of RCA product-immobilized MNPs (MNPs-RP). We employed native PAGE (10%) to evaluate the successful assembly of these DNA-RCA products (Fig. S4B†). The distinct band observed in the high molecular weight (MW) region in lane 3 confirms the successful synthesis of the DNA-RCA products. Finally, a DNA–invertase conjugate containing the complementary DNA to the tandem DNA sequence of RCA products was synthesized (Fig. S4C†) and added for hybridization, which enables the assembly of numerous invertases on the MNPs-RP to obtain MNPs-poly-invertase.
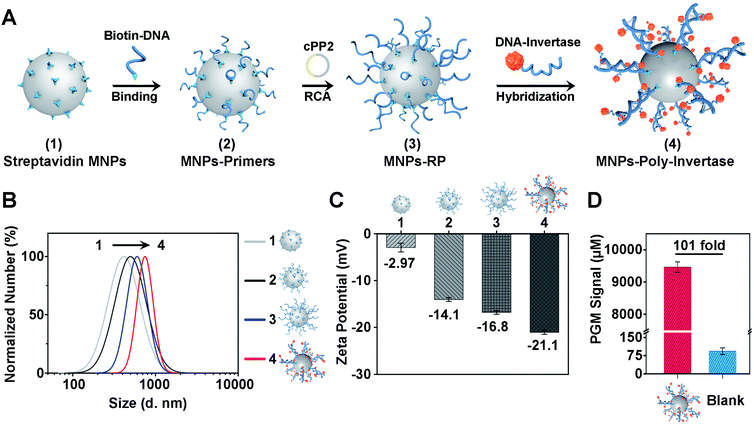 |
| Fig. 2 Programmable assembly and characterization of the poly-invertase-DNA immobilized magnetic nanoparticles (MNPs-poly-invertase). (A) Schematic of the synthesis of MNPs-poly-invertase. Hydrodynamic diameter (B) and zeta potential (C) of four MNPs: streptavidin MNPs (1), MNPs-primers (2), MNPs-RP (3) and MNPs-poly-invertase (4). (D) Comparision of PGM signals generated in the absence and presence of MNPs-poly-invertase. | |
To verify the assembly process of MNPs-poly-invertase, the dynamic light scattering (DLS) analysis was conducted, and the average hydrodynamic diameter (Dh) of streptavidin-MNPs, MNPs-primers, MNPs-RP, and MNPs-poly-invertase increased gradually from 421.0 nm to 725.0 nm (Fig. 2B), indicating the successful step-by-step assembly of poly-invertase on MNPs. It is to be noted that, compared with MNPs-RP, the Dh value of MNPs-poly-invertase, from the DLS and zeta potential measurements, showed a 25% increase which could be due to the enhanced rigidity of the DNA-RCA products after hybridization with DNA–invertase conjugates.41 In addition, to corroborate the results from DLS, zeta potential measurements were further performed in 10 mM PBS, and the ζ-potential value gradually decreased during the assembly process (Fig. 2C), revealing the increasing amount of negatively charged DNA on the MNP surface. Next, we investigate the catalytic activity of MNPs-poly-invertase by mixing 10 μL of MNPs-poly-invertase (0.5 mg mL−1) with 40 μL of sucrose reporter solution. After incubation at 37 °C for 30 min, the production of glucose was measured using a PGM. As shown in Fig. 2D, the MNPs-poly-invertase could yield nearly 101-fold more glucose from sucrose than from a blank sample. Taken together, these results confirmed the formation of highly active MNPs-poly-invertase through the RCA-mediated DNA assembly. In addition, to enable the sensitive detection of glucose, an ultra-sensitive PGM (GSI, USA) was applied with a limit of detection (LOD) of 26.0 μM (Fig. S5†), which offers more than 20-fold sensitivity enhancement over the majority of commercially available PGMs (∼0.6 mM).
