DOI:
10.1039/D4CC03163F
(Feature Article)
Chem. Commun., 2024,
60, 9692-9703
Supramolecular chemical biology: designed receptors and dynamic chemical systems
Received
27th June 2024
, Accepted 26th July 2024
First published on 27th July 2024
Abstract
Supramolecular chemistry focuses on the study of species joined by non-covalent interactions, and therefore on dynamic and relatively ill-defined structures. Despite being a well-developed field, it has to face important challenges when dealing with the selective recognition of biomolecules in highly competitive biomimetic media. However, supramolecular interactions reside at the core of chemical biology systems, since many processes in nature are governed by weak, non-covalent, strongly dynamic contacts. Therefore, there is a natural connection between these two research fields, which are not frequently related or share interests. In this feature article, I will highlight our most recent results in the molecular recognition of biologically relevant species, following different conceptual approaches from the most conventional design of elaborated receptors to the less popular dynamic combinatorial chemistry methodology. Selected illustrative examples from other groups will be also included. The discussion has been focused mainly on systems with potential biomedical applications.
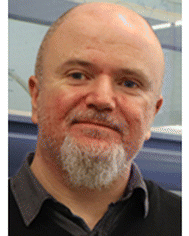
Ignacio Alfonso
| Ignacio Alfonso was initially trained as organic chemist at the University of Oviedo in Spain (PhD in 1999). He has worked in top-rated institutions in France (University of Strasbourg, short stay in 1997) and the USA (The Scripps Research Institute, postdoc in 2000–2002). After being selected for the Ramon y Cajal tenure-track contract in 2004 (Universitat Jaume I, Castellón), in 2007 he obtained a permanent position at IQAC-CSIC in Barcelona, where he created the Supramolecular Chemistry Group that he has led since then. His research interests are supramolecular chemistry, chemical biology, complex dynamic chemical systems and in general all aspects related to the molecular recognition of biorelevant species. |
Introduction
Supramolecular chemistry is a mature discipline that has found applications in different fields of science and technology, from materials science and energy to biomedicine.1 From its foundation, supramolecular chemistry has used nature as a source of inspiration since natural chemical species are often held by non-covalent contacts.2 To mention just some examples, we can recall the complementary base pairing of nucleic acids, the self-assembly of lipids in cell membranes and the specific recognition of proteins towards small molecules (enzyme substrates or cofactors) or other proteins (microtubules or protein–protein interaction regions). Thus, supramolecular concepts and approaches are also at the heart of chemical processes within a biological environment.3 On the other hand, in the last few decades, the study and modulation of biological processes with chemical tools have experienced an important development, as a way to improve their understanding at a molecular level.4 This knowledge paves the way towards more personalized and selective tools for both early diagnosis and efficient therapies.5 Accordingly, there is a close connection between the concepts and methodological approaches of supramolecular chemistry and chemical biology.6,7 By promoting parallel development in fundamental ideas and in experimental or theoretical approaches, both subdisciplines would mutually benefit and be improved.8 This feature article attempts to facilitate a dialogue between these subareas for common development in order to achieve some of the current goals in chemical biology by taking advantage of knowledge from supramolecular chemistry. As a payback, the main challenges in supramolecular chemistry could be faced by considering biomimetic benchmark systems, also leading to real-life applications in different areas related to healthcare and well-being.
Main challenges of supramolecular chemistry in biological systems
In spite of the important development of supramolecular chemistry since its early definition, challenges are still evident. Thus, for the molecular recognition or self-assembly of non-covalent complexes, these must be desolvated in order to expose the corresponding and complementary binding epitopes (Fig. 1). This unavoidable need becomes a true hurdle in the case of biological environments. The main solvent in biology is water, a highly polar, H-bond donor and acceptor solvent, and accordingly very competitive for most polar non-covalent interactions.9 Thus, hydration energies are often very high for bio-relevant targets, meaning a first barrier to be overcome for molecular recognition to be done in pure water.10 Moreover, abiotic fully synthetic receptors commonly suffer from low solubility, a propensity to aggregate or undesirable folding promoted by the presence of water (due to the well-known hydrophobic effect).11 These facts make the molecular recognition process challenging in pure water. Additional issues arise from the accurate control of important parameters in aqueous environments, such as the pH of the medium or the presence of competing salts (ionic strength), which could preclude suitable host–guest matching by protonation–deprotonation processes or shielding of electrostatic interactions.3
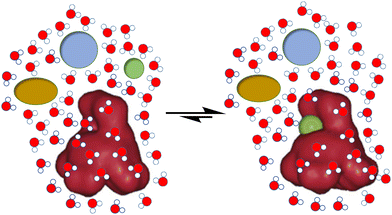 |
| Fig. 1 Schematic representation of the challenges for biomolecular recognition: strength, selectivity and competition from the aqueous medium. | |
Apart from aqueous media, other environments are key for biological processes. For instance, cellular or organelle membranes are dynamic heterogeneous media with a polarity gradient that goes from an ion-rich surface to a hydrophobic core that resembles the polarity of aliphatic alkanes. In addition, these hydrophobic barriers are present in any type of chemical biology work, whether the molecular recognition occurs within biological membranes or in the aqueous cytosol. Thus, for a synthetic receptor or ligand to play a role inside the cell, this must cross the cell membrane, taking advantage of the multiple mechanisms available.12
Another important hurdle in supramolecular chemical biology studies is the efficiency of the molecular recognition process, which is closely related to the effective concentration of the systems in the place of action. The binding constants needed for molecular recognition useful in biological media must be of the same order of magnitude as those already present in the corresponding biological network. Despite strong host–guest systems reported in literature,13 typical binding processes in conventional supramolecular chemistry are in the range of millimolar values of the dissociation constants, which is often insufficient for the potency required in chemical biology.14 This handicap is additionally complicated by the fact that in biological systems, apart from the thermodynamic strength of the complexes, the exchange rate of the binding must be considered.15 The dynamic nature of the biological systems is closely connected with the challenge that is probably most difficult to overcome in supramolecular chemical biology: selectivity. Biological processes are held by a complex network of interacting species, crowded and confined within the cell volume. This makes it necessary to design the corresponding supramolecular species that is able to bind to the given target within a dense soup of competing species. High selectivity leads to specificity, which is a key factor in this scenario. For instance, high selectivity of recognition of a substrate as an inhibitor of a biological pathway reduces the potential appearance of side effects when developing hits for therapeutic applications. Conversely, when designing a putative supramolecular sensor,16,17 specificity is fundamental for a diagnostic tool that avoids false positives or the absence of a signal in real samples.
Considering the complex process for designing supramolecular systems able to work in biological environments, the rate of success is often low, starting from tedious long syntheses with frustrating molecular recognition outcomes. In our own experience, it is advisable to take into account the inner complexity of biology as a conceptual parameter in the design.18,19 In this feature article, examples using two main approaches will be discussed. In general, the more conventional design of receptors is time consuming, but more predictable results can be obtained when starting from well-established moieties or frameworks. Alternative approaches using dynamic covalent chemistry20,21 have the advantage of less synthetic effort, since the target itself selects the best receptor. In contrast, results are more difficult to predict and many control experiments must be planned to avoid chasing false positives. Thus, dynamic combinatorial chemistry (DCC)22 proposes the creation of a dynamic mixture of interconverting species (a dynamic combinatorial library, DCL) made of building blocks joined by dynamic covalent bonds. The DCL is responsive to stimuli, and the presence of a given target biomolecule (a template) will shift the DCL to the preferred formation of the best binder (Fig. 2). This methodology allows the identification of receptors either for small molecules and ions, or for larger biomacromolecules.23 However, due to the dynamic and reversible nature of the chemical bonds joining the building blocks of the DCL, these must be either frozen or substituted by robust and non-exchangeable isosters. For instance, imines are usually reduced to the corresponding amines, and disulfides can be substituted by thioethers. This process can modify the observed selectivity or binding properties of the initially amplified members, a potential drawback of the methodological approach.
