DOI:
10.1039/D3NR03749E
(Paper)
Nanoscale, 2024,
16, 2904-2912
Unveiling protein corona formation around self-propelled enzyme nanomotors by nanoscopy†
Received
30th July 2023
, Accepted 11th November 2023
First published on 16th November 2023
Abstract
The interaction of nanoparticles with biological media is a topic of general interest for drug delivery systems and among those for active nanoparticles, also called nanomotors. Herein, we report the use of super resolution microscopy, in particular, stochastic optical reconstruction microscopy (STORM), to characterize the formation of a protein corona around active enzyme-powered nanomotors. First, we characterized the distribution and number of enzymes on nano-sized particles and characterized their motion capabilities. Then, we incubated the nanomotors with fluorescently labelled serum proteins. Interestingly, we observed a significant decrease of protein corona formation (20%) and different composition, which was studied by proteomic analysis. Moreover, motion was not hindered, as nanomotors displayed enhanced diffusion regardless of the protein corona. Elucidating how active particles interact with biological media and maintain their self-propulsion after protein corona formation will pave the way for the use of these systems in complex biological fluids in biomedicine.
Introduction
Inspired by nature, catalytic nanomotors that convert chemical energy into motion have been developed in the past decade.1 Particularly, enzymes are highly efficient natural catalysts that constitute a very promising strategy to build biocompatible micro- and nanoswimmers that self-propel using bioavailable fuels such as glucose,2–4 H2O2
5 or urea6–10 without the need for external power sources. This has opened new possibilities in the use of nanomotors for biomedical applications,11–13 and several milestones have already been reached, including enhanced anti-cancer drug delivery,14 improved cellular uptake,15 sensing16 and medical imaging.17–19 However, not all enzymes can generate active motion in a highly efficient manner, and urease is one of the most powerful enzymes to promote self-propulsion for micro- and nanoparticles.20 Urease-powered nanomotors have already been used to enhance anti-cancer drug delivery,14 and promote more efficient cellular uptake8 targeting and penetration of 3D spheroids15 and improved penetration and retention in the stomach.21 Additionally, urease-nanomotors are capable of self-propulsion within biological fluids such as urine15,18 and blood.8
Despite these exciting outcomes, some concerns have been raised for their use in biological media and potential biomedical applications.22 To tackle this issue, the challenges that all nanoparticle delivery systems face when they enter the organism should be considered. Upon nanoparticle administration, proteins, lipids and small metabolites present within the biological fluids instantly adsorb onto the nanoparticle surface, forming a protein corona that confers a new biological identity to the nanoparticles,23,24 altering their targeting capabilities,25,26 cellular uptake,27–30 circulation time and biodistribution.31 The protein corona composition and assembly strongly depend on the intrinsic properties of nanoparticles, including the size, shape and surface charges.32–34 Additionally, the characteristics of the surrounding environment can also modulate the protein corona formation, such as the pH31 or shear stress and flow.35,36 Several techniques have been used so far to analyze protein corona,37,38 with mass spectrometry-based proteomics39,40 and gel electrophoresis41 being the most extended approaches. When using these techniques, the protein corona analysis is performed in bulk. Recently, super resolution microscopy, in particular, STORM, has emerged as a powerful method to characterize the properties of nanomaterials,42 including the dynamics of protein corona formation around nanoparticles at the single particle level.43,44 Furthermore, STORM is the only current approach that allows visualization of the number and position of individual proteins, allowing for precise spatial mapping. This is particularly relevant in the case of enzyme powered micro- and nanomotors, since their enzymatic distribution is intrinsically heterogeneous, and a certain degree of heterogeneity in the formation of the protein corona around them may also be expected.45
Herein, we aimed at studying the formation of a protein corona around active urease-powered nanomotors using STORM with two main objectives: (i) to study how nanomotor activity affects the formation of the protein corona and (ii) to study how the protein corona formation affects the self-propulsion of nanomotors.
Results and discussion
Mesoporous silica nanoparticles were synthesized by using a modified Stöber method, as previously reported,15 where cetyltrimethylammonium bromide (CTAB) was employed to generate the mesopores, and triethanolamine (TEOA) and fluorescein isothiocyanate conjugated to (3-aminopropyl)triethoxysilane (FITC-APTES) were used as precursors (6
:
1 volume). The resulting particles were characterized using scanning electron microscopy (Fig. 1A), which showed monodisperse particles with a mean diameter of 434 ± 2 nm (mean ± SEM, N = 200). The inner cylindrical porous structure was verified using transmission electron microscopy (TEM, Fig. 1B) where an average pore size of 3 nm was determined. To functionalize the particles with urease, their surface was first modified with aminopropyltriethoxysilane (APTES) to provide them with available –NH2 functional groups. Urease was subsequently conjugated using glutaraldehyde (GA) as a linker (Fig. 1C). Nanomotors self-propel thanks to the conversion of urea into ammonia and carbon dioxide ((NH2)2CO + H2O → CO2 + 2NH3) by urease (Fig. 1D).45,46 To characterize the different functionalization steps, the electrophoretic mobility of the particles was monitored. Dynamic light scattering (DLS) showed that the initial unmodified particles have a surface z-potential of −29.5 ± 0.34 mV (Smoluchowski), as expected from the presence of silanol groups (Fig. 1E). After their modification with GA and urease, the z-potential of the particles was modified. As expected from the isoelectric points of the surface groups,47,48 amine modified silica yielded positive surface z-potentials and enzyme conjugation recovered the negative surface z-potential, confirming the presence of urease on the surface. Urease activity was determined by using a commercial enzyme activity assay kit (Fig. 1F).
