DOI:
10.1039/D3MD00651D
(Opinion)
RSC Med. Chem., 2024,
15, 1085-1095
AiZynth impact on medicinal chemistry practice at AstraZeneca†
Received
20th November 2023
, Accepted 15th February 2024
First published on 16th February 2024
Abstract
AstraZeneca chemists have been using the AI retrosynthesis tool AiZynth for three years. In this article, we present seven examples of how medicinal chemists using AiZynth positively impacted their drug discovery programmes. These programmes run the gamut from early-stage hit confirmation to late-stage route optimisation efforts. We also discuss the different use cases for which AI retrosynthesis tools are best suited.
Introduction
The DMTA (design, make, test, analyse) cycle is central to medicinal chemistry.1 The faster a drug discovery programme can progress through a DMTA cycle, the more iterations it can perform within a reasonable time span and the higher the likelihood of success. Artificial intelligence (AI) can be used to speed up each step of the DMTA cycle: generative AI can design thousands of molecules in a short timespan;2 deep neural networks (NN) can propose retrosyntheses of desired targets;3 image recognition tools can facilitate test readouts;4 and AI algorithms can cluster compounds in order to identify areas of underexplored chemical space.5 Indeed, at AstraZeneca, we have applied multiple tools for these purposes, including REINVENT,6,7 AiZynthFinder,8 and Lunit SCOPE IO.9
To increase the turnover of the DMTA cycle and thereby accelerate drug discovery, it is necessary to identify the rate-determining step. “Make” is the usual culprit, as each desired compound necessitates chemical synthesis, which can be slowed by limited starting material availability, unexpected side reactivity, lack of desired reactivity, difficult purification, et cetera. An efficient synthetic route to each target, starting from readily available building blocks, helps to shorten the “Make” phase and therefore the entire DMTA cycle. AI tools for retrosynthetic analysis can therefore help programmes deliver on their goals in a timely fashion.
All AstraZeneca chemists have access to a suite of tools called AiZynth, which is composed of modules that predict retrosynthetic routes and forward reactivity. The algorithms underlying most of these tools have been disclosed publicly—several having been developed by the Machine Learning for Pharmaceutical Discovery and Synthesis (MLPDS) consortium,10 in which AstraZeneca participated—but they are trained on proprietary Electronic Laboratory Notebook (ELN) data to improve their performance.11 One of these tools, AiZynthFinder, uses a NN-guided Monte Carlo Tree Search (MCTS) to predict a set of reaction templates that, in sequence, constitute multi-step retrosynthetic routes for each target molecule.‡ Because the median AiZynthFinder search takes less than three minutes to run, all target molecules that are designed for all projects at AstraZeneca are automatically sent to AiZynthFinder for retrosynthetic analysis at point of design. Over 5000 compounds per month are analysed, with the resulting routes available for chemist perusal. These proposed routes give a sense of synthetic feasibility and are routinely used at AstraZeneca to inform progression decisions. In addition, all or some of the steps from these routes have been used to deliver the targets themselves. Several such examples are described herein.
In addition to routine analogue synthesis, typically carried out on <100 mg scale, medicinal chemists must also deliver lead compounds on gram or decagram scale for in vivo experiments. In these cases, there is necessarily at least one known route to the target, but it is likely suboptimal for scale-up delivery. Typically, a combination of experimentation to improve existing steps in the route and re-designing other portions of the route is used to improve the target synthesis. For the latter, a multi-step retrosynthetic search like AiZynthFinder is usually insufficient. Instead, AiZynth deploys several tools for carrying out single-step retrosynthetic analysis.12 Within a single Graphical User Interface (GUI), a chemist can chain together these single-step results to form a bespoke retrosynthesis plan (see Supporting Information for snapshots). This is a deeper, more manual search than AiZynthFinder, and it has borne fruit for route optimisation, as described below.
Results and discussion
Design and progression decisions
“Design” and “Make” do not exist in a vacuum; they inform each other. The greater the complexity of a target, the stronger the design hypothesis must be to justify its synthesis, whereas a short retrosynthesis proposal can justify more speculative chemical space exploration. AiZynth results routinely help AstraZeneca chemists make quick synthetic feasibility assessments, which can be used to inform progression decisions, especially for the “rescue” of targets that would otherwise be parked.
On one of our programmes, REINVENT was used to design low molecular weight compounds in hopes of achieving blood–brain-barrier (BBB) penetration. Thousands of AI-generated compounds were winnowed down to several dozen, which were then passed to AiZynthFinder for rapid assessment. In parallel, a chemist quickly scanned the compounds visually and triaged them by perceived ease of synthesis. One compound, benzimidazole 1, did not fit into a straightforward cross-coupling manifold and was initially parked. AiZynthFinder proposed a one-step cyclisation from D-proline (2) and phenylenediamine 3 (Fig. 1A).13 The efficiency and feasibility of this synthesis were attractive, and the target was made in three steps from a cheaper starting material. Nitroaniline 4 was reduced to 3 and cyclised with N-Boc D-proline 5 to form benzimidazole 6, which was deprotected to deliver 1 (Fig. 1B). Unfortunately, 1 was inactive against the protein of interest and was therefore not assessed for BBB penetration.
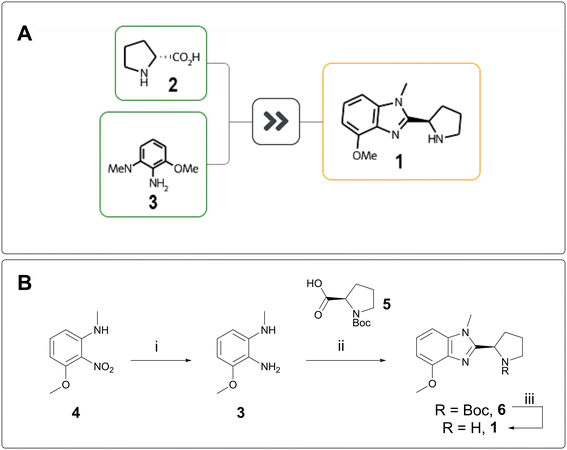 |
| Fig. 1 A) AiZynthFinder-proposed synthesis of 1. B) Laboratory synthesis of 1: i) Ni(II)Cl2·6H2O (1 equiv.), NaBH4 (4 equiv.), MeOH, 0 °C, 1 h, 96% yield; ii) isobutyl chloroformate (1 equiv.), N-methylmorpholine (1 equiv.), 5 (1 equiv.), 0 °C; then AcOH, 70 °C, 16 h, 93% yield; iii) HCl, MeOH, rt, 1 h, 92% yield. | |
An AstraZeneca project in the early hit-to-lead phase sought to improve potency by rigidifying the scaffold of compound 7.14 One design to address this moved a key R group from a pendant alkyl chain to the 1-position of an indane (compound 8), which added significant complexity (Fig. 2). In the early stages of a programme, heavy synthetic commitment is undesirable in the face of numerous hypotheses to be tested. To support the new design, a chemist used AiZynth's single-step retrosynthesis tool to predict starting materials for intermediate 9. AiZynth found a commercial indanone starting material (10) that could provide 9 by reductive amination with an ammonia source.15 The commercial availability of starting material and the plausible synthesis of the key intermediate provided enough momentum to progress the design of the rigidified molecule, and it was synthesised in two steps—oxime formation and Zn reduction—that are formally equivalent to reductive amination.16 The new scaffold was threefold more potent than the acyclic hit, providing the basis for a new chemical series that is still in active exploration.
