Exploring the selectivity of cytochrome P450 for enhanced novel anticancer agent synthesis†
Received
8th January 2024
, Accepted 28th February 2024
First published on 28th February 2024
Abstract
Cytochrome P450 monooxygenases are an extensive and unique class of enzymes, which can regio- and stereo-selectively functionalise hydrocarbons by way of oxidation reactions. These enzymes are naturally occurring but have also been extensively applied in a synthesis context, where they are used as efficient biocatalysts. Recently, a biosynthetic pathway where a cytochrome P450 monooxygenase catalyses a critical step of the pathway was uncovered, leading to the production of a number of products that display high antitumour potency. In this work, we use computational techniques to gain insight into the factors that determine the relative yields of the different products. We use conformational search algorithms to understand the substrate stereochemistry. On a machine-learned 3D protein structure, we use molecular docking to obtain a library of favourable poses for substrate–protein interaction. With molecular dynamics, we investigate the most favourable poses for reactivity on a molecular level, allowing us to investigate which protein–substrate interactions favour a given product and thus gain insight into the product selectivity.
Introduction
Biocatalysis involves the exploitation of the power and availability of naturally occurring catalysts, which can be modified to generate a wide diversity of synthetic products with high selectivity and yield via greener pathways.1 One very important component of research into biocatalysis is involved with the identification of novel enzymes and reaction cascades,2 with the enzymes then being modified or engineered to tune the reactivity in the desired way, by using some combination of rational design informed by the expected mechanism and evolutionary selection approaches. For many catalytic processes, especially those with complex regioselectivity and stereoselectivity profiles, experiments can only give limited mechanistic insight, and this often requires extensive work. Computational modelling procedures anchored on key experimental data provide an alternative source of detailed atomistic insight into reaction mechanisms, and thereby provide a deeper understanding of the origin of selectivity.3–5 This multidisciplinary and synergistic approach can be used to underpin rational fine-tuning of a biocatalytic system.
Computational approaches, however, also have significant shortcomings. Detailed atomistic studies are time consuming and do not provide quantitative predictions of rate constants or selectivity, so that the results of such studies seldom agree more than qualitatively with observed reaction outcomes. Exploration of reactivity for alternative substrates or for enzyme mutants represents considerable additional work, and given the low accuracy of computation, suffers from low predictivity. It can, however, often yield qualitative insight that rationalises observed changes in reactivity.6 Accordingly, alternative computational approaches based on machine learning can be used to predict such structure–reactivity patterns, though in this case, accurate results rely on the availability of large amounts of experimental data.7–10
Given the diversity of bioactive natural compounds with potent medicinal properties, the production of polyketide natural products has been the subject of much chemical and medical research,11,12 and these are also of great interest for biocatalysis. In vivo, polyketides are synthesised by a family of large, multidomain enzymes known as polyketide synthases (PKSs).13,14 Among other types,15,16 type I PKSs are structured like a multimodule assembly line, with each module containing a set of catalytic domains required to incorporate one carboxylic acid building block of the polyketide.17,18 A similarly structured family of multidomain enzymes are the non-ribosomal peptide synthetases (NRPSs), which produce a variety of non-ribosomal peptide natural products, many of which also have interesting medicinal properties.19,20 In addition, there are as many as 3339 known biosynthetic pathways that contain both PKS and NRPS units,21 allowing complex and highly specific products to be made. Nowadays, many of these products are made by exploiting the power of these multidomain enzymes directly, effectively using them as biocatalysts.13
In particular, a novel PKS/NRPS gene cluster (P. baetica a390T) was recently identified during an in silico screening of Pseudomonas spp.22 This gene cluster directs the biosynthesis of a number of oximidine products, which have been found to be powerful antitumour agents even on the nanomolar concentration scale.23–26 The roles of the core biosynthetic genes have been studied in great detail in ref. 22, but the exact nature of how the tailoring enzyme determines the relative yield of the different oximidine products remains unclear, hampering tuning for biocatalytic purposes. The tailoring enzyme central to the production of the oximidine products is a putative cytochrome P450 (CYP450), OxiK, which gives rise to rather selective oxidation, producing the oximidines shown in Fig. 1. Understanding the factors that lead to selectivity for these products (as opposed to the many potential oxidation products that are not formed), and that determine the competition between formation routes leading to each of them, is important for being able to tune the products formed by the novel PKS/NRPS cluster.
 |
| Fig. 1 Reactions catalysed by OxiK leading to formation of oximidine products 2–5 from reactant 1. To complete the reaction, products 3 and 5 require only 1 equivalent of O2, and products 2 and 4 require 2 equivalents of O2 and form in 2 steps. Estimated yields based on analytical data from ref. 22 are also given. | |
Both atomistic and machine-learning computational methods have been used to predict selectivity for CYP450-substrate systems.27 Such predictions are often expressed in terms of the site of metabolism (SoM), or in terms of predicted enzyme inhibition, or even in terms of expected substrate–substrate interactions.
Turning first to the machine- learning approaches, which are often implemented in rather ‘black-box’ software packages, these are designed to make quick predictions of reaction outcomes. They make use of datasets derived from atomistic calculations and from experiments,28–34 allowing SoMs for a given substrate to be proposed. Most of the existing tools of this type use datasets based on observed properties of human drug-processing CYP450s, reflecting the considerable practical importance of metabolism predictions within the drug development process. Accordingly, selectivity in biosynthetic bacterial CYP450s, such as OxiK, is not expected to be well described by these methods.
Turning now to atomistic modelling of reactivity, SoMs can be rationalised using a variety of techniques, including (but not limited to): quantum mechanics (QM), molecular mechanics molecular dynamics (MM MD), and hybrid QM/MM. Using atomistic modelling, the activation energies associated with the complete catalytic cycle of CYP450 have been elucidated.35,36 Among others, atomistic modelling of CYP450s also allows the nature of transition states, degree of enzyme stabilisation, and spin-density contributions to be understood. In many cases, bacterial CYP450s have also been investigated using atomistic modelling, with the most well-known example being P450cam.35,37–40 In principle, any CYP450 can be studied using an atomistic approach, although generating meaningful predictions via calculations and simulations is time consuming and requires user experience and care. Small changes in predicted activation free energies for competing pathways lead to large changes in predicted yields, given the exponential dependence of rate constants on free-energy barriers. Given the intrinsic large errors in atomistic modelling approaches, this means that computation of this type is better suited to qualitative rationalisation of observed selectivity profiles rather than to quantitative prediction.41,42
Atomistic modelling strategies require 3D structures of both the substrate and the enzyme involved. With careful application of conformational search techniques coupled with some experimental data, substrate structure prediction can be performed reasonably easily. However, obtaining the enzyme structure requires an X-ray crystallography study or some other similar high-quality structural determination. This requirement was, until recently, not easily satisfied. For human CYP450s, many (though not all) of the proteins corresponding to the 57 CYP450 genes have been studied using X-ray crystallography.43 However, for bacterial or fungal P450s, there is a huge mismatch between the number of known sequences and the number of available protein structures. Recently, the quality of computationally-predicted structures using machine-learning 3D structure predictors, such as RoseTTAFold and AlphaFold, has experienced spectacular improvement,44,45 such that reasonable CYP450 3D structures can now be obtained without the need for experiments. In its current form, the highly-popular AlphaFold does not include predicted coordinates for non-amino acid components such as the heme-group cofactor found in CYP450s. However, this limitation can be overcome through the use of complementary modelling tools, such as AlphaFill and FoldSeek.46,47
In this paper, we wish to explore the level of insight into the mechanism and selectivity of a bacterial CYP450, OxiK, that can be obtained using relatively straightforward computational structural modelling techniques. Specifically, we use machine-learning-based enzyme structure prediction tools (in this case, AlphaFold combined with AlphaFill for the heme cofactor) together with molecular docking and molecular dynamics to assess the enzyme structure and the enzyme–substrate positioning, ultimately to understand the observed selectivity in Fig. 1. We wish to assess the ability of such approaches to yield insights needed for the biocatalysis design process.