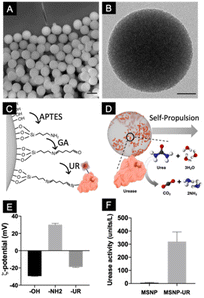 |
| Fig. 1 Fabrication and characterization of urease-powered nanomotors. (A) SEM micrograph of mesoporous silica nanoparticles. Scale bar = 500 nm. (B) Representative TEM micrograph of a mesoporous silica nanoparticle. Scale bar = 100 nm. (C) Schematic representation of the functionalization approach. (D) Schematic representation of the self-propulsion upon enzyme catalysis. (E) z-Potential characterization of the particles along the functionalization process. (F) Enzyme activity of the particles before and after their functionalization with urease. | |
To generate self-propulsion, an asymmetric distribution of the catalyst is always necessary in order to avoid a net compensation of forces. Recent works have reported that stochastic binding of enzymes onto micron-sized particles results in a non-homogeneous, patch-like distribution leading to the self-propulsion of biocatalytic micromotors without the need for physical asymmetries as the case of Janus particles.45,49 While this parameter has been thoroughly studied for micron-sized particles, which display a ballistic type of motion, little is known about nano-sized motors, which display a different type of motion known as enhanced diffusion.7 For this type of motion dynamics, several asymmetric2,3 and non-asymmetric structures have been reported.14,50,51
However, a robust and systematic analysis of the enzyme distribution onto nano-sized motors is missing. This is particularly relevant since two factors have already been demonstrated to be crucial for the self-propulsion of urease micromotors: an asymmetric distribution and the number of enzymes on the motor surface.45 Here, we used STORM to detect urease molecules bound to the nanomotor surface. For this, urease was previously labelled with a Cy5 fluorophore and a calibration to estimate the number of localisations recorded per single labelled urease was performed (Fig. S1†).45 Nanomotors functionalized with 25% labelled urease were placed onto a glass slide and allowed to precipitate until immobilized. Unbound nanomotors were washed out by replacing the remaining dispersion in PBS solution with the STORM buffer. Fig. 2A (right) shows the result of merging both conventional fluorescence and STORM images. Fig. 2B, C and S2† show representative examples of urease localizations and enzyme density, respectively. Generally, a non-uniform urease coating of nanomotors was observed (Fig. 2B and C), similar to what has been previously observed for micron-sized urease motors.45
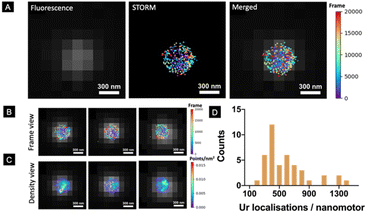 |
| Fig. 2 STORM characterization of urease molecules around the nanomotors. (A) STORM recorded localisations of Cy5-labelled enzymes are superimposed on the FITC fluorescence image for better visualization. The colour of a localisation represents the frame in which it was recorded. Scale bars are 300 nm. (B) Representative STORM images showing urease localizations in a nanomotor. (C) Corresponding images showing the localization density, where the distribution of urease can be visualized. (D) Histogram of the number of urease localisations per analysed nanomotor. The results are shown as the mean ± SD (n = 41). | |
Using STORM, we were able to determine the amount of urease molecules bound to the nanomotor surface by using a custom-made Python-based code, analyzing a minimum amount of 40 nanoparticles per case. First, the particle size was determined by using the information provided by the fluorescence images, with the mean nanoparticle radius being 254 ± 10 nm (mean ± SEM, N = 50), and these results were consistent with both TEM/SEM analysis results. STORM images enabled the quantification of urease molecules bound to the nanoparticle surface, with a mean of 584 ± 91 (mean ± SEM, N = 40).
Motion dynamics was studied by recording the nanomotors either with or without urea, at 100 mM in phosphate buffered saline (PBS 1×) solution, which is the optimal concentration of urea at which nanomotors move.14,50,52 Bright field videos were recorded for 30 s at a 25 FPS rate. Nanomotor trajectories were tracked using a custom-made Python-based code. Fig. 3A and B show the trajectories of the nanomotors with and without urea, respectively. From the trajectories, we extracted the Mean Squared Displacement (MSD), presented in Fig. 3C. For nanomotors both with and without fuel, a linear MSD was observed, which denotes a diffusive type of motion dynamics. However, in the case of the nanomotors with 100 mM urea, the slope of the MSD was significantly higher, indicating enhanced diffusion (Fig. 3C).7,53,54
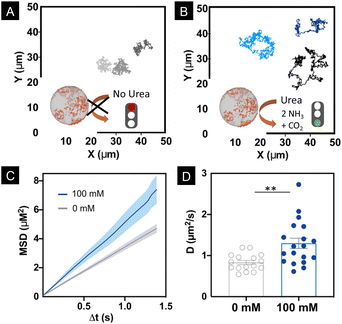 |
| Fig. 3 Motion dynamics of urease nanomotors. (A) Trajectories of nanomotors in the absence of fuel. (B) Trajectories of nanomotors in the presence of 100 mM urea. (C) Comparison between the MSD in the presence or absence of fuel. The results are shown as the mean ± SEM (n = 20). (D) Diffusion coefficient of the nanomotors calculated from the MSD. The results are shown as the mean ± SEM (n = 20). | |
Fig. 3D shows that the distribution of the obtained diffusion coefficients is significantly higher (P = 0.0035) in the case of 100 mM urea.
