DOI:
10.1039/D3NR03842D
(Paper)
Nanoscale, 2024,
16, 664-677
Recording physiological and pathological cortical activity and exogenous electric fields using graphene microtransistor arrays in vitro†
Received
1st August 2023
, Accepted 7th November 2023
First published on 15th December 2023
Abstract
Graphene-based solution-gated field-effect transistors (gSGFETs) allow the quantification of the brain's full-band signal. Extracellular alternating current (AC) signals include local field potentials (LFP, population activity within a reach of hundreds of micrometers), multiunit activity (MUA), and ultimately single units. Direct current (DC) potentials are slow brain signals with a frequency under 0.1 Hz, and commonly filtered out by conventional AC amplifiers. This component conveys information about what has been referred to as “infraslow” activity. We used gSGFET arrays to record full-band patterns from both physiological and pathological activity generated by the cerebral cortex. To this end, we used an in vitro preparation of cerebral cortex that generates spontaneous rhythmic activity, such as that occurring in slow wave sleep. This examination extended to experimentally induced pathological activities, including epileptiform discharges and cortical spreading depression. Validation of recordings obtained via gSGFETs, including both AC and DC components, was accomplished by cross-referencing with well-established technologies, thereby quantifying these components across different activity patterns. We then explored an additional gSGFET potential application, which is the measure of externally induced electric fields such as those used in therapeutic neuromodulation in humans. Finally, we tested the gSGFETs in human cortical slices obtained intrasurgically. In conclusion, this study offers a comprehensive characterization of gSGFETs for brain recordings, with a focus on potential clinical applications of this emerging technology.
1. Introduction
Many neurological conditions (e.g., stroke, traumatic brain injury, neurodegeneration, epilepsy, chronic pain) frequently exhibit alterations in brain rhythms and activity patterns. A common strategy for treatment is the restoration of physiological activity in these pathologies. For this reason, there is an urgent clinical need for the precise recording, modulation, and restoration of neural activity in the form of novel neural interfaces, which can benefit from state-of-the-art technological developments in a variety of fields, nanotechnology being a critical one.
Brain electrophysiology is the study of the electrical properties of neurons and networks ranging from whole brain electroencephalograms to single ionic channel recordings. It has been a key technique for understanding brain physiology and pathology. Historically, passive electrodes have been the most commonly used transducers for recording brain activity; however, they are highly susceptible to DC offsets and low-frequency drifts present at the electrode tissue interface, impairing the high-fidelity recording of the brain's infraslow activity and introducing high common voltage offsets. This limitation led to the standardized use of high-pass filter commercial amplifiers for extracellular recordings, while DC amplifiers were to a large extent restricted to intracellular recordings. It is for this reason that the DC or infraslow components (<1 Hz) of the extracellular signals have been widely overlooked until relatively recently.
However, from the beginning of the twenty-first century, brain imaging studies began to report on the high relevance of infraslow components for brain states, neural dynamics, cognition, and disease processes.1,2 Infraslow potentials can be physiological and linked to normal neural dynamics, like sleep,3 or as a critical mechanism for long-range communication across cortical areas.1 They are also an important marker of pathology: electrophysiological studies of seizures have reported negative DC-shifts during depolarization of pyramidal neurons, transitioning into positive shifts during the postictal period.4 Further, DC-shifts have been reported preceding the occurrence of seizures.5,6 The negative infraslow potential has also been reported as an electrophysiological correlate of infarction in human cerebral cortex.7 In recent years, graphene solution-gated field-effect transistors (gSGFETs) have emerged as a promising solution for obtaining full-band or DC-coupled recordings as well as information about the infraslow components.8 These transistors exhibit high sensitivity to local electrical potential changes, making them capable of detecting subtle variations in the electric field generated by neuronal activity.9,10 gSGFET arrays have proven useful for recording from the cerebral cortex in vivo.11,12 To our knowledge, gSGFETs have not been used to record from spontaneously active cortical slices in vitro.13,14 This preparation method offers an experimentally accessible view into the intrinsic cortical mechanisms, not only for physiological activity patterns such as slow oscillations, which are highly similar to slow waves as in slow wave sleep,15 but also for the generation of pathological patterns such as epileptiform discharges,16 and cortical spreading depression (CSD).
An increasingly common therapeutical strategy is to modulate exogenously cortical activity using transcranial direct current stimulation (tDCS), a non-invasive brain stimulation technique that involves applying electrical currents through scalp electrodes. This technique has earned significant interest as a potential therapeutic approach for various neuropsychiatric disorders.17–19 To fully control its therapeutic potential, it is crucial to enhance our understanding of the precise effects of tDCS, the intensity of the fields that actually reach the brain and their interactions with endogenous fields.20
In the current study, we have used gSGFET arrays for the first time, to our knowledge, to record physiological and pathological emergent patterns from in vitro-maintained cortical slices. This accessible preparation has allowed us to, firstly, validate the full-band recordings by obtaining simultaneous recordings using traditional passive recording techniques. Next, we systematically quantified the commonly overlooked infraslow component associated with physiological slow waves and pathological patterns such as pre-epileptic events, epileptiform activity, and cortical spreading depression. This exploration has led us to the discovery that the DC-shifts preceding epileptiform discharges occur not only in vivo but also in vitro, strongly suggesting that the origin is rooted in the local circuitry. This finding holds significant physiological relevance.
Additionally, we incorporated cortical slices from human tissue obtained during surgery into our sample. These slices were recorded for the first time using gSGFET arrays, providing valuable insights into the AC- and DC-coupled components. Finally, we harnessed the unique properties of gSGFET arrays to investigate a novel potential application—precisely measuring the exogenous electric field applied during tDCS. This measurement can be highly relevant for the design of closed-loop circuits aimed at precisely modulating brain activity.21 This comprehensive characterization of gSGFETs for recording cerebral cortex activity serves as the foundation for exploring potential clinical applications of this technique, bringing it one step closer to validation for clinical use.
2. Methods
2.1 Preparation of ferret cortical slices
Ferrets were treated in accordance with the European Union guidelines on the protection of vertebrates used for experimentation (Directive 2010/63/EU of the European Parliament and of the council of 22 September 2010). All experiments were approved by the ethics committee of the University of Barcelona. Ferrets (4–10 months old; either sex) were deeply anesthetized with isoflurane and a combination of 8 mg kg−1 of ketamine with 0.1 mg kg−1 of medetomidine before decapitation. The brain was quickly removed and placed in an ice-cold sucrose solution containing (in mM): 213 sucrose, 2.5 KCl, 1 NaH2PO4, 26 NaHCO3, 1 CaCl2, 3 MgSO4 and 10 glucose and acute coronal slices (400-μm-thick) of the occipital cortex containing visual cortical areas 17, 18, and 19 from both hemispheres were obtained.
Slices were placed in an interface-style recording chamber (Fine Science Tools, Foster City, CA) and superfused with an equal mixture of the above-mentioned sucrose solution and artificial cerebrospinal fluid (ACSF) for 30 min. The ACSF contained (in mM): 126 NaCl, 2.5 KCl, 1 NaH2PO4, 26 NaHCO3, 2 CaCl2, 2 MgSO4 and 10 glucose. Next, slices were bathed with ACSF for 1 h for stabilization. For slow oscillatory activity to spontaneously emerge, slices were superfused for at least 30 min before starting the electrophysiological recordings with an in vivo-like modified ACSF containing (in mM): 126 NaCl, 4 KCl, 1 NaH2PO4, 26 NaHCO3, 1 CaCl2, 1 MgSO4 and 10 glucose. All solutions were saturated with carbogen (95%O2 5%CO2) to a final pH of 7.4 at 34 °C.
2.2 Preparation of human cortical slices
Human cortical tissue samples were obtained from Hospital Clinic de Barcelona following surgery of patients that required temporal resection due to refractory epilepsy or tumor biopsy. Slices of 400 μm thickness were prepared following the same protocol described above for ferret slices, except for the addition of 3 mM sodium pyruvate and 0.5 mM ascorbic acid to the sucrose-substituted solution. Following electrophysiological recording, human slices were preserved in paraformaldehyde and sent for histological processing to obtain a reconstruction of the cortical layers. Ethical approval was obtained from the Hospital Clinic de Barcelona ethical committee.
2.3 Electrophysiological recordings of cortical slices in vitro
A variety of techniques were used for the electrophysiological recordings, including gSGFETs and other approaches for validation that are described next.
2.3.1 Full-bandwidth recordings with 16-channel graphene arrays of microtransistors (gSGFETs).
Full-bandwidth electrophysiological recordings were performed with flexible neural probes containing an array of 16 gSGFETs (4 × 4 array, 400-μm separation). Whereas most currently available electrodes are passive, gSGFETs are active devices that transduce local voltage changes to current, enabling full-bandwidth electrophysiological recordings.9 Neural probes were fabricated at the clean room facilities of IMB-CNM as reported by Hebert et al.8 In brief, single-layer graphene was grown by chemical vapor deposition and transferred to a silicon wafer previously coated with a polyimide layer and patterned metal traces. After defining the graphene channels (50 × 50 μm2) and before evaporating a second metal layer, UVO treatment was applied to improve the graphene-metal interface and reduce its contact resistance.22 Finally, SU-8 was used as a passivation layer and the polyimide layer was etched to define the geometry of the neural probes. Devices were gently peeled off from the wafer and inserted to zero insertion force connectors for electronic interfacing. A custom g.HIamp biosignal amplifier, (g.RAPHENE, g.tec medical engineering GmbH Austria) was used for signal acquisition at 9.6 kHz and 24 bits. The system enables simultaneous recording in two frequency bands with different gains preventing amplifier saturation; low-pass filter (LPF) (f <0.16 Hz, 104 gain) and band-pass filter (BPF) (0.16 Hz < f < 6 kHz, 106 gain).
