DOI:
10.1039/D3TB02457A
(Perspective)
J. Mater. Chem. B, 2024,
12, 2711-2719
Enzymatic micro/nanomotors in biomedicine: from single motors to swarms
Received
18th October 2023
, Accepted 19th December 2023
First published on 19th January 2024
Abstract
Micro/nanomotors (MNMs) have evolved from single self-propelled entities to versatile systems capable of performing one or multiple biomedical tasks. When single MNMs self-assemble into coordinated swarms, either under external control or triggered by chemical reactions, they offer advantages that individual MNMs cannot achieve. These benefits include intelligent multitasking and adaptability to changes in the surrounding environment. Here, we provide our perspective on the evolution of MNMs, beginning with the development of enzymatic MNMs since the first theoretical model was proposed in 2005. These enzymatic MNMs hold immense promise in biomedicine due to their advantages in biocompatibility and fuel availability. Subsequently, we introduce the design and application of single motors in biomedicine, followed by the control of MNM swarms and their biomedical applications. In the end, we propose viable solutions for advancing the development of MNM swarms and anticipate valuable insights into the creation of more intelligent and controllable MNM swarms for biomedical applications.
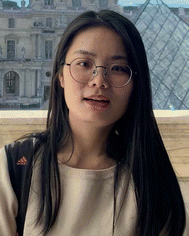
Shuqin Chen
| Shuqin Chen received her master's degree in materials science and engineering from Harbin Institute of Technology (ShenZhen), China, in 2022. She is now a PhD student at the Institute for Bioengineering of Catalonia, Spain. Her research activities focus on studying the interactions among swarms of enzymatic nanomotors. |
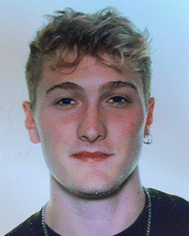
Carles Prado-Morales
| Carles Prado-Morales received his master's degree in molecular biotechnology from the University of Barcelona, Spain, in 2022. He is now a PhD student at the Institute for Bioengineering of Catalonia (IBEC), Spain. His research is focused on developing enzymatic nanomotors for biomedical applications. |
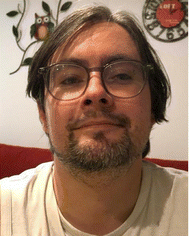
Daniel Sánchez-deAlcázar
| Daniel Sánchez deAlcázar received his PhD degree in Molecular biology and biomedicine from Basque Country University (Spain) in 2020. Since 2023 he has been working as postdoctoral fellow at the Institute for Bioengineering of Catalonia (IBEC), Spain. His research interests are focused on protein engineering for the fabrication of enzymatic nanomotors. |
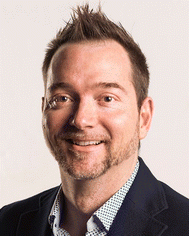
Samuel Sánchez
| Samuel obtained his PhD in Chemistry at Autonomous University of Barcelona in 2008. Currently, he is ICREA Research Professor, Group Leader and Deputy Director at the Institute for Bioengineering of Catalonia. Before that, he worked at the Max Planck Institute for Intelligent Systems Stuttgart, at the Institute for Integrative Nanosciences at IFW Dresden, Germany, and at MANA-NIMS in Japan. His group's main interests are new types of advanced robotics from nano- to mesoscale, including self-propelled nanoparticles as intelligent vehicles in biomedicine to the 3D Bioengineering of biohybrid robots and actuators. |
Introduction
First proposed in the 1950s, nanoscale machines have experienced remarkable advancements in the field of biomedicine in recent decades. Micro/nanomotors (MNMs) are tiny devices that achieve self-propulsion through converting various types of energy into mechanical force.1–7 The characteristic of self-propulsion has shown significant advantages in targeting,8,9 drug delivery,10–12 diagnostics,13,14 antibacterial,15 and in overcoming biological barriers.16 Scientists have designed and constructed various MNMs for diverse applications in the biomedical area, employing different propulsion mechanisms. These mechanisms include external stimuli like magnetic fields,17,18 electric fields,19,20 ultrasound21–23 and light,24,25 as well as internal stimuli like chemical reactions.26,27 Among the myriad of propulsion methods, utilizing enzymes as engines has emerged as a promising choice in the field of biomedical applications, primarily due to their biocompatibility and bioavailability.
The fundamental study of MNMs has evolved, shifting from a primary focus on the propulsion of individual MNMs to pairwise interactions and collective interactions. While individual units may obey simple rules, when assembled into groups in a highly ordered way, they exhibit complex and intriguing swarming behavior.28 In living systems, swarming behavior happens in various phenomena such as self-organization of bacterial colonies, cell migration, bioconvection of microbial suspensions and flocks of fish, ants, and birds. Quorum sensing plays a vital role in collective behaviors, for individuals in a swarm regulate their behaviors based on the density of their neighbors.29 Another common characteristic of swarming behavior is the ability to adapt to dynamically changing environments, which requires energy input.30 By mimicking these features, in the lab, synthetic collective active matters controlled by different energy sources have been exploited.
In this perspective, we aim at introducing how the enzymatic MNMs derives from single enzymes to enzyme driven MNMs, and how the synthetic MNMs achieve highly ordered swarms automatically or under external control. In addition, we introduce the state-of-the-artwork on applying MNMs in overcoming biofluids, biological barriers, and achieving theragnostic both in vitro and in vivo. Furthermore, the MNM swarms are gradually applied in multiple areas in biomedical applications.
