DOI:
10.1039/D3MA00449J
(Review Article)
Mater. Adv., 2023,
4, 5882-5919
Computing of neuromorphic materials: an emerging approach for bioengineering solutions
Received
22nd July 2023
, Accepted 17th October 2023
First published on 18th October 2023
Abstract
The potential of neuromorphic computing to bring about revolutionary advancements in multiple disciplines, such as artificial intelligence (AI), robotics, neurology, and cognitive science, is well recognised. This paper presents a comprehensive survey of current advancements in the use of machine learning techniques for the logical development of neuromorphic materials for engineering solutions. The amalgamation of neuromorphic technology and material design possesses the potential to fundamentally revolutionise the procedure of material exploration, optimise material architectures at the atomic or molecular level, foster self-adaptive materials, augment energy efficiency, and enhance the efficacy of brain–machine interfaces (BMIs). Consequently, it has the potential to bring about a paradigm shift in various sectors and generate innovative prospects within the fields of material science and engineering. The objective of this study is to advance the field of artificial intelligence (AI) by creating hardware for neural networks that is energy-efficient. Additionally, the research attempts to improve neuron models, learning algorithms, and learning rules. The ultimate goal is to bring about a transformative impact on AI and better the overall efficiency of computer systems.
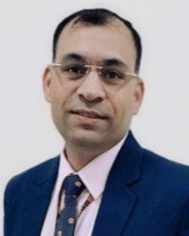
Chander Prakash
| Chander Prakash: Prof. Prakash serving Dean, Research and Development and Professor at SVKM’S Narsee Monjee Institute of Management Studies, Mumbai, India. He served as Dean, Research & Development/School of Mechanical Engineering at Lovely Professional University, India. He did his PhD in Mechanical Engineering at Panjab University, Chandigarh. His research interests are bio-manufacturing, surface modification of bio-materials and computer modeling and simulation. He has published over 350 scientific articles in peer-reviewed journals. He is a highly cited researcher with 7022 citations (H-index 47). He is one of the Top 1% of leading scientists in Mechanical and Aerospace Engineering in India, as per Research.com. |
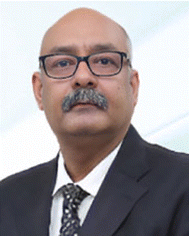
Lovi Raj Gupta
| Lavi Raj Gupta: Dr Lovi Raj Gupta is the Pro Vice Chancellor, Lovely Professional University. He holds a PhD in Bioinformatics. He did his MTech in Computer-Aided Design & Interactive Graphics from IIT, Kanpur and BE (Hons) Mechanical Engineering from MITS, Gwalior. His research interests are in the areas of Robotics, Mechatronics, Bioinformatics, Internet of Things (IoT), AI & ML using Tensor Flow (CMLE) and Gamification. He has authored 7 books along with several scientific articles. He has been appointed as Leading Scientist in Mega Project on Neuromorphic and memristive Materials by Russian Federation at Southern Federal University SFedU, Russia. |
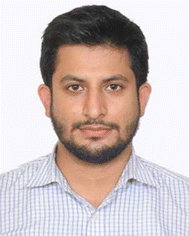
Amrinder Mehta
| Mr Amrinder Mehta is a Deputy Superintendent in the Research and Development Cell (RDC) at Lovely Professional University in Phagwara, Punjab, India. In 2015, he received his master's degree from Lovely Professional University in Phagwara, Punjab, India, and is currently pursuing his PhD Surface Engineering/Thermal Spraying is one of his research interests (HVOF, FLAME SPRAY, COLD SPRAY, AND PLASMA SPRAY). He is currently working on Nano-structured‖Multi-modal‖High entropy alloys coatings for high-temperature oxidation and corrosion resistance—Thermal Barrier Coatings (TBCs) and Microwave material processing. |
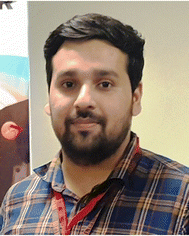
Hitesh Vasudev
| Prof. Hitesh Vasudev is working as a Professor in the Department of Mechanical Engineering, Lovely Professional University (LPU), Phagwara, Punjab, India. He has received his PhD degree from Guru Nanak Dev Engineering College, Ludhiana-India. His research areas includes Surface Engineering/Thermal Spraying. Currently working on the development of Nano-structured materials and hybrid materials. He has won the Research Excellence Award for three consecutive years (2019, 2020, 2021 & 2022) at LPU. He has published over 100 indexed papers, 15 conference papers, 4 books, and 25 book chapters. He also consistently appeared in the top 2% of researchers as per Stanford Study in 2023. |
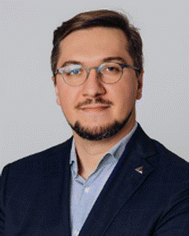
Alexander Fedotov
| Prof. Alexander Fedotov, PhD, serving Director of the Institute of Nanotechnologies, Electronics and Equipment Engineering, Southern Federal University (SFedU), Rostov-on-Don – Taganrog, Russia. Prof. Fedotov is also a leading scientist at the Research Laboratory Neuroelectronics and Memristive Nanomaterials founded at SFedU with the support of the Russian Government in 2022. His research interests lie in the field of the nanotechnology industry, micro- and nanosystems engineering, nanoelectronics, carbon nanotubes, hybrid carbon nanostructures, composite nanomaterials, and MEMS technologies. He published over 100 scientific papers, including 44 papers indexed in Scopus/WoS (Hirsch Index – 10), 101 papers indexed by RSCI. Since 2020, Prof. Fedotov is Head of the PhD Degree in Electronic component base of micro- and nanoelectronics, quantum devices. |
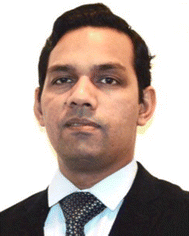
Kavindra Kumar Kesari
| Dr Kavindra Kesari is a Senior Researcher in the Department of Applied Physics, Aalto University and the University of Helsinki, Finland. He obtained a Doctoral degree in Biotechnology and received Junior and Senior Research fellowships during his Doctoral studies at Jawaharlal Nehru University, New Delhi, India. He is actively involved working in material-based neuro and cancer biology research. He has published over 150 papers (H-index 36: Google Scholar) in reputed scientific journals, 30 book chapters, 7 books, and presented over 40 papers at national and international scientific meetings. He is acting as Commissioner at the ICBE EMF, USA, since 2021 and Honorary Faculty Member for NGCEF, New South Wales, Australia, since 2020. |
1. Introduction
The human brain's ideas and architecture are used as models in the developing field of neuromorphic computing, which aims to create highly specialized and efficient computing systems.
An overview of the major milestones and advances in neuromorphic computing and intelligent computing discovery can be seen in (Fig. 1). This has allowed for the development of powerful artificial intelligence (AI) systems that can process large amounts of data quickly and accurately. The very term “neuromorphic” was coined by Carver Mead in the late 1980s. Neuromorphic computing has the potential to revolutionize the way AI systems are designed and utilized.1–3 It has already been used in a variety of applications, from medical diagnostics to autonomous vehicles.
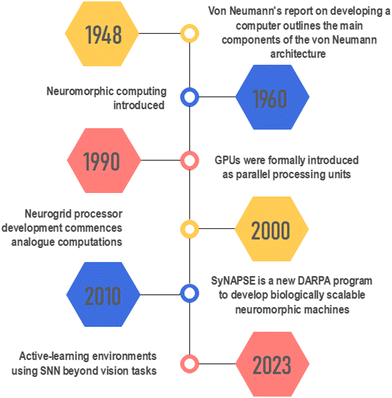 |
| Fig. 1 Intelligent computing discovery and advancement timeline.1–5 | |
The neural networks of the human brain are mimicked by these systems, also referred to as neuromorphic computers. By drawing on the brain's capacity for parallel information processing, handling complex patterns, and environment adaptation, neuromorphic computers seek to get around some of the drawbacks of conventional computing systems.4 For pattern recognition, sensory processing, and cognitive computing tasks, it uses specialized hardware and software implementations. Artificial neural networks, which are computational models that imitate the activity of organic neurons, are one of the main components of neuromorphic computers.6,7 These networks are made up of interconnected “neurons,” or nodes, that process and send data using electrical signals. Neuromorphic systems can accomplish high-performance computing using less energy than traditional von Neumann computers by emulating the parallel processing and connectivity of brain networks. Artificial intelligence, robotics, neurology, and cognitive science are a few of the domains that neuromorphic computing has the potential to change.8–11 Researchers and engineers may create more effective and intelligent systems to process and comprehend complicated data patterns, learn from experience, and adapt to new conditions by utilizing the capability of neuromorphic computers. Although neuromorphic computing exhibits enormous promise, it is still a developing area, and actual applications for neuromorphic computers are still in the planning stages.12–15
There is still hope for major developments in this field due to continuous research and advancements. Major breakthroughs in neuromorphic computing could revolutionize the way that computers are used and could open up possibilities for new and innovative applications. The potential for this technology is immense, and its development could have a profound effect on the computing industry. As seen in (Fig. 2), neuromorphic computers differ from conventional computing designs in numerous key operational ways. Here are some significant points of distinction.16
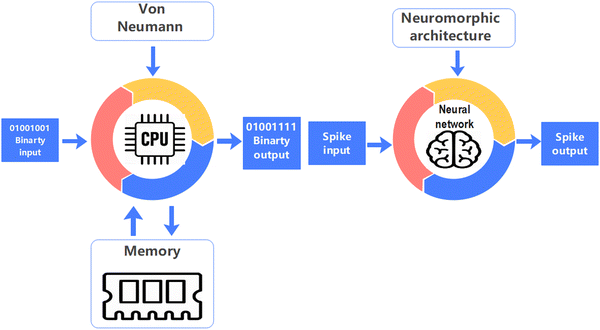 |
| Fig. 2 Von Neumann architecture vs. neuromorphic architecture. | |
Traditionally, one action at a time is carried out by traditional computers as process information sequentially. In contrast, neuromorphic computers are built to make use of parallelism and are motivated by the brain's capacity to handle several inputs at once. Complex tasks with imprecisely defined conditions and noisy input data can be processed more quickly and effectively using neuromorphic architectures since they can run calculations in parallel across numerous nodes or neurons.17–20 In order to mimic the behavior of artificial neural networks, which are made up of interconnected nodes (neurons), neuromorphic computers were created. Emulation enables neuromorphic computers to carry out more effectively tasks like pattern recognition, machine learning, and cognitive computing. Traditional computers, on the other hand, employ a more generalized architecture that is not tailored to these activities.21–23 To solve this problem, energy-efficient neuromorphic computer architectures were designed. These make use of the idea of spiking neural networks, in which calculations are based on the transmission of electrical spikes that resemble the firing of brain neurons. Compared to typical computing designs, which frequently use more energy for sequential processing and data movement, this method can dramatically minimize the amount of energy used. The ability to change their internal connections in response to experience or training makes neuromorphic computers excellent at adaptive learning. The system can learn from data and adapt to changing situations thanks to a property known as plasticity. Traditional computers do not have the natural adaptability and plasticity of neuromorphic systems, despite being capable of learning through software algorithms.24–26 Real-time integration and processing of sensory data is a strong suit for neuromorphic computers. Rapid sensory input processing is essential for decision-making in applications like robotics, where this capability is very useful. To accomplish a similar level of real-time sensory integration on traditional computers, more hardware and complicated algorithms are frequently needed. It is crucial to remember that while neuromorphic computing has several benefits, it is not meant to completely replace conventional computing architectures. It is better suited for certain tasks that benefit from parallelism, pattern recognition, and real-time adaptability, and it does not replace conventional computing methods; rather, it augments them. A more diverse and potent computer ecosystem may be possible by combining the two computing paradigms. By combining the strengths of both neuromorphic and conventional computing, it is possible to create powerful and efficient computing architectures. This could have a significant impact on the development of AI and machine learning applications that are more powerful and faster than ever before.27–29
In neuromorphic computing, most research is focused on the hardware systems, devices, and materials mentioned above. However, to fully utilize neuromorphic computers in the future, to exploit their unique computational characteristics, and to drive their hardware design, neuromorphic algorithms and applications must be utilized. Therefore, it is necessary to study and develop neuromorphic algorithms and applications that can be used to optimize the hardware design and maximize the use of neuromorphic computers, taking into account the unique computational characteristics of neuromorphic computers. Electronics, telecommunications, and computing all use analog and digital signal representation or processing techniques, which are two distinct types. Here is a quick description of each idea.30–33 Continuous signals or data that fluctuate smoothly and indefinitely over time or place are referred to as analog. In analog systems, physical quantities like voltage, current, or sound waves are used to represent information. Analog signals can take on any value within a continuous range, which distinguishes them from digital signals.34 An analog clock with moving hands, for instance, depicts time passing constantly as the hands move across the dial. Contrarily, the term “digital” describes discrete signals or data that are represented using a limited number of symbols or values. A series of 0 s and 1 s, commonly referred to as bits, are used in digital systems to represent information in binary form. Digital tools like computers can manipulate and process these bits. Digital signals are discrete in nature and have values that can only exist at certain levels. For instance, a digital clock uses incrementally changing digits to show the current time. The way information is expressed and processed is where analog and digital differ most.35–37 Digital signals are discrete and have a finite number of values, but analog signals are continuous and can theoretically have an endless number of values. Advantages of digital signals include improved precision, resistance to noise, and the capacity to store and analyze significant volumes of data. However, there are still a lot of applications for analog signals, particularly in fields like audio and video where maintaining the continuity of the signal is crucial for accurate reproduction. Analog signals are also used for control systems, where a real-time response is required. It is proposed in this work that all types of hardware implementations – digital, mixed analog-digital, and analog – are neuromorphic, but here we restrict our attention to spiking neuromorphic computers, i.e. those that implement spike-based neural networks. While analog systems are more efficient in some tasks, digital systems are more reliable and easier to scale.38–40 Digital systems can also be more easily modified, allowing for more customizability. Overall, the choice between analog and digital systems depends on the nature of the task and the desired goals.
Biomaterials are substances that have been developed to interact with living tissues and organs and other biological systems. They are suitable for use in biomedical applications because they have certain qualities. Some of the essential traits of biomaterials are depicted in (Fig. 3). Biocompatible materials are those that do not have negative effects or are hazardous when they come into contact with live tissues. They should not provoke an inflammatory response of the immune system. Bioactive qualities can be found in biomaterials, which means they can interact with biological systems and encourage particular cellular responses. Bioactive substances, for instance, can promote cell adhesion, proliferation, and differentiation. The mechanical characteristics of the tissues or organs where biomaterials are implanted should match those of the biomaterials themselves.41–44 This guarantees compatibility and lessens strain on the tissues in the area.
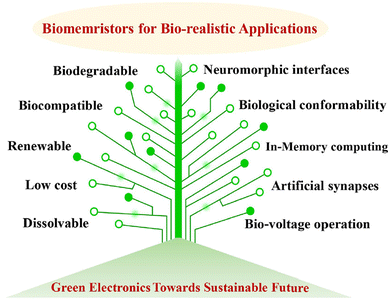 |
| Fig. 3 Overview of memristors with biomaterials for biorealistic features.45 | |
Biomaterials may need to deteriorate gradually over time depending on the use. Degradable biomaterials can be made to degrade gradually, enabling the body to progressively absorb them or regenerate tissue. For interactions with cells and tissues, biomaterials' surface characteristics are essential. Surface alterations can regulate interactions such as protein binding and cell adhesion. As shown in Fig. 4 a type of electronic device called biomaterial-based ultra-flexible artificial synaptic device imitates the operation of organic synapses present in the human brain. Biomaterials, which are substances that interact with biological systems in a functional and compatible way, are used in the construction of these devices.46 Replicating the synaptic connections between neurons in the brain in biomaterial-based ultra-flexible artificial synaptic devices is their main objective to enable them to carry out functions including learning, memory, and information processing.47
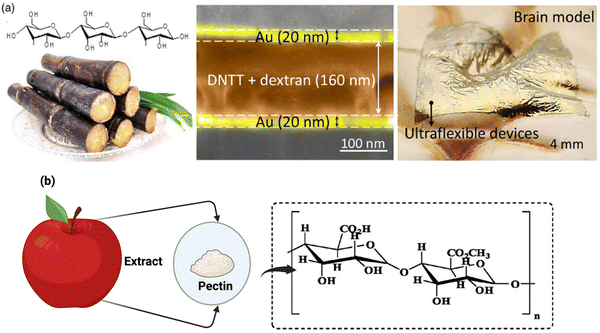 |
| Fig. 4 Biomaterial-based artificial synaptic devices with ultraflexibility: (a) ultraflexible organic synaptic transistors based on dextran;48–50 (b) organic synaptic transistors based on pectin from apples. | |
Typical components of these devices include flexible substrates, conductive substances, and synaptic elements. There are many benefits of using biomaterials in these devices. First, they can interact with biological systems without harming them or being rejected, biomaterials are biocompatible. This is crucial for creating technological innovations that can easily meld with organic tissues, including the brain. Second, biomaterials may display characteristics that are similar to those of the brain's actual synaptic connections.51–53 For instance, some biomaterials can modify electrical signals and enhance ion transport, replicating the actions of real synapses. Additionally, because of their extreme flexibility, which enables them to adapt to irregular surfaces and move with mechanical deformations, these devices are useful in areas where standard rigid electronics would be ineffective or harmful.54–57 Although this field of study is still in its early stages, engineers and scientists are working hard to create ultra-flexible artificial synaptic devices based on biomaterials.58,59 By enabling effective and biocompatible brain-inspired computing systems, these gadgets have the potential to transform such areas as neuromorphic computing, brain–machine interfaces, and artificial intelligence. These devices could also revolutionize healthcare by providing an efficient platform for drug delivery and personalized treatments.60,61 In addition, they could be used to restore motor, sensory, and cognitive function in patients with neurological diseases.
2. Integration of neuromorphic computing with material design
The amalgamation of neuromorphic computing and material design has the capacity to fundamentally transform the process of material development, resulting in the creation of innovative materials that exhibit improved characteristics and performance. Neuromorphic computing, drawing inspiration from the structural organisation of the human brain, facilitates the effective and adaptable processing of information.62 As shown in (Fig. 5) the qualities and performance of the generated materials are affected by neuromorphic computing and material design.
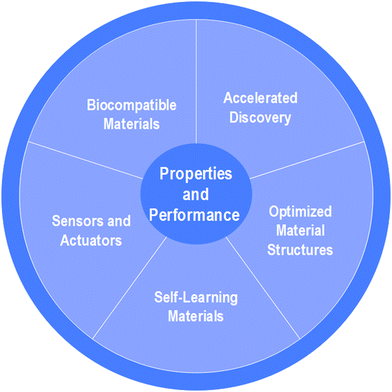 |
| Fig. 5 Material design and neuromorphic computing change the properties and performance of the materials. | |
When integrated with material design, it has the potential to influence materials in several manners. The utilisation of neuromorphic computing has the potential to greatly enhance the efficiency of material discovery by expediting the process. The utilisation of simulation and prediction techniques enables the estimation of material properties by leveraging existing data, hence mitigating the necessity for significant experimental investigations.63 The utilisation of neuromorphic algorithms enables the optimisation of material structures at the atomic or molecular scale. This phenomenon has the potential to facilitate the development of materials possessing customised characteristics, such as enhanced strength, conductivity, or thermal stability. Self-learning materials refer to educational resources that are designed to facilitate independent learning. These materials are typically created to enable individuals to acquire knowledge. Materials that are integrated with neuromorphic computing can dynamically adjust and respond to varying environmental conditions.64 Organisms possess the ability to acquire knowledge from their surroundings and modify their characteristics, accordingly, rendering them remarkably versatile and receptive. Energy efficiency is a notable characteristic of neuromorphic systems, and this attribute can be utilised to develop materials that exhibit enhanced energy efficiency. For instance, the advancements discussed can have advantageous implications for smart energy storage and conversion materials. Sensors and actuators can be effectively implemented using materials engineered with neuromorphic computing capabilities, enabling them to exhibit exceptional sensitivity and responsiveness.65 These entities possess the ability to perceive and react to alterations in their surroundings or external stimuli, rendering them highly advantageous in domains such as robotics and healthcare. The integration of neuromorphic computing with materials has the potential to enhance the development of brain–machine interfaces (BMIs). The functionality of these interfaces is contingent upon the utilisation of biocompatible materials that effectively engage with neural impulses, hence facilitating a seamless exchange of information between the brain and external equipment.66
2.1. Enhanced material characterization
The utilisation of neuromorphic approaches has the potential to increase the processes involved in material characterization, hence facilitating a more comprehensive understanding and predictive capability about the behaviour of materials across varying situations. The utilisation of materials including neuromorphic computation holds potential for application in drug discovery and delivery systems. Drug release profiles can be adjusted, resulting in enhanced efficacy and focused therapeutic interventions. The integration of neuromorphic computing into materials enables the monitoring of their health and integrity.67 These systems can identify instances of damage or deterioration, commence the necessary repair procedures, or alert users of maintenance requirements. The utilisation of neuromorphic techniques holds promise in the advancement of biocompatible materials for medical implants, as well as the development of materials that emulate biological systems for diverse applications.68 The utilisation of neuromorphic computing has the potential to expedite the exploration and development of quantum materials possessing distinctive electrical characteristics that are of utmost importance in the fields of quantum computing and advanced electronics. In general, the amalgamation of neuromorphic computing and material design exhibits potential in the development of materials that possess not only significant optimisation but also adaptability, energy efficiency, and the ability to react to dynamic circumstances.69 The adoption of an interdisciplinary approach has the potential to revolutionise multiple industries and create novel opportunities in the fields of material science and engineering. These materials have the potential to be integrated into existing devices and systems, allowing for more efficient and adaptive operations. Furthermore, the interdisciplinary approach is likely to open new areas of research and collaboration, leading to further advancements in technology.
3. Overview of neuromorphic algorithms
Artificial neural networks that imitate the structure and operation of the human brain are called neuromorphic artificial neural networks (ANNs). The phrase “neuromorphic” describes the design approach of replicating the structure and computational principles of the human brain. Neuromorphic artificial neural networks (ANNs) are built to operate on specialized hardware known as neuromorphic chips or processors, as opposed to ordinary artificial neural networks which are normally implemented on conventional computing systems. These chips utilize the parallelism and low power consumption characteristics found in biological neural networks to effectively process neural network computations. Spiking neural network models, which use discrete spikes or pulses of activity to represent and transfer information, are frequently used in neuromorphic ANNs. Traditional artificial neural networks use continuous activation levels in contrast to this. Spiking neural networks (SNN) result in various advantages including event-driven computing, effective temporal information encoding, and increased energy efficiency. These are thought to be more physiologically realistic. An emerging area of research called “neuromorphic computing” intends to create hardware and software architectures for computers that are modeled after the structure and operation of the human brain.
The word “neuromorphic” is a combination of “neuro,” which refers to the brain and nervous system, and “morphic,” which denotes the imitation or resemblance of a particular form or structure. In Table 1, biological neural networks, ANNs, and SNNs are contrasted. ANNs are composed of multiple layers of interconnected neurons. SNNs imitate the behavior of neurons in the brain using spikes of electrical signals to represent data. Both types of networks are used in machine learning for pattern recognition and data analysis. As shown in Fig. 6, biological neurons, ANNs, and SNNs differ from each other. Biological neurons are processing units in the brain, ANNs are artificial neurons that simulate the functions of biological neurons, and SNNs are a type of ANN that mimics the behavior of biological neurons using spiking signals.
