DOI:
10.1039/D2LC00783E
(Tutorial Review)
Lab Chip, 2023,
23, 818-847
Digital detection of proteins
Received
22nd August 2022
, Accepted 8th November 2022
First published on 10th January 2023
Abstract
This paper reviews methods for detecting proteins based on molecular digitization, i.e., the isolation and detection of single protein molecules or singulated ensembles of protein molecules. The single molecule resolution of these methods has resulted in significant improvements in the sensitivity of immunoassays beyond what was possible using traditional “analog” methods: the sensitivity of some digital immunoassays approach those of methods for measuring nucleic acids, such as the polymerase chain reaction (PCR). The greater sensitivity of digital protein detection has resulted in immuno-diagnostics with high potential societal impact, e.g., the early diagnosis and therapeutic intervention of Alzheimer's Disease. In this review, we will first provide the motivation for developing digital protein detection methods given the limitations in the sensitivity of analog methods. We will describe the paradigm shift catalyzed by single molecule detection, and will describe in detail one digital approach – which we call digital bead assays (DBA) – based on the capture and labeling of proteins on beads, identifying “on” and “off” beads, and quantification using Poisson statistics. DBA based on the single molecule array (Simoa) technology have sensitivities down to attomolar concentrations, equating to ∼10 proteins in a 200 μL sample. We will describe the concept behind DBA, the different single molecule labels used, the ways of analyzing beads (imaging of arrays and flow), the binding reagents and substrates used, and integration of these technologies into fully automated and miniaturized systems. We provide an overview of emerging approaches to digital protein detection, including those based on digital detection of nucleic acids labels, single nanoparticle detection, measurements using nanopores, and methods that exploit the kinetics of single molecule binding. We outline the initial impact of digital protein detection on clinical measurements, highlighting the importance of customized assay development and translational clinical research. We highlight the use of DBA in the measurement of neurological protein biomarkers in blood, and how these higher sensitivity methods are changing the diagnosis and treatment of neurological diseases. We conclude by summarizing the status of digital protein detection and suggest how the lab-on-a-chip community might drive future innovations in this field.
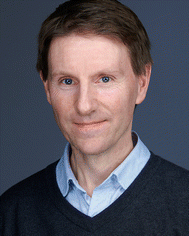 David C. Duffy | David C. Duffy is Chief Technology Officer at Quanterix Corporation. Previously, he worked at Surface Logix, Gamera Biosciences, and Unilever. He was a postdoctoral research fellow in the Department of Chemistry and Chemical Biology at Harvard University. David was the first Sir Alan Wilson Research Fellow of Emmanuel College, University of Cambridge. He received a Ph.D. in from the Department of Chemistry at the University of Cambridge, and obtained his B.A. and M.A. degrees from Selwyn College, University of Cambridge. David's scientific research has focused on measuring molecules that are difficult to detect, microfluidics, microsystem development, and surface chemistry. |
1. Introduction
In his book Rationality, the cognitive scientist and renowned science communicator Steven Pinker writes that “…better instruments, more sensitive diagnostics, more reliable forensics—is an unmitigated good, reducing errors…Enhancing sensitivity should always be our aspiration in signal detection challenges…”.1 Generations of scientists and engineers have been engaged in research and development to innovate signal detection methods that better assess the status of biological systems and diagnose diseases. The analytical methods developed cover disparate detection modalities, such as in vivo imaging (X-ray imaging and magnetic resonance imaging (MRI)), in vitro tissue staining (immunohistochemistry (IHC), fluorescence in situ hybridization (FISH), etc.), measuring concentrations of biological molecules in bodily fluids (immunoassays (IA) and polymerase chain reaction (PCR)), sequencing of DNA, and implantable devices. A common driving force behind these innovations has been the “unmitigated good” highlighted by Pinker: enhancing sensitivity. These innovations in sensitivity have been the basis of a revolution in medical diagnoses based on the measurement of specific molecules in humans. High sensitivity methods have driven a greater understanding of the molecular basis of disease and provided tools for the development of novel therapeutics. The digital detection of proteins is a recent advance that has greatly improved the sensitivity of immunoassays. The enhanced sensitivity of digital protein detection has already resulted in unique capabilities for understanding of, diagnosis of, and developing treatments for neurological diseases, such as Alzheimer's disease and multiple sclerosis, that are amongst the most devastating diseases facing humankind. In this review, we will describe the technologies underlying digital detection of proteins, how it improves the sensitivity of immunoassays, and how it has impacted clinical measurements in important diseases.
The most scalable approach to enable sensitive molecular diagnoses of as many people as possible is to measure molecules in samples that are easy to collect, such as urine, saliva, nasal swabs, and blood. The scalability of this type of test depends on low manufacturing costs of test equipment and kits, access to multiple avenues for the wide distribution of tests (hospitals, doctor's offices, clinics, homes), as well as the simplicity of sample collection in a non-invasive or minimally invasive way. The boundaries of sensitivity for highly scalable tests have been pushed the furthest for the measurement of nucleic acids (DNA and RNA) by the development of highly sensitive amplification techniques (such as PCR and loop-mediated isothermal amplification (LAMP)) and next generation sequencing (NGS).2 For example, PCR is routinely capable of detecting 10s of copies of a nucleic acid in a few microliters of sample, equating to concentrations in the attomolar (10−18 M) range. The remarkable innovations in the ultrasensitive analytical detection of nucleic acids have resulted in the field of molecular diagnostics that has revolutionized the diagnosis and management of several diseases, especially cancer and infectious diseases (ID). Improvements to the sensitivity of protein measurements – which offer very different information on the status of biological systems than DNA and RNA – has historically lagged the measurement of nucleic acids. Until recently, protein detection has been based on conventional sandwich immunoassays, especially the enzyme-linked immunosorbent assay (ELISA) first demonstrated in 1971,3 with the most sensitive methods having limits of detection in the picomolar (10−12 M) range, approximately a million times less sensitive than PCR.
The primary technical motivation for innovating more sensitive protein detection methods – to complement those available for DNA and RNA – is that protein expression provides a “real time” biological status of an organism in contrast to detection of nucleic acids that indicate the presence of a gene but often not its functional activity. DNA provides the genetic code for potential conditions to develop but you usually need to measure proteins to know if they did. An interesting consequence of the “sensitivity gap” between proteins and nucleic acids – but the need for information on the expression products of genes – is the emergence of many technologies that measure messenger RNA (mRNA). Detection of mRNA can leverage highly sensitive NA detection methods, such as PCR, while providing information on gene expression, although it is now well established that mRNA levels do not correlate well to protein expression levels.4
Greater sensitivity to the levels of protein expression also opens many analytical and clinical possibilities for scalable diagnostics.5 First, earlier detection of disease onset is possible, e.g., in cancer and ID, when far fewer copies of proteins are in circulation. Second, it becomes possible to detect the proteins in samples that are more accessible but typically contain lower protein concentrations: for example, cancer biomarkers have high expression levels in tissues (which are difficult to sample) but are diluted in blood (which is easy to sample). Third, greater sensitivity allows the profiling of proteins in healthy people as well as individuals suffering from chronic diseases. Protein expression levels often increase as a disease progresses, but with lower sensitivity assays it is often only possible to detect diagnostic proteins in sick people, an example being troponin, a marker of cardiac damage. Fourth, many therapeutics, especially those based on antibodies, target protein molecules and lower their concentration, so higher sensitivity assays enable the monitoring of those target proteins as a function of drug dose and time course. Fifth, greater sensitivity can be used to increase the speed of a test for higher abundance proteins. Sixth, greater analytical sensitivity to proteins can make it possible to measure these molecules in more challenging samples, such as stool, and in small sample volumes via the use of high dilution factors. More sensitive immunoassays, therefore, have the potential to greatly improve the power of diagnostic tests based on detecting proteins.
Given these many motivations, research into more sensitive protein detection has been intense since the first sandwich immunoassays emerged. Detection improvements in immunoassays followed a path cleared by innovations in label and optical detection technologies, with sensitivity increasing incrementally from the 1970s to 2000s moving from detection of radiometric to colorimetric to fluorescent to chemiluminescent and electrochemiluminescent labels.6 All of these methods can be viewed as analog approaches where the average signal from an ensemble of labeled protein molecules is measured using an optical system. A key concept emerged in the 1990s and 2000s, however, that offered the potential for a step change in sensitivity of protein assays similar to the way that amplification of nucleic acids by polymerases changed the game for DNA: the counting of single immunocomplexes. As Prof. David Walt has articulated this approach: “the highest resolution measurement one can make is at the single molecule level; it just does not get any better than that”.7 By focusing on the presence or absence of single molecule binding events, this approach can be viewed as a digital revolution for protein detection.
In this review, we will describe the emergence of digital detection of proteins that has led to a dramatic improvement in the sensitivity of immunoassays, and has consequently led to better understanding, diagnoses, and treatments of severe diseases. Digital protein detection draws on many disciplines needed for lab-on-a-chip systems, such as binding substrates and reagents, label chemistry, microfluidics, optics, and signal processing. One digital detection method has gained significant traction and has been widely researched: the capture and labeling of proteins on individual beads and counting on and off beads according to Poisson statistics.8 We will provide details on the developments of the different lab-on-a-chip components that have been important in the emergence of this method. We will review other approaches to digital detection of proteins that have emerged. We will describe how the greater sensitivity of digital protein detection methods has enabled unique clinical measurements, and how blood tests based on these methods have helped in the understanding and diagnoses of neurological diseases. We end by highlighting areas for future development in this field.
This review is not intended to be a comprehensive literature review on ultrasensitive protein detection or digital detection of biomolecules in general, but instead a focused summary of the key concepts and advances in ultra-sensitive protein detection methods based on the counting of single molecules or singulated ensembles of molecules. Several excellent comprehensive reviews have appeared in recent years that cover broader aspects of the approaches described here, namely, digital detection of biomolecules (Cunningham and co-workers,9 and Liu et al.10), ultrasensitive protein detection (Cohen and Walt),11 and optical detection of single molecules (Gorris and co-workers,12 and Walt7). Other authors have also provided helpful perspectives on digital detection of proteins.13,14,15,16
2. Changing the paradigm of protein detection
The original sandwich immunoassays are children of the 1970s.3 In parallel to the efforts to improve the sensitivity of these assays via brighter labels and more sensitive detection systems as described above, researchers continued to explore novel approaches in the hope of triggering a paradigm shift in protein detection as occurred in nucleic acids upon the invention of PCR. In fact, many of the approaches developed for proteins took direct inspiration from PCR, and incorporated the amplification of nucleic acid labels into immunoassays. For example, immuno-PCR was developed17 that replaced the enzyme label of ELISA with a DNA label that was amplified by PCR leading to significant improvements in sensitivity.18 Subsequently, other creative methods based on nucleic acid labels emerged such as the proximity ligation assay (PLA),19 the proximity extension assay (PEA),20 immunoassays based on rolling circle amplification labels (immuno-RCA),21 and bio-barcodes.22 While many of these methods improved the sensitivity of protein detection – often into the femtomolar (10−15 M) range23 – they have not found widespread adoption as ultrasensitive methods. This limitation is due to the inherent challenges in robust amplification of nucleic acid labels due to interfering substances in complex samples, and the imprecision associated with exponential PCR readout.24
The counting of single molecules emerged as a candidate for a paradigm shift to improve the sensitivity to proteins. While single molecule detection had been studied for a long time (especially in fluorescence microscopy), the application of these digital approaches for measuring biomolecules increased substantially around the turn of the millennium. As has often been the case, the digital detection of nucleic acids led the way via methods such as digital PCR,25 single molecule RCA at DNA arrays,26 and detection of single molecules based on single-electron transistors27 and atomic force microscopy;28 new digital detection methods for nucleic acids continue to emerge.29,30 More broadly, digital detection of biomolecules has emerged as a fertile field for innovation, as described in several reviews,9,10 including those that describe nanoparticle detection31 and single molecule biosensors.32
For proteins, digital detection was enabled by counting of single molecule labels bound to immunocomplexes. Various approaches for “single molecule counting” were initially developed based on the isolation of individual labeled immunocomplexes followed by high resolution detection of the labels. Härmä and co-workers at the University of Turku developed a method based on formation of sandwich immunocomplexes at the flat bottom of a conventional planar ELISA well plate, followed by time-resolved fluorescent (TIRF) imaging of individual europium nanoparticle labels at the well surface.33–36 This assay had a limit of detection (LOD) for PSA of ∼1 pM;34 for reference, the LOD of traditional, analog clinical immunoassays for PSA at that time was ∼3 pM.8 Another common approach was to detect using high power excitation sources and sensitive detectors the immunocomplexes formed at the bottom of flat plates where the detection antibodies were modified with fluorophores. Löscher et al.37 used a diode laser and a single-photon-counting avalanche photodiode to detect fluorescently labeled detection antibodies associated with immunocomplexes at the bottom of modified glass slides, and showed LODs in the femtomolar range. Schweitzer et al.21 imaged individual labels formed by immuno-RCA at the bottom of ELISA plates and microarray spots, demonstrating an LOD of 3 fM for PSA, about 1000-fold more sensitive than the existing clinical tests for PSA. Several single molecule imaging methods have been based on total internal reflection imaging of fluorescently labeled detection antibodies bound to immunocomplexes at planar surfaces.38–41 Researchers at Singulex modified the planar imaging approach to make use of flow-based detection by chemically releasing the detection antibodies from the bottom of the plate, flowing the solution through a capillary, and detecting the fluorescently labeled detection antibodies by using laser excitation and an avalanche photon detector.42,43 Using this approach they achieved improved sensitivities for several clinically relevant analytes, including troponin (LOD = 5.7 pM, compared to ∼60 pM for the traditional clinical immunoassay) that allowed detection of this biomarker of cardiac damage in healthy individuals.44 The instrumentation was subsequently updated to allow counting of the released single labels in the wells of a microtiter plate without the use of flow, enabling the multiplex detection of 3 interleukins.45
While these approaches demonstrated the potential of counting single immunocomplexes, assay sensitivity and robustness were limited by several factors. First, the shot noise associated with raw single label counting is high, especially when the field of view (FOV) is limited as for many of these early attempts. This “unnormalized” approach to counting single events added to imprecision and required very high precision over aspects of the assay process other than detection that impact the number of molecules detected, such as reagent concentrations, volumes, temperature, etc. Second, most methods were based on capture on planar substrates where inefficient transport of proteins to the capture antibodies can cause sensitivity to be limited kinetically.46 Third, the challenges of detecting single antibodies labeled with only a few fluorophores results in very low signal-to-noise ratios for single labels necessitating the use of expensive and complex excitation sources and detectors. Fourth, despite single label resolution, assays were often limited by non-specific binding of labeling reagents to surfaces causing high assay backgrounds, making the methods insensitive to very low concentrations of specifically labeled proteins. These challenges resulted in limited adoption of single molecule counting methods for proteins in clinical and research laboratories.
3. Digital detection of proteins captured on beads
3.1 Concept
A key concept for digital detection of proteins – the capture and labeling of low concentrations of proteins on an excess of beads, and determining the presence or absence of 1 or more labels on individual beads – was first demonstrated in our laboratory,8 and was designed to address many of the challenges in single molecule counting of proteins. Fig. 1 shows a schematic representation of this approach to digital detection of proteins that we will refer to as digital bead assays (DBA). A sample containing the protein is initially mixed with beads that are coated with capture antibodies that are specific to the target protein (Fig. 1, panel 1). At low concentrations, there are fewer protein molecules than beads and the captured proteins are distributed over the beads according to a Poisson distribution. These proteins are then labeled with a second antibody and a detectable label (Fig. 1, panel 2). The single molecule resolution of this approach is simply a statistical consequence of the Poisson distribution. For illustration, at 1 fM there are approximately 60
000 protein molecules in a 100 μL sample, and if these proteins are captured and then labeled on 500
000 beads,8 then the average number of labels per bead (μ) would be 0.12. According to the probabilities (P) predicted by the Poisson distribution equation –
– we would expect that 88.7% of the beads would have no labels (ν = 0), 10.6% of beads would be associated with a single label (ν = 1), and only 0.64% of beads would have 2 labels (ν = 2). The beads are then interrogated individually (Fig. 1, panel 3) – using imaging or flow detectors – at the characteristic wavelengths of the beads and labels. Histograms of the label intensity from the beads allow us to determine those beads that are associated with no labels (“off-beads”, ν = 0), and beads that are associated with at least 1 label (“on-beads”, ν ≥ 1), hence the digital nature of this method. From this ability to distinguish on- and off-beads, we can determine the fraction of the beads that are on (fon = on-beads ÷ total beads), which equates to 1 − Pμ (0). The histograms also yield an average intensity of all on-beads (Ībead). Fortunately, the Poisson distribution allows us to determine the average number of labels per bead (ALB) (Fig. 1, panel 4) just from counting on- and off-beads (eqn (1)), i.e., without needing to distinguish the number of labels on individual beads:47 | ALBcounting = −ln(1 − fon) | (1) |
An important distinguishing feature of this approach, therefore, was that measurement of the absence of single labels on the capture beads was as important as measuring their presence, so that fon provided an intrinsic normalization that was absent from earlier approaches to single molecule counting. At higher concentrations, where there are more protein molecules than beads, digital counting is no longer precise, and we use the average intensity to quantify the average number of labels per bead (eqn (2)): | ALBintensity = (Ībead/Īsingle) × fon | (2) |
where Īsingle is the average intensity of a single label on a bead.47 As the average number of labels per bead is proportional to the concentration of protein in the sample, we can determine concentrations by extrapolating the ALB values of samples to those of a set of calibrators (Fig. 1, panel 4). Calibration is required in DBA because the number of protein molecules captured and labeled – and hence the ALB values measured – depend on the kinetics of the binding reactions that are determined by reagent affinity, concentration, incubation times, temperature, etc., as for other immunoassays. This situation contrasts with digital PCR where calibration is, in principle, not required as the amplified target molecules are directed counted free in solution without the need for intermediate binding and labeling reactions at a surface.
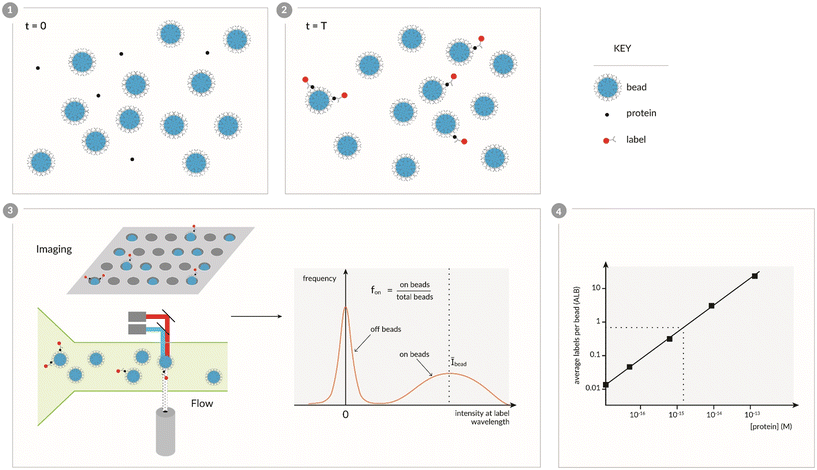 |
| Fig. 1 Digital bead assays (DBA): 1) 1000–500 000 antibody-coated beads are mixed with a sample containing the target protein; 2) at low concentrations—when the ratio of proteins to beads is <1—proteins are captured and labeled according to Poisson distribution, resulting in a fraction of beads associated with zero labels and a fraction of beads associated with at least 1 label, i.e., digitization; 3) the population of beads are then analyzed using imaging or flow detection methods to determine the fraction of beads that are associated with at least one label (fon) and the mean intensity of the labels associated with the “on” beads (Ībead); 4) fon and Ībead are converted to average number of labels per bead (“ALB”) using the Poisson distribution equation or intensity normalization. Calibration curves of ALB as a function of protein concentration are used to extrapolate the concentration of samples from their measured ALB. | |
The first demonstration of the DBA concept was based on labeling the immunocomplexes with β-galactosidase, isolating individual beads in arrays of microwells, sealing the beads in the wells in the presence of a fluorogenic substrate to the enzyme, and imaging the microwells to determine on- and off-beads (Fig. 2).8 In this case, the label was an enzyme so the digital signal was quantified by average enzymes per bead (AEB). This approach – which we called Single Molecule Arrays or Simoa – was inspired by work that showed that by confining β-galactosidase in femtoliter-sized microwells with its substrate, the accumulation of fluorescent product molecules enabled single enzyme molecules to be measured using standard microscopes.48,49 In this first report of a “digital ELISA” (Fig. 2), the ability to detect single enzyme labels allowed reductions in the concentrations of labeling reagents needed, in turn reducing the assay backgrounds, and allowed subfemtomolar concentrations of both PSA and TNF-α to be measured in serum above those backgrounds. The LOD for PSA (200 aM) of this digital ELISA represented an ∼1600-fold improvement over the clinical ultrasensitive PSA test, and was used to measure PSA in 30 patients post-radical prostatectomy who were all undetectable in the clinical test. The high sensitivity of the assays showed that the PSA concentrations in the serum of these patients varied over 3 logs from subfemtomolar to 100 s of femtomolar. This approach led to the first commercial embodiments of digital ELISA,50 and applications in numerous fields of biomedicine, especially in neurology, that are described in section 5.