The evolution of enzymatic MNMs
Enzymatic catalysis converts chemical energy in situ into mechanical energy and promotes enzyme self-propulsion31 (Fig. 1A). The first demonstration of enhanced urease diffusion, indicating substrate dependency, was reported by Sen's group.32 Subsequently, the same group revealed that enzyme motion could be directed towards areas of high substrate concentration, resembling cell behavior and exhibiting a form of chemotaxis.33 However, the enhanced diffusion of single enzymes is controversial regarding the underlying mechanisms, which is mainly attributed to various physicochemical phenomena, including diffusiophoresis,34 conformational changes,35 and exothermicity,36 along with the antichemotaxis.37 Thus, further investigation is required to address several concerns, i.e., minimizing the photophysical artifacts of fluorescence correlation spectroscopy (FCS) measurements and aligning findings with results from complementary techniques such as nuclear magnetic resonance (NMR) or dynamic light scattering (DLS).38–41
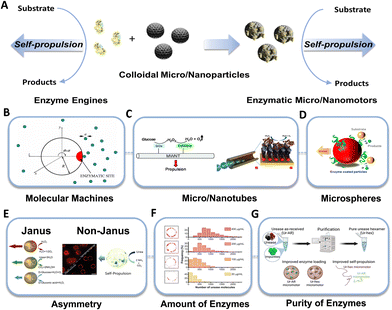 |
| Fig. 1 Motion of individual enzymes and enzymes tethered MNMs. (A) Schematics showing the main mechanisms behind the self-propulsion of individual enzymes and enzyme tethered MNMs. (B) The spherical particle with a reaction site. Reproduced with permission from ref. 42. Copyright 2005 American Physical Society. (C) Autonomous propulsion of carbon nanotubes powered by enzymes, and hybrid biocatalytic microengine. Reproduced with permission from ref. 44 and 45. Copyright 2008 Royal Society of Chemistry, Copyright 2010 American Chemical Society. (D) Micromotors powered by enzyme catalysis. Reproduced with permission from ref. 46. Copyright 2015 American Chemical Society. (E) Janus nanomotors powered by urease, catalase, and glucose oxidase, and non-Janus urease powered micromotors. Reproduced with permission from ref. 26 and 48. Copyright 2022 American Chemical Society, Copyright 2018 American Chemical Society. (F) Quantification of the number of enzymes present on the micromotors surface. Reproduced with permission from ref. 48. Copyright 2018 American Chemical Society. (G) Urease purification. Reproduced with permission from ref. 50. Copyright 2022 American Chemical Society. | |
Considering the self-propulsion capability of enzymes, the concept of enzymatic MNMs was proposed and was initially explored through theoretical demonstrations by Golestanian's group.42 In those theoretical studies, a spherical particle has a fixed single enzymatic site that releases asymmetrical reaction products, thus propelling a molecular machine (Fig. 1B). In the same year, Heller's group43 showed autonomous self-propulsion of conductive carbon fiber decorated with glucose oxidase (GOx) at one end of the fiber and bilirubin oxidase (BOD) at the opposite end. The bioelectrochemical reaction, at the air–liquid interface, promotes the flow of electrons from the anode (GOx) to the cathode (BOD) within the carbon fiber, which is essential for the fiber's motion. Furthermore, the authors claimed that a linear or spiral trajectory could be controlled by altering the GOx/BOD ratios. Subsequently Feringa's group44 experimentally demonstrated the theory of enzymatic nanotubes propelled by asymmetrical reaction products (Fig. 1C). Covalent attachment of glucose oxidase and catalase to carbon nanotubes enables the tandem catalytic conversion of the natural fuel glucose to kinetic energy. Later, Sánchez's group45 designed rolled-up microtubes propelled by covalently bounded catalase enzyme inside the micromotors’ cavity (Fig. 1C). These hybrid microengines achieved high efficiency and allow the microtubes to move at a very low concentration of fuel.
Sen's group46 experimentally demonstrated enzymatic spherical MNMs (Fig. 1D). They immobilized urease and catalase separately on polystyrene microparticles, showing diffusion enhancement in a substrate concentration-dependent manner, like the behavior exhibited by single enzymes. In the same year, Sánchez's group26 reported the first biocompatible Janus nanobots, emphasizing the significance of asymmetry in enhancing motion and supporting previous theoretical studies done by Golestanian (Fig. 1E). Similarly, Städler's team47 designed Janus silica nanoparticles driven by a harmless tandem reaction involving the glucose oxidase–catalase pair. Subsequently, Sánchez's group48 revealed that asymmetric distribution of enzymes could also be induced in non-Janus micromotors through the utilization of glutaraldehyde as a crosslinker (Fig. 1E). In a similar line, Wilson's group49 encapsulated GOx and catalase within stomatocytes, which are polymer vesicles with bowl-shape structure. This approach resulted in self-propulsion at high speed.
Some crucial parameters need to be considered when designing and constructing these enzymatic MNMs, including the amount, the purity, and asymmetric distributions of enzymes, as well as the presence of electrolytes in the media, and the size and shape of the MNMs50–54 (Fig. 1E–G). Additionally, some intrinsic features of the enzymes are equally important, i.e., molecular weight of the substrate and products,39,55 as well as high turnover number56 and the chemical binding.57 Overall, these studies collectively contributed to the evolving landscape of MNMs research, offering new avenues for exploration in biomedical applications.
Biomedical applications of MNMs
The human body is an extremely complex system with sophisticated barriers that protect it from external threats. To effectively reach the targeted area and exert their therapeutic effects, MNMs must travel through challenging environments and overcome barriers, e.g., mucus,58,59 blood brain barrier,60 skin,61,62 and eye vitreous.63 Moreover, evaluating therapeutic outcomes in a real body system is more challenging compared to simple in vitro cell models.
Stage 1: movement in complex media
Using body fluids for travelling is an arduous task, mainly due to the presence of mesh structures and high viscosity. Mesh structures limit the movement of MNMs in a size-dependent manner, requiring the same size range between MNMs and the mesh. Fisher's group64 demonstrated that helical nanopropellers with a filament diameter of about 70 nm showed advantage when move through high-viscosity solutions, with comparable velocities to that of larger micropropellers (Fig. 2A). This is mainly because these nanopropellers are of the same size range as the gel's mesh size. In case of high viscosity, acoustic propulsion has been proved to be effective for moving in complex biological fluids such as blood, mucus, or fetal bovine serum.65 Another possibility is using enzymes for modulating matrix networks, which would allow MNMs to go through it. For instance, Städler’ s group66 showed that an extracellular matrix model made of collagen fibers would not be a movement limitation when nanomotors are supplied with collagenase (Fig. 2B).
