DOI:
10.1039/D4CC03875D
(Feature Article)
Chem. Commun., 2024,
60, 14346-14359
Surface coverage and reconstruction analyses bridge the correlation between structure and activity for electrocatalysis
Received
31st July 2024
, Accepted 27th September 2024
First published on 18th November 2024
Abstract
Electrocatalysis is key to realizing a sustainable future for our society. However, the complex interface between electrocatalysts and electrolytes presents an ongoing challenge in electrocatalysis, hindering the accurate identification of effective/authentic structure–activity relationships and determination of favourable reaction mechanisms. Surface coverage and reconstruction analyses of electrocatalysts are important to address each conjecture and/or conflicting viewpoint on surface-active phases and their corresponding electrocatalytic origin, i.e., so-called structure–activity relationships. In this review, we emphasize the importance of surface states in electrocatalysis experimentally and theoretically, providing guidelines for research practices in discovering promising electrocatalysts. Then, we summarize some recent progress of how surface states determine the adsorption strengths and reaction mechanisms of occurring electrocatalytic reactions, exemplified in the electrochemical oxygen evolution reaction, oxygen reduction reaction, nitrogen reduction reaction, CO2 reduction reaction, CO2 and N2 co-reductions, and hydrogen evolution reaction. Finally, the review proposes deep insights into the in situ study of surface states, their efficient building and the application of surface Pourbaix diagrams. This review will accelerate the development of electrocatalysts and electrocatalysis theory by arousing broad consensus on the significance of surface states.
Introduction
Facing the global energy crises and climate changes, our society is witnessing a desirable revolution of energy infrastructures. Electrocatalysis has great potential to bring us a bright and sustainable future with the target of using renewable resources to convert low-cost feedstocks into value-added chemicals.1–11 Electrochemical water splitting can produce green hydrogen and oxygen.12–14 Meanwhile, electrochemical oxygen reduction can convert hydrogen into water without any carbon emissions and simultaneously release electric energy.11,15–17 The electrochemical nitrogen reduction can catalyze gaseous nitrogen into ammonia under ambient conditions using green electricity without the harsh reaction conditions of the Haber–Boash method.18–22 The mitigation of unprecedented atmospheric CO2 levels can also be achieved through electrocatalytic CO2 reduction into carbon-containing fuels.23–25 Furthermore, the coupling of electrocatalytic N2 and CO2 co-reduction can be employed to produce urea.26 Therefore, in past decades, tremendous efforts have been made toward the discovery of promising electrocatalysts, as the core of electrochemical systems, and the development of electrocatalysis theory to guide the rational design of electrocatalyst materials.
The industrial application of electrocatalysis requires electrocatalytic materials with high performance. Currently, various strategies have been developed to design highly efficient and stable electrocatalysts, including defect engineering,27–29 alloy engineering,30,31 strain engineering,32 heterostructure engineering,33,34etc. These methods have promoted the activity and selectivity of electrocatalysts, although many catalysts cannot reach the required industrial levels. However, their structure–activity relationships remain obscure because surface-active phases with meticulous design are usually different from the resting surface states (i.e., electrochemistry-induced surface coverages and/or the subsequent reconstruction) of catalysts under in situ conditions.35 Due to the electrochemically-driven water activation and ion deposition/dissolution at catalytic interfaces, newly-evolved surface states will emerge with the pre-coverage of some species,36–43 even resulting from surface reconstructions induced by existing defects (e.g., oxygen and phosphorous vacancies).44,45 Moreover, the water environment can oxidize catalytic materials (like MXenes) to form new species over surfaces.46 Such complex phenomena complicate the understanding of the origin of electrocatalysis and then limit the advance of electrocatalysts.
In very recent years, the surface state analysis of electrocatalysts has begun attracting attention in the field of electrocatalysis.36,39,43,44,47–53 In experiments, researchers have found different surface coverages and newly-formed surface-active phases under electrocatalysis compared to their as-designed surfaces.54–58 These new surface states are highly correlated to their delivered performance. Although some advanced in situ and post-reaction characterizations can provide helpful information on surface species and electronic valence of active sites,59–61 uncovering surface coverages and precise atomic structures/active sites is challenging. Fortunately, the surface Pourbaix diagrams, first proposed in electrocatalysts in the year 2008,62 have demonstrated the capacity to identify the most energetically-favourable surface states of electrocatalysts under electrocatalytic conditions, which is really helpful to the building of authentic structure–activity relationships. This will be discussed later in detail in this review work. Additionally, the presence of extensive anionic deficiency on surfaces will lead to surface reconstructions, forming new surface-active phases. Based on these scenarios, very recently, we proposed a standard research paradigm for structure–activity relationships in the investigation of electrocatalytic CO2 reduction over SnO2, which is also applicable to other electrocatalytic reactions.44
In this review, we will initially discuss how to find stable catalyst surfaces, i.e., surface states, and emphasize the core position of surface state analysis in building structure–activity relationships for electrocatalysis systems. In experiments, the in situ characterizations may still provide limited information on the understanding of surface-active species at atomic levels but can act as an important supplement for density functional theory (DFT)-based theoretical studies. Then we will discuss how surface states determine the adsorption of reaction intermediates and reaction mechanisms rather than as-synthesized surfaces through some electrochemical reactions. Finally, we propose deep insights into the in situ study of surface states, the fast and effective building of surface Pourbaix diagrams, and other applications of them.
The basic research framework for electrocatalysis
Currently, numerous electrocatalysts have been experimentally tested or theoretically screened for accelerating the energy conversion efficiency and reaction kinetics. However, authentic structure–activity relationships existing in different electrocatalytic systems remain obscure although many exciting catalysts have been claimed or predicted. The primary challenge lies in the unknown resting surface of electrocatalysts, stemming from the insufficient consideration of surface state analysis. Our group emphasized the necessity of analysing surface states through different electrochemical reactions with various classic materials, including dual-atom catalysts37 and metal oxides/nitrides/sulfides.36,39,44,48,49 In the meantime, García-Melchor and Ciotti have also discussed the importance of surface coverages of electrocatalysts under reaction conditions and that unphysical models may decelerate the discovery process of more effective and cheaper electrocatalysts.43 To provide a guideline/framework to uncover structure–activity relationships in the community of electrocatalysis, here the key research ingredients for density functional theory (DFT)-based and experimental studies are emphasized as shown in Fig. 1. As for the DFT-based studies, the surface Pourbaix diagrams should emerge as a fundamental tool to illustrate electrochemically-induced surface coverages; then sometimes, some surface species could induce the surface reconstruction to form a new phase, thus molecular dynamics (MD) simulations using various algorithms, like the constrained genetic algorithm,63,64 are needed to obtain the most stable surface phase and the evolution process of active sites. Furthermore, the MD simulation of explicit interfacial models is an effective scheme to study the surface states.46,65,66 Certainly, the surface reconstructions of electrocatalysts can be affected by many factors, including fluctuating electrolyte pH, temperature, applied potentials, and ions. Therefore, advancing more precise MD methodologies that incorporate the aforementioned factors is highly sought after, yet remains a formidable challenge. Following surface state analysis, the analysis of electrocatalytic mechanisms, including thermodynamics and kinetics, can then be conducted based on the real/resting surface states under relevant reaction conditions. In experiments, to illustrate the structure–activity relationship, it is necessary to precisely synthesize the electrocatalysts with controllable surface species and compositions, and coordination environments. Nevertheless, the surface state of active sites during electrochemical reactions may be more complex than typically assumed or designed, further complicating the identification of active sites. Therefore, during the electrocatalytic process, in situ advanced characterization techniques should be employed to understand the changes in electronic valences and surface compositions,67 which determine the activity and selectivity of electrocatalysis. Although in situ characterization techniques have been widely employed in various electrocatalytic systems, theoretical DFT-based investigation can provide powerful support to dictate the surface-active phase and the atomic-level understanding of reaction mechanisms. In elucidating structure–activity correlations, the integration of theoretical and experimental approaches is essential to advance the design of efficient electrocatalysts and foster the progression of electrocatalysis theory. In the following section, we will emphasize how surface state analysis aids in decreasing the discrepancy in different electrocatalytic systems, particularly boosting the understanding of surface-active structures and their corresponding performance, including the electrochemical oxygen evolution reaction (OER), oxygen reduction reaction (ORR), nitrogen reduction reaction (NRR), CO2 reduction reaction (CO2RR), CO2 and N2 co-reductions, and hydrogen evolution reaction (HER).
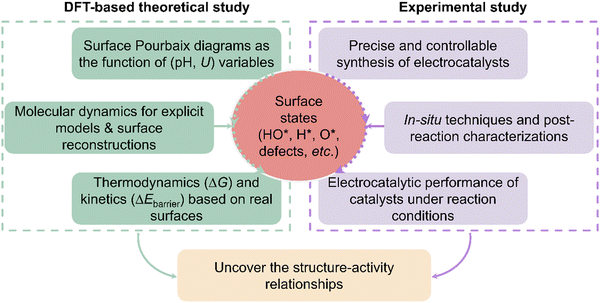 |
| Fig. 1 The proposed basic research framework for theoretical and experimental investigations to build an authentic connection between surface-active structures under the reaction conditions and the delivered performance. | |
Surface state analysis narrows down the discrepancy in electrocatalysis
OER electrocatalysis
The design of low-cost and effective electrocatalysts for water electrolysis is an open challenge. Many studies have suggested that the OER usually suffers from sluggish kinetics and instability,13,68–72 significantly making the practical implementation of electrochemical water splitting difficult and inefficient. Very recently, our group employed a doping strategy to design a series of electrocatalysts based on modified zirconium dioxide (ZrO2) with excellent thermal stability, identifying that single-atom Fe and Rh dopants showed remarkable activity enhancement towards the OER.73 The study provides crucial insights into the design and development of stable, high-activity, and low-cost OER electrocatalysts for water splitting. However, the industrial-scale application of OER electrocatalysts still faces the bottleneck of easily-changed structure–activity relationships, because the interaction between catalysts and electrolyte media is very complicated at large current densities. Achieving long-term stability and high catalytic activity requires a comprehensive understanding of electrochemically-induced surface-active structures and their impact on performance. For example, with knowledge of the surface-catalytic structure–activity relationship, Gu et al.74 tuned the surface structure of FeNi nanoparticles by rational thermal oxidation and/or reductive approaches for the OER, finding that the surface structure of catalysts in a fully oxidized or metallic state is not active, whereas the mixed-phase FeNi alloys demonstrate superior catalytic performance due to the optimized valence states of the Fe/Ni species. Bhattacharyya et al.75 employed the finite cluster models combined with constant potential-DFT and implicit solvation methods to investigate the impact of Fe substitution in cobalt(oxy)hydroxide for the OER. The surface states of the cluster catalyst models reveal that cobalt(oxy)hydroxide predominantly carries a positive charge under alkaline conditions, with nearly complete coverage of hydroxyl ligands coordinated to the Co center. This surface configuration represents the active catalytic phase for the OER. Additionally, the constant potential approach shows that at lower potentials, the O–O bond preferentially forms through the coupling of adjacent Co–OH sites, consistent with experimental findings. Substituting a surface Co atom with Fe significantly enhances the ease of oxidation compared to the unmodified structure. The existence of Fe could reduce the H+ affinity of the surface-bound –OH moieties, i.e., increased Fe–OH acidity, consequently decreasing the rate-determining step of the deprotonation of the –OH group. Analysis of surface states in finite cluster models confirms experimental results and offers deeper insights into the structure–activity relationship for the OER.
