DOI:
10.1039/D2NR06068J
(Minireview)
Nanoscale, 2023,
15, 4236-4249
Pathway of transient electronics towards connected biomedical applications
Received
30th October 2022
, Accepted 8th January 2023
First published on 9th January 2023
Abstract
Transient electronic devices have shown promising applications in hardware security and medical implants with diagnosing therapeutics capabilities since their inception. Control of the device transience allows the device to “dissolve at will” after its functional operation, leading to the development of on-demand transient electronics. This review discusses the recent developments and advantages of triggering strategies (e.g., electrical, thermal, ultrasound, and optical) for controlling the degradation of on-demand transient electronics. We also summarize bioresorbable sensors for medical diagnoses, including representative applications in electrophysiology and neurochemical sensing. Along with the profound advancements in medical diagnosis, the commencement of therapeutic systems such as electrical stimulation and drug delivery for the biomedical or medical implant community has also been discussed. However, implementing a transient electronic system in real healthcare infrastructure is still in its infancy. Many critical challenges still need to be addressed, including strategies to decouple multimodal sensing signals, dissolution selectivity in the presence of multiple stimuli, and a complete sensing–stimulation closed-loop system. Therefore, the review discusses future opportunities in transient decoupling sensors and robust transient devices, which are selective to a particular stimulus and act as hardware-based passwords. Recent advancements in closed-loop controller-enabled electronics have also been analyzed for future opportunities of using data-driven artificial intelligence-powered controllers in fully closed-loop transient systems.
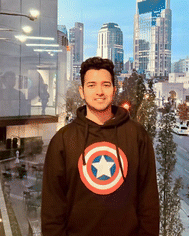 Ankan Dutta | Ankan Dutta is a PhD student under the supervision of Professor Huanyu Cheng at The Pennsylvania State University, University Park. He received his undergraduate degree from Jadavpur University in Mechanical Engineering. He has been awarded SERB Fellowships in Jadavpur University and the Indian Association for the Cultivation of Science. He has also served as a Reviewer for IEEE SMC 2022, IEEE Internet of Things Journal. His current research interests include soft robotics, transient electronics and biomedical devices such as flexible ultrasound arrays. |
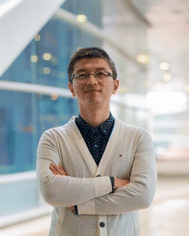 Huanyu Cheng | Prof. Huanyu Cheng earned a PhD degree from Northwestern University in 2015 and a bachelor's degree from Tsinghua University in 2010. He is the James L. Henderson, Jr Memorial Associate Professor of Engineering Science and Mechanics at Penn State University. Dr Cheng has worked on standalone stretchable sensing systems for biomedicine with over 130 peer-reviewed publications, and his work has been recognized through the reception of numerous awards. He also serves as an associate editor for the IEEE Internet of Things Journal, Computers in Biology and Medicine and as a reviewer for over 230 international journals. |
1. Introduction
Bioelectronics are an integrated part of medical implants such as artificial pacemakers. However, surgical interventions are often performed to remove the implants to avoid adverse long-term consequences after the functional operation of the implants. The recent development of medical implants with on-demand transience has largely overcome post-surgical complications. Therefore, transient electronics are widely employed in medical implants for biomedical diagnosis.1–12 Besides sensing and diagnosis, bioresorbable electronics have also been implemented for biomedical therapeutics.13–21 With the need to use advanced transient systems in real-world healthcare infrastructure, control of their operational time becomes essential. Wirelessly triggered transience using an external stimulus such as electrical, optical, or ultrasound has recently been leveraged to dissolve the implant at a controlled rate and prescribed time, thereby controlling the implant's operational time22–28 (Fig. 1a). Moreover, introducing an intelligent closed-loop controller is imperative to elevate the transient system to an advanced intelligent system for minimal clinician intervention in large-scale applications.17,18,29–32 As a first step to address this need, closed-loop systems with bioresorbable sensors and actuators have been recently developed to sense and actuate on demand.33 However, gaps and possible directions in truly data-driven intelligent, robust transient electronic systems are yet to be reported.
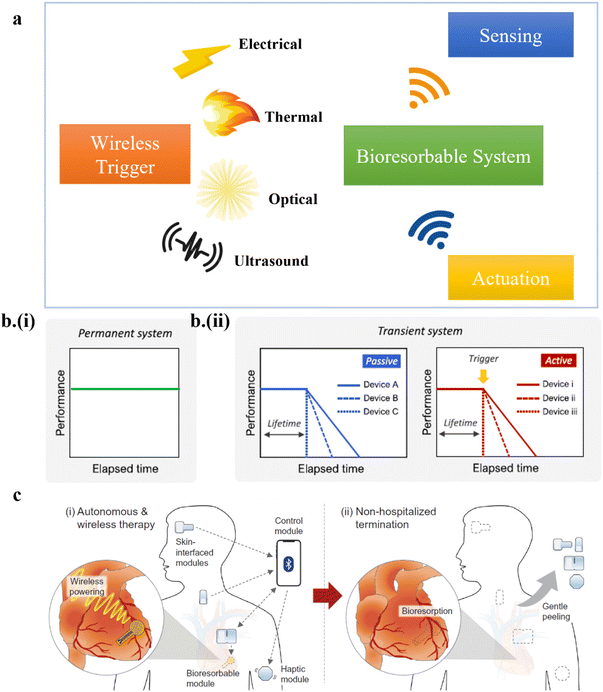 |
| Fig. 1 (a) A schematic showing on-demand transient electronics in biomedical sensing and actuation. (b) Comparison in the strategies between (i) a permanent system and (ii) passive or active transient electronics. This figure has been reproduced from ref. 24 with permission from Elsevier, copyright 2020. (c) Advantages of active transient electronics in a clinical setup. This figure has been reproduced from ref. 33 with permission from The American Association for the Advancement of Science, copyright 2022. | |
Traditional implants are fabricated using non-bioresorbable materials showing near-constant performance until their infinite operational time (Fig. 1b-i), requiring secondary surgery. On the other hand, transient electronics leverages bioresorbable substrates such as poly-lactic-co-glycolic acid (PLGA)34–46 and metals such as magnesium and zinc47–51 fabricated with standard or unconventional techniques (e.g., light-induced zinc mass transfer)52 to enable transience. The material selection, design, and external stimulus mode are crucial to determining its operational time ranging from seconds to months. However, passive transient systems have a destined degradation period, leaving no control over their operational time trajectory. On the contrary, active or on-demand transient systems could use an external triggering stimulus to initiate the onset of degradation22–27,34,35 (Fig. 1b-ii). Control over the onset of transience allows the device to “dissolve at will” after its designed usage in both inpatient and outpatient settings. The new dimension in home monitoring/treatment reduces hospital burden24,30,33 (Fig. 1c).
This review discusses the advancements in different strategies and the mechanisms underlying the dissolution dynamics of the on-demand transient electronics. Along with the advancements in the various diagnostic applications enabled by transient electronics, the review also discusses a few initial steps toward transient therapeutics such as electrical stimulation and drug delivery. By incorporating transient therapeutics with sensing capabilities, transient closed-loop systems and their possible advancement to provide autonomous biomedical therapy from recent studies are also reviewed. Lastly, limitations and challenges have been discussed, which may provide tangible pathways to implement fully robust closed-loop transient electronics in practical healthcare infrastructure.
2. Strategies of wirelessly triggered transient electronics
Degradation of materials commences immediately with the introduction of water or biofluids such as phosphate-buffered saline (PBS) solution into passive transient systems, leading to operational failure.24,34,35,38,39,42,47,53,54 In contrast, materials degrade only in the presence of an external stimulus in active transient systems, enabling control over the operation timeline of the device.14,22–25,27,55,56 The transience onset and rate can be conveniently controlled by the device structure (e.g., thickness) and constituent materials, as well as the degradation mechanism of stimuli-responsive material, to modulate the transient process and operation timeline.53,54 The dissociation of one or more bioresorbable components within the device can initiate the degradation process. On the other hand, the device can also disintegrate due to the complete degradation of the substrate supporting the device components.24 The material dissociation can be triggered by various external stimuli, ranging from etchants and temperature to ultrasound. However, triggering stimuli such as water or solvents/etchants applied physically in contact with the dissociating material are challenging to use in a wirelessly controlled setting. On the other hand, light3 or ultrasound23 can be applied externally and controlled wirelessly to trigger or initiate the dissociation of the material in the surrounding solution. The following subsections focus on the degradation mechanism and strategies exploited to trigger device disintegration wirelessly by external stimuli.
