Spiers Memorial Lecture: NMR crystallography
Received
16th August 2024
, Accepted 3rd September 2024
First published on 6th September 2024
Abstract
Chemical function is directly related to the spatial arrangement of atoms. Consequently, the determination of atomic-level three-dimensional structures has transformed molecular and materials science over the past 60 years. In this context, solid-state NMR has emerged to become the method of choice for atomic-level characterization of complex materials in powder form. In the following we present an overview of current methods for chemical shift driven NMR crystallography, illustrated with applications to complex materials.
Introduction
Chemical function is directly related to the spatial arrangement of atoms. Consequently, the determination of atomic-level three-dimensional structures, through the introduction of a range of physical methods, and most notably from single crystals by diffraction methods, has transformed molecular and materials science over the past 60 years, leading to today’s structure-based understanding of chemistry.
Despite this revolution, if the system under investigation is not periodic, or is located on a surface or at an interface, as in many functional materials, or quite simply if it presents in powder form, atomic-level three-dimensional structure determination remains a challenge. Archetypal examples are devices for solar energy conversion, drug formulations, nanoparticles, or cements. As one example, the atomic-level structures at the surfaces and interfaces in hybrid perovskite materials are thought to define the mechanisms that lead to both stabilisation and efficiency of the photo-active phases.1–4
In this context, solid-state NMR spectroscopy (in conjunction with diffraction methods and other spectroscopies) has emerged to become the method of choice for atomic-level characterization of complex materials.5 Many groups have developed NMR in this direction with notable recent successes in for example MOFs,6 cements,7 organic semiconductors,8 biomass,9 battery science,10 catalysis,11 and hybrid perovskites.12 These studies illustrate the very broad impact that NMR can have in materials chemistry when it can be deployed.
In the following, we will give a brief summary of how NMR crystallography is deployed today for powders, and describe some of the most recent developments and applications to complex materials.
Chemical shift driven NMR powder crystallography
In contrast to methods such as powder XRD13–15 or electron diffraction,16–21 NMR directly probes the local atomic environment, allowing for structural characterization without the need for long-range order. In this direction, solid-state NMR has seen spectacular progress in the last few years,5,22–25 and methods have been introduced to solve crystal structures of bulk inorganic23,26–34 or molecular solids.22,23,35–60 If isotopic enrichment is possible, NMR methods can be used that lead to complete structures, which is now well established for proteins,61–68 and with a more limited set of examples for materials.27,69–73
In most cases for organic solids and materials, isotopic labeling can be extremely costly, or the very use of a different synthetic procedure will denature the question. For molecular solids at natural isotopic abundance, methods using proton spin diffusion35,36,38 and dipolar recoupling techniques42,44–48,74,75 have nevertheless been introduced, and with the demonstration that the sensitivity gains from DNP enhanced methods could yield efficient natural abundance correlation spectra,76–78 it has been shown that quantitative carbon–carbon dipolar recoupling experiments are possible at natural abundance.79–82
However, in contrast to dipolar couplings, the chemical shift is usually by far the easiest NMR parameter to measure. Together with the development of accurate density functional theory (DFT) based methods to calculate chemical shifts,83–90 this has enabled the development of methods using chemical shifts to determine structure (often referred to as NMR crystallography).39,40,50,89,91–96 Measured chemical shifts can be compared with computational predictions, and with the current best functionals the average deviation between experiment and calculation can be as low as 1.5 ppm for carbon-13.22,89,97–101 This has now been used by many groups to validate or refine crystal structures, with the following being illustrative references.22,23,36,38,42,44–48,50,51,74,102–134 The reader is referred to, for example, the article by Brown and coworkers129 in this volume for a description of some of these applications. NMR crystallography has been recognized by the International Union of Crystallographers, and chemical shifts are increasingly combined with X-ray or electron diffraction measurements.60,94,135–150 Since the first de novo chemical shift based structure of a molecular solid solved in 2013,41 the technique has been developed and applied to a range of structures,5,22,23 from pharmaceuticals94,113,150–156 to a metal-binding site in a metalloprotein,157 capping groups on nanoparticle surfaces,95 the atomic-level structures of calcium silicate hydrates7,158,159 or to the spacer layers in two-dimensional hybrid perovskite materials.160
Remarkable examples include the determination of the structures of drug molecules in pharmaceutical formulations,127,161,162 the detailed determination of the structure of active sites in enzyme reaction pathways,59,96,163 or the precise determination of the disordered structure of amorphous drugs.54,164,165
Chemical shift driven NMR crystallography usually follows the general scheme involving the four steps shown in Fig. 1. Obviously, the core of the method relies on the experimental measurement and assignment of NMR chemical shifts. As discussed below, this step can range from straightforward, taking minutes to hours, to highly challenging, taking weeks or months. In parallel to the assignment step, candidate structures are generated, either using computational methods or using prior experimentally determined structures (typically from single crystal X-ray diffraction). Then, chemical shifts are predicted for the candidate structures, typically using either DFT calculations or machine learning models. Finally, the predictions are compared with experiment, and a structure is determined, together with attributes such as positional errors and confidence limits.