Performance of the CRISPR-PGM system for N gene detection
Once the MNPs-poly-invertase was synthesized, we then assessed its capability to amplify the PGM signals for N gene detection. As a control, the nonpolymeric invertase immobilized magnetic beads (MNPs-invertase) were prepared by direct hybridization of DNA–invertase conjugates on the MNPs-primer surface (Fig. S6A†). Assay performance was compared at an N gene concentration of 10 pM using MNPs-invertase and MNPs-poly-invertase (Fig. S6B†) as the substrate, respectively. A 6.4-fold increase of the PGM signal was observed for MNPs-poly-invertase over MNPs-invertase (Fig. S6C†), suggesting a good signal amplification capability of MNPs-poly-invertase because of the advantage of release of multiple invertase molecules with one DNA cleavage event. It should be noted that N gene-DNA, the DNA mimic of N gene-RNA from the N1 region of SARS-CoV-2, was used as the model target for CRISPR-PGM experiments because of the higher chemical stability of DNA over RNA.42 To test the hypothesis that the N gene-RNA possesses the same capability as N gene-DNA in the CRISPR-PGM system, we carried out two independent runs of CRISPR-PGM tests for N gene-RNA and N gene-DNA, respectively. No significant difference of PGM signals was observed for the same concentration of N gene-RNA and N gene-DNA (P > 0.05, Fig. S7†), confirming the validity of this strategy.
To evaluate the analytical performance of the CRISPR-PGM system, we first investigated the sensitivity of our approach for quantitative detection of N gene-DNA in PBS buffer. Fig. 3A shows that the PGM signal increased with increasing N gene-DNA concentration from 10.0 fM to 5 nM. In addition, this CRISPR-PGM system exhibited a wide linear range from 50.0 fM to 50.0 pM and showed a limit of detection (LOD) of 30.3 fM, correlating to 1.8 × 104 virus particles (VPs) per μL (based on 3σb/slope, where σb is the standard deviation of the blank samples). The LOD of our CRISPR-PGM system is comparable to that of plasmonic photothermal assays,43 but much lower than that of previous CRISPR/dCas9-based N gene sensors.44 Although the sensitivity of the CRISPR-PGM method is lower than that of other CRISPR-based sensors with optical readouts,45 the whole workflow could be finished within 3 hours, and more importantly, the glucose readout format is more user-friendly and cost-effective, thus providing a promising alternative approach for portable, fast, and self-screening of COVID-19.
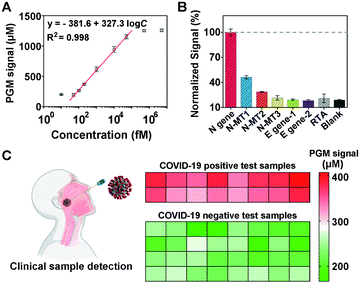 |
| Fig. 3 Performance of the CRISPR-PGM system for N gene detection. (A) The linear relationship between the PGM signal and the N gene concentration from 50 to 50 000 fM. (B) Specificity of the CRISPR-PGM system for the N gene detection. (C) Performance of the CRISPR-PGM for N gene detection in clinical samples. The heat map represents the glucose values detected using a PGM. The COVID-19 positive and negative samples were confirmed by RT-qPCR. | |
To validate the selectivity of the CRISPR-PGM system, two E (envelope) genes (E1, E2) from SARS-CoV-2 and one random target analogue (RTA) were further tested using the CRISPR-PGM system. As shown in Fig. 3B, compared with the blank samples, no significant difference of the normalized PGM signal was observed for 50 pM E1, E2, or RTA samples (P > 0.05), while the same concentration of N gene could result in about 5-fold increase of the PGM signal, suggesting that the high selectivity of CRISPR-Cas12a was maintained for the CRISPR-PGM system. To further demonstrate the specificity, we challenged the CRISPR-PGM system with different N gene mutations, including one mismatched base-contained N gene (N-MT1), two mismatched base-contained N gene (N-MT2), and three mismatched base-contained N gene (N-MT3). Compared with the perfectly matched N gene, the normalized PGM signal for N-MT1, N-MT2, and N-MT3 decreased to (46.2 ± 2.03)%, (28.6 ± 0.58)%, and (21.4 ± 2.65)%, respectively (Fig. 3B). These results indicated that the CRISPR-PGM system possesses good specificity for discriminating homologous sequences from SARS-CoV-2, even those with single nucleotide differences.
