DOI:
10.1039/D0RA09061A
(Paper)
RSC Adv., 2020,
10, 44352-44360
Investigation of the target-site resistance of EPSP synthase mutants P106T and T102I/P106S against glyphosate†
Received
23rd October 2020
, Accepted 4th December 2020
First published on 16th December 2020
Abstract
The shikimate pathway enzyme 5-enolpyruvyl shikimate-3-phosphate synthase (EPSPS) catalyzes the reaction involved in the production of amino acids essential for plant growth and survival. Thus, EPSPS is the main target of various herbicides, including glyphosate, a broad-spectrum herbicide that acts as a competitive inhibitor of phosphoenolpyruvate (PEP), which is the natural substrate of EPSPS. However, punctual mutations in the EPSPS gene have led to glyphosate resistance in some plants. Here, we investigated the mechanism of EPSPS resistance to glyphosate in mutants of two weed species, Conyza sumatrensis (mutant, P106T) and Eleusine indica (mutant, T102I/P106S), both of which have an economic impact on industrial crops. Molecular dynamics (MD) simulations and binding free energy calculations revealed the influence of the mutations on the affinity of glyphosate in the PEP-binding site. The amino acid residues of the EPSPS protein in both species involved in glyphosate resistance were elucidated as well as other residues that could be useful for protein engineering. In addition, during MD simulations, we identified conformational changes in glyphosate when complexed with resistant EPSPS, related to loss of herbicide activity and binding affinity. Our computational findings are consistent with previous experimental results and clarify the inhibitory activity of glyphosate as well as the structural target-site resistance of EPSPS against glyphosate.
Introduction
Glyphosate (N-(phosphonomethyl)glycine) is the most relevant and widely used broad-spectrum organophosphate herbicide in agriculture owing to its low cost and high efficiency.1,2 Glyphosate inhibits enolpyruvylshikimate-3-phosphate synthase (EPSPS), a transferase family enzyme that converts phosphoenolpyruvate (PEP) and shikimate-3-phosphate (S3P) to 5-enolpyruvyl shikimate-3-phosphate (EPSP) in the penultimate step of the shikimate pathway leading to the biosynthesis of aromatic amino acids.3–5 Structural studies indicate that glyphosate acts as a competitive inhibitor of PEP, mimicking an intermediate state of the EPSPS–substrate complex, thus inhibiting enzyme catalysis.6
The application of glyphosate in a wide range of industrial crops has led to the emergence of new resistant weeds worldwide.7 There are two known main mechanisms of EPSPS resistance to glyphosate: (1) target-site resistance (TSR) and (2) non-target site resistance (NTSR).8,9 In general, NTSR in weeds can be conferred as a result of the alteration of one or more physiological processes, including herbicide absorption, translocation, sequestration, and metabolism.10 The TSR mechanism is due to structural changes in the target protein, leading to a reduction in binding affinity of the ligand to the active site, thus decreasing herbicide interaction. Different EPSPS mutations have been evaluated and reported to confer resistance to glyphosate in weeds,11–18 including the double substitution Pro106Leu and Thr102Ile observed in several weed species.8,19–22 Understanding the EPSPS TSR mechanisms in mutant species could aid in the development of new commercial herbicides with less toxicity and greater efficiency.
Genetically engineered crops use EPSPS variants with high catalytic efficiency and tolerance to glyphosate, which confer an advantage over susceptible weed species.20,23 The selection of new mutations leading to alterations in the EPSPS structure could aid in the development of glyphosate-resistant plants to improve agricultural production.24–26 Conyza sumatrensis (buva) is a weed species in the botanical class Magnoliopsida. Conyza sumatrensis and C. canadensis are the most widespread species in the world and are considered the main weeds in soybean crops. The widely known resistance of C. sumatrensis EPSPS (CsEPSPS) is due to the P106T mutation.27 Eleusine indica (chicken-grass) is a weed species that widely occurs in different parts of the world. Glyphosate resistance in E. indica EPSPS (EiEPSPS) is due to the presence of the double mutation T102I/P106S (named TIPS) that recreates the commercial EPSPS structure in transgenic crops that are tolerant to first-generation glyphosate.20,28 The double mutation confers a high glyphosate resistance in E. indica mutants, approximately 180-fold greater than that of the wild-type.20
Certain environmental factors, such as compacted soil, low fertility, and high acidity, confer a competitive advantage for the development of weed species in some commercial crops. Additionally, the appearance of glyphosate resistance has caused negative economic impacts worldwide.29 Thus, computational methods have been applied to investigate the structural impact of mutations on different enzymes to analyze the stability and affinity of ligand binding, as well as possible conformational changes at the catalytic pocket that lead to impairment of enzymatic activity.30–34 Recently, we used the free energy surface and quantum mechanics/molecular mechanics (QM/MM) approach to study the catalytic mechanism of EPSPS and the individual roles of catalytic residues Asp313 and Glu341.5 In the present study, we applied different computational methods, molecular dynamics (MD) simulations and binding free energy calculations, to explore the molecular interactions involved with the site-directed mutations in the EPSPS structure of C. sumatrensis (mutant, P106T) and E. indica (mutant, T102I/P106S). Our computational results clarify the implications of these mutations on the binding affinity of glyphosate to resistant and sensitive variants of EPSPS and could further aid in the development of genetically engineered glyphosate-tolerant crop species.