2.1 Design strategy and mechanism of electrically triggered transient electronics
Electrical stimuli initiate fast and controllable triggering events in transient systems. Typically, electrical energy can be provided wirelessly to either generate an electrical spark to release corrosive agents or enable electrochemical reaction-assisted triggering of the device disintegration into the surrounding fluid.24 Though the onset of triggering is controllable, the former does not provide any control over the dynamic releasing process of corrosive agents, resulting in disintegration of the device. In contrast, the disintegration due to electrochemical reaction is controllable as it depends on the generated bias to facilitate chemical kinetics.14,53,54,57 Understanding the transience of silicon is critical to advance transient circuitries by leveraging the existing silicon-based electronics. However, accelerating the dissolution of Si nanomembranes for fast transient Si circuits is a challenge. With the application of a constant current density of 400 μA cm−2 in the galvanostatic mode using a metal–oxide–semiconductor field-effect transistor (MOSFET), lithiation was performed to provide the fast yet controllable on-demand disintegration of Si57 (Fig. 2a-i). Inhomogeneous diffusion of lithium (Li) into Si formed a vein-like LixSi network and increased its volume by ∼280%, which induced microcracks to accelerate the degradation of Si (Fig. 2a-ii and iii).
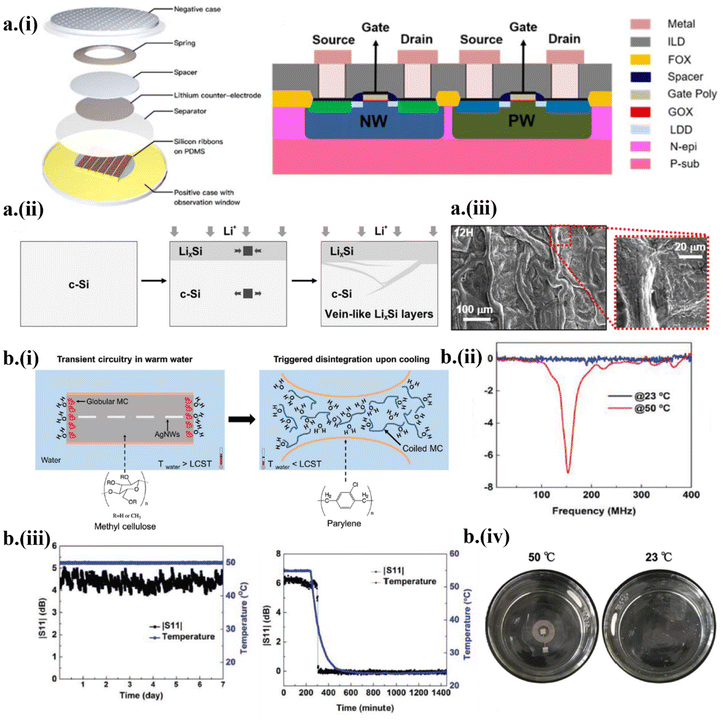 |
| Fig. 2 Strategies of wirelessly triggered on-demand transient electronics by (a) electrical or (b) temperature stimuli. (a) (i) Electrically triggered on-demand transient electronics based on a Si nanomembrane. (ii) The morphological transformation of LixSi layers upon lithiation. (iii) Morphology of the Si side of the MOSFETs after 12 h of lithiation. This figure has been reproduced from ref. 27 with permission from IOP Publishing, Ltd, copyright 2019. (b) Triggered by the temperature change, (i) the methylcellulose substrate dissolves when the solution temperature drops below the polymer's lower critical solution temperature (LCST). (ii and iii) Measured S11 return loss in warm and cold water and (iv) demonstration of transience in cold water. This figure has been reproduced from ref. 55 with permission from John Wiley and Sons, copyright 2019. | |
2.2 Design strategy and mechanism of thermally triggered transient electronics
It is intuitive to utilize water as a solvent to dissolve the substrate and as a reactant to initiate hydrolysis of the device's constitutive materials in water-triggered transient electronics.22,35,55 However, water or other etchants often need to be in contact with the device to initiate hydrolysis and are limited in the application of passive triggering transient electronics. In order to improve wireless controllability, it is imperative to combine external parameters such as the temperature of the water as wirelessly triggered stimuli to control the triggering events. As a representative example, the hydrophilicity of a thermoresponsive polymer such as methylcellulose (MC) with a lower critical solution temperature (LCST) of 50 °C was leveraged in a transient radio frequency (RF) antenna55 (Fig. 2b-i). For temperatures above the LCST, the hydrogen bonding between water molecules and polar groups of the polymer prevents random mixing, decreasing the entropy and thus favoring unspontaneous mixing due to an increase in Gibb's free energy, which induces the hydrophobic nature of MC. At a temperature below the LCST, the MC polymer becomes hydrophilic with the decreased formation enthalpy due to the formation of more hydrogen bonds, thus favoring spontaneous mixing. As a result, the antenna showed excellent mechanical and electrical stability in a warm PBS with a temperature higher than LCST (Fig. 2b-ii) but it degenerated and dissolved when the solution cooled down to be lower than LCST (Fig. 2b-iii), resulting in physical disappearance of the antenna (Fig. 2b-iv).
2.3 Design strategy and mechanism of ultrasonically triggered transient electronics
The rise of transient implantable devices has spurred the development of various transient powering sources. The implants are generally powered by batteries, taking the bulk of the device's weight. In addition, the transient battery is still in its infancy, suffering from the trade-off between the electrochemical performance and transient behavior.58,59 Therefore, wireless powering systems such as ultrasound have become attractive for transient implantable devices.23,60 However, the tissue attenuation often results in drastically reduced power transmitted to the implantable. In a recent demonstration,23 ultrasound-based acoustic energy transmission was harnessed both as wireless powering and a triggering mechanism to power an implantable triboelectric nanogenerator (TENG) (Fig. 3a). The TENG was designed with a poly(3-hydroxybutyrate-co-3-hydroxyvalerate)/polyethylene glycol (PHBV/PEG) composite membrane on top of an Mg electrode layer sandwiched between two PHBV layers (Fig. 3a-i). The implantable TENG showed a stable energy generation of 338.7 mV root mean square (RMS) using a 0.5 W cm−2, 20 kHz ultrasound probe (Fig. 3a-ii). After 60 min of operation, an increased acoustic power density of 3 W cm−2 from the ultrasound probe triggered the onset of the transient process to result in a sudden reduction in the generated voltage (Fig. 3a-iii). Thus, controlling the ultrasound intensity allowed the TENG to power (<1 W cm−2) or initiate degradation (>3 W cm−2) (Fig. 3a-iv).
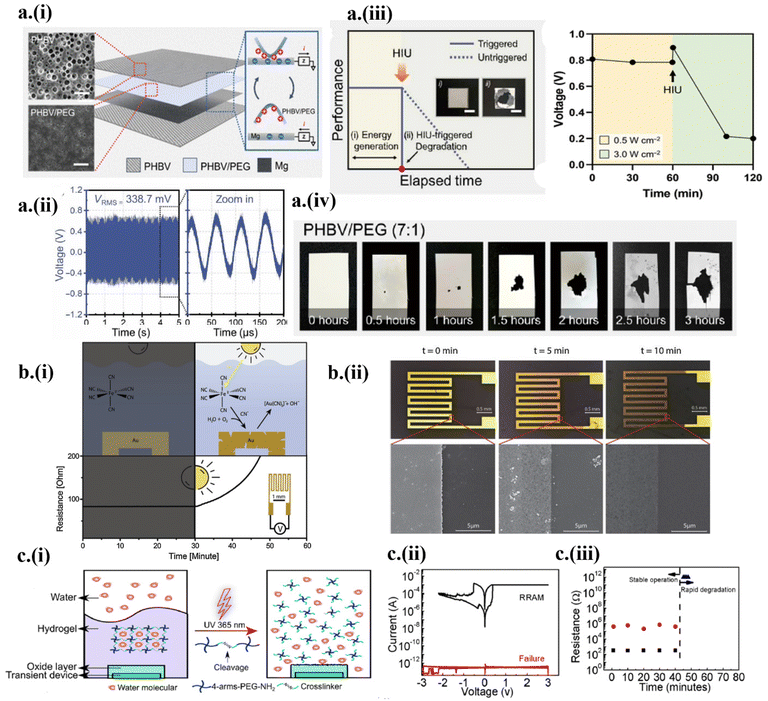 |
| Fig. 3 Wirelessly triggered on-demand transient electronics by ultrasound and light. (a) (i) The working principle of ultrasound-triggered TENG based on fully bioresorbable PHBV, PHBV/PEG, and Mg, with field-effect scanning electron microscopy (FE-SEM) images of PHBV and PHBV/PEG shown in the inset (scale 20 μm). (ii) Generated voltage from the TENG in ex vivo experiment. (iii) Voltage generated before and after the ultrasound-powered triggering event. (iv) Demonstration of different degradation stages during the triggering event. This figure has been reproduced under the terms of the Creative Commons CC BY NC License.23 Copyright 2022, The American Association for the Advancement of Science. (b) (i) A schematic showing the release of photo-initiated cyanide and gold cyanidation, which increases the resistance after the UV triggered event. (ii) Demonstration of transience and SEM images at 0, 5, and 10 min. This figure has been reproduced from ref. 56 with permission from Elsevier, copyright 2019. (c) (i) Encapsulation design of a hydrogel-oxide bilayer for controlled UV-triggered degradation. (ii) Electrical characterization of RRAM before and after the triggering event (failure). (iii) Investigation of degradation of RRAM without a light trigger. This figure has been reproduced from ref. 3 with permission from the American Chemical Society, copyright 2018. | |
2.4 Design strategy and mechanism of optically triggered transient electronics
Besides ultrasound, photo-triggerable materials (e.g., photo-acid generators or photo-induced phase transition materials) have also been recently leveraged in on-demand transient electronics systems.3,22,35,56,61 For instance, light-induced free cyanide species produced from the solar simulator oxidized the Au surface56 (Fig. 3b-i). The light-activated Au surface degradation increased with the time of light exposure, as observed in the increased resistance (Fig. 3b-ii). In another work, UV (365 nm, 300 mW cm−2) acted as a triggering external stimulus for a light-responsive hydrogel-oxide bilayer to undergo a gel-to-sol phase transition3 (Fig. 3c-i). The hydrogel layer acted as a transparent encapsulation of the Mg-based Resistive Random Access Memory (RRAM) device, where resistive switching between ON (conducting) and OFF (insulating) (ratio of >102) was achieved using oxygen vacancies-based conductive filaments (Fig. 3c-ii). The triggering of UV collapsed the hydrogel network, allowing water to pass and dissolve Mg-based RRAM (Fig. 3c-iii). The advent of transient memory devices could add an extra dimension to hardware security and zero electronics waste.