Given the highly sensitive and selective detection of N gene, the CRISPR-PGM system was further applied to detect SARS-CoV-2 from throat swab samples. We evaluated a total of 48 viral RNA samples extracted from two COVID-19 positive and four COVID-19 negative people, which were validated by RT-qPCR in the hospital laboratory. Based on the typical CRISPR-PGM workflow, the PGM signal for each sample was collected and the corresponding heat map is shown in Fig. 3C. By targeting the N gene of SARS-CoV-2, the average PGM values for the healthy people and two patients were obtained as 228 ± 29.3 (n = 32), 371 ± 23.1 (n = 8), and 342 ± 22.7 (n = 8), respectively. Based on the calibration curve of N gene detection in Fig. 3A, the correlated concentration of N gene was thus calculated to be 74.1 ± 14.6 fM, 202 ± 32.4 fM, and 165 ± 27.1 fM, respectively. Although a detectable N gene signal in healthy people was obtained due to the non-specific hydrolysis of sucrose to glucose during the CRISPR-PGM assay, a significantly higher level of N gene was observed in SARS-CoV-2 infected patients (P < 0.00001, Fig. S8†). Thus, the CRISPR-PGM system demonstrated the required sensitivity and specificity for COVID-19 screening, with 100% concordance with the results obtained by standard RT-qPCR from the clinical laboratory.
Design and performance of the CRISPR-PGM system for SARS-CoV-2 protein detection
Another challenge in current COVID-19 screening is the potential false positive due to unintended amplification of DNA/RNA contaminants.46 Therefore, serological assays for SARS-CoV-2 proteins or antibodies have been developed for complementary diagnosis. However, the detection of minute amounts of these proteins poses a considerable challenge because proteins cannot be directly amplified. To address this issue, motivated by the above success in detecting the SARS-CoV-2 N gene, we sought to adapt our CRISPR-PGM system for SARS-CoV-2 N protein detection. To do this, an antibody-assisted proximity ligation assay (PLA) was first designed47,48 and combined with CRISPR-RCA for signal amplification (Fig. 4A). Such a construct consists of two proximity probes (PPA and PPB) to target N protein, which were synthesized by conjugating two non-competitive N antibodies with specific DNA sequences via the maleimide–thiol reaction using a heterobifunctional linker, sulfo-SMCC. The synthesis process was characterized using 10% native PAGE (Fig. S9†). When the paired proximity probes bind to the same N protein in close proximity to each other, they could hybridize with two linear oligonucleotides and guide the formation of circular DNA via enzymatic DNA ligation. Then, the circular DNA could serve as the template for subsequent CRISPR-RCA reactions, producing the amplified Cas12a activators. To examine whether CRISPR-Cas12a can be activated by the N protein-mediated PLA-RCA reaction, we first performed a fluorescence assay for N protein using ssDNA-FQ as the substrate for Cas12a–crRNA (Fig. S10A†). In the absence of N protein, minimal fluorescence signal was observed (Fig. S10B†), indicating a negligible cleavage of ssDNA-FQ by the Cas12a–crRNA complex. However, a considerable fluorescence signal was observed in the presence of 50 pM N protein and more importantly showed a further increase for 200 pM N protein (Fig. S10B†), indicating the efficient collateral cleavage of ssDNA-FQ by the Cas12a–crRNA complex in response to N protein. These results suggest that only when the target N protein was present was the Cas12a activator generated from the PLA-RCA reaction, thereby confirming both target-dependent proximity ligation and subsequent activator amplification.
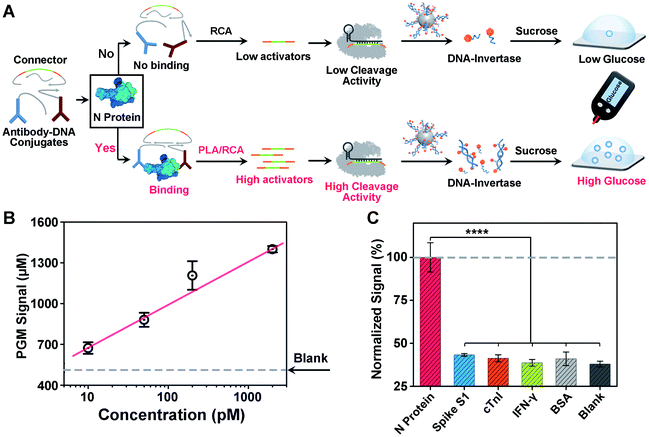 |
| Fig. 4 Design and performance of the CRISPR-PGM system for SARS-CoV-2 protein detection. (A) Working principle for the detection of N protein by the proximity ligation assisted CRISPR-PGM method. (B) PGM signal increase of the sensor in buffer for different concentrations of N protein. (C) Selectivity of N protein detection. The concentration of N protein and other competing proteins is 200 pM. Error bars represent the standard deviations of three independent measurements. **** indicated P < 0.0001. | |