Materials and methods
Structural predictions and validations of wild-type and mutant EPSPS structures
The wild-type structures of EPSPS were obtained by homology modeling using the SWISS-MODEL server35 and the crystallographic structure of Vibrio cholera EPSPS (PDB ID: 3NVS, X-ray; resolution: 1.02 Å). The resistant EPSPS models were obtained using the UCSF Chimera program36 and rotamers function. Finally, the models were validated using energy and stereochemical parameters. The energy profiles were analyzed by QMEAN,37 and the stereochemical quality was evaluated by a Ramachandran plot in the PROCHECK program,38 both available in the SWISS-MODEL server.39
Molecular dynamics simulations of EPSPS–glyphosate complexes
To analyze the variant structures of EPSPS (mutant and wild-type) complexed with the substrate shikimate-3-phosphate (S3P) and the inhibitor (glyphosate), we performed 100 ns of MD simulations in the Amber18 package. The coordinates of glyphosate and shikimate-3-phosphate were obtained from the homology model based on the crystallographic structure (PDB ID: 3NVS). First, the protonation state of the ionizable residues was analyzed by pKa calculations using the PDB2PQR server.40 Second, the ligand charges were calculated using the restrained electrostatic potentials (RESP) approach with the Hartree–Fock method and the 6-31G* basis set in the Gaussian09 program.41 Third, the Leap module of Amber18 was used to build the receptor–ligand complex parameters where the ff14SB force field42 describes the protein atoms, and the general amber force field43 treats the ligand atoms. Finally, these complexes were solvated in a cubic water box using the TIP3P explicit solvation model44 with a radius of 12.0 Å between the box and the protein surface. The counterion Na+ was added to neutralize the protein–ligand systems. Before performing the MD simulation, each protein system was minimized to reduce the overall energy using the steepest-descent and conjugate gradient algorithms.45 Minimization was performed in four steps: the first step corresponded to the minimization of the solvation waters and counterions of the system, the second minimization corresponded to the hydrogen atoms of the protein, in the third step all hydrogens and water molecules were minimized and finally, the whole system.
After minimization, heating of the systems was started at 10 K and gradually increased to 300 K over 100 ps with constant volume constraints. We carried out 200 ps of density equilibration with weak restraints on the protein–ligand complexes followed by 700 ps of constant pressure equilibration at 300 K. A Langevin thermostat was used to maintain the temperature of the system at 300 K. The SHAKE algorithm46 was used to maintain all of the H-bonds at their equilibrium distances, which allowed the use of an integration time step of 2 fs. The MD simulation was performed with 100 ns for each analyzed system.
Binding free energy calculations
The trajectories of each MD simulation were used to calculate the binding free energy of EPSPS complexed with glyphosate. We performed the molecular mechanics generalized Born surface area continuum solvation (MM/GBSA)47 method available in the Amber18 package. Using the CPPTRAJ program implemented in Amber18, the non-complexed ions and waters were removed before performing the binding free energy calculations. For both mutant and wild-type complexes, 1000 trajectory snapshots were used to compute the binding free energy values. The free energy decomposition per residue was calculated as a function of the MM/GBSA method.
Results and discussion
We used a computational approach to investigate the effects of well-known mutations on the EPSPS structure of two of the most widespread weed species with resistance to glyphosate, C. sumatrensis and E. indica (Fig. 1).
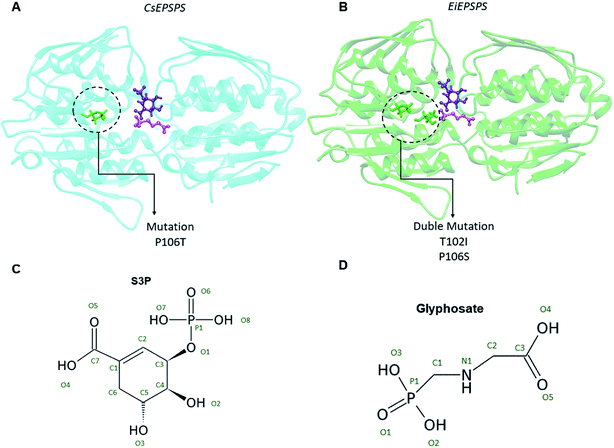 |
| Fig. 1 Location of analyzed mutations in the structures of Conyza sumatrensis 5-enolpyruvyl shikimate-3-phosphate synthase (CsEPSPS mutant, P106T, panel (A)) and Eleusine indica EPSPS (EiEPSPS double mutant, T102I/P106S, panel (B)). In the active site are the substrate shikimate-3-phosphate identified in purple (S3P) and the inhibitor glyphosate in pink. The 2D structures of S3P and glyphosate are shown in panels (C) and (D), respectively. | |
Firstly, we analyzed the root-mean-square deviation (RMSD) plot of 100 ns MD simulations. EiEPSPS structures showed that the native, sensitive variant maintained its conformational stability over the MD simulation when compared with that of the resistant form (Fig. 2). The native variant had a deviation of approximately 2.0 Å from the initial structure, whereas the resistant variant showed RMSD values slightly greater than 2.0 Å. Both the sensitive and resistant structure of CsEPSPS showed similar deviations in RMSD values, remaining stable after 60 ns of the MD trajectory (Fig. 2).