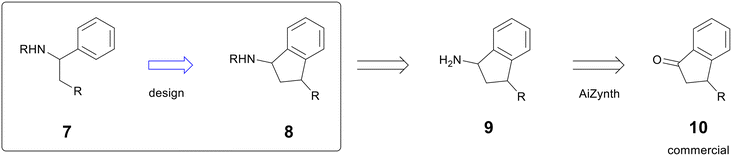 |
| Fig. 2 Example of AiZynth proposing a commercial starting material to a desired intermediate. Additional substitution has been omitted to focus on desired reactivity. | |
Analogue generation
The bread and butter of medicinal chemistry is routine analogue generation. Chemists quickly acquire knowledge and intuition about the reactivity of the core scaffolds that underpin their lead series. AI retrosynthesis can struggle to impact routine analogue generation because most compounds are designed with synthesis in mind already; chemists mentally bolt building blocks onto their core scaffolds and propose compounds after having already found commercially available precursors. However, we have found that AiZynth is particularly powerful for three use cases within the hit-to-lead and lead optimisation space: diversity-oriented synthesis (DOS), scaffold hopping, and the high priority synthesis of difficult targets or building blocks.
Diversity-oriented synthesis allows medicinal chemists to explore SAR quickly by probing a portion of chemical space with multiple analogues.17 As a project evolves, it may become desirable to alter the order of synthetic steps in order to facilitate DOS. In one case at AstraZeneca, the project team sought to deploy a late-stage Suzuki–Miyaura coupling using a borylated core. In order to deliver analogues rapidly, this core would need to be functionalised with the right-hand-side of the molecule in place, which contains an internal alkyne. An initial synthesis of this building block from bromoiodoarene 11 failed because the Miyaura borylation of alkyne 12 did not proceed (Fig. 3). At this point, a chemist used AiZynth's one-step retrosynthesis tool, which proposed an unorthodox Sonogashira coupling on boronate ester 13.18 Although deleterious Suzuki–Miyaura homocoupling or oligomerisation could be envisioned, sufficient precedent was found to carry out the proposal.19,20 This route adjustment—transposition of two fundamental transformations—was carried out successfully. Intermediate 14 has been used to deliver >70 analogues to date.
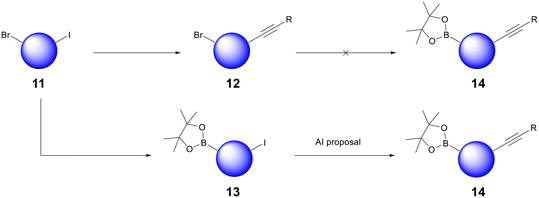 |
| Fig. 3 Example of AiZynth's impact on DOS: an unorthodox Sonogashira reaction carried out on boronate 13 without deleterious Suzuki reactivity yielded high value synthetic intermediate 14. | |
Scaffold hopping is the attempted transfer of SAR from a known series onto a related, novel series.21 As such, the synthesis of scaffold hop compounds will no longer rely on the established building blocks used for existing project routes. For one of our programmes, tetrahydro-γ-carboline 15 was proposed to introduce further sp3 character and potentially access a new vector. From a synthetic perspective, this was significantly outside of project chemistry space. AiZynthFinder proposed a one-step, three-component coupling between phenylhydrazine 16, bromide 17, and ketone 18 entailing hydrazine alkylation and subsequent Fischer indole cyclisation (Fig. 4A).22 To minimise side reactivity, this one-pot proposal was split into two steps, with isolation of hydrazine 19 before subjection to Fischer indole synthesis (Fig. 4B). The resulting tetrahydro-γ-carboline was successfully delivered using the proposed chemistry.
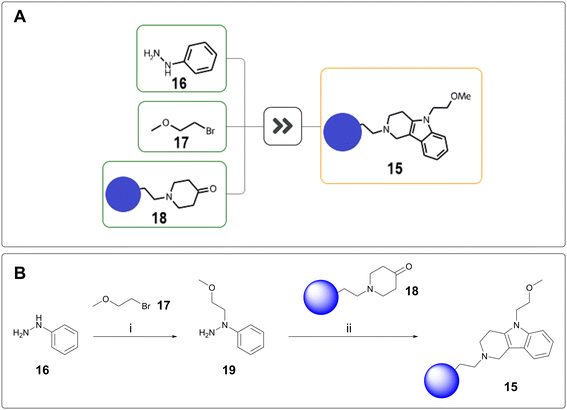 |
| Fig. 4 A) AiZynthfinder-proposed synthesis of 15. B) Laboratory synthesis of 15: i) Na2CO3 (2 equiv.), water, 100 °C, 16 h, 32% yield, 3 : 1 r.r.; ii) AcOH, 80 °C, 90 min; then HCl, 100 °C, 1 h, 13% yield. | |
Although new compounds are usually designed with an eye to commercial building blocks, it is almost inevitable that following SAR trends will lead to the design of compounds with component parts requiring bespoke synthesis. In one such case, a chemist used single step retrosynthesis for alcohol 20 (Fig. 5A). Among other suggestions, the software proposed Boc protection of 21, preceded by reductive amination with an ammonia source on aryl ketone 22.15 By expanding the retrosynthesis search back another step, the chemist found that the starting ketone was known in the literature, derived in one step from 2-bromopyridine and γ-butyrolactone.23 This sequence was used, with the addition of TBS as an orthogonal protecting group strategy, to deliver intermediate 20 and ultimately the desired target (Fig. 5B).
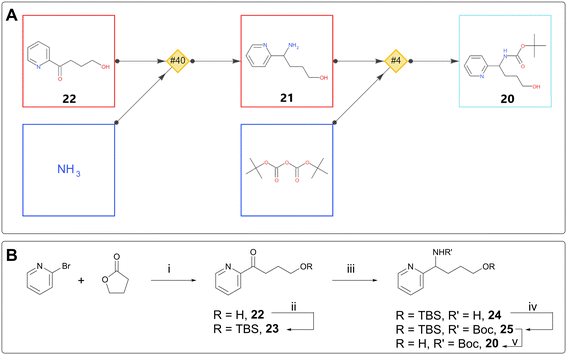 |
| Fig. 5 A) Two one-step retrosyntheses strung together to form a proposal for the synthesis of 20. The numbers in the diamond nodes represent the rank of the prediction, by feasibility. B) Laboratory synthesis of 20: i) n-BuLi (1.25 equiv.), Et2O, −78 °C to rt, 2 h, 64% yield; ii) imidazole (1.5 equiv.), TBSCl (1.2 equiv.), DCM, 0 °C to rt, 80% yield; iii) NH4OAc (10 equiv.), Na[(BH3)CN] (2 equiv.), MeCN/MeOH, 65 °C, 1 h, carried crude; iv) Boc2O (1.5 equiv.), NEt3 (3 equiv.), DCM, rt, 1 h, 30% yield; v) CuCl2 (0.2 equiv.), acetone/water, 80 °C, 2 h, 70% yield. | |
DEL off-DNA synthesis
DNA-encoded library (DEL) technology has seen extensive uptake by the pharmaceutical industry over the last 14 years.24,25 Initial hits from this screening process are not small molecules, because they are covalently linked to the DNA barcode, typically via a linear chain. In order to assess the validity of these hits, they must be made “off-DNA,” often with the linker truncated to a methyl group. Thus, the off-DNA hits are chemically distinct from the DEL hits and may require a different synthetic strategy from the library generation, especially if the building blocks for the DEL library are no longer available.