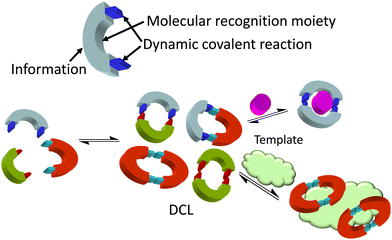 |
| Fig. 2 Dynamic combinatorial chemistry (DCC) approach to the identification of hosts for either small molecules and ions or larger biomacromolecules, used as a template. | |
Molecular recognition of ions
Ions play a fundamental role in many biological processes, such as the maintenance of cellular homeostasis or membrane potential. Accordingly, their importance in the normal function of the cell is related to serious diseases in the case of its failure. The ion imbalance mechanism can be exploited for the design and development of potential drugs.24–26 A prototypical example is the recognition and transport of chloride through lipid bilayers as a model for cell and organelle membranes.25 Recently, other ions have also attracted attention.27–30 This has become a very active research topic that combines anion molecular recognition approaches with membrane biophysical assays.31 The complexity of the systems is reflected in the fact that the process is not currently fully understood32 and relevant steps are needed in that direction.33,34 The development of efficient ion transporters is fundamental for proposing molecular tools for the replacement of the activity of defective natural transporters, as for the treatment of cystic fibrosis.35–37 Moreover, induced ion transport can be used to kill malignant cells as a mechanism of cancer chemotherapy.32,36,38 Several issues must be considered within this topic. First of all, a putative transporter must be minimally soluble to be dispersed in an aqueous medium, but hydrophobic enough to be efficiently transferred to the lipid bilayer and remain there. Moreover, ion transport is a kinetic process for which the ion must be strongly complexed to be dehydrated, but the ion-transporter complex should be labile enough to release the ion for efficient turnover. Besides, for the process to be effective, the transporter must travel between the two membrane surfaces (a polar environment) by crossing a very hydrophobic environment. This makes the overall process a complex one that depends on many physicochemical parameters like the ion binding constant, lipophilicity, diffusiveness or conformational behaviour.32 Moreover, selectivity is an issue in this case, since lack of specificity is closely related to undesirable side effects in the case of biomedical applications. Very clever and elegant approaches to implementing selectivity have recently been developed, such as the inclusion of photochemical or redox stimuli.39
The effect of the pH of the medium has been also proposed as a suitable stimulus,40 since the increased metabolism of cancer cells produces intracellular alkalinization and extracellular acidification, leading to an acidic microenvironment around solid tumours.41 Thus, receptors able to increase ion transport in slightly acidic pH values would be able to selectively kill cancer cells without affecting those healthy cells surrounded by a neutral or slightly basic medium. Several groups have developed ion transporters with such a pH-dependent transport ability,42–47 but requiring a pH-range much wider than that observed in cancer cell biology. Within this specific topic, we have been working for more than a decade with small tripodal pseudopeptidic cages that are able to strongly bind chloride anions when they are partially protonated (Fig. 3a).48 Interestingly, they showed high selectivity for chloride versus very similar fluoride and bromide anions, as demonstrated by 1H NMR titration experiments in aqueous acetonitrile and by X-ray diffraction of single crystals in the solid state.49 The selectivity is due to size matching with the inner cavity of the cage that nicely hosts chloride but is not suitable for the larger bromide halide, while the smaller fluoride allows water molecules to compete for the cage binding pocket. This size matching is reflected in the strong binding constant (Ka ≈ 104 M−1) of protonated cages towards chloride in aqueous acetonitrile, that is retained regardless of the nature of the side chain, suggesting a conserved binding pocket for the anion, as confirmed from the crystal structures of several cages (Fig. 3b). Actually, the synthesis of the cages themselves was improved when chloride was added as a template during the triple nucleophilic substitution reaction.48,49
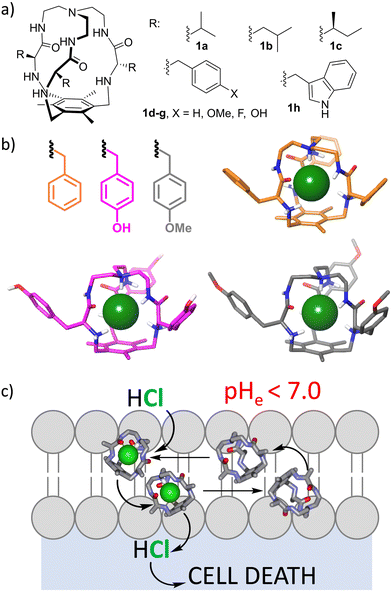 |
| Fig. 3 (a) Chemical structures of the pseudopeptidic cages able to selectively bind chloride anions when protonated. (b) Solid-state structures for the tetrachloride salt of selected cages showing the bound anion within the cage cavity as a space-filling green sphere (carbon colour code as in the depicted side chain, non-polar H atoms, crystallization solvent molecules and additional chloride anions are omitted for clarity). (c) Schematic representation of the mechanism for the H+/Cl− symport mediated by the cages, which would lead to pH-dependent cancer cell cytotoxicity. | |
The protonated cages transport chloride through lipid bilayers by an H+/Cl− symport mechanism (Fig. 3c), with differential effectivity depending on the nature of the amino acid side chain decorating the external surface of the cages. The necessary protonation allowed us to identify some cages derived from aromatic amino acids that improved their chloride transport properties when a slightly acidic pH gradient is imposed, similar to that expected around solid tumours.50 Thus, the 4-F-Phe derived cage (1f) is a better HCl transporter at slightly acidic pH values, as demonstrated by ion selective electrode and fluorescence measurements. Concomitantly, it is able to dissipate those pH gradients, impacting the overall ionic transmembrane imbalance. Satisfyingly, this property correlates with its pH-dependent cytotoxicity against human lung adenocarcinoma cells: a five-fold increase in cytotoxicity was observed for cells grown in slightly acidic pH values, mimicking those found around solid tumours.50 The intriguing effect of the fluorine atom must be related to the fine tuning of the corresponding physicochemical properties that modulate the activity of the cage as a potential therapeutical ionophore in cancer chemotherapy.
Molecular recognition of small biomolecules
Recognition of small molecules in a biomimetic environment is a hard task because their usually high hydration energy must be compensated by the interactions with the receptors. This is especially challenging when dealing with ionic51 or zwitterionic52 structures. In recent work, Quesada and co-workers described a pincer-like receptor for the lactate anion that is able to bind this hydroxy acid in competitive media.53 Moreover, this receptor transports the substrate through lipid bilayers with remarkable efficiency, making it the first example of a transporter for this type of molecule. The recognition of amino acids is also very appealing for the development of sensors or ligands able to recognize these motifs in water. For instance, receptors against N-methyl lysine54 or N-acetyl lysine55 have been reported, with potential activity in histone biology. Also the binding of proline and its transport through cell membranes have recently been described.56
In our group, we have used an alternative DCC approach for the selective and efficient recognition of cysteine amino acid, both in reduced and oxidized forms. Starting from a curiosity-generated DCL of pseudopeptidic disulfides,57 we identified the spontaneous assembly between a tripodal (2) and a bipodal (3) building block with Cys, leading to a heterotrimer stabilized by recognition of the zwitterionic form of Cys and a favoured disulfide bond within a folded compact conformation (Fig. 4). The stability of the structure was established as being dependent on parameters like pH or ionic strength, with very good correlation with the proposed recognition motif.58,59 Considering the high specificity, we used that dynamic system to prepare a dynamic covalent mixture that renders a fluorescent response in the presence of Cys and cystine, by including the monopodal building block 4 in the mixture. The presence of Cys or cystine rearranges the initially complex DCL for the formation of the very stable heterotrimer, releasing the disulfide of thiol 4, which exhibits strong excimer emission in a folded conformation in aqueous media. The excimer/monomer emission ratio can be used to quantify the amino acid and its oxidized form even in the presence of other biologically relevant thiols or a large excess of other amino acids. Remarkably, this dynamic sensing system is able to detect the amino acid even in biological human fluids like urine, as a prospective diagnostic tool for cystinuria.60
 |
| Fig. 4 DCL for Cys recognition and fluorescent sensing in a biorelevant environment. | |
Among other small biomolecules, mono- and disaccharides are especially challenging, for several reasons. First, the polyhydroxylated structure mimics a cluster of water molecules, precluding desolvation of the substrate and binding to the host. Moreover, the large number of chiral centres produces a wide range of stereoisomers with very subtle structural differences, making selectivity an especially challenging goal. Within this aspect, Davis’ group has mastered the design of extremely efficient synthetic receptors for carbohydrates with a common structural motif, the so-called temple receptors (Fig. 5).61 Over the years, Davis’ group has optimized their structure by tuning the binding epitopes complementary to the hydroxyl groups by H-bonding (red moieties in Fig. 5) and to the aliphatic core of the sugars by establishing CH–π interactions62 (blue in Fig. 5). Within this design, several hosts have been prepared. For instance, a prototypical example is macrocycle 5a, which represents a simple and accessible synthetic lectin due to its easy preparation and behaviour similar to that of natural carbohydrate-binding proteins. A combination of fluorescence, NMR and ITC rendered a modest Ka = 56 M−1, although the compound can be used to detect glucose in aqueous media. However, compound 5 shows moderate selectivity, which is a drawback for its use in a biological environment. Actually, a structurally related host 5b was described as being a very strong binder of purine and pyrimidine bases with affinities rising to 107 M−1 in an aqueous buffer.63
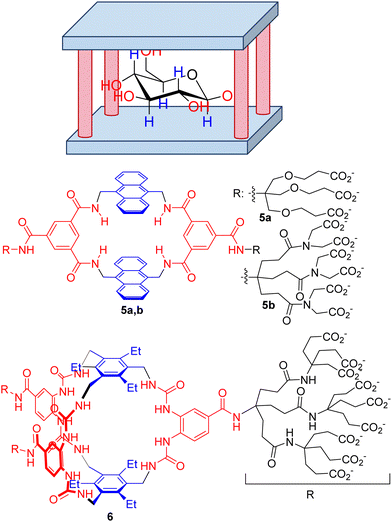 |
| Fig. 5 General design of a temple receptor for saccharides and selected examples. The polar H-bonding columns are depicted in red while the aromatic non-polar roofs and floors are blue coloured. Peripheral solubilizing groups (R), anionic at neutral pH in water, are shown in black. | |
The same group prepared more elaborate generations of temple hosts for different types of carbohydrate, mainly by the extension of the aromatic floor and roof, or by increasing the number of H-bonding columns.61 This systematic knowledge allowed them to optimize an extremely efficient receptor (6 in Fig. 5) that is characterized by minimalistic aromatic CH–π recognition motifs but a more elaborate H-bonding network to host the all-equatorial OH groups of glucose.64 The measured binding constant towards β-D-glucose went up to 18
000 M−1 with remarkable selectivity compared to other simple carbohydrates and other biomolecules and drugs. As a matter of fact, the key macrobicyclization step in the synthetic scheme of 6 can be improved by the presence of β-D-glucopyranoside, indicating an important template effect.65–67 More remarkably, the receptor is able to operate in cell culture media and in human blood serum, which clearly demonstrates the future utility of 6 in the real world for glucose sensing68 and monitoring or glucose-responsive insulin applications.