Table 1 Comparison of the characteristics of ANNs, SNNs, and biological neural networks
S. no. |
Properties |
Biological NNs |
SNNs |
ANNs |
1 |
Representation of information |
Spikes |
Spikes |
Scalars |
2 |
Learning model |
Neural plasticity |
Plasticity |
BP |
3 |
Platform |
Brain |
Neuromorphic VLSI |
VLSI |
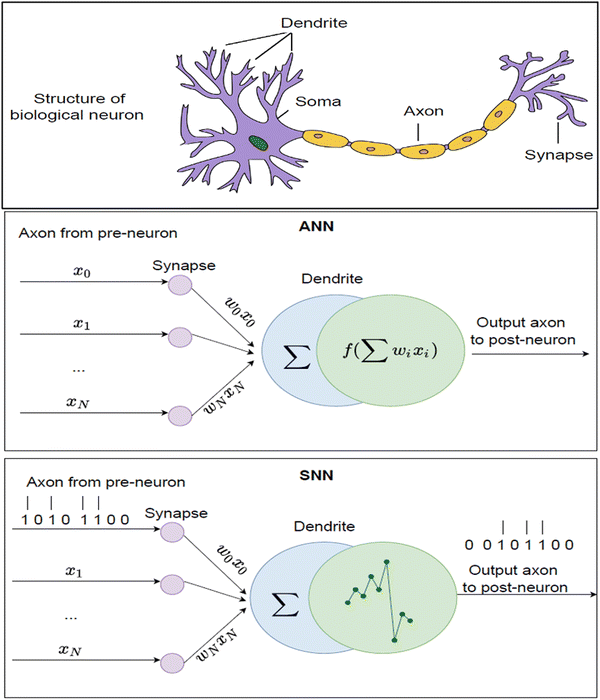 |
| Fig. 6 Analysis of the biological neuron, ANN, and SNN.70 | |
Biological neurons are connected by synapses and communicate by exchanging electrical signals. In contrast, ANNs are connected by weighted connections and communicate by exchanging numerical values. SNNs communicate by exchanging spike trains, which more closely resemble the behavior of biological neurons. These systems' specialized hardware and built-in algorithms allow them to carry out tasks like pattern recognition, sensory processing, and learning very effectively and in parallel.71–74 Algorithms for neuromorphic computers have been developed using a variety of strategies, as shown in Fig. 7.
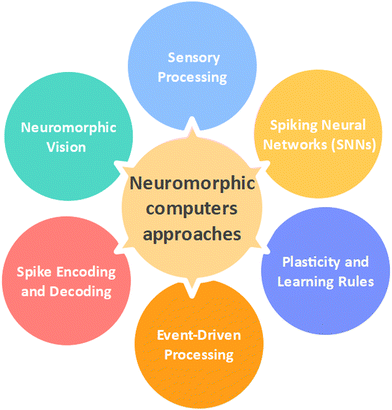 |
| Fig. 7 Neuromorphic computer approach algorithms. | |
Neuromorphic computing systems are becoming increasingly popular due to their ability to quickly process large amounts of data and perform tasks that traditional computers may not be able to do as easily. These systems also have the potential to significantly reduce energy consumption in the future. Spiking neural networks are a type of artificial neural network that simulates both the timing and behavior of individual neurons. It can benefit from the hardware's event-driven design, making it well-suited for neuromorphic computing. The firing rates and synaptic weights of the neurons are calculated by SNN algorithms to carry out operations like classification, grouping, and prediction. Plasticity mechanisms modeled after biological synapses are frequently included in neuromorphic computers, enabling them to adjust to and learn from the input data.75–78 The synaptic weights between neurons are modified by algorithms based on Hebbian learning, spike-timing-dependent plasticity (STDP), or other biologically inspired learning rules. These algorithms give the system the ability to self-organize, discover patterns, and enhance its functionality over time. The event-driven processing strategy used by neuromorphic computing is one of its fundamental characteristics. The system responds to events or changes in the input rather than processing data continuously.
To effectively manage incoming spikes or events and propagate them through the network, triggering the necessary computations and reactions, algorithms are devised. Spike encoding and decoding methods are essential because neuromorphic computers frequently work on spiking brain activity.79–81 The time and intensity of spikes are represented by spike trains, which are created by spike encoding methods from continuous data. Spike decoding algorithms, on the other hand, evaluate the spiking activity produced by the system to extract significant information or produce suitable outputs. Vision and sensory processing tasks are particularly well suited for neuromorphic computers.
To extract features and make sense of the input, hierarchical processing is a common component of the algorithms used for these applications. These algorithms make it possible to recognize objects, detect motion, and identify gestures. It is crucial to remember that research in the subject of neuromorphic computing is still ongoing, and new techniques and algorithms are constantly being created. The examples show some typical methods, but researchers may also investigate creative algorithms and modifications.83–85 This research is pushing the boundaries of what is possible and is revolutionizing the way we interact with machines. It has the potential to unlock new capabilities and applications that were previously impossible. As technology continues to evolve, it will create exciting new opportunities for exploration and development. A simplified schematic illustration demonstrating how biological nociceptors detect an external stimulus and send the signal to the brain via the spinal cord is illustrated in (Fig. 8). The illustration starts with an external stimulus operating on a particular bodily part, such as heat, pressure, or chemicals.86 Specialized sensory nerve endings called nociceptors are present throughout the body. They are in charge of recognizing and reacting to unpleasant or potentially dangerous stimuli.
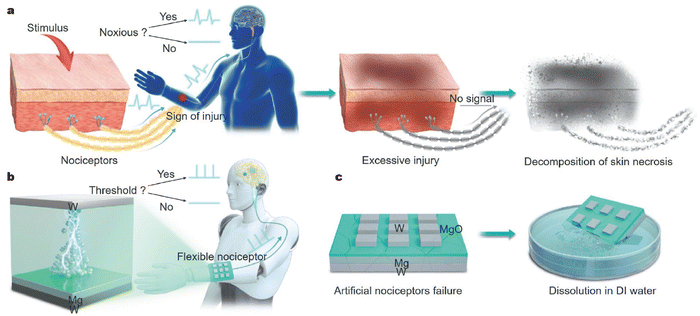 |
| Fig. 8 Diagram of biological nociceptors that detect external stimuli in (a). An action potential is transmitted from the spinal cord to the brain when the biological signal produced by painful stimuli is greater than the threshold value. Additionally, after extensive skin damage, the nociceptors lose their ability to send signals and their ability to perceive pain. The skin eventually turns necrotic, peels off, decomposes, and vanishes on its own. (b) Realization of biodegradable and biocompatible nociceptive emulators. (c) In order to mimic the skin necrosis's breakdown, the artificial nociceptors that did not work disintegrate in DI water.82 | |
The nociceptors in the afflicted area are activated when the external stimuli reach a specific threshold value. On their membrane, these nociceptors have particular receptors that react to various stimuli. The nociceptors produce electrical signals in the form of action potentials after they are engaged. An electrical impulse that travels along nerve fibers in an all-or-nothing fashion is known as an action potential. The action potential is produced by nociceptors, a type of sensory neuron, and it moves down their nerve fibers. These neurons have lengthy extensions called axons that can travel great distances to convey electrical messages. As the nociceptors enter the spinal cord, their axons converge and form bundles. Within the vertebral column, the spinal cord is a long, cylindrical substance. The nociceptors' axons join other neurons inside the spinal cord to form synapses. Electrical signals are changed into chemical messages at synapses.87–89 The presynaptic terminals of sensory neurons release neurotransmitters, which then bind to receptors on the postsynaptic neurons. The ascending spinal cord pathways receive the nociceptive signal via synaptic transmission. Higher brain areas, in particular the thalamus and somatosensory cortex, receive the signal via these pathways, which is where pain perception and interpretation take place. The transmitted signal is processed and interpreted by the brain once it gets there, which causes the impression of pain or discomfort. This knowledge is combined with other sensory and cognitive inputs by the brain to produce the proper response or behavioral output. It is crucial to remember that the many mechanisms involved in nociception and pain perception are simplified in this schematic picture. The real mechanisms are more complex and entail interacting with different neuronal types, neurotransmitters, and areas of the brain.90–93 This is why it is so important to research the neurobiological basis of pain and to develop therapies to target these underlying mechanisms. Additionally, research into the complex relationship between the mind and body can further help us to understand the subjective experience of pain.
3.1. Hardware acceleration for neural network
The use of specialized hardware and architectures created to speed up the execution of neural network algorithms is known as hardware acceleration for neural networks. The performance, effectiveness, and scalability of neural network calculations as opposed to general-purpose computing platforms like CPUs or GPUs are all goals of these hardware solutions. There are various kinds of hardware accelerators frequently utilized for neural networks, as depicted in (Fig. 9). These hardware accelerators can be designed to optimize a variety of tasks, from training and inference to data processing and feature extraction. It has the potential to drastically reduce the computational cost of neural networks, making them more accessible and efficient. GPUs have been routinely utilized to accelerate neural networks. GPUs are excellent at parallel processing and can execute large-scale matrix operations, which are essential for neural network computations.94–97 GPUs were initially created for producing visuals. GPU support in deep learning frameworks like TensorFlow and PyTorch enables neural network models to make use of GPU acceleration. Reconfigurable integrated circuits, or FPGAs, can be made to do particular computations. It has the benefit of versatility, enabling hardware architects to create designs specifically suited to neural network algorithms. When properly optimized, FPGAs can deliver exceptional performance and energy efficiency for particular neural network models.98–101
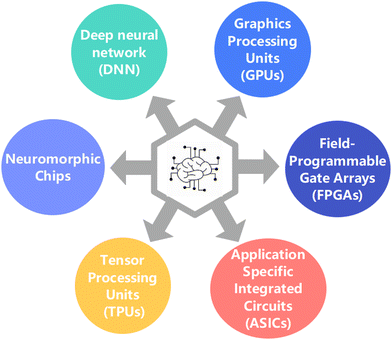 |
| Fig. 9 Types of hardware for acceleration of neural network devices. | |
ASICs are specially designed chips created for a specific use. By enhancing the hardware architecture for neural network operations, ASICs are created for neural network acceleration to offer great performance and energy efficiency. Although ASICs can improve performance noticeably, lack FPGAs' versatility. TPUs are specialized ASICs created by Google to accelerate neural network computations. TPUs are built to manage the demands of deep learning workloads and excel at executing matrix operations. This hardware is excellent for training and inference activities in neural networks being optimized for high throughput and energy efficiency.102,103 The architecture of the brain served as inspiration for the invention of neuromorphic chips, which mimic the actions of neurons and synapses.
These specialized processors imitate the massively parallel and energy-efficient processing found in the brain to speed up neural network computations. Neuromorphic chips, which are still in the research and development stage, have the potential to produce extremely effective and brain-inspired computing. The particular neural network model, performance needs, power considerations, and cost considerations are only a few of the variables that influence the choice of hardware accelerator. It is important to note that these accelerators are not mutually exclusive and that different parts of neural network computation, such as training and inference, can be optimized by combining them in a system.104–106 The design of the system is also important as it can affect performance, power consumption, and cost. It is important to consider the trade-offs between the different options before making a decision. Ultimately, the best hardware accelerator for a given task will depend on the specific neural network model and the desired performance.107 The performance metrics and applicability of different models, applications, and hardware generations can exhibit variability. According to the data shown in Table 2, a comparative analysis is conducted. The appropriateness of each accelerator is contingent upon various criteria, including the architecture of the neural network, the size of the model, the distinction between training and inference, and the specific environment in which the deployment takes place.108 Furthermore, the hardware environment is constantly changing, as newer iterations of accelerators emerge, providing enhanced levels of performance and efficiency. When making specific deployment decisions, it is crucial to evaluate these criteria in conjunction with the hardware features to ascertain the most appropriate accelerator for a certain application.109
Table 2 Comparative analysis of hardware accelerator
Hardware accelerator |
Performance metrics |
Energy efficiency |
Ideal application scenarios |
GPU |
High throughput |
Moderate to high |
Deep learning training and inference110 |
Parallel processing |
Varied based on load |
Complex, large-scale neural networks111 |
Wide ecosystem, mature support |
Suitable for data centers |
General-purpose deep learning tasks112 |
FPGA |
Low-latency, reprogrammable |
High |
Customizable neural networks113 |
Efficient for specific tasks |
Varied based on design |
Edge devices, IoT, real-time processing114 |
High hardware flexibility |
Energy-efficient custom designs |
Prototyping and research115 |
ASIC |
Extremely efficient |
Very high |
Specific, well-defined tasks116 |
High throughput |
Typically, fixed design |
Inference acceleration, dedicated AI chips117 |
Minimal power consumption |
|
Consumer electronics, embedded systems118 |
TPU |
High throughput |
Very high |
Large-scale deep learning inference119 |
Customized for neural networks |
Energy-efficient data centers |
Google cloud AI, tensor flow applications120 |
Neuromorphic chip |
Low power consumption |
Extremely high |
Brain-inspired computing, spiking networks121 |
Event-driven processing |
Ultra-low |
Neuromorphic research, cognitive computing122 |
In addition, seeking information from up-to-date documentation and analysing benchmarking results provided by hardware makers can offer a more accurate and detailed understanding of the present status of these technologies.
3.2. Design and optimization methodologies for neural network
Numerous approaches are used in the design and optimization of neural networks with the goal of enhancing their functionality, effectiveness, and generalization potential. Here are a few typical methods, as seen in (Fig. 10). Each of these approaches is intended to improve the performance of the neural network in some way.
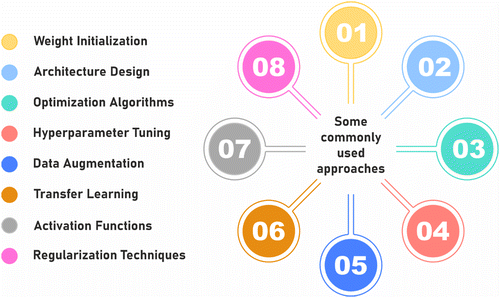 |
| Fig. 10 Commonly used approaches for optimization. | |
The number and layout of layers, the different types of neurons, and the connectivity patterns all fall under the category of a neural network's architecture. Convolutional, recurrent, or attention layers, as well as the number of layers and hyperparameters like the number of neurons or filters, must be chosen in order to design an effective architecture. To improve the architecture design, strategies like transfer learning and network pruning can be used. For effective learning, a neural network's weights must be initialized. The initial weights can be set using a variety of techniques, including random initialization. Faster convergence during training is encouraged by proper weight initialization, which helps prevent problems like vanishing or exploding gradients.123–125 The neural network models are given non-linearities via activation functions, which enables them to learn intricate patterns. Sigmoid, tanh, ReLU (rectified linear unit), and its derivatives such as Leaky ReLU and ELU (exponential linear unit), are examples of common activation functions. The ability of the network to model complicated relationships and steer clear of problems like the “vanishing gradient” problem can be impacted by selecting the proper activation function. Regularization techniques help neural networks become more generic by preventing overfitting. Techniques like dropout, batch normalization, L1 and L2 regularization (weight decay), and others can be used. Regularization enhances performance on unobserved data, reduces noise during training, and controls model complexity. During training, optimization methods are crucial in updating the weights of the network. While Adam, RMSprop, and AdaGrad offer advances in convergence time and managing complex loss landscapes, Stochastic Gradient Descent (SGD) is a widely used technique. During the optimization process, these algorithms strike a balance between the exploration and exploitation trade-offs. Different hyperparameters in neural networks, such as learning rate, batch size, and regularization strength, have a big impact on how well they perform.126–128 To obtain the optimum performance, hyperparameter tuning entails systematically looking for the finest possible combination of hyperparameters. To efficiently scour the hyperparameter space, methods like grid search, random search, or Bayesian optimization can be applied.
By performing random data transformations like rotations, translations, or flips to the input data, data augmentation techniques improve the amount and diversity of the training dataset. By exposing the neural network to a larger variety of variations and lowering the likelihood of overfitting, data augmentation aids in the neural network's ability to generalize.130–132 Transfer learning bootstraps the training of new models on smaller or related datasets by using pre-trained models on big datasets. Transfer learning can drastically save training time and boost neural network performance by transferring knowledge from the pre-trained model, especially when training data is few. To produce predictions, model ensembling mixes many neural network models. By utilizing various models and their complementing capabilities, it aids in increasing the robustness and generalization of the predictions. Prediction averages, model stacking, or the use of bagging and boosting are other assembling strategies. In a neural network, quantization reduces the precision of the weights and activations, which results in less memory consumption and faster computations. Pruning strategies find and eliminate unused links or neurons in a network, shrinking the size of the model and speeding up inference without significantly sacrificing performance. These approaches can be mixed and customized according to the particular needs and limitations of the neural network application because they are not mutually exclusive.133–135 The optimum design and optimization procedures for a particular neural network task are usually determined through empirical evaluation, iteration, and experimentation. This allows for a wide variety of neural network designs and architectures, enabling developers to choose the best approach for their particular application. Different activation functions can also be used to improve the performance of the network and to ensure that the network can learn complex patterns.136 Finally, regularization methods can be used to reduce overfitting and improve generalization. The electrical transmission of a neuronal impulse and the release of neurotransmitters into the synaptic cleft are depicted in (Fig. 11).
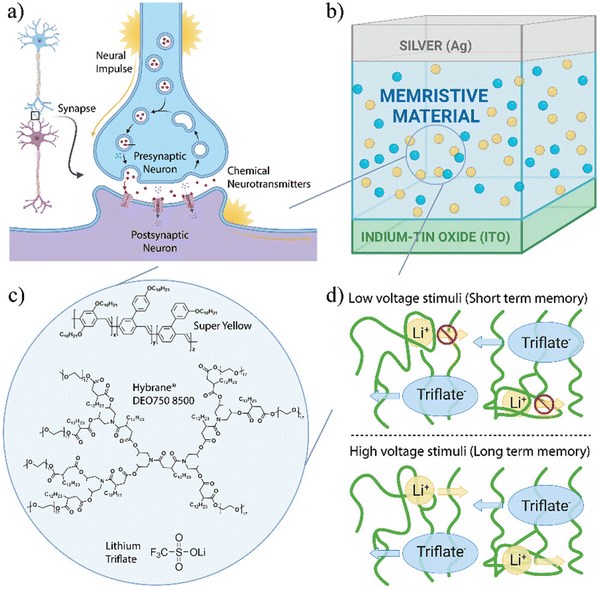 |
| Fig. 11 Release of neurotransmitters into the synaptic cleft between the presynaptic and postsynaptic neurons during the electrical transmission of a neural impulse.129 | |
The presynaptic neuron, which transmits the electrical signal or impulse, is shown first in the diagram. It is made up of a cell body, dendrites (which take input from neighboring neurons), and axons (which send output to neighboring neurons). Having the ability to act when an input signal or stimulus hits a threshold, the presynaptic neuron produces an action potential, a quick change in electrical potential.129 This electrical signal moves toward the synaptic terminal along the axon. The synaptic terminal, also known as the axon terminal, is a specialized structure that lies at the end of the axon of the presynaptic neuron. Neurotransmitter-filled synaptic vesicles can be found in this terminal. A sequence of events is started when the action potential reaches the synaptic terminal. Neurotransmitters are released into the synaptic cleft as a result of these events, which cause synaptic vesicles and the presynaptic membrane to fuse. The presynaptic and postsynaptic neurons are separated by a little region called the synaptic cleft. It divides the two neurons and blocks their direct electrical communication. Small dots in the diagram indicate the released neurotransmitters as they diffuse over the synaptic cleft. It travels from one side of the synaptic membrane to the other. The released neurotransmitters can bind to particular receptors on the postsynaptic neuron's membrane. These receptors are made to identify and respond to particular neurotransmitter molecules.137–139 When neurotransmitters attach to postsynaptic receptors, the postsynaptic neuron undergoes several chemical and electrical changes. The postsynaptic neuron can be excited or inhibited by this activation, depending on the neurotransmitter and receptor types. A postsynaptic potential is produced when neurotransmitters bind to postsynaptic receptors. The postsynaptic neuron may either depolarize (excitatory) or hyperpolarize (inhibitory) in response to this potential. The postsynaptic neuron will produce its electrical signal if the postsynaptic potential meets the threshold for an action potential. The neuronal impulse will then continue to be sent as this signal travels through the postsynaptic neuron's axon. It is crucial to highlight that the details of neurotransmitter release, receptor binding, and signal transmission are not included in this simplified representation of synaptic transmission.140–142 Even so, it offers a broad grasp of electrical impulse transmission and highlights the critical function neurotransmitters play in synaptic communication. Without neurotransmitter release, synaptic transmission would not occur. The role of neurotransmitters in the communication between neurons cannot be overstated. Thus, neurotransmitters are essential for efficient synaptic transmission of electrical impulses. These neurotransmitters are released into the synaptic cleft, which then binds to neurotransmitter receptors on the postsynaptic neuron, allowing the electrical impulse to be transmitted. Without neurotransmitters, the transmission of electrical impulses cannot take place.143,144
3.3. Synaptic transmission and the functioning of brain networks
Changes in the levels of neurotransmitters or the sensitivity of receptors can have a substantial effect on the effectiveness of synaptic communication. Consequently, this can have significant consequences for the general operation of brain networks. The impact of alterations in neurotransmitter dynamics on synaptic transmission and neural network function is illustrated in (Fig. 12).145 These changes can lead to changes in behavior, as well as malfunctions in cognitive and affective processes. Additionally, these changes can lead to the development of neurological disorders, such as anxiety and depression.146
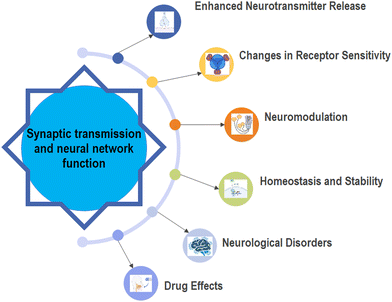 |
| Fig. 12 Pertains to synaptic transmission and the functioning of brain networks. | |
Enhanced neurotransmitter release refers to the phenomenon when there is an increase in the concentration of neurotransmitters within the synaptic vesicles or an enhancement of the processes responsible for their release. This can result in the amplification of synaptic signals, leading to better neuronal communication. This phenomenon may yield a higher degree of efficacy in the transmission of information among neurons, thereby fostering improved network connection and facilitating the process of learning.147 On the other hand, a reduction in neurotransmitter release can lead to a weakening of synaptic transmission. This phenomenon can weaken the synaptic connections between neurons, which could decrease the overall functionality of neural networks, difficulties in the process of learning, or hindered transmission of signals.148 Modifications in the sensitivity of post-synaptic receptors can yield substantial consequences. Enhanced receptor sensitivity has the potential to induce heightened neurotransmitter responses, hence optimising synaptic transmission efficiency. A reduction in sensitivity might result in a diminished reaction, compromising the efficiency of transmission. Synaptic plasticity refers to the capacity of synapses to undergo long-term potentiation or long-term depression, which involves alterations in neurotransmitter concentrations and receptor sensitivities.149 These changes play a crucial role in the strengthening or weakening of synapses over time. The aforementioned processes are fundamental to the acquisition of knowledge and the establishment of memory, hence playing a crucial role in the adaptive functioning of neural networks. Neuromodulation refers to the process by which certain neurotransmitters function as neuromodulators, exerting their influence on synaptic connections within a wider network, hence altering their strength. Changes in the amounts of neuromodulators or the sensitivities of receptors can impact the neural network's general functioning, hence altering many cognitive processes such as attention, arousal, and mood.150 The maintenance of homeostasis and stability in neuronal systems involves the regulation of neurotransmitter concentrations and receptor sensitivities by neurons and neural networks, which serves to stabilise the overall activity of the network. The dysregulation of these processes has the potential to result in various disorders, such as epilepsy, in which an overabundance of excitation impairs the stability of neural networks. Neurological and mental illnesses often exhibit changes in neurotransmitter systems.151 For instance, dysregulation of dopamine levels has been linked to the manifestation of neurological disorders such as Parkinson's disease and schizophrenia. These illnesses frequently present themselves as disturbances in the functionality and behaviour of networks. Pharmaceutical substances that specifically interact with neurotransmitter systems, such as antidepressants or anxiolytics, have the potential to influence the process of synaptic transmission and the overall functionality of neural networks.152 The therapeutic actions of medications are mostly attributed to their impact on neurotransmitter concentrations and receptor sensitivity. In brief, modifications in the levels of neurotransmitters and the sensitivities of receptors are pivotal factors in influencing the efficacy and adaptability of synaptic communication. The alterations described profoundly impact neural networks' operational capabilities, exerting influence on critical functions such as learning, memory, behaviour, and the progression of neurological and psychiatric disorders.153 Comprehending the complicated dynamics involved in synaptic transmission is of paramount importance in elucidating the intricacies of brain functionality and malfunctions. New insights into synaptic transmission can lead to the development of more effective treatments for neurological disorders and an improved understanding of brain processes. This knowledge can be used to develop new treatments for mental health disorders and to advance our understanding of neurological diseases.154
3.4. Architectural implications for memory technologies
An essential component of neural networks' effective operation is memory technology. For neural network systems, the memory technology chosen can have a big architectural impact. Here are a few important things to keep in mind, as indicated in (Fig. 13).