Pan et al.76 synthesized a highly efficient, stable noble-metal-free electrocatalyst to address the limitations in OER activity and stability. The as-synthesized Mn7.5O10Br3 catalysts can achieve a low overpotential of ∼295 mV at 10 mA cm−2 and meanwhile maintain good stability operating for >500 h. DFT-calculated bulk Pourbaix diagrams reveal that the activity and stability of Mn7.5O10Br3 arise from the formation of a MnOx passivation layer on the surface under reaction conditions (Fig. 2(a)). Compared to Mn7.5O10Br3, Mn8O10Cl3 requires a higher operational potential, resulting in a greater thermodynamic driving force to decompose. This explains why Mn–O–Br materials possess superior stability over Mn–O–Cl materials (Fig. 2(b)). The developed microkinetic volcano model predicts that the Mn–O–Br material exhibits the highest OER activity as a function of GO − GHO (Fig. 2(c)). This study reveals that the surface-active phase of Mn7.5O10Br3 enhances both stability and OER activity.
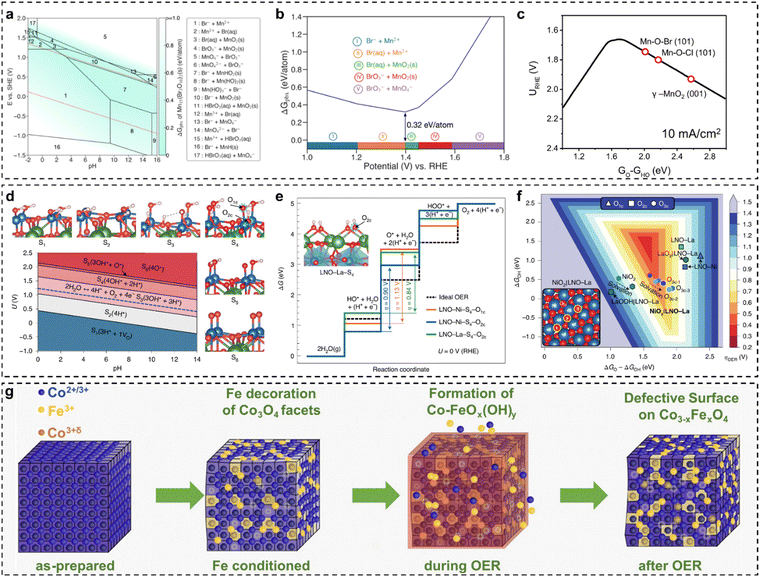 |
| Fig. 2 (a) Calculated Mn–O–Br Pourbaix diagrams with an ion concentration of 10−4 M at 298.15 K (note: the Mn ion concentration is based on the ICP-OES result, and the Lake blue colour indicates the stability of Mn7.5O10Br3); (b) calculated Pourbaix decomposition free-energy (ΔGpbx) of Mn7.5O10Br3 and the projection of ΔGpbx onto the potential axis suggests the stable species at corresponding potential regions; (c) the OER activity volcano diagram at 10 mA cm−2 as the function of GO − GHO.76 (d) Surface Pourbaix diagram of LNO–Ni (labels from S1 to S6 designate surface coverages from more reduced to more oxidized, VO < H < OH < O, VO is an oxygen vacancy); (e) calculated OER free-energy diagrams for LNO–Ni–S4 at the top site (orange) and at the bridge site (blue), and for LNO–La–S4 at the bridge site (green), are compared with an ideal catalyst (dashed line) at no bias; (f) 2D OER activity volcano map of overpotentials as the function of ΔGO − ΔGOH and ΔGOH.54 (g) Schematic diagram of the Fe interaction with cubic Co3O4 nanoparticles during the OER.56 | |
Baeumer et al.54 demonstrated how electrochemically driven surface transformations influence the identification of surface-active phases and structure–activity relationships in (001)-terminated LaNiO3 (LNO) epitaxial thin films, aiding the development of targeted OER electrocatalysts. The study showed that the Ni termination of the as-prepared state was more active than the La termination, with an overpotential difference of ∼150 mV. The surface Pourbaix diagram of the bulk LNO–Ni, as shown in Fig. 2(d), indicated that as the voltage increased, the LNO–Ni surface transitioned from reduced to more oxidized coverages. Under OER-relevant biases, the Ni-terminated surface was predominantly covered by 1 ML H* on singly coordinated O1C sites and 1/2 ML H* on doubly coordinated O2C sites. Notably, a voltage exceeding 2 V is required to achieve 1 ML
(LNO–Ni–S6). Based on surface state results, the authors assessed the theoretical OER activity of LNO with various terminations, as illustrated in Fig. 2(e). Using the stable S4 coverages for both LNO–Ni and LNO–La, it was determined that the HO* → O* transition is the potential-limiting step on both surfaces. Specifically, on LNO–Ni, the thermodynamic overpotential for the OER was η = 1.13 V at O1C and 0.90 V at O2C, while on LNO–La, there was only O2C with 0.84 V. Although LNO–La was slightly more active than LNO–Ni, both perovskite-type LaO and NiO bulk LNO(001) exhibited unfavourable O* intermediates, driving the metal sites into unfavourable Ni4+ and La3.5+ oxidation states, which contribute to the high overpotentials. Shortly, LNO(001) terminations under OER conditions tend to be highly covered by HO* and achieve O* at higher overpotentials. Theoretical OER overpotential volcano mapping (Fig. 2(f)) shows that bulk LNO(001) terminations, including LNO–Ni–S4 and LNO–La–S4, exhibit weak O* adsorption. However, transitioning to NiO2|LNO–La from the as-prepared LNO–Ni surfaces significantly reduces the overpotentials. The layered NiO2 overlayers, with their triple-coordinated O3C sites, stabilize O* and HO*, resulting in a shift to a lower and more centered position in the 2D volcano diagram. Finally, it is concluded that electrochemically driven Ni oxyhydroxide-like surfaces enhance electrochemical activity, with surface state analysis confirming the stability of the NiO2/LNO–La surface under OER conditions. Moreover, Haase et al.56 uncovered the link between surface-active structures and the enhancement of Fe decoration over Co3O4 nano-catalysts. By leveraging various operando spectroscopic techniques, the authors demonstrated that Fe decoration enhanced catalytic activity and facilitated the formation of a near-surface-active state, as shown in Fig. 2(g). Fe decoration led to observable near-surface structural transformations in the Co3O4 coherence length, indicating the formation of a Co–Fe (oxyhydr)oxide. Furthermore, in the presence of Fe decoration, the extent of Co–O near-surface oxidation was less pronounced. Incorporating Fe into a near-surface Co–Fe (oxyhydr)oxide increased the active site density, resulting in enhanced apparent OER activity. The authors also discovered that the Fe ions in the Co3−xFexO4 near-surface experienced a redox transition and accumulated oxidative charges during the OER. Following the OER, Fe incorporation into Fe-decorated Co3O4 nano-catalysts led to the formation of Co3−xFexO4 with metal defects in the spinel near-surface. This work illustrated how in situ methods can elucidate surface state evolution and its impact on electrocatalysis, revealing direct links between surface-active structures and electrochemical activity.
ORR electrocatalysis
Fuel cell technology has the promise of transforming energy infrastructures and significantly lowering carbon emissions, paving the way for a more sustainable and environmentally responsible energy future.11,77–83 Nonetheless, the structure–activity relationships become more complex due to the adsorption of reaction intermediates on the altered active sites under electrochemical conditions, resulting in discrepancies between theoretically predicted potentials/activities and experimental outcomes. Although fuel cell technology has come a long way, its reliance on Pt-based materials remains a major barrier to large-scale commercialization.84–87 At present, the ORR faces a bottleneck due to the high overpotential at cathodic electrodes, which in turn reduces the overall energy efficiency of fuel cell systems. Consequently, the development of cost-effective, stable, and efficient alternatives to Pt-group materials is a critical objective. In 2022, Kelly et al.88 incorporated field effects into the computational hydrogen electrode model to explain ORR activity trends over Pt(111), Au(111), and Au(100). They found minimal electric field influence on Pt(111), where *OH removal limits the 4e− ORR, but a significant field dependence on Au(111) and Au(100), where *OOH formation is rate-limiting. These findings aligned with experimentally observed pH dependencies and highlighted the potential of considering electric field effects to identify more effective ORR catalysts.
Transition metal oxides (TMOs) face intrinsic limitations in the ORR due to the slow O–O bond-breaking step caused by the large dipole moment of the *O intermediate.77 However, Yuan et al.89 demonstrated that low-cost, versatile zirconium nitride (ZrN) catalysts exhibit superior 4e− ORR performance, even surpassing Pt in alkaline conditions. Despite ZrN's superior ORR activity, its precise mechanism and performance origin remained unclear. To address this, Liu et al.36 employed surface state analysis, electric field effect simulations, and pH-dependent microkinetic modelling to investigate the underlying causes. To explore the origin of ORR performance, the surface Pourbaix diagram for ZrN(100) was calculated (Fig. 3(a)). Under alkaline ORR conditions (pH = 13) and 0.8 VRHE, ZrN(100) is predicted to be covered by 1 ML HO*. The 2D Pourbaix diagram (Fig. 3(b)) further indicates that this HO* coverage persists across a broad pH range, with the HO*-covered surface shown in Fig. 3(c). Next, the electric field effects on ORR intermediates were examined for both pristine and 1 ML HO*-covered ZrN(100) surfaces (Fig. 3(d) and (e)). It was found that the intrinsic dipole moment (μ) and polarizability (α) of O* are significantly reduced on the 1 ML HO*-covered ZrN(100) surface (Fig. 3(e)). The dipole properties of O* are crucial for ORR activity; an ideal catalyst should exhibit minimal dipole moment and polarizability. A larger dipole moment for O* leads to weaker O* bonding, which hinders O–O bond activation and slows the ORR.77 The pH-dependent volcano activity model (Fig. 3(f)) demonstrates that the 1 ML HO*-covered ZrN(100) exhibits exceptional ORR activity, aligning with experimental observations. The analysis result indicates the importance of determining surface states beforehand. Furthermore, the surface transformation under electrochemical conditions was extended to the Fe3N, TiN, and HfN in that these three transition metal nitrides had proven to be active in the ORR/OER. Their corresponding 2D surface Pourbaix diagrams are displayed in Fig. 3(g)–(i). All three surfaces underwent electrochemical oxidation under relevant conditions, with their atomic surface structures depicted in Fig. 3(j). These transformed surface-active phases are responsible for the activity in alkaline media, which is consistent with the experimental results.