2.3.2 Local field potential recording using 16-channel black platinum multielectrode arrays.
Extracellular local field potentials (LFP) were recorded using a 16-channel black platinum multielectrode array (MEA).23 The signal was amplified by 100 using a PGA16 Multi Channel System Amplifier (MCS, Reutlingen, Germany), digitized using a Power 1401 CED (CED, Cambridge, UK) at a sampling frequency of 5 kHz and acquired with the Spike2 software (CED).
2.3.3 DC-coupled extracellular field recording with glass pipette.
DC-coupled extracellular field recordings were obtained using glass pipettes connected to an Axoclamp-2B amplifier (Axon Instruments, CA, USA). Pipettes were pulled from borosilicate glass to a tip diameter of approximately 1–2 μm using a pipette puller. The pipettes were then filled with a solution of 150 mM NaCl to establish an electrical connection between the recorded field and the recording equipment. The filled pipette was mounted on a micromanipulator for precise positioning under the microscope. A silver–silver chloride (Ag–AgCl) wire was inserted into the back of the pipette, which was connected to the headstage of an Axoclamp-B amplifier to amplify and capture the DC-coupled extracellular field potentials.
2.4 Electronics for gSGFETs recordings
A custom biosignal amplifier system with 64 channels (g.RAPHENE, g.tec medical engineering GmbH, Austria) was utilized for signal acquisition at a sampling rate of 9.6 kHz and a resolution of 24 bits. This system enables simultaneous recording in two frequency bands with different gains to prevent amplifier saturation. It allows setting VS and VD bias voltages, along with current-to-voltage conversion for proper adjustment to the graphene transistors. Furthermore, it supports an automated IDS-VGS characterization process for each transistor, in order to determine the optimal bias point efficiently. The user interface comprises two custom-made Simulink models, incorporating g.HISYS libraries for high-speed online processing. One model performs the IDS-VGS characterization at the start of each experiment while the other is used for the setting of VS and VD bias voltages and manages the signal acquisition process during the experiments.
2.5 Cortical modulation through electrical stimulation
We applied DC electric fields by current injections ranging between −500 μA and 500 μA, in intervals of 100 μA.24,25 To create a homogenous electric field perpendicular to the cortical layers, two Ag–AgCl electrodes were placed 4–8 mm apart parallel to the cortical surface. The electrical stimulation protocols were defined in Spike2 (CED), programmed using a Power1401 ADC/DAC (CED) and converted to current through a stimulus isolator (360A, WPI, Aston, UK). Positive fields are defined as those oriented from the white matter to the cortical surface, inducing depolarization of pyramidal neurons, whereas negative fields are oriented in the opposite direction, prompting hyperpolarization.
2.6 Cortical modulation through pharmacology
To create a model of epileptic activity in vitro, we added to the bath medium 0.5–4.0 μM bicuculline methiodide (BMI), a GABAA receptor antagonist. Cortical spreading depression was induced by local application of 0.5 M KCl.
2.7 Data analysis
2.7.1 Multiunit activity.
We estimated the multiunit activity (MUA) from the LFP as previously reported.16,25
2.7.2 DC amplitude difference.
Data from four different conditions were collected for analysis, namely slow oscillation, pre-epilepsy, epilepsy and CSD. Event detection for each condition involved identifying the peaks of the events (“Ups”) in the AC signal with the scipy.signal.find_peaks function. Once identified, these were later validated through visual inspection.
To establish a temporal reference point for each event, the baseline was determined by considering the time index occurring 2 s before the event. This temporal index was used to synchronise and align the peak measurements accurately.
The DC signal, necessary for subsequent analysis, was derived from the graphene microtransistors full-band data. To obtain the DC signal, a low-pass third-order Butterworth filter was applied. The cut-off frequency for this filter was set at 0.25 Hz to isolate the desired infraslow components from the input signal.
The absolute amplitude difference between peak and baseline was computed from the DC signal. This computation aimed to quantify the magnitude of change in the infraslow amplitude during the identified events. The bottom left panel of Fig. 4 visually represents the computed absolute amplitude differences for all conditions.
The entire data processing and analysis pipeline was implemented using Python (Python Software Foundation, Wilmington, DE, USA), making extensive use of the scipy library26 for signal processing tasks.
2.7.3 Burst frequency.
In order to compute the frequency of the bursts in the absence or presence of electrical stimulation, the peaks of the events were detected in the AC (third-order Butterworth high-pass filter) signal. The frequency was then calculated for every different stimulation condition as the inverse of the time difference between consecutive events. This analysis was conducted using a custom-made Python script.
2.7.4 Spectrogram calculation.
The spectrogram of the signal was computed using a custom-made Python function based on a wavelet approach. Wavelet transforms were applied to achieve time-frequency analysis, capturing dynamic changes and frequency patterns within the data.
2.7.5 Statistical analysis.
The Mann–Whitney test (Wilcoxon rank-sum test) was used to compare across conditions in Fig. 4B and 7. The significant levels were set to *p < 0.05, **p < 0.01, ***p < 0.001 and ****p < 0.0001.
2.7.6 Signal to noise ratio quantification.
We performed a quantification of the SNR based on the methods previously described in Suarez-Perez et al. (2018).23 We used the fSNR expressed in dB defined as
and the vSNR defined as the ratio between the average peak-to-peak of the up states divided by the standard deviation of the down states.
While the fSNR gives information about the performance in terms of SNR for each frequency, the vSNR is only informing about the SNR at low frequencies (approximately 1–10 Hz). In the case of the standard deviation of the Down state, since in this period the neural activity is almost silent, the main frequency content will be due to high frequency noise mostly coming from the 50 Hz and its harmonics.
3. Results
To investigate the full-band frequency activity of the cerebral cortex in vitro, we employed 16-channel arrays of graphene solution-gated field-effect transistors (gSGFETs) which allowed the recording of cerebral cortex activity with a high spatiotemporal resolution. This approach was implemented across a total of 38 ferret cortical slices and 6 human cortical slices, each exhibiting spontaneous rhythmic activity as will be described. By combining the gSGFET full-bandwidth recordings with other well-established recording techniques based on passive electrodes as well as glass micropipettes connected to a DC amplifier, we validated both the AC (>0.1 Hz) and DC (<0.1 Hz) components of the signal.
We studied different patterns of emergent activity, including physiological activity (slow oscillations) and other cortical patterns that are models of pathological conditions, such as epileptiform discharges or CSD. In our analysis, we measured both high-frequency components and the often-neglected infraslow associated components across all patterns. Our inclusion of a sub-sample of human tissue obtained during surgery for epilepsy allowed us to test the gSGFET in conditions closer to the clinical situations, and to quantify activity patterns generated by the human cerebral cortex.
In a second part, we explored a novel use for arrays of non-ferromagnetic graphene microtransistors which is the quantification of electric fields exogenously imposed on the brain tissue. While the brain's electromagnetic stimulation is increasingly used as an intervention for different pathologies (for a review see27,28), the actual fields that reach the brain tissue and the exact mechanisms of action are still in discussion.29 We investigated the extent to which gSGFETs can be used to quantify these fields while we can measure the effect that they have on the activity patterns of the cerebral cortex.
3.1 Graphene transistor (gSGFETs) arrays: design, operating point, and recordings
gSGFET active transducers leverage graphene's electrochemical stability and local amplification to implement high-quality DC-coupled recordings, overcoming the main limitations of passive electrodes for recording ultra-low frequency brain signals.9,12 Full-band electrophysiological recordings were obtained following the procedure shown in Fig. 1A. Prior to recording, Ids–Vgs curves were obtained at constant Vds voltage (VDS = 0.05 V), the optimal bias point for signal recording was determined using a custom code (Fig. 1C, average across the arrays of the maximum of the mean normalized transconductance). Subsequent interpolation of acquired current signals into the transfer curve results in DC-coupled voltage signals. Fig. 1D shows the comparison between the transfer curve at the start and at the end of the recording session, where no significant variations were observed. Importantly, no variation in the dispersion of the CNP (charge neutrality point) is observed. The CNP is related to the capability to operate all transistors inside the array at the optimal bias voltage. A detailed electrical characterization of the performance of the devices can be found in the ESI.†
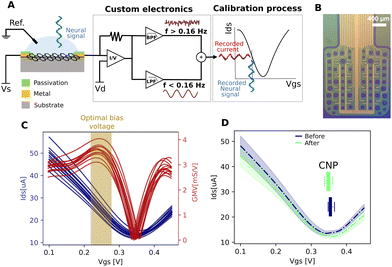 |
| Fig. 1 Electrophysiological recording with gSGFETs arrays. (A) Schematic of the gSGFET recording setup and signal postprocessing methodology. The custom electronic circuit is used to perform the in vivo characterization (transfer curve) and to record the transistor current in the LPF and BPF bands. From the combination of the two signals and considering the current-to-voltage conversion, the calibrated wide-band signal (Vsig) is obtained. (B) Optical microscope images of the active area of a 4 × 4 gSGFET array. (C) Example of transfer curve for each transistor of the array obtained in the cerebral cortex using Vds = 50 mV (Ids current in blue, normalized transconductance in red). Brown shadow shows the optimal bias voltage range for performing the recordings. (D) Mean and standard deviation of all transistors of the array before (Blue) and after (Green) of the recording session, the inset shows the dispersion of the position of the CNP (charge neutrality point, Vgs value for the minimum Ids current). | |
3.2 Using gSGFETs as a tool for measuring physiological and pathological neural events: validation against passive metal electrodes arrays
We validated the gSGFET arrays recordings of cerebral cortex activity through comparisons with single tungsten electrode recordings (n = 17), as well as black platinum arrays comprising 16 channels (n = 31) (Fig. 2A). Recordings were conducted on spontaneously active cortical slices, which spontaneously generate slow oscillations, characterized by alternating Up and Down states (periods of activity and periods of silence, respectively). As depicted in Fig. 2, both arrays were concurrently positioned over the same cortical slice, enabling us to simultaneously observe and analyse the shape and characteristics of the recorded activity.