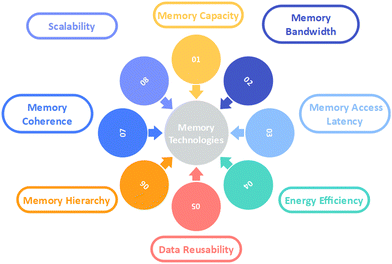 |
| Fig. 13 Some types of memory technologies. | |
To store model parameters, intermediate activations, and training data, neural networks frequently need a lot of memory. The memory technology ought to have enough capacity to satisfy the network's memory needs. A variety of memory technologies, from on-chip caches to off-chip memory modules or even distributed memory systems, offer varied capacities. In neural networks, there is a lot of data transfer between the memory and the processing units. The rate at which information can be read from or written to memory is referred to as memory bandwidth. To swiftly feed data to the processor units and avoid memory bottlenecks that could impair overall performance, high memory bandwidth is essential.155–158 The memory technology should offer sufficient bandwidth to satisfy the neural network's computing needs. The amount of time it takes to read or write data from memory is known as memory access latency. Frequent memory accesses in neural networks can cause noticeable delays and affect overall performance. On-chip caches and high-speed memory interfaces are two memory technologies with low access latency that can assist in reducing this latency and provide quicker data access.
Energy usage is a major concern when it comes to neural networks because they frequently demand large-scale memory operations. Low-power SRAM (static random access memory) and developing non-volatile memory technologies are two examples of memory technologies that have excellent energy efficiency and can assist neural network architectures using less power. Data reuse patterns in neural networks show that the same data is accessed repeatedly during various phases of computation. Cache hierarchies or scratchpad memories are examples of memory systems that facilitate effective data reuse and can lessen the frequency of memory accesses while enhancing speed. Memory hierarchies, which offer various layers of memory with variable capacities, bandwidths, and latencies, can be advantageous for neural networks. Neural networks can optimize the trade-off between capacity, bandwidth, and latency by using a hierarchy of memory technologies, including on-chip caches, high-bandwidth memory, and bigger off-chip memory.159 Memory coherence refers to providing consistency and synchronization between memory copies across various processing units or nodes in multi-node or distributed neural network systems. Data integrity in such architectures must be maintained via memory technologies that support effective memory coherence techniques, such as distributed memory systems or coherence protocols. Memory systems must facilitate scalability as neural networks get bigger and more complicated. Scalable memory technologies make it simple to increase memory space and bandwidth in order to support larger models or datasets. Technologies like memory interconnects, distributed memory systems, or memory modules with extensible capacities can help to accomplish this scalability. These considerations must be carefully taken into account when choosing memory technology for neural network topologies to strike a balance between performance, energy efficiency, and scalability. It frequently calls for a trade-off analysis based on the precise needs, limitations, and accessible technological solutions. Additionally, choices in architectural design for neural network systems are still influenced by current developments in memory technologies.160–162 This is why developers need to stay up to date with current trends and advancements in-memory technology. Furthermore, it is essential to have an in-depth knowledge of the trade-offs involved when selecting memory solutions to ensure the best performance and scalability of the neural network.
3.5. Memory bandwidth in neural networks and the frequent data transfer
The importance of memory technology advancements cannot be overstated when it comes to tackling the efficiency issues related to data transfer in neural networks. Furthermore, these advancements can have substantial architectural consequences for upcoming artificial intelligence systems.163 The influence of advances in memory capacity on neural network topologies and efficiency is seen in Fig. 14. Memory advancements allow neural networks to process more data, leading to higher accuracy rates. In addition, memory advancements can lead to more scalable AI systems, allowing for greater performance.164 Finally, memory advancements can help reduce the latency and power consumption of AI systems, making them more efficient. The concept of higher memory bandwidth refers to the increased rate at which data may be sent between the computer's memory and other components, such as the processor. Potential advancements in-memory technologies in the future could potentially yield increased memory bandwidth, hence facilitating accelerated data transfers between memory and computing units. This phenomenon can lead to a decrease in data transfer bottlenecks and expedite the process of training and inference in neural networks.165
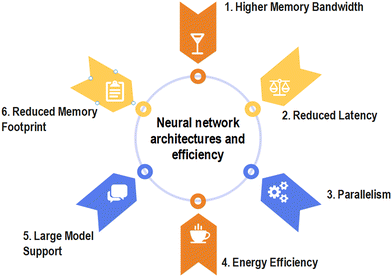 |
| Fig. 14 Bandwidth affects the architecture and performance of neural networks. | |
The concept of reduced latency refers to the decrease in the amount of time it takes for data to travel from its source to its destination advancements in memory technologies have the potential to reduce memory access latency, hence facilitating expedited data retrieval. This holds significant importance in real-time applications and when working with extensive neural network models. Parallelism is a rhetorical device that involves the repetition of grammatical structures, and words.166 The augmentation of memory bandwidth has the potential to enhance the level of parallelism in neural network topologies. Models that possess a greater number of parallel processing units can effectively process data, resulting in accelerated training and inference processes. The concept of energy efficiency refers to the ability to achieve a certain level of energy output while minimising the amount of energy input. The development of memory technologies that exhibit increased bandwidth while concurrently minimising power usage has the potential to enhance the energy efficiency of neural network hardware.167 The significance of this is paramount for mobile and edge devices that possess constrained power allocations. The concept of large model support refers to the implementation of techniques and strategies to address the challenges associated with training and deploying large-scale machine learning models. The progress in-memory technology can facilitate the utilisation of more extensive neural network models, resulting in enhanced memory capabilities. Larger models frequently exhibit superior performance, albeit necessitating increased memory bandwidth to sustain optimal efficiency.168 Advancements in memory technologies have the potential to result in decreased memory footprints for neural network models. The consideration of memory restrictions is crucial in applications that are deployed on edge devices. The concept of in-memory processing refers to the practice of doing data processing tasks directly within the computer's main memory, as opposed to the barrier between memory and processing units can be blurred by emerging memory technologies, such as resistive RAM (RRAM) and processor-in-memory (PIM) architectures.169 This has the potential to significantly improve data transfer efficiency by reducing the necessity of data movement between these units. Potential advancements in-memory technology in the future could potentially incorporate enhancements and refinements specifically tailored to neural network workloads, hence enabling the utilisation of more streamlined and effective data access patterns.170 This has the potential to enhance the utilisation of memory bandwidth. The concept of heterogeneous memory architectures refers to the use of diverse types of memory inside a computing system. The potential emergence of advanced memory technologies could potentially pave the way for the creation of heterogeneous memory architectures, wherein several forms of memory, such as high-bandwidth memory and non-volatile memory, are seamlessly integrated inside a unified system. This has the potential to provide a harmonious equilibrium between a substantial data transfer rate and the ability to accommodate a large volume of information.171 Neuromorphic computing refers to a branch of computer science that aims to develop computer systems and architectures inspired by structure and functionality. The utilisation of in-memory technologies has the potential to facilitate the advancement of neuromorphic computing systems, which aim to replicate the intricate memory and computation relationships observed in the human brain. These systems have the potential to facilitate the development of AI architectures that are both extremely efficient and inspired by the functioning of the human brain.172 AI-specific memory solutions: an exploration of memory technologies tailored for artificial intelligence applications. Memory makers have the potential to develop customised solutions that are specifically designed to meet the requirements of artificial intelligence (AI) and neural network workloads. These methods have the potential to enhance memory bandwidth and optimise access patterns for artificial intelligence (AI) activities.173 The progress made in memory technology holds the potential to effectively tackle the issues associated with data transmission efficiency in neural networks. These advancements have the potential to enhance the efficiency, speed, and energy efficiency of AI hardware architectures, hence facilitating the implementation of larger and more proficient neural network models.174 The significance of these innovations cannot be overstated, given the ongoing expansion of AI applications across diverse sectors such as autonomous vehicles, healthcare, and beyond.
4. Machine learning algorithms
Machine learning algorithms that mimic the structure and operation of the human brain are known as neuromorphic algorithms. They are necessary to carry out tasks like pattern recognition, decision-making, and learning. These algorithms create effective and scalable solutions for machine learning issues by utilizing the concepts of neuroscience and computational models of neural networks. As demonstrated by neuromorphic machine learning algorithms in (Fig. 15), these algorithms have the potential to revolutionize the field of artificial intelligence and enable machines to do complex tasks.175–177
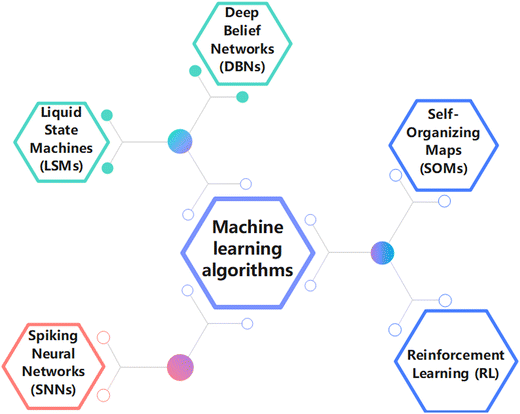 |
| Fig. 15 Neuromorphic machine learning algorithms. | |
Artificial neural networks known as “spiking neural networks” (SNNs) process information primarily using discrete-time spikes. The timing and intensity of neuronal activity are represented by spikes, means by which neurons in SNNs exchange information with one another. Modeling temporal dynamics and asynchronous processing benefit greatly from SNNs.178 Recurrent neural networks called liquid state machines (LSMs) are influenced by the dynamics of biological neural networks. It is made up of a sizable group of randomly connected neurons, or “liquid,” which gives the network a vibrant and dynamic input space. LSMs have been applied to a variety of tasks, including time-series prediction, robot control, and voice recognition.179 The capability of deep neural networks and probabilistic graphical models are combined in hierarchical generative models called deep belief networks (DBNs). These are made up of several interconnected layers of neurons, where the upper layers record increasingly abstract representations and the lower layers record low-level characteristics. DBNs can be developed using supervised learning after being trained using unsupervised learning techniques like restricted Boltzmann machines (RBMs).180 Unsupervised learning methods called self-organizing maps (SOMs) organize data based on similarity and topology. They map the high-dimensional input data onto a grid of neurons to produce a low-dimensional representation of it. SOMs have been applied to feature extraction, visualization, and clustering. Although not entirely, neuromorphic principles can be used to implement reinforcement learning (RL) algorithms.181–183
With the help of interaction with the environment and learning to base decisions on rewards or penalties, RL is a paradigm for learning. Neuromorphic RL algorithms try to imitate the adaptability and learning processes of living things. These are but a few illustrations of neuromorphic machine-learning techniques. To enhance the capabilities of artificial intelligence systems, researchers are exploring novel models and methodologies that are inspired by the brain. These models and methodologies are being used to provide AI systems with more efficient problem-solving and decision-making capabilities. This could lead to more powerful AI systems that can learn from their environment and make decisions more quickly and accurately.184–186
A popular strategy in neuromorphic systems, especially in spiking neural networks (SNNs), is to encode inputs in terms of spike rates, latency, and neuron population. Here is a brief description of the signal encoding applications of these parameters.187 The frequency or rate of spikes released by neurons over a predetermined length of time is used in this encoding strategy to represent the information. A certain feature or signal component may be more strongly present when the spike rate is higher than when it is lower, indicating either its absence or a weaker signal component. SNNs may encode a range of signal intensities or levels by adjusting the neurons' spike rates. The term “latency encoding” describes the representation of data using the precise timing or temporal pattern of spikes.188–190 The intervals between spikes contain information on the temporal organization of the input or the relative timing of events. The SNN may be made to record temporal relationships and synchronize with time-varying data because neurons can be made to respond to particular temporal patterns. Distributing a signal's representation among several neurons is a necessary step in the encoding of information in terms of the neuron population. By firing spikes in response to particular characteristics or components of the signal, each neuron in the population contributes to the overall encoding.191–193 The SNN can simultaneously encode many aspects or dimensions of the input signal by altering the activity of various neurons within the population. To provide richer representations of information in neuromorphic systems, several encoding strategies are frequently coupled. For instance, depending on the strength of a signal, a neuron population's spike rate may change, and the precise timing of spikes within that population might reveal further details about the temporal structure. In neuromorphic systems, decoding techniques are employed to extract the encoded data from spike trains.194 These algorithms decode the encoded signal and carry out operations like pattern recognition, classification, or control by analyzing the spike rates, temporal patterns, and population activity. It is crucial to keep in mind that the precise encoding and decoding techniques can change based on the application and design decisions made in a specific neuromorphic system. Different signal kinds or computing tasks may respond better to various encoding strategies.195–197 Neuromorphic computing also requires a careful consideration of the degree of the complexity of the encoding scheme, as well as the hardware resources available for implementing the decoding operations. Ultimately, the choice of encoding and decoding techniques should be tailored to the specific computing task and the available hardware resources.
5. Non-machine learning algorithms
Neuromorphic computers can be used for a variety of non-machine learning algorithms in addition to machine learning algorithms. Beyond machine learning, other computer activities might benefit from the behavior and principles of biological neural networks, which is the goal of neuromorphic computing. A few instances of non-machine learning algorithms are displayed in (Fig. 16). These algorithms, such as neural networks, incorporate biological principles of neurons and synapses to enable computers to process data more efficiently. Neuromorphic computing can also be used to identify complex patterns in data or to detect anomalies in datasets.198–200 Signal processing tasks including audio and video processing, image and voice recognition, and sensor data analysis can be performed using neuromorphic computers. By utilizing the computational ideas of neural networks, neuromorphic systems' parallel and distributed architectures can enable effective and immediate signal processing. Combinatorial optimization, resource allocation, and scheduling are a few examples of optimization issues that can be solved with neuromorphic computing. Neuromorphic systems' capacity to explore huge solution areas concurrently may have advantages in the more effective solution of challenging optimization issues. In robotics and control systems, neuromorphic computers can be utilized for motor control, sensor integration, and decision-making activities.
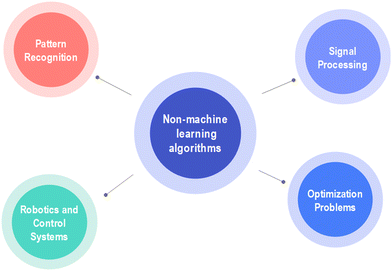 |
| Fig. 16 Neuromorphic computers for non-machine learning algorithms. | |
Spiking neural networks' event-driven design may be useful for the real-time control and feedback loops needed in robotics applications. Neuromorphic computers can be used for pattern recognition tasks in a variety of fields, such as bioinformatics, pattern matching, and anomaly detection, in addition to machine learning methods. Recognizing complicated patterns and spotting abnormalities might benefit from the capacity to record temporal dynamics and analyse data in parallel. Biological systems, such as the brain, can be studied further using neuromorphic computers.201–203 Researchers can investigate computer representations of neural processes and acquire insights into how biological neurons and networks function. It is vital to understand that while neuromorphic computers can be used for non-machine learning algorithms, the brain and neural networks serve as the primary sources of inspiration for their architecture and design. As a result, the effectiveness of these systems and their applicability for particular non-machine learning activities may vary depending on the nature of the given case and on the form in which the neuromorphic system is being implemented. Therefore, it is essential to thoroughly evaluate the architecture and design of a neuromorphic system before attempting to utilize it for a specific task. Additionally, the parameters of the system should be tuned to the particular problem to ensure optimal performance.
6. Different encoding strategies in neuromorphic systems
The utilisation of various encoding procedures holds significant importance in the representation of information inside neuromorphic systems. Neuromorphic systems endeavour to replicate the cognitive processes of the brain and frequently use techniques such as spike rates, latency, and neuron population to encode and convey information.204 The impact of each of these tactics on information representation is demonstrated in Fig. 17.
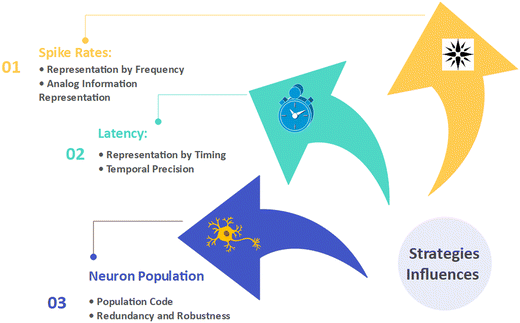 |
| Fig. 17 Neuromorphic devices the information-processing strategies influences. | |
Spike rate coding is predicated on the temporal frequency of neuronal spikes. Elevated spike rates may indicate the existence or intensity of a particular characteristic or signal. For instance, an elevated frequency of neuronal firing in response to a sensory stimulus may suggest heightened intensity or significance. Spike rate coding is a method of representing continuous variables analogously, rendering it well-suited for jobs that necessitate accurate analogue information representation. Temporal patterns refer to the fluctuations in spike rates observed over a period.205 These variations play a crucial role in encoding temporal patterns and sequences of events, hence facilitating the processing of dynamic information. The concept of latency coding involves the representation of information through the timing of neuronal spikes about a particular event or stimulus. The encoding of information is achieved through the precise timing of spike onset.206
Temporal precision refers to the ability of a coding system to accurately represent time-sensitive information and capture subtle temporal correlations between occurrences. Latency coding is known for its capacity to achieve high temporal precision. The phenomenon of synchronisation in neuronal activity is characterised by the occurrence of synchronised spikes across several neurons.207 This synchronisation can serve as an indicator of the presence of specific features or the occurrence of coordinated events within the neural network. The concept of neuron population refers to a group of interconnected neurons inside a biological system. The concept of population coding refers to the encoding of information by the combined activity of a group of neurons, as opposed to the activity of individual neurons. The scattered configuration of neuronal activations serves as a representation of information.208 The utilisation of population coding in neural systems frequently confers a greater degree of robustness to noise and fluctuations due to the redundancy of information across several neurons. The presence of redundancy within a population can contribute to the preservation of information integrity. The phenomenon of diversity is observed in populations of neurons, wherein individual neurons exhibit varying degrees of sensitivity for specific traits. This characteristic enables the encoding of intricate and multi-faceted information.209 Every encoding approach possesses distinct advantages and is tailored to forms of information representation. Spike rate coding is frequently employed in the context of continuous, analogue information and dynamic patterns. The utilisation of this technology has demonstrated utility in various domains, such as sensory processing and motor control. Latency coding demonstrates exceptional performance in tasks that necessitate accurate timing information, such as sound localisation or temporal sequence identification.210 The concept of neuron population coding exhibits a high degree of versatility, enabling it to effectively encode and represent diverse types of information. It is frequently employed in cognitive activities and intricate pattern recognition. In practical applications, neuromorphic systems can integrate many encoding schemes, which are selected based on the specific job and network architecture at hand.211 The selection of an encoding approach is contingent upon the particular demands of the application as well as the underlying biological principles that inform the development of the neuromorphic system. In general, the ability to utilise many encoding schemes enables neuromorphic systems to effectively depict and manipulate information in manners that closely resemble the intricate and adaptable nature of the human brain.
7. Electrochemical-memristor-based artificial neurons and synapses
Electrochemical memristors are of paramount importance in the advancement of artificial neurons and synapses, as they replicate the functionality exhibited by biological systems. These devices have garnered considerable interest in the field of neuromorphic engineering and play a crucial role in the development of brain-inspired computer systems.212 The following discourse presents a comprehensive overview of the core principles underlying electrochemical memristor-based artificial neurons and synapses.
7.1. Memristor basics
(a) A memristor, sometimes known as a “memory resistor,” is an electrical device with two terminals that demonstrates a non-linear correlation between the voltage applied across its terminals and the current passing through it. The memristor is a type of non-volatile memory that can be used to store data without the need for power. It is also capable of learning, allowing it to adapt to changing conditions.213 Memristors are potentially useful for many applications, including data storage, neural networks, and robotics.
(b) The distinctive characteristic of memristors is their ability to modify their resistance based on the past patterns of applied voltage or current. This characteristic enables the neural networks to retain previous states, rendering them well-suited for modeling synaptic behaviour. Memristors are also energy-efficient and capable of functioning at low temperatures.214
7.2. Neuromorphic computing
Neuromorphic computing is a paradigm in the field of artificial intelligence that seeks to replicate the intricate architecture and operational principles of the human brain. The objective of this endeavour is to construct hardware and software systems that draw inspiration from the neural networks present in the human brain. Such systems are intended to solve complex problems with greater accuracy and speed than conventional computers.215
7.3. Artificial neurons
(a) Neuromorphic systems aim to emulate the functionality of organic neurons through the construction of artificial neurons. These systems accept input signals, engage in computational processes, and produce output signals.216
(b) The utilisation of memristors in the representation of synaptic connections among artificial neurons enables the emulation of synaptic plasticity and the process of learning.217
7.4. Synaptic plasticity
(a) Synaptic plasticity pertains to the capacity of synapses, which are the interconnections between neurons, to undergo modifications in strength or weakening as a consequence of past patterns of brain activity. The process in question is a basic aspect that underlies the acquisition of knowledge and the retention of information inside biological neural systems.218
(b) Electrochemical memristors have a high degree of suitability for modeling synaptic plasticity due to their ability to replicate alterations in synaptic strength through the manipulation of their resistance.219
7.5. Learning and memory
(a) Synaptic plasticity in memristor-based synapses encompasses diverse manifestations, including long-term potentiation (LTP) and long-term depression (LTD), which are akin to the mechanisms observed in biological synapses during the processes of learning and memory formation.220
(b) The synaptic connections can acquire knowledge from input patterns and adjust their efficacy, hence facilitating the utilisation of unsupervised learning methods such as spike-timing-dependent plasticity (STDP). This plasticity allows neurons to learn and adapt to their environment, enabling them to form new connections and modify existing ones. This process is known as synaptic plasticity and is essential for the brain's ability to learn and store information.221
Memristor-based circuits, commonly known as neuromorphic circuits or memristive crossbars, are engineered to emulate the neuronal connection observed in biological brains. The circuits possess the capability to do information processing in a manner that deviates substantially from conventional von Neumann computing, hence facilitating energy-efficient and parallel processing. In essence, electrochemical memristors play a crucial role in the development of synthetic neurons and synapses for the field of neuromorphic computing.222 These systems facilitate the replication of synaptic plasticity and learning mechanisms observed in biological neural networks, presenting the possibility of effective computing methods inspired by the human brain. Scientists are currently engaged in an active investigation of these devices to propel the area of neuromorphic engineering forward and develop artificial intelligence systems that closely resemble biological counterparts.223
7.6. Nanowire-based synaptic devices for neuromorphic computing
The exploration of nanowire-based synaptic devices is a highly promising area of investigation within the realm of neuromorphic computing. These devices utilise the distinctive characteristics of nanowires to imitate the functionality of biological synapses, hence facilitating the advancement of neural network hardware that is both energy-efficient and high-performing.224 The following discourse presents a comprehensive analysis of the fundamental elements and benefits associated with nanowire-based synaptic devices in the context of neuromorphic computing.