AiZynth is well suited to providing retrosyntheses for off-DNA DEL hits. From a technical perspective, the targets tend to be amenable to short, modular syntheses; from a human perspective, many hits must be assessed for synthetic feasibility simultaneously, without the base of knowledge on existing chemical series that exists for a later-stage project. One such example from AstraZeneca concerns amide 26, an off-DNA DEL hit for an ongoing programme. The initial synthesis proposed to make 26 as a single diastereomer was eight steps long, starting from 2,3-dihydrofuran. A chemist used AiZynth for single-step retrosynthesis from a slightly modified target: stereo-undefined disubstituted tetrahydrofuran (THF) 27 (Fig. 6A). The tool proposed a simple amide coupling on amine 28, preceded by cyclisation between commercially available disubstituted THF 29 and hydroxyguanidine 30. This two-step route was obviously preferred. It was subsequently found that Boc protection of the primary amine was required to allow the cyclisation to take place. Boc-protected compound 31 is also commercially available. It was acquired and used in a three-step synthesis of desired compound 27, which was isolated as two unassigned diastereomers (Fig. 6B). Unfortunately, neither isomer was found to bind to the target protein by Surface Plasmon Resonance (SPR); however, the team was able to come to this conclusion much faster by using AiZynth output, and prioritise other hits accordingly.
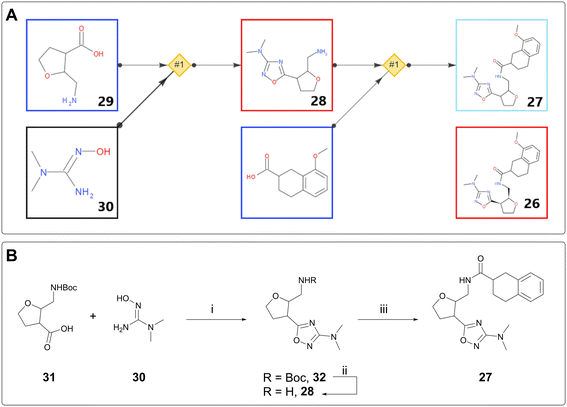 |
| Fig. 6 A) Two one-step retrosyntheses strung together for an AI-proposed synthesis of 27. Blue borders indicate commercial availability. B) Laboratory synthesis of 27: i) EDC (1.5 equiv.), HOBt (1.5 equiv.), NaHCO3 (3 equiv.), DMF, 60 °C, 3 h; ii) 3:1 DCM/TFA, rt, 2 h, 93% yield; iii) 8-methoxy-1,2,3,4-tetrahydronaphthalene-2-carboxylic acid (1 equiv.), chloro-N,N,N′,N′-tetramethylformamidinium hexafluorophosphate (1 equiv.), 1-methylimidazole (1 equiv.), DMF, rt, 16 h, 34% yield. | |
Route optimisation
The case studies above concern the delivery of milligram quantities of material for the purpose of conducting in vitro assays. In this case study, AiZynth was used to provide an alternative route to the lead compound, AZ3246 (33). A key retrosynthetic step to consider in the synthesis of AZ3246 is the disconnection between the pyrazine core and the pyrazole. Established syntheses made use of an SNAr reaction between triflate 34 and aminopyrazole 35.26 This route involved a potentially hazardous diazotisation step to form the triflate, so in parallel with de-risking the diazotisation, an alternative synthesis was considered. Namely, it was hypothesised that a cross-coupling between aminopyrazine 36 and a halopyrazole (37, 38) would achieve similar levels of efficiency to the SNAr (Fig. 7A). Halopyrazoles 37 and 38 were not known in the literature, so an AiZynth search was carried out using single-step retrosynthesis, with bromide 37 as the target. In addition to proposals that the team had already found were nonselective (NH pyrazole alkylation and electrophilic aromatic halogenation), AiZynth proposed an intriguing disconnection that no one on the team had previously thought of: decarboxylative bromination of acid 39 (Fig. 7B). This reaction template had been learned by AiZynth's NN from nineteen precedent reactions, several of which were deemed close enough to substrate 37 that the chemistry was worthy of laboratory experimentation.27–29
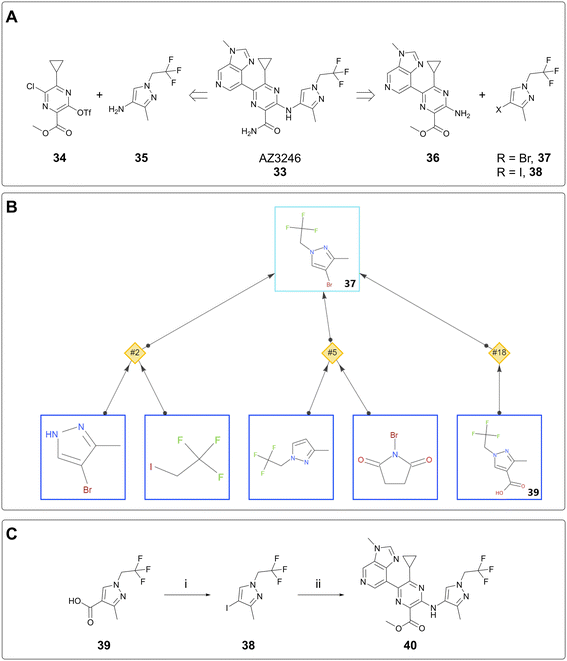 |
| Fig. 7 A) Two retrosynthetic strategies for appending pyrazole solvent tail. Left: SNAr strategy. Right: Cross-coupling strategy. B) Three proposals from AiZynth for synthesizing 37. C) Laboratory synthesis of 40via decarboxylative iodination: i) NIS (2 equiv.), LiOAc (0.2 equiv.), AcOH/water, 50 °C, 24 h, 92% yield; ii) 36 (1 equiv.), 38 (2 equiv.), CuI (0.30 equiv.), rac-trans-N1,N2-dimethylcyclohexane-1,2-diamine (0.20 equiv.), Cs2CO3 (1.5 equiv.), dioxane, 120 °C, 24 h, product detected. | |
In the event, N-bromosuccinimide (NBS) and sodium bicarbonate in DMF indeed converted pyrazole acid 39 to bromopyrazole 37 as observed by LC/MS. However, 37 was found to sublime during isolation, so iodopyrazole 38 was selected instead.§ Following condition optimisation, the decarboxylative iodination proceeded in 92% yield on >12 g (60 mmol) scale and was therefore a suitable step for preparing the pyrazole building block. This result is also the largest scale instance of an AI-proposed reaction run at AstraZeneca to date. The subsequent, human-proposed step was a Cu-catalysed Jourdan–Ullmann–Goldberg30,31 reaction between aminopyrazine 36 and iodopyrazole 38. On small scale, this reaction provided intermediate 40 (Fig. 7C), albeit not very cleanly. This reaction suffered from irreproducibility and did not prove to be scalable; extensive optimisation attempts were ultimately parked after the diazotisation/triflation sequence was thoroughly de-risked. Nevertheless, the AI-proposed decarboxylative iodination spurred optimisation of the synthesis of pyrazole acid 39, which was used in the scale-up route as reported elsewhere.26
Medicinal chemistry practice
Since 2020, when AiZynthFinder was integrated into our regular design workflow, it has generated retrosyntheses for over 130
000 molecules of interest to AstraZeneca chemists.¶ Of course, these results are only useful if viewed and evaluated by expert synthetic chemists. As the case studies above demonstrate, AiZynthFinder output can and has provided sound retrosynthetic suggestions to our chemists and has positively impacted drug discovery projects as a result. Integration with existing DMTA tools is key. Because AiZynthFinder automatically runs on all new designs, and because we have invested in the development of a user-friendly GUI, the barrier to entry is low for chemists to peruse AI proposals. Furthermore, AiZynth is integrated with our chemical inventory and commercial catalogues, allowing chemists to see at a glance which proposed starting materials and intermediates are readily available. We have also found such output to be useful for our colleagues in computational chemistry, who may not have a finely honed, intuitive sense of synthetic feasibility: a short route that terminates in commercial starting materials stands out and can be passed to synthetic chemists for closer evaluation. Thus, predicted synthetic feasibility joins the list of predicted properties that we use routinely, e.g. AZLogD (for lipophilicity) and FEP (Free Energy Perturbation; for binding affinity).32,33 We deploy such algorithmic predictions to augment human decision-making in the triage of compounds that progress from design to make.