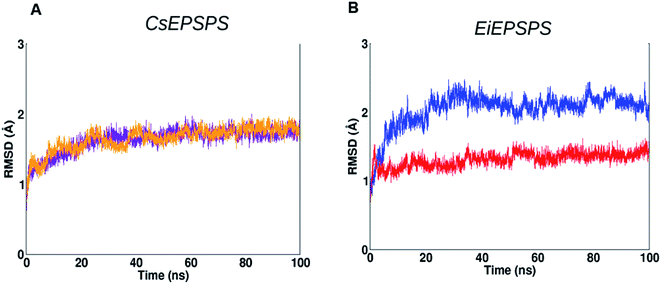 |
| Fig. 2 Root-mean-square deviation (RMSD) plots of Conyza sumatrensis 5-enolpyruvyl shikimate-3-phosphate synthase (CsEPSPS) and Eleusine indica EPSPS (EiEPSPS) variants complexed with glyphosate and shikimate-3-phosphate (S3P) obtained over 100 ns of molecular dynamics simulation. (A) CsEPSPS sensitive (orange) and resistant (purple) structures. (B) EiEPSPs sensitive (red) and resistant (blue) structures. The backbone atoms C, N, and O were used in this analysis. | |
Glyphosate complexed with resistant EPSPS variants undergoes conformational changes during the MD simulations exhibiting a condensed form
In the wild-type EPSPS of E. coli and S. pneumoniae glyphosate exists in an extended conformation.6,48 In contrast, in Agrobacterium sp. strain CP4 EPSPS glyphosate adopts a shortened conformation, which is achieved through rotation about the N–C bond adjacent to the glyphosate carboxyl group.25 Based on a previous study,25 we assumed that only the extended conformation appears to be inhibitory. Thus, we analyzed the average dihedral angle of glyphosate atoms (C1, N1, C2, and C3) acquired during the MD simulations. The reference values found in the X-ray structure showed that the angle between these atoms of glyphosate in the active form (extended conformation) was 179.71° (PDB: 2GGD);25 however, in the condensed form (inactive conformation), the angle was 56.06° (PDB: 2GGA), which does not inhibit the EPSPS activity.25
The dihedral angles obtained during the MD simulation showed that in the sensitive CsEPSPS structure, glyphosate presented an average value of 172°, whereas the resistant CsEPSPS had an average value of 37.29° (Fig. 3). Similar dihedral angle values were found for glyphosate complexed with the EiEPSPS complex.
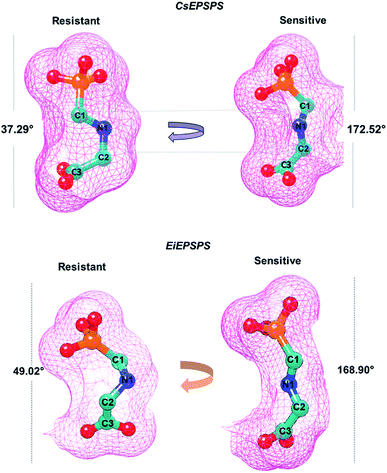 |
| Fig. 3 Average values of the dihedral angles assumed by glyphosate when complexed with resistant and sensitive Conyza sumatrensis 5-enolpyruvyl shikimate-3-phosphate synthase (CsEPSPS) and Eleusine indica EPSPS (EiEPSPS) structures. The dihedral angle values were obtained over 100 ns of molecular dynamics simulation for sensitive and resistant variants of both species. | |
The analysis of the dihedral angle values adopted by glyphosate enabled us to identify similarities with the extended conformation (active) when complexed with sensitive variants. In general, we verified a satisfactory correlation between the dihedral angles obtained from our computational analyses with those measured from the crystallographic structures of sensitive and resistant EPSPS variants.25
We noticed an increase in the average interatomic distances of the main H-bond interactions formed between glyphosate and the EPSPS structures of the wild (sensitive) and mutant (resistant) variants (Tables 1 and 2).