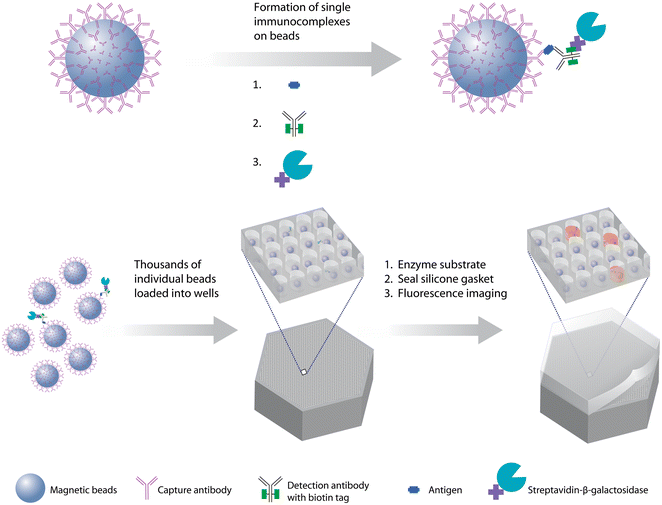 |
| Fig. 2 The first digital bead assay, based on sealing of beads associated with one or zero immunocomplexes in arrays of microwells, and counting of individual enzyme labels. The method has also become known as digital ELISA and the underlying technology as single molecule arrays (Simoa) (ref. 8). Figure reproduced with permission. | |
The detection of single enzymes on beads was pivotal for detecting subfemtomolar concentrations of proteins by a) lowering assay backgrounds, and b) allowing single captured proteins to be detected above that background. Beyond that analytical advance, this approach had several intrinsic properties that leant itself favorably to the development of robust, scalable high sensitivity immunoassays. First, determining on- and off-beads and using Poisson statistics to determine a parameter (ALB = AEB) made the method directly proportion to concentration, and allowed normalization of the signal to the total number of beads. These properties greatly helped precision compared to previous methods described above that just counted the presence of labels on a single substrate. Second, the ability to disperse the capture antibodies on beads throughout a sample resulted in highly efficient capture of the target protein,51 solving the “diffusion” problem of transport of proteins to planar capture surfaces.46 Efficient capture of the target analyte is a primary driver of sensitivity when counting single molecules, and using beads effectively meant that the capture antibodies went to the proteins rather than vice versa. Third, the approach of trapping the fluorescent product of the enzyme-substrate reaction in femtoliter wells resulted in very high signal-to-noise ratios (SNR) for single labels that had not been achieved previously. This approach allowed wide FOV detection methods based on low-cost excitation sources (lamps or LEDs) and uncooled CCD/CMOS cameras, compared to previous methods typically based on small FOV imaging and laser excitation. Fourth, this approach was based on digitizing conventional sandwich immunoassays, so could make use of existing antibody pairs, beads, and sample dilution buffers to suppress non-specific interactions, that have been optimized over the years for sensitivity and specificity in analog immunoassays. In effect, digital ELISA could “turbocharge” existing ELISA reagents. In summary, the digital detection of proteins on beads offered the potential to “capture all the proteins and detect all the proteins”, so was a compelling path to the most sensitive immunoassays possible.
The use of beads also enabled subsequent improvements that helped implementation of the assays. The ability to switch from digital counting to average intensity of beads (Fig. 1; eqn (1) and (2)) enabled a dynamic range of >3 logs that is often needed in clinical applications.47 Beads also offered a convenient path to multiplex digital detection of proteins via the use of beads that were modified with different fluorescent dyes.52,53 The use of superparamagnetic capture beads allowed the magnet pelleting and resuspension of beads in wash buffer greatly helping to reduce assay background via high dilution factors of labeling reagents.50 Many existing immuno-diagnostic systems are based on the same beads, albeit with analog detection, so existing liquid handling equipment for beads could be used to implement the digital ELISA approach.50 Lastly, and perhaps counter-intuitively, sensitivity can be further improved by reducing the number of capture beads51 by increasing ALB at a given concentration and increasing assay slope.54
Since the first description of the DBA concept in 2010, the technology has been commercialized successfully and there has been considerable research on the method. Quanterix Corporation commercialized DBA in 2014 by developing the Simoa HD-1 Analyzer50 and accompanying Simoa disk microfluidic consumable,55 along with specific reagent kits, many of which enabled detection of neurological markers in blood (section 5). The technical challenges encountered in the development and scaling of DBA included: complexity of instrumentation and consumable required to perform the single label detection on isolated beads; wide FOV, multi-wavelength imaging at high resolution; robustness of image analysis algorithms to variation in instrumentation and reagents; reproducibility of bead reagents for use in high sensitivity measurements; and, implementation of automation processes to ensure the bead incubation steps were well controlled. The next 4 subsections describe in detail the various approaches developed to address these challenges of DBA.
3.2 Single molecule labels
A key technical requirement for DBA is to detect single labels on thousands of singulated beads at reasonable cost and size of equipment: several single molecule labels have been developed to achieve this goal (Fig. 3). As mentioned above, the original approach was based on labeling biotinylated detection antibodies with streptavidin-β-galactosidase, sealing the beads in arrays of microwells in the presence of a fluorogenic substrate (resorufin-β-D-galactopyranoside; RGP), and imaging the fluorescence from the resorufin molecules generated from single enzymes in each microwell (Fig. 3A). Fluorescein di(-β-D-galactopyranoside) (FDG) has also been used as a substrate for detection of single molecules of β-galactosidase,56–58 and horse radish peroxidase (HRP) has also been used to generate the freely diffusible fluorescent products in digital ELISA.59 The step of sealing the array of microwells has been achieved using elastomeric films8 and fluorocarbon oil.55–57 While providing a single molecule label with a very high SNR, loading and sealing of beads in microwells creates complexity in the fluidic handling and consumable design. Research into alternative methods have focused on single molecule labels where the labels measured are directly associated with the beads – rather than free to diffuse away from the bead as for resorufin – to avoid the need to seal the beads in microwell arrays, and hence reduce the complexity of the detection process and consumable (Fig. 3).
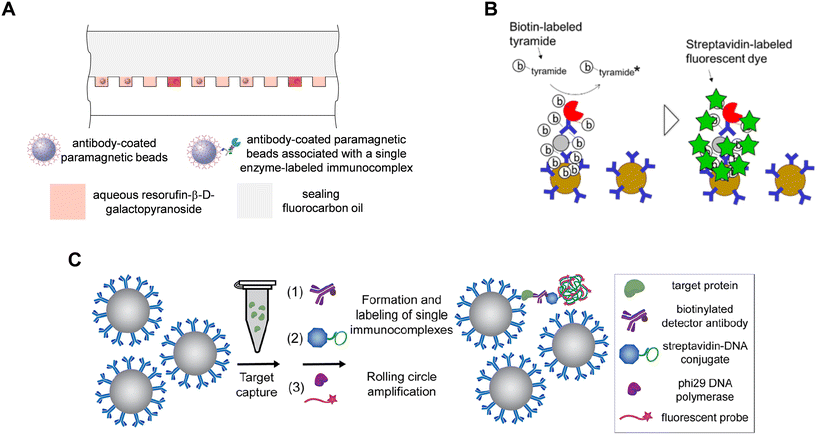 |
| Fig. 3 Approaches to detecting single molecule labels in DBA. A) Sealing of enzyme-labeled beads with diffusible substrate in arrays of microwells (ref. 55). B) Fluorescent labeling of proteins on beads via tyramide signal amplification (TSA) (ref. 60). C) Formation of DNA concatemers on beads using rolling circle amplification (RCA) followed by labeling with complementary fluorescent probes (ref. 63). Figures reproduced with permissions. | |
The tyramide signal amplification (TSA) detection chemistry has been used to perform digital ELISA without needing to seal beads in microwells (Fig. 3B).60,61 In this approach, the bead-bound immunocomplexes were labeled with streptavidin-HRP conjugates, and the HRP enzyme-substrate reaction created fluorescent tyramide radicals that react with aromatic amino acids of proteins associated with the beads, covalently labeling the beads directly with fluorophores. This approach has been used to develop digital ELISA based on detection of TSA-labeled beads using flow cytometry,60 and imaging of beads randomly dispersed on planar substrates60 and in hydrogel films.61 While TSA is a useful approach that avoids the need for microwells, the tyramide radicals are still free to diffuse in solution, so in principle can covalently label remote beads or proteins in solution leading to increased assay backgrounds.
Amplification of nucleic acid labels offers an attractive approach to single molecule counting on beads, and for some methods the detected labels are directly associated with the bead. For example, single molecule labels formed by rolling circle amplification (RCA) have been developed by the Walt lab (Fig. 3C).62,63 Unlike TSA, in RCA the fluorescent labels do not diffuse away from the beads making the method more specific and have lower backgrounds. In this approach, immunocomplexes on beads were labeled with streptavidin conjugated to a circularized DNA primer, and RCA by a polymerase was performed on all beads in bulk solution. For each single streptavidin-DNA conjugate, the RCA polymerase created a concatemer of DNA repeat sequences that were then hybridized with fluorescently labeled complementary probes, thereby directly labeling the beads with 100 s or 1000s of dye molecules. On- and off-beads were identified either by imaging of randomly dispersed beads dried in monolayer films62 or by flow cytometry.63 Another approach based on nucleic acid amplification is to perform immuno-PCR on single immunocomplexes associated with beads: Zhou proposed this concept with the beads isolated in droplets.64 While they did not report immunoassays, Ramsey and co-workers demonstrated digital detection of beads of PCR products from single primer labels on beads sealed in arrays of microwells.65
Liu and co-workers have shown proof-of-concept of another single molecule approach that localizes fluorescence on individual beads, and allows on- and off-beads to be identified using flow cytometry.66,67 In this approach, a single T4 polynucleotide kinase phosphatase catalyzes step-by-step dephosphorylation of nucleic acid substrates immobilized on the beads. Subsequently, terminal deoxynucleotidyl transferase (TdT) elongates the 3′-OH modified substrates that then bind to complementary fluorescent probes making the bead fluorescent.66 While the authors have used this approach to develop a sensitive assay for PSA based on the average intensity of a large population of beads,67 they have not yet demonstrated its use in DBA.
3.3 Analysis of beads
The detection requirement of DBA is to determine the on and off status of large number of individual capture beads (Fig. 1). Multi-wavelength imaging of arrayed beads has been the most common approach to analyzing beads, and flow systems have also been used as a detection approach. Developments in these two areas have focused on maximizing the efficiency of analyzing beads while reducing the cost and complexity of the instrumentation and consumables.
Imaging of arrayed beads.
Approaches to DBA based on arraying and imaging of beads (Fig. 4) require three key components: 1) a substrate or consumable that allows singulation of the beads; 2) an optical detection system that allows imaging of both the beads and single molecule labels; and, 3) image analysis methods that determine the location of beads, their on and off status, and their intensities. The first approach for imaging beads for digital protein detection was based on glass fiber bundle microwells arrays.8,47 In this approach, microwells were etched into the fiber bundle arrays,68 and the beads were imaged initially using a commercial off the shelf microscope and subsequently a custom microscope that allowed an 8-array fiber bundle consumable to be imaged. Standard microscopes worked well for imaging these sized arrays (50
000 wells) as high numerical aperture (NA) imaging can be achieved at these relatively smaller FOV (approximately 4 mm2). Images were analyzed using standard segmentation approaches to identify beads from their scatter in bright-field images (which also identified the location of wells), and the β-galactosidase labels were identified from the growth in intensity of beaded wells in the resorufin fluorescence image.
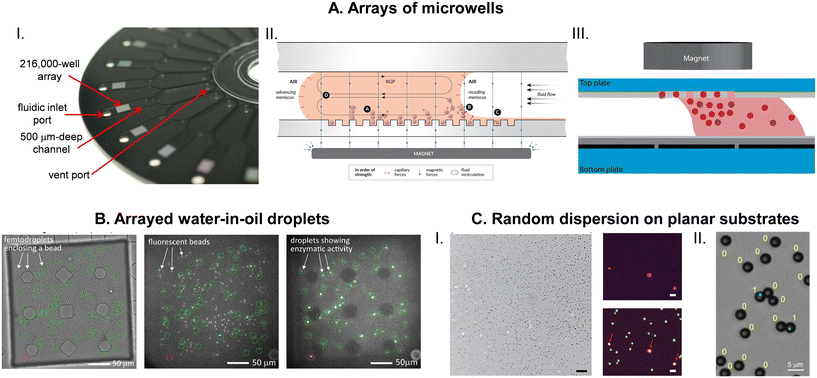 |
| Fig. 4 Approaches to isolating beads and single molecule labels in DBA using imaging detection. A) Methods based on sealing beads in arrays of microwells: I. COP disk containing 24 microwell arrays and fluidic channels for oil sealing (ref. 55); II. magnetic-meniscus sweeping (MMS) of beads over microwell arrays for high efficiency bead loading (ref. 54); III. loading of beads in HIH microwell arrays using DMF (ref. 73). B) Partitioning of beads in water-in-oil droplets and formation of droplet rafts in microfluidic chambers (ref. 76). C) Methods based on random dispersion of beads on substrates where the labels being detected do not diffuse: I. drying of monolayers of beads associated with single labels formed by RCA (ref. 62); II. Dispersion of beads associated with single labels formed by TSA on glass slides (ref. 60). Figures reproduced with permissions. | |
We subsequently reported a disk-shaped consumable for DBA that contained 24 arrays each with 239
000 microwells (Fig. 4A).55 This device was composed of two halves bonded together. The bottom half contained the microwell arrays that were molded in transparent cyclic olefin polymer (COP) using DVD replication. The top layer contained fluidic channels molded in COP loaded with carbon black. This consumable allowed the delivery of the bead-RGP mixture and subsequent oil for sealing, thereby enabling automated readout of digital ELISA on multiple arrays. The device had several benefits over the original glass fiber arrays. It was a low-cost disposable manufactured using a proven, highly scalable process (nanoscale injection molding). It had greater physical robustness than the fragile glass arrays, and the disk-shape was well suited for integration into an automated digital ELISA system.50,55 COP proved to be a good material for this application as it had low background fluorescence across a wide range of wavelengths, and its surface energy allowed both the filling of wells with beads and sealing with oil.50 This disk was also the basis for the magnetic meniscus sweeping (MMS) bead loading method (Fig. 4A) that allowed low bead numbers to be used (5000 per assay54 compared to the 500
000 beads used in the original report)8 that resulted in improvements in sensitivity sometimes in excess of 100-fold.54 In this approach, bead loading efficiency was increased by sweeping the bead-substrate solution over the microwell array with a magnet under the array. The magnet localized high concentrations of beads over the array, and high downward capillary forces at the receding meniscus pushed beads into the wells helping to improve the efficiency of bead analysis from 5% to 50%. Wider FOV optics allowed all the wells in the array to be imaged.
Imaging of the larger arrays on the disk (approximately 14 mm2) required the development of a specialized optical system that had a wider FOV than a typical commercial fluorescence microscope.69 A customized objective lens enabled a wide FOV (3.8 mm × 2.8 mm) while maintaining a high NA (0.3) to allow high efficiency of light collection across a wide range of wavelengths (488 to 850 nm). The guiding optical design metric was optical crosstalk defined as the bleeding of light energy from one well to an adjacent well. Minimizing crosstalk becomes particularly important for multiplex digital ELISA where 2 different bead types can be in adjacent wells, one bead associated with zero labels and one with 20–30 labels.52 Crosstalk from the high AEB on-bead into the off-bead has the potential to create false on-beads, and limit the dynamic range across which mixing of different bead types can work. Using this optical detection system, 7 images were acquired for each array: a bright-field image was used to identify wells using image segmentation and remove any areas of the image impacted by debris; 4 fluorescence channels were used to identify multiplex beads using histogram analysis;52 and two images spaced by 30 s were acquired at the resorufin fluorescence wavelength to identify those beads associated with at least one enzyme. For the latter, an on-bead was defined as exceeding a fixed intensity growth threshold within the beaded well between the two resorufin fluorescence images. The use of two resorufin fluorescence images and an enzyme label helped increase the SNR because enzymatically generated fluorescence changed over time and static background fluorescence could, therefore, be subtracted.
Another approach to creating arrays of microwells in polymers was based on hydrophilic wells in hydrophobic surfaces (HIH) that was developed by the Noji56 and Lammertyn57 groups. Kim et al.56 fabricated arrays of ∼1 million HIH microwells by etching a hydrophobic polymer spin coated on glass using a photolithographically defined resist mask. The hydrophilic glass bottom of the microwells helped to facilitate loading of the bead-substrate solution and stabilized the filled wells as the oil sealed against the hydrophobic surface of the well array. Bright-field and fluorescence imaging of the large array was achieved in 8 min by step-and-repeat imaging using a standard fluorescence microscope. The Lammertyn group fabricating 62
500 HIH microwells in a 4 mm2 area by patterning using dry lift-off a layer of Teflon-AF on glass.57 A bright-field image was used to identify beads, and fluorescence images at fluorescein wavelength used to distinguish on- and off-beads. On- and off-beads were determined using size thresholding filters in the open-source ImageJ software. These researchers used this approach to demonstrate assays for the nucleoprotein of influenza A57 and tau in the serum of Alzheimer's disease patents70 with LODs of 4 fM and 55 aM, respectively. This group has reported methods for fabricating the arrays of HIH microwells by imprinting using a polydimethylsiloxane (PDMS) stamp71 and by reaction injection molding72 that demonstrated their potential as cost-effective disposable consumables. Witters et al.73 integrated HIH microwell arrays with digital microfluidics (DMF) to efficiently load beads into wells (Fig. 4A). Araci et al.74 fabricated devices based on the microfluidic very large-scale integration (mVLSI) technology in which the reaction chambers for each bead can be addressed individually. They demonstrated a digital ELISA for TNF-α using this method, although the sensitivity was likely limited by the mVLSI array that only contained 400 reaction chambers. Other groups58,75 have used microwells in PDMS made using standard soft lithographic processes for performing DBA.
As mentioned above, several groups have demonstrated imaging approaches that do not require microwell arrays, where randomly deposited beads are either dried on a planar substrate,60,62 or immobilized in a hydrogel film.61 Imaging of beads for these methods were based on the standard bright-field (beads) and fluorescence (labels) imaging on conventional fluorescence microscopes, and image analysis was based on segmentation methods developed in MATLAB or ImageJ. The identification of on- and off-beads was achieved by Gaussian fits to the fluorescence intensity histogram of the two populations. These approaches require chemistries where the fluorescent product remain associated directly with the beads, and the lack of a microwell grid likely places a greater burden on image analysis to identify beads.
Another approach to singulating beads without using microwell arrays is to isolate them in water-in-oil droplets generated in microfluidic devices – a technology that has been successfully employed for performing digital PCR – and imaging self-assembled arrays of the droplets to identify on- and off-beads. Shim et al.76 first demonstrated this approach by isolating 1 μm diam. beads labeled with β-galactosidase in FDG-in-oil droplets with volumes of 5–50 fL. After formation, the droplets were moved to a 2 mm × 7 mm chamber supported by posts where they assembled into monolayers and were imaged in bright-field to identify droplet location, red fluorescence to identify beads, and green fluorescence to identify on-beads (Fig. 4B). Using this approach they imaged 20
000 droplets, 1900 of which contained beads and demonstrated a digital ELISA for PSA with an LOD of 46 fM. The efficiency of the method was low, with only 0.0016% of beads analyzed. Cohen et al.77 significantly improved the efficiency of this approach using larger droplets (pL) and optimizing the design of the droplet generation microfluidics and droplet array chamber to minimize bead loss and maximize droplet packing. They also developed image analysis algorithms to efficiently identify droplets, beads, and labels from the three images. As a result, they were able to analyze 20–30% of the 100
000 beads used in the assay, resulting in LODs of 30 aM and 20 aM for IFN-γ and IL-2, respectively. Yi et al.78 have reported a multiplex digital ELISA based on detection of on- and off-beads in droplet “rafts”, using polymeric HRP as the detection system and beads encoded by impregnation of multiple dyes. As mentioned above, droplet arrays have also been used to read out bead-based immunoassays using PCR-generated labels.64 While the author proposes this approach as a way to determine on- and off-beads, the data only contained information regarding on-beads, making it ambiguous if the assay was detecting single molecule labels.