Computational details
Protein structure preparation
The AlphaFold structure of OxiK was downloaded from the AlphaFold Protein Structure Database (entry A0A2N0DV48),48 and the heme group was incorporated using the AlphaFill web tool.46 In all cases, the heme group was modelled as hexacoordinate Por-Fe(IV)
O, known as compound I in CYP450 monooxygenase catalytic cycles.49 Hydrogens were added to the substrate and the porphyrin ring using the ‘reduce’ program from the AmberTools23 package.50 The protein’s most probable protonation state at pH 7.4 was determined using the H++ web-server,51,52 and hydrogens were added accordingly. Any discussion of the protein topology below is referencing the topology as generated here.
Sequence overlapping
Using the FASTA sequence of OxiK, a BLAST sequence alignment search of sequences with experimental structures in the Protein Data Bank was performed.53 In this search, any non-bacterial hits were filtered out, and in order to make the number of hits manageable, any hits with a BLAST score < 40 were also filtered out. On each of the hits, a root-mean-square displacement (RMSD) alignment was performed against the AlphaFold/AlphaFill OxiK structure using PyMOL.54
Conformational search
Conformational analyses were performed using the xTB extended tight-binding method together with metadynamics simulations as implemented in CREST.55,56 The conformational search was initialised with a 3D structure that was built by hand, where the experimentally determined stereochemistry was adhered to. The GFN2-xTB parameterization of the xTB method, with generalized born with surface area (GBSA) implicit treatment of water solvent, was used throughout.57 Analysis of the conformers was performed using the visual molecular dynamics (VMD) graphics package.58
Substrate parameter generation
The oximidine substrate was described with the GAFF2 forcefield.59 Partial charges were determined using the restrained electrostatic potential (RESP) fitting method using py_resp.py from AmberTools23.60 The geometry of the substrate was optimised with the GFN2-xTB Hamiltonian, with GBSA implicit solvation in chloroform, prior to RESP fitting.57 The electrostatic potential was generated at the HF/6-31G* level of theory using Gaussian16.61
Molecular docking
Molecular docking of deoxy-oximidine into the AlphaFold/AlphaFill structure was performed using the AutoDock Vina 1.2.0 software package.62,63 No flexibility was allowed in amino acid side-chains, but some flexibility was allowed for the ligand, in the form of torsional coordinate changes for some of the single bonds of the ligand. Based on the established rigidity of the ring system in the conformational search, no such changes in torsional angles were allowed within this ring. On the other hand, due to the high degree of flexibility found in the chain, all heavy-atom single-bond torsions within the chain were allowed to rotate freely. All docking procedures used charges generated herein for the substrate, charges for the heme group and Cys406 generated by Shahrokh et al.,64 and the remaining protein with the default charges using the improved AutoDock AD4 forcefield, as implemented in AutoDock 1.2.0.63
Molecular dynamics
The system (protein and substrate) was placed in a cubic box and solvated with water and sodium ions until neutral. The distance from the protein to an edge of the box was at least 15 Å. The ff19SB force field was used to describe protein residues, the OPC model was used for water and the Li and Merz 12-6 ionic forcefield for the sodium ions.65–67 Parameters for the heme group and Cys406 were taken from Shahrokh et al.64 Substrate parameters generated herein, as described above, were used.
The same equilibration procedure was used for all generated substrate positions (Table S2†). First, the positions of the solvent atoms were minimised, followed by a minimisation of the whole system. This was followed by heating under constant volume from 0 to 300 K during 20 ps, with constraints applied to all non-solvent atoms. Then the system was equilibrated to a pressure of 1 bar, first for 10 ps using a CPU and then for 1 ns with a GPU. This was followed by a series of equilibration runs under constant volume, with the constraints on the protein gradually released. For each substrate orientation, a 100 ns NVT production run was performed. Throughout, periodic boundary conditions were used together with the particle mesh Ewald algorithm and a 10 Å real-space cutoff for short-range interactions.68 Temperature was maintained using a Langevin thermostat, and pressure with an isotropic Berendsen barostat. All molecular dynamics (MD) runs employed a 2 fs time step, with the SHAKE algorithm.69 Mean interaction energies between the ligand and the protein were computed at 250 regularly-spaced snapshots drawn from the final 50 ns of the MD simulations.
Results
Structural validation
In order to validate the usage of AlphaFold for structure prediction of bacterial CYP450s, the obtained structure was compared to experimental structures for related bacterial proteins, identified using a BLAST sequence alignment search between structures in the Protein Data Bank and the sequence of OxiK. A complete table containing the results of this alignment procedure is given as Table S1.† There were a number of hits with good BLAST scores, and we note a direct correlation between the RMSD versus the AlphaFold OxiK structure and the BLAST score. In simple terms, a BLAST score is a function of two main components: the alignment of similar or identical residues, and the quantity of gaps that needed to be introduced to align the sequences.53,70 A number of the structures show good BLAST scores of >70 and have accompanying RMSD values of <3.0 Å (see Fig. 2a). Of course, this similarity in the overall fold is not unusual given that the AlphaFold model will implicitly have been trained on these structures, but it is nevertheless encouraging with regards to the global quality of the AlphaFold structure of OxiK. We see no clear correlation between the RMSD and apo/holo conformers of the enzyme, which is not unexpected given the established rigidity of the binding core of the CYP450 family of enzymes.71 The highest-scoring match (BLAST score: 85.9; RMSD (Å): 1.857) was found for PDB entry 7WZL, which is a recently crystallised high-resolution structure of CYP450 184A1 from Streptomyces avermitilis.72
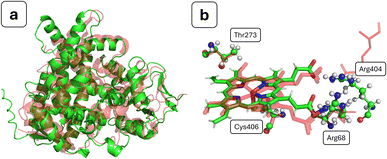 |
| Fig. 2 (a) Backbone alignment of AlphaFold entry A0A2N0DV48 (green) and PDB entry 7WZL (red); (b) heme environment with important neighbouring residues for the aligned structures; 7WZL is in red, and AlphaFold entry A0A2N0DV48 uses the indicated atomic colours. Colours: green, carbon; red, oxygen; blue, nitrogen; yellow, sulphur; orange, iron; white, hydrogen. | |
As mentioned above, the overall fold of the AlphaFold structure is expected to be quite accurate, but there are several key structural features of CYP450s that should be found in the binding core and that in principle could not be inaccurately predicted by AlphaFold. Critically, a heme group must be present to aid in the catalytic cycle, but as mentioned above, this is not added by AlphaFold in its current implementation. We have added the heme group using the tool AlphaFill. By backbone alignment with the highest BLAST score bacterial CYP450 crystal structures, we find the addition of the heme group has taken place where expected (see Fig. 2b). The heme group addition is further verified by the location of the propionate side-chains, which are known to have a key role in heme biochemistry.73 The propionates are placed in close proximity to the expected accompanying Arg residues (Arg68 & Arg404), thus neutralising the local charge density. We do, however, note that one of the propionate side-chains is unusually close to Arg404, but this contact was closely monitored during the MD simulations and was found to quickly relax without any ensuing structural perturbation. In CYP450s, a thiolate cysteine proximally coordinated to the heme group functions as an electron donor, where it aids CYP450 reactivity.74 In the structure we have generated, we find that Cys406 is within coordination distance to the heme iron (see Fig. 2b). An additional structural feature that aids in stabilisation of heme–thiolate coordination is a number (which may range from 1 to 3) of backbone peptide-bond hydrogen donors to the coordinated thiolate cysteine.75 In our structure, we find one clear candidate for hydrogen-bond donation in Ala408, and another potential hydrogen bond donor in Ala409. Following MD simulations, we see a similar behaviour, where Ala408 shows a clear hydrogen bond, whereas Ala409 is a potential hydrogen bond donor. Another overwhelmingly conserved feature among almost all CYP450s is that a Thr residue should be present in the binding pocket, where it participates in proton delivery, leading to O2 bond scission and ultimately formation of compound 1.76–78 In the structure we have generated, we find a potential candidate for this role in Thr273 (see Fig. 2b).
