DOI:
10.1039/D3NR03808D
(Minireview)
Nanoscale, 2023,
15, 17727-17738
Bridging the gap: harnessing liquid nanomachine know-how for tackling harmful airborne particulates
Received
31st July 2023
, Accepted 21st September 2023
First published on 26th September 2023
Abstract
The emergence of “nanomotors”, “nanomachines”, and “nanorobotics” has transformed dynamic nanoparticle research, driving a transition from passive to active and intelligent nanoscale systems. This review examines two critical fields: the investigation of airborne particles, significant contributors to air pollution, and the rapidly emerging domain of catalytic and field-controlled nano- and micromotors. We examine the basic concepts of nano- and micromachines in motion and envision their possible use in a gaseous medium to trap and neutralize hazardous particulates. While past studies described the application of nanotechnology and nanomotors in various scenarios, airborne nano/micromachine motion and their control have yet to be thoroughly explored. This review intends to promote multidisciplinary research on nanomachines’ propulsion and task-oriented applications, highlighting their relevance in obtaining a cleaner atmospheric environment, a critical component to consider for human health.
1. Introduction
The capacity of airborne particles to traverse large distances due to the high air drag-to-weight ratio is one of the factors that has contributed to the worsening of the situation regarding air pollution since the beginning of the industrial revolution.1 The current techniques used to facilitate the capture of particulate matter (PM), including electrostatic precipitators, nanoscale meshes, and magnetic traps, have not yet produced a universally practical and effective solution to prevent the diverse health effects of air pollution.2 The field of nanoscience has witnessed a significant evolution over the past few decades, with the shift in research focus from static nanoparticles to more dynamic and interactive terms such as “nanomotors”, “nanomachines” and “nanorobots”.3,4 These newly introduced terms brought a dynamic aspect to the field, suggesting movement, control, and tasks at the nanoscale.5 This shift in terminology has profound and far-reaching impacts, leading to a surge in research focusing on the control of nanoparticles’ speed, motion type and traveling distance, resulting in broad applications.6–8 Some common types of nanomachines include Janus spheres, nanowires, microjets and particles with chiral structures.9 Versatile methods of nanomachines’ external control have been demonstrated using ultrasound,10 electrochemical potential,11 oscillating electric (AC) field,12 magnetic field,13 light,14 and chemotaxis,15 to name a few examples. Some prominent fields of nanomachine applications are medicine, environmental science, and energy.16 For instance, nanomachines can be used for cargo delivery,17 biosensing in motion,18 minimally invasive surgery19 and environmental remediation.20,21
Airborne particulates are conceptually particulate-based machinery that achieves take-off and propulsion through various means such as chemical burning reactions,22 electrostatic charging,23 and wind power.24 Classical static techniques (e.g., high-efficiency particulate air (HEPA) filter) have been used to remove particulates and create cleaner air.25 The integration of materials, energy, and control in the context of robotics and autonomous systems could be applied to airborne particulates.26 Subsequently, the principles of dynamic nanomachines, such as electro-charged water droplets generated through electrospray atomization,27 electronetting using electrically charged droplets,28 and monodisperse charged droplets via an electrohydrodynamic device,29 can be applied to enhance the removal of PM. Air cleaning systems primarily use filtration, UV light, and ionization.30 While practical, these methods may not entirely remove ultrafine particulates less than 0.1 μm, have limitations in energy efficiency and can produce toxic byproducts. By applying nano-micromachines with incorporated catalytic segments, methods of control using magnetic fields, light, thermophoresis, electric fields, ionization and ultrasound could lead to a novel approach to enhance the effectiveness of air cleaning systems.
This review discusses the capability to control the motion, interactions and filtration of airborne particulates by deepening our understanding of the dynamic properties of nano- and micromotors, machines and robots, which can be designed to selectively capture, neutralize, and decompose harmful pollutants in the air. In this mini-review, an intersection between the established area of liquid nanomachines and the emergent challenges of airborne particulates is rigorously examined. Initially, advancements in the autonomous and controlled motion techniques of nanomotors in fluidic environments are elucidated. Subsequently, attention is directed towards airborne particulates, with a discussion of the existing challenges and potential solutions for their effective removal. An interdisciplinary approach is then employed to compare the capabilities and limitations of nanomachines in both liquid and aerial environments. In the concluding remarks, the potential of nanomachines as a promising approach for addressing the issue of harmful airborne particulates is substantiated. The overarching aim is to catalyze further scholarly inquiry and technological innovation in this critical area of airborne dynamic nanosystems and environmental science. The purpose of this review is dual-faceted: (i) to investigate the prospective utility of nanomachines in the selective capture and removal of airborne pollutants, guided by the principles of nanoscale engineering and materials science, and (ii) to critically assess how existing research in nanomachine technology, particularly the principles of nanoscale fluid dynamics and electrostatic interactions, can be extrapolated to enhance atmospheric quality. The review's purview extends beyond mere deployment of nanomachines for direct sequestration and relocation of airborne particulates. It also interrogates the translational applicability of fundamental operating mechanisms—initially conceived for nanoscale systems in fluidic environments—toward air purification strategies. In doing so, this review operates both as a comprehensive assessment of the prospective roles that nanomachines could play in advancing air quality amelioration methodologies and as a rigorous analytical critique of how extant knowledge in nanomachine science can foster innovation in alternative air purification technologies. Fig. 1a–b are dedicated to the elucidation of the pressing issues associated with air pollution in megacities. A comprehensive overview is provided, illustrating the genesis and propagation of airborne particulates characterized by a broad size distribution and complex chemical composition.
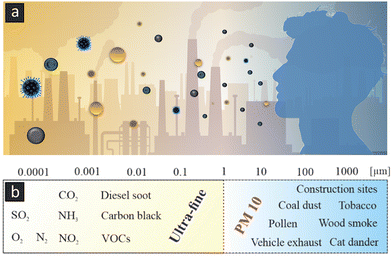 |
| Fig. 1 (a) Three-dimensional expression of a megacity with clearly occurring air contamination resulting from consequences of the industrial revolution. (b) Graphical image showing human exposure to particulates lead to significant health risks. (c) Typical size distribution and examples of PM and ultra-fine particulates.31 Images (a and b) were generated using the text-to-image AI tool Midjourney. | |
2. Nanomotors, machines and robots in liquids
2.1. Nanomotors’ autonomous motion in liquids
The fundamental principle underlying the autonomous movement of nano- and micromotors is converting chemical to mechanical energy. It can be achieved by designing the motors to catalyse specific chemical reactions, generating gaseous, proton, or ionic fluxes, which propel the nanomachines.20 In fluidic environments, the performance of these micromotors is quickly diminished due to viscous forces, resulting in a marked decrease in the Reynolds number, a dimensionless quantity that measures the ratio of inertial to viscous forces.32,33 In fluidic settings, viscous forces can indeed be a limiting factor, affecting the Reynolds number and, consequently, the operational efficiency of nanomotors. However, in gaseous environments like air, these viscous forces are significantly reduced, allowing for a higher Reynolds number and, therefore, more dynamic and rapid movements. This enables nanomotors to cover larger distances in shorter time frames, making them particularly well suited for tasks such as capturing and transporting airborne particulates. The lower resistance in air also opens up the possibility for more complex maneuvers and trajectories, which can be crucial for targeted applications such as the removal of airborne pathogens. Thus, the nanomotors’ adaptability to air-based operation is not merely a theoretical proposition but a practical advantage substantiated by the fundamental differences in fluid dynamics between air and liquid media. Self-propelled nano-/micromachines have been designed in various shapes, such as tubes, wires, rods, and spheres powered by catalytic reactions.34–37 Notable examples of nano-/micromotor mechanisms of motion include self-generated motive forces (self-electrophoresis, self-diffusiophoresis, dynamic surface tension, gas recoil), shown in Fig. 2(a–d). Self-electrophoresis, for instance, involves the asymmetric catalytic decomposition of a fuel molecule, leading to a non-uniform distribution of ions around the motor.38 This ion gradient creates an electric field, which propels the motor. This propulsion mechanism has been widely used in the design of bimetallic rod-shaped motors. On the other hand, self-diffusiophoresis exploits the concentration gradient of a solute around the motor for propulsion.39 This has been the basis of the design of Janus sphere motors, where one hemisphere of the sphere is coated with a catalyst that triggers a reaction to produce a solute.16 Bubble recoil has been employed in catalytic tubular microjets. Here, the motor catalyzes a reaction to produce gas bubbles.40 The recoil or ejection of microbubbles from the open end of the tube provides the thrust needed for propulsion. This unique mechanism has been shown to enable high speeds due to the efficient confinement of reaction–diffusion processes in large aspect ratio microtubes, which create favorable conditions for bubbles’ generation.