Saccharides have also been used as templates in DCC studies. Thus, a dynamic mixture of macrocyclic peptide disulfides was used for the molecular recognition of mono- and di-saccharides, with remarkable amplification of macrocycles containing His and Tyr amino acids (Fig. 6).69 Interestingly, two considerably different targets could be recognized, namely N-acetyl neuraminic acid (NANA) and trehalose disaccharide. Further binding studies revealed the formation of complexes with 1
:
1 and 1
:
2 receptor
:
sugar stoichiometries.70 In addition, parallel and antiparallel macrocyclic disulfides can be obtained with some differential binding behaviour. His-containing macrocycles recognize one or two molecules of NANA, while the Tyr receptor binds just one molecule of trehalose. These facts would have been difficult to predict from molecular design, underscoring the potential of DCC for discovering unexpected receptors.
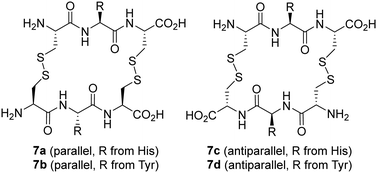 |
| Fig. 6 Disulfide peptide macrocycles identified as efficient receptors for NANA and trehalose. | |
During collaborative work, we have also contributed to the field of monosaccharide recognition by designing an extremely simple DCL of imines from isophthaldehyde and several amines and amino acids in aqueous carbonate buffer at pH 10 (Fig. 7a).71 Different aldoses and ketoses were tested as templates of the DCL of imines, and in situ reduction allowed identification and quantification of the amplified receptors, which were subsequently synthesized at a preparative scale, after reasonable structural simplification (Fig. 7b). Isothermal titration calorimetry (ITC) confirmed that the observed amplifications correlate with the binding of the ligands to the sugar structures, with 8a,b being the most efficient. Actually, 8b showed remarkable affinity (Ka ≈ 1700 M−1) and high selectivity for fructose over other monosaccharides (>50-fold) in pure water. Molecular modelling of the proposed complexes suggested that this selectivity arises from the specific H-bonding network within the anomeric moiety of the β-D-fructopyranose form in addition to several CH–π interactions with the aromatic rings of the ligand (Fig. 7c). Despite this very simple host being far from finding practical applications, this study demonstrates the ability of DCC as a mechanism to discover unexpected binding motifs and systems for challenging biomolecules.
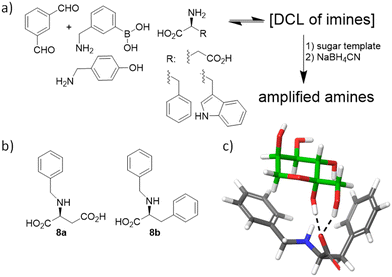 |
| Fig. 7 (a) Sugar-templated DCL. (b) Simplified monosaccharide hosts thus identified. (c) Proposed model for the interaction of 8b with β-D-fructopyranose (green C-atoms). | |
Molecular recognition of biomacromolecules
Molecular recognition of specific sequences in polypeptides is another biologically relevant but extremely difficult task.72 Apart from the general issues of biomolecular recognition, the secondary structure and conformational flexibility of polypeptides add additional complications to the design of specific hosts. Some relevant groups have proposed different receptors able to recognize sequences or solvent-exposed regions of proteins and peptides.73–81 The general background of these receptors consists of a hydrophobic cavity surrounded by polar interactions, leading to the efficient binding of specific sequences.54,75–77,82–84 In a long-term research project on the recognition of peptide sequences, we have designed large pseudopeptidic cages with a bigger cavity flanked by two aromatic rings and several polar groups (9a–d, Fig. 8a).85 The modular design of the synthetic scheme allowed us to prepare a large variety of cages decorated with different amino acid side chains. Systematic screening of binding using fluorescence and NMR titration experiments, as well as ESI-MS competition assays, identified di- and tri-peptide sequences bearing an aromatic amino acid (Phe or Tyr, but not Trp or His) as suitable substrates.86 Further structural studies (NMR, fluorescence and molecular modelling) combined with ion mobility mass spectrometry suggested the inclusion of the Tyr side chain within the inner cavity of the cage, stabilized by aryl–aryl and H-bonding interactions.87,88 Moreover, sequence selectivity was also achieved by secondary interactions between the external side chains of the pseudopeptidic cages and the amino acids surrounding the Tyr residue in the peptide substrates. Thus, complementary electrostatic contacts rendered stronger binding than those presenting residues with the same charge sign.88 Remarkably, this recognition can be translated to polypeptides containing these binding motifs, as clearly reflected when using specific random peptide co-polymers containing Tyr and different numbers of either Glu or Lys residues.89 A combination of fluorescence titrations with relaxation and diffusion-filtered NMR experiments demonstrated the selective and strong binding of large Tyr-containing polypeptides in buffered aqueous media (Fig. 8b).