Substrate conformations
In order to evaluate the lowest-energy conformers of the substrate, a conformational search, restricted to structures having the stereochemistry identified by NMR spectroscopy in ref. 22, was performed. Using the default energy window of 6 kcal mol−1, as implemented in CREST, we find a total of 289 conformers. Among these conformers, the greatest variability is observed for the side-chain. This flexibility is visually evident but was verified by measuring the high variance in two dihedral angles joining the ring system and the chain, defined as ∠C14–C15–C16–C17 and ∠O21–C15–C16–C17, respectively (Fig. S1†). The high flexibility is not unexpected given that the first carbon of the chain is not part of a conformer-defining feature, such as a double bond. We note that the observed oxidation products do not involve oxidation of the side-chain, so that prediction of its structure is not critical for the studies performed here, though for other substrates, the situation could well be different in this regard.
One very important feature of the substrate structure concerns the stereochemistry at C15, and hence of the O21 ester oxygen. The relative position of the ester group and the plane defined by the aromatic ring C1–C6 can play an important role in determining selectivity. We find that all 289 of the predicted conformers display the same relative orientation of this ester group and the aromatic ring. This consistency along all conformers means that the 12-membered ring system of the substrate is predicted to have quite a high conformational rigidity. This allows some of the observed stereochemistry of the products shown in Fig. 1 to be explained.
The computed 3D structure of deoxy-oximidine allows rationalisation of one intriguing structural feature of the product oximidine V (product 5). Formation of oximidine V presumably occurs in three steps: hydrogen abstraction from C14, formation of the C13–C14 trans-configured double bond, and addition of a hydroxyl group on C12.79 The stereochemistry of the added alcohol group cannot be easily explained as this is dependent on the position of the substrate relative to the heme group. However, the trans-configuration of the C13–C14 double bond can be explained by the high conformational rigidity of the 12-membered ring system. In every conformer, even prior to desaturation, the dihedral angle of single bond C13–C14 is such that this bond is predisposed to forming a trans-double bond upon oxidation, with an average ∠C12–C13–C14–C15 of −120°. This suggests that the formation of the trans-configured C13–C14 double bond is not as unexpected as originally presumed,22 but rather would have a lower barrier of formation with respect to the cis-configured equivalent.
Substrate docking
The primary purpose of the molecular docking procedure was to generate a library of poses that could be selected for further study by molecular dynamics. We required the set of poses to include structures that involved close proximity between the heme oxo group and several of the various oxidisable sites within the substrate. In particular, we wish to study structures that are well-suited for oxidation of the electron-rich C1–C6 aromatic ring or for oxidation of the C10–C11 or C12–C13 double bonds or the allylic C14 (these sites are in close proximity and will often be referred to below as the C10–C14 site). While the aromatic ring should a priori be quite reactive towards CYP450s, it is surprising that experiments do not show it undergoing oxidation by OxiK – this is one of the aspects we wish to understand. It should be noted that the oxidisable sites in the side-chain (such as the unsaturated bonds) are not observed to be oxidised either, but we have not explicitly included poses suited for this type of oxidation in our study. However, we do find a possible role for this side-chain and an explanation for why it is not oxidised from our MD simulations.
In total, four poses were selected for further analysis via molecular dynamics. In two of these poses, the aromatic ring was situated close to the heme oxo group, while in the other two, the C10–C14 region of the twelve-membered ring was close to it. Note that for stereochemical reasons, the other positions in the twelve-membered ring cannot easily approach the heme oxo. Of the two poses for each potential metabolism site, one was chosen to have the substrate side-chain extending into a channel close to the substrate binding pocket on the distal side of the heme group, while the other was chosen to have the side-chain within the binding pocket. Among the set of binding poses satisfying these criteria, the one with the lowest average distance to the heme oxo group for the relevant possible metabolism sites was selected.
Molecular dynamics simulations
To explore the substrate interaction further, from the 100 generated docked structures, we selected two poses where the C10–C14 region is in proximity to the heme oxo group and two poses where the aromatic ring is close to it (Fig. S2†). As mentioned before, these poses vary based on the positioning of the substrate’s side-chain. 100 ns molecular dynamics simulations were conducted for each pose.
Substrate interaction
In poses 1 and 2, the entire substrate is positioned within the hydrophobic pocket on the distal side of the heme group. After some initial fluctuations of the substrate at the start of the simulations, it stabilised in one predominant conformation with the side-chain folded back onto the rest of the substrate (Fig. 3). The binding pocket mostly consists of hydrophobic residues. Despite the substrate remaining relatively stable in terms of orientation within the active site, specific interactions with the protein environment were not observed. Only potential π-stacking interactions between nearby phenylalanine residues and the C18–C17 double bond of the substrate’s side-chain in pose 2 are possible.
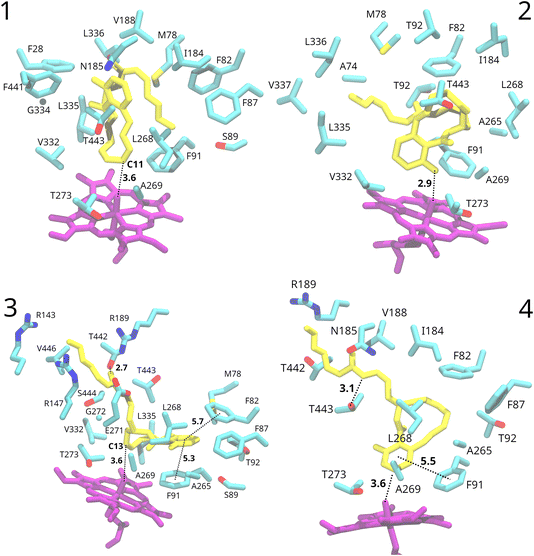 |
| Fig. 3 Protein environment around the substrate in the representative binding poses during the four MD simulations. Distances are shown in Å and represent average distances along the 100 ns of dynamics of poses 2 and 3 and the last 50 ns of dynamics of poses 1 and 4. The substrate is shown in yellow and the heme group in purple. Colours for protein residues: cyan, carbon; red, oxygen; blue, nitrogen; yellow, sulphur. | |
In the docked structures of poses 3 and 4, the substrate’s ring system is situated in the distal pocket adjacent to the heme group. However, the side-chain extends away from this pocket and is positioned inside a channel leading to the solvent, which was identified already during docking (Fig. 3). The entrance to this channel from the distal pocket is a narrow window, surrounded by residues Gly272, Leu268, Thr443, and Ser444. In both MD runs for pose 3 and pose 4, the side-chain remained extended into this tunnel, which leads towards the solvent.
In the MD run for pose 4, the side-chain remained extended towards the solvent, but significant rearrangement of the protein environment occurred. The narrow opening widened, allowing solvent water molecules to enter the binding pocket. In contrast, in pose 3, the opening mostly retained its original shape (Fig. S4†). This is consistent with the observed larger fluctuations of the substrate during the simulation in pose 4 (substrate root-mean-square fluctuations, RMSF, of 2.6 Å), where it passed through several conformations before stabilising in the second half of the simulation in one conformation. In contrast, the substrate in pose 3 maintained one predominant conformation with a small RMSF of 0.9 Å.
For pose 3, a short hydrogen bond is observed between the amide oxygen of the substrate’s side-chain and the Thr442 side-chain (average O–O distance of 2.7 Å) throughout the whole simulation (Fig. 3). Similarly, in pose 4, a hydrogen bond (3.1 Å on average) between the amide nitrogen of the substrate’s side-chain and a threonine side-chain (in this case Thr443) was noted in the final 50 ns of the simulation (Fig. 3). The extension of the side-chain towards the solvent facilitates interactions between the O-methyloxime group and charged residues on the protein surface, as well as solvent water molecules. In pose 3, the chain’s end is positioned between arginine 143, 147 and 189.