Addressing the performance of biomedical micro- and nanomotors in complex biological fluids is a critical aspect, since certain physiological conditions may hamper their motility. In this study, we monitored the motility of particles using PBS, which contains different salts and ionic species at a physiologically relevant concentration. The presence of ionic species has been recently demonstrated to impede the motion of micron-sized motors powered by urease.55 However, in the case of nano-sized motors, their motion persists in different types of media, including PBS,14,56 urine18,50 and blood,8 probably due to their different types of motion mechanisms. Nonetheless, biological fluids contain not only salts and ionic species but also other components such as proteins that might interact with the nanomotor surface. Here, to investigate the effect of the activity of nanomotors on the formation of protein corona in a quantitative manner, we incubated urease-nanomotors with Cy5-labelled serum proteins, either in the presence or absence of 100 mM urea (Fig. 4A). For this, urease nanomotors were incubated for 30 min in a solution containing non-labelled Fetal Bovine Serum (FBS), 5% Cy5 labelled FBS and 0 or 100 mM urea for the control and active samples, respectively (Fig. 4A). As in the previous experiments, green fluorescence was used to localize the nanomotor boundary and the images were superimposed with the STORM images (Fig. 4B and C), where labelled serum proteins were quantified. Fig. 4D shows a histogram depicting the distribution of FBS detection per nanomotor. In the presence of 100 mM urea, the peak of the histogram is significantly shifted to the left, indicating a lower average FBS detection per nanomotor, compared to the nanomotors without fuel. Fig. 4E shows a comparison of the average detected FBS points per nanomotor. Surprisingly, around 20% reduction on the FBS localizations was found between the control (615 ± 24 localizations, mean ± SEM) and active nanomotors (486 ± 21 localizations, mean ± s.e.m.). These results indicate a significant reduction (P = 0.002) of protein corona formation around the nanomotors when they are active. This effect could be explained by three factors. First, the urease activity of micromotors has already been reported to modify the local surrounding environment, leading to a pH increase.16,57 The formation and stability of the protein corona showed sensitivity to different pH levels58–60 and particle surface charges.61 The changes in the pH induced by the urease activity could thus result in an alteration of the physicochemical properties of the particle surface and the serum proteins, ultimately affecting the interactions between both. Second, the chemical activity of the nanomotors often leads to the creation of a shear flow around them.54 This is also a very interesting feature, since the protein corona has shown to be dependent not only on the physicochemical properties of the particles but also on the presence of shear stress and shear flow.35,36,62 Third, ionic products released from urease reaction generate ionic species around the particles,46 which may affect the electrostatic interaction between the proteins and particle surface, leading to an alteration of the protein corona. Future research is warranted in order to find the exact mechanisms underlying the changes in the protein corona around active nanomotors.
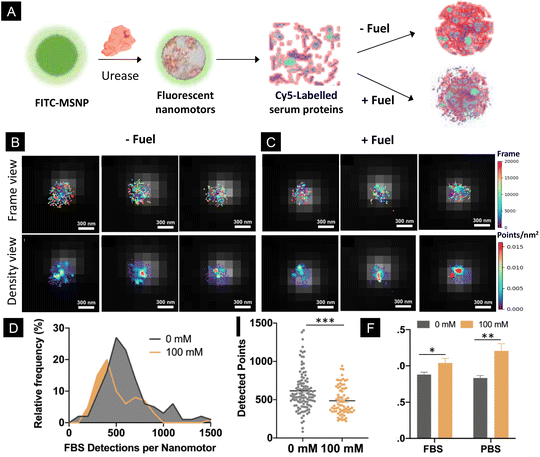 |
| Fig. 4 Protein corona formation around active nanomotors. (A) Schematic representation of the experimental approach, where fluorescent nanoparticles (FITC-MSNP) were functionalized with non-labelled urease and incubated with Cy5-labelled serum proteins either in the presence or absence of fuel. (B) Representative STORM images of nanomotors after their incubation with labelled FBS in the absence of fuel, showing FBS localizations (top) and FBS density (bottom). (C) Representative STORM images of nanomotors after their incubation with labelled FBS in the presence of fuel, showing FBS localizations (top) and FBS density (bottom). (D) Histogram of the FBS detection per nanomotor distribution. (E) Comparison of the average detected FBS proteins in nanomotors with and without urea. (F) Comparison of the diffusion coefficients of the nanomotors under different conditions. The results are shown as the mean ± SEM. | |
Since the self-propulsion of nanomotors is generated by the decomposition of urea catalyzed by urease, the presence of a protein corona on the particle surface could hamper the substrate and product exchange, limiting the motion performance. For this reason, we analyzed the motion of the nanomotors after the formation of the protein corona. We incubated the nanomotors with FBS as described previously, following the same procedure as that for the STORM imaging experiments. Then, the motors were resuspended in PBS 1× and their motion was analysed as described above. The results showed a significant increase (P = 0.047) in the diffusion coefficient of the nanomotors with 100 mM urea, constituting an increase of 18% with respect to the control (0 mM urea). This increase was slightly lower than the one observed for the nanomotors swimming in PBS, without being previously incubated with FBS, where a 44% increase in the diffusion coefficient was found. These results indicate that urease powered nanomotors can swim regardless of the diminished protein corona formation around their surface. However, their performance is slightly limited compared to that of the bare nanomotors, indicating that future strategies to further reduce protein corona might be desired.
Next, we investigated whether the activity of the nanomotors resulted in a change in protein corona composition. For this, after incubating the nanomotors with FBS for 30 min with or without fuel, we performed a proteomic analysis by digesting adsorbed proteins using a trypsin treatment, followed by protein identification using HPLC-mass spectrometry analysis. We identified a total number of 321 proteins, from which 236 protein groups were quantified. A differential expression analysis between 100 mM urea and no urea conditions was performed. Fig. 5A shows a Venn diagram of the proteins found for each condition and in common for both conditions. When we selected the 20 most abundant proteins for each condition (Fig. 5B), the changes in abundance were non-significant, although the tendency was to find a lower abundance for most proteins in the case of the urea conditions. Interestingly, for the non-urea conditions, the heat shock cognate 71 kDa protein and heat shock 70 kDa protein 1-like were among the most abundant, which was not the case for the urea conditions. Other examples are apolipoprotein C-II and ALB protein, which were among the 20 most abundant proteins under the urea conditions but not under the non-urea conditions.