Nevertheless, AI is not perfect. AiZynthFinder trades depth for speed and the results sometimes suffer accordingly. Common problems include proposals that would lead to undesired regioselectivity, functional group incompatibility, or overgeneralisation of precedented reactions to an inappropriate context.|| The tool is also not currently suited to providing retrosyntheses of large molecules like polypeptides and oligonucleotides. If the shallow AiZynthFinder search does not yield suitable results, more time-intensive AI tools within AiZynth can be brought to bear. This suite includes single-step retrosynthesis, regioselectivity prediction, and impurity prediction.34,35 This process takes longer, and we have found it to be most useful for problems like challenging, high priority targets or route optimisation. It also necessitates more training on the available tools, which can be a barrier to uptake: a core group of around 30 chemists routinely use single-step retrosynthesis within AstraZeneca, roughly a third of the number of regular users of AiZynthFinder.
During this discussion of medicinal chemistry practice, it is worth commenting on expectations. New users of AI tools in general are often disappointed by the failure of AI to live up to their expectations,36,37 and chemists' interaction with AiZynth is no exception. The first molecule that most new users test is one that they have personally synthesised recently, and AiZynthFinder rarely replicates their route exactly. Due in part to our self-imposed requirement to run fast searches, AiZynthFinder often gets close to a good route. Thus, experienced users seek inspiration from AiZynth rather than perfection. This mindset also keeps the human firmly in control of the AI-human interface, which we view as a positive aspect.38 Perhaps the most important impact of AiZynth on the day-to-day practice of medicinal chemistry at AstraZeneca is that we are now accustomed to using AI tools and we understand their limits. With three years' experience using AiZynth, AstraZeneca chemists have developed an intuition about which AI tools are best suited to each use case. Crucially, this experience also helps to inform our data science colleagues of the most impactful problems to tackle next.
Conclusions
In this paper, we have highlighted several case studies in which AstraZeneca chemists used AiZynth to impact drug discovery programmes. Its output helped us to make faster decisions, solve synthetic challenges, and consider alternative routes. We have little doubt that many of these solutions could have been found by “traditional” means, in analogy to how literature references can also be found in a physical card catalogue instead of a Reaxys, SciFinder, or Google search. Likewise, the use of AiZynth helped to inspire medicinal chemistry efforts and saved time. As fundamental research improves AI retrosynthesis further, its impact on the field of medicinal chemistry will deepen. However, no matter how many technical advances are brought to bear, the core nature of medicinal chemistry remains: it is a complex, multi-parameter optimisation problem with the ultimate aim of improving patients' lives through novel treatments. AI, like numerous other technologies before it (e.g. NMR, UPLC/MS, cross-coupling chemistry, the internet) is now a tool in the toolbox of human chemists whose dedicated hard work and creative insights drive programmes forward.
Experimental
Chemistry
General methods.
All chemicals used were reagent grade and all solvents were anhydrous unless otherwise noted. Solvents were purchased from Sigma-Aldrich. Flash column chromatography was carried out using prepacked silica cartridges and eluted using an ISCO Combiflash Rf system or Biotage Selekt system. 1H and 13C NMR spectra were recorded on either a Bruker Avance 300 MHz, a Bruker NEO 500 MHz, or a Bruker nano-AV3HD 400 MHz. 1H chemical shifts are reported in ppm relative to solvent peaks as the internal reference. J values are reported in Hz. Splitting patterns are indicated as follows: s, singlet; d, doublet; t, triplet; q, quartet; quin, quintet; m, multiplet; br, broad peak. Mass spec detection was ESI with positive/negative switching and cone voltage = 10 V.
3-Methoxy-N1-methylbenzene-1,2-diamine (3).
Sodium tetrahydroborate (0.83 g, 22 mmol) was added to a suspension of nickel(II) chloride hexahydrate (1.31 g, 5.49 mmol) and 3-methoxy-N-methyl-2-nitroaniline 4 (1.00 g, 5.49 mmol) in MeOH (25 mL) at 0 °C over a period of 10 min. The resulting solution was stirred at room temperature for 1 h. The reaction mixture was then poured into saturated aqueous NaHCO3, which was then extracted twice with EtOAc (20 mL each). The combined organics were dried over MgSO4, filtered, and concentrated. The resulting residue was purified by silica gel chromatography, using 0–30% EtOAc/petroleum ether as eluent, to afford 3 (0.800 g, 96%) as a yellow oil. 1H NMR (400 MHz, DMSO-d6) δ 2.71 (3H, d, J = 4.3, NHCH3), 3.73 (3H, s, OCH3), 4.00 (2H, br s, 2H, NH2), 4.62 (1H, br d, J = 4.3, NHCH3), 6.16 (1H, d, J = 8.0, 1.0 C(6)H), 6.31 (1H, dd, J = 8.0, 1.0, C(4)H), 6.53 (1H, t, J = 8.0, C(5)H). m/z 153.2 (M + H)+.
tert-Butyl (R)-2-(4-methoxy-1-methyl-1H-benzo[d]imidazol-2-yl)pyrrolidine-1-carboxylate (6).