These findings, especially for pose 3, suggest that poses where the side-chain is extended into the channel leading towards the solvent are more favourable. This observation also suggests a possible reason for the lack of oxidation of this side-chain by OxiK, and this motivates our decision in this preliminary work not to explore the behaviour of docked structures in which the side-chain is positioned favourably for oxidation. The presence of channels or tunnels in CYP450 enzymes is not new and they are generally associated with substrate selectivity, presumably by regulating the accessibility of certain substrates.71,80,81 There is also evidence that substrates may extend into the solvent via a channel and give stability to the holo enzyme form. By way of MD simulations, Zhuang et al. have found that the enantioselectivity towards a ketoconazole in CYP3A4 is driven in part by extension of the substrate into the bulk solvent, via a channel.82 This is experimentally supported by a crystal structure of this ketoconazole–CYP3A4 complex, where the substrate also extends into the solvent.83 In a similar vein, we have found a number of crystal structures for bacterial CYP450s with various substrates bound, showing similar extension into the bulk solvent (pdb-ids: 7GW0, 5TL8 and 1IZO).84–86 Together, this suggests that the stability of the substrate associated with a docking mode where it extends out from the heme pocket into a channel leading towards the solvent is plausible.
In both poses 3 and 4, the binding pocket mostly consists of hydrophobic residues: Gly272, Leu268, Thr443, Ser444, Ala265, Phe82, Phe91, Phe87, Met78, Leu335, Thr92, and Ser89 for pose 3, and Phe82, Phe87, Phe91, Ala265, Leu268, Ala269, and Thr273 for pose 4. Besides van der Waals contacts, the substrate engages in π-stacking interactions with neighbouring phenylalanine residues (Fig. 3). In pose 3, the substrate’s aromatic ring is sandwiched between Phe91 and Phe82, well within the π-stacking distance.87 In pose 4, the substrate’s aromatic ring forms a π-stacking interaction with Phe91, while Phe82 and Phe87 potentially interact with the C10–C11 and C12–C13 double bonds. Similar π-stacking interactions between the enzyme and the drug have been observed for other CYP450s, for example for human CYP450 2C9 with bound warfarin.88
There are significant differences in the shape of the binding pocket between pose 3 and pose 4. Fig. 4 depicts the surface of residues within 4 Å of the substrate. The fit of the substrate in pose 3 is better than in pose 4. Notably, in pose 4, an entire side of the substrate lacks adjacent protein residues due to the entrance of solvent water molecules into the binding pocket.
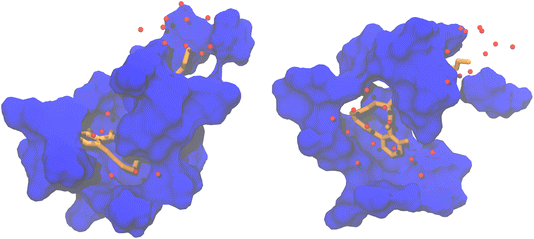 |
| Fig. 4 Shape of the binding pocket during MD simulation of pose 3 (left) and pose 4 (right). The surface of residues within 4 Å of the substrate is shown in blue. The substrate is represented as sticks in orange. Red spheres are water molecules within 4 Å of the substrate. The hydrogens of the substrate and the water molecules are omitted for clarity. | |
Considering the greater substrate fluctuation, the absence of one aromatic π-stacking interaction, and the suboptimal fit within the binding pocket, it appears that pose 3 is a better model of the likely substrate binding mode than pose 4.
To quantify our observations, we conducted an analysis of average substrate–protein interactions throughout the MD simulations for each pose, as detailed in Table 1. The analysis validates the favourability of pose 3, which exhibits van der Waals (EVDW) and electrostatic (EEEL) interactions with the protein that lead to total interaction energies Etot that are approximately 20 kcal mol−1 more favourable than those observed in other poses. Assuming the solvation energy and entropy of binding are similar for all binding poses, this would translate into pose 3 having the most favourable free energy of binding.
Table 1 Mean substrate–protein interaction (excluding solvent) values ±2 standard deviations across 250 snapshots from the final 50 ns of MD simulations for different substrate poses
Pose |
E
EEL [kcal mol−1] |
E
VDW [kcal mol−1] |
E
tot [kcal mol−1] |
1 |
−6.47 ± 4.83 |
−43.87 ± 4.33 |
−50.34 ± 5.89 |
2 |
−16.22 ± 5.87 |
−46.97 ± 5.87 |
−63.20 ± 6.33 |
3 |
−30.22 ± 9.70 |
−51.71 ± 5.25 |
−81.93 ± 11.44 |
4 |
−20.08 ± 9.68 |
−42.71 ± 6.48 |
−62.78 ± 11.40 |
Reactivity
Analysis of the products in Fig. 1 reveals the existence of three reactive positions: C14, the C13–C12 double bond, and the C11–C10 double bond. Among them, the C11–C10 double bond is apparently the least reactive based on the observed product ratios. C14 is likely the most reactive site, as product 5 likely forms following an initial hydrogen atom abstraction (HAA) on C14. Notably, all products exhibit reactivity at the C12–C13 double bond, indicating its relative reactivity. Intriguingly, no reactivity of the electron-rich aromatic ring of the substrate is observed. These trends in reactivity are the ones we wish to understand based on modelling, with the view to then being able to tune them for biocatalysis.
We evaluated the potential reactivity of the substrate during the course of the MD simulations in the four poses based on its proximity to the heme oxo group. In all poses, the substrate consistently maintained close proximity to it (Fig. 3).
In the simulation of pose 1, the distance between the heme oxo group and C10/C11 consistently fell below 3.5 Å, suggesting the potential oxidation of the C10–C11 double bond. The resulting epoxide would exhibit the same stereochemistry as assigned to product 4, but based on the structures of the other products, it seems unlikely that the C10–C11 double bond is the site of initial oxidation. It seems more likely that product 4 forms from product 3 after initial oxidation of the C12–C13 double bond.
Pose 2 shows the formation of a stable hydrogen bond between the heme oxo group and the substrate’s hydroxyl group situated on the C1–C6 aromatic ring (average distance of 2.9 Å). This placed the heme oxo group in proximity to C2, the carbon ortho to the hydroxyl group, with an average oxo-C2 distance of 3.4 Å.
In pose 4, we focused on the stable conformation observed in the last 50 ns of the simulation. The C1–C6 aromatic ring’s positioning suggested potential oxidation at C3, the carbon meta to the hydroxy group, with an average oxo-C3 distance of 3.6 Å. Additionally, the oxo-C2 distance was on average equal to 4.1 Å and occasionally dropped below 3.5 Å.
Pose 3 indicated the likely oxidation of the C12–C13 double bond, based on oxo-C12 and oxo-C13 distances frequently dropping below 3.5 Å (3.7 Å and 3.6 Å on average, respectively). The resulting epoxide would match the stereochemistry observed in product 3. Additionally, the direction of approach of the C12–C13 double bond closely resembles the proposed transition state for epoxidation of cyclohexene by compound I described by Shaik et al.36
It is interesting that pose 3, the one identified as having the most favourable interactions with the protein environment, also aligns best with the experimentally observed reactivity of OxiK. The oxidation of the substrate’s side-chain is prevented by its placement in a stabilising channel leading to the solvent, while the C1–C6 aromatic ring is held away from compound I by stabilising π-stacking interactions and steric constraints imposed by the binding pocket.
While pose 3 elucidates the epoxidation of the C12–C13 double bond observed in products 2, 3, and 4, it does not account for the formation of the major product 5, likely arising from an initial HAA at C14. The average distance between the oxo heme group and the closest hydrogen on C14 is 5.3 Å, with a minimum distance of 4.4 Å, presumably too distant to initiate a reaction. Still, the C12–C13–C14–C15 dihedral angle was on average −124° during the 100 ns of dynamics, which, as discussed earlier, already predisposes the formation of a trans C13–C14 double bond, as observed in product 5.
Conclusions
The approach favoured in this work is to use relatively computationally straightforward techniques to yield qualitative insight into the origin of selectivity in OxiK, taken as an exemplar of the class of CYP450s present in PKS/NRPS clusters. The relatively inexpensive computational analyses described here could potentially be broadened to a range of other CYP450s, or to other substrates, and could be used to generate a dataset suitable for design of a predictive model for oxidation in this class of enzymes.