Then, we further conducted similar tests and tried to detect N protein using a PGM by introducing the MNPs-poly-invertase as the substrate for Cas12a–crRNA (Fig. 4A). Compared with blank samples, the presence of N protein results in a significantly higher PGM signal in a dose-dependent manner (Fig. 4B). This observation is in agreement with the fluorescence results, which indicated that the N protein target could effectively trigger the proximity ligation assisted RCA reaction to activate the Cas12a–crRNA complex, followed by an efficient collateral cleavage of MNPs-poly-invertase that could finally catalyze the generation of a large amount of glucose. Moreover, this PLA-CRISPR-PGM system exhibited a wide dynamic range from 10 to 5000 pM and showed a limit of detection of 11.4 pM based on 3σb/slope (Fig. 4B). This LOD correlates to approximately 2.0 × 105 VPs per μL because of an average of 30–35 viral ribonucleoprotein complexes per virion.49 This sensitivity is comparable to that of several commercial ELISA kits and graphene-based electrochemical sensors50 but much higher than that of previous lateral flow sensors.51,52 The high sensitivity was due to the cooperative signal amplification by PLA-RCA, CRISPR, and poly-invertase labels. Finally, to demonstrate the selectivity of the PLA-CRISPR-PGM system for N protein detection, we performed the PGM test using different competing proteins and the glucose signals were recorded, including spike S1, cardiac troponin I (cTnI), interferon-gamma (IFN-γ), and bovine serum albumin (BSA). As shown in Fig. 4C, compared with the blank samples, no significant difference of the glucose signal was observed for competing proteins at a concentration of 50 pM, while the glucose signal in response to 50 pM N protein of SARS-CoV-2 showed a more than 3-fold increase (p < 0.0001), suggesting good specificity arising from the proximity ligation assisted CRISPR-RCA reaction. Taken together, we demonstrated that the CRISPR-PGM system could be easily reconfigured to detect a broad range of COVID-19 related biomarkers using suitable recognition probes, with high sensitivity and specificity in a POC format.
To demonstrate the potential clinical application of the PLA-CRISPR-PGM system, we further conducted a similar protein assay for SARS-CoV-2 spike protein on our PLA-CRISPR-PGM system using SARS-CoV-2 spike pseudo viruses. As shown in Fig. S11A,† a series of concentrations of pseudo virus from 0 to 2 × 106 VPs per μL were lysed with NE-PER buffer and measured with the established PLA-CRISPR-PGM system as described above, except that two non-competitive spike protein antibody–DNA conjugates were applied. Fig. S11B† shows that the PGM signal increased with increasing concentrations of pseudo virus from 0 to 2 × 106 VPs per μL, with a LOD of 9.7 × 104 VPs per μL. This LOD correlates to approximately 4.2 ± 2.4 pM spike protein because of an average of 26 ± 15 spike proteins per virion.49 These results not only verify the feasibility of our PLA-CRISPR-PGM system for the detection of SARS-CoV-2 pseudo-viruses, but also demonstrate the generality of the protein assay.
Finally, to realize the full potential of the CRISPR-PGM system and broaden its application for daily COVID-19 screening, we challenged the CRISPR-PGM system with the saliva specimens spiked with different concentrations of either N gene (Fig. S12A†) or N protein (Fig. S13A†). Fig. S12B† shows that the PGM signal increased with increasing N gene concentration from 0 to 200 pM, and a significant difference in PGM signals between 0.2 pM N gene and the blank sample was observed (p < 0.05). The LOD was calculated to be 604 fM, correlating to 3.6 × 105 VPs per μL. Similarly, when the detection of N protein in saliva samples was performed using the established PLA-CRISPR-PGM system, the PGM signal increased with increasing N protein concentration from 0 to 300 pM (Fig. S13B†), and a significant difference in PGM signals between 40 pM N protein and the blank sample was observed (p < 0.05). The LOD was calculated to be 47 pM, correlating to 8.2 × 105 VPs per μL. Since saliva is easier to collect than either blood or nasopharyngeal swab, its accessibility makes it an ideal specimen for daily COVID-19 screening.53 In addition, previous studies have showed the presence of high viral loads in the saliva specimens of infected patients.54 Therefore, our CRISPR-PGM system could provide a potential method for daily COVID-19 screening of saliva specimens.