7.6.1. Nanowire structure.
Nanowires are constructions characterised by their exceptionally small widths, often on the nanometer scale, and can be composed of either semiconducting or metallic materials. The compact dimensions of these circuits facilitate optimal utilisation of space and enable a high level of packing density in neuromorphic systems.225
7.6.2. Memristive behavior.
Numerous nanowire materials demonstrate memristive characteristics, whereby their resistance can be modulated in reaction to the application of voltage or current. The aforementioned characteristic is of utmost importance in simulating synaptic plasticity, a phenomenon in which the efficacy of synapses, i.e., the connections between neurons, can be altered in response to brain activity.226
7.6.3. Synaptic plasticity.
Nanowire-based synaptic devices can mimic many types of synaptic plasticity, including long-term potentiation (LTP) and long-term depression (LTD). These kinds of synaptic plasticity play a crucial role in the learning and memory mechanisms observed in biological brains.227
7.6.4. Energy efficiency.
One notable benefit associated with synaptic devices based on nanowires is their notable reduction in energy consumption. Synaptic processes can be executed by these devices with minimum power consumption, rendering them well-suited for energy-efficient neuromorphic hardware, particularly in systems that rely on batteries and embedded technology.228
7.6.5. Parallel processing.
The utilisation of nanowire-based synapses facilitates the concurrent processing of information, a fundamental attribute of neuromorphic computing. The implementation of parallelism has the potential to greatly enhance the efficiency of both neural network training and inference activities.229
These devices can replicate spike-timing-dependent plasticity (STDP), which is a learning rule inspired by biological synapses. The phenomenon known as spike-timing-dependent plasticity (STDP) enables synapses to undergo either strengthening or weakening, contingent upon the precise temporal relationship between pre- and post-synaptic spikes. Several nanowire materials have been investigated for their potential use in synaptic devices, such as silicon, titanium dioxide (TiO2), and chalcogenide-based materials like germanium telluride (GeTe).230 Every material possesses distinct properties and behaviours that are well-suited for various neuromorphic applications. The integration of nanowire-based synaptic devices with complementary metal–oxide–semiconductor (CMOS) technology enables the development of hybrid neuromorphic circuits, thereby leveraging the advantages offered by both technologies.231 The utilisation of nanowire-based synaptic devices has demonstrated a notable level of consistency between devices, a characteristic of utmost significance in the construction of expansive neuromorphic systems. Furthermore, it is possible to reduce their size to nanoscale dimensions, hence facilitating the advancement of densely populated neural networks.232 Although nanowire-based synaptic devices have notable benefits for neuromorphic computing, there remain obstacles that must be addressed, including device variability and dependability. Academic researchers persist in investigating diverse materials, device architectures, and fabrication procedures to augment the performance and dependability of these devices for practical applications in neuromorphic systems.233
7.7. Triboelectric nanogenerator for neuromorphic electronics
Triboelectric nanogenerators (TENGs) belong to a category of energy harvesting devices that facilitate the conversion of mechanical energy, specifically motion or vibration, into electrical energy. This conversion is achieved by leveraging the principles of the triboelectric effect and electrostatic induction.234 TENGs have been predominantly employed for energy harvesting purposes; nevertheless, their distinctive attributes have also rendered them applicable in the field of neuromorphic electronics. This paper discusses the potential utilisation of triboelectric nanogenerators (TENGs) in the field of neuromorphic electronics:
7.7.1. Energy-efficient power source.
Tunnelling field-effect transistors (TENGs) have the potential to function as highly efficient energy sources for neuromorphic circuits. Electrical energy can be generated from a variety of sources, encompassing human motion, environmental vibrations, and mechanical sensors.235
7.7.2. Self-powered neuromorphic systems.
TENGs possess the capability to facilitate the operation of self-sustaining neuromorphic systems, thereby obviating the necessity for external power supplies or batteries. Wearable neuromorphic devices and sensors can greatly benefit from the utilisation of this technology.236
7.7.3. Low-power operation.
The energy-efficient behaviour of biological brain networks is widely emulated in neuromorphic circuits, which often necessitate low power consumption. Thermoelectric nanogenerators (TENGs) have the potential to serve as a viable and energy-efficient power source for such electronic circuits.237
7.7.4. Harvesting environmental energy.
TENGs possess the capability to extract energy from the surrounding environment, rendering them well-suited for implementation in distant and autonomous neuromorphic devices situated in areas where conventional power sources are either inaccessible or unfeasible.238
7.7.5. Energy storage integration.
TENGs can be integrated with energy storage systems, such as supercapacitors or batteries, to store the gathered energy. This stored energy can then be utilised in neuromorphic circuits during instances of limited energy availability.239
TENGs possess the capability to function as sensors for biomechanical movements as well. When incorporated into wearable devices, they can record motion and mechanical data, which can then be analysed by neuromorphic circuits for a range of purposes, including health monitoring and gesture recognition.240 TENG-based neuromorphic electronics possess the capability to be seamlessly included into human-machine interfaces, enabling the utilisation of energy derived from user activities for the purpose of device control or sensory feedback provision. TENGs can facilitate the adaptation of neuromorphic circuits in accordance with the energy resources at their disposal.241 In situations where energy resources are constrained, circuits can decrease complexity and give precedence to vital tasks, thereby emulating the energy-efficient characteristics observed in biological brains. TENGs play a significant role in promoting environmental sustainability through the utilisation of mechanical energy derived from natural sources.242 This application effectively reduces the need for non-renewable energy sources for the operation of neuromorphic devices. Although TENGs have numerous benefits for neuromorphic electronics, there exist certain obstacles that necessitate attention. These include the refinement of TENG designs, the development of energy management tactics, and the seamless integration of TENGs with neuromorphic hardware and software.243 Ongoing research in this field suggests that the utilisation of TENG-powered neuromorphic systems has promise for contributing significantly to the advancement of energy-efficient and self-sustainable intelligent gadgets.
7.8. Memristive synapses for brain-inspired computing
Memristive synapses are a pivotal element within brain-inspired computing systems, commonly denoted as neuromorphic computing. The purpose of these synapses is to replicate the functioning of biological synapses, hence enabling the simulation of synaptic plasticity and the learning mechanisms observed in biological brain networks.244 This paper provides a comprehensive description of memristive synapses in the context of brain-inspired computing:
7.8.1. Memristor basics.
Memristors are electronic devices characterised by a non-linear voltage–current relationship, and are classified as two-terminal passive components. These entities possess a distinctive characteristic whereby their resistance is altered in response to the record of applied voltage or current.245
7.8.2. Synaptic plasticity.
(a) Synaptic plasticity in biological brain networks pertains to the capacity of synapses to modify their strength in response to patterns of neural activity. The phenomenon of plasticity plays a crucial role in the facilitation of learning and memory processes.246
(b) The phenomenon of memristive synapses enables the replication of many types of synaptic plasticity, including long-term potentiation (LTP) and long-term depression (LTD), which play a crucial role in facilitating learning and memory processes within biological neural networks.247
7.8.3. Learning and adaptation.
The utilisation of memristive synapses facilitates the development of brain-inspired computing systems, allowing them to acquire knowledge from input data and dynamically adjust their synaptic strengths in response. The aforementioned skill holds significant importance in the context of unsupervised learning algorithms and tasks related to pattern recognition.248
7.8.4. Spike-timing-dependent plasticity (STDP).
Spike-timing-dependent plasticity (STDP) is a learning mechanism that is derived from observations made in biological synapses. The phenomenon of spike-timing-dependent plasticity (STDP) can be emulated by memristive synapses, wherein the relative timing of pre-and post-synaptic spikes plays a crucial role in determining whether the synaptic efficacy should be augmented or diminished.249
7.8.5. Energy efficiency.
Memristive synapses are recognised for their notable energy efficiency. Neuromorphic electronics may effectively carry out synaptic processes while consuming minimum power, rendering them well-suited for low-power and energy-efficient applications.250
Parallel processing capabilities are frequently necessary in brain-inspired computer systems to effectively model neural networks. The utilisation of memristive synapses facilitates parallelism by enabling the concurrent execution of synaptic operations throughout a network of synapses. The utilisation of memristive synapses enables the emulation of a broad spectrum of synaptic behaviours, hence facilitating the realisation of various neural network topologies and learning techniques.251 Memristive synapses have been included in neuromorphic hardware platforms, specifically memristive crossbars, to emulate the connection and functionality observed in biological neural networks. These hardware platforms facilitate the advancement of computing systems that are inspired by the structure and functioning of the human brain.252 The memristive synapses play a crucial role in facilitating brain-inspired computing by enabling the replication of synaptic plasticity and learning mechanisms within artificial neural networks. The promising attributes of energy efficiency, adaptability, and diversity make them a compelling technology for the development of neuromorphic hardware that can effectively execute intricate cognitive tasks while minimising power usage.253
8. Neuromorphic materials
Neuromorphic materials belong to a category of materials that demonstrate characteristics or behaviours that bear resemblance to those observed in biological neural systems. The aforementioned materials have garnered significant interest within the domain of neuromorphic engineering and brain-inspired computing, mostly due to their capacity to replicate specific facets of neural information processing.254 The Fig. 18 demonstrates memristors based on different materials and their promising applications. In terms of conductivity, stability, and controllability, each material offers particular qualities and advantages. Here is a quick summary of these resources. Memristor fabrication frequently makes use of metal–oxide materials like hafnium oxide (HfO2) and titanium dioxide (TiO2). These have resistance-switching characteristics, are scalable, and work with traditional silicon-based circuits.255–257
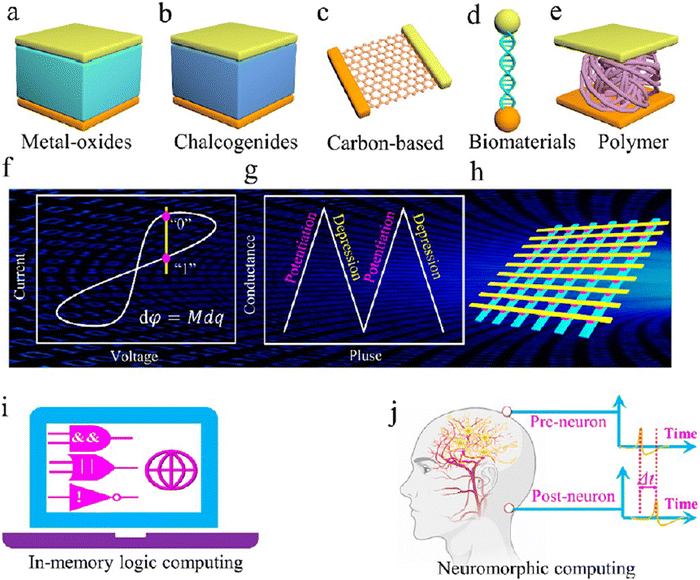 |
| Fig. 18 Schematic representation of the memristor with various materials, in (a)–(e). (f) Standard voltammetry test with the cyclic I–V characteristic. (g) Conductance evolution under pulse excitation (voltage or current), simulating the potentiation and depression of biological synapses. (h) Crossbar-structured integrated memristor device schematic diagram. (i) Diagram showing the in-memory logic computing system. (j) Diagram showing the neuromorphic computing system.255 | |
The phase-change characteristics of chalcogenides, such as germanium–antimony–tellurium (GeSbTe), are well known. Information may be stored and retained in these materials because they can flip between crystalline and amorphous states. It is frequently seen in non-volatile memory storage systems. Graphene and carbon nanotubes (CNTs) are examples of carbon-based materials with remarkable mechanical and electrical conductivity. The potential of graphene, a single layer of carbon atoms organized in a hexagonal lattice, in transparent and flexible memristor devices has been investigated. In the field of bioelectronics, natural biomaterials produced from biological sources, such as proteins, peptides, and DNA, have gained popularity.258–260 These materials can interact with biological systems, are biocompatible, and have tunable properties that can be used for neural interfaces and bio-inspired memristors. Flexible electronics, particularly memristors, utilize synthetic polymers like poly(3,4-ethylene dioxythiophene) (PEDOT) and polyvinylidene fluoride (PVDF). These materials have good mechanical flexibility, are easily processed, and work well with industrial manufacturing processes like coating and printing. It is crucial to remember that the material selection is based on the particular specifications of the gadget and the desired functionality.261–263 To improve the functionality, scalability, and integration of memristors and other electronic devices, researchers continue to investigate and develop new materials and hybrid combinations.
Through this research, scientists also hope to reduce production costs and improve the sustainability of such devices. Simultaneously, they investigate the possibility of integrating memristors with existing technology, to create novel electronic applications. A potent method that can be used to study the development and rupture of conductive metal filaments in memristors or other similar devices in real-time is the high-resolution transmission electron microscopy (HRTEM).264 HRTEM offers atomically detailed pictures of materials, enabling precise viewing and investigation of nanoscale events. HRTEM can shed light on the structural alterations and dynamics of the filament by viewing the development or rupture of conductive filaments in a memristor in real-time. As depicted in (Fig. 19), it was possible to witness the process. For HRTEM investigation, a thin cross-section of the memristor device with the conductive filament is created.
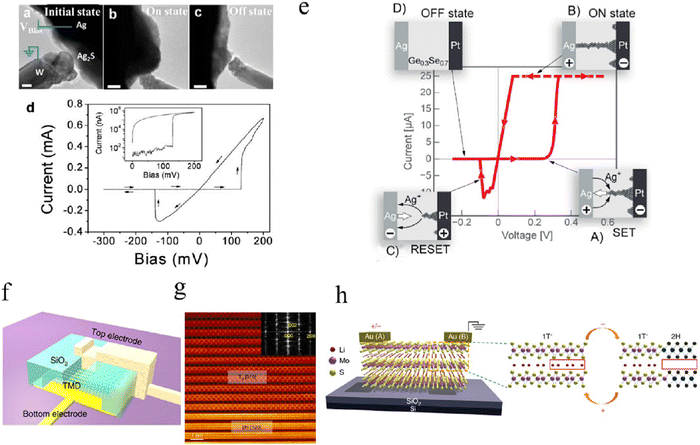 |
| Fig. 19 (a) TEM picture of an initial-state Ag2S-based memristor (a) Device's TEM picture taken at the on-state (c) TEM picture of the object in its off-state (d) Device's IV characteristics. The I–V curve is shown in the inset in a semilogarithmic scale (positive range). (e) Ag/Ag/Ge/Se/Pt device's typical I/V property and the related electrochemical metallization procedure. (f) Vertical MoTe2-based device's schematic diagram. (g) An atomic resolution image was taken using a scanning transmission electron microscope of the Td and 2H phases in a MoTe2 layer. Inset: The image is transformed using a rapid Fourier algorithm. Li+ migration-induced local 2H-1T′ phase transitions in LixMoS2 are shown schematically in (h).61,265–267 | |
To do this, the device is often thinly sectioned at the nanoscale using focused ion beam (FIB) techniques. The prepared sample is put into an HRTEM device, which illuminates the sample with a focused electron beam.60 The sample is impacted by the electron beam and the transmitted or scattered electrons that result are gathered to create a picture. Real-time monitoring of dynamic processes is possible thanks to the HRTEM instrument's ability to record images and films at a rapid frame rate. While being monitored, the conductive filament can be exposed to controlled electrical stimulation, allowing for the visualization of its creation or rupture. To comprehend the structural changes occurring in the conductive filament throughout the real-time procedure, the acquired HRTEM photos and videos can be examined. It is possible to monitor and investigate atomic-scale phenomena like the movement of individual atoms or the development of crystal formations.268 Researchers can learn important information about the dynamics and underlying mechanisms of memristor devices by utilizing HRTEM to observe the development and rupture of conductive filaments in real time. These details can help with the design and improvement of these components for memory, neuromorphic computing, and analog computing, among other uses. This information can also help identify the most reliable materials and processes to manufacture these devices. Additionally, the development of new memristor models can be accelerated by such real-time observations.269–272
As depicted on the protein conductive diagram (Fig. 20a), a conductive protein typically comprises an amino acid chain-like structure. There might be particular sections or domains that show conductivity within this structure.273 A schematic graphic would show how the protein chain is organized and would emphasize the conductive areas. It might display any redox-active groups or cofactors that are present, as well as the amino acid residues implicated in electron transfer. According to the conductive DNA pattern in (Fig. 20b), a modified DNA structure that includes conductive molecules is shown in a schematic diagram of conductive DNA. The functional groups or attachments along the DNA backbone would be represented by these conductive molecules, such as metal complexes or conjugated polymers.274Fig. 20 may show the locations of electron transport as well as the conductive modifications or moieties. A diagram of Li+ transport in phthalate starch-PVDF matrices is provided in (Fig. 20c). Lithium-ion migration can be schematically represented by a graphic of Li+ transport in a phthalate starch-PVDF matrix.275 It might display how the matrix material is organized, emphasizing the interconnected network or channels that Li+ ions can go through. The graphic could also show how different matrix elements affect the movement of Li+ ions, such as how PVDF or phthalate starch affects their diffusion or migration. The graphic should also show the different energy levels that Li+ ions need to reach to migrate through the matrix material. The properties of the Li+ ions, such as size, mass, and charge, should also be included in Fig. 20. The graphic should also include the effects of temperature, humidity, and other environmental factors on the movement of Li+ ions. Finally, the graphic should include the different types of materials used in Li-ion batteries, such as cathodes, anodes, electrolytes, and separators.
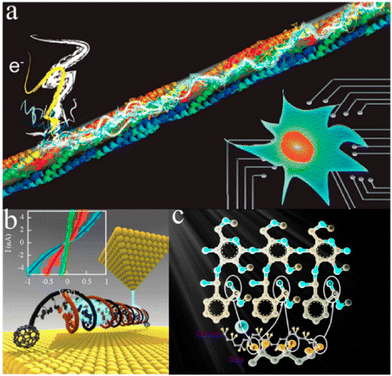 |
| Fig. 20 Diagrams of conductive proteins and DNA are shown in (a) and (b), respectively. Li+ transport in the phthalate starch/PVDF matrix is shown schematically in (c).273–275 | |
9. Challenges in neuromorphic processors between expectations and reality
A continuing issue in the industry is bridging the gap between expectations and reality in neuromorphic technology. Here are a few crucial areas where efforts are being undertaken to close this gap, as indicated in (Fig. 21). Scaling up neuromorphic hardware systems to solve bigger, more complicated issues is one of the main objectives of.276–278 The number of neurons and synapses that can currently be supported by the majority of neuromorphic hardware implementations is constrained. Scalable architectures and interconnectivity strategies are being developed by researchers to support larger networks and support more powerful calculations.
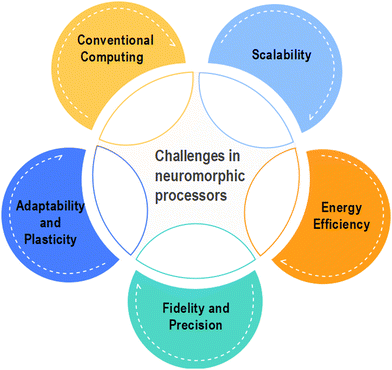 |
| Fig. 21 Neuromorphic processor challenges. | |
The goal of neuromorphic hardware is to replicate the brain's high level of energy efficiency. Even if improvements can still be made, progress can be observed. Energy usage continues to be a problem, particularly when expanding to larger networks. The energy efficiency of neuromorphic systems is being improved through the development of new circuit designs, low-power device technologies, and architectural optimizations.279–281 Another area that requires improvement is the attainment of high-fidelity and precision in neural computation. Even while existing neuromorphic hardware can mimic the essential functions of synapses and neurons, there are still disparities between them and biological systems in terms of behavior and reaction. Research is ongoing to improve the brain models and hardware circuitry's fidelity, accuracy, and precision. A fundamental feature of the brain is its capacity to adapt and learn from data in real-time. This plasticity is intended to be captured by neuromorphic technology, although there is potential for improvement. The goal of the study is to improve the adaptability, learning potential, and synaptic plasticity mechanisms in neuromorphic systems to make them more like the brain's learning and memory functions. For practical applications, it is crucial to close the gap between neuromorphic hardware and conventional computing systems.282–285 The usage of neuromorphic technology in practical applications can be facilitated by integration with already-existing computing architectures, software frameworks, and tools that can enable smooth collaboration between various computing paradigms. Although there are great expectations for neuromorphic hardware, it is important to keep in mind that the technology is still in its infancy and it may take some time before the full potential of these systems is realized. Table 3 provides an overview of how SNNs are used in computer vision.
Table 3 Use of SNNs in computer vision in recent years
S. no. |
Training paradigm |
Description |
Performance |
Ref. |
1 |
STDP and RSTDP |
It is possible to train a convolutional spiking neural network (SNN) by combining existing learning principles with spike-timing dependent plasticity (STDP). |
It makes it possible to learn more complex tasks with fewer training examples and higher accuracy. It also enables more efficient use of computing resources. |
286
|
2 |
STDP |
Spiking neural networks come in several varieties, but the lattice map spiking neural network (LM-SNN) model uses a lattice structure to represent the connectivity between neurons. |
It draws inspiration from the connection and organization present in organic neural networks. |
287
|
3 |
ANN to SNN Conversion |
To control the firing of the neurons in a more precise manner. The imbalanced threshold also helps to reduce the amount of energy required for computation, making it more efficient. |
This method can be used to simulate various types of neural networks. |
288
|
4 |
STDP and SGD |
This methodology is used to evaluate the performance of the FSHNN in a given task. The results showed that the FSHNN outperforms the conventional deep learning models in terms of predicting true positives. |
The FSHNN provides more reliable uncertainty estimates. |
289
|
5 |
Spatial envelope synthesis (SES) |
The implementation of SES on a robot platform enables it to navigate more accurately and efficiently than conventional systems. |
It has the potential to be used for a wide range of applications, such as robotics, autonomous navigation, and gaming. |
290
|
6 |
R-STDP |
This can encourage the neurons to respond more to the minority class and, thus, improve the recognition accuracy of the minority class. |
The reward adjustment in R-STDP will also increase the generalization ability of the model. |
291
|
7 |
Backpropagation |
The backpropagation SNN model was able to produce accurate 3D object detection results with minimal computational and memory requirements. |
The model was tested on a real-world dataset, showing promising results. |
292
|
SNNs are used for image recognition, object detection and segmentation, and tracking. It allows computer vision systems to have greater processing power and be more robust to changes in the environment. SNNs are also more energy-efficient than traditional deep learning models. To overcome obstacles and extend the capabilities of neuromorphic hardware, researchers, engineers, and developers must work together.284 The key to making neuromorphic hardware more powerful and efficient is to find ways to reduce energy consumption while still maintaining accuracy. Additionally, the development of software and algorithms tailored to the unique architecture of neuromorphic chips will be critical for unlocking their full potential.