Comparative analysis of the AlphaFold-generated OxiK structure with available X-ray crystallography structures revealed accurate predictions of both global structural elements and conserved features within the heme group’s local environment. The conformational search of the substrate highlighted the inherent rigidity of the substrate’s ring system, favouring the potential formation of a trans C13–C14 double bond, as observed in the major product 5 (Fig. 1). The conformational search predicts large flexibility of the substrate’s side-chain, which was also observed in the docking procedure. Consequently, it was necessary to filter the generated structures based on potential reactive sites. Despite this slight complication, the identification of a channel capable of accommodating the substrate’s side-chain emerged, potentially stabilising and preventing its oxidation.
MD simulations were conducted on several poses in order to investigate the experimentally observed preference of the enzyme to carry out oxidation of the C10–C14 region of the substrate’s 12-membered ring instead of oxidation of the C1–C6 aromatic ring. The investigation demonstrated that positioning of the substrate’s side-chain within the aforementioned channel promotes more favourable interactions with the enzyme compared to its positioning in the hydrophobic binding pocket, possibly explaining why oxidation of the side-chain was not observed experimentally. It was also found that a better general fit of the substrate inside the binding pocket can be achieved if the C10–C14 region is in proximity to the oxo heme group, as opposed to proximity of the C1–C6 aromatic ring. The most stable pose favours oxidation of the C12–C13 double bond and would lead to the formation of product 3 (Fig. 1) with matching stereochemistry. Notably, π-stacking interactions with Phe82 and Phe91 were identified as potential contributors to preventing the oxidation of the C1–C6 aromatic ring. Further investigation is necessary to completely understand the mechanism of formation of product 5 (Fig. 1).
In summary, the integration of machine-learning-based structure prediction tools, conformational search algorithms, docking, and short MD simulations proved effective in providing valuable insights into the selectivity and substrate binding in this novel bacterial CYP450. While the docking and MD simulation approach here could not be applied in a high-throughput way and does not yield immediate quantitative predictions of oxidation selectivity, it does provide useful qualitative insight into the factors that affect selectivity. Also, even though the MD simulations are computationally somewhat demanding, extension to a medium-sized set of other substrates and/or CYP450 enzymes would certainly be feasible, and the resulting dataset could be used in conjunction with experimental data to train a model able to predict oxidation profiles of different bacterial CYP450s.
Data availability
Data supporting this article have been uploaded as part of the ESI† and files for replicating molecular dynamics simulations are available via the KU Leuven Research Data Repository; see https://doi.org/10.48804/XHQXIQ.
Author contributions
Janko Čivić: conceptualisation, data curation, formal analysis, investigation, methodology, writing – original draft, writing – review & editing; Neil R. McFarlane: conceptualisation, data curation, formal analysis, investigation, methodology, writing – original draft, writing – review & editing; Jeremy N. Harvey: conceptualisation, supervision, writing – original draft, writing – review & editing; Joleen Masschelein: conceptualisation, supervision, writing – review & editing.
Conflicts of interest
There are no conflicts to declare.
Acknowledgements
Joleen Masschelein thanks FWO for finding through grant FWO - G061821N.
Notes and references
- E. L. Bell, W. Finnigan, S. P. France, A. P. Green, M. A. Hayes, L. J. Hepworth, S. L. Lovelock, H. Niikura, S. Osuna, E. Romero, K. S. Ryan, N. J. Turner and S. L. Flitsch, Biocatalysis, Nat. Rev. Methods Primers, 2021, 1(1), 46, DOI:10.1038/s43586-021-00044-z.
- D. Yi, T. Bayer, C. P. S. Badenhorst, S. Wu, M. Doerr, M. Höhne and U. T. Bornscheuer, Recent Trends in Biocatalysis, Chem. Soc. Rev., 2021, 50(14), 8003–8049, 10.1039/D0CS01575J.
- S. Ahmadi, L. Barrios Herrera, M. Chehelamirani, J. Hostaš, S. Jalife and D. R. Salahub, Multiscale Modeling of Enzymes: QM-Cluster, QM/MM, and QM/MM/MD: A Tutorial Review, Int. J. Quantum Chem., 2018, 118(9), e25558, DOI:10.1002/qua.25558.
- R. Lonsdale, J. N. Harvey and A. J. Mulholland, A Practical Guide to Modelling Enzyme-Catalysed Reactions, Chem. Soc. Rev., 2012, 41(8), 3025, 10.1039/c2cs15297e.
- A. J. Mulholland, Modelling Enzyme Reaction Mechanisms, Specificity and Catalysis, Drug Discovery Today, 2005, 10(20), 1393–1402, DOI:10.1016/S1359-6446(05)03611-1.
- M. W. Van Der Kamp and A. J. Mulholland, Computational Enzymology: Insight into Biological Catalysts from Modelling, Nat. Prod. Rep., 2008, 25(6), 1001, 10.1039/b600517a.
- D. S. Wishart, S. Tian, D. Allen, E. Oler, H. Peters, V. W. Lui, V. Gautam, Y. Djoumbou-Feunang, R. Greiner and T. O. Metz, BioTransformer 3.0—a Web Server for Accurately Predicting Metabolic Transformation Products, Nucleic Acids Res., 2022, 50(W1), W115–W123, DOI:10.1093/nar/gkac313.
- C. A. Marchant, K. A. Briggs and A. Long, In Silico Tools for Sharing Data and Knowledge on Toxicity and Metabolism: Derek for Windows, Meteor, and Vitic, Toxicol. Mech. Methods, 2008, 18(2–3), 177–187, DOI:10.1080/15376510701857320.
- N. Greene, P. N. Judson, J. J. Langowski and C. A. Marchant, Knowledge-Based Expert Systems for Toxicity and Metabolism Prediction: DEREK, StAR and METEOR, SAR QSAR Environ. Res., 1999, 10(2–3), 299–314, DOI:10.1080/10629369908039182.
- L. Ridder and M. Wagener, SyGMa: Combining Expert Knowledge and Empirical Scoring in the Prediction of Metabolites, ChemMedChem, 2008, 3(5), 821–832, DOI:10.1002/cmdc.200700312.
- D. J. Newman and G. M. Cragg, Natural Products as Sources of New Drugs over the Nearly Four Decades from 01/1981 to 09/2019, J. Nat. Prod., 2020, 83(3), 770–803, DOI:10.1021/acs.jnatprod.9b01285.
- C. Hertweck, The Biosynthetic Logic of Polyketide Diversity, Angew. Chem., Int. Ed., 2009, 48(26), 4688–4716, DOI:10.1002/anie.200806121.
- J. Staunton and K. J. Weissman, Polyketide Biosynthesis: A Millennium Review, Nat. Prod. Rep., 2001, 18(4), 380–416, 10.1039/A909079G.
- S. Smith and S.-C. Tsai, The Type I Fatty Acid and Polyketide Synthases: A Tale of Two Megasynthases, Nat. Prod. Rep., 2007, 24(5), 1041–1072, 10.1039/B603600G.
- Z. Lin and X. Qu, Emerging Diversity in Polyketide Synthase, Tetrahedron Lett., 2022, 110, 154183, DOI:10.1016/j.tetlet.2022.154183.
- B. Shen, Polyketide Biosynthesis beyond the Type I, II and III Polyketide Synthase Paradigms, Curr. Opin. Chem. Biol., 2003, 7(2), 285–295, DOI:10.1016/S1367-5931(03)00020-6.
- A. Nivina, K. P. Yuet, J. Hsu and C. Khosla, Evolution and Diversity of Assembly-Line Polyketide Synthases, Chem. Rev., 2019, 119(24), 12524–12547, DOI:10.1021/acs.chemrev.9b00525.
- T. Robbins, Y.-C. Liu, D. E. Cane and C. Khosla, Structure and Mechanism of Assembly Line Polyketide Synthases, Curr. Opin. Struct. Biol., 2016, 41, 10–18, DOI:10.1016/j.sbi.2016.05.009.
- B. R. Miller and A. M. Gulick, Structural Biology of Non-Ribosomal Peptide Synthetases, Methods Mol. Biol., 2016, 1401, 3–29, DOI:10.1007/978-1-4939-3375-4_1.