Isobutyl chloroformate (0.633 mL, 4.86 mmol) was added to a solution of (tert-butoxycarbonyl)-D-proline 5 (1.05 g, 4.86 mmol) and N-methylmorpholine (492 mg, 4.86 mmol) in THF (10 mL) at 0 °C. The reaction was stirred at 0 °C for 10 min. 3 (740 mg, 4.86 mmol) was added and the resulting solution was stirred at 0 °C for 1 h. Acetic acid (10 mL) was added and the resulting mixture was stirred at 70 °C for 16 h. The reaction mixture was poured into saturated aqueous NaHCO3, which was then extracted twice with EtOAc (20 mL each). The combined organics were dried over MgSO4, filtered, and concentrated. The resulting residue was purified by silica gel chromatography, using 0–100% EtOAc/petroleum ether as eluent, to afford 6 (1.5 g, 93%) as a yellow oil. NMR showed the presence of rotamers: 1H NMR (300 MHz, DMSO-d6) δ 0.95–1.43 (9H, m, tBu), 1.81–1.96 (2H, m, C(13)H2), 2.08–2.36 (2H, m, C(12)H2), 3.40–3.58 (2H, m, C(14)H2), 3.70–3.81 (3H, m, NCH3), 3.88 (3H, s, OCH3), 5.01–5.12 (1H, m, C(11)H), 6.64–6.74 (1H, m, C(4)H), 7.05–7.14 (2H, m, C(5,6)H). m/z 332.3 (M + H)+.
(R)-4-Methoxy-1-methyl-2-(pyrrolidin-2-yl)-1H-benzo[d]imidazole hydrochloride (1·HCl).
6 (300 mg, 0.91 mmol) was added to a solution of 3 M HCl in MeOH (1 mL, 3 mmol). The resulting mixture was stirred at room temperature for 1 h. The reaction was then concentrated. The resulting residue was purified by preparative HPLC to afford 1·HCl (224 mg, 92%) as a white solid. 1H NMR (400 MHz, DMSO-d6) δ 1.94–2.06 (1H, m, C(13)H), 2.15 (1H, dquin, J = 12.5, 6.2, C(13)H), 2.41–2.47 (2H, m, C(12)H2), 3.22–3.45 (2H, m, C(14)H2), 3.96 (3H, s, NCH3), 3.97 (3H, s, OCH3), 5.17 (1H, q, J =7.3, C(11)H), 6.95 (1H, dd, J = 7.5, 1.1, C(6)H), 7.31–7.41 (2H, m, C(4,5)H), 9.92 (1H, br s, NH2+), 11.03 (1H, br s, NH2+). m/z 232.1 (M + H)+.
1-(2-Methoxyethyl)-1-phenylhydrazine (19).
1-Bromo-2-methoxyethane 17 (3.0 mL, 32 mmol) was added to a mixture of phenylhydrazine 16 (3.2 mL, 32 mmol) and sodium carbonate (6.84 g, 64.5 mmol) in water (10 mL). The resulting mixture was stirred under reflux for 16 h. The reaction was then allowed to cool to room temperature, diluted with water (100 mL), and extracted three times with EtOAc (20 mL each). The combined organics were dried over Na2SO4, filtered, and concentrated. The resulting residue was purified by silica gel chromatography, using 5–100% EtOAc/hexanes as eluent, to afford 19 (1.729 g, 32%) as an apparent ∼3
:
1 mixture with regioisomer 1-(2-methoxyethyl)-2-phenylhydrazine. 1H NMR (500 MHz, DMSO-d6) δ 3.27 (3H, s, OCH3), 3.52 (2H, t, J = 5.3, C(9)H2), 3.59 (2H, t, J = 5.3, C(10)H2), 4.27 (2H, br s, NH2), 6.61 (1H, t, J = 7.2, C(8)H), 6.96 (2H, d, J = 7.9 C(2,3)H), 7.10–7.19 (2H, m, C(5,6)H). m/z 167.3 (M + H)+.
Tetrahydro-γ-carboline (15).
Acetic acid (10 mL) was added to a mixture of 19 (1.792 g, 10.78 mmol) and ketone 18 (10.8 mmol). The resulting mixture was stirred at 80 °C for 90 min. Concentrated aqueous HCl (5 mL, 60 mmol) was added and the resulting mixture was stirred at 100 °C for 1 h. The reaction was then allowed to cool to room temperature and concentrated. The resulting residue was purified by silica gel chromatography, using 0–25% EtOH/EtOAc as eluent. The resulting residue was further purified by reverse phase chromatography, using 2–98% MeCN/water as eluent and 0.1% formic acid as modifier, to afford 15 (14%) as a white solid.
4-((tert-Butyldimethylsilyl)oxy)-1-(pyridin-2-yl)butan-1-one (23).
TBSCl (0.515 g, 3.41 mmol) was added to a solution of 4-hydroxy-1-(pyridin-2-yl)butan-1-one 22 (0.470 g, 2.85 mmol) and imidazole (0.291 g, 4.27 mmol) in DCM (8 mL) at 0 °C. The reaction was then stirred at room temperature for 2 h. The reaction was diluted with saturated aqueous NaHCO3 (10 mL) and the layers were separated. The aqueous layer was extracted with DCM (10 mL) and the combined organics were washed with brine (10 mL), dried over Na2SO4, and concentrated. The resulting residue was purified by silica gel chromatography, using 2–6% EtOAc/hexanes as eluent, to afford 23 (0.634 g, 80%) as an amber oil. 1H NMR (500 MHz, chloroform-d) δ 0.04 (6H, s, Si(CH3)2), 0.88 (9H, s, tBu), 1.98 (2H, quin, J = 6.8, C(4)H2), 3.29 (2H, t, J = 6.8, C(3)H2), 3.73 (2H, t, J = 6.8, C(5)H2), 7.42–7.52 (1H, m, C(17)H), 7.83 (1H, t, J = 7.8, C(16)H), 8.04 (1H, d, J = 7.8, C(15)H), 8.69 (1H, d, J = 4.6, m, C(18)H). m/z 281.3 (M + H)+.
rac-4-((tert-Butyldimethylsilyl)oxy)-1-(pyridin-2-yl)butan-1-amine (24).
A mixture of 23 (0.492 g, 1.76 mmol) and ammonium acetate (1.36 g, 17.6 mmol) in MeCN (4 mL) and MeOH (4 mL) was stirred at 65 °C for 30 min. After cooling to room temperature, sodium cyanoborohydride (0.221 g, 3.52 mmol) was added. The resulting mixture was stirred at 65 °C for 1 h, then allowed to cool to room temperature and concentrated. The resulting residue was diluted with saturated aqueous NaHCO3 (6 mL) and EtOAc (12 mL). The layers were separated and the aqueous layer was extracted twice with EtOAc (10 mL each). The combined organics were dried over Na2SO4 and concentrated to afford 24 as an amber oil which was taken forward crude assuming 100% yield. m/z 281.3 (M + H)+.
rac-tert-Butyl-(4-((tert-butyldimethylsilyl)oxy)-1-(pyridin-2-yl)butyl)carbamate (25).