9.1. Challenges and interdisciplinary collaborations for the co-design of computing stack in neuromorphic computers
The comprehensive integration of hardware, software, and algorithms in neuromorphic computers is important for fully exploiting the capabilities of brain-inspired computing. The aforementioned methodology poses several obstacles and necessitates the establishment of interdisciplinary partnerships to surmount them. These joint endeavours can lead to advancements in activities such as pattern recognition and real-time processing.293 The following are the primary obstacles and collaboration elements.
9.1.1. Hardware-software co-design.
Challenge.
The process of developing hardware and software components that are closely interconnected and enhanced for neuromorphic computing. Neuromorphic computing involves mimicking the human brain's ability to process information quickly and efficiently. This technology has the potential to revolutionize the way computers work, enabling more efficient and powerful applications. Neuromorphic computing can also be used to develop new artificial intelligence (AI) applications, which could have a wide range of applications from medical diagnosis to autonomous vehicles.294
Collaboration.
The establishment of a collaborative relationship between hardware engineers and software developers is of utmost importance to guarantee the alignment of hardware architectures with the specific demands of neural simulations and learning algorithms. Such collaboration is essential for the development of efficient, reliable, and secure systems. It also helps to ensure that systems are capable of meeting the performance requirements of a given task. Ultimately, this collaboration leads to the development of better AI solutions. This, in turn, leads to improved user experience, increased profits, and a competitive edge. Furthermore, collaboration also enables organizations to share resources, ideas, and best practices.295
9.1.2. Energy efficiency.
Challenge.
The objective of this research is to design and fabricate energy-efficient neuromorphic hardware capable of executing intricate calculations while minimising power usage. This hardware will be based on the memristor technology and will have low power consumption while also being highly sensitive to electrical signals. It will be able to process large amounts of data in a short amount of time. This hardware will also be capable of learning and adapting to new input patterns, allowing it to solve complex problems with high accuracy. It will have a wide range of applications in AI and other machine learning applications.296
Collaboration.
The optimisation of energy-efficient structures and algorithms necessitates collaboration among hardware designers, materials scientists, and algorithm developers. These collaborations must be conducted early on in the design process to ensure successful outcomes. Furthermore, it is important to consider the entire life cycle of the structure, including maintenance and decommissioning. Finally, the use of simulations and modeling is necessary to understand the properties and behaviour of energy-efficient structures and algorithms. Simulations and modelling can also be used to optimise the energy efficiency of structures. It is important to ensure that the simulations and models are accurate and reliable. Finally, it is essential to consider the impact of new technologies on the energy efficiency of structures.297
9.1.3. Neuron models.
Challenge.
The objective of this study is to develop neuron models that accurately represent the behaviour of biological neurons, while also being well-suited for efficient hardware implementation. To this end, the study will explore the use of a variety of neuron architectures, including spiking neural networks, recurrent neural networks and reservoir computing. The study will also investigate the use of various optimization techniques, such as backpropagation, to improve the accuracy of the models. Finally, the study will compare the performances of the different architectures and optimization techniques. The results of this study will enable researchers to better understand which architectures and optimization techniques are best for machine learning tasks. Additionally, it will provide insights into how to improve the performances of existing models.298
Collaboration.
The refinement of neuron models and their translation into hardware-friendly representations necessitates collaborative efforts among neuroscientists, computational biologists, and hardware engineers. This collaboration is essential to ensure that models are both biologically accurate and computationally efficient. Hardware-friendly representations must also be suitable for implementation in complex neural networks. Furthermore, these efforts must be supported by appropriate infrastructure and resources. To achieve this, researchers must work together to develop and optimize algorithms, implement them in complex neural networks, and provide the necessary infrastructure. Additionally, researchers must test and evaluate these models to ensure accuracy and performance.299
9.1.4. Synaptic models.
Challenge.
The objective of this research is to create synaptic models that accurately emulate the plasticity and learning capacities shown in biological synapses. By understanding the underlying mechanisms of synaptic plasticity, researchers hope to develop artificial neural networks that can learn and adapt to their environment. This could open up new possibilities for artificial intelligence and autonomous systems. Such neural networks could be used to develop better algorithms for machine learning and artificial intelligence, as well as for other applications that require adaptability. Additionally, these models could be used to analyze and understand the brain's complex neural processes.300
Collaboration.
The convergence of neuroscientists, materials scientists, and hardware designers in a collaborative effort aims to develop memristive synapses and subsequently incorporate them into hardware platforms. This collaboration will result in the development of a new generation of neuromorphic hardware that will be able to replicate the brain's cognitive abilities. It has the potential to revolutionize artificial intelligence and offer immense potential for use in various applications. This new technology could also enable scientists to better understand how the brain works and develop new treatments for neurological disorders. Furthermore, it could lead to the development of more energy-efficient computing systems.301
9.1.5. Algorithms and learning rules.
Challenge.
The objective is to develop learning algorithms and rules that can effectively utilize the hardware's capabilities for unsupervised learning and adaptability. These algorithms and rules can then be used to develop self-learning machines and systems that can be used in a variety of applications such as healthcare, transportation, and robotics. This will open up opportunities for new products and services, as well as create jobs in the field of artificial intelligence. This will also enable businesses to automate more of their processes, resulting in greater cost savings and more efficient operations. Additionally, self-learning systems have the potential to improve security and safety, as they can quickly detect and respond to threats.302
Collaboration.
The collaboration of researchers in the field of machine learning, computer scientists, and hardware developers aims to build learning algorithms that are compatible with neuromorphic systems. These algorithms are designed to mimic the way neurons in the brain process information. The goal is to create more efficient and energy-efficient computing systems for applications such as autonomous vehicles and robots. These neuromorphic systems have the potential to revolutionize AI and open up new avenues of research and development. They are also expected to provide valuable insights into the inner workings of the brain.303
9.1.6. Scalability.
Challenge.
The primary objective is to guarantee the scalability of neuromorphic systems to effectively accommodate extensive neural networks and practical applications in real-world scenarios. To achieve this, neuromorphic systems require massive parallelism, low latency, and efficient energy consumption. Additionally, they need to implement efficient algorithms and data structures to handle large amounts of data. To do this, neuromorphic systems need to incorporate innovative hardware designs and algorithms that take advantage of the unique characteristics of biological neurons. They should also be designed with the ability to easily incorporate new algorithms and models to adapt to changing requirements. Additionally, they should also be designed to be robust and resilient to noise.304
Collaboration.
The cooperation between hardware architects and system integrators is crucial in the development of scalable systems and data management solutions. The hardware architects design the hardware components, such as processors, memory, and storage, while the system integrators assemble them to create the system. Working together, they can ensure that the system can scale as the amount of data grows. The two roles also need to be able to effectively communicate and collaborate to ensure that the system is both robust and efficient. The hardware architects need to be able to design components that meet the needs of the system integrator, and the system integrator must be able to design a system that can use the components effectively.305
9.1.7. Benchmarking and validation.
Challenge.
The objective is to develop uniform benchmarks and validation protocols for evaluating the performance and capabilities of neuromorphic systems. These benchmarks should be unbiased and should take into account both the hardware and software components of neuromorphic systems. They should also consider both synthetic and real-world scenarios. Finally, they should be regularly updated to keep up with the ever-evolving capabilities of neuromorphic systems. These benchmarks should apply to different types of neuromorphic systems, such as spiking neural networks and reservoir computing systems. Furthermore, they should allow for interoperability between different platforms, so that researchers can compare the performance of different systems.306
Collaboration.
The establishment of collaborative efforts among academics in the fields of neuromorphic computing, neurology, and application domains is imperative for delineating benchmarks and assessing the efficacy of hardware and software platforms. Such collaborative efforts can help identify potential limitations of existing approaches and suggest new directions for research and development. Moreover, this can help promote collaboration between industry and academia, leading to better commercial outcomes. This can help to create new ideas, technologies, and products that will benefit society. It can also help to foster a more innovative and collaborative culture that is essential for the continued success of businesses.307
9.2. Advancements and implications
9.2.1. Pattern recognition.
Collaborative design endeavours have the potential to facilitate the advancement of neuromorphic systems that demonstrate exceptional proficiency in tasks related to pattern recognition. These systems possess the capability to effectively analyse sensory data, rendering them well-suited for a range of applications such as image and audio recognition, natural language processing, and robotics. Furthermore, neuromorphic systems have the potential to revolutionise the field of machine learning, allowing for the development of more accurate and efficient artificial intelligence systems. Neuromorphic systems are also capable of learning in ways that traditional machine learning systems cannot, such as through unsupervised learning and reinforcement learning. This makes them an ideal candidate for a variety of applications that require a high degree of intelligence.308
9.2.2. Real-time processing.
Neuromorphic computers that have been optimised for real-time processing have the potential to facilitate several applications, including but not limited to autonomous cars, drones, and real-time data analysis. Due to their efficient low-latency and parallel processing capabilities, these systems are highly suitable for activities that necessitate prompt decision-making. These systems can also be used for natural language processing, computer vision and artificial intelligence. They are also capable of learning from their environment and adapting to changing conditions. These systems are also capable of making decisions with minimal human intervention.309 This makes these systems ideal for tasks that require quick and accurate decisions.
9.2.3. Cognitive computing.
Co-designed neuromorphic systems possess the capability to facilitate cognitive computing applications, hence enhancing machines' ability to engage in reasoning, learning, and adapting to dynamic settings with greater efficacy. The aforementioned has significant ramifications for the domains of healthcare, finance, and scientific research. These systems can be used to improve the accuracy of medical diagnoses, automate stock trading, and accelerate scientific research. Moreover, they can also be used to develop AI-powered robots that can be used in hazardous environments. AI-powered robots can also be used to perform repetitive tasks, such as manufacturing, agriculture, and mining. This can help reduce labor costs and improve the safety of workers.310
9.2.4. Energy efficiency.
The implementation of energy-efficient neuromorphic computing stacks has the potential to significantly decrease power consumption in both data centers and edge devices. This reduction in power usage can result in substantial cost savings and contribute to positive environmental outcomes. Furthermore, energy-efficient neuromorphic computing stacks have the potential to improve computing performance, reduce latency, and enable more complex AI applications. Neuromorphic computing stacks are also well-suited for applications such as natural-language processing, image recognition, and time-series analysis. These applications require large amounts of data to be processed quickly, making them ideal for energy-efficient neuromorphic computing stacks.311
9.2.5. Interdisciplinary breakthroughs.
The convergence of expertise from many disciplines can facilitate interdisciplinary advancements, hence stimulating innovation and the emergence of fresh technologies and applications. This can help to address complex challenges, such as climate change, energy shortages, and healthcare needs. Interdisciplinary collaborations can also help to create new technologies and products, as well as new ideas for solving global issues. Interdisciplinary collaborations can also help to create economic opportunities, as new markets will be created for products and services that were previously unavailable.312 Additionally, it can help to create new jobs, as people with different skill sets are needed to work together to create successful solutions. The difficulties of co-design in neuromorphic computing can result in improvements in pattern recognition, real-time processing, cognitive computing, and energy efficiency. It paves the way for intelligent systems that can emulate the complexity and adaptability of the human brain while still being effective.313
10. Disparities between neuromorphic hardware and biological systems
The issue of discrepancies between neuromorphic hardware and biological systems poses a significant difficulty within the domain of neuromorphic engineering. Researchers are now implementing diverse approaches to enhance the faithfulness, correctness, and exactness of neural computations in neuromorphic hardware, as depicted in Fig. 22.314
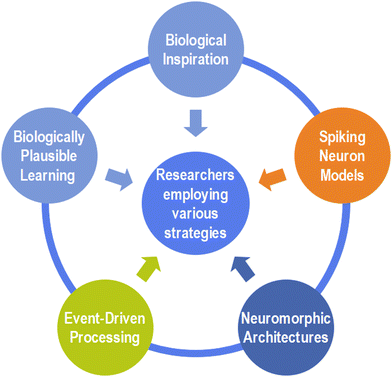 |
| Fig. 22 Researchers are improving neuromorphic hardware neural computing fidelity. | |
10.1. Biological inspiration
Academic researchers are thoroughly examining the behaviour shown by biological neurons and neural networks to acquire a deeper understanding of their functioning. This entails comprehending the intricate interaction among ion channels, synapses, and dendritic processes. Computer simulations are being used to understand better how neurons work and how different components of neural networks interact. Ultimately, this understanding will be used to create more efficient artificial neural networks.315 This knowledge is expected to lead to the development of more powerful AI systems that can learn from their environment and adapt to new situations. Such AI systems could be used for various tasks, including medical diagnoses, autonomous vehicles, and financial predictions. This has the potential to revolutionize a wide range of industries and create new opportunities for businesses.316 AI systems are poised to have a significant impact on the global economy, and the implications are yet to be fully understood.
10.2. Spiking neuron models
Spike neuron models, such as the integrate-and-fire model and the Hodgkin–Huxley model, are employed to capture the fundamental dynamics exhibited by biological neurons. These models facilitate the design of neuromorphic hardware systems replicating biological neurons' spiking behaviour. These models can also be used to study the effects of different parameters on the spiking behavior of neurons. Furthermore, they can be used to develop neural network models, which can be used to simulate complex brain functions.317 These models can also be used to identify the underlying mechanisms behind various neurological disorders, such as epilepsy and Alzheimer's disease. This can help researchers develop new treatments or therapies for these diseases. Additionally, these models can be used to develop new AI algorithms and applications. These algorithms and applications can then be used to automate tasks that were previously done by humans, such as medical diagnosis, natural language processing, and facial recognition. This automation can help save time and resources, as well as improve accuracy and precision. It can also help researchers to rapidly identify patterns and trends that were previously difficult to detect.318
10.3. Neuromorphic architectures
Researchers are currently engaged in the development of novel neuromorphic architectures with the aim of more accurately emulating the connectivity and organisational patterns observed in organic neural networks. The scope of this includes the physical realisations of neuronal layers, synapses, and connection patterns that are present within the brain. These neuromorphic architectures aim to replicate the dynamics and processing of the human brain, allowing researchers to better understand brain function and develop more efficient AI systems.319 Neuromorphic architectures can also be used for biomedical applications, such as the development of brain–machine interfaces. They can also be used to create cognitive robots that are capable of learning and adapting to their environment. Neuromorphic architectures are also being used to develop AI-powered systems for autonomous driving and robotics. They can also be used to develop AI-powered systems for natural language processing and sentiment analysis. Neuromorphic architectures can also be used to develop AI-powered systems for weather forecasting, stock market analysis, and fraud detection.320 Neuromorphic architectures can also be used to develop AI-powered systems for medical imaging, facial recognition, and natural language processing.
10.4. Event-driven processing
Numerous neuromorphic systems employ event-driven processing, a computational approach that exclusively operates when input changes or spike events occur, thereby emulating the asynchronous characteristics observed in biological brain networks. This practice contributes to the reduction of power consumption and the improvement of computing efficiency. Moreover, event-driven processing allows for the implementation of dynamic spiking neural networks, which are capable of learning and adapting to their environment.321 This approach is also advantageous for the implementation of more complex tasks, such as computer vision and natural language processing. Event-driven processing is also beneficial in reducing latency and system complexity, as well as providing a more efficient use of resources. This approach is also suitable for real-time applications, such as autonomous vehicles and robotics. Additionally, event-driven processing allows for scalability since applications can be easily extended by adding additional nodes.322 This approach is also beneficial to reduce the cost of software development, as less code is needed to implement complex tasks.
10.5. Biologically plausible learning rules
The learning algorithms and synaptic plasticity rules included in neuromorphic hardware are specifically designed to adhere to biological plausibility. This guarantees that the hardware is capable of replicating the Hebbian learning principles that are exhibited in biological synapses. This type of hardware can be beneficial in fields such as artificial intelligence, robotics, and natural language processing. It can also be used to help analyze large amounts of data quickly and accurately. Furthermore, neuromorphic hardware can be used to improve the performance of machine learning models, allowing them to learn more accurately and more quickly.323 This hardware can also be used to build neural networks and other artificial intelligence applications. It can also be used to develop more efficient and secure computing systems. The convergence of hardware engineering and neuroscience has facilitated the emergence of hardware–software co-design through collaborative endeavours.324 This methodology enables the creation of hardware platforms that are more closely linked with the specific demands of brain simulations and models. Reconfigurability is a prominent feature observed in numerous neuromorphic systems, enabling researchers to engage in the exploration of diverse neural network designs and parameters to achieve a closer alignment with biological behaviour. Hybrid systems are characterised by the integration of traditional computing components, such as central processing units (CPUs) and graphics processing units (GPUs), with neuromorphic hardware.325 This enables the performance of tasks that necessitate the utilisation of both conventional computing and brain processing, hence facilitating the integration of biological and artificial systems. Benchmarking and validation are important processes in the field of neuromorphic hardware research. These processes involve comparing the performance of neuromorphic hardware with biological data to measure any discrepancies and suggest potential areas that require further enhancement. This encompasses the evaluation of spike timing precision, network dynamics, and synaptic plasticity.326 The efficient resolution of discrepancies necessitates the crucial involvement of academics from several disciplines, including neuroscience, computer science, and materials science, in interdisciplinary collaboration. The use of a multidisciplinary approach facilitates the cultivation of a more profound comprehension of biological and artificial neural systems. The field of materials science is currently making significant contributions to the advancement of neuromorphic hardware.327 Materials exhibiting memristive characteristics can closely replicate synaptic behaviour. The use of feedback loops in neuromorphic systems enables the capacity for adaptation and self-correction by leveraging recognised discrepancies, hence enhancing accuracy progressively. Through the utilisation of these methodologies, scholars strive to reduce the disparity between neuromorphic hardware and biological systems, consequently augmenting the faithfulness, precision, and accuracy of neural computations in artificial systems and propelling the possibilities of neuromorphic computing.328
11. Opportunity of neuromorphic computers
It is a difficult and diverse process to co-design the full computing stack in neuromorphic computers, taking hardware, software, and algorithmic factors into account. The Fig. 23 shows that although it is theoretically conceivable to pursue such a strategy, there are a number of difficulties and factors to take into consideration.329–331 Utilizing specialized hardware designs and components, neuromorphic computing systems seek to replicate the composition and operation of the human brain. Co-engineering the hardware stack entails creating specialized processing units, optimizing the interconnects, and building the neural network architecture.
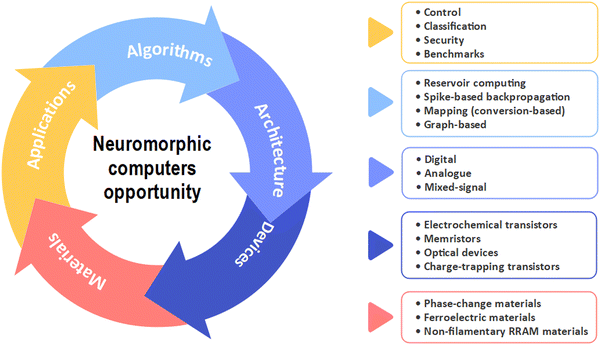 |
| Fig. 23 Possibility of co-designing the entire computing stack in neuromorphic computers. | |
This calls for knowledge in the fields of materials science, electrical engineering, and computer engineering. To maximize the capabilities of neuromorphic systems, the software stack must be jointly designed. This entails creating frameworks, libraries, and programming models that make it possible for algorithms to be effectively mapped to hardware architecture.332–334 In order to support the distinctive features of neuromorphic computing, specialized tools for neural network construction, training, and inference need to be developed or modified. In contrast to conventional computing paradigms, neuromorphic computing places a strong emphasis on the utilization of spiking neural networks and event-driven computation. It is necessary to create innovative algorithms and methods that can make use of the special properties of neuromorphic hardware to co-design the computing stack. This entails developing effective spike encoding and decoding strategies, improving neural network models, and investigating novel learning and inference techniques.335–338 Experts from a variety of fields, including neuroscience, computer science, electrical engineering, and materials science, must work together to co-design the complete computing stack in neuromorphic computers.339 This cooperation is necessary to make sure that design decisions for algorithms, software, and hardware are coordinated and mutually beneficial. It is difficult to co-design the entire computing stack in neuromorphic computers, but doing so could lead to significant improvements in computing power, particularly for tasks that benefit from neuromorphic processing, like pattern recognition, sensor data analysis, and real-time processing. These possibilities are being investigated by ongoing research, although it might be some time before a completely co-designed neuromorphic computing stack is used in everyday life.340–342 This is because these tasks require complex algorithms and architectures to be implemented, and these algorithms are difficult to design and optimize in neuromorphic computers. Additionally, it is hard to make sure that the entire stack works together seamlessly. Despite these challenges, ongoing research suggests that a co-designed neuromorphic computing stack could be used to improve computing power for certain tasks significantly.
12. Conclusions and future perspectives
Neuromorphic computing is an emerging and highly innovative discipline that has the promise of tilizerizedg multiple fields, such as artificial intelligence (AI), robotics, neurology, and cognitive science. The integration of neuromorphic and conventional computing enables the development of robust and efficient computing architectures, hence propelling the progress of artificial intelligence (AI) and machine learning (ML) applications. This research investigates the latest advancements in employing machine learning-based methodologies for the design of neuromorphic materials for engineering solutions materials. It examines the use of both neuromorphic and traditional computing techniques to enhance the efficiency and effectiveness of these materials. The combination of neuromorphic and conventional computers has the potential to facilitate the development of materials that exhibit improved characteristics and performance. Although the potential of neuromorphic computing is vast, its current development is in its nascent phases, and practical applications are currently being planned. Nevertheless, notable advancements in neuromorphic computing have the potential to fundamentally transform computer tilizeriz and facilitate the emergence of novel and ingenious applications. Neuromorphic computers have distinct characteristics that set them apart from conventional computing systems. The design of these systems is intended to exploit parallelism, drawing inspiration from the brain's capacity to process numerous inputs concurrently. Neuromorphic architectures provide exceptional performance in handling intricate problems tilizerized by imprecisely defined constraints and noisy input data, rendering them well-suited for many applications like as pattern recognition, machine learning, and cognitive computing.
In order to enhance hardware design and effectively tilize the capabilities of neuromorphic computers, it is imperative to employ neuromorphic algorithms and applications. The algorithms can be classified into two distinct categories, namely analogue and digital signal processing approaches. Analogue systems employ continuous signals that exhibit smooth variations across time or space, hence providing notable benefits such as enhanced precision and resilience against noise interference. Biomaterials possess the ability to interface with living tissues and biological systems, rendering them amenable for deployment in many medical contexts. These materials can be intentionally engineered to exhibit biocompatibility, bioactivity, and degradability, thereby enhancing their suitability for a wide range of medical applications.