3. Implantable transient electronics in diagnostic applications
By avoiding secondary surgical complications, implantable devices with a transient nature have unique application opportunities in biomedical diagnostics,22,35,61–63 including as sensors to monitor intracranial pressure1,34,62 and dopamine.5,34,63 The ideal bioresorbable implants must maintain a user-defined operational time with unchanged sensing performance for diagnosis. However, it is challenging to maintain stable sensing performance while controlling the degradation characteristics in a transient system.24,34,63,64 A few representative transient-based diagnostic devices from recent studies are discussed in the following section.
3.1 Transient electrodes for electrophysiology and temperature/pressure sensors
As an essential biomarker for neuronal disorders (e.g., epilepsy and Alzheimer's disease) and for diagnosing irregular cardiac rhythms,1,5,6,35,62 electrophysiology from transient transistor-based neural electrode arrays can provide high-resolution spatiotemporal brain mapping. Passive transient systems typically exhibit degraded electrical performance with gradually increased bulk resistance or contact impedance over time.6,47,48,65 To address this challenge, an electrocochleography (ECoG) array combined with an intracranial pressure sensor was fabricated with a Mo electrode embedded between the poly(L-lactic acid)/polycaprolactone (PLLA/PCL) substrate and encapsulation, connected to six metal contacts in the connector pad1 (Fig. 4a-i). Penicillin given to a rat induced seizures to drastically increase the amplitude of the measured ECoG signals to 1430 μV within a narrow frequency range of 3–13 Hz, compared to the ECoG data of the rat in its non-rapid eye movement (NREM) sleep state (Fig. 4a-ii). After stable operation with an impedance of 10.4 kΩ for 80 h, degradation of the ECoG electrode in PBS buffer (37 °C, pH 7.4) signaled metal hydrolysis, drastically increasing the impedance1 (Fig. 4a-iii). The localized hydrolysis initiates from Mg contacts with a weak adhesion to the polymer. The hydrolysis follows the single-layer reactive diffusion model,47 increasing the impedance as a function of reduced thickness.
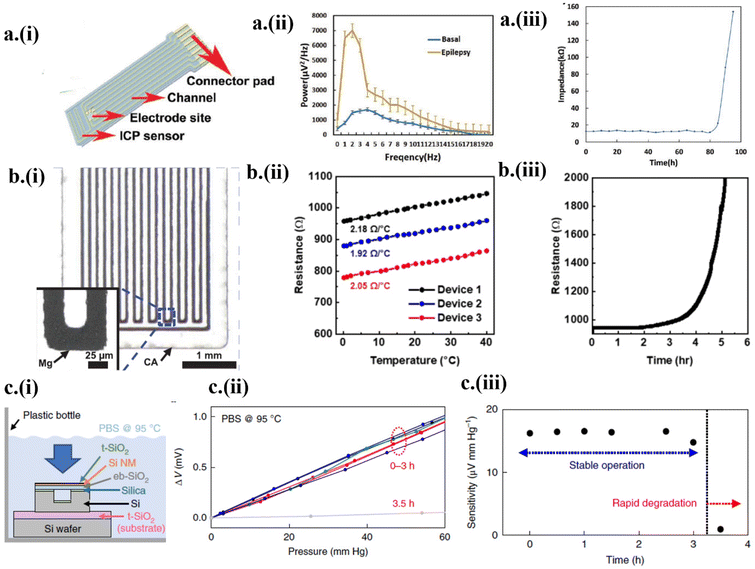 |
| Fig. 4 Transient electronics in diagnostic applications. (a) Measurements of electrophysiological signals by the ECoG device (i) designed with an intracortical pressure sensor. (ii) The power spectrum of ECoG data before and during the onset of epilepsy. (iii) Increase in impedance after the onset of degradation. This figure has been reproduced from ref. 1 with permission from John Wiley and Sons, copyright 2019. (b) (i) The design and (ii) calibration curve of the Mg-based temperature sensor with (iii) increased resistance after the initiation of the degradation process. This figure has been reproduced from ref. 21 with permission from The American Association for the Advancement of Science, copyright 2022. (c) (i) The design of the in vitro dissolution experiment with the eb-SiO2/SiNM/t-SiO2-based pressure sensor immersed in PBS solution. (ii) The linear voltage response with pressure and (iii) decreased sensitivity after 3 h due to the onset of degradation. This figure has been reproduced from ref. 66 with permission from Springer Nature, copyright 2018. | |
As a thermistor, an Mg-based temperature sensor with a width of 25 μm and length of 72 μm (Fig. 4b-i) showed a sensitivity of 2.05 ± 0.13 Ω °C−1 from 0 to 40 °C (Fig. 4b-ii).21 The encapsulation layers of POC and SiO2 provide a stable operational time of 2 h, followed by degradation in PBS (pH 7.4, 37 °C).21 The onset of hydrolysis of Mg conductors decreases its thickness following the single-layer reactive diffusion model, increasing the resistance (Fig. 4b-iii). With comparable sensitivity to conventional, commercialized pressure sensors, transient pressure sensors have been used to detect intracranial, intraocular, and blood pressure.1,22,24,35,66,67 Despite their good sensitivity, transient pressure sensors often lack stability over time. Efforts to overcome this challenge led to the recent development66 of a pressure sensor based on Si nanomembranes (NMs) sandwiched between a thermally grown Si dioxide (t-SiO2) encapsulation layer and a thick electron-beam evaporated SiO2 (eb-SiO2). The thick eb-SiO2 improved the response of the strain gauges to pressure due to the increased distance between the neutral mechanical plane and the Si NMs. With the accelerated dissolution testing setup in PBS (pH 7.4, 95 °C) (Fig. 4c-i), the complete dissociation of the encapsulating t-SiO2 layer (∼10 nm) was observed to take place within 5 h, with relatively stable performance for 3 h (Fig. 4c-ii and iii). The dissolution of the t-SiO2 encapsulation with a rate of a few hundred nanometers per day eventually leads to rapid degradation of Si NMs and bioresorbable metals due to hydrolysis. The time of stable operation could also be increased with the increased thickness of the t-SiO2 layer.
3.2 Nitric oxide and dopamine sensors
As one of the most important gaseous biomarkers for environmental and health monitoring, nitric oxide (NO) is produced in the human body to maintain a homeostatic equilibrium and provide nutrients to the tissues.4,68 With n-doped monocrystalline silicon nanomembranes (∼100 nm) and Mg (∼300 nm) electrodes encapsulated by a semipermeable membrane (Fig. 5a-i), a transient NOx sensor showed a sensitivity of 13
600% to 5 ppm NO2 at room temperature and a fast response/recovery (30/60 s).4 The linear fit between the response and gas concentration gave a limit of detection (LOD) of ∼20 ppb at room temperature (Fig. 5a-ii). The semipermeable membrane encapsulation also allowed the gas sensor to show good stability and respond to NOx in PBS solution (pH 7.4), whereas the transient gas sensor without any encapsulation dissolved in the solution with no signal (Fig. 5a-iii). Along with the degradation of the PLGA substrate, all the constituent components such as Mg electrodes and Si NMs also get dissolved via hydrolysis, leaving nontoxic end products and byproducts.