- R. D. Süssmuth and A. Mainz, Nonribosomal Peptide Synthesis—Principles and Prospects, Angew. Chem., Int. Ed., 2017, 56(14), 3770–3821, DOI:10.1002/anie.201609079.
- H. Wang, D. P. Fewer, L. Holm, L. Rouhiainen and K. Sivonen, Atlas of Nonribosomal Peptide and Polyketide Biosynthetic Pathways Reveals Common Occurrence of Nonmodular Enzymes, Proc. Natl. Acad. Sci. U. S. A., 2014, 111(25), 9259–9264, DOI:10.1073/pnas.1401734111.
- E. Vriens, D. De Ruysscher, A. N. M. Weir, S. Dekimpe, G. Steurs, A. Shemy, L. Persoons, A. R. Santos, C. Williams, D. Daelemans, M. P. Crump, A. Voet, W. De Borggraeve, E. Lescrinier and J. Masschelein, Polyketide Synthase-Mediated O-Methyloxime Formation in the Biosynthesis of the Oximidine Anticancer Agents, Angew. Chem., Int. Ed., 2023, 62(34), e202304476, DOI:10.1002/anie.202304476.
- J. W. Kim, K. Shin-ya, K. Furihata, Y. Hayakawa and H. Seto, Oximidines I and II: Novel Antitumor Macrolides from Pseudomonas Sp., J. Org. Chem., 1999, 64(1), 153–155, DOI:10.1021/jo9814997.
- Y. Hayakawa, T. Tomikawa, K. Shin-ya, N. Arao, K. Nagai and K. Suzuki, Oximidine III, a New Antitumor Antibiotic against Transformed Cells from Pseudomonas Sp. I. Taxonomy, Fermentation, Isolation, Physico-Chemical Properties and Biological Activity, J. Antibiot., 2003, 56(11), 899–904, DOI:10.7164/antibiotics.56.899.
- M. Pérez-Sayáns, J. M. Somoza-Martín, F. Barros-Angueira, J. M. G. Rey and A. García-García, V-ATPase Inhibitors and Implication in Cancer Treatment, Cancer Treat. Rev., 2009, 35(8), 707–713, DOI:10.1016/j.ctrv.2009.08.003.
- M. Huss and H. Wieczorek, Inhibitors of V-ATPases: Old and New Players, J. Exp. Biol., 2009, 212(3), 341–346, DOI:10.1242/jeb.024067.
- J. D. Tyzack and J. Kirchmair, Computational Methods and Tools to Predict Cytochrome P450 Metabolism for Drug Discovery, Chem. Biol. Drug Des., 2019, 93(4), 377–386, DOI:10.1111/cbdd.13445.
- G. Cruciani, E. Carosati, B. De Boeck, K. Ethirajulu, C. Mackie, T. Howe and R. Vianello, MetaSite: Understanding Metabolism in Human Cytochromes from the Perspective of the Chemist, J. Med. Chem., 2005, 48(22), 6970–6979, DOI:10.1021/jm050529c.
- J. Li, S. T. Schneebeli, J. Bylund, R. Farid and R. A. Friesner, IDSite: An Accurate Approach to Predict P450-Mediated Drug Metabolism, J. Chem. Theory Comput., 2011, 7(11), 3829–3845, DOI:10.1021/ct200462q.
- P. Rydberg, D. E. Gloriam, J. Zaretzki, C. Breneman and L. Olsen, SMARTCyp: A 2D Method for Prediction of Cytochrome P450-Mediated Drug Metabolism, ACS Med. Chem. Lett., 2010, 1(3), 96–100, DOI:10.1021/ml100016x.
- A. Rudik, A. Dmitriev, A. Lagunin, D. Filimonov and V. Poroikov, SOMP: Web Server for in Silico Prediction of Sites of Metabolism for Drug-like Compounds, Bioinformatics, 2015, 31(12), 2046–2048, DOI:10.1093/bioinformatics/btv087.
- M. K. Matlock, T. B. Hughes and S. J. Swamidass, XenoSite Server: A Web-Available Site of Metabolism Prediction Tool, Bioinformatics, 2015, 31(7), 1136–1137, DOI:10.1093/bioinformatics/btu761.
- S. Tian, X. Cao, R. Greiner, C. Li, A. Guo and D. S. Wishart, CyProduct: A Software Tool for Accurately Predicting the Byproducts of Human Cytochrome P450 Metabolism, J. Chem. Inf. Model., 2021, 61(6), 3128–3140, DOI:10.1021/acs.jcim.1c00144.
- M. Šícho, C. de Bruyn Kops, C. Stork, D. Svozil and J. Kirchmair, FAME 2: Simple and Effective Machine Learning Model of Cytochrome P450 Regioselectivity, J. Chem. Inf. Model., 2017, 57(8), 1832–1846, DOI:10.1021/acs.jcim.7b00250.
- S. Shaik, D. Kumar, S. P. de Visser, A. Altun and W. Thiel, Theoretical Perspective on the Structure and Mechanism of Cytochrome P450 Enzymes, Chem. Rev., 2005, 105(6), 2279–2328, DOI:10.1021/cr030722j.
- S. Shaik, S. Cohen, Y. Wang, H. Chen, D. Kumar and W. Thiel, P450 Enzymes: Their Structure, Reactivity, and Selectivity—Modeled by QM/MM Calculations, Chem. Rev., 2010, 110(2), 949–1017, DOI:10.1021/cr900121s.
- V. Guallar and R. A. Friesner, Cytochrome P450CAM Enzymatic Catalysis Cycle: A Quantum Mechanics/Molecular Mechanics Study, J. Am. Chem. Soc., 2004, 126(27), 8501–8508, DOI:10.1021/ja036123b.
- B. Meunier, S. P. de Visser and S. Shaik, Mechanism of Oxidation Reactions Catalyzed by Cytochrome P450 Enzymes, Chem. Rev., 2004, 104(9), 3947–3980, DOI:10.1021/cr020443g.
- J. Zurek, N. Foloppe, J. N. Harvey and A. J. Mulholland, Mechanisms of Reaction in Cytochrome P450: Hydroxylation of Camphor in P450cam, Org. Biomol. Chem., 2006, 4(21), 3931–3937, 10.1039/B611653A.
- J. Zheng, A. Altun and W. Thiel, Common System Setup for the Entire Catalytic Cycle of Cytochrome P450cam in Quantum Mechanical/Molecular Mechanical Studies, J. Comput. Chem., 2007, 28(13), 2147–2158, DOI:10.1002/jcc.20701.
- J. Oláh, A. J. Mulholland and J. N. Harvey, Understanding the Determinants of Selectivity in Drug Metabolism through Modeling of Dextromethorphan Oxidation by Cytochrome P450, Proc. Natl. Acad. Sci. U. S. A., 2011, 108(15), 6050–6055, DOI:10.1073/pnas.1010194108.
- R. Lonsdale, K. T. Houghton, J. Żurek, C. M. Bathelt, N. Foloppe, M. J. de Groot, J. N. Harvey and A. J. Mulholland, Quantum Mechanics/Molecular Mechanics Modeling of Regioselectivity of Drug Metabolism in Cytochrome P450 2C9, J. Am. Chem. Soc., 2013, 135(21), 8001–8015, DOI:10.1021/ja402016p.
- F. Peter Guengerich, M. R. Waterman and M. Egli, Recent Structural Insights into Cytochrome P450 Function, Trends Pharmacol. Sci., 2016, 37(8), 625–640, DOI:10.1016/j.tips.2016.05.006.
- J. Jumper, R. Evans, A. Pritzel, T. Green, M. Figurnov, O. Ronneberger, K. Tunyasuvunakool, R. Bates, A. Žídek, A. Potapenko, A. Bridgland, C. Meyer, S. A. A. Kohl, A. J. Ballard, A. Cowie, B. Romera-Paredes, S. Nikolov, R. Jain, J. Adler, T. Back, S. Petersen, D. Reiman, E. Clancy, M. Zielinski, M. Steinegger, M. Pacholska, T. Berghammer, S. Bodenstein, D. Silver, O. Vinyals, A. W. Senior, K. Kavukcuoglu, P. Kohli and D. Hassabis, Highly Accurate Protein Structure Prediction with AlphaFold, Nature, 2021, 596(7873), 583–589, DOI:10.1038/s41586-021-03819-2.