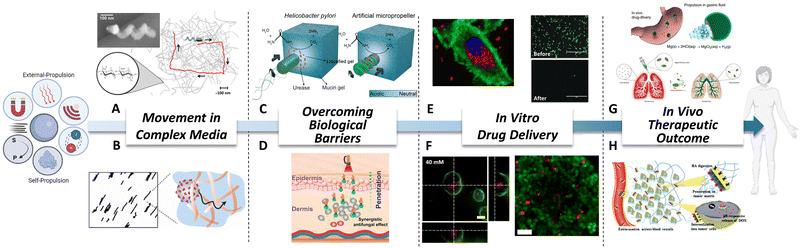 |
| Fig. 2 Biomedical advantages of MNMs. (A) Schematic of helical micropropellers moving in high viscous media while magnetically guided. Reproduced with permission from ref. 64. Copyright 2014 American Chemical Society. (B) Nanomotors functionalized with collagenase traveling through a collagen fibers network. Reproduced with permission from ref. 66. Copyright 2022 American Chemical Society. (C) Magnetic micropropellers overcoming mucus barrier by mimicking Helicobacter Pylory mechanism. Reproduced with permission from ref. 16. Copyright 2015 The Authors. (D) Near Infrared powered nanomotors actively crossing skin. Reproduced with permission from ref. 61. Copyright 2021 American Chemical Society. (E) On the left, urease-nanomotors delivering drugs intracellularly in a pH responsive manner. Reproduced with permission from ref. 70. Copyright 2019 American Chemical Society. On the right, nanowires powered by ultrasounds provoking and increasing the knock-out of GFP expression due to the delivery of Cas9/sgRNA complex. Reproduced with permission from ref. 23. Copyright 2018 Wiley-VCH Verlag GmbH & Co. KGaA, Weinheim. (F) On the left, urease-nanomotors penetrating 3D bladder cancer spheroids. Reproduced with permission from ref. 71. Copyright 2018 American Chemical Society. On the right, microswimmers reaching and killing cells in deep spheroids areas. Reproduced with permission from ref. 72. Copyright 2019 American Chemical Society. (G) On top, Mg-micromotors against gastric bacterial infections in vivo. Reproduced with permission from ref. 73. Copyright 2017 The Author(s). On bottom, hybrid microrobots were tested against acute bacterial pneumonia in a mice model. Reproduced with permission from ref. 74. Copyright 2022 The Author(s), under exclusive licence to Springer Nature Limited. (H) Janus nanorods travelling through extracellular matrix for achieving increased chemotherapy delivery and tumor diffusion in vivo. Reproduced with permission from ref. 75 Copyright 2021 Royal Society of Chemistry. | |
Stage 2: overcoming biological barriers
The presence of biological barriers in the body is considerably restricting the possible biomedical applications of nanotechnology. Nevertheless, huge steps forward have been made in the MNMs field demonstrating how active nanocarriers can overcome them. The principles that prove to be effective for nature are applicable to scientific purposes. Inspired by Helicobacter Pylori, magnetic micropropellers with urease were able to penetrate a mucus barrier, showing how externally manipulated micromotors actively and reversibly modify their surroundings16 (Fig. 2C). The significance of these applications becomes evident when considering local administration and alternative routes that offer less side effects and more efficient treatments. In the context of biological barriers, skin, which is the largest organ, plays a crucial role in safeguarding the entire body against the external environment. The outermost layer of the skin, known as the stratum corneum, effectively diminishes the bioavailability of topical formulations.67 A couple of recent approaches have used near infrared light for powering nanomotors and crossing mice skin for antifungal therapy61,62 (Fig. 2D). Moreover, nanomotors have also shown enhancement in biofilm infiltration.68 Regarding intravitreal delivery, vitreous humor acts as a barrier, impeding the access of substances to the retina. One innovative solution to this limitation involves the use of slippery helical micropropellers powered by magnetic fields. These micropropellers have shown enhanced penetration in the vitreous humor, effectively overcoming the barrier and reaching the retina.63
Stage 3: in vitro drug delivery
In the preceding lines, the advantages of active motion in crossing complex media were clearly demonstrated. In this regard, it is crucial to determine the actual effectiveness of drug delivery in the target cells using active motion. Taking advantage of drug loading capacities of MNMs, Doxorubicin was loaded into urease-nanomotors, and its release increased four-fold in the presence of fuel, showing an enhanced anticancer effect against HeLa cells.69 It is worth noting that these nanomotors also showed intelligent drug release in a pH-responsive manner when gating the pores.70 As a result, in the presence of fuel, an enhancement in the internalization and intracellular drug release was observed in 2D HeLa cell culture (Fig. 2E, left). New advanced therapies are emerging, and MNMs are successfully keeping abreast of these advancements. Ultrasound nanowires loaded with a Cas9/sgRNA complex were able to overcome physiological barriers for intra-cellular delivery of functional proteins and RNAs, achieving more than 80% GFP gene knockout compared to 30% knockout observed with the passive ones23 (Fig. 2E, right). Concerning cancer treatment, MNMs have awakened special interest as a promising strategy. Active drug-loaded urease-nanomotors exhibited enhanced penetration within bladder cancer 3D spheroids. Moreover, incorporating a targeting antibody on their surface augments penetration, leading to tumor growth inhibition71 (Fig. 2F, left). Heat delivery via incorporated magnetic nanoparticles also benefits from the increased tumor penetration. This approach facilitates localized temperature elevation within deep tumor regions, resulting in localized cell death in the tumor core72 (Fig. 2F, right).
Stage 4: in vivo therapeutic outcome
In recent years, there has been a growing interest in the application of MNMs in in vivo models to assess their potential in living organisms, following the demonstrated effectiveness and safety observed in in vitro models. To the best of our knowledge, in 2017 therapeutic magnesium micromotors for active drug delivery were successfully tested in vivo for the first time.73 In that case, magnesium-micromotors loaded with an antibiotic significantly reduced a gastric bacterial infection in mice model (Fig. 2G, top). Some years later, the same group tested the effectiveness of hybrid micromotors against acute bacterial pneumonia.74 The observed antibacterial effect in an in vivo mice model stemmed primarily from higher speed in lung fluid, uniform deep tissue distribution and enhanced retention time (Fig. 2G, bottom). Icebreaker-inspired Janus nanorods, functionalized with urease and hyaluronidase, demonstrated how the ability to travel through complex media can lead to a beneficial therapeutic outcome in vivo.75 As can be seen by increased diffusion in tumors and the consequent enhancement in chemotherapy delivery (Fig. 2H). In the case of injecting urease-nanomotors into the bladder, they reached deeper bladder tissue areas and were able to remain even after repeated urination.76 It is worth noting that no toxic effects were detected in other organs of the body throughout these studies, providing an initial safety assessment for these products.
MNM swarms driven by different types of mechanisms
When evaluating potential applications, the impact of an individual motor might not lead to desirable therapeutic outcomes. Some groups have progressed towards swarming behavior of MNMs. These MNMs function as a coordinated group, aim at a shared goal, exhibit synergistic effects and enhance their overall efficiency.
As happens at the single motor level, swarms could be configurated through external stimuli, such as magnetic fields, acoustics, light, and electric fields. Magnetic fields have the advantage of high permeability. When exposed to magnetic fields, magnetic swarms show well-controlled collective behavior, environmental adaptability and enhanced capabilities. For example, Sitti's group77 used rotating magnetic fields to induce the dynamic self-assembly of magnetic microparticles into linear chain-type swarms. Attractive and repulsive interactions among microparticles allow well-defined spatial organization. He's group78 used haematite colloidal particles for liquid, chain, vortex, and ribbon-like four main collective formations, which can flexibly transform from one to another in a confined environment, showing great potential in future biomedicine (Fig. 3A). External electrical field induces and tunes communications among colloids through dipolar interactions.79 For instance, Janus particles composed of silica and metal hemisphere polarize differently in an electric field, thus leading to self-propulsion of particles.80 When electric-field frequency is low, the particles move and collide randomly and isotropically. As the electric-field frequency increases, particles interact with each other, with their metallic side facing forwards, aligning during binary collisions and organizing into swarms (Fig. 3B). Electric fields offer advantages such as ease of generation and simplified systems compared to magnetic control, making them a generally applicable tool for studying collective behavior. Acoustic fields have noncontact characters and biocompatibility with biological systems. Wang's group81 for the first time used acoustic fields to trigger the assembly of catalytic Pt–Au nanowire motors and regulate their collective behavior (Fig. 3C). By changing the frequency of acoustic field, He's group82 demonstrated the dynamic aggregation of dandelions-like liquid metal colloidal motors. Collective behaviors could be dynamically repeated by combining acoustic field and switching UV light on and off as well. Wang's group83 controlled the aggregation of hybrid TiO2-Au microbowl motors at low pressure regions (nodes) in an acoustic pressure gradient. Upon UV light irradiation, the aggregates move away from the node center.