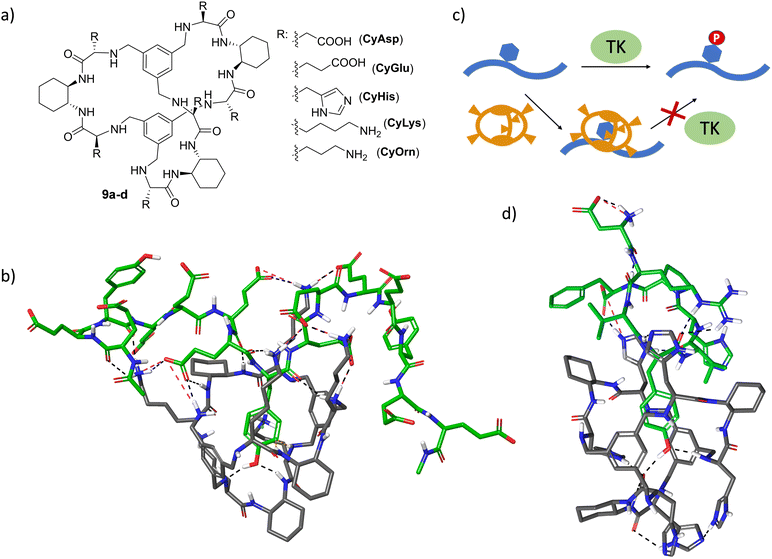 |
| Fig. 8 (a) Chemical structures of large pseudopeptidic cages 9a–d designed to bind Tyr aromatic amino acid side chains in peptides. (b) Molecular model for the interaction of CyLys with the Ac-EEYEEEEYEEEEYEE-NH2 peptide. (c) Schematic representation for the mechanism of inhibition of TK-mediated phosphorylation of Tyr-containing peptides (blue) mediated by the pseudopeptidic cages (orange). (d) Molecular model for the complex between CyHis and the Val5AngII peptide (sequence: DRVYVHPF). In (b) and (d), non-polar H-bonds are omitted, H-bonds and salt bridges are shown by black and pink dashed lines, respectively, and the peptide substrates are represented with green C-atoms. Both minimized structures show a Tyr residue included within the cage cavity with the phenolic OH setting H-bonds with different functions of the cage. | |
The efficient binding of Tyr residues has important biological implications. Thus, Tyr phosphorylation by protein Tyr kinases (TK in Fig. 8c) is a key process in cell regulation and is closely related to serious diseases.90,91 The efficient inclusion of a Tyr side chain within the cage structure protects the residue from the action of TK enzymes, as demonstrated by phosphorylation assays with different peptide sequences as substrates of Src TK.92,93 Satisfyingly, the inhibition of Tyr phosphorylation mediated by the cages correlates with their binding affinity towards the peptide substrates, as measured by fluorescence titration experiments.93 Moreover, the cages show promising substrate selectivity depending on the peptide sequence close to the Tyr, even for peptides bearing additional aromatic amino acids (Fig. 8d).93 Moreover, the generality of the strategy was demonstrated with different TK enzymes. This TK-modulation allows a defined Tyr-containing protein or peptide to be protected without affecting the activity of the TK enzyme towards a different substrate. Since a given TK is often implicated in several signalling pathways and in the modification of different proteins, this appealing approach has the potential to complement and improve the usually low specificity of available TK inhibitors.90,94
Despite polysaccharides are biomacromolecules with key functions in biological systems, their selective molecular recognition remains a scarcely explored field. Davis’ group also reported a seminal work on the design of a temple receptor able to recognize cellulose, chitin and related biopolymers.95 Thus, temple receptor 10 with extended pyrene rings and wider portals forms very stable complexes with all-equatorial β-pyranoside oligomers, as confirmed by NMR and ITC titration experiments. Very remarkably, the threading of the substrates within the host cavity (Fig. 9) was demonstrated by a combination of detailed NMR experiments (including NOE) and molecular modelling. These threaded structures are retained with polymeric cellulose and chitosan, leading to unique polypseudorotaxane complexes observable by atomic force microscopy on mica surfaces. This unprecedented molecular recognition system could facilitate the exploitation of cellulose by improving the solubility of the corresponding fibres, or the manipulation of biotechnologically relevant chitosan for tissue engineering.
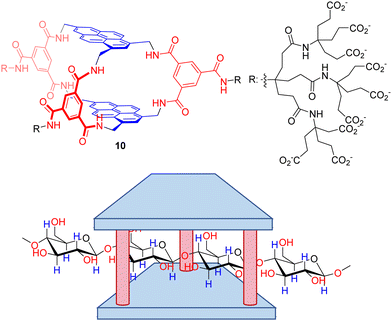 |
| Fig. 9 Macrobicyclic receptor for the binding of β-pyranoside polysaccharides in water. The molecular recognition process occurs by threading the biopolymer through the host cavity in a pseudorotaxane structure. | |
Another interesting group of polysaccharides is glycosaminoglycans (GAGs) that play an essential role in cell signalling and communication, being implicated in key serious pathological processes like cancer metastasis or viral infection.96,97 They are present in the extracellular matrix (ECM) either alone or conjugated with lipids and proteins, making them the external face of mammalian cells.98,99
GAGs are characterized by the repetition of highly anionic saccharides, leading to strongly hydrated and flexible polymers.100 These facts make them extremely difficult targets for molecular recognition by design, as they lack conventional binding pockets. Therefore, we hypothesized that GAGs could be the ideal templates for DCLs, since this approach does not require a detailed knowledge of the target structure. As a prototypical GAG, we selected heparin (see the structure in Fig. 10a) which is the biopolymer with the highest negative charge density described so far. Heparin is a broadly used drug, mainly as an anticoagulant101,102 but it is also employed in the treatment of cancer103 and infections.104 As a proof of concept, we selected a natural polyamine (spermine) that must be protonated in water at neutral pH105 and thus it would interact with the sulfates and carboxylates of the GAG.106,107 Additional CH–π interactions were implemented with a mixture of aromatic aldehydes, leading to a dynamic mixture of structurally varied imines.108In situ reductive amination of the DCL in the absence and presence of heparin rendered 11a as an amplified member of the library. We prepared the ligand at preparative scale to confirm its strong binding to heparin in the low micromolar range, using a combination of ITC, NMR and fluorescence spectroscopy. The in vitro activity of the ligand was also tested with an enzymatic cascade reaction closely related to blood coagulation (Fig. 10c), which showed the ability of 11a to reverse the inhibitory effect of heparin. Very remarkably, a closely related ligand (11d) that was not amplified in the DCC experiments shows weak binding to heparin and no in vitro activity.108 In a later study, we expanded the molecular diversity of the DCL by increasing the number and structural variety of the corresponding aldehydes. In this way, we identified two additional ligands (11b and 11c) that were more amplified than 11a in competition experiments.109 Binding studies using surface plasmon resonance (SPR) and fluorescence titrations rendered submicromolar affinity of 11b–c towards different forms of heparin. Structural studies by NMR (including DOSY) and molecular dynamics simulations (Fig. 10b) confirmed the formation of stable complexes in water, by the polyamines wrapping around the GAG and establishing polar (Coulombic contacts and H-bonds) and non-polar (CH–π) interactions. The corresponding enzymatic tests (Fig. 10c) on amplified and non-amplified members of the DCL served as a definitive validation of the screening protocol, since we found a very good correlation between the corresponding amplification factors and in vitro activity. The most efficient ligands (11b–c) were additionally assayed as heparin reversal agents in more challenging and meaningful environments. Thus, both ex vivo coagulation experiments with freshly extracted mouse blood and in vivo tests using a tail transection assay showed a dose–response efficient activity as heparin antidotes (Fig. 10d).109 Accordingly, this work demonstrated how supramolecular principles can be used for the molecular recognition of challenging biopolymer targets, even with potential applications in real life as hits towards the development of future drugs.
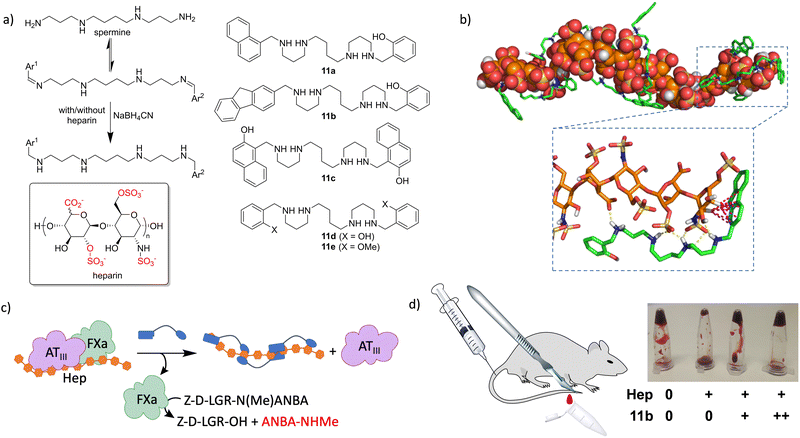 |
| Fig. 10 (a) DCL of imines from spermine and a mixture of aromatic aldehydes, by a reductive amination reaction and using heparin as a template. Some selected representative spermine structures of those studied are also shown. (b) Illustrative snapshot from molecular dynamic simulations performed with a heparin oligomeric model (orange) and six molecules of compound 11b (green). Non-polar H-atoms are omitted for clarity, H-bonds and CH–π interactions are shown by yellow and pink dashed lines, respectively. (c) Schematic representation for the enzymatic reaction used to test the in vitro activity of the identified ligands as reversal agents of heparin anticoagulant. In brief, the ternary complex [ATIII-Hep-FXa] inactivates the FXa protease, while strong heparin ligands dissociate this complex, releasing FXa, which triggers the hydrolysis of a peptide substrate mimicking those related to the coagulation cascade. (d) The biological activity of the spermine derivatives in mouse blood was additionally confirmed by ex vivo and in vivo experiments. | |
Molecular recognition and self-assembly in especially challenging biological environments
When dealing with molecular recognition in chemical biology, the ultimate goal must be to characterize supramolecular systems in living matter. This objective, despite being appealing and necessary for any type of real application, is extremely challenging as living matter is a molecularly complex and a strongly competitive environment for non-covalent interactions.