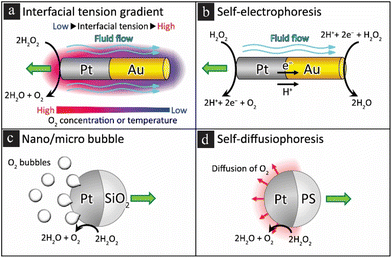 |
| Fig. 2 Propulsion mechanisms of nanorod and Janus particle-based catalytic nanomotors. (a) Proposed interfacial tension gradient and (b) self-electrophoresis-driven Pt nanomotors. (c) Microbubble-driven and (d) self-diffusiophoresis mechanisms of motion. Figure is reproduced from ref. 41 under the terms of the Creative Commons Attribution 4.0 International License (https://creativecommons.org/licenses/by/4.0). | |
2.2. Nanomotors’ motion control in liquids
The control of nano- and micromotor motion in liquid environments is a promising area of research with potential applications in various fields. In addition to chemical propulsion, nano- and micromotors can be controlled and powered using external fields such as magnetic, optical, electrical, electrochemical, and ultrasound fields.42 In addition, external stimuli can modulate the direction, speed and behavior of the devices.43,44 For instance, magnetic fields have been used to manipulate the motion of microtubes, offering the potential to perform tasks.45 Considering the effects of viscous force, thermal fluctuation, and symmetry breaking, nanomotors can achieve directional control by chemotaxis, and confined space/geometry,41 for example, electric-driven micro/nanomotors with a defective shape, i.e., golden micro/nanomotors and the longitudinally asymmetric defective golden micro/nanomotors (LA-DGMs), which exhibited controllable motion behaviors under different frequencies of an AC electric field. Nanomotors switch their direction of motion by changing the frequency of the AC field.46 Different mechanisms of motion control of nano–micromotors in liquids, including motion control using particles’ geometry, photocatalytic reactions, chemotaxis, thermophoresis, electrochemistry, electric field, ultrasound and magnetic field, are shown in Fig. 3(a–h).
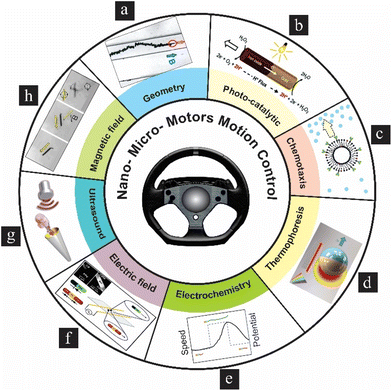 |
| Fig. 3 Various methods of nano–micromotors control in liquids: (a) geometric confinement of a microengine. Reproduced from ref. 47 with permission from American Chemical Society, copyright 2011. (b) Photocatalytic motion. Reproduced from ref. 48 with permission from the Royal Society of Chemistry, copyright 2017. (c) Chemotactic navigation. Reproduced from ref. 49 with permission from Nature Publishing Group, copyright 2019. (d) Thermophoresis-driven motion. Reproduced from ref. 50 with permission from the De Gruyter, copyright 2018. (e) Electrochemical control of nanomotor speed. Reproduced from ref. 11 with permission from the Royal Society of Chemistry, copyright 2009. (f) Electric field-driven motion. Reproduced from ref. 12 with permission from the Royal Society of Chemistry, copyright 2010. (g) Ultrasound-powered propulsion. Reproduced from ref. 51 with permission from the Wiley-VCH, copyright 2012. (h) Magnetic field-guided motion of a catalytic microengine. Reproduced from ref. 40 with permission from the Wiley-VCH, copyright 2009. | |
The chemical propulsion method uses chemical reactions to generate propulsion. For example, catalytic nanomotors can decompose hydrogen peroxide into water and oxygen,52,53 and the resulting gas bubbles propel the nanomotor, as depicted in Fig. 3(a). Photocatalytic nanomotors can be powered and controlled using specific wavelengths and intensities of light. In addition, phototactic nanomotors can move towards or away from the light source depending on their design, as illustrated in Fig. 3(b). Some nanomotors can move in response to a chemical gradient, a non-biological chemotaxis phenomenon, as indicated in Fig. 3(c). Nanomotors self-propel towards regions of higher concentration of a particular substance. It is beneficial for nanomotors used in biological environments, which can be guided toward specific cells or tissues by a chemical gradient. Temperature gradients can be used to control the motion of nanomotors. Nanomotors move from hot to cold regions in a process known as thermophoresis, as represented in Fig. 3(d). Applying an external electrochemical potential across a solution containing nanomotors can create an electric field, influencing the motion of charged nanomotors, as shown in Fig. 3(e). The speed and direction of the nanomotors can be controlled by adjusting the magnitude and polarity of the applied voltage. Even if the nanomotors are not charged, they may still respond to an oscillating electric field if polar. By oscillating the electric field, the nanomotors can be driven to move or rotate, depending on their shape and the properties of the electric field. The direction, speed, and nature of the motion (rotational vs. translational) can be controlled by adjusting the frequency, amplitude, and direction of the oscillating electric field, as indicated in Fig. 3(f). When an ultrasound wave travels through a fluid medium where nanomotors are suspended, it generates regions of compression and rarefaction due to the alternating high and low-pressure phases of the sound wave. These pressure variations can create “acoustic radiation forces”. When these forces act on nanomotors, they can induce both translational and rotational motion, as illustrated in Fig. 3(g). Nanomotors made of or coated with magnetic materials can be controlled using an external magnetic field (B). It allows for precise control of their motion and direction. Sequences of optical micrographs indicating magnetic control of the directionality of motion of a self-propelled tubular microjet engine are shown in Fig. 3(h). Each of the discussed methods has its advantages and limitations, and the choice of control method depends on the specific application and environment of the nanomotor.