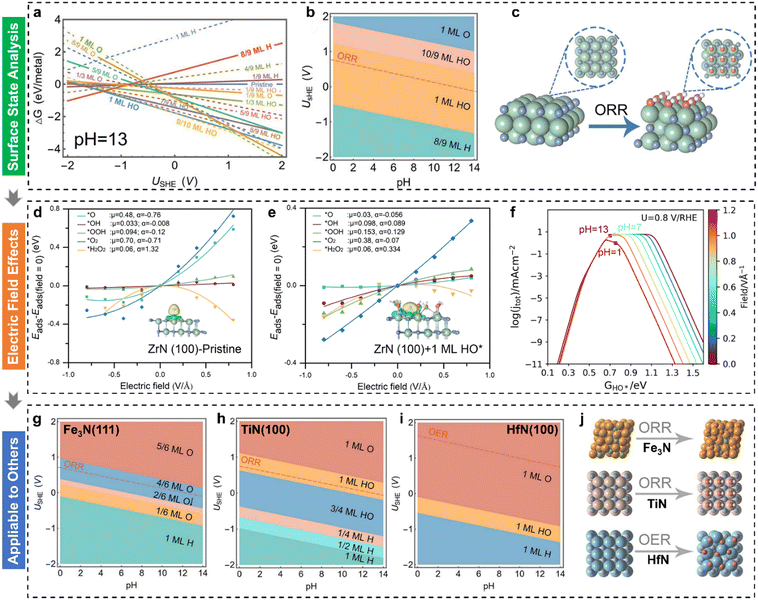 |
| Fig. 3 (a) Calculated 1D surface Pourbaix diagram as a function of applied potential (USHE) (T = 298.15 K, pH = 13); (b) calculated 2D surface Pourbaix diagram as a function of USHE and pH (T = 298.15 K); (c) optimized ZrN(100) surface structures before and after the coverage of *OH under the ORR; electric field effects on ORR intermediates with fitted parameters for (d) pristine and (e) 1 ML HO*-covered ZrN(100); (f) derived pH-dependent volcano activity model for the ORR as a function of HO* adsorption; calculated 2D surface Pourbaix diagrams as a function of USHE and pH (T = 298.15 K) for (g) Fe3N(111), (h) TiN(100), and (i) HfN(100), respectively; (j) corresponding atomic structure evolutions under ORR conditions.36 | |
NRR electrocatalysis
The nitrogen reduction reaction (NRR) under ambient conditions is viewed as a promising alternative to the industrial Haber–Bosch process, enabling the conversion of inert N2 into valuable NH3.90–94 A major challenge of the NRR lies in the scarcity of highly efficient catalysts for facilitating N2 fixation and subsequent hydrogenation. Currently, a wide range of electrocatalysts have been investigated to enhance N2 adsorption and activation, including transition metal-based materials,20,95 p-block-element-based materials,96–101 single-atom materials,102–108 dual-atom materials,109–114 and cluster metal catalysts.115,116 However, due to the lack of in-depth understanding of surface states, the authentic origin of the electrochemical NRR activity remains obscure. We have suggested that the surface states of dual-atom catalysts usually deviated from the pristine surface under electrocatalytic conditions,37 and that dual-atom catalysts may be covered by some species at the NRR window.114 Surface-induced modifications by adsorbed species can reshape the geometric configurations and alter the electronic structures of active sites, leading to variations in reaction mechanisms.
MBenes, as analogs of MXenes, have drawn extensive attention in electrocatalysis. Their surface stability under electrochemical conditions poses a significant challenge, as they are prone to oxidation. Varying surface terminations can markedly affect surface properties and reaction activity. Using Zr2B2 and Hf2B2 as examples, Gao et al.117 examined their surface states under typical NRR conditions, revealing that both MBenes become fully terminated by oxygen groups. Additionally, as the pH of the electrolyte increases, a more negative USHE potential is required to prevent the oxidation of the bare Zr2B2 and Hf2B2 surfaces. Furthermore, various single-atom metals were doped into the oxidized surfaces containing Zr(Hf) vacancies. Among these, h-Zr2B2O2–Cr exhibited the highest NRR activity, with the *NH2 → *NH3 transition identified as the potential-determining step, characterized by a maximum ΔG of 0.10 eV. However, this study overlooks the surface states of the newly formed doped surfaces, adding uncertainty to the performance of these electrocatalysts under actual reaction conditions.
Recently, our group explored the underlying mechanisms behind the exceptional performance of less-precious FeS2 catalysts for the NRR, uncovering the widespread occurrence of in situ sulfur vacancy formation in transition metal disulfides (TMS2) during electrochemical processes.39 First, according to a summary of typical TMS2 NRR catalysts reported in the literature (Fig. 4(a)), it can be seen that these TMS2 preferentially achieve the highest FE or experience a rapid FE growth at a relatively low potential (i.e., −0.4 VRHE). To gain deeper insights into the enhanced NRR performance and guide the design of modified TMS2 materials, our group selected the FeS2(111) surface—a frequently reported facet known for its higher thermodynamic stability—as a model system to interpret the experimental observations in NRR studies. Fig. 4(b) indicates that the FeS2 surface decorated with a single S vacancy is the most stable configuration within the NRR-favored potential range (e.g., ∼0.5 VRHE), suggesting that the S-vacancy can be generated in situ on the catalytic surface under these conditions. The formation of S vacancies alters the coordination environment of surface-active sites, leading to distinct NRR catalytic behaviours. Consequently, the entire NRR process on both pristine and single S-vacancy decorated FeS2 surfaces has been modelled, as illustrated in Fig. 4(c). It is seen that the S-vacancy has the excellent ability to adsorb N2, but the release of the second NH3 molecule is significantly endothermic (ΔG = 1.73 eV). The Fe near the S-vacancy site can also activate N2 and catalyze the NRR as an active site with the *N2 → *NNH step as the potential-determining step (ΔG = 1.02 eV). Compared with the stoichiometric pristine FeS2(111), the in situ generation of an S-vacancy largely boosts the NRR activity and is stable for continuous NH3 production. In addition to the FeS2(111) studied, other TMS2 materials, including MoS2(100), NiS2(210), VS2(001), SnS2(111), and VS2(011), were also investigated for the surface state analysis (Fig. 4(d)). As shown, the in situ formation of S vacancies on TMS2 surfaces is energetically favourable under NRR conditions. Moreover, Fig. 4(e) demonstrates the operating potentials of reported TMS2-based materials at their highest NRR FEs, which are all located in our predicted potential windows of the S-vacancy generation. Thus, the in situ generation of S vacancies is responsible for the observed NRR performance of TMS2 catalysts.
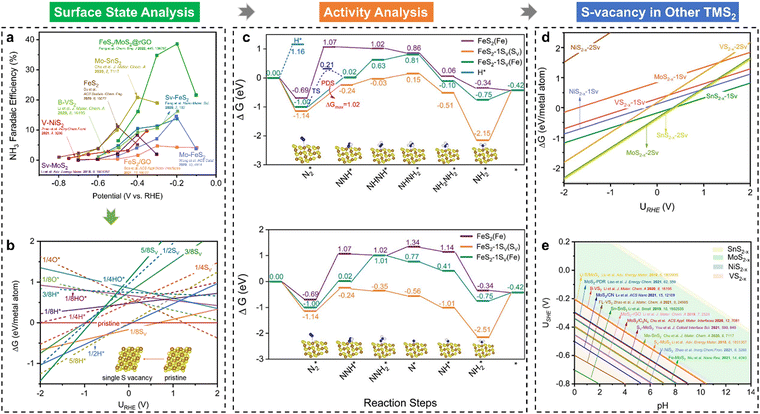 |
| Fig. 4 (a) A summary of the NRR faradaic efficiencies (FEs) of TMS2 under varying applied potentials; (b) 1D surface Pourbaix diagrams of FeS2(111) with the consideration of SV, O*, H*, and HO* groups; (c) Gibbs free energy evolutions of the NRR over FeS2(111) and FeS2−x(111)-1SV surfaces, including the alternating and distal pathways; (d) 1D surface Pourbaix diagrams of different TMS2 surfaces; (e) 2D surface Pourbaix diagram of the SV formation as a function of pH and potential, and the reported experimental operating potentials at the highest NH3 FEs are displayed in the diagram.39 | |
CO2RR electrocatalysis
The depletion of fossil fuels has intensified global climate change by driving atmospheric CO2 levels to unprecedented heights, while their non-renewable nature further underscores the urgent need for a sustainable energy supply to support human development. The electrochemical CO2 reduction reaction (CO2RR) is regarded as an environmentally sustainable and promising strategy to both mitigate excessive CO2 emissions and convert CO2 into value-added chemical feedstocks, particularly when integrated with renewable energy sources.118–124 However, the development of CO2RR electrocatalysts with tuneable activity and selectivity is hindered by the unclear structure–activity relationship that characterizes the CO2RR process. For example, (i) why can the SnO2-based electrocatalysts deliver a high faradaic efficiency of HCOOH rather than CO,125–132 and/or what surface species are accountable for the C1 product distribution?133–139 (ii) Why does C–C coupling over dual-atom catalysts underperform in CO2RR experiments, despite conventional beliefs that dual-atom sites should preferentially facilitate the formation of multi-carbon products?140–144 These critical challenges drive the need for analyzing surface-active phases under relevant conditions or employing in situ surface-sensitive characterization techniques, which can reveal the true origins of the experimental observations.