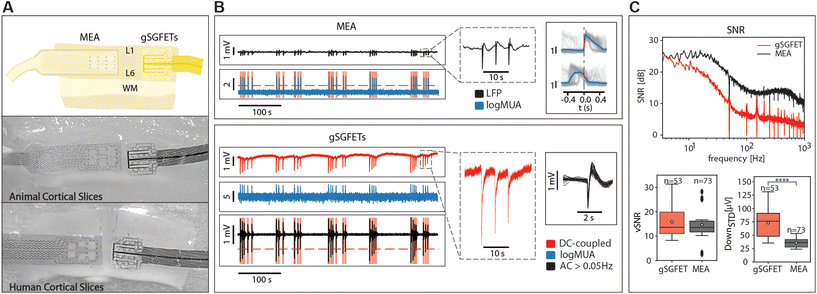 |
| Fig. 2 Graphene microtransistor arrays as a tool for measuring physiological and pathological events. (A) Experimental arrangement for the recordings performed with gSGFETs and validated against standard black platinum microelectrodes arrays (MEAs (top) in ferret (middle) and human (bottom) cortical slices in vitro. (B) Physiological events were simultaneously recorded in MEA and gSGFETs. The top trace is the local field potential (LFP) and displays pre-epileptic events that are expanded on the right hand inset. In blue is the relative firing rate or logMUA in arbritary units (see Methods), in which the events are detected and quantified. The lower part of B displays the full-band or DC-coupled recordings in red, further expanded on the right, from which the relative firing rate or logMUA is obtained. High-pass filtering (>0.05 Hz) provides the AC component. Isolated AC-events are represented on the right inset, following detection. (C) Quantification of the frequency signal-to-noise ratio (fSNR) for the 3.5–1000 Hz frequency band for the gSGFET and black platinum MEA (red and black trace, respectively)(top). Traces depict the average fSNR among a total of 53 gSGFETs and 73 black platinum electrodes. Boxplot of the voltage signal-to-noise ratio (vSNR) for the gSGFET (n = 53) and black platinum MEA (n = 73) (red and black trace, respectively. Mann–Whitney test n.s.) (bottom left). Boxplot of the standard deviation of the Down states for the gSGFET (n = 53) and black platinum MEA (n = 73) (red and black trace, respectively. Mann–Whitney test ****p < 0.0001) (bottom right). | |
Under these conditions, we studied three distinct patterns of activity: slow oscillations, pre-epileptic, and fully developed epileptic activity, as well as spontaneous and induced CSD.
Detailed descriptions of these activity patterns can be found in the subsequent section. To enhance the intensity of the discharges, we often added an inhibition blocker to the bath (0.5–4.0 μM bicuculline methiodide, BMI, a GABAA receptor blocker) that induced pre-epileptic discharges and eventually full-blown epileptiform discharges as previously described.16
Our observations revealed that the AC signal recorded with the gSGFETs closely resembled that obtained with single tungsten electrodes or the arrays of black platinum electrodes across all activity patterns (Fig. 2B). In the top, the multielectrode metal array recorded pre-epileptic, highly synchronous bursts that appeared with a low frequency (0.05–0.2 Hz), events that can be observed in more detail in the right panel. In the gSGFET recordings, the DC-coupled signal was simultaneously obtained from the same slice, evidenced by the almost simultaneous recording of the events. Full-band recordings offer additional information compared to AC recordings. Specifically, they capture the infraslow component between pre-epileptic events and the one associated with the activity, that will reveal DC-shifts on relation to epileptiform discharges (see below). It can also be seen that the shape of the individual events is different, due to the high-pass filtering in the case of the AC-coupled signals.
On the technical side, some transistors failed to produce quality recordings, resulting in a highly noisy signal. The higher noise recorded with the gSGFETs is also evidenced by the fact that when we estimate the multiunit activity (MUA) using the logMUA technique,30,31 the relative amplitude obtained with the microtransistors was often lower, due to a reduced signal-to-noise ratio at frequencies above 200 Hz.8
Additionally, we performed event detection to validate the capability of graphene to detect neuronal activity. Event detection is important for the quantification of neuronal firing. In our experiments, we observed that the detection of pre-epileptic and epileptic activity was successfully accomplished with the graphene transistors. The distinct firing pattern characteristic of these pathological states was readily captured, indicating the sensitivity and reliability of the graphene-based recording system in identifying abnormal neural activity associated with epilepsy.
However, when it came to slow oscillations, we encountered some challenges related to the small amplitude of the signal (30–40 μV) and the relative signal-to-noise ratio. Slow oscillations are low-frequency brain waves (≤1 Hz), and their detection requires a high signal-to-noise ratio to distinguish the weak neural signals from background noise. While some of the graphene transistors exhibited consistent and reliable detection of slow oscillations, others did not, possibly due to variations in device performance or tissue properties.
In order to quantitatively compare the recording devices in terms of SNR we measured the SNR at different frequencies showing that the gSGFET performance is comparable to that of the black-Pt MEA for frequencies below 10 Hz (Fig. 2C, top). This result is strengthened by the vSNR which shows no statistical difference between the gSGFET and black-Pt (Fig. 2C bottom left, average vSNR 15.9 ± 6.2 and 14.7 ± 6.7, respectively). The performance discrepancy between the two recording devices increases with the frequency. Above 10 Hz, the SNR of gSGFETs shows a decay of more than 15 dB/decade while the black-Pt MEA has a decay of 10 dB/decade. This decay in performance for higher frequencies is quantified by the standard deviation of the Down states (DOWNSTD) which gives a measure of the intrinsic noise of the device. The gSGFETs show an average DOWNSTD which is 2-fold larger than the black-Pt MEA (Fig. 2C, bottom right, average DOWNSTD 73.4 ± 25.3 μV and 35.8 ± 8.4 μV, respectively). This result is critical since the gSGFET will not be able to discern any event whose amplitude on average is below 73.4 μV.
3.3 Using gSGFETs as a tool for measuring physiological and pathological neural events: validation against DC-coupled recordings with glass pipettes
A classical way to measure DC-coupled extracellular fields is the use of glass pipettes filled with a solution of NaCl connected through a silver–silver chloride (Ag–AgCl) wire to a DC amplifier, such as an intracellular amplifier. We illustrate this recording in Fig. 3A, which represents an epileptiform discharge recorded simultaneously with a tungsten electrode (top) and a glass pipette (bottom). The glass pipette shows an infraslow component with an extracellular negative voltage of longer duration to the one recorded in AC mode (LFP), thus high-pass filtered by the amplifier. The middle trace represents the enhanced extracellular multiunit activity obtained through a high-pass filter (>200 Hz).
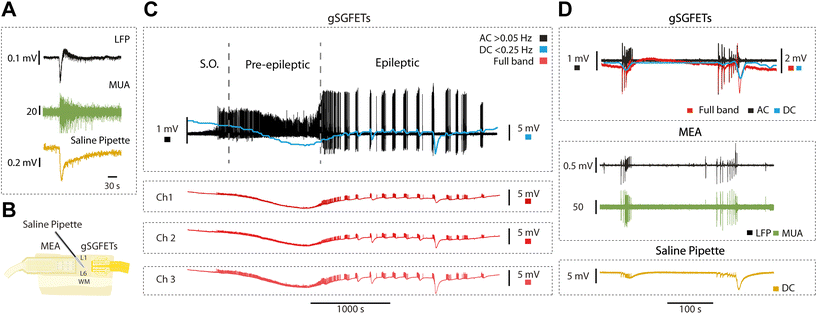 |
| Fig. 3 AC and DC components of the gSGFETs wide-band recordings and validation of the DC measures with saline pipettes. (A) Simultaneous recording of an epileptiform discharge with a tungsten electrode (AC recording) and a glass pipette connected to a DC amplifier (DC recording). LFP (black), MUA (green) and DC component (yellow). (B) Experimental arrangement for C and D, representing a cortical slice and on top of it a black platinum 16-channel array, a glass pipette in the centre and the gSGFETs array on the right. (C) Fluctuations in the AC (>0.5 Hz; black) and DC (<0.25 Hz; blue) components of the signal recorded with gSGETs over time (top) displaying pre-epileptic and epileptic discharges in the presence of 1 μM bicuculline. In red, three channels from the same array displaying the DC coupling version of the same signal (bottom). (D) Simultaneous recordings obtained with the arrangement in B with gSGFETs, MEA and saline pipette. The components and their colour code is represented in the figure. | |
The full-band or DC-coupled recording provides the infraslow or DC component along with the higher frequencies, which can then be separated using high-pass and low-pass filters. In order to further validate both components, we used the arrangement shown in Fig. 2B: an array of 16 passive black platinum electrodes, a NaCl glass pipette in the centre connected to a DC amplifier, and on the right, an array of gSGFETs (n = 4). In Fig. 3C, the recorded activity is represented in black for the AC component, obtained through a high-pass filter at 0.05 Hz. The blue represents the DC component low-pass filtered at 0.25 Hz. The red traces are the full-band DC-coupled recordings corresponding to three different microtransistors in the array. It clearly displays the negative DC-shift that is generated during the pre-epileptic discharges and that precedes the full-blown epileptic discharge.