2.3. Tasks enabled by nano–micromotors in fluids
Understanding the principles of motion at the nanoscale is crucial for harnessing the potential of nano- and micromotors in fluids, leading to various applications. In targeted drug delivery, for instance, a deep understanding of nanomotor motion under different conditions can facilitate the design of systems capable of maneuvering through complex environments. Similarly, in environmental remediation, understanding the mechanics of nanomotor movement can aid in developing systems that effectively clean polluted water or air, capturing and neutralizing contaminants. Moreover, in advanced manufacturing, knowledge of nanoscale motion can facilitate the design of nanomotors that precisely assemble nanostructures, potentially creating new materials with customized properties. Therefore, the intersection of nanotechnology and fluid dynamics, and an in-depth understanding of nanomotor motion, opens up many opportunities to address complex challenges in medicine, environmental science, and materials science. For instance, integrating photocatalytic materials, such as TiO2, has shown potential in accelerating nanomotor movement and enhancing pollutant degradation.54 The motion of Pt/AC micromotors enhances the diffusion-limited adsorption process and reduces the adsorption time threefold compared to static conditions.55 Nanomotors have evolved with intelligent designs and varied propulsion techniques, encompassing chemical, external field, enzymatic, and bio-hybrid systems. These advancements allow them to adapt and function in a range of biological settings, such as blood, tumors, eyes, and mucus.56 The hetero-silica-based micro/nanomotors have been used for drug delivery, cancer therapy, bioimaging, diagnosis, and nanosurgery.57 The advantages of nanomachines over conventional passive drug delivery systems include enhanced efficiency, precision, targeting and controllability, while limitations include biocompatibility, and toxicity, regulation and ethical issues.58 In other studies, MagRobots have demonstrated diverse propulsion techniques. These include corkscrew-like movements, traveling-wave locomotion, surface-assisted movements, and other forms of magnetic stimulation. These advanced propulsion methods have been employed in a range of applications, from targeted drug and gene delivery to cell manipulation. They've also been used in minimally invasive surgeries, biopsies, disrupting and eradicating biofilms, guided imaging for delivery, therapy, and surgery, as well as in pollution removal and sensing.59 In other examples, nanomachines containing a redox-sensitive gate (S-S-PEG) have been used for loading and releasing a dye (Ru(bpy)3Cl2) or a drug (doxorubicin) in the presence of glutathione (GSH), a reducing agent found in cells.60 The functions of biomedical micro/nanomotors include improving tissue permeability, promoting cellular uptake, loading and delivering cargo, and providing imaging contrast.
3. Particulates’ motion in the air
3.1. Particulates’ autonomous motion in the air
Aerosols are collections of solid or liquid particles suspended in a gas that significantly affect air quality and health. Airborne particulates suspended in the air comprise various substances such as dust, soot, pollen, mold spores, bacteria, viruses, cement, ash, smog, smoke, and liquid droplets.61–64 Typical smog consists of particulate matter (PM), ozone (O3), nitrogen dioxide (NO2), sulfur dioxide (SO2), carbon monoxide (CO), and volatile organic compounds (VOCs).65 Autonomous motion of airborne particulates is influenced by particles’ initial velocity, the surrounding air's turbulence, the particle's size, shape, and mass, and external forces such as gravity and electrostatic forces. The Brownian motion of nanoparticles, due to the collision with gas molecules, plays a significant role in their transport. Brownian motion is defined as the stochastic, erratic movement of particles, induced by thermal fluctuations and mediated through collisions with surrounding fluid molecules, whether in gaseous or liquid states.66 It strongly influences small particles with a diameter below 1 μm, affecting their diffusion and deposition.67 Additionally, particulates powered by chemical decomposition or explosive reactions, such as fireworks68 and atmospheric molecular reactions,69 can gain initial an motive force and speed. PM is categorized based on its size into three distinct modes: ultrafine particles, which have a diameter less than 0.1 μm; accumulating particles, which range in size from 0.1 to 2 μm; and coarse particles, which have a diameter larger than 2 μm.70 The many modes exhibit distinctions in terms of their origins, composition, and dynamic characteristics. The material-balance principle offers a systematic approach for establishing a quantitative connection between indoor particle concentrations and many elements that influence them, including emissions, ventilation, filtration, deposition, penetration, and transformation processes.71 The relative importance of these factors depends on the particle size and the indoor environment characteristics. For example, cigarette smoking and outdoor air are major sources of fine particles, while cooking and cleaning generate ultrafine and coarse particles.72 Filtration and penetration exhibit greater efficacy in removing coarse particles compared to fine particles, whereas deposition and coagulation play a more significant role in the removal of ultrafine particles when compared to other mechanisms. There are several supplementary dynamic processes that impact the amounts of particles indoors, including mixing, interzonal transit, resuspension, and phase shift.73
3.2. Particulates’ motion control in the air
Several external fields can be used to control the motion of particulates in the air. The gravitational field is the primary field that affects all particulates in the air. It constantly pulls particulates downwards, eventually causing them to fall to the ground.74 Electromagnetic fields charge particles that can be polarized and subsequently controlled using electric or magnetic fields. The magnitude and direction of these fields can be manipulated to control the trajectory and speed of the particles.75 Thermal fields or temperature changes create convective currents in the air that can carry particulates. The hotter air rises while cooler air sinks, creating motion that carries particulates.76 Overall, catalytic and combustion reactions can affect the velocity of Brownian particulates in the air. However, the exact speeds and distances traveled by such particulates depend on various factors, including particle size, medium viscosity, and the number of collisions with surrounding molecules.77 Combustion reactions can also affect the fluctuating velocity of particles, which increases with the combustion temperature. The speed of particulates in combustion reactions is influenced by factors such as the concentration of reactants, temperature, and surface area of reactants.78 Ionization refers to the process by which an atom or a molecule acquires a negative or positive charge by gaining or losing electrons. When particulates are ionized, they become charged and can be influenced by electric or magnetic fields.79 Moreover, ionized particles tend to attract or repel each other based on their charges, which can affect their motion and collective behaviors. By carefully controlling the ionization of particulates and the application of electric fields, it is possible to achieve a significant degree of control over the motion of particulates in the air. Controllable ionization of particulates can be achieved using corona discharge, ultraviolet radiation and radioactive materials.80 By applying an external electric field, the charged particles experience a force proportional to the strength of the field and the amount of their charge. If the field is static (not changing over time), particles move along the field lines until they reach a point where the field strength is zero or until they hit an obstacle. Subsequently, multiple electrodes can be assembled to create more complex electric field patterns, allowing more precise control over particle movements.
3.3. Air-filtration systems and approaches