- M. Baek, F. DiMaio, I. Anishchenko, J. Dauparas, S. Ovchinnikov, G. R. Lee, J. Wang, Q. Cong, L. N. Kinch, R. D. Schaeffer, C. Millán, H. Park, C. Adams, C. R. Glassman, A. DeGiovanni, J. H. Pereira, A. V. Rodrigues, A. A. van Dijk, A. C. Ebrecht, D. J. Opperman, T. Sagmeister, C. Buhlheller, T. Pavkov-Keller, M. K. Rathinaswamy, U. Dalwadi, C. K. Yip, J. E. Burke, K. C. Garcia, N. V. Grishin, P. D. Adams, R. J. Read and D. Baker, Accurate Prediction of Protein Structures and Interactions Using a Three-Track Neural Network, Science, 2021, 373(6557), 871–876, DOI:10.1126/science.abj8754.
- M. L. Hekkelman, I. de Vries, R. P. Joosten and A. Perrakis, AlphaFill: Enriching AlphaFold Models with Ligands and Cofactors, Nat. Methods, 2023, 20(2), 205–213, DOI:10.1038/s41592-022-01685-y.
- M. van Kempen, S. S. Kim, C. Tumescheit, M. Mirdita, J. Lee, C. L. M. Gilchrist, J. Söding and M. Steinegger, Fast and Accurate Protein Structure Search with Foldseek, Nat. Biotechnol., 2023, 42, 243–246, DOI:10.1038/s41587-023-01773-0.
- M. Varadi, S. Anyango, M. Deshpande, S. Nair, C. Natassia, G. Yordanova, D. Yuan, O. Stroe, G. Wood, A. Laydon, A. Žídek, T. Green, K. Tunyasuvunakool, S. Petersen, J. Jumper, E. Clancy, R. Green, A. Vora, M. Lutfi, M. Figurnov, A. Cowie, N. Hobbs, P. Kohli, G. Kleywegt, E. Birney, D. Hassabis and S. Velankar, AlphaFold Protein Structure Database: Massively Expanding the Structural Coverage of Protein-Sequence Space with High-Accuracy Models, Nucleic Acids Res., 2022, 50(D1), D439–D444, DOI:10.1093/nar/gkab1061.
- M. Newcomb, R. Zhang, R. E. P. Chandrasena, J. A. Halgrimson, J. H. Horner, T. M. Makris and S. G. Sligar, Cytochrome P450 Compound I, J. Am. Chem. Soc., 2006, 128(14), 4580–4581, DOI:10.1021/ja060048y.
- D. A. Case, H. M. Aktulga, K. Belfon, D. S. Cerutti, G. A. Cisneros, V. W. D. Cruzeiro, N. Forouzesh, T. J. Giese, A. W. Götz, H. Gohlke, S. Izadi, K. Kasavajhala, M. C. Kaymak, E. King, T. Kurtzman, T.-S. Lee, P. Li, J. Liu, T. Luchko, R. Luo, M. Manathunga, M. R. Machado, H. M. Nguyen, K. A. O’Hearn, A. V. Onufriev, F. Pan, S. Pantano, R. Qi, A. Rahnamoun, A. Risheh, S. Schott-Verdugo, A. Shajan, J. Swails, J. Wang, H. Wei, X. Wu, Y. Wu, S. Zhang, S. Zhao, Q. Zhu, T. E. I. Cheatham, D. R. Roe, A. Roitberg, C. Simmerling, D. M. York, M. C. Nagan, K. M. Merz Jr and AmberTools, J. Chem. Inf. Model., 2023, 63(20), 6183–6191, DOI:10.1021/acs.jcim.3c01153.
- J. C. Gordon, J. B. Myers, T. Folta, V. Shoja, L. S. Heath and A. Onufriev, H++: A Server for Estimating pKas and Adding Missing Hydrogens to Macromolecules, Nucleic Acids Res., 2005, 33(suppl_2), W368–W371, DOI:10.1093/nar/gki464.
- J. Myers, G. Grothaus, S. Narayanan and A. Onufriev, A Simple Clustering Algorithm Can Be Accurate Enoughfor Use
in Calculations of pKs in Macromolecules, Proteins: Struct., Funct., Bioinf., 2006, 63(4), 928–938, DOI:10.1002/prot.20922.
- S. F. Altschul, T. L. Madden, A. A. Schäffer, J. Zhang, Z. Zhang, W. Miller and D. J. Lipman, Gapped BLAST and PSI-BLAST: A New Generation of Protein Database Search Programs, Nucleic Acids Res., 1997, 25(17), 3389–3402, DOI:10.1093/nar/25.17.3389.
-
L. Schrödinger and W. DeLano, PyMOL, 2020, https://www.pymol.org/pymol Search PubMed.
- S. Grimme, Exploration of Chemical Compound, Conformer, and Reaction Space with Meta-Dynamics Simulations Based on Tight-Binding Quantum Chemical Calculations, J. Chem. Theory Comput., 2019, 15(5), 2847–2862, DOI:10.1021/acs.jctc.9b00143.
- P. Pracht, F. Bohle and S. Grimme, Automated Exploration of the Low-Energy Chemical Space with Fast Quantum Chemical Methods, Phys. Chem. Chem. Phys., 2020, 22(14), 7169–7192, 10.1039/C9CP06869D.
- C. Bannwarth, S. Ehlert and S. Grimme, GFN2-xTB—An Accurate and Broadly Parametrized Self-Consistent Tight-Binding Quantum Chemical Method with Multipole Electrostatics and Density-Dependent Dispersion Contributions, J. Chem. Theory Comput., 2019, 15(3), 1652–1671, DOI:10.1021/acs.jctc.8b01176.
- W. Humphrey, A. Dalke and K. Schulten, VMD: Visual Molecular Dynamics, J. Mol. Graphics, 1996, 14(1), 33–38, DOI:10.1016/0263-7855(96)00018-5.
- X. He, V. H. Man, W. Yang, T.-S. Lee and J. Wang, A Fast and High-Quality Charge Model for the next Generation General AMBER Force Field, J. Chem. Phys., 2020, 153(11), 114502, DOI:10.1063/5.0019056.
- C. I. Bayly, P. Cieplak, W. Cornell and P. A. Kollman, A Well-Behaved Electrostatic Potential Based Method Using Charge Restraints for Deriving Atomic Charges: The RESP Model, J. Phys. Chem., 1993, 97(40), 10269–10280, DOI:10.1021/j100142a004.
-
M. J. Frisch, G. W. Trucks, H. B. Schlegel, G. E. Scuseria, M. A. Robb, J. R. Cheeseman, G. Scalmani, V. Barone, G. A. Petersson, H. Nakatsuji, X. Li, M. Caricato, A. V. Marenich, J. Bloino, B. G. Janesko, R. Gomperts, B. Mennucci, H. P. Hratchian, J. V. Ortiz, A. F. Izmaylov, J. L. Sonnenberg, D. Williams-Young, F. Ding, F. Lipparini, F. Egidi, J. Goings, B. Peng, A. Petrone, T. Henderson, D. Ranasinghe, V. G. Zakrzewski, J. Gao, N. Rega, G. Zheng, W. Liang, M. Hada, M. Ehara, K. Toyota, R. Fukuda, J. Hasegawa, M. Ishida, T. Nakajima, Y. Honda, O. Kitao, H. Nakai, T. Vreven, K. Throssell, J. A. Montgomery Jr, J. E. Peralta, F. Ogliaro, M. J. Bearpark, J. J. Heyd, E. N. Brothers, K. N. Kudin, V. N. Staroverov, T. A. Keith, R. Kobayashi, J. Normand, K. Raghavachari, A. P. Rendell, J. C. Burant, S. S. Iyengar, J. Tomasi, M. Cossi, J. M. Millam, M. Klene, C. Adamo, R. Cammi, J. W. Ochterski, R. L. Martin, K. Morokuma, O. Farkas, J. B. Foresman and D. J. Fox, Gaussian16, 2016 Search PubMed.