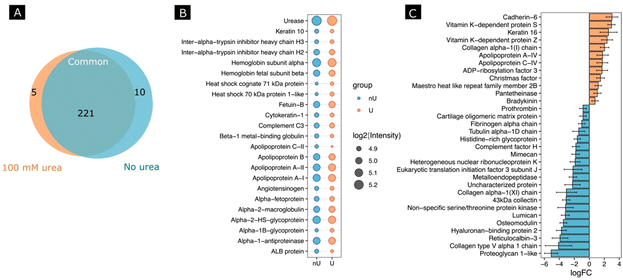 |
| Fig. 5 Proteomic analysis of protein corona composition around nanomotors when incubated for 30 min with FBS. (A) Venn diagram representing the number of proteins only present in the nanomotors exposed to urea and no urea and the number of proteins present under both conditions. (B) A dot plot displaying the most abundant proteins, according to their intensity. In each case, the results are shown as the mean log2(Intensity) of three independent biological replicates. nU: no urea; U: 100 mM urea. (C) Fold-change of the most significant proteins for the 100 mM urea conditions (light orange) with respect to the no urea (light blue) conditions. The results are shown as the mean ± SD. | |
Moreover, several significant proteins were identified using standard cutoffs for fold changes (|FC| > 1.5) and adjusted p-value (padj < 0.05) when comparing the urea and non-urea conditions (Fig. S3†). In this regard, 13 proteins were shown to be up-regulated and 21 were down-regulated (Fig. 5C).
Conclusions
This study reports for the first time the relationship behind enzyme-driven motion and protein corona, highlighting the relevance of extending these studies to the micro- and nanomotor community, since the effects on their propulsion capabilities might depend on the particle properties and their interaction with biological media. In this regard, protein corona plays a crucial role in the translation of micro-/nanomotors to biomedical applications, as it is key to determining their biodistribution. Here, we report that the protein corona is significantly reduced when the nanomotors are active, which might be desirable for certain biomedical applications, as it is an example of targeted therapeutics, where the protein corona may hamper the targeting properties of functionalized nanoparticles. Indeed, the biomedical performance of nanomotors depends not only on their mobility but also on the nanomotor–cell interactions (e.g. targeting). The protein corona impacts them both. Therefore, the following may be important key points: (i) preserving the outer surface of the nanomotors is key to maintaining their activity and motion for future biomedical applications and (ii) understanding nanomotor–protein corona interactions is very important to rationally design nanomotors for given applications such as cell targeting. In this regard, proteomic analysis revealed not only changes in the amount of proteins but also on the type of proteins that attached to the nanomotor surface. This may have significant impact on future biomedical applications such as targeted delivery of drugs, since having different proteins attached to the surface of nanomotors may influence their biodistribution and cellular uptake. Noteworthily, the changes in the protein corona upon nanomotor activity are not only qualitative but also quantitative, where the impact on different biological interactions such as cell–nanoparticle interactions will need to be considered in the near future.
Experimental section
Materials
Ethanol (EtOH, 99%), hydrochloric acid (37% in water), tetraethylorthosilicate (TEOS, 99%), triethanolamine (TEOA, 99%), cetyltrimethylammonium bromide (CTAB, 99%), methanol (MeOH, 99%), ammonium hydroxide (25% in water), 3-aminopropyltriethoxysilane (APTES, 99%), glutaraldehyde (GA, 25% in water), urease from Canavalia ensiformis (type IX, powder, 50
000–100
000 units per g solid), urease activity assay kit, fluorescein 5(6)-isothiocyanate (FITC) and urea (99.9%) were purchased from Sigma-Aldrich. Cyanine5-NHS ester was purchased from Lumiprobe and Fetal Bovine Serum (FBS) was purchased from Invitrogen.
Instruments
Transmission Electron Microscopy (TEM) images were captured under a JEOL JEM-2100 microscope. Scanning Electron Microscopy (SEM) was performed using a FEI NOVA NanoSEM 230 at 10 kV. The hydrodynamic radii and electrophoretic mobility of the particles were analysed using a Wyatt Möbius instrument coupled with an Atlas cell pressurization system. Optical videos were recorded using an inverted optical microscope (Leica DMi8) equipped with a 63× water-immersion objective. Protein quantification and enzymatic activity were measured using an Infinite M200 PRO multimode microplate reader. The STORM analysis was performed using a Nikon N-Storm system with a total internal reflection fluorescence (TIRF) configuration. The differential quantitative proteomic experiment was performed on a Dionex Ultimate 300 nanoliquid cromatograph coupled to an Orbitrap Fusion Lumos mass spectrometer (Thermo Scientific).
Fabrication of mesoporous silica nanoparticles
Type MCM-41 silica mesoporous nanoparticles were fabricated following the Stöber method.11 Briefly, a solution composed of 570 mg of CTAB, 20 mL of MilliQ water and 35 g of TEOA was prepared. The mixture was homogenized for 30 min at 95 °C, in a silicon oil bath, to allow the formation of a micellar template. Subsequently, 1.5 mL of TEOS were added dropwise, acting as the precursor for silica growth. The resulting mixture was stirred for 2 h at 95 °C. The formed particles were collected by centrifugation (10 minutes at 1350g), followed by three washes in ethanol, using the same centrifugation conditions. The CTAB was removed from the particles’ pores by suspending them in a MeOH
:
HCl mixture (10
:
0.6, 30 mL) and refluxing the solution at 80 °C for 24 h. Finally, the particles were washed thrice with ethanol (1350g, 10 minutes), sonicating 5 minutes between each centrifugation step to avoid aggregation of the particles. Fluorescent particles were obtained by mixing TEOS and FITC conjugated to (3-aminopropyl) triethoxysilane (FITC-APTES) as precursors (6
:
1 v). In order to prepare the FITC-APTES conjugate, 250 μL of APTES were mixed for 30 minutes with a solution of 2 mg of FITC in 5 mL of ethanol.12
The obtained mesoporous silica nanoparticles (MSNPs) were characterized using scanning and transmission electron microscopy (SEM and TEM), as well as dynamic light scattering (DLS) for hydrodynamic radius and zeta-potential measurements.