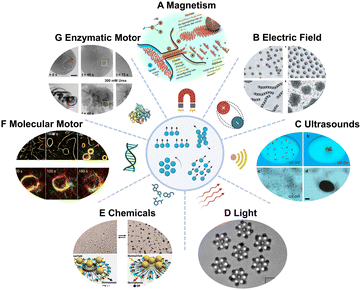 |
| Fig. 3 MNM swarms driven by different mechanisms. (A) Multimode transformations and collective manipulation of magnetic fields guided hematite colloidal microrobots. Reproduced with permission from ref. 78. Copyright 2019 The Authors, some rights reserved; exclusive licensee American Association for the Advancement of Science. (B) Different forms of Janus sphere clusters induced by electric field. Reproduced with permission from ref. 80. Copyright 2016 Springer Nature Limited. (C) Swarming behavior of chemically powered Au–Pt nanomotors under the acoustic radiation forces. Reproduced with permission from ref. 81. Copyright 2015 American Chemical Society. (D) Hierarchical self-assembly of self-spinning rotors under the control of light. Reproduced with permission from ref. 84. Copyright 2018 The Author(s). (E) Transition between exclusion and schooling behaviors caused by the addition and removal of NH3, respectively. Reproduced with permission from ref. 85. Copyright 2013 American Chemical Society. (F) Association and dissociation of microtubules controlled by DNA. Reproduced with permission from ref. 86. Copyright 2018 The Author(s). (G) Swarming behavior of enzymatic nanomotor swarms with and without fuel. Reproduced with permission from ref. 87. Copyright 2021 The Authors, some rights reserved; exclusive licensee American Association for the Advancement of Science. | |
Collective behaviors induced by light or chemicals have been intensively studied.88–90 Micro-sized particles like silver chloride (AgCl) and titania–silica Janus particles can be activated under UV light illumination. These photocatalytic reactions cause local chemical gradient and attract nearby passive silica colloids, which assembled into swarms due to self-diffusiophoretic effects.91,92 Palacci's group84 developed a phototactic swimmer based on haematite. This photocatalytic material harvests energy from hydrogen peroxide fuel and forms hierarchical superstructures through non-equilibrium pathways (Fig. 3D). Another important effect on light controlled MNMs is self-osmophoresis. Controlled by blue light, phoretic and osmotic effects in living crystals can be switched on and off conveniently.93 This results in the formation of crystallites that dynamically translate and rotate, collided, joined, and split. These light-induced collective behaviors were well studied regarding the mechanisms. However, due to the limited penetration of UV or visible light sources, they are not suitable for deep tissue biomedical applications. Chemicals as external stimulus can regulate reversible collective behaviors of MNMs in a way similar to light. Chemically powered motors are surrounded by a cloud of reactants and products that can ‘signal’ their neighbors and thus influence their interactions. Sen's group85 showed the transition of Ag3PO4 microparticles between ‘exclusion’ and ‘schooling’ by addition or removal of NH3 based on a self-diffusiophoresis mechanism (Fig. 3E). Wang's group94 demonstrated the swarming of gold microparticles when adding hydrazine into a hydrogen peroxide solution. The control of the swarms could be tailored by particles surface modification.
In addition to these extensively studied MNM swarms, swarming behavior of molecular machines and enzymatic nanomotors have also been reported. Kakugo's group86 reported DNA-functionalized kinesin-propelled microtubes, which interact with each other using DNA crosslinkers as signal input (Fig. 3F). Such behaviors could be regulated as translational or circular motion by tuning the physical properties of microtubes. Further control could be achieved by photoirradiation if azobenzene is introduced into the DNA strands.95 Enzymatic nanomotor swarms were reported for the first time by Sánchez's group87 (Fig. 3G). In the presence of fuel, urease modified mesoporous nanomotor swarms show vortices and unstable fronts, inducing enhanced fluid mixing and convection. However, the underlying mechanism of those enzymatic nanomotor swarms has not been revealed. Enzymatic MNM swarms offer distinct advantages in the field of biomedicine, particularly in terms of their ability to autonomously propel in situ without the need for external control. This autonomy can also prove beneficial when integrating enzyme-based mechanisms with external control because MNMs reduce the reliance of external input.96 For instance, swarms of magnetic motors present configurable control over their motion. Therefore, incorporating magnetic moieties may improve the controllability of swarms of enzyme motors in the future.
Biomedical applications of MNM swarms
The dynamics of MNM swarms, when controlled in a proper way, could be well applied in biomedicine. In in vitro contexts, some research groups have brought MNM swarms into practice. Sánchez's group97 explored enzymatic nanomotor swarms and their role in enhancing delivery toward HeLa cells (Fig. 4A). Taking advantage of urease-powered motion and swarming behavior, a first troop of enzymatic swarms could destroy biological barriers and reduce steric hindrance by vapor nanobubbles upon optical trigger. Then the delivery efficiency of a second troop of nanomotor swarms was 10-fold increase in the presence of fuel compared to that without fuel. Mou's group98 explored tumor treatment using nanomotors, where synthetic photonic nanorobots could actively navigate in complex environments through their controllable swarming motions. Additionally, via their responsive structural colors, these nanorobots could map local physicochemical conditions on the fly (Fig. 4B). These motile nano sensors can further guide light irradiation for local photothermal therapy in vitro.99
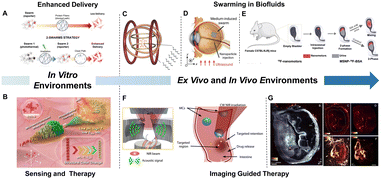 |
| Fig. 4 Biomedical applications of MNM swarms. (A) Swarms of enzymatic nanomotors for enhanced delivery. Reproduced with permission from ref. 97. Copyright 2022 The Authors. (B) Photonic nanorobot swarms for mapping-guided localized photothermal tumor therapy. Reproduced with permission from ref. 98. Copyright 2023 The Author(s). (C) Controlled swimming of a swarm of artificial bacterial flagella. Reproduced with permission from ref. 101. Copyright 2015 WILEY-VCH Verlag GmbH & Co. KGaA, Weinheim. (D). Ex vivo validations of the medium-induced swarms in bovine eyeballs. Reproduced with permission from ref. 102. Copyright 2019 The Author(s). (E) Biodistribution of enzymatic nanomotor swarms in bladders via PET-CT. Reproduced with permission from ref. 87. Copyright 2021 The Authors, some rights reserved; exclusive licensee American Association for the Advancement of Science. (F) Schematic of the micromotor capsules in the gastrointestinal tract in vivo by photoacoustic computed tomography and targeted retention and delivery. Reproduced with permission from ref. 103. Copyright 2019 The Authors, some rights reserved; exclusive licensee American Association for the Advancement of Science. (G) Nanobot swarms penetrate the bladder tumor. Reproduced with permission from ref. 104. Copyright 2023 openly licensed via a Creative Commons Attribution 4.0 International License. | |
Facilitating the clinical application of synthetic MNM swarms requires efficient platforms for real-time tracking in biofluids and deep tissues.100 Several groups have studied the movement of synthetic MNMs in biofluids. By functionalizing a NIR probe, a swarm of bacteria-like microrobotic flagella was tracked and visualized in the body of a mouse101 (Fig. 4C). In addition, it was found that while the speed of the swarms was lower in vivo than in vitro, the 3D motion could still be achieved. In another work, based on selecting the optimized swarms in specific biofluids, Zhang's group102 demonstrated the ex vivo generation of medium-induced swarms and their targeted deliveries in a bovine eyeball (Fig. 4D). Another issue to be addressed is precise on-demand control. Gao's group103 used photoacoustic computed tomography for tracking and visualizing the migration of microrobotic systems toward targeted areas in intestines in real time (Fig. 4F). It was seen how cargo-loaded micromotors could be released on-demand upon NIR irradiation.