In Fig. 3D, the simultaneous recordings with gSGFETs, MEA and saline pipette (Fig. 3B) are displayed. From the full-band obtained with gSGFETs (red trace) we obtain the equivalent to the AC band (black trace; >0.16 Hz) that we compared against recordings obtained with the passive metallic electrode array (MEA in Fig. 3D). Further, the low-pass (<0.16 Hz), DC component (red trace), was compared against the one obtained with the glass pipette (yellow trace), revealing a precise correspondence in both cases, providing an additional validation of the gSGFET methodology.
3.4 Full-band recording of cerebral cortical activity: DC and AC components in slow oscillations, epileptiform discharges and CSD
Graphene microtransistors allow the quantification of the brain's full-band signal. Extracellular AC signals include LFPs (population activity within a reach of hundreds of microns), multiunit activity and eventually single units. DC potentials are slow brain signals, with a frequency under 0.2 Hz, and commonly filtered out by conventional AC amplifiers. DC potentials, therefore, convey information about what has been referred to as “infra-slow” components of the signal. Here, we used this unique capability provided by gSGFETs to explore a part of the signal that is most commonly filtered out.
First of all, we investigated a spontaneous rhythmic activity that is generated by the cortical circuits: slow oscillations. This is a physiological pattern of activity that is equivalent to the one observed during slow wave sleep or deep anesthesia.32,33 In the same or other slices, we studied pre-epileptic activity as described in,16 following a block of GABAA-mediated inhibition, which consists in a more synchronized, higher firing rate burst. Further GABAergic blockade results in full-blown epileptiform discharges, which are an in vitro model of epilepsy.16 Next, we investigated CSD, a well-known phenomenon characterized by a massive depolarization of neurons and glial neurons that travels slowly (in the order of mm min−1) and that is followed by a long period of silence that can last minutes and even over an hour.34
We recorded the extracellular DC associated with physiological slow oscillations, an activity pattern that is highly similar to slow wave sleep. Slow oscillations consist of up states and down states (for a review, see15), and the DC change is associated with this bistability (Fig. 4A). The occurrence of rhythmic slow oscillations was detected in an oscillation in the DC (as <0.25 Hz), with an average amplitude for the event of 0.03 ± 0.02 mV (Fig. 4B and C).
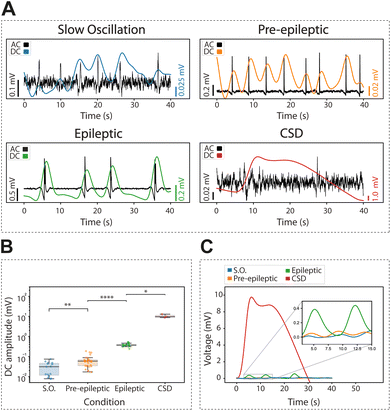 |
| Fig. 4 DC component of slow oscillations, pre-epileptic activity, epileptic activity, and cortical spreading depression (CSD). Differences in amplitude of the infra-slow across different conditions. (A) High-pass 0.5 Hz filtered (black traces) along with the corresponding low-pass 0.25 Hz filtered infra-slow (colored traces) for slow oscillations, pre-epileptic, epileptic and cortical spreading depression discharges. (B) Absolute change in amplitude of the infra-slow events with respect to their baseline for the four different conditions. Mann–Whitney test (Wilcoxon rank-sum test) *p < 0.05, **p < 0.01, ***p < 0.001 and ****p < 0.0001. (C) Representative change of the averaged infraslow component during the events across the four different conditions. | |
Next, we investigated the DC changes associated with pre-epileptic and full-blown epileptiform activity which is generated in the presence of GABAergic blockers such as bicuculline. The amplitude of the associated DC was on average 0.06 ± 0.04 mV and 0.39 ± 0.07 mV, respectively, being thus larger for epileptic discharges (Fig. 4B and C) as expected.
Finally, we induced CSD using local potassium application (0.5 M KCl). This is a relevant activity associated with pathological states such as migraine, traumatic brain injury or stroke.34,35 We observed that CSD evoked the largest DC change with an amplitude of 10.43 ± 2.23 mV, two-orders of magnitude larger than an epileptic discharge. Given its large amplitude and duration, the changes in DC associated with CSD were of the first pathological discharges characterized by gSGFETs in vivo,9 where they have also been described in association with optogenetic stimulation.36
3.5 Physiological relevance of infraslow components in different cortical patterns
We explored the DC-coupled recordings during cortical in vitro epileptiform discharges obtained by blocking inhibition (as in16). We induced pre-epileptiform activity by adding 0.5–1.0 μM of bicuculline methiodide (BMI) to the ACSF, followed by the application of 4 μM to induce full-blown epileptiform discharges. Lastly, we reverted to control ACSF to record the associated DC-shifts back to baseline.
Our investigations revealed a tight relationship between DC-shift changes observed in the full-band recordings and the occurrence of epileptiform discharges. The DC-shift displayed a potential predictive capacity for full-blown epileptiform activity. Graphene microtransistor recordings allowed for the precise visualization of DC-shifts preceding alterations in activity patterns for over 1000 s (Fig. 5A and B), a feature that was exclusively captured through DC-coupled recordings (n = 5). An average across five events is displayed in Fig. 5C.
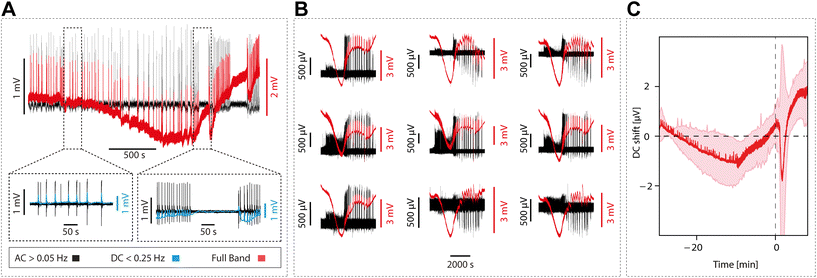 |
| Fig. 5 DC-coupled signal predicts full-blown epileptiform activity. (A) Example of the DC-coupled decay (red) superimposed on the AC component (black) starting around 1000 s before the onset of the epileptic seizure. Insets in (A) depicts a zoom in on the activity in the AC and DC components (black and blue, respectively) before the seizure (left) and at the onset of the seizure (right). (B) DC-coupled (red) and AC component (black) time course of the buildup of an epileptic seizure recorded simultaneously in 9 gSGFETs with different spatial location. (C) Waveform average of the DC-coupled for n = 5 epileptic seizures aligned at the onset of the seizure (SDM in pink). | |
Previous research in vivo had reported the occurrence of DC shifts in association with seizure onset, even preceding seizure onset,5,6 and providing information about the epileptogenic zone. However, the fact that this DC-shift preceding epileptic discharges occurs in the local circuit of a cortical slice strongly suggests that this can be explained by a local mechanism that may involve potassium accumulation and neuronal-glial interactions.6 The ability to identify and assess DC-shift as a potential predictor of epileptiform activity carries significant clinical relevance.
3.6 Cortical full-band recordings from human cerebral cortex in vitro
Even though the cerebral cortex of mammal animal models share many properties with the cerebral cortex of humans, the human cerebral cortex is more complex, thicker, has more neurons, more connections and functional differences.37–39 Given that the final objective of the graphene microtransistor arrays is to improve the neural interfaces for clinical applications, it is important to evaluate new interfaces in human tissue. However, for ethical reasons, there is less availability for this use because it requires invasive procedures that need to be justified.
We conducted recordings on brain cortical slices (n = 6) obtained from six patients with refractory epilepsy, out of which n = 4 slices presented rhythmic spontaneous activity, and they have been included here. Different activity patterns were recorded, including both spontaneous events and evoked ones by local application of glutamate.
In Fig. 6, we display both spontaneous (Fig. 6A) and evoked activity as a result of the local application of glutamate (see Methods). In both cases we represent the full-band or DC coupled recording, DC and AC components, MUA and also the spectrogram. Fig. 6C and D represent the experimental setup overlayed with the anatomical reconstruction.
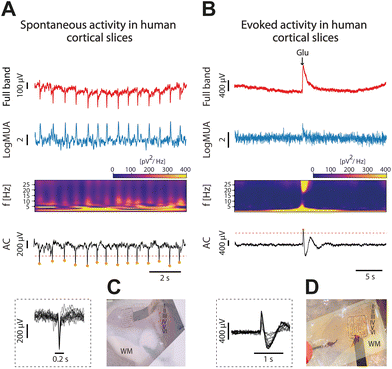 |
| Fig. 6 Graphene microtransistors for measuring human cortical activity. Spontaneous (A; left) and evoked (B; right) activity from human cortical slices in vitro recorded with graphene microtransistors. Traces of full-band signals (red), firing rate or logMUA in arbritary units (blue), spectogram and AC component of the signal (black). In the inset, single detected events are overlapped. C and D represent the experimental set-up with the overlapping anatomical reconstructions. | |
This is a small sample of human slices that are a proof of concept of the use of gSGFETs on human tissue and the successful recording of activity. However, to gain a comprehensive understanding of the complex nature of infraslow oscillations in human cortical slices, additional experiments and more extensive analyses are necessary.