Conclusion
In summary, we have demonstrated a general and versatile CRISPR-PGM system for the quantitative detection of COVID-19 related biomarkers. Using this system, a specific N gene and N protein of SARS-CoV-2 have been detected quantitatively with a single portable device. Taking advantage of both target-dependent RCA and sequence-specific recognition of CRISPR, the CRISPR-PGM system offers significant improvements in both analytical sensitivity and specificity. Moreover, integrated with a glucose meter, this method has the potential to enable rapid, low-cost, and point-of-care screening for SARS-CoV-2. These features would allow for earlier detection and more accurate screening for asymptomatic infections at home through telemedicine care. In addition, given the facile integration of various bioreceptors into the CRISPR-PGM system, the proposed method holds great promise to provide patients with a single-device solution that can quantitatively monitor multiple COVID-19 biomarkers at home. Although the CRISPR-PGM system was not fully integrated into a single step detection, as a proof-of-concept, this platform paves the way to further broaden the POC applications of PGM-based sensors. In addition, one major limitation of our study is that our experiments have thus far been conducted only with a small clinical sample size; however, our data provide a window into what a larger clinical screening might look like. It should be noted that the CRISPR-PGM system offers a sample-to-result time of approximately 3 hours; however, we expect improvements in the turnaround time with optimization of RNA extraction, Cas12a–crRNA engineering,55 and additional integration of the PGM with a lateral flow device.56 Overall, in addition to highlighting new strategies for SARS-CoV-2 detection, the results provide a starting point for the development of cost-effective home self-testing devices with the ultimate goal of daily screening and reducing the burden of the ongoing COVID-19 pandemic.
Data availability
All the data supporting this article have been included in the main text and the supplementary material.
Author contributions
R. Liu and J. J. Zhang designed the experiments. R. Liu performed the synthesis, characterization and assays. Y. S. Hu assisted to test the clinical samples. Y. He helped to perform part of the additional experiment. All authors contributed to the analysis and discussion of the results. R. Liu and J. J. Zhang wrote the manuscript. J. J. Zhang conceived and supervised this research.
Conflicts of interest
There are no conflicts to declare.
Acknowledgements
We greatly acknowledge the financial support from the National Natural Science Foundation of China (no. 22004063), Natural Science Foundation of Jiangsu Province (no. 20200303), Fundamental Research Funds for the Central Universities (020514380196, 020514380215), Innovation Fund from Nanjing University (020514913414), and start-up funds from Nanjing University (020514912226). Development of the GSI-PGM was supported by the NIH (HD092155).
Notes and references
- P. Moitra, M. Alafeef, K. Dighe, M. B. Frieman and D. Pan, ACS Nano, 2020, 14, 7617–7627 Search PubMed.
- X. Yuan, C. Yang, Q. He, J. Chen, D. Yu, J. Li, S. Zhai, Z. Qin, K. Du and Z. Chu, ACS Infect. Dis., 2020, 6, 1998–2016 Search PubMed.
- C. H. Woo, S. Jang, G. Shin, G. Y. Jung and J. W. Lee, Nat. Biomed. Eng., 2020, 4, 1168–1179 Search PubMed.
- A. Parihar, P. Ranjan, S. K. Sanghi, A. K. Srivastava and R. Khan, ACS Appl. Bio Mater., 2020, 3, 7326–7343 Search PubMed.
- L. Xu, D. Li, S. Ramadan, Y. Li and N. Klein, Biosens. Bioelectron., 2020, 170, 112673 Search PubMed.
- M. Norman, T. Gilboa, A. F. Ogata, A. M. Maley, L. Cohen, E. L. Busch, R. Lazarovits, C.-P. Mao, Y. Cai, J. Zhang, J. E. Feldman, B. M. Hauser, T. M. Caradonna, B. Chen, A. G. Schmidt, G. Alter, R. C. Charles, E. T. Ryan and D. R. Walt, Nat. Biomed. Eng., 2020, 4, 1180–1187 Search PubMed.
- J. Kang, H. Jang, G. Yeom and M.-G. Kim, Anal. Chem., 2020, 93, 992–1000 Search PubMed.
- J. Qian, S. A. Boswell, C. Chidley, Z.-x. Lu, M. E. Pettit, B. L. Gaudio, J. M. Fajnzylber, R. T. Ingram, R. H. Ward, J. Z. Li and M. Springer, Nat. Commun., 2020, 11, 5920 Search PubMed.