Table 1 Average distances of the intermolecular interactions formed between binding site residues of wild-type (sensitive) and mutant (resistant) Eleusine indica 5-enolpyruvyl shikimate-3-phosphate synthase (EiEPSPS) structures and glyphosate
Wild-type residue (atoms) |
Glyphosate atom |
Interatomic distance (Å) |
Mutant residue (atom) |
Glyphosate atom |
Interatomic distance (Å) |
Arg105 (NH1) |
O2 |
3.17 ± 0.10 |
Arg105 (NH1) |
O3 |
3.63 ± 0.52 |
Arg105 (NH2) |
O2 |
2.54 ± 0.14 |
Arg105 (NH2) |
O1 |
3.06 ± 0.23 |
Arg131 (NH1) |
O3 |
2.76 ± 0.11 |
Arg131 (NH1) |
O2 |
3.80 ± 0.55 |
Arg131 (NH2) |
O2 |
2.71 ± 0.08 |
Arg131 (NH2) |
O1 |
4.04 ± 0.78 |
Arg404 (NH2) |
O4 |
2.50 ± 0.10 |
Arg404 (NH2) |
O5 |
2.85 ± 0.15 |
Arg362 (NH2) |
O5 |
2.53 ± 0.12 |
Arg362 (NH2) |
O5 |
2.77 ± 0.10 |
Thr102 (NH1) |
O1 |
2.96 ± 0.31 |
Ile102 (NH1) |
O1 |
5.77 ± 0.74 |
Table 2 Average distances of the intermolecular interactions formed between binding site residues of wild-type (sensitive) and mutant (resistant) Conyza sumatrensis 5-enolpyruvyl shikimate-3-phosphate synthase (CsEPSPS) structures and glyphosate
Wild-type residue (atom) |
Glyphosate atom |
Interatomic distance (Å) |
Mutant residue (atom) |
Glyphosate atom |
Interatomic distance (Å) |
Gln180 (NE2) |
O4 |
2.86 ± 0.15 |
Gln180 (NE2) |
O4 |
3.20 ± 0.64 |
Arg362 (NH1) |
O5 |
3.04 ± 0.20 |
Arg362 (NH1) |
N1 |
4.56 ± 0.42 |
Arg404 (NH2) |
O4 |
2.80 ± 0.12 |
Arg404 (NH2) |
NH2 |
3.47 ± 0.59 |
Glu358 (OE2) |
N1 |
2.80 ± 0.09 |
Glu358 (OE2) |
N1 |
2.99 ± 0.41 |
Lys22 (NZ) |
O1 |
2.78 ± 0.10 |
Lys22 (NZ) |
O3 |
3.01 ± 0.54 |
Arg131 (NH1) |
O3 |
2.77 ± 0.13 |
Arg131 (NH1) |
O2 |
3.26 ± 0.49 |
Lys428 (NZ) |
O3 |
2.83 ± 0.26 |
Lys428 (NZ) |
— |
— |
The Thr102Ile mutation in the EiEPSPS structure led to the loss of some relevant interaction of glyphosate in this residue position. The distance between O1 of glyphosate and the main chain NH1 atom at position 102 increased from 2.96 to 5.77 Å when the mutation was present in the residue site (Table 1). Moreover, the side chain of Thr102 formed a H-bond between OH and O1 of glyphosate that did not exist in the mutant structure. Regarding the CsEPSPS structures, it is important to highlight that despite Pro106 not directly interacting with glyphosate, the presence of the P106T mutation induced structural changes in the CsEPSPS site. Thr106 (Fig. 4, panels A and B) in the mutant CsEPSPS interacted with Thr102, thereby reducing its interaction with glyphosate.
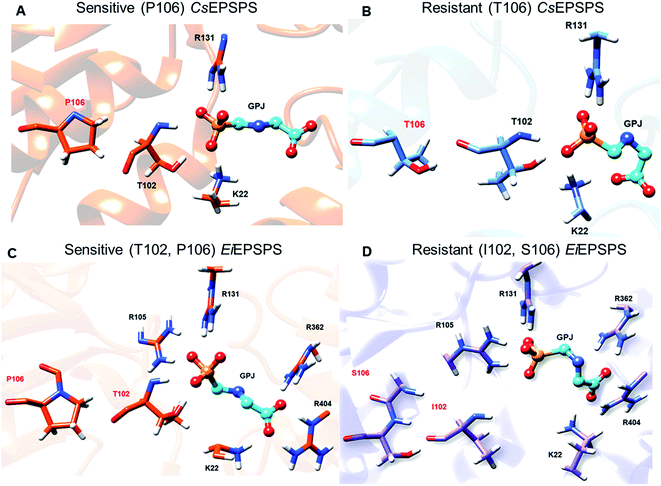 |
| Fig. 4 Close-up view of the glyphosate (GPJ) binding site of (A and C) wild-type and (B and D) mutants Conyza sumatrensis 5-enolpyruvyl shikimate-3-phosphate synthase (CsEPSPS) and Eleusine indica EPSPS (EiEPSPS). The mutated residues are highlighted in red. | |
We also observed that glyphosate changed the interaction with Lys22 due to the condensed conformation acquired during the MD simulation. Lys22 of the sensitive CsEPSPS structure interacted with the phosphate group of glyphosate, whereas the same residue formed an additional interaction with the carboxylate group of glyphosate in the resistant CsEPSPS. In contrast, we observed that in the sensitive CsEPSPS, Pro106 interacted only with Ala110 and did not influence the interaction of glyphosate with Thr102, which could be attributed to the absence of H-bond interactions between Pro106 and Thr102 (Table 1). In general, the presence of a single (P106T) or double (T102I/P106S) mutation in the resistant EPSPS causes glyphosate to assume a condensed conformation, which may be related to its lower stability throughout the simulation.