Amine functionalization of MSNPs
MSNPs were suspended in EtOH at a concentration of 2 mg ml−1. Then, APTES was added (10 μL mg−1 of particles). The suspension was placed on an end-to-end rotary mixer and kept for 24 h at room temperature. The resulting amine-modified particles (MSNP-NH2) were washed thrice with ethanol and thrice with water (1350g, 10 minutes). Some aliquots of 0.5 mL were centrifuged and air-dried to determine the amount of particles.
Urease functionalization
MSNP-NH2 of 0.25 mg mL−1 MSNP-amine particles were centrifuged and suspended in 900 L of PBS. Then, 100 μL of glutaraldehyde were added to the solution, which was kept for 3 h in the rotary mixer at room temperature. Next, the GA functionalized particles were washed thrice in PBS by centrifugation (1350g, 10 minutes) and suspended in a solution of 3 mg mL−1 urease in ×1 PBS for three hours at room temperature, under end-to-end mixing. After this, the particles were washed thrice (1350g, 10 minutes) with ×1 PBS. The redispersion of the pellet was ensured by performing thorough vortexing and sonication between the centrifugation steps.
Urease activity assay
Urease activity was studied by measuring ammonia generation using a commercial kit based on the Berthelot method,63 according to the manufacturer's instructions. For this, nanomotors in a 0.5 mg mL−1 solution were incubated with 100 mM urea. A control experiment was performed incubating nanomotors without urea.
Motion analysis
A sample of 5 μL of urease-MSNPs at 0.025 mg mL−1 concentration was mixed with 5 μL of either PBS or 100 mM urea in PBS and placed in a custom-made flow chamber in order to minimize drift.
Videos of nanomotors were recorded for 30 s at 50 fps using an inverted optical microscope, in bright field. At least 20 nanomotors were analyzed for each condition. Particle trajectories were extracted from the recorded videos using a custom-made Python script.
Urease and FBS labeling with Cy5
Urease was dispersed in 0.1 M sodium bicarbonate buffer (pH = 8.5) at 10 mg mL−1 followed by the addition of 1.5 equiv. of cyanine5 NHS ester (Lumiprobe). The reaction mixture was shaken at 300 rpm for 4 h at room temperature. Labelled proteins were then dialyzed with a 14 kDa pore size against sodium bicarbonate 3 times for 2 h while mixing at 200 rpm. The concentration of dye per protein was then quantified using a Nanodrop ND-1000 spectrophotometer.
Protein corona formation
Urease nanomotors (0.25 mg mL−1) were incubated for 30 min at 37 °C in a solution containing non-labelled Fetal Bovine Serum (FBS), 5% Cy5 labelled FBS and 0 or 100 mM urea for the control and active samples, respectively. After incubation, unbound FBS was removed by applying 7 washing steps based on centrifugation (1350g, 10 minutes).
STORM sample preparation and observation
Nanomotors were incubated in custom-made flow chambers for 15 min at room temperature in order to favour sedimentation. Then, GLOX or OxEA imaging buffers were carefully pipetted through the flow chamber so as to establish the appropriate chemical environment for the dyes to blink. The GLOX buffer was composed of PBS at pH 7.4, an oxygen-scavenging system (0.5 mg mL−1 glucose oxidase and 35 μg mL−1 catalase), glucose (5% w/v), and cysteamine (100 mM). The OxEA buffer was composed of PBS at pH 8.4, oxyrase (3% v/v) and cysteamine (100 mM). The samples were imaged under a Nikon N-Storm system with a total internal reflection fluorescence (TIRF) configuration. STORM acquisition was set to 20
000 frames at 100 fps. Cyanine5 (Cy5) and Alexa Fluor 488 (AF488) were correspondingly excited using 647 nm (160 mW) and 488 nm (80 mW) lasers. No UV light activation was employed.
STORM data post-processing
The obtained data from STORM acquisitions were filtered and analysed using custom-made Python and Matlab scripts. On the one hand, cluster centroids of the acquired localizations were automatically detected and superimposed on the FITC or brightfield low resolution images. This way, localization clusters coming from free protein aggregates could be discarded. On the other hand, the cluster shape and size were evaluated, and the number of localizations comprising them was retrieved.
Proteomic analysis
Nanomotors exposed to FBS as described above, either in the presence or absence of urea, were collected by centrifugation and washed thrice in 1 mL of 50 mM ammonium bicarbonate buffer (pH 8). Finally, 100 μL of ammonium bicarbonate was added to the pellet, and this suspension was subjected to trypsin digestion. For this, protein digestion was performed directly on the nanomotors by incubating them with 2 μg of trypsin dissolved in 300 μL of 50 mM ammonium bicarbonate buffer at 37 °C overnight (200 μL were added to the delivered volume). Then, an additional 1 μg of trypsin was added and incubated for 2 h at 37 °C. The nanomotors were pelleted by centrifugation at 2000g for 5 minutes and the supernatant was transferred to a fresh Eppendorf tube. The particles were washed once with 100 μL of 50 mM ammonium bicarbonate buffer and these washed particles were pooled with the first supernatant. Then, formic acid was added to the eluates to a final concentration of 1%. The samples were cleaned using C18 tips (PolyLC C18 tips) and the digested peptides were eluted with 80% acetonitrile and 1% TFA. Then, the samples were diluted with 20% acetonitrile and 0.25% TFA, loaded into strong cation exchange columns (SCX) and peptides were eluted in 5% NH4OH and 30% methanol. Finally the samples were evaporated to dry, reconstituted in 50 μL and diluted 1
:
8 with 3% acetonitrile and 1% formic acid aqueous solution for nanoliquid chromatography mass-spectrometry (nanoLC-MS/MS) analysis. First, the samples were loaded to a 300 μm × 5 mm PepMap100, 5 μm, 100 Å, C18 μ-precolumn (Thermo Scientific) at a flow rate of 15 μl min−1 using a Thermo Scientific Dionex Ultimate 3000 chromatographic system (Thermo Scientific). To separate the peptides, an Acclaim PEPMAP 100 C18 analytical column measuring 75 μm × 50 cm NanoViper (C18 3 μm, 100 Å, Thermo Scientific) with a 90 min run was used, comprising three consecutive steps with linear gradients from 3 to 35% B in 60 min, from 35 to 50% B in 5 min, and from 50% to 85% B in 2 min, followed by isocratic elution at 85% B in 5 min and stabilization to initial conditions (A = 0.1% FA in water and B = 0.1% FA in CH3CN). The column outlet was directly connected to an Advion TriVersa NanoMate (Advion) fitted on an Orbitrap Fusion Lumos™ Tribrid (Thermo Scientific).