Wet scavenging is one of the most efficient processes for removing aerosols from the atmosphere. Fig. 4(a) shows the main principles of in-cloud scavenging, where particles are activated as cloud condensation nuclei and absorbed by cloud water, and below-cloud scavenging. Subsequently, raindrops or snow particles capture aerosols and gas after the hydrometeors leave the clouds. A study conducted experiments to assess the mass scavenging efficiencies (MSEff) and probable factors that may affect the in-cloud and below-cloud scavenging of black carbon (BC). The research revealed that mean square errors (MSErr) of BC tend to be smaller in places at lower altitudes and increase as altitude rises. This finding provides further support for the significant influence of atmospheric aging on the chemical alteration of BC. Fig. 4(b) describes two main types of wet collectors (scrubbers) for particulate removal in gas streams: one using liquid sprays (e.g., spray towers, venturi scrubbers), and another using wetted surfaces (e.g., packed towers). A high-gravity (HiGee) reactor, which utilizes both types, has been demonstrated. Due to shear stress, the reactor produces thin liquid films and tiny droplets. The HiGee system leverages three collection mechanisms: diffusion, interception, and inertial impaction. Large particles are primarily collected via impaction and interception, and small particles are through diffusion. In addition, using charged droplets could enhance particulate attraction, facilitating more effective air cleansing. Understanding of nanoparticle motion control and several techniques can be used for air purification, including physical filters, ionizers, electrostatic and magnetic effects, humidity, and photocatalytic methods.83 Most particles are composed of organic matter, elemental carbon, sulfate and nitrate, i.e., which do not contain any “magic” property for an effective separation using external fields.84 Filtration systems, such as HEPA filters, utilize dense fibrous media to capture particles based on size and mechanical interception. The efficiency of filtration is influenced by factors such as filter material, pore size, and airflow rate.25 Electrostatic precipitation relies on applying high-voltage electrostatic fields to induce an electrostatic charge on the particles, causing them to be attracted to and deposited onto collection surfaces.75 Air ionization techniques also utilize ion generators or ionizers to produce negative ions that attach to airborne particulates, causing them to agglomerate and settle out of the air.85 The study suggested that ozone-emitting air purifiers should be avoided in indoor environments with elevated concentrations of unsaturated VOCs, and that reducing the ozone emission rate and increasing the airflow through the air purifier are essential factors for achieving the maximum possible reduction in the indoor particle level.86 Using another approach, photocatalysts, such as LiNbO3 and TiO2, can be used for air purification, which can degrade pollutants such as volatile organic compounds (VOCs) and inorganic oxides by using light energy.87 Furthermore, chemical reactions with specific sorbents can facilitate capturing and removing toxic substances and heavy metals. Metal–organic frameworks (MOFs) are promising materials for air purification due to their high porosity, tailorability, and thermal stability for removing various chemical hazards, such as chemical warfare agents, carbon monoxide, ammonia, sulfur dioxide, and others.88 Additionally, acoustic filtration using ultrasound can agglomerate micronic and sub-micron particle matter, increasing their size and making them easier to collect using conventional methods.89 Hybrid systems that incorporate multiple filtration stages, such as cyclone separators, electrostatic precipitators, and activated carbon filters, are used to maximize removal efficiency and address different particle sizes and characteristics. Fig. 5(a) describes the preparation of a ZIF-8@PDA@PDMS composite sponge using a sacrificial template and in situ growth methods. The sponge is designed for efficient and sustainable filtering of PM under harsh environments such as high temperature and humidity. The composite sponge displays excellent PM filtration performance due to the high specific surface area and porous network structure of the PDMS sponge, as well as the large number of metal sites of ZIF-8. The removal efficiency of PM2.5 or PM10 is greater than 99.8% and the sponge filter has long-term filtration stability, still achieving excellent performance after 65 hours of filtration. The composite sponge can adapt to harsh environments such as high temperature (250 °C) and high humidity (90% RH). The composite sponge filter is also regular shaped and can be customized to any shape as required.90Fig. 5(b) illustrates a novel electrostatic precipitator including dielectric coatings applied to the repelling electrodes, which enhances the efficacy of sub-micron particle removal.91 The study investigated four parameters that have an impact on performance: the voltage differential (Vc) and distance (dc) between the repelling and collecting electrodes, as well as the dielectric material and its thickness (de). The optimal performance of the novel ESP was observed when it was coated with a 60 μm thick layer of PET at a distance of 3 mm from the direct current (dc) source. The utilized breakdown voltage ranged from −4 kV (in the absence of a dielectric film) to −9 kV, resulting in an improvement in PM removal efficacy from 60% to 92%. The electrostatic precipitator (ESP) exhibited a relatively modest level of net ozone formation, ranging from 3 to 5 parts per billion (ppb). Additionally, it demonstrated a low-pressure drop, measuring in the range of 10–13 Pa, and a low energy consumption of 18 W m−2. These characteristics collectively contribute to the environmentally benign nature of the ESP.
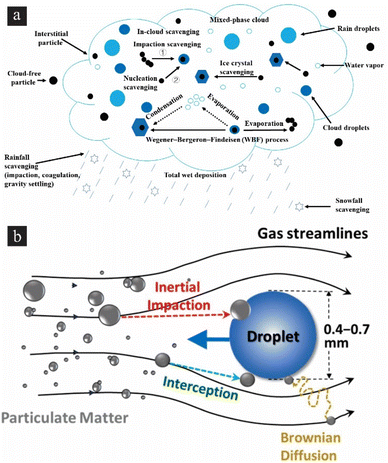 |
| Fig. 4 Particulates’ motion control in the air. (a) Main processes of wet scavenging of black carbon. Reproduced from ref. 81 under the terms of the Creative Commons Attribution License. (b) Schematic illustration of filtration mechanisms. Reproduced from ref. 82 with permission from Elsevier, copyright 2017. | |
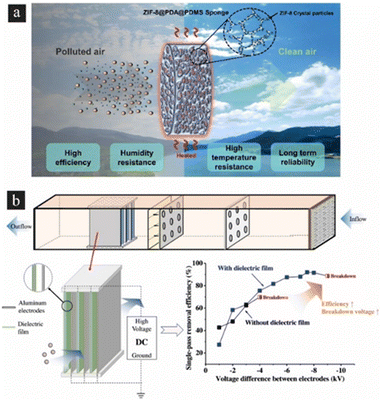 |
| Fig. 5 Air filtration materials using the (a) ZIF-8@PDA@PDMS composite sponge used under high temperature and humidity conditions. Reproduced from ref. 90 with permission from Elsevier, copyright 2023. (b) New electrostatic precipitator with dielectric coatings to efficiently remove sub-micrometer particles in the building environment. Reproduced from ref. 91 with permission from Elsevier, copyright 2020. | |
4. Interdisciplinary approach
4.1. Capture and removal of harmful particulates
Classically, fibrous filters can be applied for different slip and no-slip air flow cleaning regimes.92 Air filtration in the free molecular flow (FMF) regime is essential and challenging because it can achieve higher filtration efficiency and lower the pressure drop than conventional filters. Carbon nanotubes (CNTs) are ideal for air filters in the FMF regime because of their nanoscale diameters and high surface area. Different structural models of HEPA filters based on CNTs have been introduced, such as CNT films, CNT filters with gradient or hierarchical nanostructures, and CNT fluidized bed air filters. Theoretical and experimental studies of air filtration in the FMF regime are reviewed, focusing on the relationship between structure, pressure drop, and filtration efficiency.93 The diffusion characteristics of industrial submicron aerosol particles in indoor air using Computational Fluid Dynamics (CFD) and Discrete Particle Modelling (DPM) were studied. It was found that the main acting forces on submicron particles are drag force, Brownian force, lift force, and thermophoresis force. A recent study indicated that Brownian force should be considered when the particle diameter is below 0.3 mm, as it enhances their diffusion ability. It was shown that the smaller the particle size, the higher the heat source temperature, and the lower the particles’ initial velocity, leading to the increase of horizontal dispersity.94 Charged droplets operating as nanomotors (generated by voltage discharge) can help remove smog particulates from the air by increasing the efficiency of particle removal compared to uncharged aerosols. Water droplets and particles can exhibit various collisions and capture behaviors, depending on the particle properties (density, size, wettability) and the impact parameters (velocity, location). Multiple capture methods have been reported, such as surface capture, cavity-forming entry, skid entry, and escape.95
4.2. Air detoxification using catalytic nanomachines
Catalytic reactions and catalyst-embedded droplets or particles can effectively purify and detoxify the air, in indoor settings, industrial contexts, or broader environmental scales. The nanomotors can be designed to adsorb harmful substances onto their surfaces using an adsorption mechanism. In addition, the nanomotors can be loaded with chemicals that neutralize harmful substances or deliver neutralizing agents into the environment. The nanomotors can be guided to release these chemicals at the contamination sites.96 For instance, nanomotors containing catalytic segments such as platinum, palladium, and rhodium can be used to facilitate reactions that convert harmful gases like carbon monoxide (CO), nitrogen oxides (NOx), and unburnt hydrocarbons (HCs) into less harmful substances such as carbon dioxide (CO2), nitrogen (N2), and water (H2O). A liquid or solid catalyst can be introduced into the air as fine droplets or particles. These droplets or particles can react with harmful gases in the air, converting them into less harmful substances. This is particularly effective at removing sulfur dioxide (SO2) and nitrogen oxides (NOx) from flue gases in power plants and other industrial settings. For example, photocatalytic nanomaterials, such as titanium dioxide (TiO2), can degrade a wide range of organic pollutants in the presence of light.54 Nanomaterials can also be used to catalyze reactions that neutralize harmful gases like carbon monoxide or volatile organic compounds.