Recently, enzymatic MNMs have also been reported to show swarming behavior, which has been tracked both in vitro and in vivo. Sánchez's group87 investigated the swarming behavior of enzyme-powered nanomotors in bladders based on both optical microscopy and PET-CT (Fig. 4E). When intravesically instilled in mice bladder in vivo, the nanomotors could homogeneously distribute in the whole cavity. Urease-powered nanobot swarms were taken one step closer toward translational applications as therapeutical tools104 (Fig. 4G). By combining advanced imaging techniques, the enzymatic nanomotor swarms exhibit enhanced accumulation and penetration in an orthotopic murine model of bladder cancer. Taking full advantage of this motion-enhanced behavior, the nanomotor swarms show localized radiotherapeutic effects at doses significantly below those required to be efficient.
Outlook
The evolution of MNMs has seen significant progress from fundamental locomotion at micro/nanoscale to their biomedical applications in in vivo biomedical contexts.105 The emergence of MNM swarms has attracted increasing attention, leading to investigations into various modes of manipulation.84,92,93 Some of these swarms have demonstrated practical applications in vivo and shown clear advantages over single MNMs.104 Looking ahead here are several suggestions that may help the future development of MNMs.
First, despite the great achievements that have been acquired, at least three main points should be considered for clinical applications: simplicity, biodegradability, and reproducibility. Sophisticated designs will have hard times for reaching industry, basically because of the need of complex and expensive scalability processes. Another vital factor is that all these products must be approved by regulatory agencies like the FDA and EMA, which is not easy if there is no complete biocompatibility or significant advantages compared with current treatments. Finally, high degree of reproducibility must be achieved. Now, looking at most of the publications cited in this perspective and the current state of the art in general, they demonstrated that it is indeed feasible to use MNMs in biomedicine. However, most of these designs do not have a real translation. Now is the time to make it doable by utilizing the simplest and the most biocompatible system that is also cost-effective and scalable.
Secondly, for enzymatic MNMs, enzymes play vital role in the motion, thus making it crucial to develop and design new enzymes with enhanced properties, where motion can be optimized. One key factor in enhancing motion is the high turnover number. Currently, urease and catalase are prominent players in this field. However, these enzymes possess a drawback that hinders their effective application, particularly in biomedicine: substrate or byproduct toxicity. In this context, the advancement of artificial intelligence (AI) holds the potential to revolutionize the search for the ideal enzyme, thereby improving motion performance. By employing machine learning algorithms and computational models, AI can analyze vast amounts of data and predict enzyme properties with high accuracy.106,107 Moreover, similar to other enzyme-based therapies, there remains an issue regarding immunogenicity. One possible approach involves anchoring polymers to the surface of the enzymes.108 Another option is to reduce the immunogenicity of the protein itself through molecular biology techniques.109
Thirdly, although in some cases the mechanisms of coordinated behavior have been clarified, such as the control of magnetic swarms, there remains a lack of research concerning the mechanism of enzymatic MNM swarms. Therefore, fundamental understanding of interactions among these swarms is desired and encouraged. In addition, achieving better control over collective movement in enzymatic MNM swarms is a critical goal. In this regard, incorporating magnetic moieties and exploring collective chemotaxis could be effective ways to achieve this goal.
Conflicts of interest
There are no conflicts to declare.
Acknowledgements
The research leading to these results has received funding from the grants PID2021-128417OB-I00 and PDC2022-133753-I00 funded by MCIN/AEI/10.13039/501100011033 and, by “ERDF A way of making Europe” and European Union Next Generation EU, (Bots4BB and BOJOS projects). This project has received also funding from the European Research Council (ERC) under the European Union's Horizon 2020 research and innovation programme (grant agreement No 866348, iNanoSwarms), from the Predoctoral AGAUR-FI Joan Oró grant (2023 FI-1 00654) funded by “Secretaria d'Universitats i Recerca del Departament de Recerca i Universitats de la Generalitat de Catalunya” and by European Social Fund Plus, from the grant PRE2022-102542 funded by MCIN/AEI/10.13039/501100011033 and by “ESF Investing in your future”, and from the grant FJC2021-048024-I funded by MCIN/AEI/10.13039/501100011033 and by “European Union NextGenerationEU/PRTR”.
References
- M. Luo, S. Li, J. Wan, C. Yang, B. Chen and J. Guan, Langmuir, 2020, 36, 7005–7013 CAS.
- Z. Yang, L. Wang, Z. Gao, X. Hao, M. Luo, Z. Yu and J. Guan, ACS Nano, 2023, 17, 6023–6035 CrossRef CAS PubMed.
- J. Dai, X. Cheng, X. Li, Z. Wang, Y. Wang, J. Zheng, J. Liu, J. Chen, C. Wu and J. Tang, Adv. Funct. Mater., 2021, 31, 2106204 CrossRef CAS.
- Y. Feng, Y. Yuan, J. Wan, C. Yang, X. Hao, Z. Gao, M. Luo and J. Guan, Appl. Phys. Rev., 2021, 8, 011406 CAS.
- S. Sanchez, L. Soler and J. Katuri, Angew. Chem., Int. Ed., 2015, 54, 1414–1444 CrossRef CAS PubMed.
- S. Chen, Y. Chen, M. Fu, Q. Cao, B. Wang, W. Chen and X. Ma, J. Mater. Chem. B, 2022, 10, 7099–7107 RSC.
- H. Wang and M. Pumera, Adv. Funct. Mater., 2018, 28, 1705421 CrossRef.
- H. Xu, M. Medina-Sánchez, V. Magdanz, L. Schwarz, F. Hebenstreit and O. G. Schmidt, ACS Nano, 2018, 12, 327–337 CrossRef CAS PubMed.
- L. Yang, X. Chen, L. Wang, Z. Hu, C. Xin, M. Hippler, W. Zhu, Y. Hu, J. Li, Y. Wang, L. Zhang, D. Wu and J. Chu, Adv. Funct. Mater., 2019, 29, 1905745 CrossRef CAS.
- M. Sun, X. Fan, X. Meng, J. Song, W. Chen, L. Sun and H. Xie, Nanoscale, 2019, 11, 18382–18392 RSC.