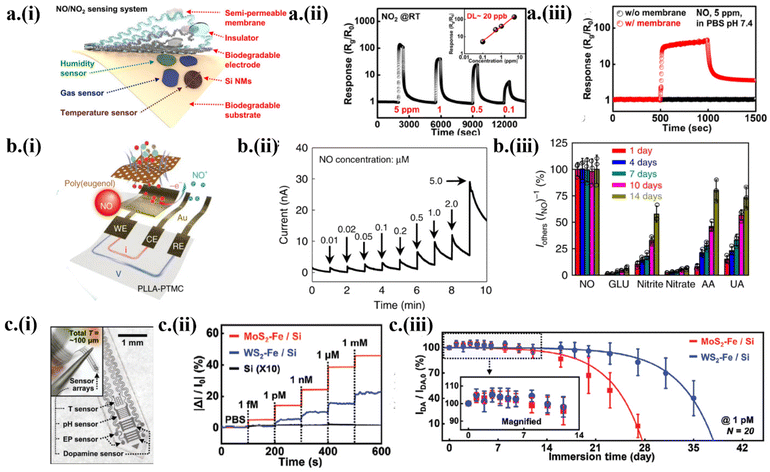 |
| Fig. 5 Transient electronics to monitor NOx and dopamine. (a) (i) The design of NOx gas integrated with temperature and humidity sensors. (ii) The linear dynamic response to various NO2 concentrations at room temperature with a linear fit to show the estimation of the limit of detection of 20 ppb. (iii) The dynamic response of the gas sensor with and without semipermeable to NO in the PBS solution. This figure has been reproduced under the terms of the Creative Commons Attribution 4.0 International License.4 Copyright 2020, Springer Nature. (b) (i) The design of the electrochemical NO sensor with Au nanomembrane electrodes on PLLA–PTMC. (ii) The dynamic electrochemical response of the sensor to varying NO concentrations (bias voltage of 0.8 V). (iii) Stability of the NO sensor selectivity showing a decrease after 7 days. This figure has been reproduced under the terms of the Creative Commons Attribution 4.0 International License.2 Copyright 2020, Springer Nature. (c) (i) Integration of a dopamine sensor with electrophysiology, temperature, and pH sensors in a neural probe. (ii) The relative current change of the neurochemical sensor to various dopamine concentrations. (iii) Change of response to 1 pM dopamine in a buffer solution for 5 weeks. This figure has been reproduced from ref. 5 with permission from John Wiley and Sons, copyright 2022. | |
Although the chemiresistive transient gas sensors may detect NOx as it adsorbs on the surface to oxidize Si NMs, more accurate real-time NOx monitoring from the solution relies on the use of chemical sensors. A transient electrochemical NO sensor was developed with Au nanomembrane electrodes and a selective membrane of poly(eugenol) film on a bioresorbable poly-L-lactic acid/poly(trimethylene carbonate) (PLLA/PTMC) copolymer substrate2 (Fig. 5b-i). By using chronoamperometry, the electrochemical sensor demonstrated a linear current response with a high sensitivity of 5.29 nA μM−1 (in 0–5 μM NO) and 4.17 nA μM−1 (in 5–100 μM NO), as well as a LOD of 3.97 nM (Fig. 5b-ii). The electrochemical NO sensor showed good selectivity with an almost linear current response to NO concentration for 7 days but the sensitivity decreased up to 1.5 times on the 14th day along with the selectivity (Fig. 5b-iii). The excellent stability over 7 days followed by a gradual decrease in the sensitivity is attributed to the stable Au nanomembrane and slow degradation rate of poly(eugenol) film and the copolymer substrate. The continuous and wireless detection of higher NO in the articular cavity of New Zealand white rabbits with anterior cruciate ligament (ACL) rupture injuries was observed to associate with the onset of osteoarthritis (OA) at the later stage.68
The electrochemical sensors can also be exploited to detect dopamine5 for neural and cardiac health monitoring, predicting diseases ranging from Parkinson's and Alzheimer's and cardiovascular diseases to cancer. For instance, a bioresorbable, wireless neurochemical system was developed based on the heterostructure of 2D molybdenum/tungsten disulfide (MoS2/WS2) nanosheets and catalytic iron nanoparticles (Fe NPs) on Si NMs5 (Fig. 5c-i). The resulting electrochemical sensor showed a linear response across the dopamine concentration from 10−15 M to 10−3 M. Compared with Fe-adsorbed Si, the 2D transition metal dichalcogenides (TMDs) in MoS2–Fe/Si and WS2–Fe/Si acted as a mediator between the Si electrodes and catalyst Fe NPs5 (Fig. 5c-ii). The excellent durability and robustness of the sensor allowed it to detect 1 pM dopamine over 40 days. The transient electrochemical sensor could retain up to 90% of the initial value over 27 days for WS2–Fe/Si and 18 days for MoS2–Fe/Si5 (Fig. 5c-iii). The relatively faster dissolution rate of MoS2 can be attributed to the thermodynamically less stable MoS2 in forming sulfate ions, favoring the forward chemical kinetics. Taken together with a wireless communication system connected to the brain-integrated probe, the simultaneously monitored dopamine and peripheral neurophysiological signals (e.g., pH, temperature, and electrophysiology) established the platform for in vivo studies of neurodegenerative diseases.
4. Implantable transient electronics in therapeutic applications
Along with sensing biomarkers, transient electronics can also deliver stimuli to modulate the biological activity or trigger drug delivery at specific target locations for therapeutics.14,24,33,35,62 Explored in neurotechnology, pancreatic and cardiac systems,13,14,62 these implantable stimulator or drug delivery systems allow the operator to control transience onset on demand.
4.1 Transient electronics-based drug-delivery systems
The implantable drug delivery system is still in its infancy in its journey towards realizing its full potential to deliver a drug at a prescribed rate and time to targeted locations to avoid off-target side effects.14,33 An ideal drug delivery system should integrate wireless control with zero leakage for avoiding unwanted delivery sites. As a first step to achieving this deterministic control over the delivery process, a programmable, bioresorbable, wireless drug delivery system based on transient polybutanedithiol 1,3,5-triallyl-1,3,5-triazine-2,4,6(1H,3H,5H)-trione penteonic anhydride (PBTPA) reservoirs with Mg active valves was developed14 (Fig. 6a-i). The wireless power harvester consisted of an Mg-based RF coil with a resonant frequency of ∼5 MHz and a rectifier circuit using a Si NM diode and Mg/SiO2/Mg capacitor14 (Fig. 6a-ii). The generated overpotential between the anode gate and cathode from the wireless power harvester accelerated the faradaic reaction at the anodic end, opening the anode gate to release the drug from the reservoir14 (Fig. 6a-iii). The increased bias decreased the time delay of the triggering onset for the drug release (Fig. 6a-iv). After the triggering onset, the diffusion-assisted drug release increased sharply and proceeded toward saturation, marking the equilibrium in drug mixing14 (Fig. 6a-v). Sequential wireless insulin triggering into the blood at different resonant frequencies in response to increased glucose (simulated from injection into the blood) demonstrated robust control of the blood glucose level14 (Fig. 6a-vi).
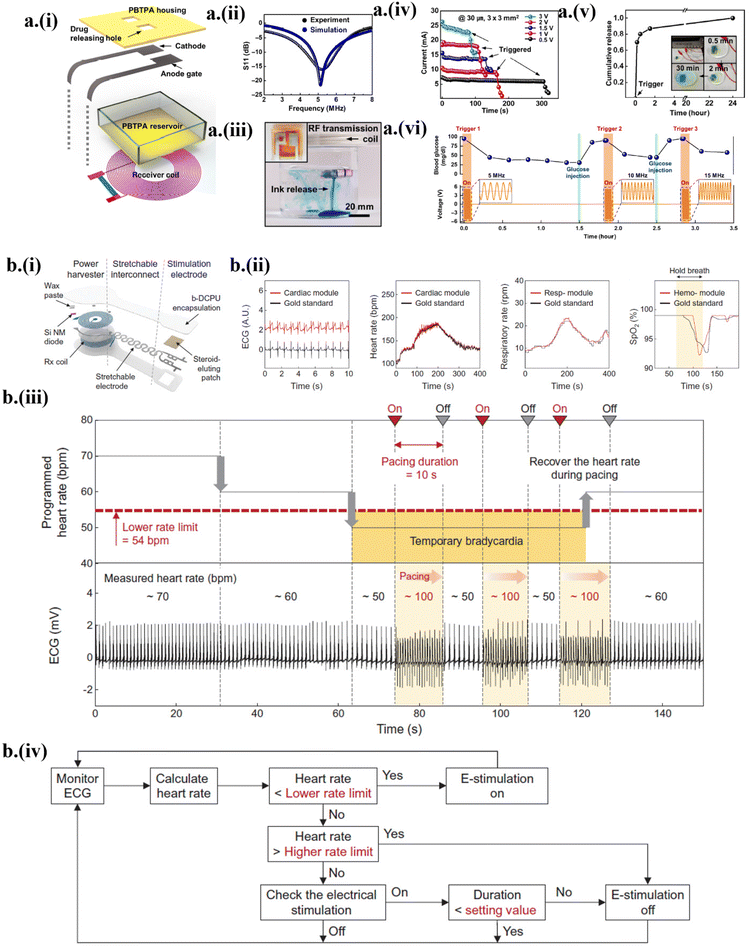 |
| Fig. 6 The transient actuating system in drug delivery and electrical stimulation. (a) (i) Design of the drug delivery system with an electrical triggering unit and bioresorbable reservoir. (ii) The return loss S11 of the Mg RF coil. (iii) Wireless controlled release of blue dye during degradation of the sensor in PBS solution. (iv) A voltage-dependent triggering event for a fixed Mg gate size. (v) Cumulative drug release after the triggered opening of the reservoir gate. (vi) Demonstration of insulin delivery to maintain the blood glucose level. This figure has been reproduced under the terms of the Creative Commons CC BY NC License.14 Copyright 2020, The American Association for the Advancement of Science. (b) (i) Bioresorbable stimulator with integrated sensing and actuating platform. (ii) Comparison of ECG, heart and respiratory rate, and SpO2 bioresorbable sensor with commercially available sensors. (iii) The triggering mechanism to maintain the average heart rate of 60 bpm based on a condition-based algorithm. (iv) Flowchart showing the condition-based algorithm to stimulate in the closed-loop system. This figure has been reproduced from ref. 33 with permission from The American Association for the Advancement of Science, copyright 2022. | |
4.2 Electrical stimulation with transient electrodes
Commercially available electrical stimulators such as the responsive neurostimulator (RNS) have been used in clinical setups to help nerve recovery and gain stability in neuronal disorders such as epilepsy and Alzheimer's disease.13,33,69 To avoid post-surgical complications from commercially rigid and non-bioresorbable stimulators,32,70 a transient stimulator with sensors to detect biomarker signals in a closed-loop system allowed automatic modulation of biological activity by stimulation based on the detected signals.32 The Si NM-based PIN diode was used to rectify the voltage generated in the Mo-based receiver coil, which generated stimulation through Mo-based electrodes33 (Fig. 6b-i). The slow dissolution rate of the bioresorbable Mo conductor (tested in PBS at 95 °C) enables a long-term (>1 month) operational lifetime. The holistic sensing system comprised skin-interfaced ECG electrodes, a microcontroller unit to calculate heart rate and respiratory rate, and a hemodynamic module to collect the SpO2 data33 (Fig. 6b-ii). The sensing data wirelessly transferred to the cloud were used to process and optimize stimulation parameters according to a threshold-based algorithm. The closed-loop system automatically initiated pacing of 100 beats per minute (bpm) when the heart rate fell below 54 bpm and ceased on-demand stimulation to maintain an average heart rate of 60 bpm33 (Fig. 6b-iii) using a condition-based algorithm (Fig. 6b-iv).