3.7 Using gSGFETs as a tool to quantify exogenously applied electric fields
Infraslow oscillations have been suggested to represent a slow, recurring modulation of gross cortical excitability.40 As such, changes in the DC component of the electrophysiological signal can be associated with changes in excitability levels. As graphene microtransistors can precisely capture changes in the DC bandwidth,9,12 gSGFETS have the potential to reveal mechanistic insights into excitability fluctuations. Furthermore, the application of DC fields to cortical tissue has been reported to modulate cortical excitability. Weak DC fields shift the somatic transmembrane voltage either towards depolarization or hyperpolarization, therefore affecting the probability of action potential firing.24 Consequently, cathodal transcranial direct current stimulation (tDCS), a non-invasive neuromodulation technique in which weak DC fields are delivered to the brain, has been suggested to decrease cortical excitability.41–45 The recording of full-bandwidth electrophysiological signals with graphene microtransistors while exogenous DC fields are applied to the cortical tissue can provide not only a direct measure of the field being applied but also a quantification of the excitability changes induced by the field itself.
Here, we applied positive and negative exogenous DC fields with intensities ranging from 100 to 500 μA while simultaneously recording the DC and AC signal components with gSGFETs (Fig. 7A and B). The AC components revealed an increase in the frequency of the oscillatory events with depolarizing fields. This protocol was repeated for slices expressing pre-epileptic and epileptiform activity induced by the application of BMI and for dead slices (by glucose elimination) (Fig. 7C). In the dead-slice condition, the DC component captures the intensity of the exogenous electric field alone, while in the active slice the DC component contains the exogenous electric field combined with the endogenous electric field due to the extracellular potentials originated by the neuronal membrane potential or activity. We recorded the DC component when the DC fields were applied in both conditions, allowing us to measure the voltage amplitude associated with each intensity amplitude, and additionally to evaluate the time course. Furthermore, by subtracting the mean DC values in these two conditions (alive-dead), we were able to isolate the changes in DC created by the endogenous field. When no external electric field is applied the average endogenous potential (EP) is around 5 μV while for an external EF of −500 μA the average EP is −15 μV. The EP is proportional to the intensity of the external electric field meaning that in absolute value, the larger the external electric field the larger the endogenous potential.
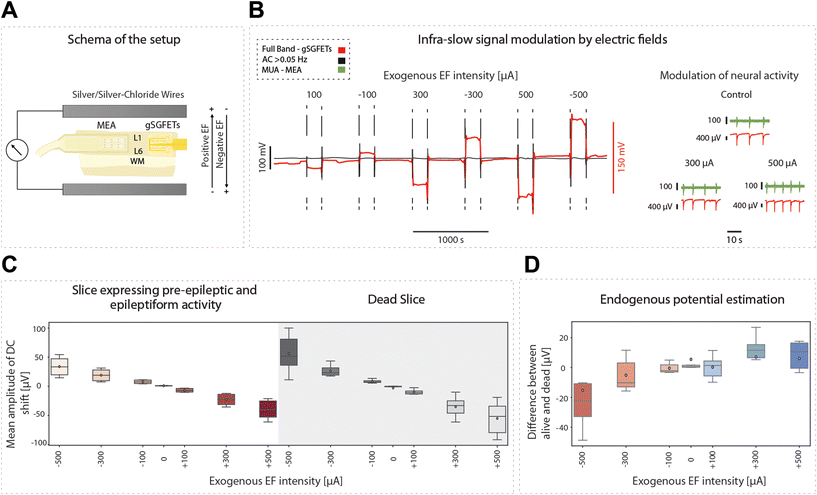 |
| Fig. 7 gSGFETs allow the quantification of exogenous and endogenous electric fields. (A) Schema of the setup for the experiments in which an exogenous electric field is applied to the cortical slice. (B) AC component (black) and DC-coupling (red) time course in a typical protocol of stimulation with positive and negative electric fields. On the right, full-band (red) and MUA (green) traces recorded with gSGFETs and MEA, respectively, both showing modulation of cortical activity with the applied field. Positive fields, by inducing depolarization of the soma of the pyramidal neurons, lead to an increase in the frequency of oscillation. (C) Mean amplitude changes of the DC component with respect to the baseline previous to the stimulation for different intensities of the electric field in the active and in the dead slice (shaded area). (D) Estimation of the endogenous electric fields computed as the difference of the mean DC component between the dead and the alive slice conditions for different intensities of exogenous electric field. | |
In the convention used, a positive sub-threshold soma polarization indicates a positive field, resulting in membrane depolarization, and a negative sub-threshold soma polarization indicates a negative field, resulting in hyperpolarization of the membrane. Somatic polarization presents a reversed polarity with the direction of the applied electric field. As such, depolarization occurs when there is a negative DC shift and hyperpolarization occurs when there is a positive DC shift (Fig. 7D).
4. Discussion
Graphene-based solution-gated field-effect microtransistor arrays have recently emerged as a promising technology that could offer some advantages over current methodologies in recording brain activity.8,9 Historically, passive electrodes have been the most used transducers for recording brain activity. However, they can lead to a non-negligible frequency-dependent attenuation and are also susceptible to DC offsets and low-frequency drifts present at the electrode-tissue interface, impairing high fidelity recording of the brain's infraslow activity and introducing high common voltage offsets. This limitation precluded the use of DC-coupled amplifiers and has promoted the use of high-pass filter ones. A key benefit of gSGFET arrays is the ability to perform DC-coupled recordings, thus providing full-band information with superior spatiotemporal resolution, as has been previously described.8,9 This innovation opens the door for the development of advanced neural interfaces with clinical applications.46 However, this emerging technology is yet to be fully characterized in terms of its capabilities and limitations, as well as the physiological relevance of the measurements it yields.
In this study, we have made significant advancements on several fronts related to the usage of gSGFETs as a new neural interface. These developments can be summarized in the following points: (1) validation of gSGFETs against passive electrode recordings; (2) full-band characterization of physiological and pathological cortical emergent patterns in vitro, including the finding of infraslow shifts preceding by hundreds of seconds epileptiform discharges; (3) first use of gSGFETs on human cortical tissue; and (4) novel use of gSGFETs for the quantification of exogenously applied electric fields and the resulting neuromodulation. Concurrently, we also examined potential problems and constraints of this technology.
Our research was carried out using an in vitro preparation of the cerebral cortex which spontaneously generates physiological activity consisting of rhythmic slow oscillations. These oscillations parallel the slow wave oscillations observed during deep non-REM sleep or profound anesthesia, as previously described.15,32 Employing in vitro measurements presents some advantages given that it provides full experimental access to the cortical tissue, allowing the combination of different technologies, as well as a direct accessibility to the cortical layers. Further, it presents a special challenge for these novel recording interfaces, given that the signals are of smaller amplitude than in vivo. Thus, our successful measurements with gSGFETs even to record the infraslow components associated with the active periods (or Up states) of physiological slow oscillations (Fig. 4) indicates their efficacy in dealing with a low signal-to-noise ratio. This ability is important for the technology's performance in real-world applications, where the need to detect small signals within noise is a common challenge.
Further, the investigation in cortical slices is obviously limited to local cortical circuits within the limits of several millimeters, what per se provides valuable physiological information regarding the underlying mechanisms of the observed phenomena. For example, we detected prominent and long-lasting (>1000 s) DC-shifts preceding seizure-like activity (Fig. 5). This feature, only detected so far in the cerebral cortex in vivo,6 strongly points to the relevance of local mechanisms underlying such DC-shifts, such as variation in extracellular potassium and the interaction with the glial role on potassium homeostasis, all of them mechanisms that would persist in a rather small piece of tissue in vitro.
Our findings have convincingly shown that both AC and DC components of the signal, as recorded using gSGFETs, are closely aligned with the measurements obtained via passive electrodes (Fig. 2 and 3). This approach presents an additional benefit related to the full-band technology: the traditional electrophysiological recording of the infraslow component utilizing glass pipettes connected to DC amplifiers is unsuitable for multichannel or chronic in vivo recordings. DC-EEG amplifiers have also been used,40 but they are highly susceptible to drifts and artifacts, electrode polarization, and so on. Nonetheless, we also encountered certain challenges with the gSGFETs, primarily arising from the use of a prototype rather than a commercial product, such as inconsistency in transistor performance and the absence of a commercial software solution for data acquisition.