To understand the CO2RR over SnO2, as one of the Sn-based materials, we proposed a standard research paradigm to uncover the surface-active species and explore their corresponding CO2RR performance.44 The proposed practice has aided in identifying the resting surface states of SnO2 under the conditions of the CO2RR, corroborated by experimental results, and building the structure–activity relationships for HCOOH and CO production. Firstly, we summarized the current origin of the CO2RR over SnO2-based materials for the CO2RR to HCOOH (Fig. 5(a)), including the (i) interfacial effect of grain boundaries, (ii) the existing oxygen vacancy, and (iii) the multivalent Sn. However, the resting/more realistic surface states of SnO2 electrocatalysts are unclear, which is closely linked to the electrochemical performance. Therefore, the surface states of SnO2(110) and (100), commonly exposed surfaces, were analyzed by the surface Pourbaix diagrams as shown in Fig. 5(b). According to the electrochemical conditions where the highest faradaic efficiency was achieved, it was found that there would be lots of oxygen vacancies
over SnO2(110) and (100) with the coverage of 1 ML
. The high density of
then would induce the surface reconstruction to form a new surface-active phase (from u-SnO2−x to s-SnO2−x). In Fig. 5(c), the selectivity analysis suggests that the electrochemically induced
could change the adsorption free energies of both H* and OCHO* (ΔGH*vs. ΔGOCHO*), but still favour the HCOOH formation. Fig. 5(d) displays the HCOOH activity volcano mapping, where s-SnO2−x(110) is the closest to the volcano peak with the highest activity, indicating that the reconstructed surface-active phase, induced by
, enhances the CO2RR to HCOOH, while pristine SnO2 (p-SnO2) possess the lowest HCOOH activity. Furthermore, the CO2RR experiments were performed to validate the theoretically uncovered surface species and reaction mechanisms. Fig. 5(e)–(f) display the high-resolution transmission electron microscope (HRTEM) images of the as-synthesized SnO2 catalysts before and after the CO2RR, evidently indicating that metallic Sn was formed due to the generation of an O-vacancy. In situ Raman (Fig. 5(g)) and attenuated total reflectance-surface enhanced infrared absorption spectroscopy (ATR-SEIRAS) spectra (Fig. 5(h)) directly suggested that the as-synthesized SnO2 catalysts were reduced and the important *OCHO intermediate was generated during the CO2RR, respectively. Finally, a standard research paradigm was proposed by us to uncover the structure–activity relationships in electrocatalysis as shown in Fig. 5(i). To conclude, the study not only uncovers the surface-active species of SnO2 but also provides a standard research paradigm for other electrocatalytic systems. The analysis of surface states and surface reconstructions is indispensable, especially in electrocatalysis.
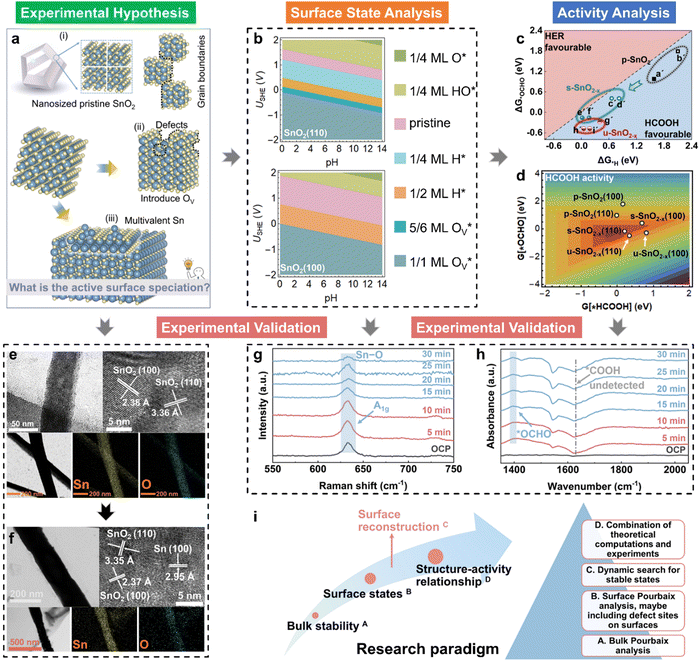 |
| Fig. 5 (a) Proposed origin of active CO2RR over SnO2 into HCOOH, including the interface effects brought by the grain boundaries of (i) nanosized SnO2, (ii) the existing defects (e.g., O vacancies), and (iii) the multivalent Sn (e.g., Sn/SnO/SnO2 heterostructures); (b) calculated 2D surface Pourbaix diagrams of SnO2(110) and (100) with different stable surface states labelled under relevant pH and USHE; (c) HCOOH selectivity analysis with the comparison of ΔG*H and ΔG*OCHO; (d) the HCOOH activity volcano model for SnO2 with different surface states; HRTEM images of the (e) as-synthesized SnO2 and (f) “SnO2-after” samples, and further the energy dispersive spectroscopy (EDS) elemental mapping of Sn and O after the CO2RR; collected in situ (g) Raman and (h) ATR-SEIRAS spectra during the CO2RR on SnO2 with a time slot of 5 min; (i) the standard research roadmap to uncover the structure–activity relationships in electrocatalysis.44 | |
Electrocatalytic C–C coupling represents a crucial advancement in achieving the synthesis of value-added multi-carbon products during the CO2RR.145 However, the dual-atom electrocatalysts, commonly considered as effective electrocatalytic materials for C–C coupling with two active sites for *CO(*CHO) intermediates, hardly catalyzed CO2 into multi-carbon products (see Fig. 6(a)). To study the reason for this unconventional phenomenon, we analyzed the CO2RR mechanisms over typical homonuclear (Fe/Fe–N6–C and Ni/Ni–N6–C) and heteronuclear (Fe/Ni–N6–C) dual-atom catalysts initialized by surface state analysis.48Fig. 6(b)–(d) illustrate their surface Pourbaix diagrams, where it can be seen that all the dual-atom catalysts studied would be covered by pre-adsorbed CO* over the bridge site under CO2RR conditions, in contrast to the unoccupied dual-atom sites. Fig. 6(e) displays the surface states of Fe/Ni–N6–C at different URHE. Notably, at potentials where URHE is below −0.06 V, pre-adsorbed *CO can form in either single- or double-sided configurations. These pre-adsorbed *CO species not only alter the electronic structures of the dual-atom active sites but also modify the underlying CO2RR mechanisms, as demonstrated in Fig. 6(f) and (g), in which the pre-adsorbed CO* in the single-side favoured the CO production and hindered the C–C coupling in both thermodynamics and kinetics and the double-side occupation could lead to the HCOOH formation. As a result of *CO pre-adsorption-induced poisoning, the formation of multi-carbon products is unfavorable on these dual-atom catalysts. Our study reveals why C–C coupling remains difficult although the precise dual-atom sites are designed. Meanwhile, the work also indicates that regulating the adsorption strength of CO* on bridge sites may be an effective way to improve the performance of CO2RR over dual-atom catalysts.
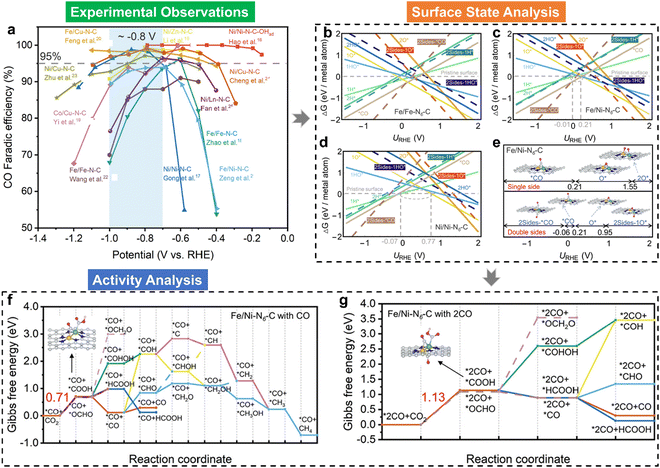 |
| Fig. 6 (a) Comparison of the faradaic efficiency of CO (FECO) for various experimentally-reported dual-atom catalysts, where the FECO data as a function of URHE were obtained from their corresponding literatures; calculated 1D surface Pourbaix diagrams of (b) Fe/Fe–N6–C, (c) Fe/Ni–N6–C, and (d) Ni/Ni–N6–C dual-atom catalysts, respectively, where the solid and dashed lines represent single- and double-side adsorption; (e) the surface states of Fe/Ni–N6–C at various potential windows, where inserts indicate the atomic configurations; Gibbs free-energy evolutions of the CO2RR over Fe/Ni–N6–C with pre-covered *CO at (f) single- and (g) double-sides, respectively.48 | |
CO2 + N2 electrocatalysis for urea
Urea (CO(NH2)2), as the first organic compound derived from inorganic raw materials, is an extremely important material in the chemical industry, and is widely used as N2 fertilizer due to its high nitrogen content (46%). Current industrial urea production relies on extreme reaction conditions, with ammonia predominantly derived from the Haber–Bosch process, which contributes significantly to the energy crisis and exacerbates climate change. Electrochemical urea synthesis via CO2 and N2 coupling under ambient conditions offers a sustainable and highly efficient approach to urea production.146–148 However, the large-scale deployment of this reaction is limited by the lack of effective electrocatalysts and complex reaction mechanisms. Zhu et al.26 theoretically investigated the catalytic activity of experimentally available metal borides (MBenes), i.e., Mo2B2, Ti2B2, and Cr2B2, and analyzed their surface structures/states and activity origin for the simultaneous electrocatalytic coupling of N2 and CO2 to produce urea (Fig. 7). These MBenes catalysts possess metal terminations (Fig. 7(a)), which can act as active sites for the activation of *N2 and *CO2. The urea synthesis mechanism is demonstrated in Fig. 7(b), including the key coupling step of *CO + *N2 → *NCON. As an optimal catalyst candidate, the catalytic surface with the necessary active sites must remain stable and resist coverage by *O/*OH species under operating conditions. Therefore, the surface Pourbaix diagrams of the three MBenes were constructed (Fig. 7(c)–(e)), which revealed that 2D Mo2B2 and Cr2B2 possess high electrochemical stability against the surface oxidation, while the 2D Ti2B2 would be occupied by *O/*OH species under working conditions. Although all three MBenes can effectively activate N2 and CO2 on their basal surface, the *CO intermediate can be easily formed through the *CO2 → *CO process, and the limiting potentials of urea electro-synthesis, comparable to that of Pd–Cu alloys, are in the appropriate range of −0.49 to −0.65 V, the Ti2B2 catalyst is not an optimal candidate for urea production because the active basal plane will be covered by *O/*OH groups. Therefore, this work strongly reaffirms that surface states under operating conditions must be carefully considered when designing active and selective catalysts. The interaction between the designed surface and the electrolyte medium can lead to the formation of new, complex phases, resulting in unanticipated structure–activity relationships that may deviate from initial expectations.