- O. Trott and A. J. Olson, AutoDock Vina: Improving the Speed and Accuracy of Docking with a NewScoring
Function, Efficient Optimization and Multithreading, J. Comput. Chem., 2010, 31(2), 455–461, DOI:10.1002/jcc.21334.
- J. Eberhardt, D. Santos-Martins, A. F. Tillack and S. Forli, AutoDock Vina 1.2.0: New Docking Methods, Expanded Force Field, and Python Bindings, J. Chem. Inf. Model., 2021, 61(8), 3891–3898, DOI:10.1021/acs.jcim.1c00203.
- K. Shahrokh, A. Orendt, G. S. Yost and T. E. Cheatham III, Quantum Mechanically Derived AMBER-Compatible Heme Parameters for Various States of the Cytochrome P450 Catalytic Cycle, J. Comput. Chem., 2012, 33(2), 119–133, DOI:10.1002/jcc.21922.
- C. Tian, K. Kasavajhala, K. A. A. Belfon, L. Raguette, H. Huang, A. N. Migues, J. Bickel, Y. Wang, J. Pincay, Q. Wu and C. Simmerling, ff19SB: Amino-Acid-Specific Protein Backbone Parameters Trained against Quantum Mechanics Energy Surfaces in Solution, J. Chem. Theory Comput., 2020, 16(1), 528–552, DOI:10.1021/acs.jctc.9b00591.
- Y. Xiong, P. S. Shabane and A. V. Onufriev, Melting Points of OPC and OPC3 Water Models, ACS Omega, 2020, 5(39), 25087–25094, DOI:10.1021/acsomega.0c02638.
- A. Sengupta, Z. Li, L. F. Song, P. Li and K. M. Merz Jr, Parameterization of Monovalent Ions for the OPC3, OPC, TIP3P-FB, and TIP4P-FB Water Models, J. Chem. Inf. Model., 2021, 61(2), 869–880, DOI:10.1021/acs.jcim.0c01390.
- H. G. Petersen, Accuracy and Efficiency of the Particle Mesh Ewald Method, J. Chem. Phys., 1995, 103(9), 3668–3679, DOI:10.1063/1.470043.
- D. J. Tobias and C. L. Brooks III, Molecular Dynamics with Internal Coordinate Constraints, J. Chem. Phys., 1988, 89(8), 5115–5127, DOI:10.1063/1.455654.
- S. F. Altschul, W. Gish, W. Miller, E. W. Myers and D. J. Lipman, Basic Local Alignment Search Tool, J. Mol. Biol., 1990, 215(3), 403–410, DOI:10.1016/S0022-2836(05)80360-2.
- P. Urban, T. Lautier, D. Pompon and G. Truan, Ligand Access Channels in Cytochrome P450 Enzymes: A Review, Int. J. Mol. Sci., 2018, 19(6), 1617, DOI:10.3390/ijms19061617.
-
V. C. Kim, D. G. Kim, S. G. Lee, G. H. Lee, S. A. Lee and L. W. Kang, Crystal Structure of Cytochrome P450 184A1 from Streptomyces Avermitilis, 2023 Search PubMed.
- V. Guallar and B. Olsen, The Role of the Heme Propionates in Heme Biochemistry, J. Inorg. Biochem., 2006, 100(4), 755–760, DOI:10.1016/j.jinorgbio.2006.01.019.
- C. Aldag, I. A. Gromov, I. García-Rubio, K. von Koenig, I. Schlichting, B. Jaun and D. Hilvert, Probing the Role of the Proximal Heme Ligand in Cytochrome P450cam by Recombinant Incorporation of Selenocysteine, Proc. Natl. Acad. Sci. U. S. A., 2009, 106(14), 5481–5486, DOI:10.1073/pnas.0810503106.
- S. Yoshioka, T. Tosha, S. Takahashi, K. Ishimori, H. Hori and I. Morishima, Roles of the Proximal Hydrogen Bonding Network in Cytochrome P450cam-Catalyzed Oxygenation, J. Am. Chem. Soc., 2002, 124(49), 14571–14579, DOI:10.1021/ja0265409.
- H. Zhang, H. Lin, C. Kenaan and P. F. Hollenberg, Targeting of the Highly Conserved Threonine 302 Residue of Cytochromes P450 2B Family during Mechanism-Based Inactivation by Aryl Acetylenes, Arch. Biochem. Biophys., 2011, 507(1), 135–143, DOI:10.1016/j.abb.2010.09.006.
- I. G. Denisov, T. M. Makris, S. G. Sligar and I. Schlichting, Structure and Chemistry of Cytochrome P450, Chem. Rev., 2005, 105(6), 2253–2278, DOI:10.1021/cr0307143.
- D. Hamdane, H. Zhang and P. Hollenberg, Oxygen Activation by Cytochrome P450 Monooxygenase, Photosynth. Res., 2008, 98(1–3), 657–666, DOI:10.1007/s11120-008-9322-1.
- P. R. Ortiz de Montellano, Hydrocarbon Hydroxylation by Cytochrome P450 Enzymes, Chem. Rev., 2010, 110(2), 932–948, DOI:10.1021/cr9002193.
- V. Cojocaru, P. J. Winn and R. C. Wade, The Ins and Outs of Cytochrome P450s, Biochim. Biophys. Acta, Gen. Subj., 2007, 1770(3), 390–401, DOI:10.1016/j.bbagen.2006.07.005.
- L. J. Kingsley and M. A. Lill, Substrate Tunnels in Enzymes: Structure–Function Relationships and Computational Methodology, Proteins: Struct., Funct., Bioinf., 2015, 83(4), 599–611, DOI:10.1002/prot.24772.
- S. Zhuang, L. Zhang, T. Zhan, L. Lu, L. Zhao, H. Wang, J. A. Morrone, W. Liu and R. Zhou, Binding Specificity Determines the Cytochrome P450 3A4 Mediated Enantioselective Metabolism of Metconazole, J. Phys. Chem. B, 2018, 122(3), 1176–1184, DOI:10.1021/acs.jpcb.7b11170.
- M. Ekroos and T. Sjögren, Structural Basis for Ligand Promiscuity in Cytochrome P450 3A4, Proc. Natl. Acad. Sci. U. S. A., 2006, 103(37), 13682–13687, DOI:10.1073/pnas.0603236103.
-
O. Keita, S. Osami, A. Yuichiro and S. Hiroshi, Structure of the Manganese Protoporphyrin IX-Reconstituted CYP102A1 Heme Domain with N-Palmitoyl-L-Phenylalanine, 2023 Search PubMed.
-
L. M. Podust, G. Jennings, C. Calvet-Alvarez and A. Debnath, Structure of the Naegleria Fowleri CYP51 at 1.7 Angstroms Resolution, 2017 Search PubMed.
- D.-S. Lee, A. Yamada, H. Sugimoto, I. Matsunaga, H. Ogura, K. Ichihara, S. Adachi, S.-Y. Park and Y. Shiro, Substrate Recognition and Molecular Mechanism of Fatty Acid Hydroxylation by Cytochrome P450 from Bacillus Subtilis: Crystallographic, Spectroscopic, And Mutational Studies, J. Biol. Chem., 2003, 278(11), 9761–9767, DOI:10.1074/jbc.M211575200.
- G. B. McGaughey, M. Gagné and A. K. Rappé, π-Stacking Interactions: Alive and Well in Proteins, J. Biol. Chem., 1998, 273(25), 15458–15463, DOI:10.1074/jbc.273.25.15458.
- P. A. Williams, J. Cosme, A. Ward, H. C. Angove, D. Matak Vinković and H. Jhoti, Crystal Structure of Human Cytochrome P450 2C9 with Bound Warfarin, Nature, 2003, 424(6947), 464–468, DOI:10.1038/nature01862.
|
This journal is © The Royal Society of Chemistry 2024 |
Click here to see how this site uses Cookies. View our privacy policy here.