The significance of infraslow components of brain activity has gained importance in brain dynamics since the early 2000s, largely driven by findings in fMRI data.2,47,48 This has revealed a crucial role of infraslow rhythmic activity in coordinating brain function and maintaining synchrony across various cortical regions. These infraslow oscillations (ISOs), or fluctuations in brain activity below 0.1 Hz, appear to be the orchestrators of neural processes that span large-scale networks in the brain.49,50 Evidence from fMRI studies indicates that infraslow oscillations are linked to the brain's default mode network (DMN), a large-scale network active during resting states, and that disruptions in this activity are associated with various neurological and psychiatric conditions, including Alzheimer's disease, schizophrenia, and autism, suggesting potential diagnostic and therapeutic implications.51–53
However, the electrophysiological basis of infraslow oscillations and the precise underlying mechanisms are not yet fully understood, although simultaneous laminar electrophysiology and brain imaging reveal a distinct propagation of infraslow activity from lower rhythms like delta activity.2 While fMRI provides a macroscopic view of these slow rhythms, it does not directly measure neuronal activity but rather changes in blood flow and oxygenation, which indirectly reflect neuronal activity. It is for this reason that is critical to further investigate these phenomena at the electrophysiological level, measuring the electrical activity of neurons in order to find out how the infraslow oscillations or components are generated at the cellular and circuit level, how they interact with faster neuronal activity, and how they contribute to cortical function and behaviour.
Regarding the electrophysiological correlates of the infraslow component, a large part of the available information is from epilepsy and its associated infraslow components.4–6,40,54 There has been documentation of the infraslow rhythms related to the periods of responsiveness to external auditory stimuli during slow-wave sleep.3 In this study we have described a low amplitude component in the infraslow band associated with physiological slow oscillations (Fig. 4). We have also described the AC and DC components associated with highly synchronous, pre-epileptic bursts generated in the presence of a GABAA blocker,16 as well as that associated with epileptiform activity, the latter being of larger amplitude than the former. To our knowledge, this is the first full-band description of all these events in cortical slices.
Interestingly, we have found that the change in the infraslow component precedes for hundreds of seconds the occurrence of full-blown epileptiform discharges, suggesting that there is an extracellular change which is detected in the DC-coupled recordings which is associated with an increased excitability that leads to the discharge (Fig. 5). This type of DC-shift preceding seizures has been described in vivo,5 however, its finding in cortical slices points towards local mechanisms as a cause. This infraslow change, therefore, can be used as a biomarker of this pathological activity.
Another relevant pathological cortical discharge is CSD, a wave of neuronal and glial depolarization that travels slowly across the cortex, followed by a prolonged suppression of neural activity. There is a well-known association of the pathological activity patterns to migraine, traumatic brain injury and stroke between others.35,55,56 CSD being a high amplitude signal, had already been characterized in vivo using gSGFET arrays.8,36 In the current study we have also measured this pathological discharge but now in a local circuit in vitro (Fig. 3 and 4), where the DC response has the largest amplitude of all the activity patterns detected. CSDs can occur in the slices spontaneously during epilepsy, or as a consequence of local potassium injection. This mechanism can be similar to that inducing CSDs in brain lesions, where raising extracellular potassium is involved.57
4.1 Full-band recordings from human cerebral cortex in vitro
Given that one of the main objectives of graphene microtransistor arrays is to enhance neural interfaces for clinical applications, it becomes critical to test these interfaces on human tissue. This will help ensure that the devices can effectively capture the intricate and highly specialized neural networks found in humans.
Despite the significant similarities between the mammalian cerebral cortex and that of humans, such as its layered structure and basic cellular composition, the human cerebral cortex has higher complexity. This complexity is reflected in several aspects including its thickness, neuron density, and richness of connectivity, among others. For example, a human pyramidal cell receives on average 40
000 inputs, while a mouse one receives around 10
000 inputs.37–39 However, testing in humans is obviously more restricted for ethical reasons, since access to the brain requires invasive procedures that require substantial justification due to the potential risks to the patient. Furthermore, all testing takes place in the context of neurological diseases, and never on healthy brains. Consequently, the availability of human tissue for this purpose is relatively limited.
Epilepsy surgery often requires the removal of brain tissue, which would otherwise be discarded. This tissue provides a valuable opportunity to obtain cortical slices and to record from them in vitro, an avenue that has been taken to learn from human cortex.17,58,59 We have used this preparation here to test the graphene microtransistor arrays in the human cortex in vitro, recording both spontaneous rhythmic activity and evoked responses, in a context that is much closer to their intended final use in humans. In this way, it may be possible to advance the development of neural interfaces while respecting the necessary ethical boundaries of human research.
4.2 Using gSGFETs to measure exogenously applied electric fields for neuromodulation
Finally, we have explored a new use of gSGFETs that also has clinical applications, namely the local quantification of exogenously applied electric fields in the context of neuromodulation. The field of brain stimulation has attracted significant attention in the community in the last two decades. Non-invasive strategies such as transcranial magnetic stimulation (TMS), transcranial direct current stimulation (tDCS), and transcranial alternating current stimulation (tACS) have been the subject of a vast number of studies since then. There is accumulated evidence of the value of the brain's electromagnetic activation/inhibition through non-invasive brain stimulation in many different applications (from pain to stroke recovery, among others; for a review see Brunoni et al.27). However, the spatial resolution is low (several millimeters), and the exact mechanisms are not well understood. Furthermore, some serious objections have been made with respect to the actual electric fields reaching neurons, versus those decaying in skin and skull (up to 75% of the signal).29 We considered that the DC-couple capabilities of gSGFET arrays could be used to measure imposed electric fields as well as the resulting impact on cortical activity patterns. We have demonstrated that this use is possible (Fig. 7), albeit sometimes for long (>30 s) and high-intensity electric fields (>400 μA) there are instabilities. Future developments in this technology should allow us to integrate gSGFETs in brain interfaces for clinical applications.
5. Conclusions
Graphene-based solution-gated field-effect transistors have the unique capability to capture DC- and AC-coupled or full-band recordings. Leveraging on this property of gSGFETs, we investigated various cortical activity patterns.
First, we validated the recordings obtained with gSGFETs. Simultaneous recordings of different activity patterns were carried out in in vitro cortical slices with three types of systems: gSGFET arrays, passive metallic microelectrode arrays, and glass pipettes filled with NaCl connected to a DC amplifier. This facilitated the recording of full-band AC and DC components of activity, respectively, thereby validating gSGFET recording.
gSGFET technology enabled the quantification of the infraslow component associated with the physiological activity of slow oscillations – as in slow-wave sleep- (ca. 30 μV), pre-epileptic activity (ca. 60 μV), epileptiform activity (ca. 400 μV), and CSD (ca. 10 mV), the latter being an activity related to migraines and brain lesions. We detected long-lasting (around 1000 s) DC-shifts preceding epileptic discharges. Such DC shifts have been described in vivo, but never previously in vitro. The detection of such a slow event in vitro is of significant physiological value, as it indicates that the underlying mechanism is reliant on local circuitry. Furthermore, we report on the first recordings of spontaneous and evoked responses from human cortical slices using gSGFETs, bringing this technology one step closer to its validation for clinical use.
In this study we have also demonstrated a new potential use for gSGFETs: the quantification of exogenously applied electric fields and their resulting effects on cortical activity. This innovative use of gSGFETs opens doors to future clinical applications in neural interfaces, brain stimulation, and closed-loop devices.
However, certain limitations of gSGFETs persist, such as the inhomogeneity of the transistors, as well as instabilities and distortions during the measurement of large amplitude (>400 μA) electric fields. Additionally, their performance in the high-frequency domain could be improved. These limitations will need to be addressed in the future.
Author contributions
Nathalia Cancino-Fuentes: experiments, data analysis, and writing. Arnau Manasanch: data analysis and writing. Joana Covelo: writing and analysis. Alex Suarez-Perez: data analysis and writing. Enrique Fernandez: calibration of transistors. Stratis Matsoukis: provide graphene amplifier and equipment integration and writing. Xavi Illa: development of transistors. Anton Guimerà-Brunet: development of transistors, writing and data analysis. Maria V. Sanchez-Vives: experimental design, supervision, analysis, paper writing and funding acquisition.
Conflicts of interest
There are no conflicts to declare.
Acknowledgements
This research has been funded by the European Union's Horizon 2020 research and innovation programme under Grant Agreement No. 881603 (GrapheneCore3) and No. 785219 (GrapheneCore2) to all teams. The IDIBAPS team was also funded by CORTICOMOD PID2020-112947RB-I00 funded by MCIN/AEI/10.13039/501100011033 and by Departament de Recerca i Universitats de la Generalitat de Catalunya (AGAUR 2021-SGR-01165). IDIBAPS is funded by the CERCA program (Generalitat de Catalunya). The IDIBAPS team thanks the Hospital Clinic of Barcelona for the active collaboration to obtain human tissue (Drs. Roldán, Conde, Rumiá and Carreño). J. C. is funded by EU Horizon 2020 Marie Skłodowska-Curie No 860563 (euSNN MSCA-ITN). The fabrication of the gSGFET arrays made use of the Spanish ICTS Network MICRONANOFABS, partially supported by MICINN and the ICTS NANBIOSIS, specifically by the Micro-NanoTechnology Unit U8 of the CIBER-BBN. C. N. M. also acknowledge funding from the Generalitat de Catalunya (2021SGR00495), by the Spanish MICIN PID2021-126117NA-I00, and by CIBER-BBN (CB06/01/0049). The authors acknowledge the financial support provided by CIBER-BBN and the Instituto de Salud Carlos III with assistance from the European Regional Development.
References
- M. E. Raichle, A. M. MacLeod, A. Z. Snyder, W. J. Powers, D. A. Gusnard and G. L. Shulman, Proc. Natl. Acad. Sci. U. S. A., 2001, 98, 676–682 CrossRef CAS PubMed.
- A. Mitra, A. Kraft, P. Wright, B. Acland, A. Z. Snyder, Z. Rosenthal, L. Czerniewski, A. Bauer, L. Snyder, J. Culver, J.-M. Lee and M. E. Raichle, Neuron, 2018, 98, 297–305 CrossRef CAS PubMed e6.