(i) The objective of biomaterial-based ultra-flexible artificial synaptic devices is to emulate the functionalities of biological synapses seen in the human brain. These devices possess biocompatibility, enabling them to engage with biological systems without inducing any adverse effects. Synaptic connections in the brain can be emulated, thereby facilitating the modification of electrical signals and augmenting ion transport. During the nascent phases of their development, these devices possess the capacity to revolutionise the fields of neuromorphic computing, brain–machine interfaces, and artificial intelligence. Furthermore, there is potential for them to significantly transform the healthcare sector through the provision of streamlined platforms for drug administration and tailored therapeutic interventions.
(ii) The integration of neuromorphic computing with material design has the potential to bring about significant transformations in the field of material development, leading to the creation of novel materials that exhibit enhanced features and performance characteristics. Neuromorphic computing, drawing inspiration from the anatomical organisation of the brain, facilitates the efficient processing of information. When the integration of material design occurs, it has the potential to greatly enhance the process of material discovery, optimise the structures of materials at the atomic or molecular level, and facilitate the development of self-learning materials.
(iii) Neuromorphic artificial neural networks (ANNs) replicate the anatomical and functional characteristics of the human brain. Neuromorphic chips or processors are utilised in their operations, which are characterised by specialised hardware designed to effectively handle neural network computations through parallelism and low power consumption. Spiking neural networks (SNNs) are frequently employed in neuromorphic artificial neural networks (ANNs), providing many benefits such as event-driven computation, efficient encoding of temporal information, and enhanced energy efficiency.
(iv) The incorporation of neuromorphic computing into the field of material design holds significant promise for transformative advancements across various industries, while also presenting innovative prospects within the domains of material science and engineering. The integration of these materials into current devices and systems allows for enhanced operational efficiency and adaptability.
(v) Electrochemical memristors play a vital role in the advancement of artificial neurons and synapses, as they are capable of emulating the fundamental operations exhibited by biological systems. These gadgets exhibit energy efficiency and possess the ability to operate well at low-temperature conditions. The utilisation of memristive synapses facilitates the replication of synaptic plasticity and learning mechanisms that are evident in biological brain networks. These devices exhibit a distinctive relationship between resistance and current and are recognised for their high energy efficiency, rendering them well-suited for applications that need low power consumption and energy conservation.
(vi) Synaptic devices based on nanowires emulate the functionality of biological synapses, providing advantages such as compact size, memristive properties, and the ability to exhibit synaptic plasticity. Neuromorphic hardware benefits from the ability of these devices to efficiently process information while consuming minimum power, rendering them highly suitable for energy-efficient applications. Triboelectric nanogenerators (TENGs) are devices that transform mechanical energy into electrical energy, thereby functioning as energy-efficient power sources and sensors for biomechanical movements.
(vii) The optimisation of energy-efficient neuromorphic hardware, neuron models, learning algorithms, and learning rules necessitates the collaboration of hardware designers, materials scientists, algorithm developers, and neuroscientists. The collective endeavours can result in inventive solutions that have extensive applicability, spanning several domains such as artificial intelligence and healthcare.
(viii) The issue of scalability poses a significant obstacle in the field of neuromorphic computing, necessitating advancements in hardware architecture, energy efficiency, and the seamless integration of neuromorphic technology with traditional computing systems. The establishment of standardised benchmarks and validation methodologies is crucial in order to reliably evaluate the performance of neuromorphic systems.
(ix) In summary, neuromorphic computing exhibits considerable promise as a topic that holds the capacity to bring about transformative advancements in artificial intelligence, materials science, and diverse industrial sectors. The collaboration of diverse teams is essential in order to fully leverage their potential and effectively address various difficulties. The objective of this study is to develop energy-efficient neuromorphic hardware and algorithms to foster creativity and augment computer capabilities across many applications.
Authors’ contributions
CP, HV, AM, KK, and LRG conceptualized the idea and listed the table of contents for the manuscript. HV and AM collected the literature and relevant information. CP, HV, AM, RM, EK, AF, VS, KK, and LRG prepared the original draft of the manuscript. KK, RM, EK, AF, and VS reviewed and edited the original draft of the manuscript. All authors read and approved the final manuscript.
Availability of data and materials
Data sharing does not apply to this article as no datasets were generated or analysed during the current study.
Conflicts of interest
Authors declare that they have no competing interests.
Acknowledgements
The reported study was funded by the Russian Federation Government (Agreement No. 075-15-2022-1123).
References
- J. Von Neumann, IEEE Ann. Hist. Comput., 1993, 15, 27–75 Search PubMed.
- C. Mead, Proc. IEEE, 1990, 78, 1629–1636 CrossRef.
- L. Chua, IEEE Trans. Circuit Theory, 1971, 18, 507–519 Search PubMed.
- A. Krizhevsky, I. Sutskever and G. E. Hinton, Commun. ACM, 2017, 60, 84–90 CrossRef.
- P. A. Merolla, J. V. Arthur, R. Alvarez-Icaza, A. S. Cassidy, J. Sawada, F. Akopyan, B. L. Jackson, N. Imam, C. Guo and Y. Nakamura, Science, 2014, 345, 668–673 CrossRef CAS PubMed.
- S. Moradi, N. Qiao, F. Stefanini and G. Indiveri, IEEE Trans. Biomed. Circuits Syst., 2017, 12, 106–122 Search PubMed.
- A. Vanarse, A. Osseiran and A. Rassau, Sensors, 2017, 17, 2591 CrossRef PubMed.
- T. Ferreira de Lima, B. J. Shastri, A. N. Tait, M. A. Nahmias and P. R. Prucnal, Nanophotonics, 2017, 6, 577–599 Search PubMed.
- Y. Van De Burgt, E. Lubberman, E. J. Fuller, S. T. Keene, G. C. Faria, S. Agarwal, M. J. Marinella, A. Alec Talin and A. Salleo, Nat. Mater., 2017, 16, 414–418 CrossRef CAS PubMed.
- B. Rajendran, A. Sebastian, M. Schmuker, N. Srinivasa and E. Eleftheriou, IEEE Signal Process. Mag., 2019, 36, 97–110 Search PubMed.
- M. A. Zidan, A. Chen, G. Indiveri and W. D. Lu, J. Electroceram., 2017, 39, 4–20 CrossRef CAS.
- C. S. Thakur, R. Wang, T. J. Hamilton, R. Etienne-Cummings, J. Tapson and A. van Schaik, IEEE Trans. Circuits Syst. I: Regular Pap., 2017, 65, 1174–1184 Search PubMed.
-
B. J. Shastri, A. N. Tait, T. F. de Lima, M. A. Nahmias, H.-T. Peng and P. R. Prucnal, 2017, preprint, arXiv:1801.00016 DOI:10.48550/arXiv.1801.00016.
- M. Davies, N. Srinivasa, T.-H. Lin, G. Chinya, Y. Cao, S. H. Choday, G. Dimou, P. Joshi, N. Imam and S. Jain, IEEE Micro, 2018, 38, 82–99 Search PubMed.
- E. Chicca and G. Indiveri, Appl. Phys. Lett., 2020, 116, 120501 CrossRef CAS.
- M. Rasouli, Y. Chen, A. Basu, S. L. Kukreja and N. V. Thakor, IEEE Trans. Biomed. Circuits Syst., 2018, 12, 313–325 Search PubMed.
- M. Pfeiffer and T. Pfeil, Front. Neurosci., 2018, 12, 774 CrossRef PubMed.
- M. Davies, Symposium on VLSI Circuits, 2021, 1–2, DOI:10.23919/VLSICircuits52068.2021.9492385.
- J. Yoo and M. Shoaran, Curr. Opin. Biotechnol, 2021, 72, 95–101 CrossRef CAS PubMed.
- G. Indiveri, Neuromorphic Comput. Eng., 2021, 1, 010401, DOI:10.48550/arXiv.1911.02385.
- M. Davies, Nat. Mach. Intell., 2019, 1, 386–388 CrossRef.
- T. F. De Lima, H.-T. Peng, A. N. Tait, M. A. Nahmias, H. B. Miller, B. J. Shastri and P. R. Prucnal, J. Light Technol., 2019, 37, 1515–1534 Search PubMed.
-
F. Ortiz, E. Lagunas, W. Martins, T. Dinh, N. Skatchkovsky, O. Simeone, B. Rajendran, T. Navarro and S. Chatzinotas, 39th International Communications Satellite Systems Conference (ICSSC), 2022.
-
H. F. Langroudi, T. Pandit, M. Indovina and D. Kudithipudi, Digital neuromorphic chips for deep learning inference: a comprehensive study, in Applications of Machine Learning, ed. M. E. Zelinski, T. M. Taha, J. Howe, A. A. Awwal and K. M. Iftekharuddin, SPIE, sep 2019, p. 9. [Online], 2019 DOI:10.1117/12.2529407.
- L. Salt, D. Howard, G. Indiveri and Y. Sandamirskaya, IEEE Trans. Neural Networks Learn. Syst., 2019, 31, 3305–3318 Search PubMed.
-
C. Mayr, S. Hoeppner and S. Furber, arXiv, 2019, preprint, arXiv:1911.02385 DOI:10.48550/arXiv.1911.02385.
- X. Xu, W. Han, M. Tan, Y. Sun, Y. Li, J. Wu, R. Morandotti, A. Mitchell, K. Xu and D. J. Moss, IEEE J. Sel. Top. Quantum Electron., 2022, 29, 1–12 Search PubMed.
-
P. Blouw, X. Choo, E. Hunsberger and C. Eliasmith, Proceedings of the 7th annual neuro-inspired computational elements workshop, 2019.
-
C. M. Vineyard, S. Green, W. M. Severa and Ç. K. Koç, Proceedings of the International Conference on Neuromorphic Systems, 2019.
- S. G.-C. Carrillo, E. Gemo, X. Li, N. Youngblood, A. Katumba, P. Bienstman, W. Pernice, H. Bhaskaran and C. D. Wright, APL Mater., 2019, 7, 091113 CrossRef.
- L. Wang, W. Liao, S. L. Wong, Z. G. Yu, S. Li, Y. F. Lim, X. Feng, W. C. Tan, X. Huang and L. Chen, Adv. Funct. Mater., 2019, 29, 1901106 CrossRef.
-
A. Ussa, L. Della Vedova, V. R. Padala, D. Singla, J. Acharya, C. Z. Lei, G. Orchard, A. Basu and B. Ramesh, arXiv, 2019, preprint, arXiv:1910.09806 DOI:10.48550/arXiv.1910.09806.
- C. Wan, K. Xiao, A. Angelin, M. Antonietti and X. Chen, Adv. Intell. Syst., 2019, 1, 1900073 CrossRef.
- A. Vanarse, A. Osseiran, A. Rassau and P. van der Made, Sensors, 2019, 19, 4831 CrossRef CAS PubMed.
-
M. Zamani, M. Ronchini, H. A. Huynh, H. Farkhani and F. Moradi, IEEE International Symposium on Circuits and Systems (ISCAS), 2021.
- C. Frenkel, J.-D. Legat and D. Bol, IEEE Trans. Biomed. Circuits Syst., 2019, 13, 999–1010 Search PubMed.
- Y. Lee and T.-W. Lee, Acc. Chem. Res., 2019, 52, 964–974 CrossRef CAS PubMed.
- S. Miao, G. Chen, X. Ning, Y. Zi, K. Ren, Z. Bing and A. Knoll, Front. Neurorob., 2019, 13, 38 CrossRef PubMed.
-
G. Orchard, E. P. Frady, D. B. D. Rubin, S. Sanborn, S. B. Shrestha, F. T. Sommer and M. Davies, IEEE Workshop on Signal Processing Systems (SiPS), 2021 Search PubMed.
- J. D. Smith, A. J. Hill, L. E. Reeder, B. C. Franke, R. B. Lehoucq, O. Parekh, W. Severa and J. B. Aimone, Nat. Electron., 2022, 5, 102–112 CrossRef.
-
C. Ostrau, C. Klarhorst, M. Thies and U. Rückert, Proceedings of the Neuro-inspired Computational Elements Workshop, 2020 Search PubMed.
-
G. Rutishauser, R. Hunziker, A. Di Mauro, S. Bian, L. Benini and M. Magno, arXiv, 2023, preprint, arXiv:2302.07957 DOI:10.48550/arXiv.2302.07957.
-
K. A. Bharadwaj, 3rd International conference on Electronics, Communication and Aerospace Technology (ICECA), 2019.
- D. Marković, A. Mizrahi, D. Querlioz and J. Grollier, Nat. Rev. Phys., 2020, 2, 499–510 CrossRef.
- J. Xu, X. Zhao, X. Zhao, Z. Wang, Q. Tang, H. Xu and Y. Liu, J. Mater. Chem., 2022, 2, 2200028 CAS.
-
J. Plank, C. Rizzo, K. Shahat, G. Bruer, T. Dixon, M. Goin, G. Zhao, J. Anantharaj, C. Schuman and M. Dean, Advanced Electronic Materials, The TENNLab suite of LIDAR-based control applications for recurrent, spiking, neuromorphic systems, Oak Ridge National Lab. (ORNL), Oak Ridge, TN (United States), 2019 Search PubMed.
- V. Erokhin, Bionanoscience, 2020, 10, 834–847 CrossRef.
- Y. Yang, X. Zhao, S. Wang, C. Zhang, H. Sun, F. Xu, Y. Tong, Q. Tang and Y. Liu, J. Mater. Chem., 2020, 8, 16542–16550 RSC.
- Y. Yang, X. Zhao, C. Zhang, Y. Tong, J. Hu, H. Zhang, M. Yang, X. Ye, S. Wang and Z. Sun, Adv. Funct. Mater., 2020, 30, 2006271 CrossRef CAS.
- C. Zhang, F. Xu, X. Zhao, M. Zhang, W. Han, H. Yu, S. Wang, Y. Yang, Y. Tong, Q. Tang and Y. Liu, Nano Energy, 2022, 95, 107001 CrossRef CAS.
-
F. Corradi, S. Pande, J. Stuijt, N. Qiao, S. Schaafsma, G. Indiveri and F. Catthoor, International Joint Conference on Neural Networks (IJCNN), 2019.
-
T. Mikawa, R. Yasuhara, K. Katayama, K. Kouno, T. Ono, R. Mochida, Y. Hayata, M. Nakayama, H. Suwa, Y. Gohou and T. Kakiage, Neuromorphic Computing Based on Analog ReRAM as Low Power Solution for Edge Application, in 2019 IEEE 11th International Memory Workshop (IMW); Monterey, USA, May 12–15, IEEE, 2019, pp 1–4 DOI:10.1109/IMW.2019.8739720.
-
J. B. Aimone, W. Severa and C. M. Vineyard, Proceedings of the International Conference on Neuromorphic Systems, 2019.
- S. W. Cho, S. M. Kwon, M. Lee, J.-W. Jo, J. S. Heo, Y.-H. Kim, H. K. Cho and S. K. Park, Nano Energy, 2019, 66, 104097 CrossRef CAS.
-
A. M. Zyarah, K. Gomez and D. Kudithipudi, IEEE Transactions on Computers, Springer, 2020, 69, 1099–1112 Search PubMed.
- H. Han, H. Yu, H. Wei, J. Gong and W. Xu, Small, 2019, 15, 1900695 CrossRef PubMed.
- F. Liao, F. Zhou and Y. Chai, J. Semicond., 2021, 42, 013105 CrossRef.
-
Y. Ma, E. Donati, B. Chen, P. Ren, N. Zheng and G. Indiveri, 2nd IEEE International Conference on Artificial Intelligence Circuits and Systems (AICAS), 2020.
- J. Aimone, P. Date, G. Fonseca-Guerra, K. Hamilton, K. Henke, B. Kay, G. Kenyon, S. Kulkarni, S. Mniszewski and M. Parsa, Neuromorphic Comput., 2022, 1–8 Search PubMed.
- C. Zhang, Y. Li, F. Yu, G. Wang, K. Wang, C. Ma, X. Yang, Y. Zhou and Q. Zhang, Nano Energy, 2023, 109, 108274 CrossRef CAS.
- R. Waser, R. Dittmann, G. Staikov and K. Szot, Adv. Mater., 2009, 21, 2632–2663 CrossRef CAS PubMed.
-
I. K. Schuller, R. Stevens, R. Pino and M. Pechan, Neuromorphic computing–from materials research to systems architecture roundtable, USDOE Office of Science (SC) (United States), 2015 Search PubMed.
- S. Najmaei, A. L. Glasmann, M. A. Schroeder, W. L. Sarney, M. L. Chin and D. M. Potrepka, Mater. Today Commun., 2022, 59, 80–106 CrossRef CAS.
- B. J. Shastri, A. N. Tait, T. Ferreira de Lima, W. H. Pernice, H. Bhaskaran, C. D. Wright and P. R. Prucnal, Nat. Photonics, 2021, 15, 102–114 CrossRef CAS.
- M. Xu, X. Chen, Y. Guo, Y. Wang, D. Qiu, X. Du, Y. Cui, X. Wang and J. Xiong, Adv. Mater., 2023, 2301063 CrossRef PubMed.
- Z. Zhang, D. Yang, H. Li, C. Li, Z. Wang, L. Sun and H. Yang, Neuromorphic Comput. Eng., 2022, 2(3), 032004, DOI:10.1088/2634-4386/ac8a6a.
- G. Indiveri, B. Linares-Barranco, R. Legenstein, G. Deligeorgis and T. Prodromakis, Nanotechnology, 2013, 24, 384010 CrossRef PubMed.
- J. Bian, Z. Cao and P. Zhou, Appl. Phys. Rev., 2021, 8, 041313, DOI:10.1063/5.0067352.
- B. Sun, T. Guo, G. Zhou, S. Ranjan, Y. Jiao, L. Wei, Y. N. Zhou and Y. A. Wu, Mater. Today Phys., 2021, 18, 100393 CrossRef CAS.
- K. Yamazaki, V.-K. Vo-Ho, D. Bulsara and N. Le, Brain Sci., 2022, 12, 863 CrossRef PubMed.
- S. Bolat, G. Torres Sevilla, A. Mancinelli, E. Gilshtein, J. Sastre, A. Cabas Vidani, D. Bachmann, I. Shorubalko, D. Briand and A. N. Tiwari, Sci. Rep., 2020, 10, 16664 CrossRef CAS PubMed.
-
V. Gupta, G. Lucarelli, S. Castro, T. Brown and M. Ottavi, Integrated Systems In Nanoscale, 2019.
- M. V. DeBole, B. Taba, A. Amir, F. Akopyan, A. Andreopoulos, W. P. Risk, J. Kusnitz, C. O. Otero, T. K. Nayak and R. Appuswamy, Computer, 2019, 52, 20–29 Search PubMed.
- A. Rubino, C. Livanelioglu, N. Qiao, M. Payvand, G. Indiveri and S. I. R. Papers, IEEE Trans. Circuits, 2020, 68, 45–56 Search PubMed.
- C. Bartolozzi, G. Indiveri and E. Donati, Nat. Commun., 2022, 13, 1024 CrossRef CAS PubMed.
- V. Kornijcuk and D. S. Jeong, Adv. Intell. Syst., 2019, 1, 1900030 CrossRef.
- T. Wunderlich, A. F. Kungl, E. Müller, A. Hartel, Y. Stradmann, S. A. Aamir, A. Grübl, A. Heimbrecht, K. Schreiber and D. Stöckel, Front. Neurosci., 2019, 13, 260 CrossRef PubMed.
- A. Opala, S. Ghosh, T. C. Liew and M. Matuszewski, Phys. Rev. Appl., 2019, 11, 064029 CrossRef CAS.
-
V. R. Leite, Z. Su, A. M. Whatley and G. Indiveri, arXiv, 2022, preprint, arXiv:2203.00655 DOI:10.48550/arXiv.2203.00655.
- F. Zhou, Z. Zhou, J. Chen, T. H. Choy, J. Wang, N. Zhang, Z. Lin, S. Yu, J. Kang and H.-S. P. Wong, Nat. Nanotechnol., 2019, 14, 776–782 CrossRef CAS PubMed.
- S. Choi, J. Yang and G. Wang, Adv. Mater. Processes, 2020, 32, 2004659 CrossRef CAS PubMed.
- Y. Cao, S. Wang, R. Wang, Y. Xin, Y. Peng, J. Sun, M. Yang, X. Ma, L. Lv and H. Wang, Sci. China Mater., 2023, 1–9 Search PubMed.
- K. Sozos, C. Mesaritakis and A. Bogris, IEEE J. Quantum Electron., 2021, 57, 1–7 Search PubMed.
-
M. Sharifshazileh, K. Burelo, T. Fedele, J. Sarnthein and G. Indiveri, 26th IEEE International Conference on Electronics, Circuits and Systems (ICECS), 2019.
- Z.-Y. He, T.-Y. Wang, J.-L. Meng, H. Zhu, L. Ji, Q.-Q. Sun, L. Chen and D. W. Zhang, Mater. Horiz., 2021, 8, 3345–3355 RSC.
-
M. Evanusa and Y. Sandamirskaya, Proceedings of the IEEE/CVF Conference on Computer Vision and Pattern Recognition, 2019.
- L. A. Camuñas-Mesa, B. Linares-Barranco and T. Serrano-Gotarredona, Materials, 2019, 12, 2745 CrossRef PubMed.
- J. Feldmann, N. Youngblood, C. D. Wright, H. Bhaskaran and W. H. Pernice, Nature, 2019, 569, 208–214 CrossRef CAS PubMed.
- D. Moss, IEEE Techrxiv, 2022, 220611, DOI:10.36227/techrxiv.20055623.v1.
- C. D. Wright, H. Bhaskaran and W. H. Pernice, MRS Bull., 2019, 44, 721–727 CrossRef.
- B. Shi, N. Calabretta and R. Stabile, IEEE J. Sel. Top. Quantum Electron., 2019, 26, 1–11 Search PubMed.
-
T. Chou, W. Tang, J. Botimer and Z. Zhang, ACM International Symposium on Microarchitecture, 2019.
- L. Zhang, Z. Tang, J. Fang, X. Jiang, Y.-P. Jiang, Q.-J. Sun, J.-M. Fan, X.-G. Tang and G. Zhong, Appl. Surf. Sci., 2022, 606, 154718 CrossRef CAS.
- T. Paul, T. Ahmed, K. K. Tiwari, C. S. Thakur and A. Ghosh, 2D Mater., 2019, 6, 045008 CrossRef CAS.
- S. Majumdar, H. Tan, Q. H. Qin and S. van Dijken, Adv. Electron. Mater., 2019, 5, 1800795 CrossRef.
- C. Yakopcic, T. M. Taha, D. J. Mountain, T. Salter, M. J. Marinella and M. McLean, IEEE Trans. Comput.-Aided Des. Integr. Circuits Syst., 2019, 39, 1084–1095 Search PubMed.
-
R. Patton, P. Date, S. Kulkarni, C. Gunaratne, S.-H. Lim, G. Cong, S. R. Young, M. Coletti, T. E. Potok and C. D. Schuman, 2022 IEEE/ACM Redefining Scalability for Diversely Heterogeneous Architectures Workshop (RSDHA): IEEE, 2022, p. 22–8.
- J. Wang, G. Cauwenberghs and F. D. Broccard, IEEE Trans. Biomed. Eng., 2019, 67, 1831–1840 Search PubMed.
- T. Dalgaty, M. Payvand, F. Moro, D. R. Ly, F. Pebay-Peyroula, J. Casas, G. Indiveri and E. Vianello, Apl Mater., 2019, 7, 081125 CrossRef.