5. Conclusions and future directions
Since the initial demonstration of transient devices a little more than ten years ago, different bioresorbable materials and unconventional fabrication approaches have been explored to enable controlled degradation or disintegration of the device at a prescribed rate or time. After the investigations of strategies for controlling the on-demand degradation of the device, stimulating devices have been further integrated with sensors to yield a transient closed-loop system that performs stimulation according to condition-based algorithms.33 However, the if–else algorithms result in inefficient and impotent control logic. A pre-defined threshold-based decision-making controller almost always operates in a limited scope in practical settings, as it is unaware of past medical conditions and present environmental scenarios. A closed-loop system based on a more practical and artificial intelligence (AI)-powered controller is therefore imperative.32 Lessons can be learned from other biomedical closed-loop systems. For instance, an implantable pulse generator could carry out epidural electrical stimulation (EES) of the spinal cord with a spatiotemporally adaptive trigger controller.71 The sensing system consisted of the electromyography (EMG) response of the leg muscle and the whole-body ground reaction. With the option of a pre-programmed open-loop or closed-loop, the wireless communication module carried out the EES with dynamic control over EES parameters71 (Fig. 7a-i). Only with the introduction of a closed-loop spatiotemporal EES, could the participants voluntarily walk and reintroducing EES after a stoppage resumed the participant's locomotion71 (Fig. 7a-ii). Similar to spinal cord stimulation, closed-loop deep brain stimulation (DBS) or neuromodulation was recently reported as well. With measured local field potential (LFP) to automatically detect pain in a closed-loop system, a brain–machine interface was set up to optogenetically or electrically perform DBS69 (Fig. 7b-i). The multiregional neural interface from the anterior cingulate cortex (ACC) and somatosensory cortex (S1) could detect and treat acute evoked and chronic pain. By computing frequency-dependent LFP power features, a real-time neural decoder based on a Markovian-driven state space model (unsupervised learning) was designed to decode the pain onset69 (Fig. 7b-ii), which applied the DBS to reduce the acute thermal pain69 (Fig. 7b-iii).
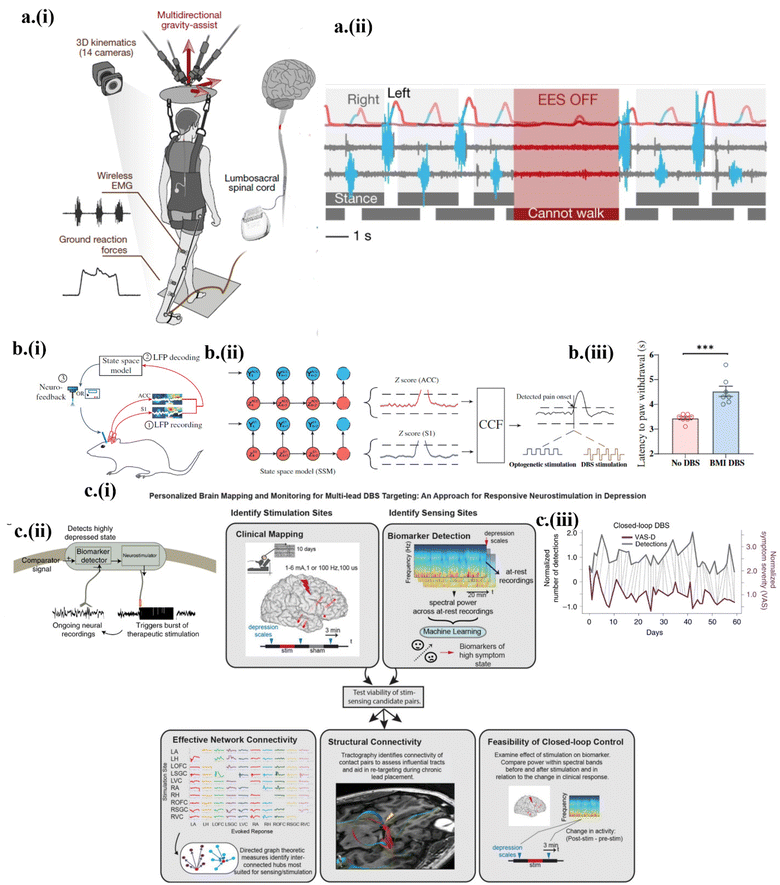 |
| Fig. 7 On-demand closed-loop transient systems. (a) Epidural electrical stimulation (EES) of the spinal cord with (i) the control module to (ii) demonstrate spatiotemporal EES for voluntary walking. This figure has been reproduced from ref. 71 with permission from Springer Nature, copyright 2018. (b) The closed-loop brain–machine interface with (i) the control module based on the detection of low field potential for deep brain stimulation (DBS). (ii) Representation of the Markovian-driven state space model to detect pain onset. (iii) Demonstration of improved latency with the brain–machine interface-based DBS. This figure has been reproduced from ref. 69 with permission from The American Association for the Advancement of Science, copyright 2022. (c) Closed-loop neuromodulation relies on (i) the sequential algorithm optimizing the location of the biomarker, validating the network and structural connectivity and the stimulation parameters. (ii) The NeuroPace RNS system with biomarker detection and stimulation. (iii) Aligning biomarker detection with VAS rating using dynamic time wrapping for demonstrated improvement due to biomarker-driver stimulation. This figure has been reproduced from ref. 32 with permission from Springer Nature, copyright 2021. | |
Along with intelligent closed loop-based stimulation, a protocol of sequential searching for biomarker signals and optimizing stimulation parameters has also been effective in complicated, biological systems. As an example, biomarker-driven closed-loop DBS was carried out to treat the major depressive disorder (MDD).32 Unlike Parkinson's disease or epilepsy, MDD requires personalized neural circuit targeting, which results in the patient's own physiological biomarkers-based trigger or stimulation. The stimulus-response mapping was initially performed for the emotion neural circuit using stereoelectroencephalography (SEEG) electrodes at important regions such as the amygdala, hippocampus, and ventral capsule/ventral striatum (VC/VS). The SEEG spectral activity identified amygdala gamma power as a sufficient biomarker. With the right VC/VS as the selected stimulation site, the correlation between the stimulation parameters and the amygdala gamma power was analyzed32 (Fig. 7c-i). According to the correlation, the FDA-approved NeuroPace RNS system was used with two separate sensing and stimulation leads simultaneously placed in the amygdala and VC/VS region for stimulation with the optimized parameters32 (Fig. 7c-ii). The implementation of closed-loop neuromodulation resulted in significant improvement in the symptom severity according to the visual analog scale (VAS) scales32 (Fig. 7c-iii).