- W. Chen, R. Jiang, X. Sun, S. Chen, X. Liu, M. Fu, X. Yan and X. Ma, Chem. Mater., 2022, 34, 7543–7552 CrossRef CAS.
- L. Wang, P. Guo, D. Jin, Y. Peng, X. Sun, Y. Chen, X. Liu, W. Chen, W. Wang, X. Yan and X. Ma, ACS Nano, 2023, 17, 5095–5107 CrossRef CAS PubMed.
- S. Chen, X. Sun, M. Fu, X. Liu, S. Pang, Y. You, X. Liu, Y. Wang, X. Yan and X. Ma, Biomaterials, 2022, 288, 121744 CrossRef CAS PubMed.
- X. Yan, Q. Zhou, M. Vincent, Y. Deng, J. Yu, J. Xu, T. Xu, T. Tang, L. Bian, Y.-X. J. Wang, K. Kostarelos and L. Zhang, Sci. Robot., 2017, 2, 1155 CrossRef PubMed.
- X. Arqué, M. D. T. Torres, T. Patiño, A. Boaro, S. Sánchez and C. Fuente-Nunez, ACS Nano, 2022, 16, 7547–7558 CrossRef PubMed.
- D. Walker, B. T. Käsdorf, H. H. Jeong, O. Lieleg and P. Fischer, Sci. Adv., 2016, 1, e1500501 CrossRef PubMed.
- Y. Chen, R. Pan, Y. Wang, P. Guo, X. Liu, F. Ji, J. Hu, X. Yan, G. P. Wang, L. Zhang, Y. Sun and X. Ma, Adv. Funct. Mater., 2022, 32, 2200600 CrossRef CAS.
- Y. Wang, Y. Liu, Y. Li, D. Xu, X. Pan, Y. Chen, D. Zhou, B. Wang, H. Feng and X. Ma, Research, 2020, 2020 Search PubMed.
- J. Guo, J. J. Gallegos, A. R. Tom and D. Fan, ACS Nano, 2018, 12, 1179–1187 CrossRef CAS PubMed.
- R. Zhuang, D. Zhou, X. Chang, Y. Mo, G. Zhang and L. Li, Appl. Mater. Today, 2022, 26, 101314 CrossRef.
- T. Xu, L. P. Xu and X. Zhang, Appl. Mater. Today, 2017, 9, 493–503 CrossRef.
- D. Zhou, Y. Gao, J. Yang, Y. C. Li, G. Shao, G. Zhang, T. Li and L. Li, Adv. Sci., 2018, 5, 1800122 CrossRef PubMed.
- M. Hansen-Bruhn, B. E. de Ávila, M. Beltrán-Gastélum, J. Zhao, D. E. Ramírez-Herrera, P. Angsantikul, K. Vesterager Gothelf, L. Zhang and J. Wang, Angew. Chem., Int. Ed., 2018, 130, 2687–2691 CrossRef.
- L. Shao and M. Käll, Adv. Funct. Mater., 2018, 28, 1706272 CrossRef.
- R. Dong, Y. Cai, Y. Yang, W. Gao and B. Ren, Acc. Chem. Res., 2018, 51, 1940–1947 CrossRef CAS PubMed.
- X. Ma, A. Jannasch, U. R. Albrecht, K. Hahn, A. Miguel-López, E. Schäffer and S. Sánchez, Nano Lett., 2015, 15, 7043–7050 CrossRef PubMed.
- X. Ma, K. Hahn and S. Sánchez, J. Am. Chem. Soc., 2015, 137, 4976–4979 CrossRef CAS PubMed.
- H. Wang and M. Pumera, Chem. Soc. Rev., 2020, 49, 3211–3230 RSC.
- T. Bäuerle, A. Fischer, T. Speck and C. Bechinger, Nat. Commun., 2018, 9, 3232–3239 CrossRef PubMed.
- W. Wang, W. Duan, S. Ahmed, A. Sen and T. E. Mallouk, Acc. Chem. Res., 2015, 48, 1938–1946 CrossRef CAS PubMed.
- S. Gáspár, Nanoscale, 2014, 6, 7757–7763 RSC.
- H. S. Muddana, S. Sengupta, T. E. Mallouk, A. Sen and P. J. Butler, J. Am. Chem. Soc., 2010, 132, 2110–2111 CrossRef CAS PubMed.
- S. Sengupta, K. K. Dey, H. S. Muddana, T. Tabouillot, M. E. Ibele, P. J. Butler and A. Sen, J. Am. Chem. Soc., 2013, 135, 1406–1414 CrossRef CAS PubMed.
- J. Agudo-Canalejo, P. Illien and R. Golestanian, Nano Lett., 2018, 18, 2711–2717 CrossRef CAS PubMed.
- P. Illien, X. Zhao, K. K. Dey, P. J. Butler, A. Sen and R. Golestanian, Nano Lett., 2017, 17, 4415–4420 CrossRef CAS PubMed.
- C. Riedel, R. Gabizon, C. A. M. Wilson, K. Hamadani, K. Tsekouras, S. Marqusee, S. Pressé and C. Bustamante, Nature, 2015, 517, 227–230 CrossRef CAS PubMed.
- A. Y. Jee, S. Dutta, Y. K. Cho, T. Tlusty and S. Granick, Proc. Natl. Acad. Sci. U. S. A., 2018, 115, 14–18 CrossRef CAS PubMed.
- J. P. Günther, G. Majer and P. Fischer, J. Chem. Phys., 2019, 150, 124201 CrossRef PubMed.
- A. Y. Jee, T. Tlusty and S. Granick, Proc. Natl. Acad. Sci. U. S. A., 2020, 117, 29435–29441 CrossRef CAS PubMed.
- J. P. Günther, M. Börsch and P. Fischer, Acc. Chem. Res., 2018, 51, 1911–1920 CrossRef PubMed.
- A. Y. Jee, K. Chen, T. Tlusty, J. Zhao and S. Granick, J. Am. Chem. Soc., 2019, 141, 20062–20068 CrossRef CAS PubMed.
- R. Golestanian, T. B. Liverpool and A. Ajdari, Phys. Rev. Lett., 2005, 94, 220801 CrossRef PubMed.
- N. Mano and A. Heller, J. Am. Chem. Soc., 2005, 127, 11574–11575 CrossRef CAS PubMed.
- D. Pantarotto, W. R. Browne and B. L. Feringa, Chem. Commun., 2008, 1533–1535 RSC.
- S. Sanchez, A. A. Solovev, Y. Mei and O. G. Schmidt, J. Am. Chem. Soc., 2010, 132, 13144–13145 CrossRef CAS PubMed.
- K. K. Dey, X. Zhao, B. M. Tansi, W. J. Méndez-Ortiz, U. M. Córdova-Figueroa, R. Golestanian and A. Sen, Nano Lett., 2015, 15, 8311–8315 CrossRef CAS PubMed.
- P. Schattling, B. Thingholm and B. Städler, Chem. Mater., 2015, 27, 7412–7418 CrossRef CAS.