Di-tert-butyl dicarbonate (0.577 g, 2.64 mmol) was added to a solution of 24 (0.494 g, 1.76 mmol) and triethylamine (0.736 mL, 5.28 mmol) in DCM (6 mL). The resulting solution was stirred at room temperature for 1 h and then diluted with water (6 mL). They layers were separated and the organic solution was washed with brine (6 mL), then dried over Na2SO4 and concentrated. The resulting residue was purified by silica gel chromatography, using 2–20% EtOAc/hexanes as eluent, to afford 25 (0.200 g, 30%) as an amber oil. 1H NMR (500 MHz, chloroform-d) δ 0.02 (6H, s, Si(CH3)2), 0.87 (9H, s, Si(tBu)), 1.44 (9H, s, CO(tBu)), 1.47–1.61 (2H, m, C(9)H2), 1.75–1.99 (2H, m, C(8)H2), 3.60 (2H, t, J = 6.3 C(10)H2), 4.76 (1H, br q, J = 6.6, C(7)H), 5.74 (1H, br s, NH), 7.12–7.26 (2H, m, C(3,5)H), 7.12–7.26 (1H, m, C(4)H), 8.56 (1H, br d, J = 4.4, C(6)H). m/z 381.3 (M + H)+.
rac-tert-Butyl-(4-hydroxy-1-(pyridin-2-yl)butyl)carbamate (20).
Copper(II) chloride (14 mg, 0.11 mmol) was added to a solution of 25 (0.2 g, 0.53 mmol) in acetone (3 mL) and water (0.15 mL). The resulting mixture was stirred at 80 °C for 2 hours. The reaction was then concentrated and the resulting residue was purified by silica gel chromatography, using 1–5% MeOH/DCM as eluent, to afford 20 (0.098 g, 70%) as a light green gum. 1H NMR (500 MHz, chloroform-d) δ 1.44 (9H, s, tBu), 1.52–1.65 (2H, m, C(11)H2), 1.77–1.97 (2H, m, C(10)H2), 3.55–3.73 (2H, m, C(12)H2), 4.81 (1H, br q, J = 6.9, C(9)H), 5.77 (1H, br d, J = 6.3, NH), 7.13–7.21 (1H, m, C(17)H), 7.24 (1H, d, J = 7.8, C(15)H), 7.65 (1H, t, J = 7.6, C(16)H), 8.54 (1H, d, J = 4.6, C(18)H). m/z 267.2 (M + H)+.
tert-Butyl ((3-(3-(dimethylamino)-1,2,4-oxadiazol-5-yl)tetrahydrofuran-2-yl)methyl)carbamate (32).
HOBt (281 mg, 1.83 mmol) was added to a mixture of 2-(((tert-butoxycarbonyl)amino)methyl)tetrahydrofuran-3-carboxylic acid 31 (300 mg, 1.22 mmol), 2-hydroxy-1,1-dimethylguanidine 30 (126 mg, 1.22 mmol), EDC hydrochloride (352 mg, 1.83 mmol), and sodium bicarbonate (308 mg, 3.67 mmol) in DMF (5 mL). The resulting mixture was stirred at 60 °C for 3 h. The reaction mixture was diluted with water (50 mL) and extracted three times with EtOAc (20 mL each). The combined organics were washed with brine (100 mL), dried over Na2SO4, filtered, and concentrated to afford 32 (0.200 g, 52%) as a yellow oil, which was used directly without further purification. 1H NMR (300 MHz, DMSO-d6) 1.34 (9H, s, tBu), 1.66–1.87 (1H, m, C(7)H), 2.03–2.18 (1H, m, C(7)H), 2.58–2.70 (2H, m, C(9)H2), 2.72 (3H, s, NCH3), 2.72 (3H, s, NCH3), 2.76–2.84 (1H, m, C(8)H), 3.75–3.89 (1H, m, C(6)H), 4.05 (1H, td, J = 8.21, 4.13 C(6)H), 5.09 (1H, d, J = 7.15, C(4)H), 6.87 (1H, br t, J = 5.09 NH). m/z 313.2 (M + H)+.
5-(2-(Aminomethyl)tetrahydrofuran-3-yl)-N,N-dimethyl-1,2,4-oxadiazol-3-amine (28).
TFA (2 mL) was added to a solution of 32 (190 mg, 0.61 mmol) in DCM (6 mL). The resulting mixture was stirred at room temperature for 2 h. The reaction was then concentrated to afford 28 (0.120 g, 93%), which was used directly without further purification. m/z 213.2 (M + H)+.
N-((3-(3-(Dimethylamino)-1,2,4-oxadiazol-5-yl)tetrahydrofuran-2-yl)methyl)-8-methoxy-1,2,3,4-tetrahydronaphthalene-2-carboxamide (27).
Chloro-N,N,N′,N′-tetramethylformamidinium hexafluorophosphate (145 mg, 0.520 mmol) was added to a solution of 28 (110 mg, 0.52 mmol), 8-methoxy-1,2,3,4-tetrahydronaphthalene-2-carboxylic acid (107 mg, 0.520 mmol), and 1-methylimidazole (43 mg, 0.52 mmol) in DMF (3 mL). The resulting mixture was stirred at room temperature for 16 h. The reaction was then diluted with water (100 mL) and extracted three times with EtOAc (20 mL each). The combined organics were washed with brine (150 mL), dried over Na2SO4, filtered, and concentrated. The resulting residue was purified by preparative HPLC, using an XSelect CSH Fluoro-Phenyl, 30 mm × 150 mm, 5 μm column, 27–37% MeCN/water as eluent, and 0.1% formic acid as modifier, to afford the (unassigned) diastereomers of 27 (0.039 g, 19%) and (0.032 g, 15%), each as a white solid. Isomer 1: 1H NMR (300 MHz, DMSO-d6) δ 1.42–1.64 (1H, m, C(19)H), 1.74–1.94 (1H, m, C(19)H), 2.05–2.23 (1H, m, C(20)H), 2.24–2.46 (3H, m, C(20)H, C(27)H2), 2.63–2.81 (3H, m, C(18)H, C(10)H2), 2.89 (6H, s, N(CH3)2), 3.20–3.41 (3H, m, C(9)H, C(14)H2), 3.73 (3H, s, OCH3), 3.78–3.99 (2H, m, C(11)H2), 4.07 (1H, q, J = 6.0, C(13)H), 6.65 (1H, d, J = 7.7, C(24)H), 6.71 (1H, d, J = 8.1, C(22)H), 7.04 (1H, t, J = 7.9, C(23)H), 7.99–8.15 (1H, m, NH). m/z 401.1 (M + H)+. Isomer 2: 1H NMR (300 MHz, DMSO-d6) δ 1.36–1.67 (1H, m, C(19)H), 1.74–1.92 (1H, m, C(19)H), 2.17–2.46 (4H, m, C(20)H2, C(27)H2), 2.64–2.83 (4H, m, C(10)H2, C(18)H, C(14)H), 2.90 (3H, s, NCH3), 2.91 (3H, s, NCH3), 3.08–3.21 (1H, m, C(14)H), 3.64–3.82 (5H, m, OCH3, C(11)H2), 4.00–4.23 (2H, m, C(13)H, C(9)H), 6.65 (1H, d, J = 7.5, C(24)H), 6.71 (1H, d, J = 8.3, C(22)H), 7.04 (1H, t, J = 7.9, C(23)H), 7.91 (1H, t, J = 5.4, NH). m/z 401.1 (M + H)+.
4-Iodo-3-methyl-1-(2,2,2-trifluoroethyl)-1H-pyrazole (38).