- S. Lecci, L. M. J. Fernandez, F. D. Weber, R. Cardis, J.-Y. Chatton, J. Born and A. Lüthi, Sci. Adv., 2017, 3, e1602026 CrossRef PubMed.
- P. Modur, Ann. Indian Acad. Neurol., 2014, 17, 99 CrossRef PubMed.
- K. Kanazawa, R. Matsumoto, H. Imamura, M. Matsuhashi, T. Kikuchi, T. Kunieda, N. Mikuni, S. Miyamoto, R. Takahashi and A. Ikeda, Clin. Neurophysiol., 2015, 126, 47–59 CrossRef PubMed.
- A. Ikeda, H. Takeyama, C. Bernard, M. Nakatani, A. Shimotake, M. Daifu, M. Matsuhashi, T. Kikuchi, T. Kunieda, R. Matsumoto, T. Kobayashi and K. Sato, Neurosci. Res., 2020, 156, 95–101 CrossRef PubMed.
- J. Lückl, C. L. Lemale, V. Kola, V. Horst, U. Khojasteh, A. I. Oliveira-Ferreira, S. Major, M. K. L. Winkler, E.-J. Kang, K. Schoknecht, P. Martus, J. A. Hartings, J. Woitzik and J. P. Dreier, Brain, 2018, 141, 1734–1752 CrossRef PubMed.
- C. Hébert, E. Masvidal-Codina, A. Suarez-Perez, A. B. Calia, G. Piret, R. Garcia-Cortadella, X. Illa, E. Del Corro Garcia, J. M. De la Cruz Sanchez, D. V. Casals, E. Prats-Alfonso, J. Bousquet, P. Godignon, B. Yvert, R. Villa, M. V. Sanchez-Vives, A. Guimerà-Brunet and J. A. Garrido, Adv. Funct. Mater., 2018, 28, 1703976 CrossRef.
- E. Masvidal-Codina, X. Illa, M. Dasilva, A. B. Calia, T. Dragojević, E. E. Vidal-Rosas, E. Prats-Alfonso, J. Martínez-Aguilar, J. M. De la Cruz, R. Garcia-Cortadella, P. Godignon, G. Rius, A. Camassa, E. Del Corro, J. Bousquet, C. Hébert, T. Durduran, R. Villa, M. V. Sanchez-Vives, J. A. Garrido and A. Guimerà-Brunet, Nat. Mater., 2019, 18, 280–288 CrossRef CAS PubMed.
- B. M. Blaschke, N. Tort-Colet, A. Guimerà-Brunet, J. Weinert, L. Rousseau, A. Heimann, S. Drieschner, O. Kempski, R. Villa, M. V. Sanchez-Vives and J. A. Garrido, 2D Mater., 2017, 4, 025040 CrossRef.
-
A. Guimera-Brunet, E. Masvidal-Codina, X. Illa, M. Dasilva, A. Bonaccini-Calia, E. Prats-Alfonso, J. Martinez-Aguilar, J. M. De la Cruz, R. Garcia-Cortadella, N. Schaefer, A. Barbero, P. Godignon, G. Rius, E. Del Corro, J. Bousquet, C. Hebert, R. Wykes, M. V. Sanchez-Vives, R. Villa and J. A. Garrido, in 2019 IEEE International Electron Devices Meeting (IEDM), IEEE, 2019, pp. 18.3.1–18.3.4.
- A. Bonaccini Calia, E. Masvidal-Codina, T. M. Smith, N. Schäfer, D. Rathore, E. Rodríguez-Lucas, X. Illa, J. M. De la Cruz, E. Del Corro, E. Prats-Alfonso, D. Viana, J. Bousquet, C. Hébert, J. Martínez-Aguilar, J. R. Sperling, M. Drummond, A. Halder, A. Dodd, K. Barr, S. Savage, J. Fornell, J. Sort, C. Guger, R. Villa, K. Kostarelos, R. C. Wykes, A. Guimerà-Brunet and J. A. Garrido, Nat. Nanotechnol., 2022, 17, 301–309 CrossRef CAS PubMed.
- C. Capone, B. Rebollo, A. Muñoz, X. Illa, P. Del Giudice, M. V. Sanchez-Vives and M. Mattia, Cereb. Cortex, 2019, 29, 319–335 CrossRef PubMed.
- M. V. Sanchez-Vives, Curr. Opin. Physiol., 2020, 15, 217–223 CrossRef.
- M. V. Sanchez-Vives, M. Massimini and M. Mattia, Neuron, 2017, 94, 993–1001 CrossRef CAS PubMed.
- M. V. Sanchez-Vives, M. Mattia, A. Compte, M. Perez-Zabalza, M. Winograd, V. F. Descalzo and R. Reig, J. Neurophysiol., 2010, 104, 1314–1324 CrossRef PubMed.
- J. Covelo, A. Camassa, J. M. Sanchez-Sanchez, A. Barbero-Castillo, R. M. Robles, N. Cancino-Fuentes, A. Manasanch, S. Tapia-Gonzalez, M. Carreño, J. Rumià Arboix, E. Conde, P. Roldán, J. DeFelipe and M. V. Sanchez-Vives, Clin. Neurophysiol., 2023, 148, e58–e59 CrossRef.
- M. Bikson, P. Grossman, C. Thomas, A. L. Zannou, J. Jiang, T. Adnan, A. P. Mourdoukoutas, G. Kronberg, D. Truong, P. Boggio, A. R. Brunoni, L. Charvet, F. Fregni, B. Fritsch, B. Gillick, R. H. Hamilton, B. M. Hampstead, R. Jankord, A. Kirton, H. Knotkova, D. Liebetanz, A. Liu, C. Loo, M. A. Nitsche, J. Reis, J. D. Richardson, A. Rotenberg, P. E. Turkeltaub and A. J. Woods, Brain Stimul., 2016, 9, 641–661 CrossRef PubMed.
- M. A. Jog, C. Anderson, A. Kubicki, M. Boucher, A. Leaver, G. Hellemann, M. Iacoboni, R. Woods and K. Narr, Sci. Rep., 2023, 13, 2841 CrossRef CAS PubMed.
- B. Rebollo, B. Telenczuk, A. Navarro-Guzman, A. Destexhe and M. V. Sanchez-Vives, Sci. Adv., 2021, 7, eabc7772 CrossRef PubMed.
- M. D'Andola, M. Giulioni, V. Dante, P. Del Giudice and M. V. Sanchez-Vives, J. Neuroeng. Rehabil., 2019, 16, 7 CrossRef PubMed.
- N. Schaefer, R. Garcia-Cortadella, J. Martínez-Aguilar, G. Schwesig, X. Illa, A. Moya Lara, S. Santiago, C. Hébert, G. Guirado, R. Villa, A. Sirota, A. Guimerà-Brunet and J. A. Garrido, 2D Mater., 2020, 7, 025046 CrossRef CAS.
- A. Suarez-Perez, G. Gabriel, B. Rebollo, X. Illa, A. Guimerà-Brunet, J. Hernández-Ferrer, M. T. Martínez, R. Villa and M. V. Sanchez-Vives, Front. Neurosci., 2018, 12, 862 CrossRef PubMed.
- F. Fröhlich and D. A. McCormick, Neuron, 2010, 67, 129–143 CrossRef PubMed.
-
M. D'Andola, J. Weinert, M. Mattia and M. Sanchez-Vives, bioRxiv, 2018, 246819, DOI:10.1101/246819.
- P. Virtanen, R. Gommers, T. E. Oliphant, M. Haberland, T. Reddy, D. Cournapeau, E. Burovski, P. Peterson, W. Weckesser, J. Bright, S. J. van der Walt, M. Brett, J. Wilson, K. J. Millman, N. Mayorov, A. R. J. Nelson, E. Jones, R. Kern, E. Larson, C. J. Carey, İ. Polat, Y. Feng, E. W. Moore, J. VanderPlas, D. Laxalde, J. Perktold, R. Cimrman, I. Henriksen, E. A. Quintero, C. R. Harris, A. M. Archibald, A. H. Ribeiro, F. Pedregosa, P. van Mulbregt, A. Vijaykumar, A. Pietro Bardelli, A. Rothberg, A. Hilboll, A. Kloeckner, A. Scopatz, A. Lee, A. Rokem, C. N. Woods, C. Fulton, C. Masson, C. Häggström, C. Fitzgerald, D. A. Nicholson, D. R. Hagen, D. V. Pasechnik, E. Olivetti, E. Martin, E. Wieser, F. Silva, F. Lenders, F. Wilhelm, G. Young, G. A. Price, G.-L. Ingold, G. E. Allen, G. R. Lee, H. Audren, I. Probst, J. P. Dietrich, J. Silterra, J. T. Webber, J. Slavič, J. Nothman, J. Buchner, J. Kulick, J. L. Schönberger, J. V. de Miranda Cardoso, J. Reimer, J. Harrington, J. L. C. Rodríguez, J. Nunez-Iglesias, J. Kuczynski, K. Tritz, M. Thoma, M. Newville, M. Kümmerer, M. Bolingbroke, M. Tartre, M. Pak, N. J. Smith, N. Nowaczyk, N. Shebanov, O. Pavlyk, P. A. Brodtkorb, P. Lee, R. T. McGibbon, R. Feldbauer, S. Lewis, S. Tygier, S. Sievert, S. Vigna, S. Peterson, S. More, T. Pudlik, T. Oshima, T. J. Pingel, T. P. Robitaille, T. Spura, T. R. Jones, T. Cera, T. Leslie, T. Zito, T. Krauss, U. Upadhyay, Y. O. Halchenko and Y. Vázquez-Baeza, Nat. Methods, 2020, 17, 261–272 CrossRef CAS PubMed.