Thr102Ile substitution has a great impact on the stability of the binding pocket of EiEPSPS, and Arg131 and Arg362 contribute highly to glyphosate affinity in both sensitive enzymes
In the present study, we calculated the binding free energy of the EPSPS variants complexed with glyphosate using MM/GBSA method (Table 3). Thereafter, we compared the half-maximal inhibitory concentration (IC50) values obtained from the enzymatic activity inhibition assays.20,27,49 The energy values obtained by computational methods for glyphosate complexed with EPSPS structures showed a similar trend to the values obtained by the inhibition assays. Owing to the conformational stability of the structure verified in the last 10 ns of the MD simulation, we selected this interval of the trajectory to perform the binding free energy (ΔGbind) calculations (Table 3).
Table 3 Binding free energy (kcal mol−1) values obtained using molecular mechanics generalized Born surface area (MM/GBSA) method for both Conyza sumatrensis 5-enolpyruvyl shikimate-3-phosphate synthase (CsEPSPS) and Eleusine indica EPSPS (EiEPSPS) structures complexed with glyphosate and compared with the experimental half-maximal inhibitory concentration IC50.20,27 Electrostatic and van der Waals components (kcal mol−1) of the calculated binding free energies calculated for CsEPSPS and EiEPSPS structures complexed with glyphosate are shown in Table S1
Structural variants |
CsEPSPS |
EiEPSPS |
ΔGGBSA |
IC50 (μM) |
ΔGGBSA |
IC50 (μM) |
Sensitive |
−95.80 ± 0.47 |
13.55 |
−126.04 ± 0.47 |
20.0 |
Resistant |
−75.64 ± 0.48 |
106.11 |
−75.67 ± 0.56 |
52 938 |
The analysis of pairwise decomposition of residue interaction energies in the wild-type EiEPSPS structure demonstrated that Thr102 as well as some residues of the binding pocket (Arg105, Arg131, Lys358, Arg362, and Arg404) showed a relevant energetic contribution to the complex affinity (Fig. 5). However, in the double-mutant EiEPSPS structure, Ile102 and Ser106 interacted with each other. Thus, there was no H-bond interaction between the residue at position 102 and the phosphate group of glyphosate, as observed in wild-type EiEPSPS (Fig. 4).
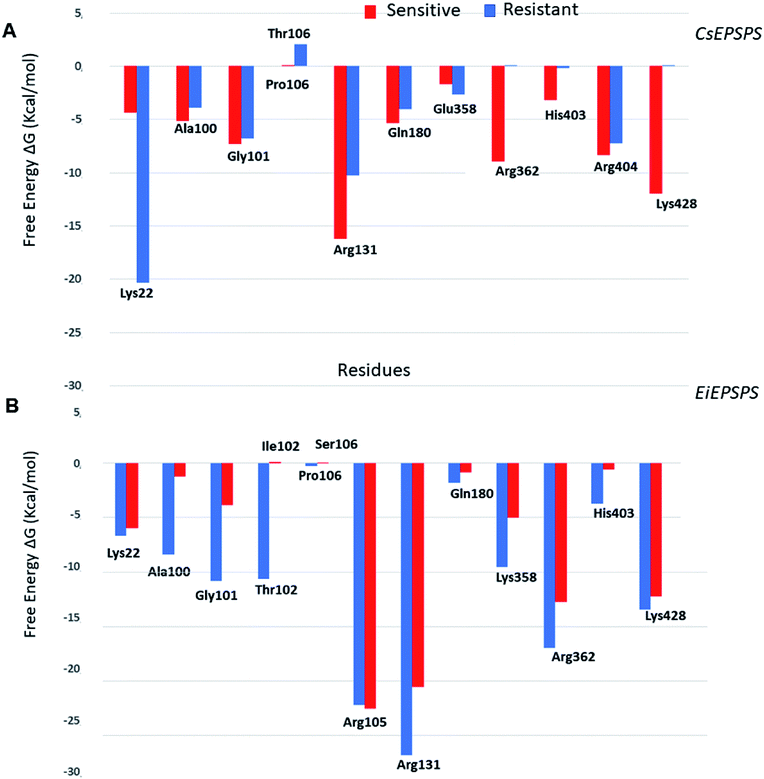 |
| Fig. 5 Pairwise decomposition of residue interactions energies of (A) Conyza sumatrensis 5-enolpyruvyl shikimate-3-phosphate synthase (CsEPSPS) and (B) Eleusine indica EPSPS (EiEPSPS) mutant (resistant, blue) and wild-type structures (sensitive, red). | |
The Thr102Ile substitution had a great impact on the affinity of the binding pocket due to the differences in the chemical properties of the mutated residues. Threonine is a polar residue, whereas isoleucine is a hydrophobic (nonpolar), branched-chain, and aliphatic residue. Analysis of the binding free energy decomposition plot (Fig. 5) revealed that Thr102 in the sensitive form contributed energetically to the stability of the complex with a value of −10.61 kcal mol−1, whereas in the resistant structure, Ile102 did not influence the affinity of the complex. In contrast, Gly101 showed a lower contribution of −3.85 kcal mol−1. These results agree with previous evidence of residue mutation in the binding site of the EiEPSPS structure.50 Jingbo Li et al. (2018), using a computational approach, proposed that a mutation in EiEPSPS (Thr102Ser) resulted in development of resistance against glyphosate due to energetic changes in the PEP binding site. Additionally, our results showed that these energetic changes included the contributions of Ala100, Gly101, and Thr/Ile102 in the EiEPSPS active site.