Flow detection.
The Issadore group developed an integrated system for DBA where the beads were isolated in water-in-oil droplets, and the droplets were analyzed by flowing them in a microchannel over an imager based on a mobile phone and complex video analysis algorithms.59 In this approach, beads labeled with HRP were isolated in 23 pL droplets of a fluorogenic substrate to HRP, and the droplets were imaged at high speed in flow using a time domain-encoded approach at 3 excitation wavelengths. This approach allowed beads to be analyzed at 100 MHz with assay sensitivities similar to existing commercial digital ELISAs. Recently, Yue et al.79 reported a novel microfluidic device that improved the efficiency of placing single beads in single droplets by particle ordering using Dean flow, overcoming the inefficiency of using a Poisson distribution to isolate single beads in droplets.
Commercial flow cytometers have been used to analyze beads in DBA.60,63,66 Flow cytometers have been used extensively to analyze other bead-based immunoassay formats, albeit at high concentrations where every bead needs at least hundreds of label molecules bound to it to be detected.80 The use of flow cytometry in DBA has been enabled by the development of single molecule labels that are sufficiently bright and remain associated with the bead during flow analysis. The use of flow cytometry has several benefits over imaging approaches. First, the method does not require a specific consumable to be developed for isolating and detecting beads. Second, the number of beads analyzed is not limited by the “real estate” of microwells or the FOV of an imaging system. Third, crosstalk is theoretically lower as beads can be more widely spaced compared to microwell arrays. Finally, this approach can leverage the existing infrastructure of flow cytometry that is predominantly used to analyze cells. Flow cytometric analysis of digitized beads also has some potential pitfalls, including clogging of channels by aggregated beads, and the need for extra care to avoid cross contamination of beads between samples.
Akama et al.60 first reported the use of flow cytometry for analyzing digital immunoassay beads. Single immunocomplexes on beads were labeled using TSA, and the beads were analyzed on a FACSVerse (BD Biosciences). Singulated beads were identified by gating the forward scatter and side scatter intensities, and on-beads were identified based on exceeding a threshold in a fluorescence channel that measured the TSA label. Recently, Wu et al.63 deployed their single molecule RCA label chemistry to allow multiplex detection of on- and off-beads on a flow cytometer. Forward and side scatter were used to identify the overall bead population, and gating in multiple channels was used to identify individual sub-populations of dyed beads. On- and off-beads were identified from fitting 2 Gaussian profiles to the fluorescence intensity of the beads at the wavelength of the RCA probe. They utilized the high efficiency read out of flow cytometry to analyze a high fraction of the beads used in the assay (50%), enabling them to adopt a low bead number approach that increases assay slope and improves sensitivity.54 They demonstrated an 8-plex of cytokines with LODs ranging from 6 aM to 230 aM, and demonstrated improved detectability in clinical samples compared to digital ELISA using higher bead numbers. As described above, Zhang et al.66 use the T4 polynucleotide kinase phosphatase label to distinguish on- and off-beads in a flow cytometer.
3.4 Binding reagents
The base substrate for DBA has been spherical superparamagnetic beads to which the capture binding molecules are attached (Fig. 1). The capture binding molecules are usually antibodies to enable detection of specific proteins, but several other types of binding reagents have been used in DBA to detect different classes of molecules (Fig. 5). Several commercial sources of these beads are available that use different manufacturing methods to incorporate iron into the polymeric structure of the bead. Each type of bead is usually available in multiple surface chemistries to attach capture reagents. Most of the digital detection methods developed have used polystyrene-based beads with a diameter of ∼3 μm, although some approaches used 1 μm diam. beads.74,76 ∼3 μm diam. beads was a propitious choice for digital ELISA. First, the fabrication and imaging of microwells or droplets over a sufficient FOV is relatively straightforward at this length scale. Second, each bead can be modified with 105–106 antibodies per bead, such that for the 5000 to 500
000 capture beads typically used to get a Poisson distribution at subfemtomolar concentration there is sufficiently high antibody concentrations to allow efficient capture of proteins.51 The most important properties of these beads for application in digital protein detection are: 1) monomericity, i.e., low aggregation; 2) consistency in bead shape and size; and, 3) the number and availability of the coupling groups on the surface of the beads. Fortunately, manufacturers such as Agilent (Lodestar beads) and Thermo Fischer (Dynal beads) have developed processes that provide this level of control of bead properties.
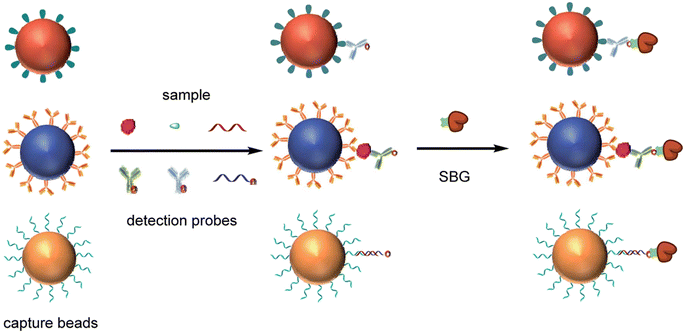 |
| Fig. 5 Examples of different capture binding molecules used in DBA (ref. 91). Figure reproduced with permission. | |
The most common way to attach binding reagents to these beads is via random coupling of amine groups on the antibodies to COOH groups on the beads via a crosslinker. This approach has the benefit of allowing high concentrations of antibodies to be loaded onto the beads without loss of binding at the variable region as most isotypes have several amines in their constant region. Other surfaces such as tosyl81 and epoxy60 have also been used to attach antibodies to the beads. Multiplexing beads adds an extra complexity to the design of the beads, as sufficient dye must be incorporated into the bead for it to be detected without interfering with the signal molecule detection channel. Conventional fluorescent magnetic beads that have dyes impregnated in their cores tend to be too bright for this application. To address this challenge, dyes were attached at low concentrations to the COOH groups on the beads before attachment of the capture antibodies.52
Detection antibodies are usually biotinylated via modification of free amines on the constant region of the antibody.82 These molecules are then labeled with conjugates composed of streptavidin and the single molecule label. For these streptavidin conjugates, it is important that the molecules are close to monomeric and not crosslinked. Commercial sources of streptavidin conjugates used in analog methods tend to contain crosslinked aggregates leading to high non-specific binding and heterogeneous single molecule signals. As a result, researchers developing DBA often develop custom monomeric conjugates.8,62
The sensitivity of DBA depends on the concentrations, affinity, and specificity of the binding reagents used. Kinetic models that incorporate these parameters allow researchers to predict ALB for particular reagents, and the comparison of these predictions to experimental data has led to significant insights into the design of DBA.51 Specifically, by measuring ALB and knowing the total number of beads in an assay, DBA allow us to determine straightforwardly the number of molecules detected and, therefore, the overall efficiency of the assay (= number of labels detected ÷ number of protein molecules in the sample). Based on this information, it is possible to test kinetic models of immunocomplex formation that depend on variables such as on- and off-rates of antibodies and reagents, reagent concentrations, number of beads, incubation times, etc. These models can then be used to make predictions on assay performance as function of these variables, allowing the sensitivity of assays to be optimized systematically. For example, we reported a kinetic model that predicted AEB for the formation of immunocomplexes on beads.51 The model was based on bimolecular association and dissociation for each step of the immunocomplex formation, with the assumption that kinetics were not limited by diffusion of the proteins to the beads. From knowledge of the concentration of the protein and reagents, the number of beads, the kon and koff of each interaction, and the time of binding, we were able to predict AEB and compare these values to experimental data. Despite the simplicity and assumptions of the model – and the limited amount of data on kon and koff – the predicted AEB values were close to experimental data, and allowed some critical insights into the parameters important in detection. Most notably the model predicted that using fewer beads would result in greater sensitivity if: a) the on-rates of the capture antibodies can compensate for the drop in antibody concentration caused by using fewer beads, and still result in a high efficiency of protein capture on the beads; and, b) the background of the assay does not change when using fewer beads. The underlying basis of the improvement in sensitivity was that the DBA signal = molecules/beads that increases at a fixed concentration by using fewer beads. So, if background is unchanged then the signal-to-background or slope of the assay – and therefore its sensitivity – increases with fewer beads. We demonstrated this effect by testing beads numbers down to <2000 beads until too few beads could be detected for quantification.54 The improvement in sensitivity varied between different proteins in a way consistent with the kinetic model. For some proteins, this approach allowed LODs at subattomolar concentrations, i.e., down to as few as 6 proteins in a 200 μL sample. These observations were supported by subsequent reports of improved sensitivity using very low bead numbers in another DBA.63 These demonstrations indicate that the digital bead protein detection offers a viable path to the most sensitive immunoassays possible.
In general, the kinetic model and experimental data supporting it indicated the importance of the kinetic properties of the capture antibody in these assays. Capture antibodies must have high enough on-rates to kinetically overcome the use of low bead numbers that drive down antibody concentration while increasing the slope of the assay signal, whereas detection antibodies can be incubated at high concentrations to overcome kinetic deficiencies, if they do not add excessive backgrounds to the assays. Tables 1 and 2 illustrate how the on- and off-rates of the binding of the protein to the capture antibodies can affect both the capture efficiency of the protein and the expected benefit going to lower bead assays, respectively.
Table 1 Percentage of proteins captured on beads as a function of the on- and off-rates of the capture antibody–protein interaction as per the biomolecular association model described in ref. 51. The calculations used 500
000 capture beads with 274
000 antibodies per bead, and beads incubated with 0.1 mL of sample for 10
000 s at a protein concentration of 0.1 fM. High efficiency capture (≥95%) conditions are highlighted in bold text. The combinations of kon and koff of equal affinity equal to Kd ∼ 0.1 nM are italic
|
k
off (s−1) |
3.1 × 10−2 |
3.1 × 10−3 |
3.1 × 10−4 |
3.1 × 10−5 |
3.1 × 10−6 |
3.1 × 10−7 |
3.1 × 10−8 |
k
on (M−1 s−1) |
2.7 × 102 |
0% |
0% |
0% |
1% |
1% |
1% |
1%
|
2.7 × 103 |
0% |
0% |
2% |
5% |
6% |
6%
|
6% |
2.7 × 104 |
0% |
2% |
16% |
40% |
45%
|
45% |
46% |
2.7 × 105 |
2% |
16% |
66% |
95%
|
99%
|
100%
|
100%
|
2.7 × 106 |
16% |
66% |
95%
|
99%
|
100%
|
100%
|
100%
|
2.7 × 107 |
66% |
95%
|
99%
|
100%
|
100%
|
100%
|
100%
|
2.7 × 108 |
95%
|
99%
|
100%
|
100%
|
100%
|
100%
|
100%
|
Table 2 Fold increase in AEB going from 500
000 to 5000 capture beads as a function of the on- and off-rates of the capture antibody–protein interaction as per the biomolecular association model described in ref. 51 and 54. The calculations assumed 274
000 antibodies per bead, and beads incubated with 0.1 mL of sample for 10
000 s at a protein concentration of 0.1 fM. Increases in AEB that approach the maximum theoretically possible (100-fold) are highlighted in bold text. The combinations of kon and koff of equal affinity equal to Kd ∼ 0.1 nM are italic
|
k
off (s−1) |
3.1 × 10−2 |
3.1 × 10−3 |
3.1 × 10−4 |
3.1 × 10−5 |
3.1 × 10−6 |
3.1 × 10−7 |
3.1 × 10−8 |
k
on (M−1 s−1) |
2.7 × 102 |
1.0 |
1.0 |
1.0 |
1.0 |
1.0 |
1.0 |
1.0
|
2.7 × 103 |
1.0 |
1.0 |
1.0 |
1.0 |
1.0 |
1.0
|
1.0 |
2.7 × 104 |
1.0 |
1.0 |
1.2 |
1.3 |
1.3
|
1.3 |
1.3 |
2.7 × 105 |
1.0 |
1.2 |
2.8 |
5.3
|
5.8 |
5.9 |
5.9 |
2.7 × 106 |
1.2 |
2.9 |
16.7
|
39.9 |
44.9 |
45.5 |
45.5 |
2.7 × 107 |
2.9 |
17.1
|
66.3 |
95.0
|
99.3
|
99.7
|
99.8
|
2.7 × 108 |
17.1
|
66.3 |
95.2
|
99.5
|
99.9
|
100.0
|
100.0
|
A different perspective was provided by Dinh et al.83 who tested 6 different variable region, heavy-chain antibody binding domains (VHHs) to botulinum neurotoxin – for which they measured on- and off-rates – as both capture and detection reagents in digital ELISA. They identified the off-rate of the detection antibodies as a key determinant of assay sensitivity. The on-rates of the capture antibodies were not an important factor in sensitivities for these reagents, likely because the kon varied by less than 1 log across the 6 capture molecules, whereas koff values spanned more than 3 logs. Clearly, further studies of how binding affinities affect ALB are needed to give a holistic understanding of how the sensitivity of DBA can be optimized.
The importance of the binding reagents on the performance of the digital bead assays has led to efforts to engineer their properties. Aptamers—nucleic acids that are selected from libraries to bind to specific proteins—have been used in DBA, although so far they have only performed well as detection reagents and not as capture reagents.58,84 The reasons for the limitations of aptamers as capture reagents is not clear, although it may be due to differences in the kinetic properties of these reagents or how they present on the capture substrate compared to antibodies.
The DBA concept has been applied to single molecule counting of different types of molecules, including different classes of proteins, by changing the capture and detection reagents. For example, the detection of serological antibodies was enabled by using antigens as the capture reagents on beads. An impressive demonstration of this capability was provided by Norman et al.85 who immobilized 4 viral antigens from SARS-CoV-2 onto 4 different dye-encoded beads, and used multiple different anti-isotype detection reagents to IgG, IgM, and IgA to serologically profile the blood of COVID-19 patients. These researchers also combined serological and viral antigen detection in a single assay.86 By formatting competitive assays in the digital format, it is possible to detect small molecules using DBA,87 although as the number of enzymes per bead increases with decreasing concentrations of target analyte in a competitive assay, the sensitivity benefits of digital counting are not obvious. Going beyond the boundaries of immunoassays, nucleic acids can also be detected with PCR level sensitivity using nucleic acids as capture and detection reagents.88–90 The Walt lab developed a multiplex DBA that combined digital detection of a protein, a small molecule, and a nucleic acid (Fig. 5).91
3.5 Integration of assay steps with single label counting
An important aspect of DBA that is not often described in detail is the process for performing the capture and labeling steps (panels 1–2 in Fig. 1) prior to detection of the beads. If these assay steps are not performed correctly, the assays may have high backgrounds and will likely be imprecise: the benefits of single label counting will be lost, and the assays will not be sensitive. The nature of DBA and their high sensitivity place more exacting demands on the systems that perform the assay steps compared to those used for performing traditional, analog immunoassays of lower sensitivity. The systems that perform the assay steps must be designed to achieve three specific goals: 1) efficient dispersion of beads into the total volume of the sample or reagent; 2) low residual volumes after the sample or reagent are removed; and, 3) low bead loss.
Efficient dispersion of beads is important so that the beads are evenly distributed throughout the liquid and molecules distribute over the beads according to Poisson statistics. Incomplete or inconsistent dispersion of beads can lead to non-Poisson distribution of molecules over the beads, subpopulations of bead signals, and imprecise results. Bead dispersion is typically achieved using active agitation of the sample-bead mixture, e.g., using an orbital shaker for microtiter plates54,90 or a customized shaker.50 Low residual volume (<2 μL from an initial 100 μL) is particularly important after the final labeling step, as the concentration of labels is reduced by serial dilution of multiple wash steps. High residual volumes would result in insufficient dilution of the label and elevated background signals from free enzymes trapped in beaded microwells. Low residual volumes have been achieved by using needles to aspirate the supernatant liquid from tapered cuvettes or wells.54,50 Bead loss at each pelleting-aspiration step of the assay must be <1% for the cumulative bead loss to be <10%. If bead loss during the assay steps exceeds this value, then there may be insufficient beads for a quantitative measurement. For the most sensitive assays based on using very low numbers of beads,54,63 minimizing bead loss becomes even more critical to maintain sufficient number of beads and positive beads. These methods were based on approaches that yielded high bead read efficiencies (∼50%).
In general, there are three approaches to performing the assay steps: manual pipetting, robotic pipetting systems and washers, and microfluidics (Fig. 6); systems often combine 2 or 3 of these approaches. We have reported a fully automated system for performing digital ELISA that was based on robotic pipetting and customized equipment for washer and shaking the beads (Fig. 6B).50 This approach included a microfluidic consumable for the delivery of beads to the arrays of microwells that could be integrated into the automated robotic system.55 By requiring minimal manual involvement in the assays steps, this system was able to achieve high sensitivity and precise assays, and a samples-in/results-out workflow for the user. The precise timing of each step in the assay also minimized imprecision in AEB caused by dissociation of detection antibodies from immunocomplexes.51 Full automation using pipettors resulted in this instrument being relatively large and floor-standing. Chiba et al.92 recently reported a desktop fully-automated analyzer for performing Simoa assays using a pipettor-based system for bead incubation and washing, and microwell arrays in COP; this system was used to measure antigen from SARS-CoV-2 in nasopharyngeal swabs. A more flexible automation approach was reported that separated the instrumentation that performed the assay and bead detection steps (Fig. 6A).54,90 Beads, samples, and reagents were manually pipetted into 96-well microtiter plates, beads were resuspended by shaking the plates on an orbital shaker, and beads were washed using a dedicated 96-well plate washer modified with a customized magnet. As a result, the bead imager could fit on a benchtop. The system was designed to make the assay results robust to user variability in the manual pipetting steps, but the inclusion of manual steps does increase the potential for more variation in timing compared to an automated system.
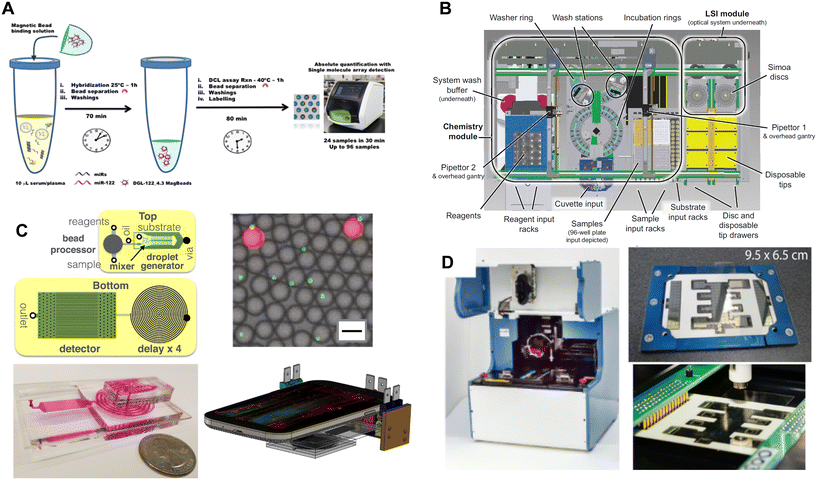 |
| Fig. 6 Approaches to integrating the assay and single molecule detection steps in DBA. A) Manual pipetting of reagents and automated washing (ref. 90). B) Fully automated system for assays and imaging (ref. 50). C) Miniaturized assay processing based on pressure-driven microfluidics and flow detection on a mobile phone of beads in water-in-oil droplets (ref. 59). D) Miniaturized assay processing based on DMF (ref. 81). Figures reproduced with permissions. | |
Microfluidics offers a path to fully automated DBA in much smaller, lower cost equipment. These approaches will likely be necessary for DBA to achieve widespread adoption outside of clinical and research laboratories. The most impressive demonstration of the potential of integrating DBA with microfluidics was provided by the Issadore group (Fig. 6C).59 They demonstrated a system that performed the assay steps and bead detection steps in a single microfluidic device. The assay steps were performed in a bead processor based on a semi-permeable membrane, beads were then isolated in substrate-in-oil droplets, and the droplets were imaged in a flow channel by a mobile phone imager as described above. Using this device, they achieved subfemtomolar LODs that matched the sensitivity of the commercial assays performed on the floor-standing unit. This group has since used this technology to detect single extracellular vesicles by capturing them on magnetic beads via their surface antigens.93 The Lammertyn group have focused on the use of electrowetting-on-dielectric (EWOD) techniques for automating the steps of DBA in digital microfluidic (DMF) devices.73,81 EWOD has been used previously to automate analog bead-based immunoassays,94,95 as well as other analytical methods such as PCR and NGS sample prep. Lammertyn et al.73 have used EWOD combined with magnets to load beads into arrays of HIH microwells and demonstrate digital ELISA. Recently, this group reported an integrated DMF-Simoa device that allowed the assay steps and detection process to be performed in a semi-automated fashion (Fig. 6D).81 They used this system and the high sensitivity of DBA to demonstrate an assay for thyroid stimulating hormone (TSH) that was fast (5 min sample incubation) and used a small sample volume (1.1 μL) to demonstrate the potential of DBA for point-of-care applications. The digital assay for TSH was twice as sensitive as the corresponding Abbott ARCHITECT assay despite using 135-fold less sample volume.