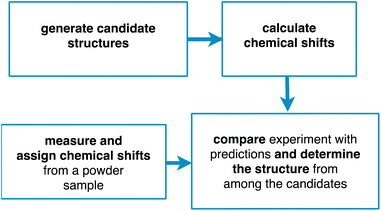 |
| Fig. 1 A scheme of the principal steps involved in chemical shift driven NMR crystallography. | |
In the following we will describe each of these steps in more detail. We will focus on the determination of organic molecular solids using isotropic chemical shifts, but the method can be adapted to include chemical shift anisotropy or to hybrid inorganic materials or oxides,5,22,23,34 and we will illustrate the methods described with examples primarily from our group. We will also focus on structure determination, rather than structure validation. While the distinction between the two is not always clear, here we will assume that structure determination does not use any prior structural information, while structure validation most commonly refers to the verification (or not) of a hypothesis derived from a pre-existing experimentally determined crystal structure.
Candidate structure generation
De novo structure determination currently requires first the generation of a large ensemble of credible candidate structures.39–41 For crystalline materials this is usually achieved with some form of computational crystal structure prediction (CSP) protocol.33,166–172 Methods to predict crystal structures have been developed primarily with the objective of understanding the energy landscape of crystal structures, and were first primarily used as a tool to help ensure that all the important crystal forms of a given drug molecule had been found experimentally. This area has come of age over the last 20 years, and rapid progress continues to be made, as can be seen from the ongoing series of blind tests.173 The capacity for CSP has increased to include the larger molecules typically found in molecular drugs, having conformational flexibility and including hydrates and solvates of pharmaceutical sized molecules (and we note that CSP can equally be performed for inorganic/ionic or hybrid structures).
From the experimental structure determination perspective, CSP provides an ideal tool to generate an ensemble of possible candidate structures. Notably, while in prediction type applications, accurately ranking structures within a set according to predicted energy is often considered as critical, nevertheless from the experimental structure determination perspective it does not really matter what the predicted energies are, as long as the experimentally present structure is included in the set.
Our objective is not to provide a detailed review of CSP, which can be found elsewhere,171 but we note that further improvements in CSP methods, as they become faster, more accurate, and accessible for larger and larger molecules, will directly lead to consequent improvements in CSP based NMR crystallography.
Chemical shift predictions
Once candidate structures have been generated, the next step is to compute predicted chemical shifts for the ensemble of candidates.39–41
Plane-wave density functional theory (DFT) methods.
While the principles of computing chemical shifts were introduced in the 1950s,124,174–176 the challenge here is that high accuracy is required in order to capture the effects of particular conformations and packing arrangements of the molecular building blocks on the chemical shifts, and to allow the identification of the correct structure among a set of potential candidates based on a comparison between computed and measured chemical shifts.
In this respect, a revolution in solid-state NMR has occurred with the introduction of accurate methods to calculate chemical shifts,83–85 in particular using DFT methods developed for periodic systems based on the gauge-including projector augmented wave (GIPAW) approach.87,88,177 Using a Perdew–Burke–Ernzerhof (PBE) functional178 with a dispersion correction179 GIPAW can achieve root-mean-square error (RMSE) between experiment and calculation of ∼2.5 ppm for 13C and ∼0.4 ppm for 1H.22,34,39,90,124,180 This accuracy has been key in enabling the very rapid development of chemical shift based NMR crystallography over the last 20 years.