N-Iodosuccinimide (27.0 g, 120 mmol) was added to a solution of 3-methyl-1-(2,2,2-trifluoroethyl)-1H-pyrazole-4-carboxylic acid 39 (12.5 g, 60.1 mmol), and lithium acetate (0.793 g, 12.01 mmol), in acetic acid (125 mL) and water (25 mL). The resulting mixture was stirred at 50 °C for 24 h. The reaction was then diluted with water (125 mL) and MTBE (125 mL) and stirred for 10 min. The layers were separated and the aqueous layer was extracted twice with MTBE (60 mL each). The combined organic layers were washed with a solution of sodium thiosulfate pentahydrate (59.6 g, 240 mmol) in water (200 mL) upon which the solution turned from dark brown to yellow. The layers were separated and the organic layer was washed twice with water (125 mL each), twice with saturated aqueous NaHCO3 (125 mL each), and once with brine (125 mL), then dried by passing through a phase separation cartridge. The resulting solution was concentrated by rotary evaporation under 50 mbar at 30 °C for 45 min to afford 38 (15.95 g, 92%) as a yellow solid. 1H NMR (400 MHz, DMSO-d6) δ 2.14 (3H, s, CH3), 5.04 (2H, q, J = 9.1, CH2), 7.92 (1H, s, CH). m/z 290.9 (M + H)+.
Methyl 5-cyclopropyl-3-((3-methyl-1-(2,2,2-trifluoroethyl)-1H-pyrazol-4-yl)amino)-6-(1-methyl-1H-benzo[d]imidazol-4-yl)pyrazine-2-carboxylate hydrochloride (40·HCl).
Under nitrogen, a vial was charged with methyl 3-amino-5-cyclopropyl-6-(3-methyl-3H-imidazo[4,5-c]pyridin-7-yl)pyrazine-2-carboxylate 36 (160 mg, 0.49 mmol), 38 (286 mg, 0.99 mmol), caesium carbonate (241 mg, 0.74 mmol), copper(I) iodide (28.2 mg, 0.15 mmol), rac-trans-N1,N2-dimethylcyclohexane-1,2-diamine (0.015 mL, 0.10 mmol), and 1,4-dioxane (5 mL). The medium was purged with nitrogen, then heated to 120 °C for 24 h. The reaction was then added to a 0 °C solution of thionyl chloride (0.720 mL, 9.87 mmol) in MeOH (20 mL). The resulting mixture was stirred at room temperature for 18 h, then concentrated. The resulting residue was purified by C18 reverse phase chromatography, using 0–100% MeOH/water as eluent and 0.01% aqueous HCl as modifier, to afford 40·HCl as a yellow solid, though purity was low. m/z 487.2 (M + H)+
AiZynth
GitHub
AiZynthFinder is open source and can be found on GitHub at https://github.com/MolecularAI/aizynthfinder. AiZynthTrain is an open-source tool that can be used to train AiZynthFinder on datasets of interest; it can be found on GitHub at https://github.com/MolecularAI/aizynthtrain.
Training
All AiZynth searches described above were carried out on our internal version of the tool, which is trained on both publicly available and proprietary data. As has been described elsewhere, the inclusion of proprietary ELN data improves model performance.11
Citing precedent
AiZynth learns by generalising reported reactions into reaction templates, which are then applied to input molecules. Each template is associated with the list of reactions that AiZynth used to learn that template. These lists are not exhaustive catalogues of all instances of the reaction type in the literature, nor do they necessarily include the first report of a given reaction. When describing AiZynthFinder proposals, we have chosen to cite the earliest precedent from each respective template.
Author contributions
Conceptualization: JDS, AM, TR, BS, CT, REZ. Investigation: JDS, RH, GL, YL, CER, HR, TR, YZ, REZ. Writing – original draft: JDS. Writing – review & editing: JDS.
Conflicts of interest
All authors of this article except YL and YZ are present or former employees of AstraZeneca and may have or have had a financial stake in the performance of the company.
Acknowledgements
AstraZeneca is a former member of the Machine Learning for Pharmaceutical Discovery and Synthesis (MLPDS) Consortium, which developed the ASKCOS tool on which some of AiZynth's modules are based. AiZynthFinder was developed by AstraZeneca and the work described herein was solely internally funded. The authors wish to acknowledge the excellent IT team that supported AiZynth within AstraZeneca during the time from which our examples were drawn, especially Alla Bushoy, Max Liu, Pavel Smirnov, and Simon Stoddart. Thanks also to present and past members of our internal Business Reference Group for AiZynth and CAZP, especially Samuel Genheden, Per-Ola Norrby, and Nessa Carson.
Notes and references
- A. T. Plowright, C. Johnstone, J. Kihlberg, J. Pettersson, G. Robb and R. A. Thompson, Drug Discovery Today, 2012, 17, 56–62 CrossRef CAS PubMed.
- D. Merk, L. Friedrich, F. Grisoni and G. Schneider, Mol. Inf., 2018, 37, 1700153 CrossRef PubMed.
- Y. Jiang, Y. Yu, M. Kong, Y. Mei, L. Yuan, Z. Huang, K. Kuang, Z. Wang, H. Yao, J. Zou, C. W. Coley and Y. Wei, Engineering, 2022, 25, 32–50 CrossRef.
- S. Lin, K. Schorpp, I. Rothenaigner and K. Hadian, Drug Discovery Today, 2020, 25, 1348–1361 CrossRef CAS PubMed.
- X. Li, Y. Xu, H. Yao and K. Lin, J. Cheminf., 2020, 12, 42 CAS.
- T. Blaschke, J. Arús-Pous, H. Chen, C. Margreitter, C. Tyrchan, O. Engkvist, K. Papadopoulos and A. Patronov, J. Chem. Inf. Model., 2020, 60, 5918–5922 CrossRef CAS PubMed.
- M. Olivecrona, T. Blaschke, O. Engkvist and H. Chen, J. Cheminf., 2017, 9, 48 Search PubMed.
- S. Genheden, A. Thakkar, V. Chadimová, J.-L. Reymond, O. Engkvist and E. Bjerrum, J. Cheminf., 2020, 12, 70 Search PubMed.
- S. Park, C.-Y. Ock, H. Kim, S. Pereira, S. Park, M. Ma, S. Choi, S. Kim, S. Shin, B. J. Aum, K. Paeng, D. Yoo, H. Cha, S. Park, K. J. Suh, H. A. Jung, S. H. Kim, Y. J. Kim, J.-M. Sun, J.-H. Chung, J. S. Ahn, M.-J. Ahn, J. S. Lee, K. Park, S. Y. Song, Y.-J. Bang, Y.-L. Choi, T. S. Mok and S.-H. Lee, J. Clin. Oncol., 2022, 40, 1916–1928 CrossRef CAS PubMed.
- T. J. Struble, J. C. Alvarez, S. P. Brown, M. Chytil, J. Cisar, R. L. DesJarlais, O. Engkvist, S. A. Frank, D. R. Greve, D. J. Griffin, X. Hou, J. W. Johannes, C. Kreatsoulas, B. Lahue, M. Mathea, G. Mogk, C. A. Nicolaou, A. D. Palmer, D. J. Price, R. I. Robinson, S. Salentin, L. Xing, T. Jaakkola, W. H. Green, R. Barzilay, C. W. Coley and K. F. Jensen, J. Med. Chem., 2020, 63, 8667–8682 CrossRef CAS PubMed.
- S. Genheden, P.-O. Norrby and O. Engkvist, J. Chem. Inf. Model., 2023, 63, 1841–1846 CrossRef CAS PubMed.