In addition to the closed-loop system or system-level integration of transient electronics, there are still a few fundamental challenges that need to be addressed. For example, multiple sensing units (e.g., temperature, pH, and pressure) in the transient device may affect each other so decoupling of the sensing data would need to be performed.72 Furthermore, with the advent of on-demand transience, the selectivity of multimodal transient electronic devices in the presence of more than one external (active and passive) stimuli is often ignored. For example, transient devices that disintegrate faster in electrical (active) stimuli and comparatively slower in optical (passive) stimuli are expected to dissociate at an unexpected rate in the presence of both stimuli. Moreover, it becomes difficult to control the influence of passive stimuli on disintegration of the device, presenting challenges for its use and robustness in real-world applications. An intuitive strategy to solve this issue is to incorporate multilayer encapsulations with materials with complementary dissociation-selectivity towards stimuli. For example, materials that dissociate in electrical and water stimuli and materials with electrical and optical stimuli-specific dissolution may form a multi-layer encapsulation to implement transience that can only be triggered by an electrical stimulus, increasing the selectivity towards electrical triggered transience. Employing specific sequence-based external stimuli can result in a complete dissociation of the transient device with composite encapsulations.64 The sequence of stimuli-selective materials in a composite forms an intelligent hardware-based password to trigger dissolution rather than a destined degradation, adding an extra dimension to the security. The ultimate practical, robust transient electronics system hinges on the mature developments in closed-loop therapeutics, the decoupling mechanism, and dissolution selectivity as discussed above. Although considerable advancements have been made, the field of intelligent transient electronics systems is still in its infancy. However, the introduction of intelligent, robust transient electronics systems will open up opportunities from hardware security to biomedical diagnosis and therapeutics in the real world.
Conflicts of interest
The authors declare that there are no competing financial interests that could appear to influence this work.
Acknowledgements
HC acknowledges the support provided by NIH (award no. R21EB030140, U01DA056242, and R61HL154215), NSF (grant no. ECCS-1933072), and Penn State University.
References
- K. Xu, S. Li, S. Dong, S. Zhang, G. Pan, G. Wang, L. Shi, W. Guo, C. Yu and J. Luo, Adv. Healthc. Mater., 2019, 8, 1801649 CrossRef PubMed.
- R. Li, H. Qi, Y. Ma, Y. Deng, S. Liu, Y. Jie, J. Jing, J. He, X. Zhang, L. Wheatley, C. Huang, X. Sheng, M. Zhang and L. Yin, Nat. Commun., 2020, 11, 3207 CrossRef CAS PubMed.
- S. Zhong, X. Ji, L. Song, Y. Zhang and R. Zhao, ACS Appl. Mater. Interfaces, 2018, 10, 36171–36176 CrossRef CAS PubMed.
- G. J. Ko, S. D. Han, J. K. Kim, J. Zhu, W. B. Han, J. Chung, S. M. Yang, H. Cheng, D. H. Kim, C. Y. Kang and S. W. Hwang, NPG Asia Mater., 2020, 12, 71 CrossRef CAS.
- S. M. Yang, J. H. Shim, H. U. Cho, T. M. Jang, G. J. Ko, J. Shim, T. H. Kim, J. Zhu, S. Park, Y. S. Kim, S. Y. Joung, J. C. Choe, J. W. Shin, J. H. Lee, Y. M. Kang, H. Cheng, Y. Jung, C. H. Lee, D. P. Jang and S. W. Hwang, Adv. Mater., 2022, 34, 2108203 CrossRef CAS PubMed.
- S. K. Kang, R. K. J. Murphy, S. W. Hwang, S. M. Lee, D. V. Harburg, N. A. Krueger, J. Shin, P. Gamble, H. Cheng, S. Yu, Z. Liu, J. G. McCall, M. Stephen, H. Ying, J. Kim, G. Park, R. C. Webb, C. H. Lee, S. Chung, D. S. Wie, A. D. Gujar, B. Vemulapalli, A. H. Kim, K. M. Lee, J. Cheng, Y. Huang, S. H. Lee, P. V. Braun, W. Z. Ray and J. A. Rogers, Nature, 2016, 530, 71–76 CrossRef CAS PubMed.
- C. M. Boutry, Y. Kaizawa, B. C. Schroeder, A. Chortos, A. Legrand, Z. Wang, J. Chang, P. Fox and Z. Bao, Nat. Electron., 2018, 1, 314–321 CrossRef.
- H. Lopez Hernandez, S. K. Kang, O. P. Lee, S. W. Hwang, J. A. Kaitz, B. Inci, C. W. Park, S. Chung, N. R. Sottos, J. S. Moore, J. A. Rogers and S. R. White, Adv. Mater., 2014, 26, 7637–7642 CrossRef PubMed.
- T. Lei, M. Guan, J. Liu, H. C. Lin, R. Pfattner, L. Shaw, A. F. McGuire, T. C. Huang, L. Shao, K. T. Cheng, J. B. H. Tok and Z. Bao, Proc. Natl. Acad. Sci. U. S. A., 2017, 114, 5107–5112 CrossRef CAS PubMed.
- P. S. Liu and H. F. Tse, J. Arrhythm., 2013, 29, 314–319 CrossRef.
- R. J. Vetter, J. C. Williams, J. F. Hetke, E. A. Nunamaker and D. R. Kipke, IEEE Trans. Biomed. Eng., 2004, 51, 896–904 CrossRef PubMed.
- H. U. Chung, A. Y. Rwei, A. Hourlier-Fargette, S. Xu, K. H. Lee, E. C. Dunne, Z. Xie, C. Liu, A. Carlini, D. H. Kim, D. Ryu, E. Kulikova, J. Cao, I. C. Odland, K. B. Fields, B. Hopkins, A. Banks, C. Ogle, D. Grande, J. B. Park, J. Kim, M. Irie, H. Jang, J. H. Lee, Y. Park, J. Kim, H. H. Jo, H. Hahm, R. Avila, Y. Xu, M. Namkoong, J. W. Kwak, E. Suen, M. A. Paulus, R. J. Kim, B. V. Parsons, K. A. Human, S. S. Kim, M. Patel, W. Reuther, H. S. Kim, S. H. Lee, J. D. Leedle, Y. Yun, S. Rigali, T. Son, I. Jung, H. Arafa, V. R. Soundararajan, A. Ollech, A. Shukla, A. Bradley, M. Schau, C. M. Rand, L. E. Marsillio, Z. L. Harris, Y. Huang, A. Hamvas, A. S. Paller, D. E. Weese-Mayer, J. Y. Lee and J. A. Rogers, Nat. Med., 2020, 26, 418–429 CrossRef CAS PubMed.
- G. Lee, E. Ray, H. J. Yoon, S. Genovese, Y. S. Choi, M. K. Lee, S. Şahin, Y. Yan, H. Y. Ahn, A. J. Bandodkar, J. Kim, M. Park, H. Ryu, S. S. Kwak, Y. H. Jung, A. Odabas, U. Khandpur, W. Z. Ray, M. R. MacEwan and J. A. Rogers, Sci. Adv., 2022, 8, eabp9169 CrossRef CAS PubMed.
- J. Koo, S. B. Kim, Y. S. Choi, Z. Xie, A. J. Bandodkar, J. Khalifeh, Y. Yan, H. Kim, M. K. Pezhouh, K. Doty, G. Lee, Y. Y. Chen, S. M. Lee, D. D'Andrea, K. Jung, K. H. Lee, K. Li, S. Jo, H. Wang, J. H. Kim, J. Kim, S. G. Choi, W. J. Jang, Y. S. Oh, I. Park, S. S. Kwak, J. H. Park, D. Hong, X. Feng, C. H. Lee, A. Banks, C. Leal, H. M. Lee, Y. Huang, C. K. Franz, W. Z. Ray, M. MacEwan, S. K. Kang and J. A. Rogers, Sci. Adv., 2020, 6, 20 Search PubMed.
- M. Prakasam, J. Locs, K. Salma-Ancane, D. Loca, A. Largeteau and L. Berzina-Cimdina, J. Funct. Biomater., 2017, 8, 44 CrossRef PubMed.
- J. Koo, M. R. MacEwan, S. K. Kang, S. M. Won, M. Stephen, P. Gamble, Z. Xie, Y. Yan, Y. Y. Chen, J. Shin, N. Birenbaum, S. Chung, S. B. Kim, J. Khalifeh, D. V. Harburg, K. Bean, M. Paskett, J. Kim, Z. S. Zohny, S. M. Lee, R. Zhang, K. Luo, B. Ji, A. Banks, H. M. Lee, Y. Huang, W. Z. Ray and J. A. Rogers, Nat. Med., 2018, 24, 1830–1836 CrossRef CAS PubMed.
- C. Li, C. Guo, V. Fitzpatrick, A. Ibrahim, M. J. Zwierstra, P. Hanna, A. Lechtig, A. Nazarian, S. J. Lin and D. L. Kaplan, Nat. Rev. Mater., 2020, 5, 61–81 CrossRef.