The binding free energy values obtained indicated that the resistant CsEPSPS variant (mutant) showed a weaker affinity than the sensitive variant to glyphosate. These results are consistent with experimental data showing that sensitive CsEPSPS is inhibited by 13.5 μM glyphosate, whereas the resistant form is inhibited by a much higher concentration, 106 μM, which is much higher than the recommended glyphosate treatment dose.27 We also observed a trend in theoretical free energy values when compared with the experimental inhibitory activity. The energy values obtained from the MM/GBSA method showed a ratio of 1.26 for resistant CsEPSPS in the presence of the mutation. The residues Gly101, Arg131, Arg362, Arg404, and Lys428 of the sensitive CsEPSPS (Fig. 5) interact with glyphosate, contributing to the greater binding affinity.
The loss of interaction between Lys428 and glyphosate may explain its lack of energy contribution in the resistant CsEPSPS structure when compared with that of the sensitive form in which Lys428 contributed to the binding affinity with the energy of −11.98 kcal mol−1. Regarding the resistant CsEPSPS structure, we noted that residues Lys22, Ala100, Gly101, Arg131, Gln180, and Arg404 contributed to glyphosate affinity at the binding site. In addition, it is important to highlight that Lys22 showed a greater energy contribution (−20.33 kcal mol−1). This high energetic contribution occurred due to the loss of an interaction between glyphosate and Arg362, which led to reduced interaction with Arg404 throughout the MD simulation. This interaction impairment induced glyphosate to assume the condensed conformation, thus interacting more strongly with Lys22. Snapshots of the MD trajectory when glyphosate (GPJ) loses its interaction with Arg362 are shown in Fig. 6.
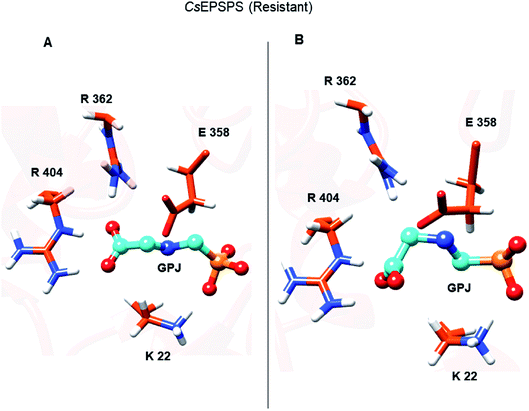 |
| Fig. 6 (A) Glyphosate (GPJ) complexed with EPSPS binding site interacting with residue Arg362 during the first 30 ns of molecular dynamics simulation. (B) GPJ assuming the condensed conformation in the last 70 ns of the molecular dynamics trajectory. | |
Although the P106T mutation is located on the superficies of the cavity that forms the EPSPS binding site, this substitution promotes a stable surface that accommodates the other residues involved in the interaction of PEP, thus allowing substrate-binding.51 Our computational results demonstrate that the target-site resistance mechanism of CsEPSPS and EiEPSPS is related to the loss of binding affinity of glyphosate caused by the residue mutations, which leads to the loss of relevant interatomic interactions.
Conclusion
Computational analyses in this study demonstrated that sensitive EPSPS structures complexed with glyphosate correspond with the most energy-stable complexes, and the resistant EPSPS showed less affinity for the herbicide. Additionally, we demonstrated that the glyphosate structure undergoes conformational changes during MD simulations when complexed with resistant EPSPS, showing a condensed form that reduces the binding affinity of glyphosate. Our computational findings are consistent with previous experimental results and clarify the inhibitory activity of glyphosate as well as the target-site resistance of the EPSPS structure against this herbicide. Regarding the molecular mechanism of resistance of the analyzed mutations, we found that the Thr102Ile substitution strongly decreased the affinity of EiEPSPS to glyphosate. Thus, we were able to identify the EPSPS amino acid residues involved in binding glyphosate in the resistant weed species as well as other residues with potential for protein engineering. These findings could aid in the development of new commercial herbicides with less toxicity and greater efficiency.
Conflicts of interest
There are no conflicts to declare.
Acknowledgements
We are grateful to the Santos Dumont Supercomputer from the Laboratório Nacional de Computação Científica (LNCC) for providing the supercomputing facilities and to Pró-Reitoria de Pesquisa e Pós-Graduação (PROPESP/UFPA) for the financial support to improve the quality of this manuscript. E. C. M. F. and K. S. C. are grateful for the scholarship from the Brazilian funding agency, Coordenação de Aperfeiçoamento de Pessoal de Nível Superior (CAPES).