4.3. Application of external fields to remove particulates
High-frequency sound waves can be used to agitate airborne particulates, causing them to coalesce and fall out of the air. This approach is similar to ultrasound-propelled nanomotors in a liquid medium.10 If the airborne particulates are made of or coated with a magnetic material, a magnetic field could be used to control their motion. An oscillating electric field could trap and control the motion of charged airborne particulates, similar to how it is used to control the motion of nanomotors.46 Unlike in fluids,11 electrochemical potentials cannot be used for particulates motion control and air purification. In fluidic environments, electrochemical potentials serve as an effective means to control the motion of particles, including nanomachines. This is largely due to the presence of ions in the fluid, which interact with the electrochemical gradients to produce forces that can be harnessed for motion control. However, this mechanism is not directly translatable to air for several reasons. Air, being a gaseous medium, lacks the ionic constituents that are essential for electrochemical interactions. In fluids, charged particles move in response to an electric field, but in air, the absence of a continuous ionic medium makes it challenging to establish a stable electrochemical gradient for particle control. Even if ionization is used to create a medium for electrochemical interactions, the energy required to maintain such a state would be impractically high. This makes the use of electrochemical potentials in air both inefficient and unsustainable for long-term applications. Given these challenges, application of electrochemical potentials is not a viable method for controlling particulate motion in air. However, photoelectrochemical oxidation (PECO) has been recently demonstrated for air purification.97 PECO is an innovative air purification process that uses light to activate a catalyst, which then oxidizes and destroys pollutants and organic matter as small as 0.1 nm, which cannot be removed by conventional filters. Using another approach, thermophoresis effects have been shown to be responsible for dust accumulation on solar panels, where the temperature gradient between the solar panel and the ambient air influences the transport and deposition of airborne dust particles. Thermophoretic force, which drives particles from hot to cold regions, can reduce the dust accumulation of sub-micrometer-size particles on the solar panel whose temperature is a few tens of degrees higher than the ambient air in the daytime.98 A numerical study of airborne particle dynamics in vortices near curved electrode surfaces has been performed, combining effects of aerodynamic and electrostatic forces. The direction and strength of air circulation and electric fields can influence particle capture, deposition, and flight manipulation differently. It was found that co-vortices improve the electrostatic capture of particles, while counter-vortices deteriorate it or trap particles in the air. Depending on their size, inertia, and dielectrophoretic force, particles can exhibit different trajectories, such as vortex trapping, inertial limit cycles, spillage, and inverse cyclonic separation.99
4.4. Comparison of nanomachines in the air and liquid
There are some similarities and differences of motive forces of nanomachines in the air and liquid. Several essential characteristics can lead to a better understanding of particles in the air and liquid, as summarized in Table 1. Both gliding and coasting leverage the kinetic energy accumulated in the vehicle's mass, essentially its inertia, to maintain motion. However, this energy gradually dissipates due to opposing forces like air drag, rolling resistance, and gravity. Accepting a spherical size of the nanomotor, the air drag force equals F = 6πμrpv, where v is the nanomotor velocity, μ is the medium viscosity and rp is the radius of machine. Subsequently, the rate at which the nanomotor would stop equals a = F/m, where m is the nanomotor mass.100 The deceleration can be used to calculate the drifting time (t) and distance (d) using the equation d = vt − 0.5(at)2. For instance, if the motive force is no longer applied, the nanomachine drifts for a longer time and distance in the air than water, due to the lower viscosity of air. However, even in the air, the drifting distance can be in the order of nanometers. This highlights the negligible inertia of nanoparticles, which allows them to stop almost instantaneously when the motive force is removed. The mean square displacement of a nanomachine due to Brownian motion depends on the diffusion coefficient (D) and (t) the time 〈x2〉 = 2Dt. For example, for a 10 nm nanomachine propelling in water by diffusion during 1 second (D = 2.5 × 10−10 m2 s−1), the root-mean-square displacement equals
. Accepting D = 5 × 10−6 m2 s−1, the root-mean-square displacement in the air equals
. It demonstrates that nanomachines in the air have significant dynamic behaviors. Furthermore, both nanomachines in the air and liquid experience buoyancy. Both air and liquid create drag forces on moving particles, which opposes the motion of the nanomachine and depends on the object's speed, size, shape, viscosity and density of the fluid. Gravity is a significant motive force for particulates in the air, but it is less critical for nanomachines in fluids. Surface tension force is generally more relevant in liquids. Interfacial forces are typically less relevant in the air due to the lower density and intermolecular forces. Chemical gradients, self-electrophoresis, self-diffusiophoresis, bubble recoil, and surface tension are specific to nanomotors in liquids and are irrelevant to air propulsion. Bubble recoil (liquid) is relevant to power nanomotors in liquids. It involves the recoil or ejection of gaseous microbubbles, and drives motion in the opposite direction (according to Newton's third law). Since air is less dense than liquid, an equivalent force in the air requires the creation of “steam” at much higher fuel decomposition rates and temperature, resulting in high-speed propulsion of man-made rockets and jet engines. Photochemical reactions involve the absorption of light, which can also lead to chemical changes in the particles and potentially influence their motion. While the distinct similar motive mechanisms can drive particulates in the air and liquid, there are significant differences. It is noteworthy that motive forces can interact and depend on factors like the scale of the particles, the reaction rates, the strength of fields and the surrounding medium.
Table 1 Characteristics of nano- and micromachines in the air and liquid. Note: specific values are presented for the general consideration and does not cover all existing conditions
Characteristic |
Particulates in the air |
Nanomotors in a fluid |
Ref. |
Size range, nm |
0.4–100 |
10–1000 |
101 and 102 |
Max. absolute speed, m s−1 |
∼80 |
∼1 × 10−3 |
103 and 104 |
Max. relative speed, bl s−1 |
∼8 × 108 |
∼1 × 103 |
104 and 105 |
Medium viscosity, Pa s |
∼1.8 × 10−5 (air at 20 C) |
∼1 × 10−3 (water) |
106 and 107 |
Medium density, kg m−3 |
∼1.2 (air at 20 C) |
∼1000 (water) |
108 and 109 |
Mean square displacement (e.g. 10 nm particle, time, time 1 s), m |
∼3.2 × 10−3 |
∼7.1 × 10−6 |
110
|
Coasting, drifting distance (for 1 μm diameter motor moving at 30 μm s−1), m |
∼1 × 10−7 |
∼2 × 10−12 |
100
|
Motive force |
Gravity, buoyancy, air drag, chemical decomposition and photochemical reactions |
Chemical gradients, self-electrophoresis, self-diffusiophoresis, bubble recoil, buoyancy, surface tension, fluid drag |
9 and 111 |
Methods of external control |
Electric field, light, temperature, magnetic field, ultrasound, aerodynamic forces, mechanical forces, optical tweezers |
Electric field, light, temperature, magnetic field, ultrasound, electrochemical potential, chemical gradients, pH gradients, optical tweezers, flow fields |
43 and 111 |
5. Conclusions
For decades, “nanoparticle” has been an established term in nanoscience, but its breadth has limited its potential to excite the next generation of nanomachine enthusiasts. The emergence of man-made “nanomotors”, “nanomachines”, and “nanorobotics” has transformed static nanoparticle research, driving a transition from passive to active and potentially intelligent nanoscale systems. This linguistic and conceptual shift has successfully created nanoparticle motion control systems, which have applications in domains such as targeted drug delivery and environmental remediation. A similar shift in perspective could stimulate advances in controlling airborne particulates as humanity faces expanding air pollution. It is projected that by adopting the terminology “nanomotors, machines, and robots”, studies on not only monitoring airborne particles but also innovative mitigation approaches could be established. While extensive studies have been conducted on the adverse health effects of air particles and the advantages of air cleaning systems, a research gap remains in implementing engineered nano-micromachines for controlling particulates. Controlling nano-micromachines using catalytic processes, magnetic fields, light, thermophoresis, electric fields, electrochemical potential, and ultrasound might give novel approaches for catching and neutralizing air particles. Conventional air filtration technologies are inherently static, exhibiting limited efficacy in spatiotemporally heterogeneous environments due to their localized mode of operation. These systems may be proficient at filtering air passing through a designated volume, yet they fall short in mitigating pollutants that bypass the filtration network, entering habitable zones via alternate ingress points such as fenestrations or door apertures. It is in these challenging environments that the dynamic, adaptive nature of nanoscale machines becomes particularly advantageous for air purification. Specifically, liquid-phase nanomachines—when appropriately engineered for airborne applications—can disseminate rapidly throughout a volumetric airspace, utilizing real-time sensing and feedback mechanisms to identify, target, and sequester airborne contaminants. Such dynamic functionality equips airborne nanomachines with a superior capacity for mitigating indoor air pollution, especially in settings where pollution sources are characterized by temporal and spatial variability. It can result in more efficient air cleaning systems and decrease the health hazards connected with air pollution. Nanomotors can travel through the air, capture particulates, and transport them to a collecting station. First and foremost, this new concept can be based on hazardous particle classification and then the optimization strategy for their liquidation by passive or active air micro-nanomachines. As a promising station for collection, classification, and mass measurements of airborne PM samples, lab-on-a-chip (LOC) devices have demonstrated the ability to perform airborne PM monitoring and detection using different operating principles with simple and low-cost devices with fast response times, and the capacity to become portable in identifying specific airborne PM components has recently shown remarkable progress.112 After determining the class and hazards of PM, the multidisciplinary methods and techniques in this review, and sampling strategies and analytical techniques for the assessment of airborne micro and nano plastics113 can be used for PM collecting and removal. Considering the practicality of employing nanomotors for air purification, several key parameters must be scrutinized. The time required for a single nanomotor to capture an airborne particle is contingent upon multiple factors, including particle dimensions, the velocity of the nanomotor, and the prevailing environmental conditions. To achieve a noticeable impact on air quality in a standard residential room, a swarm comprising a large number of nanomotors would be necessary. While the immediate application of this technology may appear most relevant for the capture of airborne pathogens, given their potential health impact, the inherent scalability and adaptability of nanomotors should not be underestimated. These attributes make nanomachines a promising technology for a wide range of air purification applications. In addition, nanomotors might be designed to selectively bind to specific surfaces of particulates. If the nanomotors can be controlled, they may be able to react to changes in the environment in real time. Some nanomotors might contain catalysts that convert hazardous contaminants into less harmful molecular species. In conclusion, it is crucial to emphasize that man-made flying nanomotors, machines, and robots remain primarily conceptual and have not yet been explored.