- F. C. Bauer, D. R. Muir and G. Indiveri, IEEE Trans. Biomed. Circuits Syst., 2019, 13, 1575–1582 Search PubMed.
- S. Buccelli, Y. Bornat, I. Colombi, M. Ambroise, L. Martines, V. Pasquale, M. Bisio, J. Tessadori, P. Nowak and F. Grassia, IScience, 2019, 19, 402–414 CrossRef PubMed.
-
G. Tang, N. Kumar, R. Yoo and K. Michmizos, IEEE International Conference on Intelligent Robots and Systems (IROS), 2021.
- G. Haessig, X. Berthelon, S.-H. Ieng and R. Benosman, Sci. Rep., J. Mater. Chem., 2019, 9, 3744 Search PubMed.
-
M. Martini, N. Khan, Y. Bi, Y. Andreopoulos, H. Saki and M. Shikh-Bahaei, IEEE International Conference on Acoustics, Speech and Signal Processing (ICASSP), 2020.
- D. Moss, Proc. IEEE, 2022, 2022060179, DOI:10.20944/preprints202206.0179.v1.
- M. Bouvier, A. Valentian, T. Mesquida, F. Rummens, M. Reyboz, E. Vianello and E. Beigne, ACM J. Emerging Technol. Comput. Syst., 2019, 15, 1–35 CrossRef.
-
R. Shrestha, R. Bajracharya, A. Mishra and S. Kim, Artificial Intelligence and Hardware Accelerators, Springer, 2023, pp. 95–125 Search PubMed.
-
Y. Hui, J. Lien and X. Lu, International Symposium on Benchmarking, Measuring and Optimization, 2019.
-
Z. Pan and P. Mishra, arXiv, 2023, preprint, arXiv:2305.04887.
-
T. Gale, M. Zaharia, C. Young and E. Elsen, International Conference for High Performance Computing, Networking, Storage and Analysis, 2020.
-
K. Hazelwood, S. Bird, D. Brooks, S. Chintala, U. Diril, D. Dzhulgakov, M. Fawzy, B. Jia, Y. Jia and A. Kalro, IEEE International Symposium on High Performance Computer Architecture (HPCA), 2018.
-
S. Koppula, L. Orosa, A. G. Yağlıkçı, R. Azizi, T. Shahroodi, K. Kanellopoulos and O. Mutlu, Proceedings of the 52nd Annual IEEE/ACM International Symposium on Microarchitecture, 2019.
- E. E. Khoda, D. Rankin, R. T. de Lima, P. Harris, S. Hauck, S.-C. Hsu, M. Kagan, V. Loncar, C. Paikara and R. Rao, Mach. Learn.: Sci. Technol., 2023, 4, 025004 Search PubMed.
-
Z. Que, M. Loo, H. Fan, M. Pierini, A. Tapper and W. Luk, International Conference on Field-Programmable Logic and Applications (FPL), 2022.
-
J. Wang, Q. Lou, X. Zhang, C. Zhu, Y. Lin and D. Chen, ACM/SIGDA international symposium on field-programmable gate arrays, 2018.
- M. P. Véstias, IEEE, J. Solid State Circ., 2019, 12, 154 Search PubMed.
- M. A. Talib, S. Majzoub, Q. Nasir and D. Jamal, J. Supercomput., 2021, 77, 1897–1938 CrossRef.
-
Y. Chen, J. He, X. Zhang, C. Hao and D. Chen, Proceedings of the 2019 ACM/SIGDA International Symposium on Field-Programmable Gate Arrays, 2019, pp. 73–82 DOI:10.1145/3289602.3293915.
-
L. Song, F. Chen, Y. Zhuo, X. Qian, H. Li and Y. Chen, IEEE International Symposium on High Performance Computer Architecture (HPCA), 2020.
-
M. Abadi, P. Barham, J. Chen, Z. Chen, A. Davis, J. Dean, M. Devin, S. Ghemawat, G. Irving and M. Isard, OSDI'16: Proceedings of the 12th USENIX conference on Operating Systems Design and Implementation, 2016, pp. 265–283, https://dl.acm.org/doi/10.5555/3026877.3026899#sec-cit.
-
X. Wu, V. Saxena and K. Zhu, IEEE Neural Networks, 2015.
-
Z. Cai and X. Li, IEEE International Conference on Artificial Intelligence and Industrial Design (AIID), 2021.
- P. M. Solomon, Solid-State Electron., 2019, 155, 82–92 CrossRef CAS.
- D. Ivanov, A. Chezhegov, M. Kiselev, A. Grunin and D. Larionov, Front. Neurosci., 2022, 16, 1513 Search PubMed.
- S. F. Müller-Cleve, V. Fra, L. Khacef, A. Pequeño-Zurro, D. Klepatsch, E. Forno, D. G. Ivanovich, S. Rastogi, G. Urgese, F. Zenke and C. Bartolozzi, Braille letter reading: A benchmark for spatio-temporal pattern recognition on neuromorphic hardware, Front. Neurosci., 2022, 16, 951164 CrossRef PubMed.
-
J. C. Thiele, O. Bichler, A. Dupret, S. Solinas and G. Indiveri, International Joint Conference on Neural Networks (IJCNN), IEEE, 2019, pp. 1–8 DOI:10.1109/IJCNN.2019.8852360.
-
C. Ostrau, C. Klarhorst, M. Thies and U. Rückert, FastPath 2019 - International Workshop on Performance Analysis of Machine Learning Systems, Madison, Wisconsin, USA, 2019urn:nbn:de:0070-pub-29353281.
-
F. Barchi, G. Urgese, E. Macii and A. Acquaviva, 26th IFIP WG 10.5/IEEE International Conference on Very Large Scale Integration, 2019.
- C. D. Prado-Socorro, S. Giménez-Santamarina, L. Mardegan, L. Escalera-Moreno, H. J. Bolink, S. Cardona-Serra and E. Coronado, Adv. Electron. Mater., 2022, 8, 2101192 CrossRef CAS.
- Y.-H. Lin, C.-H. Wang, M.-H. Lee, D.-Y. Lee, Y.-Y. Lin, F.-M. Lee, H.-L. Lung, K.-C. Wang, T.-Y. Tseng and C.-Y. Lu, IEEE Trans. Electron Devices, 2019, 66, 1289–1295 CAS.
- K. Sozos, A. Bogris, P. Bienstman, G. Sarantoglou, S. Deligiannidis and C. Mesaritakis, Commun. Eng., 2022, 1, 24 CrossRef.
-
P. Stark, J. Weiss, R. Dangel, F. Horst, J. Geler-Kremer and B. J. Offrein, Optical Fiber Communication Conference, 2021 Search PubMed.
- W. Guo, M. E. Fouda, A. M. Eltawil and K. N. Salama, Front. Neurosci., 2021, 15, 638474 CrossRef PubMed.
- J. Park, J. Lee and D. Jeon, IEEE, J. Solid State Circ., 2019, 55, 108–119 Search PubMed.
-
A. Tripathi, M. Arabizadeh, S. Khandelwal and C. S. Thakur, IEEE International Symposium on Circuits and Systems (ISCAS), 2019.
-
P. Date, Combinatorial neural network training algorithm for neuromorphic computing, Rensselaer Polytechnic Institute, 2019 Search PubMed.
- X. Sheng, C. E. Graves, S. Kumar, X. Li, B. Buchanan, L. Zheng, S. Lam, C. Li and J. P. Strachan, Adv. Electron. Mater., 2019, 5, 1800876 CrossRef.
-
D. Moss, Proc. 11775 SPIE Optics + Optoelectronics Symposium, Prague (EOO21), OO107-8, 2021, 107, 11775-1, https://ssrn.com/abstract=3930751 Search PubMed.
- H.-L. Park and T.-W. Lee, Org. Electron., 2021, 98, 106301 CrossRef CAS.
- S. Yu, IEEE J. Explor. Solid-State Comput. Devices Circuits, 2019, 5, ii–iii Search PubMed.
-
M. Liehr, J. Hazra, K. Beckmann, W. Olin-Ammentorp, N. Cady, R. Weiss, S. Sayyaparaju, G. Rose and J. Van Nostrand, Proceedings of the International Conference on Neuromorphic Systems, 2019.
- E. Jokar, H. Abolfathi and A. Ahmadi, IEEE Trans. Biomed. Circuits Syst., 2019, 13, 454–469 Search PubMed.
-
M. Davies, Proceedings of Neuro Inspired Computing Elements, 2019 Search PubMed.
- C. D. Schuman, S. R. Kulkarni, M. Parsa, J. P. Mitchell, P. Date and B. Kay, Nat. Comput. Sci., 2022, 2, 10–19 CrossRef.
- Y. Zhan, R. C. Paolicelli, F. Sforazzini, L. Weinhard, G. Bolasco, F. Pagani, A. L. Vyssotski, A. Bifone, A. Gozzi and D. Ragozzino, Nat. Neurosci., 2014, 17, 400–406 CrossRef CAS PubMed.
- M. Stampanoni Bassi, E. Iezzi, L. Gilio, D. Centonze and F. Buttari, Int. J. Mol. Sci., 2019, 20, 6193 CrossRef PubMed.
- C. W. Lynn and D. S. Bassett, Nat. Rev. Phys., 2019, 1, 318–332 CrossRef.
- S. Marinelli, B. Basilico, M. C. Marrone and D. Ragozzino, Semin. Cell Dev. Biol., 2019, 94, 138–151 CrossRef PubMed.
- C. Seguin, O. Sporns and A. Zalesky, Nat. Rev. Neurosci., 2023, 1–18 Search PubMed.
- E. L. Lameu, E. E. Macau, F. Borges, K. C. Iarosz, I. L. Caldas, R. R. Borges, P. Protachevicz, R. L. Viana and A. M. Batista, Eur. Phys. J. Spec. Top., 2018, 227, 673–682 CrossRef.
- S. D. Glasgow, R. McPhedrain, J. F. Madranges, T. E. Kennedy and E. S. Ruthazer, Front. Synaptic Neurosci., 2019, 20 CrossRef CAS PubMed.
- D. Cosgrove, O. Mothersill, K. Kendall, B. Konte, D. Harold, I. Giegling, A. Hartmann, A. Richards, K. Mantripragada and M. Owen, Neuropsychopharmacology, 2017, 42, 2612–2622 CrossRef CAS PubMed.
- B. Sakmann, Exp. Physiol., 2017, 102, 489–521 CrossRef PubMed.
- J.-O. Hollnagel, T. Cesetti, J. Schneider, A. Vazetdinova, F. Valiullina-Rakhmatullina, A. Lewen, A. Rozov and O. Kann, iScience, 2020, 23, 101316 CrossRef CAS PubMed.
- F. Brückerhoff-Plückelmann, J. Feldmann, C. D. Wright, H. Bhaskaran and W. H. Pernice, J. Appl. Phys., 2021, 129, 151103 CrossRef.
-
J. Acharya, A. U. Caycedo, V. R. Padala, R. R. S. Sidhu, G. Orchard, B. Ramesh and A. Basu, 2019 32nd IEEE International System-on-Chip Conference
(SOCC), 2019, pp. 318–323 DOI:10.1109/SOCC46988.2019.1570553690.
- L. Steffen, D. Reichard, J. Weinland, J. Kaiser, A. Roennau and R. Dillmann, Front. Neurorob., 2019, 13, 28 CrossRef PubMed.
-
V. Baruzzi, G. Indiveri and S. P. Sabatini, Proceedings of the 15th International Joint Conference on Computer Vision, Imaging and Computer Graphics Theory and Applications, 2020.
- F. Sun, Q. Lu, S. Feng and T. Zhang, ACS Nano, 2021, 15, 3875–3899 CrossRef CAS PubMed.
- S. Oh, H. Hwang and I. Yoo, APL Mater., 2019, 7, 091109 CrossRef.
- A. Lakshmi, A. Chakraborty and C. S. Thakur, Wiley Interdiscip. Rev.: Data Min. Knowl. Discovery, 2019, 9, e1310 Search PubMed.
- T. Wan, S. Ma, F. Liao, L. Fan and Y. Chai, Sci. China Inf. Sci., 2022, 65, 1–14 Search PubMed.
- X. Peng, R. Liu, S. Yu and S. I. R. Papers, IEEE Trans. Circuits, 2019, 67, 1333–1343 Search PubMed.
-
M. Peemen, A. A. Setio, B. Mesman and H. Corporaal, IEEE 31st international conference on computer design (ICCD), 2013.
- Y. Chen, Y. Xie, L. Song, F. Chen and T. Tang, Eng. Failure Anal., 2020, 6, 264–274 Search PubMed.
- Y. S. Lee and T. H. Han, IEEE Access, 2021, 9, 68561–68572 Search PubMed.
- J. Wang, J. Lin and Z. Wang, IEEE Trans. Circuits Syst., 2017, 65, 1941–1953 Search PubMed.
-
Y. Ma, N. Suda, Y. Cao, J.-S. Seo and S. Vrudhula, Field programmable logic and applications (FPL), 2016.
-
C. Zhang, P. Li, G. Sun, Y. Guan, B. Xiao and J. Cong, ACM/SIGDA international symposium on field-programmable gate arrays, 2015.
- S. M. Nabavinejad, M. Baharloo, K.-C. Chen, M. Palesi, T. Kogel and M. Ebrahimi, IEEE J. Emerging Sel. Top. Circuits, 2020, 10, 268–282 Search PubMed.
- S. Ambrogio, P. Narayanan, H. Tsai, R. M. Shelby, I. Boybat, C. Di Nolfo, S. Sidler, M. Giordano, M. Bodini and N. C. Farinha, Nature, 2018, 558, 60–67 CrossRef CAS PubMed.
- A. Dundar, J. Jin, B. Martini and E. Culurciello, IEEE Trans. Neural Networks Learn. Syst., 2016, 28, 1572–1583 Search PubMed.
-
N. Suda, V. Chandra, G. Dasika, A. Mohanty, Y. Ma, S. Vrudhula, J.-S. Seo and Y. Cao, Proceedings of the ACM/SIGDA international symposium on field-programmable gate arrays, 2016.
-
S. Lym, E. Choukse, S. Zangeneh, W. Wen, S. Sanghavi and M. Erez, Proceedings of the International Conference for High Performance Computing, 2019.
-
D. Owen-Newns, W. Zhang, J. Alanis, J. Bueno, J. Robertson, M. Hejda and A. Hurtado, in Abstract Book of the 5th International Conference on Applications of Optics and Photonics, ed. M. F. P. C. M. Costa, 2022, pp. 146–147.
- L. Q. Guo, H. Han, L. Q. Zhu, Y. B. Guo, F. Yu, Z. Y. Ren, H. Xiao, Z. Y. Ge and J. N. Ding, ACS Appl. Mater. Interfaces, 2019, 11, 28352–28358 CrossRef CAS PubMed.
- M. Bernert and B. Yvert, Int. J. Neural Syst., 2019, 29, 1850059 CrossRef PubMed.
- J. D. Nunes, M. Carvalho, D. Carneiro and J. S. Cardoso, IEEE Access, 2022, 10, 60738–60764 Search PubMed.
- F. Osisanwo, J. Akinsola, O. Awodele, J. Hinmikaiye, O. Olakanmi and J. Akinjobi, Int. J. Computer Trends Technol., 2017, 48, 128–138 CrossRef.
-
A. Fischer and C. Igel, Computer Vision, and Applications: 17th Iberoamerican Congress, 2012.
- S. Höppner, B. Vogginger, Y. Yan, A. Dixius, S. Scholze, J. Partzsch, F. Neumärker, S. Hartmann, S. Schiefer, G. Ellguth and S. I. R. Papers, IEEE Trans. Circuits, 2019, 66, 2973–2986 Search PubMed.
-
D. K. Gopalakrishnan, A. Ravishankar and H. Abdi, Artificial Intelligence, Productivity Press, 2020, pp. 307–319 Search PubMed.
-
C. D. Schuman, J. S. Plank, G. Bruer and J. Anantharaj, International Joint Conference on Neural Networks (IJCNN), 2019.
-
M. Molendijk, K. Vadivel, F. Corradi, G.-J. van Schaik, A. Yousefzadeh and H. Corporaal, Industrial Artificial Intelligence Technologies and Applications, 2022, pp. 21–34 Search PubMed.
-
A. Yousefzadeh, S. Hosseini, P. Holanda, S. Leroux, T. Werner, T. Serrano-Gotarredona, B. L. Barranco, B. Dhoedt and P. Simoens, IEEE International Conference on Artificial Intelligence Circuits and Systems (AICAS), 2019.
- Q. Chen, X. Zhang, Y. Liu, Y. Yan, R. Yu, X. Wang, Z. Lin, H. Zeng, L. Liu and H. Chen, Nano Energy, 2022, 94, 106931 CrossRef CAS.
- R. G. Melko, G. Carleo, J. Carrasquilla and J. I. Cirac, Nat. Phys., 2019, 15, 887–892 Search PubMed.
-
V. R. Leite, Z. Su, A. M. Whatley and G. Indiveri, in Biomedical Circuits and Systems Conference, (BioCAS), 2022, IEEE, 2022, pp. 364–368.
- L. Shao, H. Wang, Y. Yang, Y. He, Y. Tang, H. Fang, J. Zhao, H. Xiao, K. Liang, M. Wei and W. Xu, ACS Appl. Mater. Interfaces, 2019, 11, 12161–12169 CrossRef CAS PubMed.
- Q. Xia and J. J. Yang, Nat. Mater., 2019, 18, 309–323 CrossRef CAS PubMed.
- J. Ajayan, D. Nirmal, B. K. Jebalin I.V and S. Sreejith, Microelectronics J, 2022, 130, 105634 CrossRef.
- B. R. Gaines, IEEE Des. Test, 2020, 38, 16–27 Search PubMed.
-
S.-Y. Sun, H. Xu, J. Li, H. Liu and Q. Li, International Joint Conference on Neural Networks (IJCNN), 2019.
- D. C. Mocanu, E. Mocanu, P. H. Nguyen, M. Gibescu and A. Liotta, Mach. Learn.: Sci. Technol., 2016, 104, 243–270 CrossRef.
-
J. N. Tripathi, B. Kumar and D. Junjariya, IEEE International Symposium on Circuits and Systems (ISCAS), 2022.
-
A. R. Aslam and M. A. B. Altaf, IEEE International Symposium on Circuits and Systems (ISCAS), 2019.
- Y. Alkabani, M. Miscuglio, V. J. Sorger and T. El-Ghazawi, IEEE Photonics J., 2020, 12, 1–14 Search PubMed.
-
S. Spiga, A. Sebastian, D. Querlioz and B. Rajendran, Memristive Devices for Brain-Inspired Computing: From Materials, Devices, and Circuits to Applications-Computational Memory, Deep Learning, and Spiking Neural Networks, Woodhead Publishing, 2020 Search PubMed.
- Y.-X. Hou, Y. Li, Z.-C. Zhang, J.-Q. Li, D.-H. Qi, X.-D. Chen, J.-J. Wang, B.-W. Yao, M.-X. Yu and T.-B. Lu, ACS Nano, 2020, 15, 1497–1508 CrossRef PubMed.
- A. Mehonic, A. Sebastian, B. Rajendran, O. Simeone, E. Vasilaki and A. J. Kenyon, Adv. Intell. Syst., 2020, 2, 2000085 CrossRef.
- S. Afshar, T. J. Hamilton, L. Davis, A. Van Schaik and D. J. I. S. J. Delic, IEEE Sens. J., 2020, 20, 7677–7691 CAS.
- A. Grübl, S. Billaudelle, B. Cramer, V. Karasenko and J. Schemmel, J. Signal Process. Syst., 2020, 92, 1277–1292 CrossRef.
- E. E. Tsur and M. Rivlin-Etzion, Neurocomputing, 2020, 374, 54–63 CrossRef.
-
C. Schuman, C. Rizzo, J. McDonald-Carmack, N. Skuda and J. Plank, Proceedings of the International Conference on Neuromorphic Systems, 2022.
- M. Wu, Q. Zhuang, K. Yao, J. Li, G. Zhao, J. Zhou, D. Li, R. Shi, G. Xu and Y. Li, InfoMat, 2023, e12472 CrossRef.
- M. Hejda, J. Robertson, J. Bueno and A. Hurtado, J. Phys.: Photonics, 2020, 2, 044001 Search PubMed.
- D. Zendrikov, S. Solinas and G. Indiveri, Neuromorphic Comput. Eng., 2023, 3, 034002 CrossRef.
- A. Vanarse, A. Osseiran and A. Rassau, IEEE Instrum. Meas. Mag., 2019, 22, 4–9 Search PubMed.
- N. Khan, K. Iqbal and M. G. Martini, IEEE Internet Things J., 2020, 8, 596–609 Search PubMed.
- J. Timcheck, S. B. Shrestha, D. B. D. Rubin, A. Kupryjanow, G. Orchard, L. Pindor, T. Shea and M. Davies, Neuromorphic Comput. Eng. Failure Anal., 2023, 3, 034005 CrossRef.
- G. Indiveri and S.-C. Liu, Proc. IEEE, 2015, 103, 1379–1397 CAS.
- P. C. Harikesh, C.-Y. Yang, D. Tu, J. Y. Gerasimov, A. M. Dar, A. Armada-Moreira, M. Massetti, R. Kroon, D. Bliman and R. Olsson, Nat. Commun., 2022, 13, 901 CrossRef CAS PubMed.
- T. Otero, J. Martinez and J. Arias-Pardilla, Electrochim. Acta, 2012, 84, 112–128 CrossRef CAS.
- E. Wlaźlak, D. Przyczyna, R. Gutierrez, G. Cuniberti and K. Szaciłowski, Jpn. J. Appl. Phys., 2020, 59, SI0801 CrossRef.
- R. Yu, E. Li, X. Wu, Y. Yan, W. He, L. He, J. Chen, H. Chen and T. Guo, ACS Appl. Mater. Interfaces, 2020, 12, 15446–15455 CrossRef CAS PubMed.
- G. Yushan, Z. Junyao, L. Dapeng, S. Tongrui, W. Jun, L. Li, D. Shilei, Z. Jianhua, Y. Zhenglong and H. Jia, Chin. Chem. Lett., 2023, 108582 CrossRef.
-
M. Ansari, S. M. A. Rizvi and S. Khan, International Conference on Electrical, Electronics, and Optimization Techniques (ICEEOT), 2016.
- J. Qiu, J. Cao, X. Liu, P. Chen, G. Feng, X. Zhang, M. Wang and Q. Liu, IEEE Electron Device Lett., 2022, 44, 176–179 Search PubMed.
- P. Monalisha, A. P. Kumar, X. R. Wang and S. Piramanayagam, ACS Appl. Mater. Interfaces, 2022, 14, 11864–11872 CrossRef CAS PubMed.
-
S. E. Lee, S. B. Simons, S. A. Heldt, M. Zhao, J. P. Schroeder, C. P. Vellano, D. P. Cowan, S. Ramineni, C. K. Yates and Y. Feng, Proc. Natl. Acad. Sci., 2010, 107, 16994–16998 Search PubMed.
- H. Chun, J. Kim, J. Yu and S. Han, IEEE Access, 2020, 8, 81789–81799 Search PubMed.
- H. Wang, Q. Zhao, Z. Ni, Q. Li, H. Liu, Y. Yang, L. Wang, Y. Ran, Y. Guo and W. Hu, Adv. Mater., 2018, 30, 1803961 CrossRef PubMed.