- D. Wang, S. He, X. Wang, Y. Yan, J. Liu, S. Wu, S. Liu, Y. Lei, M. Chen, L. Li, J. Zhang, L. Zhang, X. Hu, X. Zheng, J. Bai, Y. Zhang, Y. Zhang, M. Song and Y. Tang, Nat. Biomed. Eng., 2020, 4, 1150–1158 Search PubMed.
- E. Xiong, L. Jiang, T. Tian, M. Hu, H. Yue, M. Huang, W. Lin, Y. Jiang, D. Zhu and X. Zhou, Angew. Chem., Int. Ed., 2020, 60, 5307–5315 Search PubMed.
- F. Tian, C. Liu, J. Q. Deng, Z. W. Han, L. Zhang, Q. H. Chen and J. S. Sun, Sci. China: Chem., 2020, 63, 1498–1506 Search PubMed.
- T. Tian, B. Shu, Y. Jiang, M. Ye, L. Liu, Z. Guo, Z. Han, Z. Wang and X. Zhou, ACS Nano, 2020, 15, 1167–1178 Search PubMed.
- C. M. Ackerman, C. Myhrvold, S. G. Thakku, C. A. Freije, H. C. Metsky, D. K. Yang, S. H. Ye, C. K. Boehm, T. S. F. Kosoko-Thoroddsen, J. Kehe, T. G. Nguyen, A. Carter, A. Kulesa, J. R. Barnes, V. G. Dugan, D. T. Hung, P. C. Blainey and P. C. Sabeti, Nature, 2020, 582, 277–282 Search PubMed.
- J. P. Broughton, X. D. Deng, G. X. Yu, C. L. Fasching, V. Servellita, J. Singh, X. Miao, J. A. Streithorst, A. Granados, A. Sotomayor-Gonzalez, K. Zorn, A. Gopez, E. Hsu, W. Gu, S. Miller, C. Y. Pan, H. Guevara, D. A. Wadford, J. S. Chen and C. Y. Chiu, Nat. Biotechnol., 2020, 38, 870–874 Search PubMed.
- M. Patchsung, K. Jantarug, A. Pattama, K. Aphicho, S. Suraritdechachai, P. Meesawat, K. Sappakhaw, N. Leelahakorn, T. Ruenkam, T. Wongsatit, N. Athipanyasilp, B. Eiamthong, B. Lakkanasirorat, T. Phoodokmai, N. Niljianskul, D. Pakotiprapha, S. Chanarat, A. Homchan, R. Tinikul, P. Kamutira, K. Phiwkaow, S. Soithongcharoen, C. Kantiwiriyawanitch, V. Pongsupasa, D. Trisrivirat, J. Jaroensuk, T. Wongnate, S. Maenpuen, P. Chaiyen, S. Kamnerdnakta, J. Swangsri, S. Chuthapisith, Y. Sirivatanauksorn, C. Chaimayo, R. Sutthent, W. Kantakamalakul, J. Joung, A. Ladha, X. Jin, J. S. Gootenberg, O. O. Abudayyeh, F. Zhang, N. Horthongkham and C. Uttamapinant, Nat. Biomed. Eng., 2020, 4, 1140–1149 Search PubMed.
- X. Ding, K. Yin, Z. Li, R. V. Lalla, E. Ballesteros, M. M. Sfeir and C. Liu, Nat. Commun., 2020, 11, 4711 Search PubMed.
- J. Arizti-Sanz, C. A. Freije, A. C. Stanton, B. A. Petros, C. K. Boehm, S. Siddiqui, B. M. Shaw, G. Adams, T.-S. F. Kosoko-Thoroddsen, M. E. Kemball, J. N. Uwanibe, F. V. Ajogbasile, P. E. Eromon, R. Gross, L. Wronka, K. Caviness, L. E. Hensley, N. H. Bergman, B. L. MacInnis, C. T. Happi, J. E. Lemieux, P. C. Sabeti and C. Myhrvold, Nat. Chem., 2020, 11, 5921 Search PubMed.