A twin database search with two separate software, Thermo Proteome Discoverer v2.3.0.480 (PD) and MaxQuant v1.6.2.6a (MQ) was performed. The search engine nodes used were Sequest HT for PD and Andromeda for MQ. The databases used in the search was SwissProt Human (release 2019 01) including contaminants and the user proteins. A differential expression analysis between the 100 mM urea and no urea conditions was performed by applying a principal component analysis, and using standard cutoffs for fold changes (|FC| > 1.5) and adjusted p-values (padj < 0.05).
Author contributions
The manuscript was written through contributions of all authors.
Conflicts of interest
The authors declare no conflicts of interest.
Acknowledgements
This work was supported by the European Union's Horizon 2020 Research and Innovation Program, under the MarieSkłodowska-Curie Individual Fellowship (843998 to TP), and the Irène Curie Fellowship (TP). S. S. thanks the European Research Council (ERC) under the European Union's Horizon 2020 Research and Innovation Programme (grant agreement no 866348, i-NanoSwarms), PID2021-128417OB-I00 and PDC2022-133753-I00 funded by MCIN/AEI/10.13039/501100011033 and by “ERDF A way of making Europe” and European Union Next Generation EU (Bots4BB and BOJOS projects). S. S. thanks the “La Caixa” Foundation under the grant agreement LCF/PR/HR21/52410022 for project “BLADDEBOTS”. S. P. and L.A acknowledge the financial support by the Spanish Ministry of Science and Innovation (PID2019-109450RB-I00/AEI /10.13039/501100011033), European Research Council/Horizon 2020 (ERC-StG-757397), and the “la Caixa” Foundation (ID 100010434). Mass spectrometry/Proteomics was performed at the IRB Barcelona Mass Spectrometry and Proteomics Core Facility, which is a member of ProteoRed, PRB3-ISCIII, supported by grant PRB3 (IPT17/0019 – ISCIII-SGEFI/ERDF) and is granted in the framework of the 2014–2020 ERDF Operational Programme in Catalonia, co-financed by the European Regional Development Fund (ERDF, Reference: IU16-015983).
The IBEC team thanks the CERCA program by the Generalitat de Catalunya, the Secretaria d'Universitats i Recerca del Departament d'Empresa i Coneixement de la Generalitat de Catalunya through the project 2021 SGR 01606, and the “Centro de Excelencia Severo Ochoa”, funded by Agencia Estatal de Investigación (CEX2018-000789-S).
References
- S. Sánchez, L. Soler and J. Katuri, Angew. Chem., Int. Ed., 2015, 54, 1414–1444 CrossRef.
- P. S. Schattling, M. A. Ramos-Docampo, V. Salgueiriño and B. Städler, ACS Nano, 2017, 11, 3973–3983 CrossRef CAS.
- X. Ma, A. Jannasch, U.-R. Albrecht, K. Hahn, A. Miguel-López, E. Schäffer and S. Sánchez, Nano Lett., 2015, 15, 7043–7050 CrossRef PubMed.
- Y. Ji, X. Lin, Z. Wu, Y. Wu, W. Gao and Q. He, Angew. Chem., 2019, 131, 12328–12333 CrossRef.
- L. K. E. A. Abdelmohsen, M. Nijemeisland, G. M. Pawar, G.-J. A. Janssen, R. J. M. Nolte, J. C. M. van Hest, D. A. Wilson, E. S. Nanomotor, L. K. E. A. Abdelmohsen, M. Nijemeisland, G. M. Pawar, G.-J. A. Janssen, R. J. M. Nolte, J. C. M. Van Hest and D. A. Wilson, ACS Nano, 2016, 10, 2652–2660 CrossRef CAS PubMed.
- X. Ma, A. C. Hortelao, T. Patiño and S. Sanchez, ACS Nano, 2016, 10, 9111–9122 CrossRef CAS PubMed.
- T. Patiño, X. Arqué, R. Mestre, L. Palacios and S. Sánchez, Acc. Chem. Res., 2018, 51, 2662–2671 CrossRef.
- S. Tang, F. Zhang, H. Gong, F. Wei, J. Zhuang, E. Karshalev, B. Esteban-Fernández de Ávila, C. Huang, Z. Zhou, Z. Li, L. Yin, H. Dong, R. H. Fang, X. Zhang, L. Zhang and J. Wang, Sci. Rob., 2020, 5, eaba6137 CrossRef.
- Z. Ye, Y. Wang, S. Liu, D. Xu, W. Wang and X. Ma, J. Am. Chem. Soc., 2021, 143, 15063–15072 CrossRef CAS.
- D. Xu, J. Hu, X. Pan, S. Sánchez, X. Yan and X. Ma, ACS Nano, 2021, 15, 11543–11554 CrossRef CAS.
- C. Gao, Y. Wang, Z. Ye, Z. Lin, X. Ma and Q. He, Adv. Mater., 2021, 33, 2000512 CrossRef CAS.
- Z. Wu, Y. Chen, D. Mukasa, O. S. Pak and W. Gao, Chem. Soc. Rev., 2020, 49, 8088–8112 RSC.
- H. Yuan, X. Liu, L. Wang and X. Ma, Bioact. Mater., 2021, 6, 1727–1749 CAS.