- P. Torren Peraire, A. K. Hassen, S. Genheden, J. Verhoeven, D. Clevert, M. Preuss and I. V. Tetko, Digital Discovery, 2024 10.1039/D3DD00252G.
- M. A. Phillips, J. Chem. Soc., 1929, 2820–2828, 10.1039/JR9290002820.
- A. Y. S. Balazs, R. J. Carbajo, N. L. Davies, Y. Dong, A. W. Hird, J. W. Johannes, M. L. Lamb, W. McCoull, P. Raubo, G. R. Robb, M. J. Packer and E. Chiarparin, J. Med. Chem., 2019, 62, 9418–9437 CrossRef CAS PubMed.
- R. Leuckart and H. Janssen, Ber. Dtsch. Chem. Ges., 1889, 22, 1409–1413 CrossRef.
- K. Abiraj and D. C. Gowda, J. Chem. Res., 2003, 2003, 332–334 CrossRef.
- W. R. J. D. Galloway, A. Isidro-Llobet and D. R. Spring, Nat. Commun., 2010, 1, 80 CrossRef PubMed.
- K. Sonogashira, Y. Tohda and N. Hagihara, Tetrahedron Lett., 1975, 16, 4467–4470 CrossRef.
- E. K. Perttu, M. Arnold and P. M. Iovine, Tetrahedron Lett., 2005, 46, 8753–8756 CrossRef CAS.
- J. Kulhánek, F. Bureš and M. Ludwig, Beilstein J. Org. Chem., 2009, 5, 11 Search PubMed.
- H. Sun, G. Tawa and A. Wallqvist, Drug Discovery Today, 2012, 17, 310–324 CrossRef CAS PubMed.
- H. Ohno, H. Tanaka and T. Takahashi, Synlett, 2004, 2004, 508–511 Search PubMed.
- L. Miao, S. C. DiMaggio and M. L. Trudell, Synthesis, 2010, 2010, 91–97 CrossRef.
- M. A. Clark, R. A. Acharya, C. C. Arico-Muendel, S. L. Belyanskaya, D. R. Benjamin, N. R. Carlson, P. A. Centrella, C. H. Chiu, S. P. Creaser, J. W. Cuozzo, C. P. Davie, Y. Ding, G. J. Franklin, K. D. Franzen, M. L. Gefter, S. P. Hale, N. J. V. Hansen, D. I. Israel, J. Jiang, M. J. Kavarana, M. S. Kelley, C. S. Kollmann, F. Li, K. Lind, S. Mataruse, P. F. Medeiros, J. A. Messer, P. Myers, H. O'Keefe, M. C. Oliff, C. E. Rise, A. L. Satz, S. R. Skinner, J. L. Svendsen, L. Tang, K. van Vloten, R. W. Wagner, G. Yao, B. Zhao and B. A. Morgan, Nat. Chem. Biol., 2009, 5, 647–654 CrossRef CAS PubMed.
- P. R. Fitzgerald and B. M. Paegel, Chem. Rev., 2021, 121, 7155–7177 CrossRef CAS PubMed.
-
S. Nilsson Lill, K. Giblin, J. G. Kettle, F. W. Goldberg, N. P. Grimster, L. Morrill, R. Escobar, A. Metrano, K. Song, J. Sheppeck, R. E. Ziegler, Y. Wu, L. Sha, D. Wu, T. Grebe, A. Mfuh, A. Balazs and J. Shields, WO2023001794A1, 2023.
- O. S. Attaryan, G. A. Akopyan, K. S. Badalyan and G. V. Asratyan, Russ. J. Gen. Chem., 2007, 77, 307–308 CrossRef CAS.
- V. M. Vinogradov, T. I. Cherkasova, I. L. Dalinger and S. A. Shevelev, Russ. Chem. Bull., 1993, 42, 1552–1554 CrossRef.
-
R. Bonjouklian, R. D. Dally, A. de Dios, M. F. Del Prado Catalina, C. Dominguez-Fernandez, C. Jaramillo Aguado, B. Lopez de Uralde-Garmendia, C. Montero Salgado and T. A. Shepherd, WO2005080380A1, 2005.
- I. Goldberg, Ber. Dtsch. Chem. Ges., 1906, 39, 1691–1692 CrossRef.
- J. A. Olson and K. M. Shea, Acc. Chem. Res., 2011, 44, 311–321 CrossRef CAS PubMed.
- P. Bruneau and N. R. McElroy, J. Chem. Inf. Model., 2006, 46, 1379–1387 CrossRef CAS PubMed.
- D. H. O'Donovan, C. Gregson, M. J. Packer, R. Greenwood, K. G. Pike, S. Kawatkar, A. Bloecher, J. Robinson, J. Read, E. Code, J. H. Hsu, M. Shen, H. Woods, P. Barton, S. Fillery, B. Williamson, P. B. Rawlins and S. K. Bagal, Bioorg. Med. Chem. Lett., 2021, 39, 127904 CrossRef PubMed.
- Y. Guan, C. W. Coley, H. Wu, D. Ranasinghe, E. Heid, T. J. Struble, L. Pattanaik, W. H. Green and K. F. Jensen, Chem. Sci., 2021, 12, 2198–2208 RSC.
- C. W. Coley, W. Jin, L. Rogers, T. F. Jamison, T. S. Jaakkola, W. H. Green, R. Barzilay and K. F. Jensen, Chem. Sci., 2019, 10, 370–377 RSC.
- D. B. Shank, C. Graves, A. Gott, P. Gamez and S. Rodriguez, Comput. Hum. Behav., 2019, 98, 256–266 CrossRef.
- S. M. Jones-Jang and Y. J. Park, J. Comput.-Mediat. Commun., 2023, 28, zmac029 CrossRef.
- J. Bongard and M. Levin, Front. Ecol. Evol., 2021, 9, 650726 CrossRef.
Footnotes |
† Electronic supplementary information (ESI) available: NMR spectra of new compounds and snapshots of the AiZynth GUI. See DOI: https://doi.org/10.1039/d3md00651d |
‡ In addition to the application of machine-learned templates, the MCTS also applies a database of AstraZeneca ELN and publicly available reactions in an attempt to find exact matches to the target molecule and its proposed intermediates. In this way a multi-step retrosynthesis generated by AiZynthFinder can comprise both experimental and predicted reactions. |
§ Iodopyrazole 38 also sublimes, but at a higher temperature and lower pressure than bromopyrazole 37, rendering it more suitable for isolation. |
¶ The reader will notice that for several of the examples above, a successful application of AiZynth to make the target molecules or improve the desired route did not succeed in addressing potency challenges or other project goals. This is to be expected, as the overwhelming majority of molecules made by medicinal chemists for drug discovery programmes are found to be wanting in one or more dimensions. |
|| Early problems also included protection/deprotection cycles, which had to be intentionally penalised in order to focus AiZynth on productive chemistry. We have found that protecting group strategy is still best decided by the chemist. Thus, the AI proposals discussed in the case studies do not make heavy use of protecting groups, whereas several of the laboratory syntheses do. |
|
This journal is © The Royal Society of Chemistry 2024 |
Click here to see how this site uses Cookies. View our privacy policy here.