Author contributions
The manuscript was written through contributions of all authors. All authors have given approval to the final version of the manuscript.
Conflicts of interest
There are no conflicts to declare.
Acknowledgements
This work was supported by the National Key Technologies R&D Program of China (2021YFA0715302 and 2021YFE0191800), the National Natural Science Foundation of China (61975035 and 52150610489), and the Science and Technology Commission of Shanghai Municipality (22ZR1405000). Large language model GPT 4.0 was instrumental in refining the early versions of the manuscript. Text-to-image AI Midjourney software was instrumental in creating Fig. 1(a and b) visual representation of the main concept.
References
- A. L. Power, R. K. Tennant, A. G. Stewart, C. Gosden, A. T. Worsley, R. Jones and J. Love, Sci. Rep., 2023, 13, 8964 CrossRef CAS PubMed.
- E. Cheek, V. Guercio, C. Shrubsole and S. Dimitroulopoulou, Sci. Total Environ., 2021, 766, 142585 CrossRef CAS PubMed.
- B. Kherzi and M. Pumera, Nanoscale, 2016, 8, 17415–17421 RSC.
- W. Wang and T. E. Mallouk, ACS Nano, 2021, 15, 15446–15460 CrossRef CAS PubMed.
- X. Lyu, X. Liu, C. Zhou, S. Duan, P. Xu, J. Dai, X. Chen, Y. Peng, D. Cui and J. Tang, J. Am. Chem. Soc., 2021, 143, 12154–12164 CrossRef CAS PubMed.
- H. Zhu, S. Nawar, J. G. Werner, J. Liu, G. Huang, Y. Mei, D. A. Weitz and A. A. Solovev, J. Phys.: Condens. Matter, 2019, 31, 214004 CrossRef CAS PubMed.
- L. L. Adams, D. Lee, Y. Mei, D. A. Weitz and A. A. Solovev, Adv. Mater. Interfaces, 2020, 7, 1901583 CrossRef CAS.
- H. Ning, Y. Zhang, H. Zhu, A. Ingham, G. Huang, Y. Mei and A. A. Solovev, Micromachines, 2018, 9, 75 CrossRef PubMed.
- M. Liu and K. Zhao, Micromachines, 2021, 12, 687 CrossRef PubMed.
- Y. Zhu, Y. Liu, K. Khan, G. Arkin, A. K. Tareen, Z. Xie, T. He, L. Su, F. Guo, X. Lai, J. Xu and J. Zhang, Mater. Today Adv., 2023, 17, 100330 CrossRef CAS.
- P. Calvo-Marzal, K. M. Manesh, D. Kagan, S. Balasubramanian, M. Cardona, G.-U. Flechsig, J. Posner and J. Wang, Chem. Commun., 2009, 4509–4511 RSC.
- P. Calvo-Marzal, S. Sattayasamitsathit, S. Balasubramanian, J. R. Windmiller, C. Dao and J. Wang, Chem. Commun., 2010, 46, 1623–1624 RSC.
- S. Khoee, S. Moayeri and M. A. Charsooghi, Langmuir, 2021, 37, 10668–10682 CrossRef CAS PubMed.
- X. Li, Y. Zhao, D. Wang and X. Du, Colloids Surf., A, 2023, 658, 130712 CrossRef CAS.
- K. T. Krist, A. Sen and W. G. Noid, J. Chem. Phys., 2021, 155, 164902 CrossRef CAS PubMed.
-
Z. Yang, D. A. Aarts, Y. Chen, S. Jiang, P. Hammond, I. Kretzschmar, H.-J. Schneider, M. Shahinpoor, A. H. Muller and C. Xu, Janus particle synthesis, self-assembly and applications, Royal Society of Chemistry, 2012 Search PubMed.
- X. Xiong, X. Huang, Y. Liu, A. Feng, Z. Wang, X. Cheng, Z. He, S. Wang, J. Guo and X. Yan, Chem. Eng. J., 2022, 445, 136576 CrossRef CAS.
- Biocompatible micromotors for biosensing | SpringerLink, https://link.springer.com/article/10.1007/s00216-022-04287-x, (accessed July 23, 2023).
- W. Xi, A. A. Solovev, A. N. Ananth, D. H. Gracias, S. Sanchez and O. G. Schmidt, Nanoscale, 2013, 5, 1294–1297 RSC.
- M. Safdar, S. U. Khan and J. Jänis, Adv. Mater., 2018, 30, 1703660 CrossRef PubMed.
- X. Chang, Y. Feng, B. Guo, D. Zhou and L. Li, Nanoscale, 2022, 14, 219–238 RSC.
- S. Tomaz, J.-L. Jaffrezo, O. Favez, E. Perraudin, E. Villenave and A. Albinet, Atmos. Environ., 2017, 161, 144–154 CrossRef CAS.
- K. De Bruijne, S. Ebersviller, K. G. Sexton, S. Lake, D. Leith, R. Goodman, J. Jetters, G. W. Walters, M. Doyle-Eisele and R. Woodside, Inhalation Toxicol., 2009, 21, 91–101 CrossRef CAS PubMed.
- W. D. Hafner and R. A. Hites, Environ. Sci. Technol., 2005, 39, 7817–7825 CrossRef CAS PubMed.
- P. M. Bluyssen, M. Ortiz and D. Zhang, Build. Environ., 2021, 188, 107475 CrossRef PubMed.
-
S. M. Walsh, M. S. Strano and S. C. Stanton, Robot. Syst. Auton. Platf. Adv. Mater. Manuf., 2019, pp. 19–46 Search PubMed.