- A. D. Mickle, S. M. Won, K. N. Noh, J. Yoon, K. W. Meacham, Y. Xue, L. A. McIlvried, B. A. Copits, V. K. Samineni, K. E. Crawford, D. H. Kim, P. Srivastava, B. H. Kim, S. Min, Y. Shiuan, Y. Yun, M. A. Payne, J. Zhang, H. Jang, Y. Li, H. H. Lai, Y. Huang, S. I. Park, R. W. Gereau and J. A. Rogers, Nature, 2019, 565, 361–365 CrossRef CAS PubMed.
- Y. S. Choi, R. T. Yin, A. Pfenniger, J. Koo, R. Avila, K. Benjamin Lee, S. W. Chen, G. Lee, G. Li, Y. Qiao, A. Murillo-Berlioz, A. Kiss, S. Han, S. M. Lee, C. Li, Z. Xie, Y. Y. Chen, A. Burrell, B. Geist, H. Jeong, J. Kim, H. J. Yoon, A. Banks, S. K. Kang, Z. J. Zhang, C. R. Haney, A. V. Sahakian, D. Johnson, T. Efimova, Y. Huang, G. D. Trachiotis, B. P. Knight, R. K. Arora, I. R. Efimov and J. A. Rogers, Nat. Biotechnol., 2021, 39, 1228–1238 CrossRef CAS PubMed.
- Y. S. Choi, Y. Y. Hsueh, J. Koo, Q. Yang, R. Avila, B. Hu, Z. Xie, G. Lee, Z. Ning, C. Liu, Y. Xu, Y. J. Lee, W. Zhao, J. Fang, Y. Deng, S. M. Lee, A. Vázquez-Guardado, I. Stepien, Y. Yan, J. W. Song, C. Haney, Y. S. Oh, W. Liu, H. J. Yun, A. Banks, M. R. MacEwan, G. A. Ameer, W. Z. Ray, Y. Huang, T. Xie, C. K. Franz, S. Li and J. A. Rogers, Nat. Commun., 2020, 11, 5990 CrossRef CAS PubMed.
- J. T. Reeder, Z. Xie, Q. Yang, M. H. Seo, Y. Yan, Y. Deng, K. R. Jinkins, S. R. Krishnan, C. Liu, S. McKay, E. Patnaude, A. Johnson, Z. Zhao, M. J. Kim, Y. Xu, I. Huang, R. Avila, C. Felicelli, E. Ray, X. Guo, W. Z. Ray, Y. Huang, M. R. MacEwan and J. A. Rogers, Science, 2022, 377, 109–115 CrossRef CAS PubMed.
- C. You, H. Zhao, Q. Guo and Y. Mei, MRS Bull., 2020, 45, 129–134 CrossRef.
- D. M. Lee, N. Rubab, I. Hyun, W. Kang, Y. J. Kim, M. Kang, B. O. Choi and S. W. Kim, Sci. Adv., 2022, 8, 8423 CrossRef PubMed.
- G. Lee, Y. S. Choi, H. J. Yoon and J. A. Rogers, Matter, 2020, 3, 1031–1052 CrossRef.
- J. W. Shin, J. Chan Choe, J. H. Lee, W. B. Han, T. M. Jang, G. J. Ko, S. M. Yang, Y. G. Kim, J. Joo, B. H. Lim, E. Park and S. W. Hwang, ACS Nano, 2021, 15, 19310–19320 CrossRef CAS PubMed.
- J. Ausra, M. Madrid, R. T. Yin, J. Hanna, S. Arnott, J. A. Brennan, R. Peralta, D. Clausen, J. A. Bakall, I. R. Efimov and P. Gutruf, Sci. Adv., 2022, 8, eabq7469 CrossRef CAS PubMed.
- Y. Chen, H. Wang, Y. Zhang, R. Li, C. Chen, H. Zhang, S. Tang, S. Liu, X. Chen, H. Wu, R. Lv, X. Sheng, P. Zhang, S. Wang and L. Yin, Nanotechnology, 2019, 30, 394002 CrossRef CAS PubMed.
- C. M. Boutry, H. Chandrahalim, P. Streit, M. Schinhammer, A. C. Hänzi and C. Hierold, Philos. Trans. R. Soc., A, 2012, 370, 2418–2432 CrossRef CAS PubMed.
- R. Proietti, G. Manzoni, L. di Biase, G. Castelnuovo, L. Lombardi, C. Fundarò, N. Vegliante, G. Pietrabissa, P. Santangeli, R. A. Canby, A. Sagone, M. Viecca and A. Natale, Pacing Clin. Electrophysiol., 2012, 35, 990–998 CrossRef PubMed.
- C. G. Kanakry, Sci. Transl. Med., 2020, 12, eabb2779 CrossRef.
- R. Hovorka, Diabetic Med., 2006, 23, 1–12 CrossRef CAS PubMed.
- K. W. Scangos, A. N. Khambhati, P. M. Daly, G. S. Makhoul, L. P. Sugrue, H. Zamanian, T. X. Liu, V. R. Rao, K. K. Sellers, H. E. Dawes, P. A. Starr, A. D. Krystal and E. F. Chang, Nat. Med., 2021, 27, 1696–1700 CrossRef CAS PubMed.
- Y. S. Choi, H. Jeong, R. T. Yin, R. Avila, A. Pfenniger, J. Yoo, J. Y. Lee, A. Tzavelis, Y. J. Lee, S. W. Chen, H. S. Knight, S. Kim, H. Y. Ahn, G. Wickerson, A. Vázquez-Guardado, E. Higbee-Dempsey, B. A. Russo, M. A. Napolitano, T. J. Holleran, L. A. Razzak, A. N. Miniovich, G. Lee, B. Geist, B. Kim, S. Han, J. A. Brennan, K. Aras, S. S. Kwak, J. Kim, E. A. Waters, X. Yang, A. Burrell, K. S. Chun, C. Liu, C. Wu, A. Y. Rwei, A. N. Spann, A. Banks, D. Johnson, Z. J. Zhang, C. R. Haney, S. H. Jin, A. V. Sahakian, Y. Huang, G. D. Trachiotis, B. P. Knight, R. K. Arora, I. R. Efimov and J. A. Rogers, Science, 2022, 376, 1006–1012 CrossRef CAS PubMed.
- R. Li, L. Wang, D. Kong and L. Yin, Bioact. Mater., 2017, 3, 322–333 CrossRef PubMed.
- J. S. Shim, J. A. Rogers and S. K. Kang, Mater. Sci. Eng., R, 2021, 145, 100624 CrossRef.
- L. Yin, X. Huang, H. Xu, Y. Zhang, J. Lam, J. Cheng and J. A. Rogers, Adv. Mater., 2014, 26, 3879–3884 CrossRef CAS PubMed.
- Y. H. Jung, H. Zhang, S. Gong and Z. Ma, Semicond. Sci. Technol., 2017, 32, 063002 CrossRef.
- Q. Yang, S. Lee, Y. Xue, Y. Yan, T. L. Liu, S. K. Kang, Y. J. Lee, S. H. Lee, M. H. Seo, D. Lu, J. Koo, M. R. MacEwan, R. T. Yin, W. Z. Ray, Y. Huang and J. A. Rogers, Adv. Funct. Mater., 2020, 30, 1910718 CrossRef CAS.
- S. W. Hwang, X. Huang, J. H. Seo, J. K. Song, S. Kim, S. Hage-Ali, H. J. Chung, H. Tao, F. G. Omenetto, Z. Ma and J. A. Rogers, Adv. Mater., 2013, 25, 3526–3531 CrossRef CAS PubMed.
- X. Huang, Y. Liu, S. W. Hwang, S. K. Kang, D. Patnaik, J. F. Cortes and J. A. Rogers, Adv. Mater., 2014, 26, 7371–7377 CrossRef CAS PubMed.
- Y. Choi, J. Koo and J. A. Rogers, MRS Bull., 2020, 45, 103–112 CrossRef.
- S. W. Hwang, D. H. Kim, H. Tao, T. I. Kim, S. Kim, K. J. Yu, B. Panilaitis, J. W. Jeong, J. K. Song, F. G. Omenetto and J. A. Rogers, Adv. Funct. Mater., 2013, 23, 4087–4093 CrossRef CAS.
- J.-K. Chang, Proc. Natl. Acad. Sci. U. S. A., 2017, 114, E5522–E5529 CAS.
- Q. Yang, T. Wei, R. T. Yin, M. Wu, Y. Xu, J. Koo, Y. S. Choi, Z. Xie, S. W. Chen, I. Kandela, S. Yao, Y. Deng, R. Avila, T. L. Liu, W. Bai, Y. Yang, M. Han, Q. Zhang, C. R. Haney, K. Benjamin Lee, K. Aras, T. Wang, M. H. Seo, H. Luan, S. M. Lee, A. Brikha, N. Ghoreishi-Haack, L. Tran, I. Stepien, F. Aird, E. A. Waters, X. Yu, A. Banks, G. D. Trachiotis, J. M. Torkelson, Y. Huang, Y. Kozorovitskiy, I. R. Efimov and J. A. Rogers, Nat. Mater., 2021, 20, 1559–1570 CrossRef CAS PubMed.