- T. Patiño, N. Feiner-Gracia, X. Arqué, A. Miguel-López, A. Jannasch, T. Stumpp, E. Schäffer, L. Albertazzi and S. Sánchez, J. Am. Chem. Soc., 2018, 140, 7896–7903 CrossRef PubMed.
- L. K. E. A. Abdelmohsen, M. Nijemeisland, G. M. Pawar, G. J. A. Janssen, R. J. M. Nolte, J. C. M. Van Hest and D. A. Wilson, ACS Nano, 2016, 10, 2652–2660 CrossRef CAS PubMed.
- M. Valles, S. Pujals, L. Albertazzi and S. Sánchez, ACS Nano, 2022, 16, 5615–5626 CrossRef CAS PubMed.
- X. Arqué, X. Andrés, R. Mestre, B. Ciraulo, J. Ortega Arroyo, R. Quidant, T. Patiño and S. Sánchez, Research, 2020, 2020 Search PubMed.
- T. Patino, X. Arqué, R. Mestre, L. Palacios and S. Sánchez, Acc. Chem. Res., 2018, 51, 2662–2671 CrossRef CAS PubMed.
- M. De Corato, X. Arqué, T. Patinõ, M. Arroyo, S. Sánchez and I. Pagonabarraga, Phys. Rev. Lett., 2020, 124, 108001 CrossRef CAS PubMed.
- X. Ma, X. Wang, K. Hahn and S. Sánchez, ACS Nano, 2016, 10, 3597–3605 CrossRef CAS PubMed.
- X. Arqué, A. Romero-Rivera, F. Feixas, T. Patiño, S. Osuna and S. Sánchez, Nat. Commun., 2019, 10, 2826–2837 CrossRef PubMed.
- S. Ghosh, F. Mohajerani, S. Son, D. Velegol, P. J. Butler and A. Sen, Nano Lett., 2019, 19, 6019–6026 CrossRef CAS PubMed.
- L. Wang, M. Marciello, M. Estévez-Gay, P. E. D. Soto Rodriguez, Y. Luengo Morato, J. Iglesias-Fernández, X. Huang, S. Osuna, M. Filice and S. Sánchez, Angew. Chem., Int. Ed., 2020, 59, 21080–21087 CrossRef CAS PubMed.
- W. Ban, J. Qu, L. Cai, J. Fu, W. Wu, H. Song, C. Yu and J. Tang, Appl. Mater. Today, 2023, 34, 101916 CrossRef.
- Y. Cao, S. Liu, Y. Ma, L. Ma, M. Zu, J. Sun, F. Dai, L. Duan and B. Xiao, Small, 2022, 18, 2203466 CrossRef CAS PubMed.
- A. Joseph, C. Contini, D. Cecchin, S. Nyberg, L. Ruiz-Perez, J. Gaitzsch, G. Fullstone, X. Tian, J. Azizi, J. Preston, G. Volpe and G. Battaglia, Sci. Adv., 2017, 3, e170036 Search PubMed.
- X. Ji, H. Yang, W. Liu, Y. Ma, J. Wu, X. Zong, P. Yuan, X. Chen, C. Yang, X. Li, H. Lin, W. Xue and J. Dai, ACS Nano, 2021, 15, 14218–14228 CrossRef CAS PubMed.
- L. Liu, S. Li, K. Yang, Z. Chen, Q. Li, L. Zheng, Z. Wu, X. Zhang, L. Su, Y. Wu and J. Song, Nano Lett., 2023, 23, 3929–3938 CrossRef CAS PubMed.
- Z. Wu, J. Troll, H.-H. Jeong, Q. Wei, M. Stang, F. Ziemssen, Z. Wang, M. Dong, S. Schnichels, T. Qiu and P. Fischer, Sci. Adv., 2018, 4, 4388 CrossRef PubMed.
- D. Schamel, A. G. Mark, J. G. Gibbs, C. Miksch, K. I. Morozov, A. M. Leshansky and P. Fischer, ACS Nano, 2014, 8, 8794–8801 CrossRef CAS PubMed.
- A. Aghakhani, A. Pena-Francesch, U. Bozuyuk, H. Cetin, P. Wrede and M. Sitti, Sci. Adv., 2022, 8, 5126 CrossRef PubMed.
- M. A. Ramos Docampo, N. Wang, S. Pendlmayr and B. Städler, ACS Appl. Nano Mater., 2022, 5, 14622–14629 CrossRef CAS.
- J. Hadgraft and M. E. Lane, Int. J. Pharm., 2016, 514, 52–57 CrossRef CAS PubMed.
- J. Peng, S. Xie, K. Huang, P. Ran, J. Wei, Z. Zhang and X. Li, J. Mater. Chem. B, 2022, 10, 4189–4202 RSC.
- A. C. Hortelão, T. Patiño, A. Perez-Jiménez, À. Blanco and S. Sánchez, Adv. Funct. Mater., 2018, 28, 1705086 CrossRef.
- A. Llopis-Lorente, A. Garciá-Fernández, N. Murillo-Cremaes, A. C. Hortelaõ, T. Patinõ, R. Villalonga, F. Sancenón, R. Martínez-Máñez and S. Sánchez, ACS Nano, 2019, 13, 12171–12183 CrossRef CAS PubMed.
- A. C. Hortelao, R. Carrascosa, N. Murillo-Cremaes, T. Patino and S. Sánchez, ACS Nano, 2019, 13, 429–439 CrossRef CAS PubMed.
- M. A. Ramos-Docampo, M. Fernández-Medina, E. Taipaleenmäki, O. Hovorka, V. Salgueirinõ and B. Städler, ACS Nano, 2019, 13, 12192–12205 CrossRef CAS PubMed.
- B. E. F. De Ávila, P. Angsantikul, J. Li, M. Angel Lopez-Ramirez, D. E. Ramírez-Herrera, S. Thamphiwatana, C. Chen, J. Delezuk, R. Samakapiruk, V. Ramez, L. Zhang and J. Wang, Nat. Commun., 2017, 8, 272–280 CrossRef PubMed.
- F. Zhang, J. Zhuang, Z. Li, H. Gong, B. E. F. de Ávila, Y. Duan, Q. Zhang, J. Zhou, L. Yin, E. Karshalev, W. Gao, V. Nizet, R. H. Fang, L. Zhang and J. Wang, Nat. Mater., 2022, 21, 1324–1332 CrossRef CAS PubMed.
- Z. Zhang, D. Zhang, B. Qiu, W. Cao, Y. Liu, Q. Liu and X. Li, Nanoscale, 2021, 13, 6545–6557 RSC.
- H. Choi, S. H. Cho and S. K. Hahn, ACS Nano, 2020, 14, 6683–6692 CrossRef CAS PubMed.
- B. Yigit, Y. Alapan and M. Sitti, Adv. Sci., 2019, 6, 1801837 CrossRef PubMed.
- H. Xie, M. Sun, X. Fan, Z. Lin, W. Chen, L. Wang, L. Dong and Q. He, Sci. Robot., 2019, 4, 8006 CrossRef PubMed.
- M. E. Leunissen, H. R. Vutukuri and A. Van Blaaderen, Adv. Mater., 2009, 21, 3116–3120 CrossRef CAS.