An important consideration for manual, robotic, and microfluidic approaches is the pre-analytical treatment of samples, often called “sample prep”. Stated simply, DBA works well if the target proteins are in solution and largely free of particulates. Particulates tend to cause beads to aggregate and interfere with protein capture and labeling steps, so they need to be removed. For example, serum and plasma samples are usually centrifuged to remove particulates before beads are added. Apart from centrifuging or filtering out particulates and dilution in buffer, DBA requires little sample preparation compared to PCR, for example, that requires the nucleic acid to be purified from the sample. So, for example, toxins from C. diff. bacteria have been detected using digital ELISA simply by diluting stool 20-fold in an extraction buffer and filtering to remove all solid matter.96
4. Emerging approaches to digital protein detection
Besides DBA, there have been many recent innovations in the measurement of proteins based on single molecule detection. These innovations often result from solving three technical challenges: discrimination of single labels with high SNR; spatial isolation of the labeled proteins; and detection of large numbers of single labels. Table 3 highlights different approaches developed to overcome these challenges by the methods described in this section. The methods developed can be classified into 5 categories (Fig. 7) that we describe in turn: digital counting of nucleic acid labels, single nanoparticle labels, use of nanopore arrays, exploiting the kinetics of single binding events, and other methods.
Table 3 Summary of the approaches taken to solve the 3 main technical challenges in developing digital protein detection methods. Illustrative references are provided for each approach; the main text contains more comprehensive references
Discrimination of single labels |
Spatial isolation of labels |
Detection of many single labels |
Enzymes confined in wells8 or droplets76 |
Microwell arrays55,140 |
Wide FOV, high resolution multi-wavelength imaging69 |
Amplification of nucleic acid labels in droplets64 |
Arrays of droplets77 |
Flow combined with laser-induced fluorescence43 |
Labels directly associated with beads60,63 |
Flow of droplets59 |
Electrical126 |
Nanoparticles33,103 |
Random dispersed arrays62 |
Wide FOV plasmonic imaging104 |
DNA nanostructures127 |
Nanopore arrays126 |
Scatter imaging119 |
Laser induced fluorescence of bound labels39 |
Flow cytometry60 |
Coincidence detection113 |
Laser induced fluorescence of released labels42 |
Nanostructured surfaces135 |
Time-resolved fluorescence (TIRF) microscopy37 |
Particle motion119,120 |
|
Mass spectrometry142 |
Differential kinetics of specifically bound labels123 |
|
Transmission electron microscopy113 |
Nanopore blockade125 |
|
Surface plasmon imaging123 |
Nanopore translocation127 |
|
|
Microbubbles137 |
|
|
Diamond nitrogen vacancy centers141 |
|
|
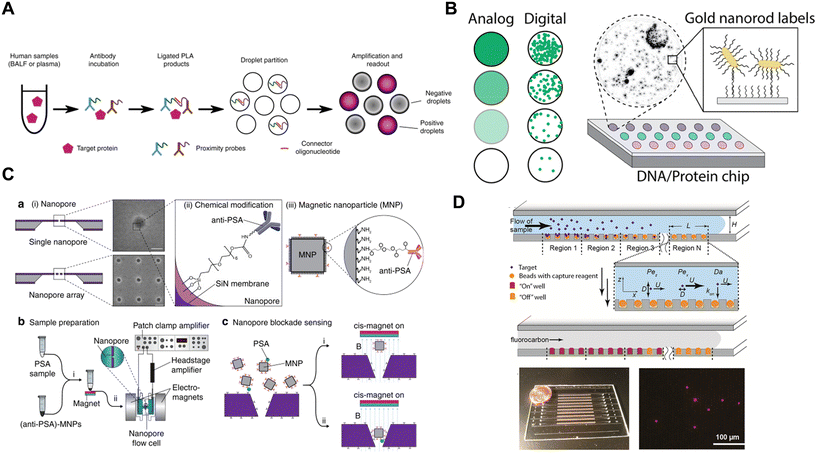 |
| Fig. 7 Examples of emerging methods for digital detection of proteins. A) Digital readout of nucleic acid labels (ref. 98). B) Nanoparticle labels (ref. 104). C) Nanopore detection (ref. 125). D) Exploiting the kinetics of binding of single labels (ref. 131). Figures reproduced with permissions. | |
4.1 Digital counting of nucleic acid labels
As mentioned in section 2, a common approach to improving the sensitivity of immunoassays has been to label detection antibodies with a nucleic acid and detect the products of the amplified labels, using methods such as immuno-PCR, PLA, and immuno-RCA; some of these approaches have been digitized. The original developers of PLA digitized the method to improve the precision of the assay over the conventional readout of the ligation product.24 They formed immunocomplexes on magnetic beads, with 2 detection antibodies providing the pair of nucleic acids for ligation. The ligation product was released from the beads, circularized, and amplified using RCA. The resulting concatemers were labeled with fluorescent probes, and RCA concatemers were counted in a microfluidic flow channel using laser-induced fluorescence detection. They demonstrated an assay for IL-6 with an LOD of 5 fM and an average coefficient of variation (CV) of 7% compared to 18% for the conventional PCR readout method, a promising advance that addresses the imprecision of analog PLA. The Tay group97,98 have also reported a digital PLA method that they used to detect proteins from single cells (Fig. 7A). They lysed mammalian cells and performed PLA in solution using two nucleic acid-labeled antibodies. After digestion of the proteins, the double-stranded ligated DNA was distributed over 20
000 water-in-oil droplets and droplet digital PCR performed in a commercial microfluidic device to quantify the amount of label. Using this method, they achieved femtomolar LODs for 3 different proteins. These authors improved the sensitivity of the method 55-fold by developing a microfluidic device for manipulating single cells and performing digital PCR that reduced the dilution needed for handling of the sample in the original microtiter plate assay.99 Byrnes et al.100 have also reported a digital droplet immunoassay based on PLA readout in polydisperse droplets, and used it to develop a wash-free assay for IL-8 with picomolar detection limits. Schröder et al.101 have digitized immuno-PCR using water-in-oil microdroplets, calling it digital droplet immuno-PCR (ddIPCR). They formed oligonucleotide-labeled immunocomplexes at the surface of an ELISA plate, chemically released the label from the surface, partitioned the labels into droplets using a commercial droplet generator, performed PCR on the droplets, and then analyzed the on/off status of the droplets using a commercial droplet reader. By digitizing the assay, they demonstrated an average CV of 5% for ddIPCR assays for IL-2 and IL-6.
4.2 Single nanoparticle labels
Many of the early attempts at single label counting for protein detection were based on nanoparticles, and this approach has continued to be extensively explored. Farka et al.102 have provided a comprehensive review of all the different types of nanoparticles used in immunoassays.
The Ünlü group developed an immunoassay based on counting individual gold nanoparticles (AuNPs).103 In this method, capture antibodies were immobilized on a functionalized silicon dioxide surface and proteins were captured from undiluted serum or whole blood. 40 nm AuNPs were functionalized with detection antibodies that bound to the captured proteins. Scatter from individual AuNP labels was measured using interferometric reflectance imaging sensor (IRIS)—a method that has the potential for wide FOV, low-cost imaging of single nanoparticle labels—and they demonstrated femtomolar LODs for β-lactoglobulin. As for other surface-based counting methods, the labels must be spatially separated to enable counting of single labels. As a result, very wide FOV are needed for sensitive detection, and these authors provided an analysis of LOD as a function of sensor area. Subsequently, this group improved this approach to allow scanning of wider FOV using IRIS and used gold nanorods to enable counting of single labels in protein microarrays (Fig. 7B).104 Jing et al.105 used plasmonic imaging to detect single AuNPs in immunocomplexes formed at functionalized gold-coated glass surfaces, and demonstrated a digital immunoassay for procalcitonin. This imaging method was able to track the binding and unbinding of individual particles. These authors extended the method by generating gradients in signal as the sample flowed over the capture surface in a microfluidic channel.106 This gradient approach allowed the authors to actively correct for assay background within each sample, and they demonstrated an assay for troponin with an LOD of 6 pg mL−1, comparable to clinical high sensitivity assays. Belushkin et al.107 used a similar approach and plasmonic hole arrays to count individual AuNP labels using a portable plasmonic imager. Cunningham and co-workers developed a method based on AuNPs coated in capture antibodies that—after binding to the protein target—form a sandwich immunocomplex with secondary antibodies immobilized on a plasmonic crystal biosensor.108 Optical coupling of the plasmon resonance of bound AuNP with the resonance of the plasmonic crystal quenches the resonance reflection of the sensor, so individual particles could be counted. They integrated the detection scheme into a microfluidic device for capture and digital counting of the AuNP labels, and were able to detect p24 in serum down to 40 fM,108 and to detect antibodies to SARS-CoV-2 in the blood of COVID-19 patients.109 Gao et al.110 used plasmonic imaging to detect single silver nanocube labels in a microfluidic integrated device, and used machine learning to analyze images to profile cytokine storm in COVID-19 patients.
As well as methods based on scatter of nanoparticles, other more complex optical techniques have been used to detect single nanoparticles in immunocomplexes. Nie and co-workers used single-molecule coincidence fluorescence detection to demonstrate a homogeneous immunoassay based on 40 nm fluorescent nanoparticle and quantum dot labels.111 Liu et al.112 also made use of two antibodies labeled with quantum dots, and detected the dimers formed by sandwich immunocomplexes using transmission electron microscopy. They extended this approach to enable homogeneous assays where coincidence detection of AuNPs modified with detection antibodies and quantum dots modified with capture antibodies were detected using dark-field and fluorescence microscopy, respectively.113 Gite et al.114 developed a digital immunoassay where one antibody was modified with 500 nm fluorescent particles and the other antibody was modified with a magnetic particle. After the formation of sandwich immunocomplexes in solution, the complexes were pulled down to a surface using a magnet and the individual fluorescent particles counted using a wide FOV imager. Using this method, they demonstrated an assay for Toxin B from C. diff. with an LOD of 45 pg mL−1.
Gorris and co-workers have pioneered the use of photon up conversion nanoparticles (UCNPs) as single molecule labels for digital assays at planar surfaces. UCNPs are lanthanide-doped nanocrystals that are excited by near-IR wavelengths and emit in the visible spectrum. As a result, these labels have lower optical backgrounds than fluorescent labels, improving the signal-to-noise ratio needed to measure single labels. These workers demonstrated a digitized immunoassay for PSA assay with an LOD of 42 fM based on imaging UCNP-labeled detection antibodies at the surface of a polystyrene microtiter plate.115 They subsequently improved the sensitivity of the assay to 0.8 fM by labeling a biotinylated detection antibody with a streptavidin-UCNP conjugate that was modified to reduce non-specific binding.116 One significant downside to the use of UCNPs is the high excitation energies needed for the multiphoton up conversion process. High energy light sources typically increase the size and cost of instrumentation, and limit the FOV of labels imaged.
In additional to conventional optical detection of nanoparticles, the kinetics of motion of nanoparticles has been used as the signal transduction method for digital assays.117,118 Tekin et al.119 patterned arrays of antibody-functionalized 1 μm magnetic beads on a surface. Larger (2.8 μm) magnetic beads coated in a second antibody were mixed with sample in a microfluidic device, and then flowed over the array of smaller beads. The larger beads associated with protein could overcome the drag forces and remained bound to the smaller beads; those beads without sufficient target molecules did not bind as strongly and were washed from the surface. Detection was performed by scatter images to determine the number of bound large beads. An LOD for TNF-α of 6 fM was reported, although the slope of the signal response was low and the CVs high. In a similar approach, Akama et al.120 first captured proteins on antibody-coated magnetic nanoparticles and delivered them to arrays of femtoliter wells functionalized with a second antibody. They used bright-field imaging of the nanoparticles to observe their Brownian motion. Based on the mean square displacement (MSD) analysis, they were able to identify beads bound via immunocomplex from those not bound. They developed an assay for PSA with an LOD of 5 fM, and subsequently improved the sensitivity of the method by normalizing the MSD to bead fluorescence121 and demonstrated a multiplex assay.122 Recently, Zeng et al.123 used magnetic and electrical forces to accelerate the transport of antibody-coated particles to the capture surface, and have provided a theoretical model for these measurements.124 They measured the interaction lifetimes of the particles with the surface using surface plasmon resonance imaging, and were able to distinguish specifically bound particles from those binding non-specifically.
4.3 Nanopore detection
The detection of DNA molecules passing through nanopores has received significant attention for sequencing. Nanopores have also been used to detect single protein molecules, although quantification of low concentrations of proteins by this method has been hampered by the globular nature of proteins making nanopore selectivity for specific proteins a challenge, and long process times for sufficient numbers of proteins to translocate through the nanopores. Gooding and co-workers elegantly addressed these challenges by developing a nanopore blockade sensor for detecting large numbers of individual proteins (Fig. 7C).125,126 In this approach, magnetic nanoparticles were modified with an antibody to PSA and used to efficiently capture the target protein from a sample. After washing, the nanoparticles were delivered via a flow cell to a solid-state nanopore array in a SiN membrane modified with a second antibody to PSA. The particles blocked the nanopores giving rise to a positive signal, and specificity to target-bound particles was achieved using a counter magnetic force: particles that did not form a sandwich complex with the antibody in the nanopore were ejected by magnetic force; particles bound via immunocomplex to the nanopore were not ejected. This assay had an LOD of 0.8 fM PSA. He et al.127 also used solid-state nanopores to develop a digital immunoassay for thyroid stimulating hormone (TSH). These authors did not directly detect the bound protein, but instead formed a sandwich on magnetic beads using an oligonucleotide-labeled detection antibody. The ssDNA was released using UV light and used to catalyze the formation of DNA nanostructure dumbbells from DNA probes. These solutions were then analyzed by translocation through solid-state nanopores with the current change associated with a probe assigned “0” and with a dumbbell assigned “1”.
4.4 Exploiting the kinetics of binding of single labels
Several groups have used the kinetic properties of measuring single labels to enhance the performance of immunoassays. Kurabayashi and co-workers have adapted DBA to use the high sensitivity of digital detection to enable faster measurements of higher abundance markers.128–130 In this approach—which they call pre-equilibrium digital ELISA (PEdELISA)—capture beads were pre-loaded into arrays of microwells rather than dispersed throughout the sample. A mixture of sample and detection antibodies, SA-HRP, and a chemifluorescent substrate to HRP were sequentially flowed over the arrays to form digitized immunocomplexes, the arrays were sealed with oil, and fluorescently imaged. The images generated were analyzed using machine learning methods130 to yield the average number of immunocomplexes per bead as for DBA. Rather than make use of single molecule detection to make the most sensitive assays, these authors used the high sensitivity to reach detectable signals from higher abundance markers more rapidly. They have used this approach to detect a 4-plex of cytokines in the serum of COVID-19 patients undergoing cytokine storm,128 and CAR-T patients experiencing cytokine release syndrome.129 Ismagilov and co-workers have also made use of flow of sample over pre-formed beads-in-microwell arrays arranged in microchannels to exploit Brownian trapping effects in these assays (Fig. 7D).131 The concentration dependence of Brownian trapping along the length of the bead array effectively converted the temporal variation of signal on the beads to a spatial distribution, allowing the authors to maintain high sensitivity of DBA with an increased dynamic range.
The difference in dissociation kinetics of specifically bound and non-specifically bound single labels has been exploited to lower the background of these assays in a method called single-molecule recognition through equilibrium Poisson sampling (SiMREPS).132,133 In SiMREPS, the binding of a fluorescently labeled detection antibody fragment to the surface is measured using TIRF microscopy. The kinetic profile of fluorescent signals from labels that specifically bind to a protein analyte specifically bound to a capture antibody differ from those non-specifically bound to the surface, allowing better discrimination of specific binding and lower assay backgrounds. As mentioned above the combination of single label sensitivity and reduction in backgrounds are the key features of the most sensitive immunoassays, and SiMREPS is designed to achieve both. The authors used the method to develop assays for several proteins with LODs at low femtomolar and subfemtomolar concentrations, and allowed them to quantify TNF-α in 2 μL of sample from patients undergoing CAR-T therapy.132 A similar approach has been reported by Zeng et al.123 who also used the differential kinetics of labels bound specifically and non-specifically at a surface to improve the specificity of single molecule protein detection. Nanoparticle label tracking has also been used to improve the discrimination of specifically bound target molecules as described above.119–121,123
4.5 Other approaches
Outside of these 4 specific categories, other methods have been developed to enable single molecule detection of proteins for quantitative assays. In one approach, the enhanced optical fields generated by nanostructures, such as microtoroid resonators134 and nanoneedles,135 can enable single molecule detection. For example, Liang et al.135 have reported a method for detecting p-tau captured on arrays of nanoneedles based on Poisson distribution analysis of enzyme labels bound to individual binding sites as described previously.49,136 In this approach, individual immunocomplexes were labeled with HRP and precipitation of an insoluble product of the enzyme-substrate reaction changed the refractive index of the associated nanoneedle. Dark field imaging of the nanoneedle array was used to determine the fraction of “on” needles and to quantify concentration. Wang and co-workers have used the microbubbles generated in the presence of hydrogen peroxide by single platinum nanoparticles (PtNP) labels associated with immunocomplexes on beads.137,138 In principle, this assay could have been quantified using Poisson statistics as for DBA, but the authors chose to use imaging on a smart phone to count the number of bubbles and their total area. This approach enabled them to demonstrate assays for PSA and SARS-CoV-2 antigen with LODs of 2.1 fM and 10.6 fM, respectively.
Some groups have taken a similar approach as Simoa based on trapping enzymes in microwell arrays, but instead of forming the immunocomplexes on beads they are formed on planar capture surfaces. For example, Wang et al.139 and Piraino et al.140 have used pneumatic sealing of PDMS microwell arrays against glass surfaces in integrated microfluidic devices to digitize immunocomplexes formed on planar surfaces. Atallah et al.141 reported the detection of single immunocomplexes using diamond nitrogen vacancy centers, where two different magnetic bead labels on the antibodies that respond differently to the magnetic field can, therefore, make use of coincidence optical detection. They used this approach to develop an assay for IL-6 with an LOD of 2 fM to measure the protein in the serum of COVID-19 patients. Two recent publications have used mass spectrometry to count single labels in bead-based immunoassays, one approach based on TSA142 and one using gold and silver nanoparticles.143
4.6 Characteristics of robust, sensitive digital protein detection methods
Many of these innovative digital detection methods overcome the 3 technical challenges highlighted above, i.e., they enable detection of large numbers of spatially isolated single labels of proteins. The practical application of these innovations as robust methods for detecting very low concentrations of proteins depends, however, on them also achieving several other important characteristics:
1) Assay response—how signal varies with concentration of protein—must have a high signal-to-background ratio to enable precise sensitive detection. It is not sufficient to simply detect single labeled proteins. This requirement means that the assay must have a high slope (log increases in concentration must result in log increases in signal) and low backgrounds. High slope is achieved by highly efficient capture and labeling of proteins driven by high concentrations of high affinity capture antibodies. Low backgrounds are achieved by low non-specific binding between the labeling reagents and capture molecules and—in the case of heterogenous assays—the binding surfaces being effectively washed. Methods that have low capture efficiencies, low slopes, and high backgrounds will not result in sensitive assays even if they are able to count single labels. The use of beads in digital assays generally provides high slope (resulting from efficient capture from high concentrations of antibodies distributed throughout the sample) and low backgrounds (from washing). Methods based on planar substrates can achieve high sensitivity, but efficiencies are limited by transport of proteins to the surfaces and there are greater challenges in washing to minimize backgrounds. Homogenous assays can also achieve high slopes and low backgrounds, but can be prone to interference from non-specific binding of components in the sample as washing is not used.