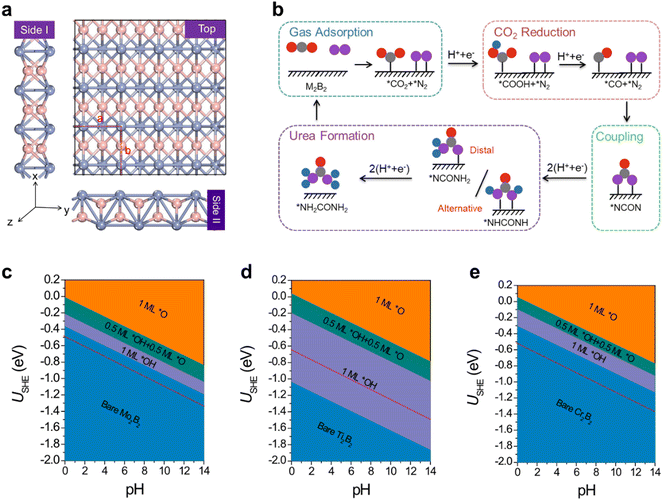 |
| Fig. 7 (a) The top and side views of three MBenes with the red dashed lines of a unit cell (pink: boron atoms; blue: metal atoms); (b) the schematic diagram of the reaction mechanism of urea production through the *CO and *N2 coupling (the gray, red, pink, and blue balls represent C, O, N, and H atoms, respectively); the surface Pourbaix diagrams of (c) Mo2B2, (d) Ti2B2, and (e) Cr2B2 with the SHE and pH as variables and the red dashed line represents the limiting potential of urea electro-synthesis; the thermodynamically stable surface states are highlighted by orange (*O), green (*O + *OH), and purple (*OH).26 | |
HER electrocatalysis
Developing efficient catalysts for the hydrogen evolution reaction (HER) is significant for a sustainable hydrogen economy.149–152 MXenes (transition metal carbides, carbonitrides, and nitrides), an important family of two-dimensional (2D) materials, have drawn tremendous attention for HER electrocatalysis due to the large surface area, hydrophilic nature, fast electron transport, and diverse chemical compositions.150–154 Usually, MXenes are synthesized by etching the A elements in their bulk MAX phases, which leads to the inevitable coverage by O, OH, and/or F atoms.155–162 Additionally, our report has suggested that the pH and potentials of interest can induce different surface-covered species, and even vacancies and H-covered surfaces at a low potential window.40 The coverage of different species/newly-formed active sites can significantly impact the surface properties of MXenes and HER performance. Therefore, Gao et al.151 systematically studied the HER activity of MXenes with the termination of O/OH groups. The surface Pourbaix diagrams indicated that Ti2C, V2C, and Ti3C2 would be terminated by 0.5 ML O* and 0.5 ML HO* groups, while Nb2C and Nb4C3 would be fully terminated by O* groups under the reaction potential window, as shown in Fig. 8(a). Then, the hydrogen adsorption free energy (ΔGH*) at varying coverages over O*-terminated MXenes was calculated, as shown in Fig. 8(b). Ideally, the ΔGH* should be close to 0 for a catalyst with high HER performance. In Fig. 8(b), it is found that the average ΔGH* over the *O-termination of Ti2C for θ ranging from 1/8 to 4/8 is close to 0, indicating that Ti2CO2 could offer the optimal binding strength for H* species over a wide range of hydrogen coverages. Therefore, Ti2CO2 is anticipated to exhibit the highest HER activity among the O*-terminated MXenes studied. The research highlights that O*-termination modulates H adsorption strength, influencing reaction kinetics in the HER.
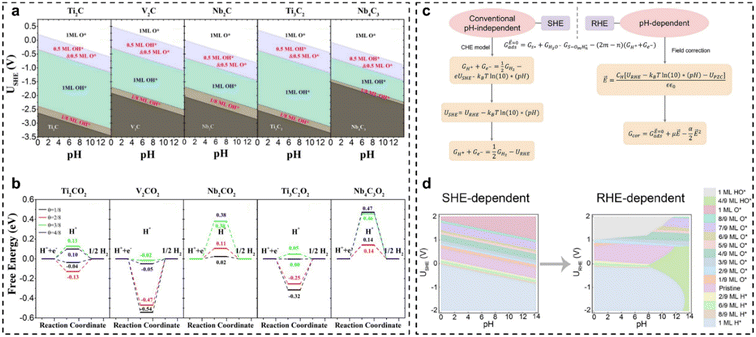 |
| Fig. 8 (a) Calculated surface Pourbaix diagrams of Ti2C, V2C, Nb2C, Ti3C2, and Nb4C3; (b) free-energy evolutions of the HER at different H* coverages (θ = 1/8, 2/8, 3/8, and 4/8) occurring on Ti2CO2, V2CO2, Nb2CO2, Ti3C2O2, and Nb4C3O2 under standard conditions.151 (c) Schematic illustration of the RHE scale-dependent method; (d) calculated classic SHE-dependent and RHE-dependent surface Pourbaix diagrams for Pt(111).50 | |
In catalyst design and electrocatalysis mechanism analysis, the impact of electrochemically induced surface coverage is a crucial factor. The surface Pourbaix diagram is a key tool, offering critical insights into adsorbate coverage from electrochemical potential-driven water activation. However, the classic standard hydrogen electrode (SHE)-dependent surface Pourbaix diagrams are sometimes inconsistent with the experimentally observed surface coverages. This is because some critical factors, illustrated in Fig. 8(c), need to be considered to minimize the discrepancy between theoretical predictions and experimental observations. Within this context, very recently, our group revisited the surface Pourbaix diagram of Pt(111) based on the reversible hydrogen electrode (RHE)-scale by considering the energetics computed by DFT with the Bayesian Error Estimation Functional with van der Waals corrections (BEEF-vdW), the electric field effects, the derived adsorption-induced dipole moment and polarizability, and the potential of zero-charge. As shown in Fig. 8(d), the RHE-dependent surface Pourbaix diagram effectively reduces discrepancies between the theoretical prediction and experimental results for Pt(111),163 particularly under neutral–alkaline, moderate-potential conditions. To be specific, under alkaline HER conditions at slightly negative potentials on the RHE scale, the Pt(111) surface is primarily covered by HO* rather than being pristine or H*-covered, which can be helpful to illustrate the alkaline HER mechanism and design optimum catalysts for accelerated HER rate.
Summary and outlook
In electrocatalysis, surface states of electrocatalysts are complex and often change with the reaction conditions, consequently delivering distinct experimental observations. Thus, effective electrocatalyst design and structure–activity relationship understanding must prioritize surface state analysis, as exemplified in the above-mentioned OER/ORR, NRR, CO2RR, CO2 + N2 electrocatalysis, and HER. As for the surface state analysis, the surface Pourbaix diagram can be analyzed based on the SHE and/or RHE scale. Sometimes, calculating the pH-dependent surface Pourbaix diagram at the RHE scale can minimize the discrepancy between theoretical predictions and experimental observations, particularly under high-pH conditions. In short, the effect of electrochemically-induced surface states should not be overlooked when designing catalysts and identifying electrocatalysis mechanisms.
Additionally, in situ characterization techniques would exert positive effects on the understanding of surface structures, because the application of in situ characterization techniques can provide real-time insights into the evolution of surface states under operational conditions. For example, Chen et al.,55 using operando NAP-XPS, demonstrated that nickel gadolinium-doped ceria cermet electrodes undergo partial reduction, forming a core–shell structure with metallic nickel in the shell and NiO beneath. This structure improved i–V polarization curves. Thus, beyond theoretical DFT frameworks, advanced in situ techniques have significant potential for revealing surface states, bridging the gap between electrocatalysis performance and catalyst surface structures.
Given the importance of surface structures, developing precise and efficient methods for calculating surface Pourbaix diagrams is highly desirable. Currently, surface Pourbaix diagram analysis is prohibitively expensive for real-scale systems.164 To address challenges in large catalytic systems, a bond-type embedded crystal graph convolutional neural network (BECGCNN) model was recently developed.165 With the aid of the BECGCNN model, Bang et al.165 constructed reliable Pourbaix diagrams for large-size nanoparticles (approximately 4.8 nm in diameter, up to 6525 atoms). Additionally, Han et al.166 proposed a Bayesian evolutionary multitasking framework to accelerate the understanding of surface phase diagrams. The integration of Bayesian statistics with evolutionary multitasking enables the efficient generation of surface phase diagrams for complex systems.
Finally, surface state analysis of catalysts has been instrumental in revealing the stability of catalytic materials, greatly aiding in the design of dissolution- and oxidation-resistant catalysts. For example, Li et al.167 discovered that adding Ag atoms to the Pd nanoparticle surface can inhibit surface Pd oxidation and dissolution through surface Pourbaix diagrams. Therefore, surface state analysis under reaction conditions is essential not only for the understanding of the structure–activity relationships, but also for designing optimal catalysts for industrial applications.
Author contributions
Z. G. drafted the manuscript. Z. G., T. W., J. X., A. C., and H. L. revised the manuscript together, and J. X., A. C., and H. L. supervised the project.
Data availability
No primary research results, software or code have been included and no new data were generated or analysed as part of this review.
Conflicts of interest
There are no conflicts to declare.
Acknowledgements
This work is supported by the National Key Research and Development Program of China (No. 2021YFA1202700), the Postdoctoral Fellowship Program of CPSF under the Grant Number (GZC20241472), JSPS KAKENHI (No. JP23K13703 & JP23KF0102), and AIMR Fusion Research.
References
- V. Rosca, M. Duca, M. T. de Groot and M. T. Koper, Chem. Rev., 2009, 109, 2209–2244 Search PubMed.
- E. J. Popczun, C. G. Read, C. W. Roske, N. S. Lewis and R. E. Schaak, Angew. Chem., Int. Ed., 2014, 53, 5427–5430 Search PubMed.
- J. Zhang and L. Dai, ACS Catal., 2015, 5, 7244–7253 CrossRef CAS.
- C. Hu and L. Dai, Angew. Chem., Int. Ed., 2016, 55, 11736–11758 CrossRef CAS PubMed.
- Z. W. Seh, J. Kibsgaard, C. F. Dickens, I. Chorkendorff, J. K. Nørskov and T. F. Jaramillo, Science, 2017, 355, eaad4998 CrossRef.
- H. Jin, C. Guo, X. Liu, J. Liu, A. Vasileff, Y. Jiao, Y. Zheng and S.-Z. Qiao, Chem. Rev., 2018, 118, 6337–6408 CrossRef CAS.
- C. Hu and L. Dai, Adv. Mater., 2019, 31, 1804672 Search PubMed.
- Y. Sun, S. Sun, H. Yang, S. Xi, J. Gracia and Z. J. Xu, Adv. Mater., 2020, 32, 2003297 CrossRef CAS PubMed.
- Y. Li, H. Wang, C. Priest, S. Li, P. Xu and G. Wu, Adv. Mater., 2021, 33, 2000381 CrossRef CAS.
- X. Jia, Z. Yu, F. Liu, H. Liu, D. Zhang, E. Campos dos Santos, H. Zheng, Y. Hashimoto, Y. Chen, L. Wei and H. Li, Adv. Sci., 2024, 11, 2305630 CrossRef CAS.
- D. Zhang, Z. Wang, F. Liu, P. Yi, L. Peng, Y. Chen, L. Wei and H. Li, J. Am. Chem. Soc., 2024, 146, 3210–3219 Search PubMed.
- J. Rossmeisl, A. Logadottir and J. K. Nørskov, Chem. Phys., 2005, 319, 178–184 Search PubMed.
- E. Fabbri and T. J. Schmidt, ACS Catal., 2018, 8, 9765–9774 CrossRef CAS.
- Z. Pei, H. Zhang, D. Luan and X. W. Lou, Matter, 2023, 6, 4128–4144 Search PubMed.
- J. K. Nørskov, J. Rossmeisl, A. Logadottir, L. Lindqvist, J. R. Kitchin, T. Bligaard and H. Jónsson, J. Phys. Chem. B, 2004, 108, 17886–17892 CrossRef.