- P. Fozouni, S. Son, M. Diaz de Leon Derby, G. J. Knott, C. N. Gray, M. V. D'Ambrosio, C. Zhao, N. A. Switz, G. R. Kumar, S. I. Stephens, D. Boehm, C.-L. Tsou, J. Shu, A. Bhuiya, M. Armstrong, A. R. Harris, P.-Y. Chen, J. M. Osterloh, A. Meyer-Franke, B. Joehnk, K. Walcott, A. Sil, C. Langelier, K. S. Pollard, E. D. Crawford, A. S. Puschnik, M. Phelps, A. Kistler, J. L. DeRisi, J. A. Doudna, D. A. Fletcher and M. Ott, Cell, 2020, 183, 323–333 Search PubMed.
- P. Pokhrel, C. Hu and H. Mao, ACS Sens., 2020, 5, 2283–2296 Search PubMed.
- I. A. Mattioli, A. Hassan, O. N. Oliveira, Jr. and F. N. Crespilho, ACS Sens., 2020, 5, 3655–3677 Search PubMed.
- D. B. Larremore, B. Wilder, E. Lester, S. Shehata, J. M. Burke, J. A. Hay, M. Tambe, M. J. Mina and R. Parker, Sci. Adv., 2021, 7, eabd5393 Search PubMed.
- B. Udugama, P. Kadhiresan, H. N. Kozlowski, A. Malekjahani, M. Osborne, V. Y. Li, H. Chen, S. Mubareka, J. B. Gubbay and W. C. Chan, ACS Nano, 2020, 14, 3822–3835 Search PubMed.
- K. Mao, H. Zhang and Z. Yang, Biosens. Bioelectron., 2020, 169, 112617 Search PubMed.
- H. Lukas, C. Xu, Y. Yu and W. Gao, ACS Nano, 2020, 14, 16180–16193 Search PubMed.
- A. Fernández, ACS Pharmacol. Transl. Sci., 2020, 4, 403–405 Search PubMed.
- J. E. Van Dongen, J. T. W. Berendsen, R. D. M. Steenbergen, R. M. F. Wolthuis, J. C. T. Eijkel and L. I. Segerink, Biosens. Bioelectron., 2020, 166, 112445 Search PubMed.
- M. Sharafeldin and J. J. Davis, Anal. Chem., 2021, 93, 184–197 Search PubMed.
- J. Cheong, H. Yu, C. Y. Lee, J.-U. Lee, H.-J. Choi, J.-H. Lee, H. Lee and J. Cheon, Nat. Biomed. Eng., 2020, 4, 1159–1167 Search PubMed.
- N. Singh, Biosens. Bioelectron., 2021, 180, 113111 Search PubMed.
- A. Suea-Ngam, L. Bezinge, B. Mateescu, P. D. Howes, A. J. deMello and D. A. Richards, ACS Sens., 2020, 5, 2701–2723 Search PubMed.
- D. C. Christodouleas, B. Kaur and P. Chorti, ACS Cent. Sci., 2018, 4, 1600–1616 Search PubMed.
- T. Miesler, C. Wimschneider, A. Brem and L. Meinel, ACS Biomater. Sci. Eng., 2020, 6, 2709–2725 Search PubMed.
- J. J. Zhang, T. Lan and Y. Lu, TrAC, Trends Anal. Chem., 2020, 124, 115782 Search PubMed.
- Y. Xiang and Y. Lu, Nat. Chem., 2011, 3, 697–703 Search PubMed.
- L. Yan, Z. Zhu, Y. Zou, Y. S. Huang, D. W. Liu, S. S. Jia, D. M. Xu, M. Wu, Y. Zhou, S. Zhou and C. J. Yang, J. Am. Chem. Soc., 2013, 135, 3748–3751 Search PubMed.
- J. J. Zhang and Y. Lu, Angew. Chem., Int. Ed., 2018, 57, 9702–9706 Search PubMed.
- S. H. Gong, Y. Y. Chen, W. Pan, N. Li and B. Tang, Chem. Commun., 2020, 56, 8850–8853 Search PubMed.
- H. Y. Kim, C. Y. Lee, H. Kim, K. S. Park and H. G. Park, Analyst, 2020, 145, 5578–5583 Search PubMed.
- D. Huang, Z. Shi, J. Qian, K. Bi, M. Fang and Z. Xu, Biotechnol. Bioeng., 2021, 118, 1587–1596 Search PubMed.
- P. Khan, L. M. Aufdembrink and A. E. Engelhart, ACS Synth. Biol., 2020, 9, 2861–2880 Search PubMed.