2) The reagents, consumables, and instrumentation used must be stable, low cost, and low complexity. Methods based on homogeneous amplification of nucleic acids are particularly attractive from this standpoint as they are based on well-established reagents and instrumentation from molecular diagnostics, and have a simple “mix-and-read” assay workflow. Nanoparticle detection based on scattering is attractive in terms of simplifying the detection technology using low-cost imaging.
3) The assay processing steps before single label detection must be simple and precise. This aspect of developing digital protein assays is often overlooked, as researchers focus on the challenges of detecting singulated labels in the first place. If the assay steps are not done with precision or high efficiency then the benefits from counting single labels will be lost in high noise and low assay slope. Methods that use existing approaches to the assay processing steps or have developed new approaches are attractive in this respect.
4) Labels must be free to diffuse and bind to proteins evenly and reproducibly. Biological labels, such as enzymes, diffuse freely and consistently label proteins. For methods based on larger, non-biological labels, such as engineered particles, more care needs to be taken to ensure that the labels are well dispersed and able to bind homogeneously to proteins.
5. Impact of digital protein detection
The advent of digital protein detection and the consequent increases in the sensitivity of immunoassays has resulted in unique measurements in biomedicine that provide a path to significant benefits to society. While the field is still nascent, the “unmitigated good” of more sensitivity to proteins will clearly continue to result from new diagnostic measurements that were not possible previously. Digital protein detection has, for examples, enabled:5 the detection of neurodegeneration years before symptoms of Alzheimer's Disease arise;144 detection of damage to cardiac tissue in subclinical populations;145 the more specific diagnosis of deadly infectious diseases than possible with nucleic acid detection by measuring proteins from active viruses and bacteria;96,146,147 and, the ability to predict years before current methods those cancer patients in remission whose cancer will recur.148 Given the burgeoning literature of using digital protein detection—DBA in particular—for clinical and diagnostic measurements,5 it is not possible to provide comprehensive coverage of these new clinical applications within this technology review. Instead, we will highlight three key aspects of applying digital protein detection that have combined to result in high impact applications in biomedicine. These perspectives may provide guidance for researchers developing new analytical methods based on detection of single protein molecules.
5.1 Development of customized digital proteins assays (“homebrew”)
Once a sensitive digital detection technology has been developed, a common roadblock to its adoption is the challenge of developing a wide range of assays to enable researchers to explore a breadth of clinical applications. The success of PCR can be attributed partly to the relative ease with which biological researchers can develop their own assays, as well as the exquisite sensitivity of the method. Similarly, a critical aspect to the adoption of digital protein methods has been the ability of clinical researchers to develop their own highly sensitive assays based on their own (often proprietary) binding reagents that are tailored to their specific clinical application and sample type. We call this type of custom assay development “homebrew”, and it unlocks the creativity of clinicians, biologists, and chemists to develop their own unique “apps” based on digital protein detection. DBA provides a convenient way for researchers to develop homebrew assays as the protocols to conjugate capture antibodies to beads and to biotinylate detection antibodies are straightforward and can be performed in bulk outside of the devices.149 Once proof of principle of DBA has been performed using homebrew methods, it often results in the development of more analytically validated kits that can be used for clinical validation and use. Digital detection methods that are “closed” or provide significant technical challenges to custom assay development will make it difficult to explore broad clinical applications.
Researchers at Genentech have described the methods and challenges associated with DBA homebrew, and used it to develop an assay for IL-13, a biomarker and therapeutic target in asthma and COPD.149 They compared the performance of their homebrew DBA for IL-13 with a corresponding commercial kit and a third protein detection platform. One particularly noteworthy application of DBA that was catalyzed by homebrew was the detection of neurofilament light (NfL) in blood by Kuhle and co-workers,150 whose impact will be described in more detail below. A common use of homebrew is to enable assay development on sample types of clinical interest that pose significant analytical challenges where the flexibility of customized assay development can be critical to enable quantitative measurements. For example, Göpfert and co-workers developed a DBA assay for measuring VEGF-A in aqueous humor from the eyes of human subjects.151 They used the greater sensitivity of this digital assay to measure reductions in the concentration of VEGF in the ocular fluid caused by administration of a therapeutic antibody that targets this growth factor; conventional bead immunoassays were not able to detect these reductions. The sensitivity of homebrew DBA has also been used to measure proteins in other challenging samples where protein concentrations and sample volumes may be low, such as breath condensate,152 sweat,153 saliva,154 and stool.96 Another application of homebrew has been to detect the proteins in single cells, where the number of proteins expressed when single cells are lysed in microliter volumes are within the sensitivity of digital protein assays.155,156,147
5.2 Applications in clinical translation
Digital protein detection has had its greatest initial impact in the translation of known biomarkers into clinical use, as opposed to the discovery of potential new biomarkers early in research. Clinical translation of biomarkers requires measurements with high precision and accuracy (as well as high sensitivity) of relatively few proteins in many samples, corresponding to the sweet spot of digital detection. A requirement for these measurements, therefore, is the availability of high-quality antibody pairs. In contrast, de novo discovery of biomarkers requires qualitative or semi-quantitative measurements of large number of proteins in small numbers of samples. Methods based on mass spectrometry (that does not require antibodies)157 or immunoassay platforms based on large libraries of binding reagents (for example, aptamers158 or antibodies labeled with nucleic acids159) are better suited for biomarker discovery measurements.
A good example of the application of DBA in clinical translation is in the measurement of neurological biomarkers in blood, described in detail below. Another widespread application has been in mid- to late-stage clinical trials of therapeutics, especially antibodies therapeutics where the target molecule is usually a protein. These measurements need high precision and accuracy, especially to quantitatively measure the effect of the candidate therapeutic on the concentration of the target protein in a physiological fluid. The natural progression of these applications in clinical translation and pharmaceutical clinical trials is for these markers to become diagnostic tests, as has started to happen in Alzheimer's disease and viral and serology tests for COVID-19.
5.3 Measurement of neurological markers in blood
The greatest impact of digital protein detection so far has been in neurology. Clinical chemists had discovered promising biomarkers in cerebral spinal fluid (CSF)—such as tau, amyloid peptides, and neurofilaments—that made the diagnosis of diseases such as AD and multiple sclerosis (MS) possible.160 The field of neurological diagnosis was, however, limited by a lack of sufficiently sensitive methods to measure these critical biological molecules in readily accessible samples from humans. In short, there were no blood tests for the brain. As a result, diagnoses of diseases such as AD were based on magnetic resonance imaging of tracers in brains (expensive and difficult to scale), the measurement of the biomarkers in CSF (difficult sample collection), or, unfortunately, post-mortem examination of patients' brains. The large increase in sensitivity of DBA offered clinical researchers in neurology the ability to measure diagnostic biomarkers in blood. Henrik Zetterberg and Kaj Blennow—neurological clinical researchers in Sweden—lit the touch paper when they used Simoa to measure tau161 and Aβ-42 (ref. 162) in the blood of patients who had undergone neurological damage after hypoxia. With collaborators, they subsequently used the homebrew approach to develop blood-based digital immunoassays for many neurological protein biomarkers.163 Subsequent to these initial studies, the neurology research community adopted DBA broadly for measuring biomarkers such as phosphorylated tau, amyloid peptides, neurofilament light (NfL), and α-synuclein in many diseases and neurological conditions, such as AD, MS, Parkinson's disease, and traumatic brain injury. The value of these measurements was also quickly picked up by pharmaceutical companies who had candidate therapeutics in development that targeted the same molecules or pathways. As a result, the sensitivity of digital detection was able to create synergies between therapeutics and diagnostics. In its most powerful application, the ability to use digital assays to identify people early in neurodegeneration before symptoms arise144 allows therapeutics to be administered early enough so that they can impact disease progression.164
This review cannot efficiently summarize the impact of digital protein detection in neurology, but several excellent reviews are available. For example, Thebault and co-workers describe the biology of NfL and how digital immunoassays have allowed it to become a general biomarker of neurodegeneration akin to the use of troponin as a marker of cardiac damage.165 They highlight the importance of DBA to MS diagnosis and the development of therapeutics. Zetterberg and Blennow describe how blood-based biomarkers enabled by digital protein detection is “democratizing” the diagnosis of AD by offering low-cost and non-invasive testing for the first time.163 These authors have also reviewed the history of protein biomarkers for AD, and how greater sensitivity enabled measurements in blood.166 Mielke et al.167 also review how the measurement of tau, amyloid peptides, and NfL have impacted the understanding of AD. The measurement of phosphorylated forms of tau using digital protein detection has emerged as very specific diagnostics of AD. Ding et al.168 have provided a meta-analysis of clinical studies that measured p-tau-181 in AD patients using DBA, and researchers at Eli Lilly and Janssen have highlighted the potential of digital protein detection of p-tau-217 to help diagnose AD and guide administration of new therapies for AD.169 In summary, the ability of DBA to enable these unique molecular measurements to diagnose devastating diseases was catalyzed by assay sensitivity allowing translation of discovery biomarkers to use as clinical measurements, and the availability of a homebrew path to unlock the creativity of clinical researchers.
6. The current state and future of digital protein detection
In its first 20 years or so, digital detection of proteins has shown promise as a path to highly sensitive immunoassays. These assays have led to several unique clinical research and diagnostic measurements, especially the measurement of neurological markers in blood. It remains to be seen whether digital detection will have the same impact on protein diagnostics that PCR and NGS have had in diagnoses based on detection of nucleic acids, but the outlook is promising. Given the lack of methods for the direct molecular amplification of proteins, the counting of single protein molecules seems to be the front-runner in the race. Approaches to next-generation protein sequencing170 and CRISPR-based detection of proteins171 may provide alternative approaches to highly sensitive protein detection, although these methods may also benefit from single molecule detection.
In terms of analytical performance, the single molecule counting approach of DBA has achieved attomolar sensitivities,54,63 with limits of detection down to about 10 protein molecules in a 200 μL sample, comparable to PCR for nucleic acid detection.54 Theoretical models of DBA show that this sensitivity can be achieved assuming favorable binding affinities for the capture and detection antibodies.51,54 Antibody engineering approaches will, therefore, presumably be needed to achieve attomolar sensitivity across all proteins, in the same way that scalable synthesis of nucleic acids enabled the widespread adoption of PCR and NGS.
The main question for the future of digital protein detection, therefore, seems to be whether these technologies can be delivered in scalable formats that enable mass adoption and maximize the impact on human health. Currently, the commercial embodiments of digital protein detection require large, complex, and expensive equipment, and the method has been confined to specialized clinical and research settings. For wider adoption, digital protein detection technologies need to be developed into smaller, easier to use, and low-cost equipment and consumables; we have described the first few efforts in this direction.59,81 The innovations in miniaturization and microfluidics that might emerge from the “Lab-On-A-Chip” community172 would appear, therefore, to be a critical to the ultimate impact of digital protein detection. These innovations will need to encompass sample and reagent handling, optics, consumable manufacturing, and system integration if digital protein detection is to have the same impact on health that technologies such as lateral flow and glucose sensing have had in home testing and as wearables, respectively. Judging by the rapid pace of progress in digital protein detection in the last 10 years, and the creativity of the LOC community, this dream may be within reach.
Conflicts of interest
The author is an employee of Quanterix Corporation, and owns stock and stock options in Quanterix that manufactures and sells instrumentation and assay kits based on digital ELISA.
Acknowledgements
We thank Anne Tsimboukis (E-mail: anne@tsimboukisdesign.com) for rendering Fig. 1.
References
-
S. Pinker, Rationality: what it is, why it seems scarce, why it matters, Viking, New York, 2021, 1st edn, pp. 215–216 Search PubMed.
- D. Khodakov, C. Wang and D. Y. Zhang, Diagnostics based on nucleic acid sequence variant profiling: PCR, hybridization, and NGS approaches, Adv. Drug Delivery Rev., 2016, 105(Pt A), 3–19, DOI:10.1016/j.addr.2016.04.005 , Epub 2016 Apr 16. PMID: 27089811.
- E. Engvall and P. Perlmann, Enzyme-linked immunosorbent assay (ELISA). Quantitative assay of immunoglobulin G, Immunochemistry, 1971, 8(9), 871–874, DOI:10.1016/0019-2791(71)90454-x , PMID: 5135623.
- S. H. Payne, The utility of protein and mRNA correlation, Trends Biochem. Sci., 2015, 40(1), 1–3, DOI:10.1016/j.tibs.2014.10.010 , Epub 2014 Nov 23. PMID: 25467744; PMCID: PMC4776753.
- D. C. Duffy, Short Keynote Paper: Single Molecule Detection of Protein Biomarkers to Define the Continuum From Health to Disease, IEEE J. Biomed. Health Inform., 2020, 24(7), 1864–1868, DOI:10.1109/JBHI.2020.2971553 , Epub 2020 Feb 4. PMID: 32031955.
-
The Immunoassay Handbook, ed. D. Wild, Elsevier, Oxford, UK, 4th edn, 2013 Search PubMed.
- D. R. Walt, Optical methods for single molecule detection and analysis, Anal. Chem., 2013, 85(3), 1258–1263, DOI:10.1021/ac3027178 , Epub 2012 Dec 19. PMID: 23215010; PMCID: PMC3565068.
- D. M. Rissin, C. W. Kan, T. G. Campbell, S. C. Howes, D. R. Fournier, L. Song, T. Piech, P. P. Patel, L. Chang, A. J. Rivnak, E. P. Ferrell, J. D. Randall, G. K. Provuncher, D. R. Walt and D. C. Duffy, Single-molecule enzyme-linked immunosorbent assay detects serum proteins at subfemtomolar concentrations, Nat. Biotechnol., 2010, 28(6), 595–599, DOI:10.1038/nbt.1641 , Epub 2010 May 23. PMID: 20495550; PMCID: PMC2919230.
- Q. Huang, N. Li, H. Zhang, C. Che, F. Sun, Y. Xiong, T. D. Canady and B. T. Cunningham, Critical Review: digital resolution biomolecular sensing for diagnostics and life science research, Lab Chip, 2020, 20(16), 2816–2840, 10.1039/d0lc00506a , Epub 2020 Jul 23. PMID: 32700698; PMCID: PMC7485136.
- H. Liu and Y. Lei, A critical review: Recent advances in "digital" biomolecule detection with single copy sensitivity, Biosens. Bioelectron., 2021, 177, 112901, DOI:10.1016/j.bios.2020.112901 , Epub 2021 Jan 4. PMID: 33472132; PMCID: PMC7836387.
- L. Cohen and D. R. Walt, Highly Sensitive and Multiplexed Protein Measurements, Chem. Rev., 2019, 119(1), 293–321, DOI:10.1021/acs.chemrev.8b00257 , Epub 2018 Aug 28. PMID: 30152694.
- Z. Farka, M. J. Mickert, M. Pastucha, Z. Mikušová, P. Skládal and H. H. Gorris, Advances in Optical Single-Molecule Detection: En Route to Supersensitive Bioaffinity Assays, Angew. Chem., Int. Ed., 2020, 59(27), 10746–10773, DOI:10.1002/anie.201913924 , Epub 2020 Apr 15. PMID: 31869502; PMCID: PMC7318240.
- Y. Zhang and H. Noji, Digital Bioassays: Theory, Applications, and Perspectives, Anal. Chem., 2017, 89(1), 92–101, DOI:10.1021/acs.analchem.6b04290 , Epub 2016 Dec 1.
- W. Fan, D. Liu, W. Ren and C. Liu, Trends of Bead Counting-Based Technologies Toward the Detection of Disease-Related Biomarkers, Front. Chem., 2020, 8, 600317, DOI:10.3389/fchem.2020.600317 , PMID: 33409266; PMCID: PMC7779676.
- A. S. Basu, Digital Assays Part II: Digital Protein and Cell Assays, SLAS Technol., 2017, 22(4), 387–405, DOI:10.1177/2472630317705681 , Epub 2017 May 22. PMID: 28548029.
- E. Macchia, K. Manoli, C. Di Franco, G. Scamarcio and L. Torsi, New trends in single-molecule bioanalytical detection, Anal. Bioanal. Chem., 2020, 412(21), 5005–5014, DOI:10.1007/s00216-020-02540-9 , Epub 2020 Mar 17. PMID: 32185439; PMCID: PMC7338812.
- T. Sano, C. L. Smith and C. R. Cantor, Immuno-PCR: very sensitive antigen detection by means of specific antibody-DNA conjugates, Science, 1992, 258(5079), 120–122, DOI:10.1126/science.1439758 , PMID: 1439758.
- C. M. Niemeyer, M. Adler and R. Wacker, Immuno-PCR: high sensitivity detection of proteins by nucleic acid amplification, Trends Biotechnol., 2005, 23(4), 208–216, DOI:10.1016/j.tibtech.2005.02.006 , PMID: 15780713.
- S. Fredriksson, M. Gullberg, J. Jarvius, C. Olsson, K. Pietras, S. M. Gústafsdóttir, A. Ostman and U. Landegren, Protein detection using proximity-dependent DNA ligation assays, Nat. Biotechnol., 2002, 20(5), 473–477, DOI:10.1038/nbt0502-473 , PMID: 11981560.
- M. Lundberg, A. Eriksson, B. Tran, E. Assarsson and S. Fredriksson, Homogeneous antibody-based proximity extension assays provide sensitive and specific detection of low-abundant proteins in human blood, Nucleic Acids Res., 2011, 39(15), e102, DOI:10.1093/nar/gkr424 , Epub 2011 Jun 6. PMID: 21646338; PMCID: PMC3159481.
- B. Schweitzer, S. Wiltshire, J. Lambert, S. O'Malley, K. Kukanskis, Z. Zhu, S. F. Kingsmore, P. M. Lizardi and D. C. Ward, Immunoassays with rolling circle DNA amplification: a versatile platform for ultrasensitive antigen detection, Proc. Natl. Acad. Sci. U. S. A., 2000, 97(18), 10113–10119, DOI:10.1073/pnas.170237197 , PMID: 10954739; PMCID: PMC27732.
- J. M. Nam, C. S. Thaxton and C. A. Mirkin, Nanoparticle-based bio-bar codes for the ultrasensitive detection of proteins, Science, 2003, 301(5641), 1884–1886, DOI:10.1126/science.1088755 , PMID: 14512622.
- M. Gullberg, S. M. Gústafsdóttir, E. Schallmeiner, J. Jarvius, M. Bjarnegård, C. Betsholtz, U. Landegren and S. Fredriksson, Cytokine detection by antibody-based proximity ligation, Proc. Natl. Acad. Sci. U. S. A., 2004, 101(22), 8420–8424, DOI:10.1073/pnas.0400552101 , Epub 2004 May 21. PMID: 15155907; PMCID: PMC420409.
- R. Ke, R. Y. Nong, S. Fredriksson, U. Landegren and M. Nilsson, Improving precision of proximity ligation assay by amplified single molecule detection, PLoS One, 2013, 8(7), e69813, DOI:10.1371/journal.pone.0069813 , PMID: 23874999; PMCID: PMC3713053.
- B. Vogelstein and K. W. Kinzler, Digital PCR, Proc. Natl. Acad. Sci. U. S. A., 1999, 96(16), 9236–9241, DOI:10.1073/pnas.96.16.9236 , PMID: 10430926; PMCID: PMC17763.
- O. Ericsson, J. Jarvius, E. Schallmeiner, M. Howell, R. Y. Nong, H. Reuter, M. Hahn, J. Stenberg, M. Nilsson and U. Landegren, A dual-tag microarray platform for high-performance nucleic acid and protein analyses, Nucleic Acids Res., 2008, 36(8), e45, DOI:10.1093/nar/gkn106 , Epub 2008 Mar 16. PMID: 18346972; PMCID: PMC2377440.
- L. C. Brousseau 3rd., Label-free "digital detection" of single-molecule DNA hybridization with a single electron transistor, J. Am. Chem. Soc., 2006, 128(35), 11346–11347, DOI:10.1021/ja063022f , PMID: 16939245; PMCID: PMC2533726.
- S. Husale, H. H. Persson and O. Sahin, DNA nanomechanics allows direct digital detection of complementary DNA and microRNA targets, Nature, 2009, 462(7276), 1075–1078, DOI:10.1038/nature08626 , Epub 2009 Dec 13. PMID: 20010806; PMCID: PMC2966338.