- V. Stamenkovic, B. S. Mun, K. J. J. Mayrhofer, P. N. Ross, N. M. Markovic, J. Rossmeisl, J. Greeley and J. K. Nørskov, Angew. Chem., Int. Ed., 2006, 45, 2897–2901 Search PubMed.
- C. F. Dickens, C. Kirk and J. K. Nørskov, J. Phys. Chem. C, 2019, 123, 18960–18977 CrossRef CAS.
- R. Shi, X. Zhang, G. I. N. Waterhouse, Y. Zhao and T. Zhang, Adv. Energy Mater., 2020, 10, 2000659 Search PubMed.
- W. Tong, B. Huang, P. Wang, Q. Shao and X. Huang, Natl. Sci. Rev., 2021, 8 Search PubMed.
- T. Wang, Z. Guo, X. Zhang, Q. Li, A. Yu, C. Wu and C. Sun, J. Mater. Sci. Technol., 2023, 140, 121–134 CrossRef CAS.
- A. R. Singh, B. A. Rohr, J. A. Schwalbe, M. Cargnello, K. Chan, T. F. Jaramillo, I. Chorkendorff and J. K. Nørskov, ACS Catal., 2017, 7, 706–709 Search PubMed.
- B. Chang, Z. Cao, Y. Ren, C. Chen, L. Cavallo, F. Raziq, S. Zuo, W. Zhou, Y. Han and H. Zhang, ACS Nano, 2024, 18, 288–298 Search PubMed.
- J. T. Feaster, C. Shi, E. R. Cave, T. Hatsukade, D. N. Abram, K. P. Kuhl, C. Hahn, J. K. Nørskov and T. F. Jaramillo, ACS Catal., 2017, 7, 4822–4827 CrossRef CAS.
- L. D. Chen, M. Urushihara, K. Chan and J. K. Nørskov, ACS Catal., 2016, 6, 7133–7139 CrossRef CAS.
- A. A. Peterson, F. Abild-Pedersen, F. Studt, J. Rossmeisl and J. K. Nørskov, Energy Environ. Sci., 2010, 3, 1311–1315 RSC.
- X. Zhu, X. Zhou, Y. Jing and Y. Li, Nat. Commun., 2021, 12, 4080 CrossRef CAS PubMed.
- A. Zhang, Y. Liang, H. Zhang, Z. Geng and J. Zeng, Chem. Soc. Rev., 2021, 50, 9817–9844 Search PubMed.
- Y. Jia, Y. Zhang, H. Xu, J. Li, M. Gao and X. Yang, ACS Catal., 2024, 14, 4601–4637 CrossRef CAS.
- X. Yan, L. Zhuang, Z. Zhu and X. Yao, Nanoscale, 2021, 13, 3327–3345 RSC.
- P. Zhang, S. Hong, N. Song, Z. Han, F. Ge, G. Dai, H. Dong and C. Li, Chin. Chem. Lett., 2024, 35, 109073 CrossRef CAS.
- J.-T. Ren, L. Chen, H.-Y. Wang and Z.-Y. Yuan, Chem. Soc. Rev., 2023, 52, 8319–8373 Search PubMed.
- X. Yang, Y. Wang, X. Tong and N. Yang, Adv. Energy Mater., 2022, 12, 2102261 Search PubMed.
- S. Pal, T. Ahmed, S. Khatun and P. Roy, ACS Appl. Energy Mater., 2023, 6, 7737–7784 CrossRef CAS.
- Z. Zhang, P. Liu, Y. Song, Y. Hou, B. Xu, T. Liao, H. Zhang, J. Guo and Z. Sun, Adv. Sci., 2022, 9, 2204297 CrossRef CAS PubMed.
- C. Lucky and M. Schreier, ACS Nano, 2024, 18, 6008–6015 CrossRef CAS PubMed.
- H. Liu, D. Zhang, S. M. Holmes, C. D'Agostino and H. Li, Chem. Sci., 2023, 14, 9000–9009 RSC.
- W. Yang, Z. Jia, B. Zhou, L. Wei, Z. Gao and H. Li, Commun. Chem., 2023, 6, 6 CrossRef CAS PubMed.
- M. Cho, J. T. Song, S. Back, Y. Jung and J. Oh, ACS Catal., 2018, 8, 1178–1185 Search PubMed.
- T. Wang, Z. Guo, H. Oka, A. Kumatani, C. Liu and H. Li, J. Mater. Chem. A, 2024, 12, 8438–8446 RSC.
- H. Liu, X. Jia, A. Cao, L. Wei, C. D’agostino and H. Li, J. Chem. Phys., 2023, 158, 124705 Search PubMed.
- J. Duan, S. Chen, C. A. Ortíz-Ledón, M. Jaroniec and S.-Z. Qiao, Angew. Chem., Int. Ed., 2020, 59, 8181–8186 CrossRef CAS.
- A. S. Dobrota, N. V. Skorodumova, S. V. Mentus and I. A. Pašti, Electrochim. Acta, 2022, 412, 140155 CrossRef CAS.
- A. Ciotti and M. García-Melchor, Curr. Opin. Electrochem., 2023, 42, 101402 CrossRef CAS.
- Z. Guo, Y. Yu, C. Li, E. Campos dos Santos, T. Wang, H. Li, J. Xu, C. Liu and H. Li, Angew. Chem., Int. Ed., 2024, 63, e202319913 Search PubMed.
- X. Wang, X. Liu, S. Wu, K. Liu, X. Meng, B. Li, J. Lai, L. Wang and S. Feng, Nano Energy, 2023, 109, 108292 Search PubMed.
- P. Hou, Y. Tian, Y. Xie, F. Du, G. Chen, A. Vojvodic, J. Wu and X. Meng, Angew. Chem., Int. Ed., 2023, 62, e202304205 CrossRef CAS.
- M. López, K. S. Exner, F. Viñes and F. Illas, Adv. Theor. Simul., 2023, 6, 2200217 CrossRef.
- W. Yang, Z. Jia, B. Zhou, L. Chen, X. Ding, L. Jiao, H. Zheng, Z. Gao, Q. Wang and H. Li, ACS Catal., 2023, 13, 9695–9705 CrossRef CAS.
- J.-T. Jiang, Z. Guo, S.-K. Deng, X. Jia, H. Liu, J. Xu, H. Li and L.-H. Cheng, ChemSusChem, 2024, e202400827, DOI:10.1002/cssc.202400827.
- H. Liu, D. Zhang, Y. Wang and H. Li, Langmuir, 2024, 40, 7632–7638 Search PubMed.
- S. Pan, H. Li, T. Wang, Y. Fu, S. Wang, Z. Xie, L. Wei, H. Li and N. Li, ACS Catal., 2024, 13814–13824, DOI:10.1021/acscatal.4c03088,.
- H. Du, T. Wang, M. Li, Z. Yin, R. Lv, M. Zhang, X. Wu, Y. Tang, H. Li and G. Fu, Adv. Mater., 2024, 2411090, DOI:10.1002/adma.202411090,.
- A. Martini, D. Hursán, J. Timoshenko, M. Rüscher, F. Haase, C. Rettenmaier, E. Ortega, A. Etxebarria and B. Roldan Cuenya, J. Am. Chem. Soc., 2023, 145, 17351–17366 CrossRef CAS PubMed.
- C. Baeumer, J. Li, Q. Lu, A. Y.-L. Liang, L. Jin, H. P. Martins, T. Duchoň, M. Glöβ, S. M. Gericke, M. A. Wohlgemuth, M. Giesen, E. E. Penn, R. Dittmann, F. Gunkel, R. Waser, M. Bajdich, S. Nemšák, J. T. Mefford and W. C. Chueh, Nat. Mater., 2021, 20, 674–682 CrossRef CAS PubMed.
- D. Chen, D. K. Niakolas, V. Papaefthimiou, E. Ioannidou, S. G. Neophytides and S. Zafeiratos, J. Catal., 2021, 404, 518–528 Search PubMed.
- F. T. Haase, E. Ortega, S. Saddeler, F.-P. Schmidt, D. Cruz, F. Scholten, M. Rüscher, A. Martini, H. S. Jeon, A. Herzog, U. Hejral, E. M. Davis, J. Timoshenko, A. Knop-Gericke, T. Lunkenbein, S. Schulz, A. Bergmann and B. Roldan Cuenya, Energy Environ. Sci., 2024, 17, 2046–2058 RSC.
- P. P. Lopes, D. Y. Chung, X. Rui, H. Zheng, H. He, P. Farinazzo Bergamo Dias Martins, D. Strmcnik, V. R. Stamenkovic, P. Zapol, J. F. Mitchell, R. F. Klie and N. M. Markovic, J. Am. Chem. Soc., 2021, 143, 2741–2750 CrossRef CAS PubMed.
- Y. Sun, J. Wang, S. Xi, J. Shen, S. Luo, J. Ge, S. Sun, Y. Chen, J. V. Hanna, S. Li, X. Wang and Z. J. Xu, Nat. Commun., 2023, 14, 2467 CrossRef CAS PubMed.
- J.-Y. Ye, Y.-X. Jiang, T. Sheng and S.-G. Sun, Nano Energy, 2016, 29, 414–427 CrossRef CAS.
- Y. Yang, S. Louisia, S. Yu, J. Jin, I. Roh, C. Chen, M. V. Fonseca Guzman, J. Feijóo, P.-C. Chen, H. Wang, C. J. Pollock, X. Huang, Y.-T. Shao, C. Wang, D. A. Muller, H. D. Abruña and P. Yang, Nature, 2023, 614, 262–269 CrossRef CAS.
- Y. Deng, J. Zhao, S. Wang, R. Chen, J. Ding, H.-J. Tsai, W.-J. Zeng, S.-F. Hung, W. Xu, J. Wang, F. Jaouen, X. Li, Y. Huang and B. Liu, J. Am. Chem. Soc., 2023, 145, 7242–7251 CrossRef CAS.
- H. A. Hansen, J. Rossmeisl and J. K. Nørskov, Phys. Chem. Chem. Phys., 2008, 10, 3722–3730 RSC.
- E. Campos dos Santos, R. Sato, K. Kisu, K. Sau, X. Jia, F. Yang, S.-I. Orimo and H. Li, Chem. Mater., 2023, 35, 5996–6004 CrossRef CAS.
- Q. Zhu, A. R. Oganov, C. W. Glass and H. T. Stokes, Acta Cryst., 2012, B68, 215–226 Search PubMed.
- H. Zhao, X. Lv and Y.-G. Wang, Adv. Sci., 2023, 10, 2303677 CrossRef CAS PubMed.
- Z. Zhang, J. Li and Y.-G. Wang, Acc. Chem. Res., 2024, 57, 198–207 CrossRef CAS.
- X. Cao, D. Tan, B. Wulan, K. S. Hui, K. N. Hui and J. Zhang, Small Methods, 2021, 5, 2100700 CrossRef CAS PubMed.