- J. Kim, J. J. Kim, S. Park, J. Kim and S. J. Lee, Atmos. Pollut. Res., 2023, 14, 101711 CrossRef CAS.
-
S. Zhang, H. Liu, N. Tang, J. Yu and B. Ding, in Electrospinning: Nanofabrication and Applications, Elsevier, 2019, pp. 249–282 Search PubMed.
- K. Lee, K. I. Lee, S. Y. Jeon and S. Kim, Adv. Powder Technol., 2019, 30, 190–198 CrossRef CAS.
- E. Tapia-Brito, J. Riffat, Y. Wang, Y. Wang, A. M. Ghaemmaghami, C. M. Coleman, M. T. Erdinç and S. Riffat, Build. Environ., 2023, 240, 110422 CrossRef PubMed.
- L. Jin, X. Luo, P. Fu and X. Li, Natl. Sci. Rev., 2017, 4, 593–610 CrossRef CAS.
- J. J. Abbott, K. E. Peyer, M. C. Lagomarsino, L. Zhang, L. Dong, I. K. Kaliakatsos and B. J. Nelson, Int. J. Robot. Res., 2009, 28, 1434–1447 CrossRef.
- D. Walker, M. Kubler, K. I. Morozov, P. Fischer and A. M. Leshansky, Nano Lett., 2015, 15, 4412–4416 CrossRef CAS PubMed.
- F. Mou, Y. Li, C. Chen, W. Li, Y. Yin, H. Ma and J. Guan, Small, 2015, 11, 2564–2570 CrossRef CAS PubMed.
- J. Li, Q. Xiao, J.-Z. Jiang, G.-N. Chen and J.-J. Sun, RSC Adv., 2014, 4, 27522–27525 RSC.
- S. Fournier-Bidoz, A. C. Arsenault, I. Manners and G. A. Ozin, Chem. Commun., 2005, 441–443 RSC.
- M. Safdar, T. D. Minh, N. Kinnunen and J. Jänis, ACS Appl. Mater. Interfaces, 2016, 8, 32624–32629 CrossRef CAS PubMed.
- S. Ebbens, D. A. Gregory, G. Dunderdale, J. R. Howse, Y. Ibrahim, T. B. Liverpool and R. Golestanian, Europhys. Lett., 2014, 106, 58003 CrossRef.
- W. F. Paxton, A. Sen and T. E. Mallouk, Chem. – Eur. J., 2005, 11, 6462–6470 CrossRef CAS PubMed.
- A. A. Solovev, Y. Mei, E. Bermúdez Ureña, G. Huang and O. G. Schmidt, Small, 2009, 5, 1688–1692 CrossRef CAS PubMed.
- D. Yamamoto and A. Shioi, KONA Powder Part. J., 2015, 32, 2–22 CrossRef.
- K. Xu and B. Liu, Beilstein J. Nanotechnol., 2021, 12, 756–765 CrossRef PubMed.
- S. Yu, Y. Cai, Z. Wu and Q. He, View, 2021, 2, 20200113 CrossRef.
-
T. Shabatina and V. Bochenkov, Smart Nanosystems for Biomedicine, Optoelectronics and Catalysis, BoD – Books on Demand, 2020 Search PubMed.
- T. Xu, W. Gao, L.-P. Xu, X. Zhang and S. Wang, Adv. Mater., 2017, 29, 1603250 CrossRef PubMed.
- R. Zhuang, D. Zhou, X. Chang, Y. Mo, G. Zhang and L. Li, Appl. Mater. Today, 2022, 26, 101314 CrossRef.
- S. Sanchez, A. A. Solovev, S. M. Harazim and O. G. Schmidt, J. Am. Chem. Soc., 2011, 133, 701–703 CrossRef CAS PubMed.
- D. Zhou, L. Ren, Y. C. Li, P. Xu, Y. Gao, G. Zhang, W. Wang, T. E. Mallouk and L. Li, Chem. Commun., 2017, 53, 11465–11468 RSC.
- A. Somasundar, S. Ghosh, F. Mohajerani, L. N. Massenburg, T. Yang, P. S. Cremer, D. Velegol and A. Sen, Nat. Nanotechnol., 2019, 14, 1129–1134 CrossRef CAS PubMed.
- Z. Zhan, F. Wei, J. Zheng, W. Yang, J. Luo and L. Yao, Nanotechnol. Rev., 2018, 7, 555–581 CrossRef CAS.
- D. Kagan, M. J. Benchimol, J. C. Claussen, E. Chuluun-Erdene, S. Esener and J. Wang, Angew. Chem., Int. Ed., 2012, 51, 7519–7522 CrossRef CAS PubMed.
- S. Naeem, F. Naeem, J. Liu, V. A. B. Quiñones, J. Zhang, L. He, G. Huang, A. A. Solovev and Y. Mei, Chem. – Asian J., 2019, 14, 2431–2434 CrossRef CAS PubMed.
- S. Naeem, F. Naeem, M. Manjare, F. Liao, V. A. Bolaños Quiñones, G. S. Huang, Y. Li, J. Zhang, A. A. Solovev and Y. F. Mei, Appl. Phys. Lett., 2019, 114, 033701 CrossRef.
- S. W. Verbruggen, J. Photochem. Photobiol., C, 2015, 24, 64–82 CrossRef CAS.
- C. M. Oral, M. Ussia and M. Pumera, J. Phys. Chem. C, 2021, 125, 18040–18045 CrossRef CAS.
- H. Choi, J. Yi, S. H. Cho and S. K. Hahn, Biomaterials, 2021, 279, 121201 CrossRef CAS PubMed.
- M. Yan, L. Xie, J. Tang, K. Liang, Y. Mei and B. Kong, Chem. Mater., 2021, 33, 3022–3046 CrossRef CAS.
- G. Tezel, S. S. Timur, F. Kuralay, R. N. Gürsoy, K. Ulubayram, L. Öner and H. Eroğlu, J. Drug Targeting, 2021, 29, 29–45 CrossRef CAS PubMed.
- H. Zhou, C. C. Mayorga-Martinez, S. Pané, L. Zhang and M. Pumera, Chem. Rev., 2021, 121, 4999–5041 CrossRef CAS PubMed.
- P. Díez, E. Lucena-Sánchez, A. Escudero, A. Llopis-Lorente, R. Villalonga and R. Martinez-Manez, ACS Nano, 2021, 15, 4467–4480 CrossRef PubMed.
- P. Luo, H.-G. Ni, L.-J. Bao, S.-M. Li and E. Y. Zeng, Environ. Sci. Technol., 2014, 48, 13793–13799 CrossRef CAS PubMed.
- Y. Pan, S. Tian, X. Li, Y. Sun, Y. Li, G. R. Wentworth and Y. Wang, Sci. Total Environ., 2015, 537, 9–22 CrossRef CAS PubMed.
- P. Smichowski and D. R. Gómez, Appl. Spectrosc. Rev., 2023, 1–27 CrossRef.
- I. Vouitsis, J. Portugal, A. Kontses, H. L. Karlsson, M. Faria, K. Elihn, A. T. Juárez-Facio, F. Amato, B. Piña and Z. Samaras, Atmos. Environ., 2023, 119698 CrossRef CAS.
- P. Schmitt-Kopplin, A. Gelencser, E. Dabek-Zlotorzynska, G. Kiss, N. Hertkorn, M. Harir, Y. Hong and I. Gebefügi, Anal. Chem., 2010, 82, 8017–8026 CrossRef CAS PubMed.
- F. de A. Lima, G. B. Medeiros, P. A. M. Chagas, M. L. Aguiar and V. G. Guerra, Powders, 2023, 2, 259–298 CrossRef.