- A. C. Hortelão, T. Patiño, A. Perez-Jiménez, À. Blanco and S. Sánchez, Adv. Funct. Mater., 2017, 28, 1705086 CrossRef.
- A. C. Hortelão, R. Carrascosa, N. Murillo-Cremaes, T. Patiño and S. Sánchez, ACS Nano, 2019, 13, 429–439 CrossRef.
- T. Patino, A. Porchetta, A. Jannasch, A. Lladó, T. Stumpp, E. Schäffer, F. Ricci and S. Sánchez, Nano Lett., 2019, 19, 3440–3447 CrossRef CAS.
- A. C. Hortelao, C. Simó, M. Guix, S. Guallar-Garrido, E. Julián, D. Vilela, L. Rejc, P. Ramos-Cabrer, U. Cossío, V. Gómez-Vallejo, T. Patiño, J. Llop and S. Sánchez, Sci. Rob., 2021, 6, eabd2823 CrossRef.
- H. Choi, S. H. Cho and S. K. Hahn, ACS Nano, 2020, 14, 6683–6692 CrossRef CAS.
- S. Pané, J. Puigmartí-Luis, C. Bergeles, X. Z. Chen, E. Pellicer, J. Sort, V. Počepcová, A. Ferreira and B. J. Nelson, Adv. Mater. Technol., 2019, 4, 1–16 Search PubMed.
- X. Arqué, A. Romero-Rivera, F. Feixas, T. Patiño, S. Osuna and S. Sánchez, Nat. Commun., 2019, 10, 2826 CrossRef PubMed.
- H. Choi, S. H. Jeong, T. Y. Kim, J. Yi and S. K. Hahn, Bioact. Mater., 2022, 9, 54–62 CAS.
- W. Wang and C. Zhou, Adv. Healthcare Mater., 2020, 2001236 Search PubMed.
- M. P. Monopoli, C. Åberg, A. Salvati and K. A. Dawson, Nat. Nanotechnol., 2012, 7, 779–786 CrossRef CAS.
- K. A. Dawson and Y. Yan, Nat. Nanotechnol., 2021, 16, 229–242 CrossRef CAS PubMed.
- H. Mohammad-Beigi, Y. Hayashi, C. M. Zeuthen, H. Eskandari, C. Scavenius, K. Juul-Madsen, T. Vorup-Jensen, J. J. Enghild and D. S. Sutherland, Nat. Commun., 2020, 11, 4535 CrossRef CAS PubMed.
- A. Salvati, A. S. Pitek, M. P. Monopoli, K. Prapainop, F. B. Bombelli, D. R. Hristov, P. M. Kelly, C. Åberg, E. Mahon and K. A. Dawson, Nat. Nanotechnol., 2013, 8, 137–143 CrossRef CAS.
- F. Wang, L. Yu, M. P. Monopoli, P. Sandin, E. Mahon, A. Salvati and K. a. Dawson, Nanomedicine, 2013, 9, 1159–1168 CrossRef CAS.
- Y. Yan, K. T. Gause, M. M. J. Kamphuis, C.-S. Ang, N. M. O'Brien-Simpson, J. C. Lenzo, E. C. Reynolds, E. C. Nice and F. Caruso, ACS Nano, 2013, 7, 10960–10970 CrossRef CAS PubMed.
- S. Ritz, S. Schöttler, N. Kotman, G. Baier, A. Musyanovych, J. Kuharev, K. Landfester, H. Schild, O. Jahn, S. Tenzer and V. Mailänder, Biomacromolecules, 2015, 16, 1311–1321 CrossRef CAS PubMed.
- A. Lesniak, F. Fenaroli, M. P. Monopoli, C. Åberg, K. A. Dawson and A. Salvati, ACS Nano, 2012, 6, 5845–5857 CrossRef CAS PubMed.
- F. S. M. Tekie, M. Hajiramezanali, P. Geramifar, M. Raoufi, R. Dinarvand, M. Soleimani and F. Atyabi, Sci. Rep., 2020, 10, 9664 CrossRef.
- C. D. Walkey, J. B. Olsen, F. Song, R. Liu, H. Guo, D. W. H. Olsen, Y. Cohen, A. Emili and W. C. W. Chan, ACS Nano, 2014, 8, 2439–2455 CrossRef CAS.
- S. Tenzer, D. Docter, J. Kuharev, A. Musyanovych, V. Fetz, R. Hecht, F. Schlenk, D. Fischer, K. Kiouptsi, C. Reinhardt, K. Landfester, H. Schild, M. Maskos, S. K. Knauer and R. H. Stauber, Nat. Nanotechnol, 2013, 8, 772–781 CrossRef CAS.
- R. García-Álvarez, M. Hadjidemetriou, A. Sánchez-Iglesias, L. M. Liz-Marzán and K. Kostarelos, Nanoscale, 2018, 10, 1256–1264 RSC.
- S. Palchetti, D. Pozzi, A. L. Capriotti, G. La Barbera, R. Z. Chiozzi, L. Digiacomo, G. Peruzzi, G. Caracciolo and A. Laganà, Colloids Surf., B, 2017, 153, 263–271 CrossRef CAS.
- D. T. Jayaram, S. M. Pustulka, R. G. Mannino, W. A. Lam and C. K. Payne, Biophys. J., 2018, 115, 209–216 CrossRef CAS PubMed.
- C. Carrillo-Carrion, M. Carril and W. J. Parak, Curr. Opin. Biotechnol, 2017, 46, 106–113 CrossRef CAS PubMed.
- A. L. Capriotti, G. Caracciolo, C. Cavaliere, V. Colapicchioni, S. Piovesana, D. Pozzi and A. Laganà, Chromatographia, 2014, 77, 755–769 CrossRef CAS.