- S. Zhao, Y. Wang, J. Dong, C.-T. He, H. Yin, P. An, K. Zhao, X. Zhang, C. Gao, L. Zhang, J. Lv, J. Wang, J. Zhang, A. M. Khattak, N. A. Khan, Z. Wei, J. Zhang, S. Liu, H. Zhao and Z. Tang, Nat. Energy, 2016, 1, 16184 Search PubMed.
- X. Ren, T. Wu, Y. Sun, Y. Li, G. Xian, X. Liu, C. Shen, J. Gracia, H.-J. Gao, H. Yang and Z. J. Xu, Nat. Commun., 2021, 12, 2608 CrossRef CAS.
- S. Lyu, C. Guo, J. Wang, Z. Li, B. Yang, L. Lei, L. Wang, J. Xiao, T. Zhang and Y. Hou, Nat. Commun., 2022, 13, 6171 CrossRef CAS.
- Y. Hao, Z. Guo, H. Cheng, C. Yao, S. Cheng, L. Yi and H. Li, J. Colloid Interface Sci., 2023, 650, 1949–1957 CrossRef CAS.
- C. Feng, M. B. Faheem, J. Fu, Y. Xiao, C. Li and Y. Li, ACS Catal., 2020, 10, 4019–4047 CrossRef CAS.
- Y. Zhang, T. Wang, L. Mei, R. Yang, W. Guo, H. Li and Z. Zeng, Nano-Micro Lett., 2024, 16, 180 CrossRef CAS.
- X. Gu, Z. Liu, M. Li, J. Tian and L. Feng, Appl. Catal., B, 2021, 297, 120462 CrossRef CAS.
- K. Bhattacharyya and A. A. Auer, J. Phys. Chem. C, 2022, 126, 18623–18635 CrossRef CAS.
- S. Pan, H. Li, D. Liu, R. Huang, X. Pan, D. Ren, J. Li, M. Shakouri, Q. Zhang, M. Wang, C. Wei, L. Mai, B. Zhang, Y. Zhao, Z. Wang, M. Graetzel and X. Zhang, Nat. Commun., 2022, 13, 2294 CrossRef CAS PubMed.
- H. Li, S. Kelly, D. Guevarra, Z. Wang, Y. Wang, J. A. Haber, M. Anand, G. T. K. K. Gunasooriya, C. S. Abraham, S. Vijay, J. M. Gregoire and J. K. Nørskov, Nat. Catal., 2021, 4, 463–468 CrossRef CAS.
- S. Yang, Y. J. Tak, J. Kim, A. Soon and H. Lee, ACS Catal., 2017, 7, 1301–1307 CrossRef CAS.
- A. Kulkarni, S. Siahrostami, A. Patel and J. K. Nørskov, Chem. Rev., 2018, 118, 2302–2312 CrossRef CAS.
- X. X. Wang, D. A. Cullen, Y.-T. Pan, S. Hwang, M. Wang, Z. Feng, J. Wang, M. H. Engelhard, H. Zhang, Y. He, Y. Shao, D. Su, K. L. More, J. S. Spendelow and G. Wu, Adv. Mater., 2018, 30, 1706758 CrossRef PubMed.
- Y. Wang, Y.-J. Tang and K. Zhou, J. Am. Chem. Soc., 2019, 141, 14115–14119 CrossRef CAS.
- L. Yang, J. Shui, L. Du, Y. Shao, J. Liu, L. Dai and Z. Hu, Adv. Mater., 2019, 31, 1804799 CrossRef.
- D. Zhang, Y. Hirai, K. Nakamura, K. Ito, Y. Matsuo, K. Ishibashi, Y. Hashimoto, H. Yabu and H. Li, Chem. Sci., 2024, 15, 5123–5132 Search PubMed.
- R. Lin, X. Cai, H. Zeng and Z. Yu, Adv. Mater., 2018, 30, 1705332 CrossRef PubMed.
- J. Wu and H. Yang, Acc. Chem. Res., 2013, 46, 1848–1857 CrossRef CAS.
- H. Niu, C. Xia, L. Huang, S. Zaman, T. Maiyalagan, W. Guo, B. You and B. Y. Xia, Chin. J. Catal., 2022, 43, 1459–1472 CrossRef CAS.
- L. Wang, A. Holewinski and C. Wang, ACS Catal., 2018, 8, 9388–9398 CrossRef CAS.
- S. R. Kelly, C. Kirk, K. Chan and J. K. Nørskov, J. Phys. Chem. C, 2020, 124, 14581–14591 CrossRef CAS.
- Y. Yuan, J. Wang, S. Adimi, H. Shen, T. Thomas, R. Ma, J. P. Attfield and M. Yang, Nat. Mater., 2020, 19, 282–286 CrossRef CAS PubMed.
- D. Bao, Q. Zhang, F. L. Meng, H. X. Zhong, M. M. Shi, Y. Zhang, J. M. Yan, Q. Jiang and X. B. Zhang, Adv. Mater., 2017, 29 Search PubMed.
- G. F. Chen, S. Ren, L. Zhang, H. Cheng, Y. Luo, K. Zhu, L. X. Ding and H. Wang, Small Methods, 2018, 3, 1800337 CrossRef.
- S. K. Sahoo, J. Heske, M. Antonietti, Q. Qin, M. Oschatz and T. D. Kühne, ACS Appl. Energy Mater., 2020, 3, 10061–10069 CrossRef CAS PubMed.
- H. Jin, S. S. Kim, S. Venkateshalu, J. Lee, K. Lee and K. Jin, Adv. Sci., 2023, 10, 2300951 CrossRef CAS.
- D. Chanda, R. Xing, T. Xu, Q. Liu, Y. Luo, S. Liu, R. A. Tufa, T. H. Dolla, T. Montini and X. Sun, Chem. Commun., 2021, 57, 7335–7349 RSC.
- D. Yang, T. Chen and Z. Wang, J. Mater. Chem. A, 2017, 5, 18967–18971 RSC.
- Z. Chen, J. Zhao, L. Yin and Z. Chen, J. Mater. Chem. A, 2019, 7, 13284–13292 RSC.
- Z. Guo, L. Jasin Arachchige, S. Qiu, X. Zhang, Y. Xu, S. J. Langford and C. Sun, Nanoscale, 2021, 13, 14935–14944 RSC.
- Z. Guo, T. Wang, H. Liu, S. Qiu, X. Zhang, Y. Xu, S. J. Langford and C. Sun, Appl. Surf. Sci., 2022, 572, 151441 CrossRef CAS.
- Z. Guo, S. Qiu, H. Li, Y. Xu, S. J. Langford and C. Sun, Diamond Relat. Mater., 2021, 111, 108210 CrossRef CAS.
- C. Liu, Q. Li, J. Zhang, Y. Jin, D. R. MacFarlane and C. Sun, J. Phys. Chem. C, 2018, 122, 25268–25273 CrossRef CAS.
- C. Liu, Q. Li, C. Wu, J. Zhang, Y. Jin, D. R. MacFarlane and C. Sun, J. Am. Chem. Soc., 2019, 141, 2884–2888 CrossRef CAS PubMed.
- Z. Guo, C. Liu, C. Sun, J. Xu, H. Li and T. Wang, ChemCatChem, 2023, 15, e202300669 CrossRef CAS.
- C. Liu, H. Zheng, T. Wang, Z. Guo, F. Zhu, H. Xie, G. Qin, H. Li and S. Li, J. Mater. Sci. Technol., 2023, 159, 244–250 CrossRef CAS.
- L. Zhang, H. Zhou, X. Yang, S. Zhang, H. Zhang, X. Yang, X. Su, J. Zhang and Z. Lin, Angew. Chem., Int. Ed., 2023, 135, e202217473 CrossRef.
- Y. Li, J. Li, J. Huang, J. Chen, Y. Kong, B. Yang, Z. Li, L. Lei, G. Chai, Z. Wen, L. Dai and Y. Hou, Angew. Chem., Int. Ed., 2021, 60, 9078–9085 CrossRef CAS PubMed.
- X.-F. Li, Q.-K. Li, J. Cheng, L. Liu, Q. Yan, Y. Wu, X.-H. Zhang, Z.-Y. Wang, Q. Qiu and Y. Luo, J. Am. Chem. Soc., 2016, 138, 8706–8709 CrossRef CAS PubMed.
- X. Chen, X. Zhao, Z. Kong, W.-J. Ong and N. Li, J. Mater. Chem. A, 2018, 6, 21941–21948 RSC.
- C. Liu, Q. Li, J. Zhang, Y. Jin, D. R. MacFarlane and C. Sun, J. Mater. Chem. A, 2019, 7, 4771–4776 RSC.
- C. Liu, H. Zheng, T. Wang, X. Zhang, Z. Guo and H. Li, Phys. Chem. Chem. Phys., 2023, 25, 13126–13135 RSC.
- X. Lv, W. Wei, B. Huang, Y. Dai and T. Frauenheim, Nano Lett., 2021, 21, 1871–1878 CrossRef CAS.
- B. Wang, S. Huang, L. Yang, Q. Fu and Y. Bu, J. Phys. Chem. C, 2021, 125, 14253–14262 CrossRef CAS.
- M. Liang, X. Shao and H. Lee, Chem. – Eur. J., 2024, 30, e202302843 CrossRef CAS PubMed.
- X. Liu, Z. Wang, G. Feng, Y. Sun, X. Zhang, X. Chen, R. Sa, Q. Li, C. Sun and Z. Ma, Chem. – Eur. J., 2024, 30, e202303148 CrossRef CAS.
- Y. Zhang, Z. Yu, F. She, L. Wei, Z. Zeng and H. Li, J. Colloid Interface Sci., 2023, 640, 983–989 CrossRef CAS.
- C. Yao, N. Guo, S. Xi, C.-Q. Xu, W. Liu, X. Zhao, J. Li, H. Fang, J. Su, Z. Chen, H. Yan, Z. Qiu, P. Lyu, C. Chen, H. Xu, X. Peng, X. Li, B. Liu, C. Su, S. J. Pennycook, C.-J. Sun, J. Li, C. Zhang, Y. Du and J. Lu, Nat. Commun., 2020, 11, 4389 CrossRef CAS PubMed.
- H. Yang, D. Luo, R. Gao, D. Wang, H. Li, Z. Zhao, M. Feng and Z. Chen, Phys. Chem. Chem. Phys., 2021, 23, 16707–16717 RSC.
- Y. Gao, E. Wang, Y. Zheng, J. Zhou and Z. Sun, Energy Mater. Adv., 2023, 4, 0039 CrossRef.
- W. Liu, P. Zhai, A. Li, B. Wei, K. Si, Y. Wei, X. Wang, G. Zhu, Q. Chen, X. Gu, R. Zhang, W. Zhou and Y. Gong, Nat. Commun., 2022, 13, 1877 CrossRef CAS PubMed.