- A. R. Brunoni, M. A. Nitsche, N. Bolognini, M. Bikson, T. Wagner, L. Merabet, D. J. Edwards, A. Valero-Cabre, A. Rotenberg, A. Pascual-Leone, R. Ferrucci, A. Priori, P. S. Boggio and F. Fregni, Brain Stimul., 2012, 5, 175–195 CrossRef PubMed.
- A. J. Bullard, B. C. Hutchison, J. Lee, C. A. Chestek and P. G. Patil, Neuromodulation, 2020, 23, 411–426 CrossRef PubMed.
- M. Vöröslakos, Y. Takeuchi, K. Brinyiczki, T. Zombori, A. Oliva, A. Fernández-Ruiz, G. Kozák, Z. T. Kincses, B. Iványi, G. Buzsáki and A. Berényi, Nat. Commun., 2018, 9, 483 CrossRef PubMed.
- R. Reig, M. Mattia, A. Compte, C. Belmonte and M. V. Sanchez-Vives, J. Neurophysiol., 2010, 103, 1253–1261 CrossRef CAS PubMed.
-
R. Gutzen, G. De Bonis, C. De Luca, E. Pastorelli, C. Capone, A. L. A. Mascaro, F. Resta, A. Manasanch, F. S. Pavone, M. V. Sanchez-Vives, M. Mattia, S. Grün, P. S. Paolucci and M. Denker, arXiv 2023, arXiv:2211.08527, 1–33, DOI:10.48550/arXiv.2211.08527.
- M. V. Sanchez-Vives and D. A. McCormick, Nat. Neurosci., 2000, 3, 1027–1034 CrossRef CAS PubMed.
- M. Steriade, A. Nunez and F. Amzica, J. Neurosci., 1993, 13, 3252–3265 CrossRef CAS PubMed.
- J. M. Smith, D. P. Bradley, M. F. James and C. L.-H. Huang, Biol. Rev., 2006, 81, 457 CrossRef PubMed.
- A. C. Charles and S. M. Baca, Nat. Rev. Neurol., 2013, 9, 637–644 CrossRef PubMed.
- E. Masvidal-Codina, T. M. Smith, D. Rathore, Y. Gao, X. Illa, E. Prats-Alfonso, E. Del Corro, A. B. Calia, G. Rius, I. Martin-Fernandez, C. Guger, P. Reitner, R. Villa, J. A. Garrido, A. Guimerà-Brunet and R. C. Wykes, J. Neural Eng., 2021, 18, 055002 CrossRef PubMed.
- S. Hunt, Y. Leibner, E. J. Mertens, N. Barros-Zulaica, L. Kanari, T. S. Heistek, M. M. Karnani, R. Aardse, R. Wilbers, D. B. Heyer, N. A. Goriounova, M. B. Verhoog, G. Testa-Silva, J. Obermayer, T. Versluis, R. Benavides-Piccione, P. de Witt-Hamer, S. Idema, D. P. Noske, J. C. Baayen, E. S. Lein, J. DeFelipe, H. Markram, H. D. Mansvelder, F. Schürmann, I. Segev and C. P. J. de Kock, Cereb.Cortex, 2023, 33, 2857–2878 CrossRef PubMed.
- J. DeFelipe, Front. Neuroanat., 2011, 5, 1–17 Search PubMed.
- N. A. Goriounova, D. B. Heyer, R. Wilbers, M. B. Verhoog, M. Giugliano, C. Verbist, J. Obermayer, A. Kerkhofs, H. Smeding, M. Verberne, S. Idema, J. C. Baayen, A. W. Pieneman, C. P. de Kock, M. Klein and H. D. Mansvelder, eLife, 2018, 7, e41714 CrossRef PubMed.
- S. Vanhatalo, J. M. Palva, M. D. Holmes, J. W. Miller, J. Voipio and K. Kaila, Proc. Natl. Acad. Sci. U. S. A., 2004, 101, 5053–5057 CrossRef CAS PubMed.
- H. L. Kaye, D. San-Juan, R. Salvador, M. C. Biagi, L. Dubreuil-Vall, U. Damar, A. Pascual-Leone, G. Ruffini, M. M. Shafi and A. Rotenberg, J. Clin. Neurophysiol., 2023, 40, 53–62 CrossRef PubMed.
- D. San-Juan, L. Morales-Quezada, A. J. Orozco Garduño, M. Alonso-Vanegas, M. F. González-Aragón, D. A. Espinoza López, R. Vázquez Gregorio, D. J. Anschel and F. Fregni, Brain Stimul., 2015, 8, 455–464 CrossRef PubMed.
- D. Yang, Q. Wang, C. Xu, F. Fang, J. Fan, L. Li, Q. Du, R. Zhang, Y. Wang, Y. Lin, Z. Huang, H. Wang, C. Chen, Q. Xu, Y. Wang, Y. Zhang, Z. Zhang, X. Zhao, X. Zhao, T. Li, C. Liu, Y. Niu, Q. Zhou, Q. Zhou, Y. Duan, X. Liu, T. Yu, Q. Xue, J. Li, X. Dai, J. Han, C. Ren, H. Xu, N. Li, J. Zhang, N. Xu, K. Yang and Y. Wang, Brain Stimul., 2020, 13, 109–116 CrossRef PubMed.
- D. Reato, A. Rahman, M. Bikson and L. C. Parra, J. Neurosci., 2010, 30, 15067–15079 CrossRef CAS PubMed.
- A. Rahman, D. Reato, M. Arlotti, F. Gasca, A. Datta, L. C. Parra and M. Bikson, J. Physiol., 2013, 591, 2563–2578 CrossRef CAS PubMed.
- R. C. Wykes, E. Masvidal–Codina, A. Guimerà–Brunet and J. A. Garrido, Clin. Transl. Med., 2022, 12, e968 CrossRef PubMed.
- A. Mitra, A. Z. Snyder, E. Tagliazucchi, H. Laufs and M. E. Raichle, eLife, 2015, 4, e10781 CrossRef PubMed.
- M. L. Kringelbach, A. R. McIntosh, P. Ritter, V. K. Jirsa and G. Deco, Trends Cognit Sci., 2015, 19, 616–628 CrossRef PubMed.
- M. Senden, N. Reuter, M. P. van den Heuvel, R. Goebel and G. Deco, Neuroimage, 2017, 146, 561–574 CrossRef PubMed.
- C. Favaretto, M. Allegra, G. Deco, N. V. Metcalf, J. C. Griffis, G. L. Shulman, A. Brovelli and M. Corbetta, Nat. Commun., 2022, 13, 5069 CrossRef CAS PubMed.
- Y. I. Sheline, M. E. Raichle, A. Z. Snyder, J. C. Morris, D. Head, S. Wang and M. A. Mintun, Biol. Psychiatry, 2010, 67, 584–587 CrossRef CAS PubMed.
- S. Li, N. Hu, W. Zhang, B. Tao, J. Dai, Y. Gong, Y. Tan, D. Cai and S. Lui, Front. Mol. Psychiatry, 2019, 10, 482 CrossRef PubMed.
- Y. I. Sheline and M. E. Raichle, Biol. Psychiatry, 2013, 74, 340–347 CrossRef PubMed.
- A. Ikeda, K. Terada, N. Mikuni, R. C. Burgess, Y. Comair, W. Taki, T. Hamano, J. Kimura, H. O. Lüders and H. Shibasaki, Epilepsia, 1996, 37, 662–674 CrossRef CAS PubMed.
- J. P. Dreier and C. Reiffurth, Neuron, 2015, 86, 902–922 CrossRef CAS PubMed.
- M. Lauritzen, J. P. Dreier, M. Fabricius, J. A. Hartings, R. Graf and A. J. Strong, J. Cereb. Blood Flow Metab., 2011, 31, 17–35 CrossRef PubMed.
- Y. Katayama, D. P. Becker, T. Tamura and D. A. Hovda, J. Neurosurg., 1990, 73, 889–900 CAS.
- H. Mohan, M. B. Verhoog, K. K. Doreswamy, G. Eyal, R. Aardse, B. N. Lodder, N. A. Goriounova, B. Asamoah, A. B. C. B. Brakspear, C. Groot, S. van der Sluis, G. Testa-Silva, J. Obermayer, Z. S. R. M. Boudewijns, R. T. Narayanan, J. C. Baayen, I. Segev, H. D. Mansvelder and C. P. J. de Kock, Cereb. Cortex, 2015, 25, 4839–4853 CrossRef PubMed.
- R. Csercsa, B. Dombovári, D. Fabó, L. Wittner, L. Erőss, L. Entz, A. Sólyom, G. Rásonyi, A. Szűcs, A. Kelemen, R. Jakus, V. Juhos, L. Grand, A. Magony, P. Halász, T. F. Freund, Z. Maglóczky, S. S. Cash, L. Papp, G. Karmos, E. Halgren and I. Ulbert, Brain, 2010, 133, 2814–2829 CrossRef PubMed.
|
This journal is © The Royal Society of Chemistry 2024 |
Click here to see how this site uses Cookies. View our privacy policy here.