In this context and as a step further in the development of GAG ligands, we wanted to demonstrate the concept with structures other than heparin. As mentioned before, GAGs form part of the ECM of mammalian cells, and their structure and composition depend strongly on the cell type.110 Moreover, in certain pathological processes like cancer metastasis111 or host–pathogen infection,112 the nature and composition of the ECM suffer important changes, with the GAG composition being a fingerprint for the characterization of the cell type or its stage of progression in pathology. With this idea in mind, we hypothesized that the native ECM of living cells could be used as a template in a similar DCL of imines against GAGs (Fig. 11).
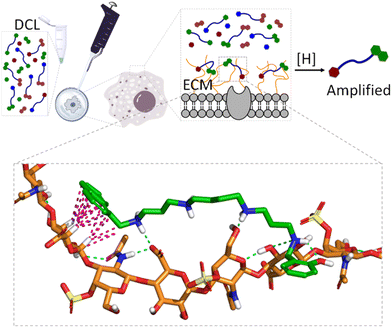 |
| Fig. 11 Schematic representation of live-cell-templated DCC experiment targeting the GAGs of the ECM. The inset shows a model interaction between the amplified member of the library (11a) and chondroitin sulfate A (CDS-A), the main GAG in the ECM of A549 cells used for the DCC experiments. In the model, non-polar H atoms are omitted, CDS-A and 11a are represented with orange and green C-atoms, respectively. On the other hand, H-bonds and CH–π interactions are depicted with blue and pink dashed lines, respectively. | |
Gratifyingly, the reductive amination reaction of a DCL generated from spermine and a small selection of aromatic aldehydes showed strong amplification of 11a in the presence of human lung adenocarcinoma (A549) cells.113 The amplification pattern varies with the type of cancer cell (e.g. HeLa cells), suggesting that the DCC approach could be used as a cell-type fingerprint. Moreover, experiments performed in the presence of protamine (a small Arg-rich protein) as a competing binder, or with A549 cells grown with an inhibitor of ECM sulfation, supressed the observed amplifications. These experiments identified GAGs as the binding motives for the DCL. On-cell NMR experiments with 11a (amplified ligand) and 11e (non-amplified ligand) confirmed their reversible non-covalent binding to the ECM, being much stronger for 11a. Since chondroitin sulfate A (CDS-A) is the most abundant GAG in A549 cells, their strong binding to 11a was confirmed by SPR and NMR, while it was illustrated by molecular dynamics simulations that revealed the proposed polar and non-polar interactions (Fig. 11, inset). This work shows that living cells can be used in DCC and introduce supramolecular chemistry concepts to an especially relevant and meaningful environment for chemical biology.
The non-covalent self-assembly of designed building blocks inside living cells has also been used to target different organelles like the nucleus or mitochondria, controlling cell fate and viability. Short peptide sequences,114,115 DNA nanostructures116 or abiotic disulfides117,118 can be exploited in this regard. The key issue here is triggering self-assembly to occur within a precise location and timing,119 using chemical117,118 or enzymatic114,115 stimuli (Fig. 12). There is a promising future for this type of system for cancer treatment, while intracellular characterization of the assemblies still represents a challenge in which fluorescence imaging or in-cell NMR might play a key role.
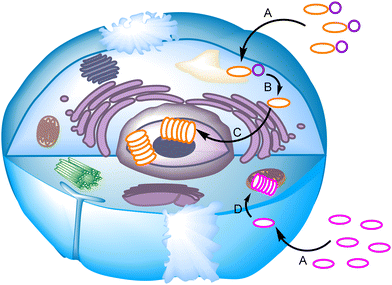 |
| Fig. 12 Schematic representation of selected mechanisms for the self-assembly process inside a live cell. A chemical entity (orange oval) with a modifiable function (purple circle) enters the cell (A) and is transformed by a chemical or enzymatic reaction (B) to be transferred and aggregated in the nucleus (C). On the other hand, a species-targeting mitochondrion (pink oval) is internalized in the cell (A) and accumulated in this organelle, inducing aggregation due to a much higher local concentration (D). | |
As a final example of the elegant use of molecular recognition for the control of an extremely complex biological process, a recent contribution was reported in the field of bacterial infection. Thus, cationic pillarenes can be used to efficiently encapsulate chemical signals (homoserine lactones) for the quorum sensing of pathological bacteria, disrupting their intercommunication.120,121 This binding effect precludes biofilm formation and improves healing in open-wound in vivo models. This inspiring supramolecular approach paves the way towards an alternative mechanism to fight antimicrobial resistance to conventional drugs, which is one of the main current concerns in human health.122,123
Conclusions
The molecular recognition of biorelevant species is an essential topic of research in chemical biology. Thus, processes like the reading and transference of genetic information, cell communication, enzymatic activity or even the integrity of the cell rest on the establishment of non-covalent interactions between chemical entities. Since chemical biology focuses on the study, understanding and intervention of biological processes from a chemical perspective, supramolecular chemistry has concomitantly influenced chemical biology. Despite their intrinsic connectivity, there are still important hurdles for classical supramolecular chemistry to surmount before it can step into the field of chemical biology. The efficiency, specificity and, especially, the water compatibility of the supramolecular systems are essential in this regard. Also, temporal and spatial control over the supramolecular process can be an issue in the case of complex biological media. Different approaches for the design of biorelevant molecular recognition systems have been described in the literature, all of them having pros and cons. In our own experience, rational design usually renders more predictable outcomes while requiring higher synthetic efforts and sometimes leading to frustrating results. Dynamic covalent chemistry methodologies are interesting alternatives to speed up the identification of hits, although less predictable results and false positives are frequently obtained. The templated synthesis of designed receptors represents a somewhat hybrid possibility, as already illustrated in the syntheses of several hosts (i.e.1a–h or 6). In any case, no methodology is perfect and keeping in mind all the accessible options is strongly recommended.
Methodological connectivity between supramolecular chemistry and chemical biology is also evident when reviewing the experimental techniques or concepts supporting the hypotheses used in both disciplines. Moreover, the establishment of clear structure–activity relationships will benefit advances in the joint multidisciplinary field.
The final transfer of research to real-life applications requires testing of the supramolecular systems in meaningful biological environments, which imposes additional hurdles. To that end, sensitive assays in complex matrices like cells, organoids, tissues or even living animals must be developed. With regard to that, the early introduction of the matrix in the development of molecular recognition systems is desirable, for which methodological alternatives like live-cell-templated DCC or the use of high-resolution imaging techniques are especially appealing.
Data availability
This manuscript is submitted to be considered as a Feature Article and, accordingly it does not contain original research. Therefore, no new data needs to become available.
Conflicts of interest
There are no conflicts to declare.
Acknowledgements
I wish to thank all the past and current members of the group, especially those who have contributed to the development of this chemistry and appear as co-authors in the corresponding cited references from our group. Far from being an exhaustive revision of the literature in this emerging field, I apologize beforehand to those authors who can feel excluded from the references cited in this Feature Article. Financial support from the Spanish Research Agency within the Spanish Ministry of Science, Innovation and Universities (PID2021-128411NB-I00, MCIU/AEI/10.13039/501100011033, and FEDER/EU) is gratefully acknowledged.
Notes and references
- J.-M. Lehn, Science, 2002, 295, 2400–2403 CrossRef CAS PubMed.
- J.-M. Lehn, Science, 1993, 260, 1762–1763 CrossRef CAS PubMed.
-
B. Smith, Synthetic Receptors for Biomolecules, The Royal Society of Chemistry, 2015 Search PubMed.
-
Nat. Chem. Biol., 2015, 11, 378–379 Search PubMed.
- K. L. Morrison and G. A. Weiss, Nat. Chem. Biol., 2006, 2, 3–6 CrossRef CAS PubMed.
- K. Petkau-Milroy and L. Brunsveld, Org. Biomol. Chem., 2013, 11, 219–232 RSC.
- I. Insua and J. Montenegro, Chem., 2020, 6, 1652–1682 CAS.
- L. M. Salonen, M. Ellermann and F. Diederich, Angew. Chem., Int. Ed., 2011, 50, 4808–4842 CrossRef CAS PubMed.
- S. Mondal and B. Bagchi, Curr. Opin. Struct. Biol., 2022, 77, 102462 CrossRef CAS PubMed.
- J. Dong and A. P. Davis, Angew. Chem., Int. Ed., 2021, 60, 8035–8048 CrossRef CAS PubMed.