- Y. Xiong, Q. Huang, T. D. Canady, P. Barya, S. Liu, O. H. Arogundade, C. M. Race, C. Che, X. Wang, L. Zhou, X. Wang, M. Kohli, A. M. Smith and B. T. Cunningham, Photonic crystal enhanced fluorescence emission and blinking suppression for single quantum dot digital resolution biosensing, Nat. Commun., 2022, 13(1), 4647, DOI:10.1038/s41467-022-32387-w , PMID: 35941132; PMCID: PMC9360002.
- F. Ekiz Kanik, I. Celebi, D. Sevenler, K. Tanriverdi, N. Lortlar Ünlü, J. E. Freedman and M. S. Ünlü, Attomolar sensitivity microRNA detection using real-time digital microarrays, Sci. Rep., 2022, 12(1), 16220, DOI:10.1038/s41598-022-19912-z , PMID: 36171215; PMCID: PMC9519543.
- M. Cretich, G. G. Daaboul, L. Sola, M. S. Ünlü and M. Chiari, Digital detection of biomarkers assisted by nanoparticles: application to diagnostics, Trends Biotechnol., 2015, 33(6), 343–351, DOI:10.1016/j.tibtech.2015.03.002 , Epub 2015 Apr 17. PMID: 25896126.
- N. Akkilic, S. Geschwindner and F. Höök, Single-molecule biosensors: Recent advances and applications, Biosens. Bioelectron., 2020, 151, 111944, DOI:10.1016/j.bios.2019.111944 , Epub 2019 Dec 9. PMID: 31999573.
- H. Härmä, T. Soukka and T. Lövgren, Europium nanoparticles and time-resolved fluorescence for ultrasensitive detection of prostate-specific antigen, Clin. Chem., 2001, 47(3), 561–568 CrossRef , PMID: 11238312.
- T. Soukka, J. Paukkunen, H. Härmä, S. Lönnberg, H. Lindroos and T. Lövgren, Supersensitive time-resolved immunofluorometric assay of free prostate-specific antigen with nanoparticle label technology, Clin. Chem., 2001, 47(7), 1269–1278 CrossRef CAS , PMID: 11427459.
- H. Härmä, P. Tarkkinen, T. Soukka and T. Lövgren, Miniature single-particle immunoassay for prostate-specific antigen in serum using recombinant Fab fragments, Clin. Chem., 2000, 46(11), 1755–1761 CrossRef , PMID: 11067810.
- H. Härmä, T. Soukka, S. Lönnberg, J. Paukkunen, P. Tarkkinen and T. Lövgren, Zeptomole detection sensitivity of prostate-specific antigen in a rapid microtitre plate assay using time-resolved fluorescence, Luminescence, 2000, 15(6), 351–355, DOI:10.1002/1522-7243(200011/12)15:6<351::AID-BIO624>3.0.CO;2-3 , PMID: 11114110.
- F. Löscher, S. Böhme, J. Martin and S. Seeger, Counting of single protein molecules at interfaces and application of this technique in early-stage diagnosis, Anal. Chem., 1998, 70(15), 3202–3205, DOI:10.1021/ac971378r , PMID: 11013721.
- J. Li, W. Xie, N. Fang and E. S. Yeung, Single-molecule immunosorbent assay as a tool for human immunodeficiency virus-1 antigen detection, Anal. Bioanal. Chem., 2009, 394(2), 489–497, DOI:10.1007/s00216-009-2712-1 , Epub 2009 Mar 8. PMID: 19267241.
- L. A. Tessler, J. G. Reifenberger and R. D. Mitra, Protein quantification in complex mixtures by solid phase single-molecule counting, Anal. Chem., 2009, 81(17), 7141–7148, DOI:10.1021/ac901068x , PMID: 19601620.
- R. Schmidt, J. Jacak, C. Schirwitz, V. Stadler, G. Michel, N. Marmé, G. J. Schütz, J. D. Hoheisel and J. P. Knemeyer, Single-molecule detection on a protein-array assay platform for the exposure of a tuberculosis antigen, J. Proteome Res., 2011, 10(3), 1316–1322, DOI:10.1021/pr101070j , Epub 2011 Feb 16. PMID: 21247063.
- E. Burgin, A. Salehi-Reyhani, M. Barclay, A. Brown, J. Kaplinsky, M. Novakova, M. A. Neil, O. Ces, K. R. Willison and D. R. Klug, Absolute quantification of protein copy number using a single-molecule-sensitive microarray, Analyst, 2014, 139(13), 3235–3244, 10.1039/c4an00091a , PMID: 24676423.
- A. H. Wu, N. Fukushima, R. Puskas, J. Todd and P. Goix, Development and preliminary clinical validation of a high sensitivity assay for cardiac troponin using a capillary flow(single molecule) fluorescence detector, Clin. Chem., 2006, 52(11), 2157–2159, DOI:10.1373/clinchem.2006.073163 , PMID: 18061987.
- J. Todd, B. Freese, A. Lu, D. Held, J. Morey, R. Livingston and P. Goix, Ultrasensitive flow-based immunoassays using single-molecule counting, Clin. Chem., 2007, 53(11), 1990–1995, DOI:10.1373/clinchem.2007.091181 , Epub 2007 Sep 21. PMID: 17890441.
- A. H. Wu, Q. A. Lu, J. Todd, J. Moecks and F. Wians, Short- and long-term biological variation in cardiac troponin I measured with a high-sensitivity assay: implications for clinical practice, Clin. Chem., 2009, 55(1), 52–58, DOI:10.1373/clinchem.2008.107391 , Epub 2008 Nov 6. PMID: 18988755.
- M. Gilbert, R. Livingston, J. Felberg and J. J. Bishop, Multiplex single molecule counting technology used to generate interleukin 4, interleukin 6, and interleukin 10 reference limits, Anal. Biochem., 2016, 503, 11–20, DOI:10.1016/j.ab.2016.03.008 , Epub 2016 Mar 24. PMID: 27019152.
- C. I. Tobos, S. Kim, D. M. Rissin, J. M. Johnson, S. Douglas, S. Yan, S. Nie, B. Rice, K. J. Sung, H. D. Sikes and D. C. Duffy, Sensitivity and binding kinetics of an ultra-sensitive chemiluminescent enzyme-linked immunosorbent assay at arrays of antibodies, J. Immunol. Methods, 2019, 474, 112643, DOI:10.1016/j.jim.2019.112643 , Epub 2019 Aug 8. PMID: 31401067.
- D. M. Rissin, D. R. Fournier, T. Piech, C. W. Kan, T. G. Campbell, L. Song, L. Chang, A. J. Rivnak, P. P. Patel, G. K. Provuncher, E. P. Ferrell, S. C. Howes, B. A. Pink, K. A. Minnehan, D. H. Wilson and D. C. Duffy, Simultaneous detection of single molecules and singulated ensembles of molecules enables immunoassays with broad dynamic range, Anal. Chem., 2011, 83(6), 2279–2285, DOI:10.1021/ac103161b , Epub 2011 Feb 23. PMID: 21344864; PMCID: PMC3056883.
- Y. Rondelez, G. Tresset, K. V. Tabata, H. Arata, H. Fujita, S. Takeuchi and H. Noji, Microfabricated arrays of femtoliter chambers allow single molecule enzymology, Nat. Biotechnol., 2005, 23(3), 361–365, DOI:10.1038/nbt1072 , Epub 2005 Feb 20. PMID: 15723045.
- D. M. Rissin and D. R. Walt, Digital concentration readout of single enzyme molecules using femtoliter arrays and Poisson statistics, Nano Lett., 2006, 6(3), 520–523, DOI:10.1021/nl060227d , PMID: 16522055.
- D. H. Wilson, D. M. Rissin, C. W. Kan, D. R. Fournier, T. Piech, T. G. Campbell, R. E. Meyer, M. W. Fishburn, C. Cabrera, P. P. Patel, E. Frew, Y. Chen, L. Chang, E. P. Ferrell, V. von Einem, W. McGuigan, M. Reinhardt, H. Sayer, C. Vielsack and D. C. Duffy, The Simoa HD-1 Analyzer: A Novel Fully Automated Digital Immunoassay Analyzer with Single-Molecule Sensitivity and Multiplexing, J. Lab. Autom., 2016, 21(4), 533–547, DOI:10.1177/2211068215589580 , Epub 2015 Jun 15. PMID: 26077162.
- L. Chang, D. M. Rissin, D. R. Fournier, T. Piech, P. P. Patel, D. H. Wilson and D. C. Duffy, Single molecule enzyme-linked immunosorbent assays: theoretical considerations, J. Immunol. Methods, 2012, 378(1–2), 102–115, DOI:10.1016/j.jim.2012.02.011 , Epub 2012 Feb 20. PMID: 22370429; PMCID: PMC3327511.
- D. M. Rissin, C. W. Kan, L. Song, A. J. Rivnak, M. W. Fishburn, Q. Shao, T. Piech, E. P. Ferrell, R. E. Meyer, T. G. Campbell, D. R. Fournier and D. C. Duffy, Multiplexed single molecule immunoassays, Lab Chip, 2013, 13(15), 2902–2911, 10.1039/c3lc50416f , PMID: 23719780.
- A. J. Rivnak, D. M. Rissin, C. W. Kan, L. Song, M. W. Fishburn, T. Piech, T. G. Campbell, D. R. DuPont, M. Gardel, S. Sullivan, B. A. Pink, C. G. Cabrera, D. R. Fournier and D. C. Duffy, A fully-automated, six-plex single molecule immunoassay for measuring cytokines in blood, J. Immunol. Methods, 2015, 424, 20–27, DOI:10.1016/j.jim.2015.04.017 , Epub
2015 May 7. PMID: 25960176.
- C. W. Kan, C. I. Tobos, D. M. Rissin, A. D. Wiener, R. E. Meyer, D. M. Svancara, A. Comperchio, C. Warwick, R. Millington, N. Collier and D. C. Duffy, Digital enzyme-linked immunosorbent assays with sub-attomolar detection limits based on low numbers of capture beads combined with high efficiency bead analysis, Lab Chip, 2020, 20(12), 2122–2135, 10.1039/d0lc00267d , Epub 2020 May 11. PMID: 32391827.
- C. W. Kan, A. J. Rivnak, T. G. Campbell, T. Piech, D. M. Rissin, M. Mösl, A. Peterça, H. P. Niederberger, K. A. Minnehan, P. P. Patel, E. P. Ferrell, R. E. Meyer, L. Chang, D. H. Wilson, D. R. Fournier and D. C. Duffy, Isolation and detection of single molecules on paramagnetic beads using sequential fluid flows in microfabricated polymer array assemblies, Lab Chip, 2012, 12(5), 977–985, 10.1039/c2lc20744c , Epub 2011 Dec 16. PMID: 22179487.
- S. H. Kim, S. Iwai, S. Araki, S. Sakakihara, R. Iino and H. Noji, Large-scale femtoliter droplet array for digital counting of single biomolecules, Lab Chip, 2012, 12(23), 4986–4991, 10.1039/c2lc40632b , PMID: 22961607.
- K. Leirs, P. Tewari Kumar, D. Decrop, E. Pérez-Ruiz, P. Leblebici, B. Van Kelst, G. Compernolle, H. Meeuws, L. Van Wesenbeeck, O. Lagatie, L. Stuyver, A. Gils, J. Lammertyn and D. Spasic, Bioassay Development for Ultrasensitive Detection of Influenza A Nucleoprotein Using Digital ELISA, Anal. Chem., 2016, 88(17), 8450–8458, DOI:10.1021/acs.analchem.6b00502 , Epub 2016 Aug 15. PMID: 27487722.
- C. Ge, J. Feng, J. Zhang, K. Hu, D. Wang, L. Zha, X. Hu and R. Li, Aptamer/antibody sandwich method for digital detection of SARS-CoV2 nucleocapsid protein, Talanta, 2022, 236, 122847, DOI:10.1016/j.talanta.2021.122847 , ISSN 0039-9140.
- V. Yelleswarapu, J. R. Buser, M. Haber, J. Baron, E. Inapuri and D. Issadore, Mobile platform for rapid sub-picogram-per-milliliter, multiplexed, digital droplet detection of proteins, Proc. Natl. Acad. Sci. U. S. A., 2019, 116(10), 4489–4495, DOI:10.1073/pnas.1814110116 , Epub 2019 Feb 14. PMID: 30765530; PMCID: PMC6410864.
- K. Akama, K. Shirai and S. Suzuki, Droplet-Free Digital Enzyme-Linked Immunosorbent Assay Based on a Tyramide Signal Amplification System, Anal. Chem., 2016, 88(14), 7123–7129, DOI:10.1021/acs.analchem.6b01148 , Epub 2016 Jun 30. PMID: 27322525.
- A. M. Maley, P. M. Garden and D. R. Walt, Simplified Digital Enzyme-Linked Immunosorbent Assay Using Tyramide Signal Amplification and Fibrin Hydrogels, ACS Sens., 2020, 5(10), 3037–3042, DOI:10.1021/acssensors.0c01661 , Epub 2020 Oct 1. PMID: 32988208.
- C. Wu, P. M. Garden and D. R. Walt, Ultrasensitive Detection of Attomolar Protein Concentrations by Dropcast Single Molecule Assays, J. Am. Chem. Soc., 2020, 142(28), 12314–12323, DOI:10.1021/jacs.0c04331 , Epub 2020 Jun 30. PMID: 32602703; PMCID: PMC7368998.
- C. Wu, T. J. Dougan and D. R. Walt, High-Throughput, High-Multiplex Digital Protein Detection with Attomolar Sensitivity, ACS Nano, 2022, 16(1), 1025–1035, DOI:10.1021/acsnano.1c08675 , Epub 2022 Jan 14. PMID: 35029381, PMCID: PMC9499451.
- W. Zhou, Development of immunomagnetic droplet-based digital immuno-PCR for the quantification of prostate specific antigen, Anal. Methods, 2018, 10, 3690–3695 RSC.
- W. H. Henley, N. A. Siegfried and J. M. Ramsey, Spatially isolated reactions in a complex array: using magnetic beads to purify and quantify nucleic acids with digital and quantitative real-time PCR in thousands of parallel microwells, Lab Chip, 2020, 20(10), 1771–1779, 10.1039/d0lc00069h , PMID: 32347869.
- L. Zhang, W. Fan, D. Jia, Q. Feng, W. Ren and C. Liu, Microchamber-Free Digital Flow Cytometric Analysis of T4 Polynucleotide Kinase Phosphatase Based on Single-Enzyme-to-Single-Bead Space-Confined Reaction, Anal. Chem., 2021, 93(44), 14828–14836, DOI:10.1021/acs.analchem.1c03724 , Epub 2021 Oct 29. PMID: 34713697.
- L. Zhu, D. Chen, X. Lu, Y. Qi, P. He, C. Liu and Z. Li, An ultrasensitive flow cytometric immunoassay based on bead surface-initiated template-free DNA extension, Chem. Sci., 2018, 9(32), 6605–6613, 10.1039/c8sc02752h , PMID: 30310592; PMCID: PMC6115634.
- P. Pantano and D. R. Walt, Ordered Nanowell Arrays, Chem. Mater., 1996, 8(12), 2832–2835, DOI:10.1021/cm9603314.
-
W. McGuigan, D. R. Fournier, G. W. Watson, L. Walling, B. Gigante, D. C. Duffy, D. M. Rissin, C. W. Kan, R. E. Meyer, T. Piech and M. W. Fishburn, The Optics Inside an Automated Single Molecule Array Analyzer, in SPIE, Proceedings of the SPIE Conference: Advanced Biomedical and Clinical Diagnostic Systems XII, ed. T. Vo-Dinh, A. Mahadevan-Jansen and W. S. Grundfest, SPIE, Bellingham, WA, San Francisco, CA, 2014 Search PubMed.
- E. Pérez-Ruiz, D. Decrop, K. Ven, L. Tripodi, K. Leirs, J. Rosseels, M. van de Wouwer, N. Geukens, A. De Vos, E. Vanmechelen, J. Winderickx, J. Lammertyn and D. Spasic, Digital ELISA for the quantification of attomolar concentrations of Alzheimer's disease biomarker protein Tau in biological samples, Anal. Chim. Acta, 2018, 1015, 74–81, DOI:10.1016/j.aca.2018.02.011 , Epub 2018 Feb 17. PMID: 29530254.
- D. Decrop, G. Pardon, L. Brancato, D. Kil, R. Zandi Shafagh, T. Kokalj, T. Haraldsson, R. Puers, W. van der Wijngaart and J. Lammertyn, Single-Step Imprinting of Femtoliter Microwell Arrays Allows Digital Bioassays with Attomolar Limit of Detection, ACS Appl. Mater. Interfaces, 2017, 9(12), 10418–10426, DOI:10.1021/acsami.6b15415 , Epub 2017 Mar 15. PMID: 28266828.
- R. Zandi Shafagh, D. Decrop, K. Ven, A. Vanderbeke, R. Hanusa, J. Breukers, G. Pardon, T. Haraldsson, J. Lammertyn and W. van der Wijngaart, Reaction injection molding of hydrophilic-in-hydrophobic femtolitre-well arrays, Microsyst. Nanoeng., 2019, 5, 25, DOI:10.1038/s41378-019-0065-2 , PMID: 31231538; PMCID: PMC6545322.
- D. Witters, K. Knez, F. Ceyssens, R. Puers and J. Lammertyn, Digital microfluidics-enabled single-molecule detection by printing and sealing single magnetic beads in femtoliter droplets, Lab Chip, 2013, 13(11), 2047–2054, 10.1039/c3lc50119a , PMID: 23609603.
- I. E. Araci, M. Robles and S. R. Quake, A reusable microfluidic device provides continuous measurement capability and improves the detection limit of digital biology, Lab Chip, 2016, 16(9), 1573–1578, 10.1039/c6lc00194g , PMID: 27072314.
- J. Sun, J. Hu, T. Gou, X. Ding, Q. Song, W. Wu, G. Wang, J. Yin and Y. Mu, Power-free polydimethylsiloxane femtoliter-sized arrays for bead-based digital immunoassays, Biosens. Bioelectron., 2019, 139, 111339, DOI:10.1016/j.bios.2019.111339 , Epub 2019 May 20. PMID: 31132722.
- J. U. Shim, R. T. Ranasinghe, C. A. Smith, S. M. Ibrahim, F. Hollfelder, W. T. Huck, D. Klenerman and C. Abell, Ultrarapid generation of femtoliter microfluidic droplets for single-molecule-counting immunoassays, ACS Nano, 2013, 7(7), 5955–5964, DOI:10.1021/nn401661d , Epub 2013 Jul 8. PMID: 23805985.
- L. Cohen, N. Cui, Y. Cai, P. M. Garden, X. Li, D. A. Weitz and D. R. Walt, Single Molecule Protein Detection with Attomolar Sensitivity Using Droplet Digital Enzyme-Linked Immunosorbent Assay, ACS Nano, 2020, 14(8), 9491–9501, DOI:10.1021/acsnano.0c02378 , Epub 2020 Jul 6. PMID: 32589401.
- J. Yi, Z. Gao, Q. Guo, Y. Wu, T. Sun, Y. Wang, H. Zhou, H. Gu, J. Zhao and H. Xu, Multiplexed Digital ELISA in Picoliter Droplets Based on Enzyme Signal Amplification Block and Precisely Decoding Strategy: A Universal and Practical Biodetection Platform, Sens. Actuators, B, 2022, 132214 CrossRef.
- X. Yue, X. Fang, T. Sun, J. Yi, X. Kuang, Q. Guo, Y. Wang, H. Gu and H. Xu, Breaking through the Poisson Distribution: A compact high-efficiency droplet microfluidic system for single-bead encapsulation and digital immunoassay detection, Biosens. Bioelectron., 2022, 114384 CrossRef PubMed.
- H. Y. Hsu, T. O. Joos and H. Koga, Multiplex microsphere-based flow cytometric platforms for protein analysis and their application in clinical proteomics - from assays to results, Electrophoresis, 2009, 30(23), 4008–4019, DOI:10.1002/elps.200900211 , PMID: 19960465.
- K. Leirs, F. Dal Dosso, E. Perez-Ruiz, D. Decrop, R. Cops, J. Huff, M. Hayden, N. Collier, K. X. Z. Yu, S. Brown and J. Lammertyn, Bridging the Gap between Digital Assays and Point-of-Care Testing: Automated, Low Cost, and Ultrasensitive Detection of Thyroid Stimulating Hormone, Anal. Chem., 2022, 94(25), 8919–8927, DOI:10.1021/acs.analchem.2c00480 , Epub 2022 Jun 10. PMID: 35687534.