- H. Lee, D. G. Ryu, G. Lee, M. K. Song, H. Moon, J. Lee, J. Ryu, J. H. Kang, J. Suh and S. Kim, Adv. Electron. Mater., 2022, 8, 2200378 CrossRef CAS.
- X. Chen, B. Chen, P. Zhao, V. A. Roy, S.-T. Han and Y. Zhou, Mater. Futures, 2023, 2, 023501 CrossRef.
-
W. Chung, M. Si and D. Y. Peide, IEEE International Electron Devices Meeting (IEDM), 2018.
- C. Ebenhoch and L. Schmidt-Mende, Adv. Electron. Mater., 2021, 7, 2000950 CrossRef CAS.
- X. Chen, B. Chen, B. Jiang, T. Gao, G. Shang, S. T. Han, C. C. Kuo, V. A. Roy and Y. Zhou, Adv. Funct. Mater., 2023, 33, 2208807 CrossRef CAS.
- C. Yoon, G. Oh and B. H. Park, Nanomaterials, 2022, 12, 1728 CrossRef CAS PubMed.
- M. L. Varshika, F. Corradi and A. Das, Electronics, 2022, 11, 1610 CrossRef.
- S. Umesh and S. Mittal, J. Syst. Archit., 2019, 97, 349–372 CrossRef.
- D. Chabi, D. Querlioz, W. Zhao and J.-O. Klein, ACM J. Emerging Technol. Comput. Syst., 2014, 10, 1–20 CrossRef.
- D. Wang, S. Zhao, R. Yin, L. Li, Z. Lou and G. Shen, Npj Flexible Electron., 2021, 5, 13 CrossRef CAS.
- G. Milano, E. Miranda, M. Fretto, I. Valov and C. Ricciardi, ACS Appl. Mater. Interfaces, 2022, 14, 53027–53037 CrossRef CAS PubMed.
- Z. L. Wang, Adv. Energy Mater., 2020, 10, 2000137 CrossRef CAS.
- P.-Y. Feng, Z. Xia, B. Sun, X. Jing, H. Li, X. Tao, H.-Y. Mi and Y. Liu, ACS Appl. Mater. Interfaces, 2021, 13, 16916–16927 CrossRef CAS PubMed.
- J. K. Han, I. W. Tcho, S. B. Jeon, J. M. Yu, W. G. Kim and Y. K. Choi, Adv. Sci., 2022, 9, 2105076 CrossRef CAS PubMed.
- S. A. Han, W. Seung, J. H. Kim and S.-W. Kim, ACS Energy Lett., 2021, 6, 1189–1197 CrossRef CAS.
- Z. L. Wang, T. Jiang and L. Xu, Nano Energy, 2017, 39, 9–23 CrossRef CAS.
- Y. Zi, J. Wang, S. Wang, S. Li, Z. Wen, H. Guo and Z. L. Wang, Nat. Commun., 2016, 7, 10987 CrossRef CAS PubMed.
- K. Dong and Z. L. Wang, J. Semicond., 2021, 42, 101601 CrossRef CAS.
- Y. Han, W. Wang, J. Zou, Z. Li, X. Cao and S. Xu, Nano Energy, 2020, 76, 105008 CrossRef CAS.
- X. Cheng, W. Tang, Y. Song, H. Chen, H. Zhang and Z. L. Wang, Nano Energy, 2019, 61, 517–532 CrossRef CAS.
- S. Niu, Y. S. Zhou, S. Wang, Y. Liu, L. Lin, Y. Bando and Z. L. Wang, Nano Energy, 2014, 8, 150–156 CrossRef CAS.
- G. Milano, G. Pedretti, K. Montano, S. Ricci, S. Hashemkhani, L. Boarino, D. Ielmini and C. Ricciardi, Nat. Mater., 2022, 21, 195–202 CrossRef CAS PubMed.
- Y. Lv, H. Chen, Q. Wang, X. Li, C. Xie and Z. Song, Front. Neurorob., 2022, 16, 948386 CrossRef PubMed.
- M. Payvand, F. Moro, K. Nomura, T. Dalgaty, E. Vianello, Y. Nishi and G. Indiveri, Nat. Commun., 2021, 13, 1–12 Search PubMed.
-
M. S. Hasan, C. D. Schuman, J. S. Najem, R. Weiss, N. D. Skuda, A. Belianinov, C. P. Collier, S. A. Sarles and G. S. Rose, IEEE 13th Dallas Circuits and Systems Conference (DCAS), 2018.
- Y. Li and K.-W. Ang, Adv. Intell. Syst., 2021, 3, 2000137 CrossRef.
-
T. Marukame, J. Sugino, T. Kitamura, K. Ishikawa, K. Takahashi, Y. Tamura, R. Berdan, K. Nomura and Y. Nishi, IEEE International Symposium on Circuits and Systems (ISCAS), 2019.
-
J. Liu, H. Huo, W. Hu and T. Fang, International Conference on Machine Learning and Computing, 2018.
- N. Garg, I. Balafrej, T. C. Stewart, J.-M. Portal, M. Bocquet, D. Querlioz, D. Drouin, J. Rouat, Y. Beilliard and F. Alibart, Front. Neurosci., 2022, 16, 983950 CrossRef PubMed.
- A. Y. Morozov, K. K. Abgaryan and D. L. Reviznikov, Chaos, Solitons Fractals, 2021, 143, 110548 CrossRef.
- P. Subin, P. Midhun, A. Antony, K. Saji and M. Jayaraj, Mater. Today Commun., 2022, 33, 104232 CrossRef CAS.
- A. Williamson, L. Schumann, L. Hiller, F. Klefenz, I. Hoerselmann, P. Husar and A. Schober, Nanoscale, 2013, 5, 7297–7303 RSC.
- T. Guo, B. Sun, S. Ranjan, Y. Jiao, L. Wei, Y. N. Zhou and Y. A. Wu, ACS Appl. Mater. Interfaces, 2020, 12, 54243–54265 CrossRef CAS PubMed.
- M. Kumar, J. Lim, S. Kim and H. Seo, ACS Nano, 2020, 14, 14108–14117 CrossRef CAS PubMed.
-
G. Tang, N. Kumar and K. P. Michmizos, IEEE/RSJ International Conference, IEEE International Conference on Intelligent Robots and Systems (IROS), 2020.
-
R. Massa, A. Marchisio, M. Martina and M. Shafique, International Joint Conference on Neural Networks (IJCNN), 2020.
- S. Dutta, C. Schafer, J. Gomez, K. Ni, S. Joshi and S. Datta, Front. Neurosci., 2020, 14, 634 CrossRef PubMed.
- S. W. Cho, S. M. Kwon, Y.-H. Kim and S. K. Park, Adv. Intell. Syst., 2021, 3, 2000162 CrossRef.
- A. Lugnan, A. Katumba, F. Laporte, M. Freiberger, S. Sackesyn, C. Ma, E. Gooskens, J. Dambre and P. Bienstman, APL Photonics, 2020, 5, 020901 CrossRef.
- T. Ferreira De Lima, A. N. Tait, A. Mehrabian, M. A. Nahmias, C. Huang, H.-T. Peng, B. A. Marquez, M. Miscuglio, T. El-Ghazawi and V. J. Sorger, Nanophotonics, 2020, 9, 4055–4073 CrossRef CAS.
- Q.-X. Li, T.-Y. Wang, X.-L. Wang, L. Chen, H. Zhu, X.-H. Wu, Q.-Q. Sun and D. W. Zhang, Nanoscale, 2020, 12, 23150–23158 RSC.
- Y. Gong, Y. Wang, R. Li, J.-Q. Yang, Z. Lv, X. Xing, Q. Liao, J. Wang, J. Chen and Y. Zhou, J. Mater. Chem., 2020, 8, 2985–2992 CAS.
- Z. Xu, Y. Bando, W. Wang, X. Bai and D. Golberg, ACS Nano, 2010, 4, 2515–2522 CrossRef CAS PubMed.
- F. Zhang, H. Zhang, S. Krylyuk, C. A. Milligan, Y. Zhu, D. Y. Zemlyanov, L. A. Bendersky, B. P. Burton, A. V. Davydov and J. Appenzeller, Nat. Mater., 2019, 18, 55–61 CrossRef CAS PubMed.
- X. Zhu, D. Li, X. Liang and W. D. Lu, Nat. Mater., 2019, 18, 141–148 CrossRef CAS PubMed.
- S. Subramanian Periyal, M. Jagadeeswararao, S. E. Ng, R. A. John and N. Mathews, Adv. Mater. Technol., 2020, 5, 2000514 CrossRef CAS.
- X. Zhang, Y. Zhou, K. M. Song, T.-E. Park, J. Xia, M. Ezawa, X. Liu, W. Zhao, G. Zhao and S. Woo, J. Phys.: Condens. Matter, 2020, 32, 143001 CrossRef CAS PubMed.
- R. Stagsted, A. Vitale, J. Binz, L. Bonde Larsen and Y. Sandamirskaya, Robot. Sci. Syst., 2020, 74–82 Search PubMed.
-
O. Moreira, A. Yousefzadeh, F. Chersi, A. Kapoor, R.-J. Zwartenkot, P. Qiao, G. Cinserin, M. A. Khoei, M. Lindwer and J. Tapson, 2nd IEEE International Conference on Artificial Intelligence Circuits and Systems (AICAS), 2020.
- Q. Yu, S. Li, H. Tang, L. Wang, J. Dang and K. C. Tan, IEEE Trans. Cybern., 2020, 52, 1364–1376 Search PubMed.
- N. L. Ing, M. Y. El-Naggar and A. I. Hochbaum, J. Phys. Chem., 2018, 122, 10403–10423 CrossRef CAS PubMed.
- K. L. Jiménez-Monroy, N. Renaud, J. Drijkoningen, D. Cortens, K. Schouteden, C. Van Haesendonck, W. J. Guedens, J. V. Manca, L. D. Siebbeles and F. C. Grozema, J. Phys. Chem. A, 2017, 121, 1182–1188 CrossRef PubMed.
- M. Xie, L. Li, Y. Zhang, J. Du, Y. Li, Y. Shan and H. Zheng, Ionics, 2020, 26, 1109–1117 CrossRef CAS.
- X. Fu, T. Li, B. Cai, J. Miao, G. N. Panin, X. Ma, J. Wang, X. Jiang, Q. Li and Y. Dong, Light: Sci. Appl., 2023, 12, 39 CrossRef CAS PubMed.
- K. M. Oikonomou, I. Kansizoglou and A. Gasteratos, Machines, 2023, 11, 162 CrossRef.
- I. Polykretis, L. Supic and A. Danielescu, Neuromorphic Comput. Eng. Failure Anal., 2023, 3, 014013 CrossRef.
- F. Huang, F. Fang, Y. Zheng, Q. You, H. Li, S. Fang, X. Cong, K. Jiang, Y. Wang and C. Han, Nano Res., 2023, 16, 1304–1312 CrossRef CAS.
- J. Timchek, S. B. Shrestha, D. B. D. Rubin, A. Kupryjanow, G. Orchard, L. Pindor, T. Shea and M. Davies, Neuromorph. Comput. Eng., 2023, 3, 034005 CrossRef.
- A. Ussa, C. S. Rajen, T. Pulluri, D. Singla, J. Acharya, G. F. Chuanrong, A. Basu and B. Ramesh, IEEE Trans. Neural Networks Learn. Syst., 2023 DOI:10.48550/arXiv.1910.09806.
- M. U. Khan, J. Kim, M. Y. Chougale, R. A. Shaukat, Q. M. Saqib, S. R. Patil, B. Mohammad and J. Bae, Adv. Intell. Syst., 2023, 5, 2200281 CrossRef.
- N. Prudnikov, S. Malakhov, V. Kulagin, A. Emelyanov, S. Chvalun, V. Demin and V. Erokhin, Biomimetics, 2023, 8, 189 CrossRef CAS PubMed.
- J. Chung, K. Park, G. I. Kim, J. B. An, S. Jung, D. H. Choi and H. J. Kim, Appl. Surf. Sci., 2023, 610, 155532 CrossRef CAS.
- K. Udaya Mohanan, S. Cho and B.-G. Park, Appl. Intell., 2023, 53, 6288–6306 CrossRef.
- M. Mozafari, M. Ganjtabesh, A. Nowzari-Dalini, S. J. Thorpe and T. Masquelier, Pattern Recognition, 2019, 94, 87–95 CrossRef.
- H. Hazan, D. J. Saunders, D. T. Sanghavi, H. Siegelmann and R. Kozma, Ann. Math. Artif. Intell., 2020, 88, 1237–1260 CrossRef.
-
S. Kim, S. Park, B. Na and S. Yoon, Proceedings of the AAAI conference on artificial intelligence, 2020.
- B. Chakraborty, X. She and S. Mukhopadhyay, IEEE Trans. Image Process., 2021, 30, 9014–9029 Search PubMed.
- F. Galluppi, J. Conradt, T. Stewart, C. Eliasmith, T. Horiuchi, J. Tapson, B. Tripp, S. Furber and R. Etienne-Cummings, IEEE Biomedical Circuits and Systems Conference (BioCAS), 2012, 91, DOI:10.1109/BioCAS.2012.6418493.
-
Z. Jiang, R. Otto, Z. Bing, K. Huang and A. Knoll, IEEE/RSJ International Conference on Intelligent Robots and Systems (IROS), 2020.
-
C. M. Parameshwara, S. Li, C. Fermüller, N. J. Sanket, M. S. Evanusa and Y. Aloimonos, 2021 IEEE/RSJ International Conference on Intelligent Robots and Systems (IROS), 2021, pp. 3414–3420 DOI:10.1109/IROS51168.2021.9636506.
-
C. M. Parameshwara, S. Li, C. Fermüller, N. J. Sanket, M. S. Evanusa and Y. Aloimonos, 2022 IEEE International Meeting for Future of Electron Devices, Kansai (IMFEDK), 2021, pp. 1–5 DOI:10.1109/IMFEDK56875.2022.9975370.
-
R. Kabrick, D. Roa, S. Raskar, J. M. M. Diaz and G. Gao, Univ. Delaware, Newark, DE, USA, Tech. Rep. CAPSL Technical Memo, 2020, 136.
-
M. Descour, D. Stracuzzi, J. Tsao, J. Weeks, A. Wakeland, D. Schultz and W. Smith, AI-Enhanced Co-Design for Next-Generation Microelectronics: Innovating Innovation (Workshop Report), Sandia National Lab. (SNL-NM), Albuquerque, NM (United States), 2021.
- Y. Zhang, P. Qu and W. Zheng, Tsinghua Sci. Technol., 2021, 26, 664–673 Search PubMed.
-
J. Ang, A. A. Chien, S. D. Hammond, A. Hoisie, I. Karlin, S. Pakin, J. Shalf and J. S. Vetter, Reimagining codesign for advanced scientific computing: Report for the ascr workshop on reimagining codesign, USDOE Office of Science (SC) (United States), 2022.
- S. Zhu, T. Yu, T. Xu, H. Chen, S. Dustdar, S. Gigan, D. Gunduz, E. Hossain, Y. Jin and F. Lin, Intell. Comput., 2023, 2, 0006 CrossRef.
- Y. Chen, H. H. Li, C. Wu, C. Song, S. Li, C. Min, H.-P. Cheng, W. Wen and X. Liu, Integration, 2018, 61, 49–61 CrossRef.
- M. M. Ziegler, K. Kailas, X. Zhang and R. V. Joshi, IEEE J. Emerging Sel. Top. Circuits Syst., 2019, 9, 435–438 Search PubMed.
-
E. E. Tsur, Neuromorphic Engineering: The Scientist's, Algorithms Designer's and Computer Architect's Perspectives on Brain-Inspired Computing, CRC Press, 2021 Search PubMed.
- V. K. Sangwan, S. E. Liu, A. R. Trivedi and M. C. Hersam, Matter, 2022, 5, 4133–4152 CrossRef.
-
S. Yu, in Neuro-inspired Computing Using Resistive Synaptic Devices, Springer, 2017, pp. 1–15 Search PubMed.
- G. Li, L. Deng, H. Tang, G. Pan, Y. Tian, K. Roy and W. Maass, TechRxiv. Preprint., 2023 DOI:10.36227/techrxiv.21837027.v1.
-
G. Finocchio, S. Bandyopadhyay, P. Lin, G. Pan, J. J. Yang, R. Tomasello, C. Panagopoulos, M. Carpentieri, V. Puliafito and J. Åkerman, arXiv, 2023, preprint arXiv:2301.06727 DOI:10.48550/arXiv.2301.06727.
-
A. Iosup, F. Kuipers, A. L. Varbanescu, P. Grosso, A. Trivedi, J. Rellermeyer, L. Wang, A. Uta and F. Regazzoni, arXiv, 2022, preprint arXiv:2206.03259 DOI:10.48550/arXiv.2206.03259.
-
G. Cauwenberghs, J. Cong, X. S. Hu, S. Joshi, S. Mitra, W. Porod and H.-S. P. Wong, Proc. IEEE, 2023, 111, 561–574 Search PubMed.
-
G. K. Thiruvathukal, Y.-H. Lu, J. Kim, Y. Chen and B. Chen, Low-power computer vision: improve the efficiency of artificial intelligence, CRC Press, 2022 Search PubMed.
- T. Baba, Y.-a Shimada, S. Kawamura, M. Matoba, T. Fukushima, S. Fujii, T. Nagano, Y. Katsumata, N. Kochi and Y. Kimura, Jpn. J. Appl. Phys., 2020, 59, 050503 CrossRef CAS.
- L. Witt, M. Heyer, K. Toyoda, W. Samek and D. Li, IEEE Internet Things J., 2022, 3642–3663, DOI:10.1109/JIOT.2022.3231363.
- W. Liu, Z. Wang, X. Liu, N. Zeng, Y. Liu and F. E. Alsaadi, Neurocomputing, 2017, 234, 11–26 CrossRef.
-
X. He, T. Liu, F. Hadaeghi and H. Jaeger, The 9th International IEEE EMBS Conference on Neural Engineering (NER), San Francisco, CA, USA, 2019, https://tianlinliu.com/files/poster_ner2019.pdf.
-
J. Knechtel, Hardware security for and beyond CMOS technology: an overview on fundamentals, applications, and challenges, in Proceedings of the 2020 International Symposium on Physical Design ACM, 2020, pp. 75–86.
- J. Partzsch and R. Schuffny, IEEE Trans. Neural Networks, 2011, 22, 919–935 Search PubMed.
- C. Ostrau, C. Klarhorst, M. Thies and U. Rückert, Front. Neurosci., 2022, 16, 873935 CrossRef PubMed.
- J. Hasler and B. Marr, Front. Neurosci., 2013, 7, 118 Search PubMed.
-
T. Oess, M. Löhr, C. Jarvers, D. Schmid and H. Neumann, 2nd IEEE International Conference on Artificial Intelligence Circuits and Systems (AICAS), 2020.
- D. Brüderle, E. Müller, A. P. Davison, E. Muller, J. Schemmel and K. Meier, Front. Neuroinf., 2009, 3, 362 Search PubMed.
-
P. U. Diehl and M. Cook, International Joint Conference on Neural Networks (IJCNN), 2014.
- R. Wang, C. S. Thakur, G. Cohen, T. J. Hamilton, J. Tapson and A. van Schaik, IEEE Trans. Biomed. Circuits Syst., 2017, 11, 574–584 Search PubMed.
-
P. U. Diehl, G. Zarrella, A. Cassidy, B. U. Pedroni and E. Neftci, IEEE International Conference on Rebooting Computing (ICRC), 2016.
- G. Indiveri and Y. Sandamirskaya, IEEE Signal Process. Mag., 2019, 36, 16–28 Search PubMed.
- A. Kurenkov, S. Fukami and H. Ohno, J. Appl. Phys., 2020, 128, 010902, DOI:10.1063/5.0009482.
- N. Risi, A. Aimar, E. Donati, S. Solinas and G. Indiveri, Front. Neurorob., 2020, 14, 568283 CrossRef PubMed.
- C. M. Niu, S. K. Nandyala and T. D. Sanger, Front. Comput. Neurosci., 2014, 8, 141 Search PubMed.
- S. Davies, F. Galluppi, A. D. Rast and S. B. Furber, Neural Networks, 2012, 32, 3–14 CrossRef CAS PubMed.
- M. Osswald, S.-H. Ieng, R. Benosman and G. Indiveri, Sci. Rep., 2017, 7, 40703 CrossRef CAS PubMed.
- S. Moradi and R. Manohar, J. Phys. D: Appl. Phys., 2018, 52, 014003 CrossRef.
- M. Ronchini, Y. Rezaeiyan, M. Zamani, G. Panuccio and F. Moradi, J. Neural Eng., 2023, 20, 036002 CrossRef PubMed.
- Y. Cai, F. Wang, X. Wang, S. Li, Y. Wang, J. Yang, T. Yan, X. Zhan, F. Wang and R. Cheng, Adv. Funct. Mater., 2023, 33, 2212917 CrossRef CAS.
- N. R. Kheirabadi, A. Chiolerio, K. Szaciłowski and A. Adamatzky, Chem. Phys. Chem., 2023, 24, e202200390 CrossRef CAS PubMed.
- F. Wen, C. Wang and C. Lee, Nano Res., 2023, 16, 11801–11821 CrossRef.
-
P. Agarwal and M. Alam, 7th International Conference on Intelligent Computing and Control Systems (ICICCS), 2023.
- J. Chen, N. Skatchkovsky and O. Simeone, IEEE Trans. Cognit. Commun. Networking, 2023, 9(2), 252–265 Search PubMed.
-
Y. Sun, J. Wu, M. Tan, X. Xu, Y. Li, R. Morandotti, A. Mitchell and D. Moss, in CLEO 2023, Technical Digest Series (Optica Publishing Group, 2023), paper SM1P.1, https://doi.org/10.1364/CLEO_SI.2023.SM1P.1 Search PubMed.
-
N. Zins, Y. Zhang, C. Yu and H. An, Frontiers of Quality Electronic Design (QED) AI, IoT and Hardware Security, Springer, 2023, pp. 259–296 Search PubMed.
- T. Lim, S. Lee, J. Lee, H. Choi, B. Jung, S. Baek and J. Jang, Adv. Funct. Mater., 2023, 33, 2212367 CrossRef CAS.
- D. Kumar, H. Li, U. K. Das and A. M. SyedEl-Atab, Adv. Mater., 2023, 2300446 CrossRef CAS PubMed.
- A. Bicaku, M. Sapounaki, A. Kakarountas and S. K. Tasoulis, J. Low Power Electron. Appl., 2023, 13, 10 CrossRef.
- D. L. Manna, A. Vicente-Sola, P. Kirkland, T. J. Bihl and G. Di Caterina, Eng. Appl. Neural Networks, 2023, 2, 044009, DOI:10.1088/2634-4386/ac999b.
- A. Hazan, B. Ratzker, D. Zhang, A. Katiyi, M. Sokol, Y. Gogotsi and A. Karabchevsky, Adv. Mater., 2023, 2210216 CrossRef CAS PubMed.
- S. Battistoni, R. Carcione, E. Tamburri, V. Erokhin, M. L. Terranova and S. Iannotta, Adv. Mater. Technol., 2023, 2201555 CrossRef CAS.
Footnote |
† Authors equally contributed. |
|
This journal is © The Royal Society of Chemistry 2023 |
Click here to see how this site uses Cookies. View our privacy policy here.