- N. B. Rego and A. J. Patel, Annu. Rev. Condens. Matter Phys., 2022, 13, 303–324 CrossRef CAS.
- I. D. Alves, C.-Y. Jiao, S. Aubry, B. Aussedat, F. Burlina, G. Chassaing and S. Sagan, Biochim. Biophys. Acta, Biomembr., 2010, 1798, 2231–2239 CrossRef CAS PubMed.
- S. Sarkar, P. Ballester, M. Spektor and E. A. Kataev, Angew. Chem., Int. Ed., 2023, 62, e202214705 CrossRef CAS PubMed.
- P. L. Kastritis and A. M. J. J. Bonvin, J. R. Soc., Interface, 2013, 10, 20120835 CrossRef PubMed.
- H.-X. Zhou, Q. Rev. Biophys., 2010, 43, 219–293 CrossRef PubMed.
- L. Motiei and D. Margulies, Acc. Chem. Res., 2023, 56, 1803–1814 CrossRef CAS PubMed.
- E. V. Anslyn, J. Org. Chem., 2007, 72, 687–699 CrossRef CAS PubMed.
- I. Alfonso, Chem. Commun., 2016, 52, 239–250 RSC.
- J. Solà, C. Jimeno and I. Alfonso, Chem. Commun., 2020, 56, 13273–13286 RSC.
- J.-M. Lehn and A. V. Eliseev, Science, 2001, 291, 2331–2332 CrossRef CAS PubMed.
- O. Ramström and J.-M. Lehn, Nat. Rev. Drug Discovery, 2002, 1, 26–36 CrossRef PubMed.
- P. T. Corbett, J. Leclaire, L. Vial, K. R. West, J.-L. Wietor, J. K. M. Sanders and S. Otto, Chem. Rev., 2006, 106, 3652–3711 CrossRef CAS PubMed.
- A. Herrmann, Chem. Soc. Rev., 2014, 43, 1899–1933 RSC.
- I. Alfonso and R. Quesada, Chem. Sci., 2013, 4, 3009–3019 RSC.
- P. A. Gale, J. T. Davis and R. Quesada, Chem. Soc. Rev., 2017, 46, 2497–2519 RSC.
- P. A. Gale, R. Pérez-Tomás and R. Quesada, Acc. Chem. Res., 2013, 46, 2801–2813 CrossRef CAS PubMed.
- L. Martínez-Crespo, S. H. Hewitt, N. A. De Simone, V. Šindelář, A. P. Davis, S. Butler and H. Valkenier, Chem. – Eur. J., 2021, 27, 7367–7375 CrossRef PubMed.
- L. Martínez-Crespo and H. Valkenier, ChemPlusChem, 2022, 87, e202200266 CrossRef PubMed.
- A. Cataldo, K. Norvaisa, L. Halgreen, S. E. Bodman, K. Bartik, S. J. Butler and H. Valkenier, J. Am. Chem. Soc., 2023, 145, 16310–16314 CrossRef CAS PubMed.
- L. Jing, E. Deplazes, J. K. Clegg and X. Wu, Nat. Chem., 2024, 16, 335–342 CrossRef CAS PubMed.
- R. Quesada, Chem., 2019, 5, 1924–1926 CAS.
- S.-H. Park, S.-H. Park, E. N. W. Howe, J. Y. Hyun, L.-J. Chen, I. Hwang, G. Vargas-Zuñiga, N. Busschaert, P. A. Gale, J. L. Sessler and I. Shin, Chem., 2019, 5, 2079–2098 CAS.
- X. Wu, J. R. Small, A. Cataldo, A. M. Withecombe, P. Turner and P. A. Gale, Angew. Chem., Int. Ed., 2019, 58, 15142–15147 CrossRef CAS PubMed.
- S. Cheung, D. Wu, H. C. Daly, N. Busschaert, M. Morgunova, J. C. Simpson, D. Scholz, P. A. Gale and D. F. O’Shea, Chem., 2018, 4, 879–895 CAS.
- X. Wu, E. N. W. Howe and P. A. Gale, Acc. Chem. Res., 2018, 51, 1870–1879 CrossRef CAS PubMed.
- N. Akhtar, O. Biswas and D. Manna, Chem. Commun., 2020, 56, 14137–14153 RSC.
- N. Busschaert and P. A. Gale, Angew. Chem., Int. Ed., 2013, 52, 1374–1382 CrossRef CAS PubMed.
- X.-H. Yu, X.-Q. Hong, Q.-C. Mao and W.-H. Chen, Eur. J. Med. Chem., 2019, 184, 111782 CrossRef CAS PubMed.
- M. Ahmad, S. A. Gartland and M. J. Langton, Angew. Chem., Int. Ed., 2023, 62, e202308842 CrossRef CAS PubMed.
- N. Kumar and N. Madhavan, Org. Biomol. Chem., 2023, 21, 5892–5905 RSC.
-
K. M. Bailey, J. W. Wojtkowiak, A. I. Hashim and R. J. Gillies, in Current Challenges in Personalized Cancer Medicine, ed. K. S. M. Smalley, Academic Press, 2012, vol. 65, pp. 63–107 Search PubMed.
- E. N. W. Howe, N. Busschaert, X. Wu, S. N. Berry, J. Ho, M. E. Light, D. D. Czech, H. A. Klein, J. A. Kitchen and P. A. Gale, J. Am. Chem. Soc., 2016, 138, 8301–8308 CrossRef CAS PubMed.
- N. Busschaert, R. B. P. Elmes, D. D. Czech, X. Wu, I. L. Kirby, E. M. Peck, K. D. Hendzel, S. K. Shaw, B. Chan, B. D. Smith, K. A. Jolliffe and P. A. Gale, Chem. Sci., 2014, 5, 3617–3626 RSC.
- R. B. P. Elmes, N. Busschaert, D. D. Czech, P. A. Gale and K. A. Jolliffe, Chem. Commun., 2015, 51, 10107–10110 RSC.
- L. A. Marchetti, T. Krämer and R. B. P. Elmes, Org. Biomol. Chem., 2022, 20, 7056–7066 RSC.
- A. Roy, D. Saha, P. S. Mandal, A. Mukherjee and P. Talukdar, Chem. – Eur. J., 2017, 23, 1241–1247 CrossRef CAS PubMed.
- E. N. W. Howe, V.-V. T. Chang, X. Wu, M. Fares, W. Lewis, L. K. Macreadie and P. A. Gale, Biochim. Biophys. Acta, Biomembr., 2022, 1864, 183828 CrossRef CAS PubMed.
- I. Martí, J. Rubio, M. Bolte, M. I. Burguete, C. Vicent, R. Quesada, I. Alfonso and S. V. Luis, Chem. - Eur. J., 2012, 18, 16728–16741 CrossRef PubMed.
- I. Martí, M. Bolte, M. I. Burguete, C. Vicent, I. Alfonso and S. V. Luis, Chem. - Eur. J., 2014, 20, 7458–7464 CrossRef PubMed.
- L. Tapia, Y. Pérez, M. Bolte, J. Casas, J. Solà, R. Quesada and I. Alfonso, Angew. Chem., Int. Ed., 2019, 58, 12465–12468 CrossRef CAS PubMed.
- P. A. Gale, E. N. W. Howe and X. Wu, Chem., 2016, 1, 351–422 CAS.
- I. Alfonso and J. Solà, Chem. - Asian J., 2020, 15, 986–994 CrossRef CAS PubMed.
- D. Alonso-Carrillo, A. Arias-Betancur, I. Carreira-Barral, P. Fontova, V. Soto-Cerrato, M. García-Valverde, R. Pérez-Tomás and R. Quesada, iScience, 2023, 26, 107898 CrossRef CAS PubMed.
- J. E. Beaver and M. L. Waters, ACS Chem. Biol., 2016, 11, 643–653 CrossRef CAS PubMed.
- M. Orlandini, A. Pedrini, D. Marchetti, Y. Li, G. Aragay, E. Dalcanale, P. Ballester and R. Pinalli, Chem. – Eur. J., 2024, 30, e202303715 CrossRef CAS PubMed.
- L. Martínez-Crespo, J. L. Sun-Wang, A. F. Sierra, G. Aragay, E. Errasti-Murugarren, P. Bartoccioni, M. Palacín and P. Ballester, Chem., 2020, 6, 3054–3070 Search PubMed.
- J. Solà, M. Lafuente, J. Atcher and I. Alfonso, Chem. Commun., 2014, 50, 4564–4566 RSC.