References
- S. O. Duke and S. B. Powles, Pest Manage. Sci., 2008, 64, 319–325 Search PubMed
. - S. O. Duke, Pest Manage. Sci., 2018, 74, 1027–1034 Search PubMed
. - H. Maeda and N. Dudareva, Annu. Rev. Plant Biol., 2012, 63, 73–105 Search PubMed
. - S. O. Duke, J. Agric. Food Chem., 2011, 59, 5835–5841 Search PubMed
. - A. M. dos Santos, A. H. Lima, C. N. Alves and J. Lameira, J. Phys. Chem. B, 2017, 121, 8626–8637 Search PubMed
. - E. Schönbrunn, S. Eschenburg, W. A. Shuttleworth, J. V. Schloss, N. Amrhein, J. N. S. Evans and W. Kabsch, Proc. Natl. Acad. Sci. U. S. A., 2001, 98, 1376–1380 Search PubMed
. - E. Bracamonte, P. T. Fernández-Moreno, F. Barro and R. de Prado, Front. Plant Sci., 2016, 7, 1845 Search PubMed
. - J. Gherekhloo, P. T. Fernández-Moreno, R. Alcántara-De La Cruz, E. Sánchez-González, H. E. Cruz-Hipolito, J. A. Domínguez-Valenzuela and R. De Prado, Sci. Rep., 2017, 7, 6702 Search PubMed
. - J. A. Sikorski and K. J. Gruys, Acc. Chem. Res., 1997, 30, 2–8 Search PubMed
. - M. Jugulam and C. Shyam, Plants, 2019, 8, 417 Search PubMed
. - I. Heap and S. O. Duke, Pest Manage. Sci., 2018, 74, 1040–1049 Search PubMed
. - V. E. Perotti, A. S. Larran, V. E. Palmieri, A. K. Martinatto, C. E. Alvarez, D. Tuesca and H. R. Permingeat, Pest Manage. Sci., 2019, 75, 1242–1251 Search PubMed
. - T. A. Gaines, W. Zhang, D. Wang, B. Bukun, S. T. Chisholm, D. L. Shaner, S. J. Nissen, W. L. Patzoldt, P. J. Tranel, A. S. Culpepper, T. L. Grey, T. M. Webster, W. K. Vencill, R. D. Sammons, J. Jiang, C. Preston, J. E. Leach and P. Westra, Proc. Natl. Acad. Sci. U. S. A., 2010, 107, 1029–1034 Search PubMed
. - M. J. García, C. Palma-Bautista, J. G. Vazquez-Garcia, A. M. Rojano-Delgado, M. D. Osuna, J. Torra and R. De Prado, Sci. Rep., 2020, 10, 17681 Search PubMed
. - S. Nasr Ramzi, M. M. Sohani, R. Shirzadian-Khorramabad, J. Asghari and M. Amininasab, Plant Gene, 2020, 22, 100225 Search PubMed
. - T. C. Ramalho, M. S. Caetano, E. F. F. Da Cunha, T. C. S. Souza and M. V. J. Rocha, J. Biomol. Struct. Dyn., 2009, 27, 195–207 Search PubMed
. - M. Yanniccari, M. E. Gómez-Lobato, C. Istilart, C. Natalucci, D. O. Giménez and A. M. Castro, Front. Ecol. Evol., 2017, 5, 123 Search PubMed
. - R. Alcántara-de la Cruz, A. M. Rojano-Delgado, M. J. Giménez, H. E. Cruz-Hipolito, J. A. Domínguez-Valenzuela, F. Barro and R. De Prado, Front. Plant Sci., 2016, 7, 1742 Search PubMed
. - J. Chen, H. Huang, C. Zhang, S. Wei, Z. Huang, J. Chen and X. Wang, Planta, 2015, 242, 859–868 Search PubMed
. - Q. Yu, A. Jalaludin, H. Han, M. Chen, R. D. Sammons, S. B. Powles, R. Douglas Sammons and S. B. Powles, Plant Physiol., 2015, 167, 1440–1447 Search PubMed
. - R. Alcántara-de la Cruz, P. T. Fernández-Moreno, C. V. Ozuna, A. M. Rojano-Delgado, H. E. Cruz-Hipolito, J. A. Domínguez-Valenzuela, F. Barro and R. de Prado, Front. Plant Sci., 2016, 7, 1492 Search PubMed
. - M. S. Bell, A. G. Hager and P. J. Tranel, Weed Sci., 2013, 61, 460–468 Search PubMed
. - Y. Dong, E. Ng, J. Lu, T. Fenwick, Y. Tao, S. Bertain, M. Sandoval, E. Bermudez, Z. Hou, P. Patten, M. Lassner and D. Siehl, J. Biol. Chem., 2019, 294, 716–725 Search PubMed
. - L. Pollegioni, E. Schonbrunn and D. Siehl, FEBS J., 2011, 278, 2753–2766 Search PubMed
. - T. Funke, H. Han, M. L. Healy-Fried, M. Fischer and E. Schönbrunn, Proc. Natl. Acad. Sci. U. S. A., 2006, 103, 13010–13015 Search PubMed
. - Y. Dong, X. Jin, Q. Tang, X. Zhang, J. Yang, X. Liu, J. Cai, X. Zhang, X. Wang and Z. Wang, Front. Plant Sci., 2017, 8, 885 Search PubMed