- L. Cohen and D. R. Walt, Evaluation of Antibody Biotinylation Approaches for Enhanced Sensitivity of Single Molecule Array(Simoa) Immunoassays, Bioconjugate Chem., 2018, 29(10), 3452–3458, DOI:10.1021/acs.bioconjchem.8b00601 , Epub 2018 Oct 1. PMID: 30272951.
- T. L. Dinh, K. C. Ngan, C. B. Shoemaker and D. R. Walt, Using Antigen-antibody Binding Kinetic Parameters to Understand Single-Molecule Array Immunoassay Performance, Anal. Chem., 2016, 88(23), 11335–11339, DOI:10.1021/acs.analchem.6b03192 , Epub 2016 Nov 8. PMID: 27779850.
- D. Wu, E. Katilius, E. Olivas, M. Dumont Milutinovic and D. R. Walt, Incorporation of Slow Off-Rate Modified Aptamers Reagents in Single Molecule Array Assays for Cytokine Detection with Ultrahigh Sensitivity, Anal. Chem., 2016, 88(17), 8385–8389, DOI:10.1021/acs.analchem.6b02451 , Epub 2016 Aug 23. PMID: 27529794.
- M. Norman, T. Gilboa, A. F. Ogata, A. M. Maley, L. Cohen, E. L. Busch, R. Lazarovits, C. P. Mao, Y. Cai, J. Zhang, J. E. Feldman, B. M. Hauser, T. M. Caradonna, B. Chen, A. G. Schmidt, G. Alter, R. C. Charles, E. T. Ryan and D. R. Walt, Ultrasensitive high-resolution profiling of early seroconversion in patients with COVID-19, Nat. Biomed. Eng., 2020, 4(12), 1180–1187, DOI:10.1038/s41551-020-00611-x , Epub 2020 Sep 18. PMID: 32948854; PMCID: PMC7498988.
- A. F. Ogata, A. M. Maley, C. Wu, T. Gilboa, M. Norman, R. Lazarovits, C. P. Mao, G. Newton, M. Chang, K. Nguyen, M. Kamkaew, Q. Zhu, T. E. Gibson, E. T. Ryan, R. C. Charles, W. A. Marasco and D. R. Walt, Ultra-Sensitive Serial Profiling of SARS-CoV-2 Antigens and Antibodies in Plasma to Understand Disease Progression in COVID-19 Patients with Severe Disease, Clin. Chem., 2020, 66(12), 1562–1572, DOI:10.1093/clinchem/hvaa213 , PMID: 32897389; PMCID: PMC7499543.
- X. Wang, L. Cohen, J. Wang and D. R. Walt, Competitive Immunoassays for the Detection of Small Molecules Using Single Molecule Arrays, J. Am. Chem. Soc., 2018, 140(51), 18132–18139, DOI:10.1021/jacs.8b11185 , Epub 2018 Dec 11. PMID: 30495929.
- L. Song, D. Shan, M. Zhao, B. A. Pink, K. A. Minnehan, L. York, M. Gardel, S. Sullivan, A. F. Phillips, R. B. Hayman, D. R. Walt and D. C. Duffy, Direct detection of bacterial genomic DNA at sub-femtomolar concentrations using single molecule arrays, Anal. Chem., 2013, 85(3), 1932–1939, DOI:10.1021/ac303426b , Epub 2013 Jan 18. PMID: 23331316.
- L. Cohen, M. R. Hartman, A. Amardey-Wellington and D. R. Walt, Digital direct detection of microRNAs using single molecule arrays, Nucleic Acids Res., 2017, 45(14), e137, DOI:10.1093/nar/gkx542 , PMID: 28637221; PMCID: PMC5737668.
- B. López-Longarela, E. E. Morrison, J. D. Tranter, L. Chahman-Vos, J. F. Léonard, J. C. Gautier, S. Laurent, A. Lartigau, E. Boitier, L. Sautier, P. Carmona-Saez, J. Martorell-Marugan, R. J. Mellanby, S. Pernagallo, H. Ilyine, D. M. Rissin, D. C. Duffy, J. W. Dear and J. J. Díaz-Mochón, Direct Detection of miR-122 in Hepatotoxicity Using Dynamic Chemical Labeling Overcomes Stability and isomiR Challenges, Anal. Chem., 2020, 92(4), 3388–3395, DOI:10.1021/acs.analchem.9b05449 , Epub 2020 Jan 27. PMID: 31939284.
- X. Wang and D. R. Walt, Simultaneous detection of small molecules, proteins and microRNAs using single molecule arrays, Chem. Sci., 2020, 11(30), 7896–7903, 10.1039/d0sc02552f , PMID: 34094160; PMCID: PMC8163101.
- R. Chiba, K. Miyakawa, K. Aoki, T. J. Morikawa, Y. Moriizumi, T. Degawa, Y. Arai, O. Segawa, K. Tanaka, H. Tajima, S. Arai, H. Yoshinaga, R. Tsukada, A. Tani, H. Fuji, A. Sato, Y. Ishii, K. Tateda, A. Ryo and T. Yoshimura, Development of a Fully Automated Desktop Analyzer and Ultrahigh Sensitivity Digital Immunoassay for SARS-CoV-2 Nucleocapsid Antigen Detection, Biomedicines, 2022, 10(9), 2291, DOI:10.3390/biomedicines10092291 , PMID: 36140390; PMCID: PMC9496537.
- Z. Yang, Y. Atiyas, H. Shen, M. J. Siedlik, J. Wu, K. Beard, G. Fonar, J. P. Dolle, D. H. Smith, J. H. Eberwine, D. F. Meaney and D. A. Issadore, Ultrasensitive Single Extracellular Vesicle Detection Using High Throughput Droplet Digital Enzyme-Linked Immunosorbent Assay, Nano Lett., 2022, 22(11), 4315–4324, DOI:10.1021/acs.nanolett.2c00274 , Epub 2022 May 19. PMID: 35588529.
- R. S. Sista, A. E. Eckhardt, V. Srinivasan, M. G. Pollack, S. Palanki and V. K. Pamula, Heterogeneous immunoassays using magnetic beads on a digital microfluidic platform, Lab Chip, 2008, 8(12), 2188–2196, 10.1039/b807855f , Epub 2008 Oct 14. PMID: 19023486; PMCID: PMC2726047.
- K. Choi, A. H. Ng, R. Fobel, D. A. Chang-Yen, L. E. Yarnell, E. L. Pearson, C. M. Oleksak, A. T. Fischer, R. P. Luoma, J. M. Robinson, J. Audet and A. R. Wheeler, Automated digital microfluidic platform for magnetic-particle-based immunoassays with optimization by design of experiments, Anal. Chem., 2013, 85(20), 9638–9646, DOI:10.1021/ac401847x , Epub 2013 Aug 26. PMID: 23978190.
- L. Song, M. Zhao, D. C. Duffy, J. Hansen, K. Shields, M. Wungjiranirun, X. Chen, H. Xu, D. A. Leffler, S. P. Sambol, D. N. Gerding, C. P. Kelly and N. R. Pollock, Development and Validation of Digital Enzyme-Linked Immunosorbent Assays for Ultrasensitive Detection and Quantification of Clostridium difficile Toxins in Stool, J. Clin. Microbiol., 2015, 53(10), 3204–3212, DOI:10.1128/JCM.01334-15 , Epub 2015 Jul 22. PMID: 26202120; PMCID: PMC4572538.
- C. Albayrak, C. A. Jordi, C. Zechner, J. Lin, C. A. Bichsel, M. Khammash and S. Tay, Digital Quantification of Proteins and mRNA in Single Mammalian Cells, Mol. Cell, 2016, 61(6), 914–924, DOI:10.1016/j.molcel.2016.02.030 , PMID: 26990994.
- M. F. Abasıyanık, K. Wolfe, H. Van Phan, J. Lin, B. Laxman, S. R. White, P. A. Verhoef, G. M. Mutlu, B. Patel and S. Tay, Ultrasensitive digital quantification of cytokines and bacteria predicts septic shock outcomes, Nat. Commun., 2020, 11(1), 2607, DOI:10.1038/s41467-020-16124-9 , PMID: 32451375; PMCID: PMC7248118.
- J. Lin, C. Jordi, M. Son, H. Van Phan, N. Drayman, M. F. Abasiyanik, L. Vistain, H. L. Tu and S. Tay, Ultra-sensitive digital quantification of proteins and mRNA in single cells, Nat. Commun., 2019, 10(1), 3544, DOI:10.1038/s41467-019-11531-z , PMID: 31391463; PMCID: PMC6685952.
- S. A. Byrnes, T. Huynh, T. C. Chang, C. E. Anderson, J. J. McDermott, C. I. Oncina, B. H. Weigl and K. P. Nichols, Wash-Free, Digital Immunoassay in Polydisperse Droplets, Anal. Chem., 2020, 92(5), 3535–3543, DOI:10.1021/acs.analchem.9b02526 , Epub 2020 Feb 12. PMID: 31999432.
- H. Schröder, M. Grösche, M. Adler, M. Spengler and C. M. Niemeyer, Immuno-PCR with digital readout, Biochem. Biophys. Res. Commun., 2017, 488(2), 311–315, DOI:10.1016/j.bbrc.2017.04.162 , Epub 2017 May 5. PMID: 28483527.
- Z. Farka, T. Juřík, D. Kovář, L. Trnková and P. Skládal, Nanoparticle-Based Immunochemical Biosensors and Assays: Recent Advances and Challenges, Chem. Rev., 2017, 117(15), 9973–10042, DOI:10.1021/acs.chemrev.7b00037 , Epub 2017 Jul 28. PMID: 28753280.
- M. R. Monroe, G. G. Daaboul, A. Tuysuzoglu, C. A. Lopez, F. F. Little and M. S. Ünlü, Single nanoparticle detection for multiplexed protein diagnostics with attomolar sensitivity in serum and unprocessed whole blood, Anal. Chem., 2013, 85(7), 3698–3706, DOI:10.1021/ac4000514 , Epub 2013 Mar 20. PMID: 23469929; PMCID: PMC3690328.
- D. Sevenler, G. G. Daaboul, F. Ekiz Kanik, N. L. Ünlü and M. S. Ünlü, Digital Microarrays: Single-Molecule Readout with Interferometric Detection of Plasmonic Nanorod Labels, ACS Nano, 2018, 12(6), 5880–5887, DOI:10.1021/acsnano.8b02036 , Epub 2018 May 21. PMID: 29756761.
- W. Jing, Y. Wang, Y. Yang, Y. Wang, G. Ma, S. Wang and N. Tao, Time-Resolved Digital Immunoassay for Rapid and Sensitive Quantitation of Procalcitonin with Plasmonic Imaging, ACS Nano, 2019, 13(8), 8609–8617, DOI:10.1021/acsnano.9b02771 , Epub 2019 Jul 9. PMID: 31276361; PMCID: PMC7008466.
- W. Jing, Y. Wang, C. Chen, F. Zhang, Y. Yang, G. Ma, E. H. Yang, C. L. N. Snozek, N. Tao and S. Wang, Gradient-Based Rapid Digital Immunoassay for High-Sensitivity Cardiac Troponin T(hs-cTnT) Detection in 1 μL Plasma, ACS Sens., 2021, 6(2), 399–407, DOI:10.1021/acssensors.0c01681 , Epub 2020 Oct 9. PMID: 32985183; PMCID: PMC7914138.
- A. Belushkin, F. Yesilkoy, J. J. González-López, J. C. Ruiz-Rodríguez, R. Ferrer, A. Fàbrega and H. Altug, Rapid and Digital Detection of Inflammatory Biomarkers Enabled by a Novel Portable Nanoplasmonic Imager, Small, 2020, 16(3), e1906108, DOI:10.1002/smll.201906108 , Epub 2019 Dec 12. PMID: 31830370.
- C. Che, N. Li, K. D. Long, M. Á. Aguirre, T. D. Canady, Q. Huang, U. Demirci and B. T. Cunningham, Activate capture and digital counting(AC + DC) assay for protein biomarker detection
integrated with a self-powered microfluidic cartridge, Lab Chip, 2019, 19(23), 3943–3953, 10.1039/c9lc00728h , Epub 2019 Oct 23. PMID: 31641717.
- B. Zhao, C. Che, W. Wang, N. Li and B. T. Cunningham, Single-step, wash-free digital immunoassay for rapid quantitative analysis of serological antibody against SARS-CoV-2 by photonic resonator absorption microscopy, Talanta, 2021, 225, 122004, DOI:10.1016/j.talanta.2020.122004 , Epub 2020 Dec 23. PMID: 33592744; PMCID: PMC7833826.
- Z. Gao, Y. Song, T. Y. Hsiao, J. He, C. Wang, J. Shen, A. MacLachlan, S. Dai, B. H. Singer, K. Kurabayashi and P. Chen, Machine-Learning-Assisted Microfluidic Nanoplasmonic Digital Immunoassay for Cytokine Storm Profiling in COVID-19 Patients, ACS Nano, 2021, 15(11), 18023–18036, DOI:10.1021/acsnano.1c06623 , Epub 2021 Oct 29. PMID: 34714639; PMCID: PMC8577373.
- A. Agrawal, C. Zhang, T. Byassee, R. A. Tripp and S. Nie, Counting single native biomolecules and intact viruses with color-coded nanoparticles, Anal. Chem., 2006, 78(4), 1061–1070, DOI:10.1021/ac051801t , PMID: 16478096.
- X. Liu, C. Huang, X. Dong, A. Liang, Y. Zhang, Q. Zhang, Q. Wang and H. Gai, Asynchrony of spectral blue-shifts of quantum dot based digital homogeneous immunoassay, Chem. Commun., 2018, 54(93), 13103–13106, 10.1039/c8cc06754f , PMID: 30397699.
- X. Liu, X. Lin, X. Pan and H. Gai, Multiplexed Homogeneous Immunoassay Based on Counting Single Immunocomplexes together with Dark-Field and Fluorescence Microscopy, Anal. Chem., 2022, 94(15), 5830–5837, DOI:10.1021/acs.analchem.1c05269 , Epub 2022 Apr 5. PMID: 35380795.
- S. Gite, D. Archambault, M. P. Cappillino, D. Cunha, V. Dorich, T. Shatova, A. Tempesta, B. Walsh, J. A. Walsh, A. Williams, J. E. Kirby, J. Bowers and D. Straus, A Rapid, Accurate, Single Molecule Counting Method Detects Clostridium difficile Toxin B in Stool Samples, Sci. Rep., 2018, 8(1), 8364, DOI:10.1038/s41598-018-26353-0 , PMID: 29849171; PMCID: PMC5976643.
- Z. Farka, M. J. Mickert, A. Hlaváček, P. Skládal and H. H. Gorris, Single Molecule Upconversion-Linked Immunosorbent Assay with Extended Dynamic Range for the Sensitive Detection of Diagnostic Biomarkers, Anal. Chem., 2017, 89(21), 11825–11830, DOI:10.1021/acs.analchem.7b03542 , Epub 2017 Oct 10. PMID: 28949515.
- M. J. Mickert, Z. Farka, U. Kostiv, A. Hlaváček, D. Horák, P. Skládal and H. H. Gorris, Measurement of Sub-femtomolar Concentrations of Prostate-Specific Antigen through Single-Molecule Counting with an Upconversion-Linked Immunosorbent Assay, Anal. Chem., 2019, 91(15), 9435–9441, DOI:10.1021/acs.analchem.9b02872 , Epub 2019 Jul 15. PMID: 31246416.
- D. Sevenler, J. Trueb and M. S. Ünlü, Beating the reaction limits of biosensor sensitivity with dynamic tracking of single binding events, Proc. Natl. Acad. Sci. U. S. A., 2019, 116(10), 4129–4134, DOI:10.1073/pnas.1815329116 , Epub 2019 Feb 19. PMID: 30782809; PMCID: PMC6410873.
- D. Gopalan and P. R. Nair, Dynamic Tracking Biosensors: Finding Needles in a Haystack, ACS Sens., 2020, 5(5), 1374–1380, DOI:10.1021/acssensors.0c00083 , Epub 2020 Apr 17. PMID: 32253912.
- H. C. Tekin, M. Cornaglia and M. A. Gijs, Attomolar protein detection using a magnetic bead surface coverage assay, Lab Chip, 2013, 13(6), 1053–1059, 10.1039/c3lc41285g , PMID: 23392210.
- K. Akama, N. Iwanaga, K. Yamawaki, M. Okuda, K. Jain, H. Ueno, N. Soga, Y. Minagawa and H. Noji, Wash- and Amplification-Free Digital Immunoassay Based on Single-Particle Motion Analysis, ACS Nano, 2019, 13(11), 13116–13126, DOI:10.1021/acsnano.9b05917 , Epub 2019 Nov 4. PMID: 31675215.
- K. Akama and H. Noji, Multiparameter single-particle motion analysis for homogeneous digital immunoassay, Analyst, 2021, 146(4), 1303–1310, 10.1039/d0an02056g , Epub 2020 Dec 23. PMID: 33367316.
- K. Akama and H. Noji, Multiplexed homogeneous digital immunoassay based on single-particle motion analysis, Lab Chip, 2020, 20(12), 2113–2121, 10.1039/d0lc00079e , Epub 2020 Apr 29. PMID: 32347266.
- Q. Zeng, X. Zhou, Y. Yang, Y. Sun, J. Wang, C. Zhai, J. Li and H. Yu, Dynamic single-molecule sensing by actively tuning binding kinetics for ultrasensitive biomarker detection, Proc. Natl. Acad. Sci. U. S. A., 2022, 119(10), e2120379119, DOI:10.1073/pnas.2120379119 , Epub 2022 Mar 1. PMID: 35238650; PMCID: PMC8916011.
- Y. Yang, Q. Zeng, Q. Luo, C. Wang and H. Yu, Dynamic Single-Molecule Sensors: A Theoretical Study, ACS Sens., 2022, 7(7), 2069–2074, DOI:10.1021/acssensors.2c00929 , Epub 2022 Jun 16. PMID: 35709486.
- K. Chuah, Y. Wu, S. R. C. Vivekchand, K. Gaus, P. J. Reece, A. P. Micolich and J. J. Gooding, Nanopore blockade sensors for ultrasensitive detection of proteins in complex biological samples, Nat. Commun., 2019, 10(1), 2109, DOI:10.1038/s41467-019-10147-7 , PMID: 31068594; PMCID: PMC6506515.
- Y. Wu, K. Chuah and J. J. Gooding, Evaluating the sensing performance of nanopore blockade sensors: A case study of prostate-specific antigen assay, Biosens. Bioelectron., 2020, 165, 112434, DOI:10.1016/j.bios.2020.112434 , Epub 2020 Jul 8. PMID: 32729547.
- L. He, D. R. Tessier, K. Briggs, M. Tsangaris, M. Charron, E. M. McConnell, D. Lomovtsev and V. Tabard-Cossa, Digital immunoassay for biomarker concentration quantification using solid-state nanopores, Nat. Commun., 2021, 12(1), 5348, DOI:10.1038/s41467-021-25566-8 , PMID: 34504071; PMCID: PMC8429538.
- Y. Song, Y. Ye, S. H. Su, A. Stephens, T. Cai, M. T. Chung, M. K. Han, M. W. Newstead, L. Yessayan, D. Frame, H. D. Humes, B. H. Singer and K. Kurabayashi, A digital protein microarray for COVID-19 cytokine storm monitoring, Lab Chip, 2021, 21(2), 331–343, 10.1039/d0lc00678e , Epub 2020 Nov 19. PMID: 33211045; PMCID: PMC7855944.
- Y. Song, E. Sandford, Y. Tian, Q. Yin, A. G. Kozminski, S. H. Su, T. Cai, Y. Ye, M. T. Chung, R. Lindstrom, A. Goicochea, J. Barabas, M. Olesnavich, M. Rozwadowski, Y. Li, H. B. Alam, B. H. Singer, M. Ghosh, S. W. Choi, M. Tewari and K. Kurabayashi, Rapid single-molecule digital detection of protein biomarkers for continuous monitoring of systemic immune disorders, Blood, 2021, 137(12), 1591–1602, DOI:10.1182/blood.2019004399 , PMID: 33275650; PMCID: PMC8065241.
- Y. Song, J. Zhao, T. Cai, A. Stephens, S. H. Su, E. Sandford, C. Flora, B. H. Singer, M. Ghosh, S. W. Choi, M. Tewari and K. Kurabayashi, Machine learning-based cytokine microarray digital immunoassay analysis, Biosens. Bioelectron., 2021, 180, 113088, DOI:10.1016/j.bios.2021.113088 , Epub 2021 Feb 20. PMID: 33647790; PMCID: PMC7896497.