- J. E. Blume, W. C. Manning, G. Troiano, D. Hornburg, M. Figa, L. Hesterberg, T. L. Platt, X. Zhao, R. A. Cuaresma, P. A. Everley, M. Ko, H. Liou, M. Mahoney, S. Ferdosi, E. M. Elgierari, C. Stolarczyk, B. Tangeysh, H. Xia, R. Benz, A. Siddiqui, S. A. Carr, P. Ma, R. Langer, V. Farias and O. C. Farokhzad, Nat. Commun., 2020, 11, 3662 CrossRef CAS.
- L. Papafilippou, A. Claxton, P. Dark, K. Kostarelos and M. Hadjidemetriou, Nanoscale, 2020, 12, 10240–10253 RSC.
- M. P. Monopoli, D. Walczyk, A. Campbell, G. Elia, I. Lynch, F. Baldelli Bombelli and K. A. Dawson, J. Am. Chem. Soc., 2011, 133, 2525–2534 CrossRef CAS.
- S. Pujals, N. Feiner-Gracia, P. Delcanale, I. Voets and L. Albertazzi, Nat. Rev. Chem., 2019, 3, 68–84 CrossRef.
- N. Feiner-Gracia, M. Beck, S. Pujals, S. Tosi, T. Mandal, C. Buske, M. Linden and L. Albertazzi, Small, 2017, 13, 1701631 CrossRef.
- A. M. Clemments, P. Botella and C. C. Landry, J. Am. Chem. Soc., 2017, 139, 3978–3981 CrossRef CAS PubMed.
- T. Patiño, N. Feiner-Gracia, X. Arqué, A. Miguel-López, A. Jannasch, T. Stumpp, E. Schäffer, L. Albertazzi and S. Sánchez, J. Am. Chem. Soc., 2018, 140, 7896–7903 CrossRef.
- M. De Corato, X. Arqué, T. Patiño, M. Arroyo, S. Sánchez and I. Pagonabarraga, Phys. Rev. Lett., 2020, 124, 108001 CrossRef CAS.
- Z. Wu, H. Xiang, T. Kim, M. S. Chun and K. Lee, J. Colloid Interface Sci., 2006, 304, 119–124 CrossRef CAS.
- J. B. Sumner and D. B. Hand, J. Am. Chem. Soc., 1929, 51, 1255–1260 CrossRef CAS.
- R. A. J. Post, D. van der Zwaag, G. Bet, S. P. W. Wijnands, L. Albertazzi, E.
W. Meijer and R. W. van der Hofstad, Nat. Commun., 2019, 10, 1663 CrossRef CAS.
- A. C. Hortelao, R. Carrascosa, N. Murillo-Cremaes, T. Patino, S. Sánchez, A. C. Hortelão, R. Carrascosa, N. Murillo-Cremaes, T. Patiño and S. Sánchez, ACS Nano, 2019, 13, 429–439 CrossRef CAS PubMed.
- K. K. Dey, X. Zhao, B. M. Tansi, W. J. Méndez-Ortiz, U. M. Córdova-Figueroa, R. Golestanian and A. Sen, Nano Lett., 2015, 15, 8311–8315 CrossRef CAS.
- X. Arqué, A. Romero-Rivera, F. Feixas, T. Patiño, S. Osuna and S. Sánchez, Nat. Commun., 2019, 10, 2826 CrossRef.
- G. Dunderdale, S. Ebbens, P. Fairclough and J. Howse, Langmuir, 2012, 28, 10997–11006 CrossRef CAS.
- J. R. Howse, R. A. L. Jones, A. J. Ryan, T. Gough, R. Vafabakhsh and R. Golestanian, Phys. Rev. Lett., 2007, 99, 8–11 CrossRef.
- X. Arqué, X. Andrés, R. Mestre, B. Ciraulo, J. Ortega Arroyo, R. Quidant, T. Patiño and S. Sánchez, Research, 2020, 2020, 1–14 CrossRef PubMed.
- A. Llopis-Lorente, A. García-Fernández, N. Murillo-Cremaes, A. C. Hortelão, T. Patiño, R. Villalonga, F. Sancenón, R. Martínez-Máñez and S. Sánchez, ACS Nano, 2019, 13, 12171–12183 CrossRef CAS.
- D. Walker, B. T. Kasdorf, H. H. H.-H. Jeong, O. Lieleg, P. Fischer, B. T. Käsdorf, H. H. H.-H. Jeong, O. Lieleg and P. Fischer, Sci. Adv., 2015, 1, e1500501–e1500501 CrossRef.
- W. Wang, Z. Huang, Y. Li, W. Wang, J. Shi, F. Fu, Y. Huang, X. Pan and C. Wu, Acta Pharm. Sin. B, 2021, 11, 1030–1046 CrossRef CAS.
- M. Raoufi, M. J. Hajipour, S. M. Kamali Shahri, I. Schoen, U. Linn and M. Mahmoudi, Nanoscale, 2018, 10, 1228–1233 RSC.
- V. Gorshkov, J. A. Bubis, E. M. Solovyeva, M. V. Gorshkov and F. Kjeldsen, Environ. Sci.: Nano, 2019, 6, 1089–1098 RSC.
- M. Lundqvist, J. Stigler, G. Elia, I. Lynch, T. Cedervall and K. A. Dawson, Proc. Natl. Acad. Sci. U. S. A., 2008, 105, 14265–14270 CrossRef CAS.
- D. Bonvin, U. Aschauer, D. T. L. Alexander, D. Chiappe, M. Moniatte, H. Hofmann and M. Mionić Ebersold, Small, 2017, 13, 1700409 CrossRef.
- C. J. Patton and S. R. Crouch, Anal. Chem., 1977, 49, 464–469 CrossRef CAS.
Footnotes |
† Electronic supplementary information (ESI) available: Additional Experimental section and proteomic results (PDF). See DOI: https://doi.org/10.1039/d3nr03749e |
‡ These authors contributed equally to this work. |
|
This journal is © The Royal Society of Chemistry 2024 |
Click here to see how this site uses Cookies. View our privacy policy here.