- Y. S. Choi, J. Koo, Y. J. Lee, G. Lee, R. Avila, H. Ying, J. Reeder, L. Hambitzer, K. Im, J. Kim, K. M. Lee, J. Cheng, Y. Huang, S. K. Kang and J. A. Rogers, Adv. Funct. Mater., 2020, 30, 2000941 CrossRef CAS.
- S. W. Hwang, J. K. Song, X. Huang, H. Cheng, S. K. Kang, B. H. Kim, J. H. Kim, S. Yu, Y. Huang and J. A. Rogers, Adv. Mater., 2014, 26, 3905–3911 CrossRef CAS PubMed.
- L. Yin, H. Cheng, S. Mao, R. Haasch, Y. Liu, X. Xie, S. W. Hwang, H. Jain, S. K. Kang, Y. Su, R. Li, Y. Huang and J. A. Rogers, Adv. Funct. Mater., 2014, 24, 645–658 CrossRef CAS.
- H. Ryu, M. H. Seo and J. A. Rogers, Adv. Healthc. Mater., 2021, 10, 2002236 CrossRef CAS PubMed.
- S. K. Kang, G. Park, K. Kim, S. W. Hwang, H. Cheng, J. Shin, S. Chung, M. Kim, L. Yin, J. C. Lee, K. M. Lee and J. A. Rogers, ACS Appl. Mater. Interfaces, 2015, 7, 9297–9305 CrossRef CAS PubMed.
- L. Teng, S. Ye, S. Handschuh-Wang, X. Zhou, T. Gan, X. Zhou, L. Teng, S. C. Ye, S. Handschuh-Wang, X. H. Zhou, T. S. Gan and X. C. Zhou, Adv. Funct. Mater., 2019, 29, 1808739 CrossRef.
- Q. Guo, J. Koo, Z. Xie, R. Avila, X. Yu, X. Ning, H. Zhang, X. Liang, S. B. Kim, Y. Yan, M. R. MacEwan, H. M. Lee, A. Song, Z. Di, Y. Huang, Y. Mei and J. A. Rogers, Adv. Funct. Mater., 2019, 29, 1905451 CrossRef CAS.
- N. Yi, Y. Gao, A. Lo Verso, J. Zhu, D. Erdely, C. Xue, R. Lavelle and H. Cheng, Mater. Today, 2021, 50, 24–34 CrossRef CAS PubMed.
- R. Li, H. Cheng, Y. Su, S. W. Hwang, L. Yin, H. Tao, M. A. Brenckle, D. H. Kim, F. G. Omenetto, J. A. Rogers and Y. Huang, Adv. Funct. Mater., 2013, 23, 3106–3114 CrossRef CAS.
- S. K. Kang, S. W. Hwang, H. Cheng, S. Yu, B. H. Kim, J. H. Kim, Y. Huang and J. A. Rogers, Adv. Funct. Mater., 2014, 24, 4427–4434 CrossRef CAS.
- X. Zhang, C. M. Weber and L. M. Bellan, Adv. Mater. Technol., 2019, 4, 1900528 CrossRef CAS.
- W. D. Chen, S. K. Kang, W. J. Stark, J. A. Rogers and R. N. Grass, Sens. Actuators, B, 2019, 282, 52–59 CrossRef CAS.
- Y. Chen, H. Wang, Y. Zhang, R. Li, C. Chen, H. Zhang, S. Tang, S. Liu, X. Chen, H. Wu, R. Lv, X. Sheng, P. Zhang, S. Wang and L. Yin, Nanotechnology, 2019, 30, 394002 CrossRef CAS PubMed.
- L. A. Wehner, N. Mittal, T. Liu and M. Niederberger, ACS Cent. Sci., 2021, 7, 231–244 CrossRef CAS PubMed.
- C. Dagdeviren, S. W. Hwang, Y. Su, S. Kim, H. Cheng, O. Gur, R. Haney, F. G. Omenetto, Y. Huang and J. A. Rogers, Small, 2013, 9, 3398–3404 CrossRef CAS PubMed.
- R. Hinchet, H. J. Yoon, H. Ryu, M. K. Kim, E. K. Choi, D. S. Kim and S. W. Kim, Science, 2019, 365, 491–494 CrossRef CAS PubMed.
- R. Jamshidi, M. Taghavimehr, Y. Chen, N. Hashemi and R. Montazami, Adv. Sustainable Syst., 2022, 6, 2100057 CrossRef.
- A. Fanelli and D. Ghezzi, Curr. Opin. Biotechnol, 2021, 72, 22–28 CrossRef CAS PubMed.
- W. B. Han, H. Lee, J.-W. Shin, S.-W. Hwang, W. B. Han, J. H. Lee, J.-W. Shin and S.-W. Hwang, Adv. Mater., 2020, 32, 2002211 CrossRef CAS PubMed.
- S. Wei, J. Jiang, L. Sun, J. Li, T. H. Tao, Z. Zhou, S. Wei, J. Jiang, L. Sun, T. H. Tao, Z. Zhou and J. Li, Adv. Mater., 2022, 34, 2201035 CrossRef CAS PubMed.
- Q. Yang, T. L. Liu, Y. Xue, H. Wang, Y. Xu, B. Emon, M. Wu, C. Rountree, T. Wei, I. Kandela, C. R. Haney, A. Brikha, I. Stepien, J. Hornick, R. A. Sponenburg, C. Cheng, L. Ladehoff, Y. Chen, Z. Hu, C. Wu, M. Han, J. M. Torkelson, Y. Kozorovitskiy, M. T. A. Saif, Y. Huang, J. K. Chang and J. A. Rogers, Nat. Electron., 2022, 5, 526–538 CrossRef CAS.
- J. Shin, Y. Yan, W. Bai, Y. Xue, P. Gamble, L. Tian, I. Kandela, C. R. Haney, W. Spees, Y. Lee, M. Choi, J. Ko, H. Ryu, J. K. Chang, M. Pezhouh, S. K. Kang, S. M. Won, K. J. Yu, J. Zhao, Y. K. Lee, M. R. MacEwan, S. K. Song, Y. Huang, W. Z. Ray and J. A. Rogers, Nat. Biomed. Eng., 2019, 3, 37–46 CrossRef CAS PubMed.
- D. Lu, Y. Yan, Y. Deng, Q. Yang, J. Zhao, M. H. Seo, W. Bai, M. R. MacEwan, Y. Huang, W. Z. Ray and J. A. Rogers, Adv. Funct. Mater., 2020, 30, 2003754 CrossRef CAS.
- Y. Deng, H. Qi, Y. Ma, S. Liu, M. Zhao, Z. Guo, Y. Jie, R. Zheng, J. Jing, K. Chen, H. Ding, G. Lv, K. Zhang, R. Li, H. Cheng, L. Zhao, X. Sheng, M. Zhang and L. Yin, Proc. Natl. Acad. Sci. U. S. A., 2022, 119, e2208060119 CrossRef CAS PubMed.
- G. Sun, F. Zeng, M. McCartin, Q. Zhang, H. Xu, Y. Liu, Z. S. Chen and J. Wang, Sci. Transl. Med., 2022, 14, eabm5868 CrossRef CAS PubMed.
- G. K. Bergey, M. J. Morrell, E. M. Mizrahi, A. Goldman, D. King-Stephens, D. Nair, S. Srinivasan, B. Jobst, R. E. Gross, D. C. Shields, G. Barkley, V. Salanova, P. Olejniczak, A. Cole, S. S. Cash, K. Noe, R. Wharen, G. Worrell, A. M. Murro, J. Edwards, M. Duchowny, D. Spencer, M. Smith, E. Geller, R. Gwinn, C. Skidmore, S. Eisenschenk, M. Berg, C. Heck, P. van Ness, N. Fountain, P. Rutecki, A. Massey, C. O'Donovan, D. Labar, R. B. Duckrow, L. J. Hirsch, T. Courtney, F. T. Sun and C. G. Seale, Neurology, 2015, 84, 810–817 CrossRef CAS PubMed.
- F. B. Wagner, J. B. Mignardot, C. G. Le Goff-Mignardot, R. Demesmaeker, S. Komi, M. Capogrosso, A. Rowald, I. Seáñez, M. Caban, E. Pirondini, M. Vat, L. A. McCracken, R. Heimgartner, I. Fodor, A. Watrin, P. Seguin, E. Paoles, K. van den Keybus, G. Eberle, B. Schurch, E. Pralong, F. Becce, J. Prior, N. Buse, R. Buschman, E. Neufeld, N. Kuster, S. Carda, J. von Zitzewitz, V. Delattre, T. Denison, H. Lambert, K. Minassian, J. Bloch and G. Courtine, Nature, 2018, 563, 65–71 CrossRef CAS PubMed.
- R. Yang, W. Zhang, N. Tiwari, H. Yan, T. Li and H. Cheng, Adv. Sci., 2022, 9, 2202470 CrossRef PubMed.
|
This journal is © The Royal Society of Chemistry 2023 |
Click here to see how this site uses Cookies. View our privacy policy here.