- J. Yan, M. Han, J. Zhang, C. Xu, E. Luijten and S. Granick, Nat. Mater., 2016, 15, 1095–1099 CrossRef CAS PubMed.
- T. Xu, F. Soto, W. Gao, R. Dong, V. Garcia-Gradilla, E. Magaña, X. Zhang and J. Wang, J. Am. Chem. Soc., 2015, 137, 2163–2166 CrossRef CAS PubMed.
- Z. Li, H. Zhang, D. Wang, C. Gao, M. Sun, Z. Wu and Q. He, Angew. Chem., Int. Ed., 2020, 59, 19884–19888 CrossRef CAS PubMed.
- S. Tang, F. Zhang, J. Zhao, W. Talaat, F. Soto, E. Karshalev, C. Chen, Z. Hu, X. Lu, J. Li, Z. Lin, H. Dong, X. Zhang, A. Nourhani and J. Wang, Adv. Funct. Mater., 2019, 29, 1809003 CrossRef.
- A. Aubret, M. Youssef, S. Sacanna and J. Palacci, Nat. Phys., 2018, 14, 1114–1118 Search PubMed.
- W. Duan, R. Liu and A. Sen, J. Am. Chem. Soc., 2013, 135, 1280–1283 CrossRef CAS PubMed.
- J. J. Keya, R. Suzuki, A. M. R. Kabir, D. Inoue, H. Asanuma, K. Sada, H. Hess, A. Kuzuya and A. Kakugo, Nat. Commun., 2018, 9, 453–460 CrossRef PubMed.
- A. C. Hortelao, C. Simó, M. Guix, S. Guallar-Garrido, E. Julián, D. Vilela, L. Rejc, P. Ramos-Cabrer, U. Cossío, V. Gómez-Vallejo, T. Patiño, J. Llop and S. Sánchez, Sci. Robot., 2021, 6, 2823 CrossRef PubMed.
- J. Zheng, J. Chen, Y. Jin, Y. Wen, Y. Mu, C. Wu, Y. Wang, P. Tong, Z. Li, X. Hou and J. Tang, Nature, 2023, 617, 499–506 CrossRef CAS PubMed.
- B. Dai, J. Wang, Z. Xiong, X. Zhan, W. Dai, C. C. Li, S. P. Feng and J. Tang, Nat. Nanotechnol., 2016, 11, 1087–1092 CrossRef CAS PubMed.
- C. Wu, J. Dai, X. Li, L. Gao, J. Wang, J. Liu, J. Zheng, X. Zhan, J. Chen, X. Cheng, M. Yang and J. Tang, Nat. Nanotechnol., 2021, 16, 288–295 CrossRef CAS PubMed.
- M. Ibele, T. E. Mallouk and A. Sen, Angew. Chem., Int. Ed., 2009, 121, 3358–3362 CrossRef.
- D. P. Singh, U. Choudhury, P. Fischer and A. G. Mark, Adv. Mater., 2017, 29, 1701328 CrossRef PubMed.
- S. Jeremie Palacci, A. Preska Steinberg, D. J. Pine and P. M. Chaikin, Science, 2013, 339, 933–936 CrossRef PubMed.
- D. Kagan, S. Balasubramanian and J. Wang, Angew. Chem., Int. Ed., 2011, 123, 523–526 CrossRef.
- M. Akter, J. J. Keya, K. Kayano, A. M. R. Kabir, D. Inoue, H. Hess, K. Sada, A. Kuzuya, H. Asanuma and A. Kakugo, Sci. Robot., 2022, 7, 0677 Search PubMed.
- S. Palagi and P. Fischer, Nat. Rev. Mater., 2018, 3, 113–124 CrossRef CAS.
- J. C. Fraire, M. Guix, A. C. Hortelao, N. Ruiz-González, A. C. Bakenecker, P. Ramezani, C. Hinnekens, F. Sauvage, S. C. De Smedt, K. Braeckmans and S. Sánchez, ACS Nano, 2023, 17, 7180–7193 CrossRef CAS PubMed.
- L. Li, Z. Yu, J. Liu, M. Yang, G. Shi, Z. Feng, W. Luo, H. Ma, J. Guan and F. Mou, Nano-Micro Lett., 2023, 15, 141–159 CrossRef PubMed.
- J. Liu, L. Li, C. Cao, Z. Feng, Y. Liu, H. Ma, W. Luo, J. Guan and F. Mou, ACS Nano, 2023, 17, 16731–16742 CrossRef CAS PubMed.
- D. Jin, Q. Wang, K. F. Chan, N. Xia, H. Yang, Q. Wang, S. Chun, H. Yu and L. Zhang, Sci. Adv., 2023, 9, 9278 CrossRef PubMed.
- A. Servant, F. Qiu, M. Mazza, K. Kostarelos and B. J. Nelson, Adv. Mater., 2015, 27, 2981–2988 CrossRef CAS PubMed.
- J. Yu, D. Jin, K. F. Chan, Q. Wang, K. Yuan and L. Zhang, Nat. Commun., 2019, 10, 5631–5642 CrossRef CAS PubMed.
- Z. Wu, L. Li, Y. Yang, P. Hu, Y. Li, S.-Y. Yang, L. V. Wang and W. Gao, Sci. Robot., 2019, 4, 0613 Search PubMed.
- C. Simó, M. Serra-Casablancas, A. Hortelao, V. Di Carlo, S. Guallar-Garrido, S. Plaza-Garcia, P. Ramos-Cabrer, B. Yagüe, L. Aguado, L. Bardía, S. Tosi, A. Martín, T. Patiño, E. Julián, J. Colombelli, J. Llop and S. Sánchez, Nat. Nanotechnol., 2024 DOI:10.1038/s41565-023-01577-y.
- X. Xia, Y. Li, X. Xiao, Z. Zhang, C. Mao, T. Li and M. Wan, Small, 2023, 2306191 CrossRef PubMed.
- B. Markus, G. C. C. K. Andreas, K. Arkadij, L. Stefan, O. Gustav, S. Elina and S. Radka, ACS Catal., 2023, 13, 14454–14469 CrossRef CAS PubMed.
- S. Mazurenko, Z. Prokop and J. Damborsky, ACS Catal., 2020, 10, 1210–1223 CrossRef CAS.
- P. W. Lee, S. A. Isarov, J. D. Wallat, S. K. Molugu, S. Shukla, J. E. P. Sun, J. Zhang, Y. Zheng, M. Lucius Dougherty, D. Konkolewicz, P. L. Stewart, N. F. Steinmetz, M. J. A. Hore and J. K. Pokorski, J. Am. Chem. Soc., 2017, 139, 3312–3315 CrossRef CAS PubMed.
- K. E. Griswold and C. Bailey-Kellogg, Curr. Opin. Struct. Biol., 2016, 39, 79–88 CrossRef CAS PubMed.
|
This journal is © The Royal Society of Chemistry 2024 |
Click here to see how this site uses Cookies. View our privacy policy here.