-
A. Maynard and P. K. Hopke, in Handbook of Indoor Air Quality, Springer, 2022, pp. 247–274 Search PubMed.
- H. Wang, K. Kawamura and D. Shooter, Environ. Sci. Technol., 2006, 40, 5257–5262 CrossRef CAS PubMed.
- M. Shiraiwa, K. Selzle and U. Pöschl, Free Radic. Res., 2012, 46, 927–939 CrossRef CAS PubMed.
- A. I. Calvoa, C. Alvesa, A. Castrob, V. Pontc, A. M. Vicentea and R. Fraileb, Atmos. Res., 2012, 120–121 Search PubMed.
- I. Santiasih, J. Hermana and D. B. Supriadi, J. Appl. Environ. Biol. Sci., 2012, 2, 625–633 Search PubMed.
- G. Loupa, D. Karali and S. Rapsomanikis, Air Qual., Atmos. Health, 2019, 12, 1449–1457 CrossRef CAS.
- C. Liu, S. Shi, C. Weschler, B. Zhao and Y. Zhang, Aerosol Sci. Technol., 2013, 47, 125–136 CrossRef CAS.
- S. Murakami, S. Kato, S. Nagano and Y. Tanaka, ASHRAE Trans., 1992, 98, 82–97 Search PubMed.
- M. Sillanpää, M. D. Geller, H. C. Phuleria and C. Sioutas, J. Aerosol Sci., 2008, 39, 335–347 CrossRef.
- K. Ravindra, R. Sokhi and R. Van Grieken, Atmos. Environ., 2008, 42, 2895–2921 CrossRef CAS.
- D. Fino, S. Bensaid, M. Piumetti and N. Russo, Appl. Catal., A, 2016, 509, 75–96 CrossRef CAS.
- Y. Huang, H. Shen, H. Chen, R. Wang, Y. Zhang, S. Su, Y. Chen, N. Lin, S. Zhuo and Q. Zhong, Environ. Sci. Technol., 2014, 48, 13834–13843 CrossRef CAS PubMed.
- A. Hartmann and M. Kriegel, Results Eng., 2022, 100528 CrossRef CAS.
- E. Sysolyatina, A. Mukhachev, M. Yurova, M. Grushin, V. Karalnik, A. Petryakov, N. Trushkin, S. Ermolaeva and Y. Akishev, Plasma Processes Polym., 2014, 11, 315–334 CrossRef CAS.
- Y. Yang, Y. Fu, Q. Lin, F. Jiang, X. Lian, L. Li, Z. Wang, G. Zhang, X. Bi and X. Wang, Atmosphere, 2019, 10, 175 CrossRef CAS.
- S.-Y. Pan, P. Wang, Q. Chen, W. Jiang, Y.-H. Chu and P.-C. Chiang, J. Cleaner Prod., 2017, 149, 540–556 CrossRef CAS.
- M. Ogrizek, A. Kroflič and M. Šala, Trends Environ. Anal. Chem., 2022, 33, e00155 CrossRef CAS.
- E. S. Galvão, J. M. Santos, A. T. Lima, N. C. Reis Jr., M. T. D. Orlando and R. M. Stuetz, Chemosphere, 2018, 199, 546–568 CrossRef PubMed.
- D. Z. Bezabeh, H. A. Bamford, M. M. Schantz and S. A. Wise, Anal. Bioanal. Chem., 2003, 375, 381–388 CrossRef CAS PubMed.
- A. Alshawa, A. R. Russell and S. A. Nizkorodov, Environ. Sci. Technol., 2007, 41, 2498–2504 CrossRef CAS PubMed.
- R. K. Nath, M. F. M. Zain and M. Jamil, Renewable Sustainable Energy Rev., 2016, 62, 1184–1194 CrossRef CAS.
- J. B. DeCoste and G. W. Peterson, Chem. Rev., 2014, 114, 5695–5727 CrossRef CAS PubMed.
- H. Liu, Z. Li, H. Tan, F. Yang, P. Feng, Y. Du, M. Ren and M. Dong, Fuel, 2021, 287, 119549 CrossRef CAS.
- Y. Gou, Y. Hao, W. Cai, J. Huang and Y. Lai, Particuology, 2023, 76, 141–150 CrossRef CAS.
- J. Mo, E. Tian and J. Pan, Sustain. Cities Soc., 2020, 55, 102063 CrossRef.
- S. A. Hosseini and H. V. Tafreshi, Powder Technol., 2011, 212, 425–431 CrossRef CAS.
- P. Li, C. Wang, Y. Zhang and F. Wei, Small, 2014, 10, 4543–4561 CrossRef CAS PubMed.
- C. Li, H. Wang, C. W. Yu and D. Xie, Indoor Built Environ., 2022, 31, 17–30 CrossRef CAS.
- N. B. Speirs, J. L. Belden and A. M. Hellum, J. Fluid Mech., 2023, 958, A40 CrossRef CAS.
- W. Gao and J. Wang, ACS Nano, 2014, 8, 3170–3180 CrossRef CAS PubMed.
- N. G. Rao, A. Kumar, J. S. Wong, R. Shridhar and D. Y. Goswami, Allergy Rhinol., 2018, 9, 2152656718781609 Search PubMed.
- S. Oh, B. W. Figgis and S. Rashkeev, Sol. Energy, 2020, 211, 412–417 CrossRef.
- P. Srinivasula and R. M. Thaokar, J. Electrost., 2023, 122, 103781 CrossRef.
-
B. Rogers, J. Adams and S. Pennathur, Nanotechnology: understanding small systems, Crc Press, 2014 Search PubMed.
- S. F. I. Abdillah and Y.-F. Wang, Environ. Res., 2023, 218, 115061 CrossRef CAS PubMed.
-
A. A. Solovev, Nanomembranes Mater. Prop. Appl., 2022, pp. 253–285 Search PubMed.
- S. Sanchez, A. N. Ananth, V. M. Fomin, M. Viehrig and O. G. Schmidt, J. Am. Chem. Soc., 2011, 133, 14860–14863 CrossRef CAS PubMed.
- L. A. Twisdale Jr., J. Struct. Div., 1978, 104, 1611–1630 CrossRef.
- J. Li, I. Rozen and J. Wang, ACS Nano, 2016, 10, 5619–5634 CrossRef CAS PubMed.
-
A. K. Al_Omari, H. F. I. Saied and O. G. Avrunin, in Image Processing and Communications Challenges 3, Springer, 2011, pp. 303–310 Search PubMed.
- S. Sengupta, K. K. Dey, H. S. Muddana, T. Tabouillot, M. E. Ibele, P. J. Butler and A. Sen, J. Am. Chem. Soc., 2013, 135, 1406–1414 CrossRef CAS PubMed.
- S. Abdalla and L. Cavaleri, J. Geophys. Res.: Oceans, 2002, 107, 17–11 CrossRef.
- P. Dhar, S. Narendren, S. S. Gaur, S. Sharma, A. Kumar and V. Katiyar, Int. J. Biol. Macromol., 2020, 158, 1020–1036 CrossRef CAS PubMed.
- G. Dunderdale, S. Ebbens, P. Fairclough and J. Howse, Langmuir, 2012, 28, 10997–11006 CrossRef CAS PubMed.
- A. D. Fusi, Y. Li, A. Llopis-Lorente, T. Patiño, J. C. van Hest and L. K. Abdelmohsen, Angew. Chem., 2023, 135, e202214754 CrossRef.
- S. Ezrre, M. A. Reyna, C. Anguiano, R. L. Avitia and H. Márquez, Biosensors, 2022, 12, 191 CrossRef CAS PubMed.
- A. Azari, J. A. Vanoirbeek, F. Van Belleghem, B. Vleeschouwers, P. H. Hoet and M. Ghosh, Environ. Int., 2023, 107885 CrossRef CAS PubMed.
|
This journal is © The Royal Society of Chemistry 2023 |
Click here to see how this site uses Cookies. View our privacy policy here.