. - I. Amaro-Blanco, P. T. Fernández-Moreno, M. D. Osuna-Ruiz, F. Bastida and R. De Prado, Pest Manage. Sci., 2018, 74, 1925–1937 Search PubMed
. - H. Han, M. M. Vila-Aiub, A. Jalaludin, Q. Yu and S. B. Powles, Plant Cell Environ., 2017, 40, 3031–3042 Search PubMed
. - S. Bonny, Environ. Manag., 2016, 57, 31–48 Search PubMed
. - J. Neves Cruz, K. S. da Costa, T. A. A. de Carvalho and N. A. N. de Alencar, J. Biomol. Struct. Dyn., 2020, 38, 1425–1434 Search PubMed
. - P. Kumar, P. Kumari, S. G. Sachan and R. Poddar, Comput. Biol. Chem., 2018, 76, 245–255 Search PubMed
. - E. Fazel-Najafabadi, E. Vahdat Ahar, S. Fattahpour and M. Sedghi, Comput. Biol. Chem., 2015, 59, 48–55 Search PubMed
. - C. H. S. Costa, A. R. S. Oliveira, A. M. dos Santos, K. S. da Costa, A. H. L. e. Lima, C. N. Alves and J. Lameira, J. Biomol. Struct. Dyn., 2019, 37, 4374–4383 Search PubMed
. - M. D. de Oliveira, J. de O. Araújo, J. M. P. Galúcio, K. Santana and A. H. Lima, J. Mol. Graph. Model., 2020, 101, 107735 Search PubMed
. - M. Biasini, S. Bienert, A. Waterhouse, K. Arnold, G. Studer, T. Schmidt, F. Kiefer, T. G. Cassarino, M. Bertoni, L. Bordoli, T. Schwede, F. Keifer, C. Gallo, M. Bertoni, L. Bordoli, S. Torsten, F. Kiefer, T. G. Cassarino, M. Bertoni, L. Bordoli and T. Schwede, Nucleic Acids Res., 2014, 42, W252–W258 Search PubMed
. - E. F. Pettersen, T. D. Goddard, C. C. Huang, G. S. Couch, D. M. Greenblatt, E. C. Meng and T. E. Ferrin, J. Comput. Chem., 2004, 25, 1605–1612 Search PubMed
. - P. Benkert, M. Künzli and T. Schwede, Nucleic Acids Res., 2009, 37, W510–W514 Search PubMed
. - R. A. Laskowski, M. W. MacArthur, D. S. Moss and J. M. Thornton, J. Appl. Crystallogr., 1993, 26, 283–291 Search PubMed
. - F. Kiefer, K. Arnold, M. Künzli, L. Bordoli and T. Schwede, Nucleic Acids Res., 2009, 37(1), D387–D392 Search PubMed
. - T. J. Dolinsky, J. E. Nielsen, J. A. McCammon and N. A. Baker, Nucleic Acids Res., 2004, 32, W665–W667 Search PubMed
. - M. J. e. a. Frisch, A. J. Nathan and A. Scobell, Gaussian, Inc.Wallingford CT, 2009, 2–3
. - J. A. Maier, C. Martinez, K. Kasavajhala, L. Wickstrom, K. E. Hauser and C. Simmerling, J. Chem. Theory Comput., 2015, 11, 3696–3713 Search PubMed
. - J. Wang, R. M. Wolf, J. W. Caldwell, P. A. Kollman and D. A. Case, J. Comput. Chem., 2004, 25, 1157–1174 Search PubMed
. - W. L. Jorgensen, J. Am. Chem. Soc., 1981, 103, 335–340 Search PubMed
. - M. R. Hestenes and E. Stiefel, J. Res. Natl. Bur. Stand., 1952, 49, 409 Search PubMed
. - J.-P. Ryckaert, G. Ciccotti and H. J. C. Berendsen, J. Comput. Phys., 1977, 23, 327–341 Search PubMed
. - T. Hou, J. Wang, Y. Li and W. Wang, J. Chem. Inf. Model., 2011, 51, 69–82 Search PubMed
. - H. Park, J. L. Hilsenbeck, H. J. Kim, W. A. Shuttleworth, Y. H. Park, J. N. Evans and C. Kang, Mol. Microbiol., 2004, 51, 963–971 Search PubMed
. - C. Mao, H. Xie, S. Chen, B. E. Valverde and S. Qiang, Planta, 2016, 243, 321–335 Search PubMed
. - J. Li, Q. Peng, H. Han, A. Nyporko, T. Kulynych, Q. Yu and S. Powles, J. Agric. Food Chem., 2018, 66(30), 7880–7888 Search PubMed
. - L. F. S. M. Timmers, A. M. S. Neto, R. W. Montalvão, L. A. Basso, D. S. Santos and O. Norberto de Souza, J. Mol. Model., 2017, 23, 197 Search PubMed
.
Footnote |
† Electronic supplementary information (ESI) available. See DOI: 10.1039/d0ra09061a |
|
This journal is © The Royal Society of Chemistry 2020 |
Click here to see how this site uses Cookies. View our privacy policy here.