- M. Lafuente, J. Atcher, J. Solà and I. Alfonso, Chem. - Eur. J., 2015, 21, 17002–17009 CrossRef CAS PubMed.
- M. Lafuente, I. Alfonso and J. Solà, ChemSystemsChem, 2019, 1, 25–31 CrossRef CAS.
- M. Lafuente, J. Solà and I. Alfonso, Angew. Chem., Int. Ed., 2018, 57, 8421–8424 CrossRef CAS PubMed.
- A. P. Davis, Chem. Soc. Rev., 2020, 49, 2531–2545 RSC.
- J. L. Asensio, A. Ardá, F. J. Cañada and J. Jiménez-Barbero, Acc. Chem. Res., 2013, 46, 946–954 CrossRef CAS PubMed.
- D. Van Eker, S. K. Samanta and A. P. Davis, Chem. Commun., 2020, 56, 9268–9271 RSC.
- R. A. Tromans, T. S. Carter, L. Chabanne, M. P. Crump, H. Li, J. V. Matlock, M. G. Orchard and A. P. Davis, Nat. Chem., 2019, 11, 52–56 CrossRef CAS PubMed.
- S. Anderson, H. L. Anderson and J. K. M. Sanders, Acc. Chem. Res., 1993, 26, 469–475 CrossRef CAS.
- R. Vilar, Angew. Chem., Int. Ed., 2003, 42, 1460–1477 CrossRef CAS PubMed.
- S. J. Rowan, S. J. Cantrill, G. R. L. Cousins, J. K. M. Sanders and J. F. Stoddart, Angew. Chem., Int. Ed., 2002, 41, 898–952 CrossRef PubMed.
- R. A. Tromans, S. K. Samanta, A. M. Chapman and A. P. Davis, Chem. Sci., 2020, 11, 3223–3227 RSC.
- M. Rauschenberg, S. Bomke, U. Karst and B. J. Ravoo, Angew. Chem., Int. Ed., 2010, 49, 7340–7345 CrossRef CAS PubMed.
- M. Rauschenberg, S. Bandaru, M. P. Waller and B. J. Ravoo, Chem. – Eur. J., 2014, 20, 2770–2782 CrossRef CAS PubMed.
- M. Alena-Rodriguez, M. Fernandez-Villamarin, I. Alfonso and P. M. Mendes, Org. Biomol. Chem., 2024, 22, 3854–3859 RSC.
- M. W. Peczuh and A. D. Hamilton, Chem. Rev., 2000, 100, 2479–2494 CrossRef CAS PubMed.
- R. E. McGovern, H. Fernandes, A. R. Khan, N. P. Power and P. B. Crowley, Nat. Chem., 2012, 4, 527–533 CrossRef CAS PubMed.
- P. B. Crowley, Acc. Chem. Res., 2022, 55, 2019–2032 CrossRef CAS PubMed.
- S. van Dun, C. Ottmann, L.-G. Milroy and L. Brunsveld, J. Am. Chem. Soc., 2017, 139, 13960–13968 CrossRef CAS PubMed.
- P. Suating, L. B. Kimberly, M. B. Ewe, S. L. Chang, J. M. Fontenot, P. R. Sultane, C. W. Bielawski, D. A. Decato, O. B. Berryman, A. B. Taylor and A. R. Urbach, J. Am. Chem. Soc., 2024, 146, 7649–7657 CrossRef CAS PubMed.
- P. Suating, M. B. Ewe, L. B. Kimberly, H. D. Arman, D. J. Wherritt and A. R. Urbach, Chem. Sci., 2024, 15, 5133–5142 RSC.
- Z. Hirani, H. F. Taylor, E. F. Babcock, A. T. Bockus, C. D. Varnado, C. W. Bielawski and A. R. Urbach, J. Am. Chem. Soc., 2018, 140, 12263–12269 CrossRef CAS PubMed.
- L. C. Smith, D. G. Leach, B. E. Blaylock, O. A. Ali and A. R. Urbach, J. Am. Chem. Soc., 2015, 137, 3663–3669 CrossRef CAS PubMed.
- F.-G. Klärner and T. Schrader, Acc. Chem. Res., 2013, 46, 967–978 CrossRef PubMed.
- T. Schrader, G. Bitan and F.-G. Klärner, Chem. Commun., 2016, 52, 11318–11334 RSC.
- H. Wang, R. S. Dawber, P. Zhang, M. Walko, A. J. Wilson and X. Wang, Chem. Sci., 2021, 12, 5977–5993 RSC.
- M.-H. Le, E. S. Taghuo K. and T. Schrader, Chem. Commun., 2022, 58, 2954–2966 RSC.
- H. Shahpasand-Kroner, I. Siddique, R. Malik, G. R. Linares, M. I. Ivanova, J. Ichida, T. Weil, J. Münch, E. Sanchez-Garcia, F.-G. Klärner, T. Schrader and G. Bitan, Pharmacol. Rev., 2023, 75, 263–308 CrossRef CAS PubMed.
- A. Moure, S. V. Luis and I. Alfonso, Chem. - Eur. J., 2012, 18, 5496–5500 CrossRef CAS PubMed.
- E. Faggi, A. Moure, M. Bolte, C. Vicent, S. V. Luis and I. Alfonso, J. Org. Chem., 2014, 79, 4590–4601 CrossRef CAS PubMed.
- E. Faggi, C. Vicent, S. V. Luis and I. Alfonso, Org. Biomol. Chem., 2015, 13, 11721–11731 RSC.
- L. Tapia, Y. Pérez, J. Solà, S. V. Luis, I. Alfonso and C. Vicent, Analyst, 2022, 147, 5546–5556 RSC.
- N. Solozabal, L. Tapia, J. Solà, Y. Pérez and I. Alfonso, Bioconjug. Chem., 2023, 34, 2345–2357 CrossRef CAS PubMed.
- M. D. Haskell, J. K. Slack, J. T. Parsons and S. J. Parsons, Chem. Rev., 2001, 101, 2425–2440 CrossRef CAS PubMed.
- F. H. Igney and P. H. Krammer, Nat. Rev. Cancer, 2002, 2, 277–288 CrossRef CAS PubMed.
- E. Faggi, Y. Pérez, S. V. Luis and I. Alfonso, Chem. Commun., 2016, 52, 8142–8145 RSC.
- L. Tapia, N. Solozabal, J. Solà, Y. Pérez, W. T. Miller and I. Alfonso, Chem. – Eur. J., 2021, 27, 9542–9549 CrossRef CAS PubMed.
- V. C. Yan, H. E. Butterfield, A. H. Poral, M. J. Yan, K. L. Yang, C.-D. Pham and F. L. Muller, Trends Cancer, 2020, 6, 924–941 CrossRef CAS PubMed.
- T. J. Mooibroek, J. M. Casas-Solvas, R. L. Harniman, C. M. Renney, T. S. Carter, M. P. Crump and A. P. Davis, Nat. Chem., 2016, 8, 69–74 CrossRef CAS PubMed.
- A. Berdiaki, M. Neagu, E.-M. Giatagana, A. Kuskov, A. M. Tsatsakis, G. N. Tzanakakis and D. Nikitovic, Biomolecules, 2021, 11, 395 CrossRef CAS PubMed.
- E. Kamhi, E. J. Joo, J. S. Dordick and R. J. Linhardt, Biol. Rev., 2013, 88, 928–943 CrossRef PubMed.
- I. Capila and R. J. Linhardt, Angew. Chem., Int. Ed., 2002, 41, 390–412 CrossRef CAS.
- N. K. Karamanos, Z. Piperigkou, A. D. Theocharis, H. Watanabe, M. Franchi, S. Baud, S. Brézillon, M. Götte, A. Passi, D. Vigetti, S. Ricard-Blum, R. D. Sanderson, T. Neill and R. V. Iozzo, Chem. Rev., 2018, 118, 9152–9232 CrossRef CAS PubMed.
- S. Khan, J. Gor, B. Mulloy and S. J. Perkins, J. Mol. Biol., 2010, 395, 504–521 CrossRef CAS PubMed.
- N. S. Gandhi and R. L. Mancera, Drug Discovery Today, 2010, 15, 1058–1069 CrossRef CAS PubMed.
- D. Imberti, W. Ageno, R. Manfredini, F. Fabbian, R. Salmi, R. Duce and M. Gallerani, Thromb. Res., 2012, 129, 418–425 CrossRef CAS PubMed.
- C. Tieken and H. H. Versteeg, Thromb. Res., 2016, 140, 148–153 CrossRef PubMed.