- A. Savelyev, C. K. Materese and G. A. Papoian, J. Am. Chem. Soc., 2011, 133, 19290–19293 Search PubMed.
- C. Xue, S. X. Zhang, C. H. Ouyang, D. R. Chang, B. J. Salena, Y. F. Li and Z. S. Wu, Angew. Chem., Int. Ed., 2018, 57, 9739–9743 Search PubMed.
- G. G. Qiu, Z. B. Gai, Y. L. Tao, J. Schmitt, G. A. Kullak-Ublick and J. Wang, ACS Nano, 2020, 14, 5268–5277 Search PubMed.
- J. Moon, H.-J. Kwon, D. Yong, I.-C. Lee, H. Kim, H. Kang, E.-K. Lim, K.-S. Lee, J. Jung, H. G. Park and T. Kang, ACS Sens., 2020, 5, 4017–4026 Search PubMed.
- B. Pang, J. Xu, Y. Liu, H. Peng, W. Feng, Y. Cao, J. Wu, H. Xiao, K. Pabbaraju, G. Tipples, M. A. Joyce, H. A. Saffran, D. L. Tyrrell, H. Zhang and X. C. Le, Anal. Chem., 2020, 92, 16204–16212 Search PubMed.
- W. Feng, A. M. Newbigging, C. Le, B. Pang and X. C. Le, Anal. Chem., 2020, 92, 10196–10209 Search PubMed.
- O. Soderberg, M. Gullberg, M. Jarvius, K. Ridderstrale, K. J. Leuchowius, J. Jarvius, K. Wester, P. Hydbring, F. Bahram, L. G. Larsson and U. Landegren, Nat. Methods, 2006, 3, 995–1000 Search PubMed.
- R. Liu, L. He, Y. Hu, Z. Luo and J. Zhang, Chem. Sci., 2020, 11, 12157–12164 Search PubMed.
- H. P. Yao, Y. T. Song, Y. Chen, N. P. Wu, J. L. Xu, C. J. Sun, J. X. Zhang, T. H. Weng, Z. Y. Zhang, Z. G. Wu, L. F. Cheng, D. R. Shi, X. Y. Lu, J. L. Lei, M. Crispin, Y. G. Shi, L. J. Li and S. Li, Cell, 2020, 183, 730–738 Search PubMed.
- R. M. Torrente-Rodriguez, H. Lukas, J. Tu, J. Min, Y. Yang, C. Xu, H. B. Rossiter and W. Gao, Matter, 2020, 3, 1981–1998 Search PubMed.
- H.-Y. Kim, J.-H. Lee, M. J. Kim, S. C. Park, M. Choi, W. Lee, K. B. Ku, B. T. Kim, E. Changkyun Park, H. G. Kim and S. I. Kim, Biosens. Bioelectron., 2021, 175, 112868 Search PubMed.
- J.-H. Lee, M. Choi, Y. Jung, S. K. Lee, C.-S. Lee, J. Kim, J. Kim, N. H. Kim, B.-T. Kim and H. G. Kim, Biosens. Bioelectron., 2021, 171, 112715 Search PubMed.
- D. Ter-Ovanesyan, T. Gilboa, R. Lazarovits, A. Rosenthal, X. Yu, J. Z. Li, G. M. Church and D. R. Walt, Anal. Chem., 2021, 93, 5365–5370 Search PubMed.
- H. Yousefi, A. Mahmud, D. R. Chang, J. Das, S. Gomis, J. B. Chen, H. S. Wang, T. Been, L. Yip, E. Coomes, Z. J. Li, S. Mubareka, A. McGeer, N. Christie, S. Gray-Owen, A. Cochrane, J. M. Rini, E. H. Sargent and S. O. Kelley, J. Am. Chem. Soc., 2021, 143, 1722–1727 Search PubMed.
- L. T. Nguyen, B. M. Smith and P. K. Jain, Nat. Commun., 2020, 11, 4906 Search PubMed.
- J. J. Zhang, Z. Shen, Y. Xiang and Y. Lu, ACS Sens., 2016, 1, 1091–1096 Search PubMed.
Footnote |
† Electronic supplementary information (ESI) available: Experimental section and synthetic procedures. See DOI: 10.1039/d1sc00512j |
|
This journal is © The Royal Society of Chemistry 2021 |
Click here to see how this site uses Cookies. View our privacy policy here.