- S. Ge, W. Liu, T. Schlappi and R. F. Ismagilov, Digital, ultrasensitive, end-point protein measurements with large dynamic range via Brownian trapping with drift, J. Am. Chem. Soc., 2014, 136(42), 14662–14665, DOI:10.1021/ja507849b , Epub 2014 Oct 7. PMID: 25289692.
- T. Chatterjee, A. Knappik, E. Sandford, M. Tewari, S. W. Choi, W. B. Strong, E. P. Thrush, K. J. Oh, N. Liu, N. G. Walter and A. Johnson-Buck, Direct kinetic fingerprinting and digital counting of single protein molecules, Proc. Natl. Acad. Sci. U. S. A., 2020, 117(37), 22815–22822, DOI:10.1073/pnas.2008312117 , Epub 2020 Aug 31. PMID: 32868420; PMCID: PMC7502736.
- S. Mandal, Z. Li, T. Chatterjee, K. Khanna, K. Montoya, L. Dai, C. Petersen, L. Li, M. Tewari, A. Johnson-Buck and N. G. Walter, Direct Kinetic Fingerprinting for High-Accuracy Single-Molecule Counting of Diverse Disease Biomarkers, Acc. Chem. Res., 2021, 54(2), 388–402, DOI:10.1021/acs.accounts.0c00621 , Epub 2020 Dec 31. PMID: 33382587; PMCID: PMC8752314.
- A. M. Armani, R. P. Kulkarni, S. E. Fraser, R. C. Flagan and K. J. Vahala, Label-free, single-molecule detection with optical microcavities, Science, 2007, 317(5839), 783–787, DOI:10.1126/science.1145002 , Epub 2007 Jul 5.
- F. Liang, K. Baldyga, Q. Quan, A. Khatri, S. Choi, J. Wiener-Kronish, O. Akeju, B. M. Westover, K. Cody, Y. Shen, E. R. Marcantonio and Z. Xie, Preoperative Plasma Tau-PT217 and Tau-PT181 Are Associated With Postoperative Delirium, Ann. Surg., 2022 DOI:10.1097/SLA.0000000000005487 , Epub ahead of print. PMID: 35794069.
- D. M. Rissin and D. R. Walt, Digital readout of target binding with attomole detection limits via enzyme amplification in femtoliter arrays, J. Am. Chem. Soc., 2006, 128(19), 6286–6287, DOI:10.1021/ja058425e , PMID: 16683771.
- H. Chen, Z. Li, L. Zhang, P. Sawaya, J. Shi and P. Wang, Quantitation of Femtomolar-Level Protein Biomarkers Using a Simple Microbubbling Digital Assay and Bright-Field Smartphone Imaging, Angew. Chem., Int. Ed., 2019, 58(39), 13922–13928, DOI:10.1002/anie.201906856 , Epub 2019 Aug 21. PMID: 31344297; PMCID: PMC7211056.
- H. Chen, Z. Li, S. Feng, M. Richard-Greenblatt, E. Hutson, S. Andrianus, L. J. Glaser, K. G. Rodino, J. Qian, D. Jayaraman, R. G. Collman, A. Glascock, F. D. Bushman, J. S. Lee, S. Cherry, A. Fausto, S. R. Weiss, H. Koo, P. M. Corby, A. Oceguera, U. O'Doherty, A. L. Garfall, D. T. Vogl, E. A. Stadtmauer and P. Wang, Femtomolar SARS-CoV-2 Antigen Detection Using the Microbubbling Digital Assay with Smartphone Readout Enables Antigen Burden Quantitation and Tracking, Clin. Chem., 2021, 68(1), 230–239, DOI:10.1093/clinchem/hvab158 , PMID: 34383886; PMCID: PMC8436368.
- T. Wang, M. Zhang, D. D. Dreher and Y. Zeng, Ultrasensitive microfluidic solid-phase ELISA using an actuatable microwell-patterned PDMS chip, Lab Chip, 2013, 13(21), 4190–4197, 10.1039/c3lc50783a , PMID: 23989677.
- F. Piraino, F. Volpetti, C. Watson and S. J. Maerkl, A Digital-Analog Microfluidic Platform for Patient-Centric Multiplexed Biomarker Diagnostics of Ultralow Volume Samples, ACS Nano, 2016, 10(1), 1699–1710, DOI:10.1021/acsnano.5b07939 , Epub 2016 Jan 12. PMID: 26741022.
- J. Atallah, D. Archambault, J. D. Randall, A. Shepro, L. E. Styskal, D. R. Glenn, C. B. Connolly, K. Katsis, K. Gallagher, M. Ghebremichael and M. K. Mansour, Rapid Quantum Magnetic IL-6 Point-of-Care Assay in Patients Hospitalized with COVID-19, Diagnostics, 2022, 12(5), 1164, DOI:10.3390/diagnostics12051164 , PMID: 35626318; PMCID: PMC9139897.
- S. Beck, D. Shin, S. J. Kim, P. N. Hedde and W. Zhao, Digital Protein Detection in Bulk Solutions, ACS Omega, 2022, 7(42), 37714–37723, DOI:10.1021/acsomega.2c04666 , PMID: 36312374; PMCID: PMC9608401.
- Z. Huang, X. Zhao, J. Hu, C. Zhang, X. Xie, R. Liu and Y. Lv, Single-Nanoparticle Differential Immunoassay for Multiplexed Gastric Cancer Biomarker Monitoring, Anal. Chem., 2022, 94(37), 12899–12906, DOI:10.1021/acs.analchem.2c03013 , Epub 2022 Sep 7. PMID: 36069220.
- O. Preische, S. A. Schultz, A. Apel, J. Kuhle, S. A. Kaeser, C. Barro, S. Gräber, E. Kuder-Buletta, C. LaFougere, C. Laske, J. Vöglein, J. Levin, C. L. Masters, R. Martins, P. R. Schofield, M. N. Rossor, N. R. Graff-Radford, S. Salloway, B. Ghetti, J. M. Ringman, J. M. Noble, J. Chhatwal, A. M. Goate, T. L. S. Benzinger, J. C. Morris, R. J. Bateman, G. Wang, A. M. Fagan, E. M. McDade, B. A. Gordon and M. Jucker, Dominantly Inherited Alzheimer Network. Serum neurofilament dynamics predicts neurodegeneration and clinical progression in presymptomatic Alzheimer's disease, Nat. Med., 2019, 25(2), 277–283, DOI:10.1038/s41591-018-0304-3 , Epub 2019 Jan 21. PMID: 30664784; PMCID: PMC6367005.
- M. N. Lyngbakken, T. Vigen, H. Ihle-Hansen, J. Brynildsen, T. Berge, O. M. Rønning, A. Tveit, H. Røsjø and T. Omland, Cardiac troponin I measured with a very high sensitivity assay predicts subclinical carotid atherosclerosis: The Akershus Cardiac Examination 1950 Study, Clin. Biochem., 2021, 93, 59–65, DOI:10.1016/j.clinbiochem.2021.04.005 , Epub 2021 Apr 20. PMID: 33861986.
- G. Wu, M. Swanson, A. Talla, D. Graham, J. Strizki, D. Gorman, R. J. Barnard, W. Blair, O. S. Søgaard, M. Tolstrup, L. Østergaard, T. A. Rasmussen, R. P. Sekaly, N. M. Archin, D. M. Margolis, D. J. Hazuda and B. J. Howell, HDAC inhibition induces HIV-1 protein and enables immune-based clearance following latency reversal, JCI Insight, 2017, 2(16), e92901, DOI:10.1172/jci.insight.92901 , PMID: 28814661; PMCID: PMC5621903.
- C. P. B. Passaes, T. Bruel, J. Decalf, A. David, M. Angin, V. Monceaux, M. Muller-Trutwin, N. Noel, K. Bourdic, O. Lambotte, M. L. Albert, D. Duffy, O. Schwartz and A. Sáez-Cirión, Ultrasensitive HIV-1 p24 Assay Detects Single Infected Cells and Differences in Reservoir Induction by Latency Reversal Agents, J. Virol., 2017, 91(6), e02296-16, DOI:10.1128/JVI.02296-16 , PMID: 28077644; PMCID: PMC5331803.
- H. Lepor, C. D. Cheli, R. P. Thiel, S. S. Taneja, J. Laze, D. W. Chan, L. J. Sokoll, L. Mangold and A. W. Partin, Clinical evaluation of a novel method for the measurement of prostate-specific antigen, AccuPSA(TM) , as a predictor of 5-year biochemical recurrence-free survival after radical prostatectomy: results of a pilot study, BJU Int., 2012, 109(12), 1770–1775, DOI:10.1111/j.1464-410X.2011.10568.x , Epub 2011 Oct 12. PMID: 21992499.
- G. Sperinde, M. Hokom, K. Peng and S. K. Fischer, Bioanalytical challenges in development of ultrasensitive Home Brew assays: a case study using IL-13, Bioanalysis, 2019, 11(11), 1045–1054, DOI:10.4155/bio-2019-0012 , PMID: 31251105.
- J. Kuhle, C. Barro, U. Andreasson, T. Derfuss, R. Lindberg, Å. Sandelius, V. Liman, N. Norgren, K. Blennow and H. Zetterberg, Comparison of three analytical platforms for quantification of the neurofilament light chain in blood samples: ELISA, electrochemiluminescence immunoassay and Simoa, Clin. Chem. Lab. Med., 2016, 54(10), 1655–1661, DOI:10.1515/cclm-2015-1195 , PMID: 27071153.
- J. C. Göpfert, A. Reiser, V. A. Carcamo Yañez, A. Pohle, U. Wessels, A. Heine, T. O. Joos, C. Petit-Frère, E. Nogoceke and K. G. Stubenrauch, Development and evaluation of an ultrasensitive free VEGF-A immunoassay for analysis of human aqueous humor, Bioanalysis, 2019, 11(9), 875–886, DOI:10.4155/bio-2019-0044 , Epub 2019 May 9. PMID: 31070047.
- J. D. Pleil, M. M. Angrish and M. C. Madden, Immunochemistry for high-throughput screening of human exhaled breath condensate(EBC) media: implementation of automated Quanterix SIMOA instrumentation, J. Breath Res., 2015, 9(4), 047108, DOI:10.1088/1752-7155/9/4/047108 , PMID: 26658359.
- M. D. Hladek, S. L. Szanton, Y. E. Cho, C. Lai, C. Sacko, L. Roberts and J. Gill, Using sweat to measure cytokines in older adults compared to younger adults: A pilot study, J. Immunol. Methods, 2018, 454, 1–5, DOI:10.1016/j.jim.2017.11.003 , Epub 2017 Nov 8. PMID: 29128425; PMCID: PMC5818291.
- D. Ter-Ovanesyan, T. Gilboa, R. Lazarovits, A. Rosenthal, X. Yu, J. Z. Li, G. M. Church and D. R. Walt, Ultrasensitive Measurement of Both SARS-CoV-2 RNA and Antibodies from Saliva, Anal. Chem., 2021, 93(13), 5365–5370, DOI:10.1021/acs.analchem.1c00515 , Epub 2021 Mar 23. PMID: 33755419.
- S. M. Schubert, S. R. Walter, M. Manesse and D. R. Walt, Protein Counting in Single Cancer Cells, Anal. Chem., 2016, 88(5), 2952–2957, DOI:10.1021/acs.analchem.6b00146 , Epub 2016 Feb 11. PMID: 26813414.
- A. Saxena, P. K. Dagur, A. Desai and J. P. McCoy Jr., Ultrasensitive Quantification of Cytokine Proteins in Single Lymphocytes From Human Blood Following ex-vivo Stimulation, Front. Immunol., 2018, 9, 2462, DOI:10.3389/fimmu.2018.02462 , PMID: 30405640; PMCID: PMC6206239.
- T. Sajic, Y. Liu and R. Aebersold, Using data-independent, high-resolution mass spectrometry in protein biomarker research: perspectives and clinical applications, Proteomics: Clin. Appl., 2015, 9(3–4), 307–321, DOI:10.1002/prca.201400117 , Epub 2015 Feb 23. PMID: 25504613.
- L. Gold, D. Ayers, J. Bertino, C. Bock, A. Bock, E. N. Brody, J. Carter, A. B. Dalby, B. E. Eaton, T. Fitzwater, D. Flather, A. Forbes, T. Foreman, C. Fowler, B. Gawande, M. Goss, M. Gunn, S. Gupta, D. Halladay, J. Heil, J. Heilig, B. Hicke, G. Husar, N. Janjic, T. Jarvis, S. Jennings, E. Katilius, T. R. Keeney, N. Kim, T. H. Koch, S. Kraemer, L. Kroiss, N. Le, D. Levine, W. Lindsey, B. Lollo, W. Mayfield, M. Mehan, R. Mehler, S. K. Nelson, M. Nelson, D. Nieuwlandt, M. Nikrad, U. Ochsner, R. M. Ostroff, M. Otis, T. Parker, S. Pietrasiewicz, D. I. Resnicow, J. Rohloff, G. Sanders, S. Sattin, D. Schneider, B. Singer, M. Stanton, A. Sterkel, A. Stewart, S. Stratford, J. D. Vaught, M. Vrkljan, J. J. Walker, M. Watrobka, S. Waugh, A. Weiss, S. K. Wilcox, A. Wolfson, S. K. Wolk, C. Zhang and D. Zichi, Aptamer-based multiplexed proteomic technology for biomarker discovery, PLoS One, 2010, 5(12), e15004, DOI:10.1371/journal.pone.0015004 , PMID: 21165148; PMCID: PMC3000457.
- B. C. Carlyle, R. R. Kitchen, Z. Mattingly, A. M. Celia, B. A. Trombetta, S. Das, B. T. Hyman, P. Kivisäkk and S. E. Arnold, Technical Performance Evaluation of Olink Proximity Extension Assay for Blood-Based Biomarker Discovery in Longitudinal Studies of Alzheimer's Disease, Front. Neurol., 2022, 13, 889647, DOI:10.3389/fneur.2022.889647 , PMID: 35734478; PMCID: PMC9207419.
- J. H. Kang, M. Korecka, M. J. Figurski, J. B. Toledo, K. Blennow, H. Zetterberg, T. Waligorska, M. Brylska, L. Fields, N. Shah, H. Soares, R. A. Dean, H. Vanderstichele, R. C. Petersen, P. S. Aisen, A. J. Saykin, M. W. Weiner, J. Q. Trojanowski, L. M. Shaw and A.'s. D. N. Initiative, The Alzheimer's Disease Neuroimaging Initiative 2 Biomarker Core: A review of progress and plans, Alzheimer's Dementia, 2015, 11(7), 772–791, DOI:10.1016/j.jalz.2015.05.003 , PMID: 26194312; PMCID: PMC5127404.
- J. Randall, E. Mörtberg, G. K. Provuncher, D. R. Fournier, D. C. Duffy, S. Rubertsson, K. Blennow, H. Zetterberg and D. H. Wilson, Tau proteins in serum predict neurological outcome after hypoxic brain injury from cardiac arrest: results of a pilot study, Resuscitation, 2013, 84(3), 351–356, DOI:10.1016/j.resuscitation.2012.07.027 , Epub 2012 Aug 9. PMID: 22885094.
- H. Zetterberg, E. Mörtberg, L. Song, L. Chang, G. K. Provuncher, P. P. Patel, E. Ferrell, D. R. Fournier, C. W. Kan, T. G. Campbell, R. Meyer, A. J. Rivnak, B. A. Pink, K. A. Minnehan, T. Piech, D. M. Rissin, D. C. Duffy, S. Rubertsson, D. H. Wilson and K. Blennow, Hypoxia due to cardiac arrest induces a time-dependent increase in serum amyloid β levels in humans, PLoS One, 2011, 6(12), e28263, DOI:10.1371/journal.pone.0028263 , Epub 2011 Dec 14. PMID: 22194817; PMCID: PMC3237426.
- H. Zetterberg and K. Blennow, Blood Biomarkers: Democratizing Alzheimer's Diagnostics, Neuron, 2020, 106(6), 881–883, DOI:10.1016/j.neuron.2020.06.004 , PMID: 32553204.
- L. Álvarez-Sánchez, C. Peña-Bautista, M. Baquero and C. Cháfer-Pericás, Novel Ultrasensitive Detection Technologies for the Identification of Early and Minimally Invasive Alzheimer's Disease Blood Biomarkers, J. Alzheimer's Dis., 2022, 86(3), 1337–1369, DOI:10.3233/JAD-215093 , PMID: 35213367.
- S. Thebault, R. A. Booth and M. S. Freedman, Blood Neurofilament Light Chain: The Neurologist's Troponin?, Biomedicines, 2020, 8(11), 523, DOI:10.3390/biomedicines8110523 , PMID: 33233404; PMCID: PMC7700209.
- K. Blennow and H. Zetterberg, Biomarkers for Alzheimer's disease: current status and prospects for the future, J. Intern. Med., 2018, 284(6), 643–663, DOI:10.1111/joim.12816 , Epub 2018 Aug 19. PMID: 30051512.
- D. Li and M. M. Mielke, An Update on Blood-Based Markers of Alzheimer's Disease Using the SiMoA Platform, Neurol. Ther., 2019, 8(Suppl 2), 73–82, DOI:10.1007/s40120-019-00164-5 , Epub 2019 Dec 12. PMID: 31833025; PMCID: PMC6908531.
- X. Ding, S. Zhang, L. Jiang, L. Wang, T. Li and P. Lei, Ultrasensitive assays for detection of plasma tau and phosphorylated tau 181 in Alzheimer's disease: a systematic review and meta-analysis, Transl. Neurodegener., 2021, 10(1), 10, DOI:10.1186/s40035-021-00234-5 , PMID: 33712071; PMCID: PMC7953695.
- C. Groot, C. Cicognola, D. Bali, G. Triana-Baltzer, J. L. Dage, M. J. Pontecorvo, H. C. Kolb, R. Ossenkoppele, S. Janelidze and O. Hansson, Diagnostic and prognostic performance to detect Alzheimer's disease and clinical progression of a novel assay for plasma
p-tau217, Alzheimers Res. Ther., 2022, 14(1), 67, DOI:10.1186/s13195-022-01005-8.
- B. D. Reed, M. J. Meyer, V. Abramzon, O. Ad, O. Ad, P. Adcock, F. R. Ahmad, G. Alppay, J. A. Ball, J. Beach, D. Belhachemi, A. Bellofiore, M. Bellos, J. F. Beltrán, A. Betts, M. W. Bhuiya, K. Blacklock, R. Boer, D. Boisvert, N. D. Brault, A. Buxbaum, S. Caprio, C. Choi, T. D. Christian, R. Clancy, J. Clark, T. Connolly, K. F. Croce, R. Cullen, M. Davey, J. Davidson, M. M. Elshenawy, M. Ferrigno, D. Frier, S. Gudipati, S. Hamill, Z. He, S. Hosali, H. Huang, L. Huang, A. Kabiri, G. Kriger, B. Lathrop, A. Li, P. Lim, S. Liu, F. Luo, C. Lv, X. Ma, E. McCormack, M. Millham, R. Nani, M. Pandey, J. Parillo, G. Patel, D. H. Pike, K. Preston, A. Pichard-Kostuch, K. Rearick, T. Rearick, M. Ribezzi-Crivellari, G. Schmid, J. Schultz, X. Shi, B. Singh, N. Srivastava, S. F. Stewman, T. R. Thurston, T. R. Thurston, P. Trioli, J. Tullman, X. Wang, Y. C. Wang, E. A. G. Webster, Z. Zhang, J. Zuniga, S. S. Patel, A. D. Griffiths, A. M. van Oijen, M. McKenna, M. D. Dyer and J. M. Rothberg, Real-time dynamic single-molecule protein sequencing on an integrated semiconductor device, Science, 2022, 378(6616), 186–192, DOI:10.1126/science.abo7651 , Epub 2022 Oct 13, PMID: 36227977.
- I. Lee, S. J. Kwon, M. Sorci, P. S. Heeger and J. S. Dordick, Highly Sensitive Immuno-CRISPR Assay for CXCL9 Detection, Anal. Chem., 2021, 93(49), 16528–16534, DOI:10.1021/acs.analchem.1c03705 , Epub 2021 Dec 4. PMID: 34865465; PMCID: PMC9012118.
-
L. Skolrood, Y. Wang, S. Zhang and Q. Wei, Single-molecule and particle detection on true portable microscopy platforms, Sensors and Actuators Reports, 2022, vol. 4, p. 100063, ISSN 2666–0539, DOI:10.1016/j.snr.2021.100063.
|
This journal is © The Royal Society of Chemistry 2023 |
Click here to see how this site uses Cookies. View our privacy policy here.