- J. Zhang, C. Guo, S. Fang, X. Zhao, L. Li, H. Jiang, Z. Liu, Z. Fan, W. Xu, J. Xiao and M. Zhong, Nat. Commun., 2023, 14, 1298 CrossRef CAS.
- S. Nitopi, E. Bertheussen, S. B. Scott, X. Liu, A. K. Engstfeld, S. Horch, B. Seger, I. E. L. Stephens, K. Chan, C. Hahn, J. K. Nørskov, T. F. Jaramillo and I. Chorkendorff, Chem. Rev., 2019, 119, 7610–7672 CrossRef CAS PubMed.
- S. Garg, M. Li, A. Z. Weber, L. Ge, L. Li, V. Rudolph, G. Wang and T. E. Rufford, J. Mater. Chem. A, 2020, 8, 1511–1544 RSC.
- J.-J. Wang, X.-P. Li, B.-F. Cui, Z. Zhang, X.-F. Hu, J. Ding, Y.-D. Deng, X.-P. Han and W.-B. Hu, Rare Met., 2021, 40, 3019–3037 CrossRef CAS.
- B. Chang, H. Pang, F. Raziq, S. Wang, K.-W. Huang, J. Ye and H. Zhang, Energy Environ. Sci., 2023, 16, 4714–4758 RSC.
- X. Zhang, W. Huang, L. Yu, M. García-Melchor, D. Wang, L. Zhi and H. Zhang, Carbon Energy, 2024, 6, e362 CrossRef CAS.
- X. Wang, D. Wu, X. Kang, J. Zhang, X.-Z. Fu and J.-L. Luo, J. Energy Chem., 2022, 71, 159–166 CrossRef CAS.
- B. Ning, M. Liu, Y. Hu, H. Jiang and C. Li, Dalton Trans., 2022, 51, 3512–3519 RSC.
- C. Lin, Z. Xu, X. Kong, H. Zheng, Z. Geng and J. Zeng, ChemNanoMat, 2022, 8, e202200020 CrossRef CAS.
- M. K. Kim, H. Lee, J. H. Won, W. Sim, S. J. Kang, H. Choi, M. Sharma, H.-S. Oh, S. Ringe, Y. Kwon and H. M. Jeong, Adv. Funct. Mater., 2022, 32, 2107349 CrossRef CAS.
- Y. Zhang, H. Xu, D. Niu, X. Zhang and Y. Zhang, ChemSusChem, 2021, 14, 2769–2779 CrossRef CAS.
- B. Zhang, S. Chen, B. Wulan and J. Zhang, Chem. Eng. J., 2021, 421, 130003 CrossRef CAS.
- Y. Yuan, K. Sheng, G. Zhuang, Q. Li, C. Dou, Q.-J. Fang, W.-W. Zhan, H. Gao, D. Sun and X. Han, Chem. Commun., 2021, 57, 8636–8639 RSC.
- J. Li, J. Jiao, H. Zhang, P. Zhu, H. Ma, C. Chen, H. Xiao and Q. Lu, ACS Sustainable Chem. Eng., 2020, 8, 4975–4982 CrossRef CAS.
- K. V. Daele, D. Arenas-Esteban, D. Choukroun, S. Hoekx, A. Rossen, N. Daems, D. Pant, S. Bals and T. Breugelmans, ChemElectroChem, 2023, 10, e202201024 CrossRef CAS.
- Y. Zang, T. Liu, H. Li, P. Wei, Y. Song, C. Cheng, D. Gao, Y. Song, G. Wang and X. Bao, Chem. Eng. J., 2022, 446, 137444 CrossRef CAS.
- C. Salvini, M. Re Fiorentin, F. Risplendi, F. Raffone and G. Cicero, J. Phys. Chem. C, 2022, 126, 14441–14447 CrossRef CAS.
- Z. Yang, C. Yang, J. Han, W. Zhao, S. Shao, S. Li, H. Gao, H. Xie and X. Zhang, J. Mater. Chem. A, 2021, 9, 19681–19686 RSC.
- X. Cao, B. Wulan, B. Zhang, D. Tan and J. Zhang, J. Mater. Chem. A, 2021, 9, 14741–14751 RSC.
- H. Hu, L. Gui, W. Zhou, J. Sun, J. Xu, Q. Wang, B. He and L. Zhao, Electrochim. Acta, 2018, 285, 70–77 CrossRef CAS.
- B. Kumar, V. Atla, J. P. Brian, S. Kumari, T. Q. Nguyen, M. Sunkara and J. M. Spurgeon, Angew. Chem., Int. Ed., 2017, 56, 3645–3649 CrossRef CAS PubMed.
- Y. Li, B. Wei, M. Zhu, J. Chen, Q. Jiang, B. Yang, Y. Hou, L. Lei, Z. Li, R. Zhang and Y. Lu, Adv. Mater., 2021, 33, 2102212 CrossRef CAS.
- Q. Hao, H.-x Zhong, J.-z Wang, K.-h Liu, J.-m Yan, Z.-h Ren, N. Zhou, X. Zhao, H. Zhang, D.-x Liu, X. Liu, L.-w Chen, J. Luo and X.-b Zhang, Nat. Synth., 2022, 1, 719–728 CrossRef.
- Y.-N. Gong, C.-Y. Cao, W.-J. Shi, J.-H. Zhang, J.-H. Deng, T.-B. Lu and D.-C. Zhong, Angew. Chem., Int. Ed., 2022, 61, e202215187 CrossRef CAS.
- X. Zhao, K. Zhao, Y. Liu, Y. Su, S. Chen, H. Yu and X. Quan, ACS Catal., 2022, 12, 11412–11420 CrossRef CAS.
- M. Feng, X. Wu, H. Cheng, Z. Fan, X. Li, F. Cui, S. Fan, Y. Dai, G. Lei and G. He, J. Mater. Chem. A, 2021, 9, 23817–23827 RSC.
- W. Ma, S. Xie, T. Liu, Q. Fan, J. Ye, F. Sun, Z. Jiang, Q. Zhang, J. Cheng and Y. Wang, Nat. Catal., 2020, 3, 478–487 CrossRef CAS.
- C. Yang, Z. Li, J. Xu, Y. Jiang and W. Zhu, Green Chem., 2024, 26, 4908–4933 RSC.
- Y. Wang, D. Chen, C. Chen and S. Wang, Acc. Chem. Res., 2024, 57, 247–256 CrossRef CAS PubMed.
- C. Chen, X. Zhu, X. Wen, Y. Zhou, L. Zhou, H. Li, L. Tao, Q. Li, S. Du, T. Liu, D. Yan, C. Xie, Y. Zou, Y. Wang, R. Chen, J. Huo, Y. Li, J. Cheng, H. Su, X. Zhao, W. Cheng, Q. Liu, H. Lin, J. Luo, J. Chen, M. Dong, K. Cheng, C. Li and S. Wang, Nat. Chem., 2020, 12, 717–724 CrossRef CAS PubMed.
- W. Huang, T. Bo, S. Zuo, Y. Wang, J. Chen, S. Ould-Chikh, Y. Li, W. Zhou, J. Zhang and H. Zhang, SusMat, 2022, 2, 466–475 CrossRef CAS.
- C. Ling, L. Shi, Y. Ouyang and J. Wang, Chem. Mater., 2016, 28, 9026–9032 CrossRef CAS.
- G. Gao, A. P. O’Mullane and A. Du, ACS Catal., 2017, 7, 494–500 CrossRef CAS.
- X. Yang, N. Gao, S. Zhou and J. Zhao, Phys. Chem. Chem. Phys., 2018, 20, 19390–19397 RSC.
- C. Ling, L. Shi, Y. Ouyang, Q. Chen and J. Wang, Adv. Sci., 2016, 3, 1600180 CrossRef PubMed.
- Z. W. Seh, K. D. Fredrickson, B. Anasori, J. Kibsgaard, A. L. Strickler, M. R. Lukatskaya, Y. Gogotsi, T. F. Jaramillo and A. Vojvodic, ACS Energy Lett., 2016, 1, 589–594 CrossRef CAS.
- M. Alhabeb, K. Maleski, T. S. Mathis, A. Sarycheva, C. B. Hatter, S. Uzun, A. Levitt and Y. Gogotsi, Angew. Chem., Int. Ed., 2018, 57, 5444–5448 CrossRef CAS PubMed.
- M. Naguib, M. Kurtoglu, V. Presser, J. Lu, J. Niu, M. Heon, L. Hultman, Y. Gogotsi and M. W. Barsoum, Adv. Mater., 2011, 23, 4248–4253 CrossRef CAS PubMed.
- A. Thakur, N. Chandran B. S, K. Davidson, A. Bedford, H. Fang, Y. Im, V. Kanduri, B. C. Wyatt, S. K. Nemani, V. Poliukhova, R. Kumar, Z. Fakhraai and B. Anasori, Small Methods, 2023, 7, 2300030 CrossRef CAS PubMed.
- P. Urbankowski, B. Anasori, T. Makaryan, D. Er, S. Kota, P. L. Walsh, M. Zhao, V. B. Shenoy, M. W. Barsoum and Y. Gogotsi, Nanoscale, 2016, 8, 11385–11391 RSC.
- M. Ghidiu, M. Naguib, C. Shi, O. Mashtalir, L. M. Pan, B. Zhang, J. Yang, Y. Gogotsi, S. J. L. Billinge and M. W. Barsoum, Chem. Commun., 2014, 50, 9517–9520 RSC.
- J. Zhu, J. Zhang, R. Lin, B. Fu, C. Song, W. Shang, P. Tao and T. Deng, Chem. Commun., 2021, 57, 12611–12614 RSC.
- A. E. Ghazaly, H. Ahmed, A. R. Rezk, J. Halim, P. O. Å. Persson, L. Y. Yeo and J. Rosen, ACS Nano, 2021, 15, 4287–4293 CrossRef CAS PubMed.
- Z. Sun, M. Yuan, L. Lin, H. Yang, C. Nan, H. Li, G. Sun and X. Yang, ACS Mater. Lett., 2019, 1, 628–632 CrossRef CAS.
- I. T. McCrum and M. T. M. Koper, Nat. Energy, 2020, 5, 891–899 CrossRef CAS.
- P. G. Ghanekar, S. Deshpande and J. Greeley, Nat. Commun., 2022, 13, 5788 CrossRef CAS PubMed.
- K. Bang, D. Hong, Y. Park, D. Kim, S. S. Han and H. M. Lee, Nat. Commun., 2023, 14, 3004 CrossRef CAS PubMed.
- S. Han, S. Lysgaard, T. Vegge and H. A. Hansen, npj Comput. Mater., 2023, 9, 139 CrossRef CAS.
- Z. Li, F. Chen, W. Bian, B. Kou, Q. Wang, L. Guo, T. Jin, Q. Tang and B. Pan, Electrochim. Acta, 2021, 386, 138465 CrossRef CAS.
|
This journal is © The Royal Society of Chemistry 2024 |
Click here to see how this site uses Cookies. View our privacy policy here.