- C. C. La, L. E. Takeuchi, S. Abbina, S. Vappala, U. Abbasi and J. N. Kizhakkedathu, Biomacromolecules, 2020, 21, 2595–2621 CrossRef CAS PubMed.
- P. Gans, A. Sabatini and A. Vacca, Talanta, 1996, 43, 1739–1753 CrossRef CAS PubMed.
- C. Bazzicalupi, A. Bencini, A. Bianchi, M. Cecchi, B. Escuder, V. Fusi, E. Garcia-España, C. Giorgi, S. V. Luis, G. Maccagni, V. Marcelino, P. Paoletti and B. Valtancoli, J. Am. Chem. Soc., 1999, 121, 6807–6815 CrossRef CAS.
- A. De Robertis, C. De Stefano, A. Gianguzza and S. Sammartano, Talanta, 1999, 48, 119–126 CrossRef CAS PubMed.
- M. Corredor, D. Carbajo, C. Domingo, Y. Pérez, J. Bujons, A. Messeguer and I. Alfonso, Angew. Chem., Int. Ed., 2018, 57, 11973–11977 CrossRef CAS PubMed.
- D. Carbajo, Y. Pérez, M. Guerra-Rebollo, E. Prats, J. Bujons and I. Alfonso, J. Med. Chem., 2022, 65, 4865–4877 CrossRef CAS PubMed.
- N. Fujitani, J. Furukawa, K. Araki, T. Fujioka, Y. Takegawa, J. Piao, T. Nishioka, T. Tamura, T. Nikaido, M. Ito, Y. Nakamura and Y. Shinohara, Proc. Natl. Acad. Sci., 2013, 110, 2105–2110 CrossRef CAS PubMed.
- A. Pudełko, G. Wisowski, K. Olczyk and E. M. Koźma, FEBS J., 2019, 286, 1815–1837 CrossRef PubMed.
- A. H. Bartlett and P. W. Park, Expert Rev. Mol. Med., 2010, 12, e5 CrossRef PubMed.
- D. Carbajo, Y. Pérez, J. Bujons and I. Alfonso, Angew. Chem., Int. Ed., 2020, 59, 17202–17206 CrossRef CAS PubMed.
- S. Liu, Q. Zhang, A. N. Shy, M. Yi, H. He, S. Lu and B. Xu, J. Am. Chem. Soc., 2021, 143, 15852–15862 CrossRef CAS PubMed.
- S. Liu, Q. Zhang, H. He, M. Yi, W. Tan, J. Guo and B. Xu, Angew. Chem., Int. Ed., 2022, 61, e202210568 CrossRef CAS PubMed.
- F. Li, Y. Liu, Y. Dong, Y. Chu, N. Song and D. Yang, J. Am. Chem. Soc., 2022, 144, 4667–4677 CrossRef CAS PubMed.
- S. Kim, B. Jana, E. M. Go, J. E. Lee, S. Jin, E.-K. An, J. Hwang, Y. Sim, S. Son, D. Kim, C. Kim, J.-O. Jin, S. K. Kwak and J.-H. Ryu, ACS Nano, 2021, 15, 14492–14508 CrossRef CAS PubMed.
- S. Kim, J.-B. Chae, D. Kim, C.-W. Park, Y. Sim, H. Lee, G. Park, J. Lee, S. Hong, B. Jana, C. Kim, H. Chung and J.-H. Ryu, J. Am. Chem. Soc., 2023, 145, 21991–22008 CrossRef CAS PubMed.
- M. T. Jeena, L. Palanikumar, E. M. Go, I. Kim, M. G. Kang, S. Lee, S. Park, H. Choi, C. Kim, S.-M. Jin, S. C. Bae, H. W. Rhee, E. Lee, S. K. Kwak and J.-H. Ryu, Nat. Commun., 2017, 8, 26 CrossRef CAS PubMed.
- C. Jonkergouw, N. K. Beyeh, E. Osmekhina, K. Leskinen, S. M. Taimoory, D. Fedorov, E. Anaya-Plaza, M. A. Kostiainen, J. F. Trant, R. H. A. Ras, P. Saavalainen and M. B. Linder, Nat. Commun., 2023, 14, 2141 CrossRef CAS PubMed.
- O. I. V. Luotonen, E. Osmekhina, E. Anaya-Plaza, S. Kaabel, A. L. Harmat, M. Sammalkorpi, C. Jonkergouw, M. B. Linder and M. A. Kostiainen, Cell Rep. Phys. Sci., 2024, 5, 102089 CrossRef CAS.
- L. S. J. Roope, R. D. Smith, K. B. Pouwels, J. Buchanan, L. Abel, P. Eibich, C. C. Butler, P. S. Tan, A. S. Walker, J. V. Robotham and S. Wordsworth, Science, 2019, 364, eaau4679 CrossRef CAS PubMed.
- C. J. L. Murray, K. S. Ikuta, F. Sharara, L. Swetschinski, G. Robles Aguilar, A. Gray, C. Han, C. Bisignano, P. Rao, E. Wool, S. C. Johnson, A. J. Browne, M. G. Chipeta, F. Fell, S. Hackett, G. Haines-Woodhouse, B. H. Kashef Hamadani, E. A. P. Kumaran, B. McManigal, S. Achalapong, R. Agarwal, S. Akech, S. Albertson, J. Amuasi, J. Andrews, A. Aravkin, E. Ashley, F.-X. Babin, F. Bailey, S. Baker, B. Basnyat, A. Bekker, R. Bender, J. A. Berkley, A. Bethou, J. Bielicki, S. Boonkasidecha, J. Bukosia, C. Carvalheiro, C. Castañeda-Orjuela, V. Chansamouth, S. Chaurasia, S. Chiurchiù, F. Chowdhury, R. Clotaire Donatien, A. J. Cook, B. Cooper, T. R. Cressey, E. Criollo-Mora, M. Cunningham, S. Darboe, N. P. J. Day, M. De Luca, K. Dokova, A. Dramowski, S. J. Dunachie, T. Duong Bich, T. Eckmanns, D. Eibach, A. Emami, N. Feasey, N. Fisher-Pearson, K. Forrest, C. Garcia, D. Garrett, P. Gastmeier, A. Z. Giref, R. C. Greer, V. Gupta, S. Haller, A. Haselbeck, S. I. Hay, M. Holm, S. Hopkins, Y. Hsia, K. C. Iregbu, J. Jacobs, D. Jarovsky, F. Javanmardi, A. W. J. Jenney, M. Khorana, S. Khusuwan, N. Kissoon, E. Kobeissi, T. Kostyanev, F. Krapp, R. Krumkamp, A. Kumar, H. H. Kyu, C. Lim, K. Lim, D. Limmathurotsakul, M. J. Loftus, M. Lunn, J. Ma, A. Manoharan, F. Marks, J. May, M. Mayxay, N. Mturi, T. Munera-Huertas, P. Musicha, L. A. Musila, M. M. Mussi-Pinhata, R. N. Naidu, T. Nakamura, R. Nanavati, S. Nangia, P. Newton, C. Ngoun, A. Novotney, D. Nwakanma, C. W. Obiero, T. J. Ochoa, A. Olivas-Martinez, P. Olliaro, E. Ooko, E. Ortiz-Brizuela, P. Ounchanum, G. D. Pak, J. L. Paredes, A. Y. Peleg, C. Perrone, T. Phe, K. Phommasone, N. Plakkal, A. Ponce-de-Leon, M. Raad, T. Ramdin, S. Rattanavong, A. Riddell, T. Roberts, J. V. Robotham, A. Roca, V. D. Rosenthal, K. E. Rudd, N. Russell, H. S. Sader, W. Saengchan, J. Schnall, J. A. G. Scott, S. Seekaew, M. Sharland, M. Shivamallappa, J. Sifuentes-Osornio, A. J. Simpson, N. Steenkeste, A. J. Stewardson, T. Stoeva, N. Tasak, A. Thaiprakong, G. Thwaites, C. Tigoi, C. Turner, P. Turner, H. R. van Doorn, S. Velaphi, A. Vongpradith, M. Vongsouvath, H. Vu, T. Walsh, J. L. Walson, S. Waner, T. Wangrangsimakul, P. Wannapinij, T. Wozniak, T. E. M. W. Young Sharma, K. C. Yu, P. Zheng, B. Sartorius, A. D. Lopez, A. Stergachis, C. Moore, C. Dolecek and M. Naghavi, Lancet, 2022, 399, 629–655 CrossRef CAS PubMed.
|
This journal is © The Royal Society of Chemistry 2024 |
Click here to see how this site uses Cookies. View our privacy policy here.