While GIPAW accuracy is sufficient in many cases, increased accuracy of chemical shift predictions will directly lead to increased discriminating power for structure determination by NMR. As a result, there has been a large volume of research aimed at further improving the accuracy of chemical shift predictions with first principles methods. Most of this activity has been directed to using more accurate functionals, which rapidly becomes very computationally costly in the GIPAW formalism. To avoid this, hybrid or double hybrid functionals have been used in cluster or fragment-based approaches. With such calculations, the RMSE between experiment and calculation can be as low as 1.5 ppm for 13C and 0.2 ppm for 1H.89,97–101,181,182
In general, one should bear in mind that the accuracy ranges given above are averages, and that some structures can lead to significant outliers.183 Moreover, note that chemical shift calculations depend very strongly on the model structure being used as input. In this regard, if the input is an experimental X-ray diffraction structure, then at least the 1H positions should always be optimized prior to calculating chemical shifts, preferably using the same framework as used for the chemical shift calculations.124,184 In some cases it is prudent to compare consistency between the results from structures where only 1H positions have been optimized to results where the positions of all the heavy atoms have also been optimized. More generally, for candidate structures that have been generated computationally, thought must be given to the level of theory used for structural optimization as compared to the level of theory for the chemical shift computation.90,185
Finally, we note that the methods above all compute structures without accounting for finite temperature, meaning that they will not correctly reproduce temperature dependent shifts. To address this, methods have been introduced using averaging over vibrational modes or over snapshots taken from ab initio molecular dynamics simulations186 or using path integral molecular dynamics.99,187,188 While finite temperature effects are a significant practical issue, these approaches currently remain impractical in most cases due to the computational cost. One promising path forward is to use machine learned potentials and chemical shifts for this purpose.189
Machine learning models.
In summary, DFT based methods generally offer a good tradeoff between accuracy and computational cost for computing chemical shifts in small periodic structures. However, the computational cost of DFT methods severely limits the size of systems accessible, preventing the study of large or disordered systems.
To address this limitation of first principles calculations, in recent years machine-learning models have become popular to bypass intensive quantum-mechanical calculations in many areas of chemistry. Indeed, chemical shifts were first predicted in solutions from large experimental databases, and machine-learned models of experimental shifts have met with considerable success, in both small molecules and proteins,190–199 and are widely used today.
In contrast, for solids there are no equivalent experimental databases that would be large enough to train prediction models. As an alternative to training models on experimental data, machine-learning models can be built using databases constructed using DFT methods,200,201 and this has been applied for chemical shifts in isolated molecules.200,202–206 For chemical shifts in solids, an early example of this approach was demonstrated for the specific case of silicas,207 and more recently ML models have been developed for molecular solids58,208–210 and oxides.211,212
Such approaches have proven able to yield chemical shifts with accuracy similar to DFT at a fraction of the computational cost, allowing applications to large ensembles of large systems.
Chemical shifts in molecular solids present a particular challenge because of the diversity of organic chemistry, and the subtle dependence of shifts on conformations and the effects of crystal packing. In this context we have previously introduced ShiftML,58 a machine-learning model of chemical shifts trained on GIPAW DFT data for structures from the Cambridge structural database (CSD).213 The current version, ShiftML2,208 was trained on GIPAW DFT chemical shifts for an extended set of over 14
000 structures containing any of 12 common elements (H, C, N, O, S, F, P, Cl, Na, Ca, Mg and K), and composed of roughly equal amounts of relaxed and thermally perturbed structures of crystals extracted from the CSD. It allows fast predictions of chemical shifts for any molecular solid containing those atoms with accuracy that is comparable to DFT, and in particular for predictions on distorted structures, or for structures that are geometry optimized using other methods. For example, ShiftML2 yields an RMSE between predicted and experimentally measured 1H chemical shifts of 0.47 ppm for a benchmark set of 13 organic molecular solids, as compared to 0.35 ppm using DFT.208Fig. 2 shows a comparison of DFT-computed shieldings and predictions with the ShiftML2 model for 1H, 13C and 15N.
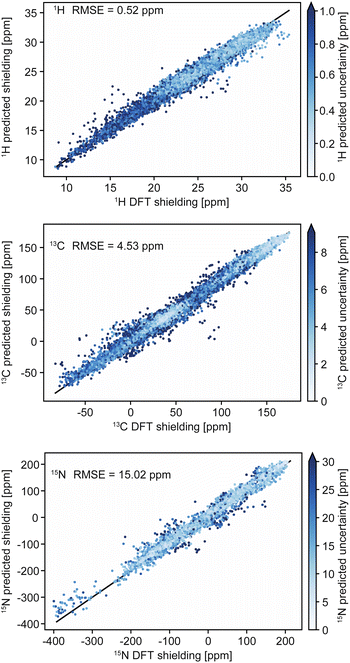 |
| Fig. 2 Comparison of DFT-computed shieldings with predictions from the ShiftML2 model for 1H, 13C and 15N. The color scale shows the predicted uncertainty of the ShiftML2 prediction. Adapted from ref. 208. | |
Structure determination
Once candidate structures have been generated together with associated predicted chemical shifts, structure determination is then in principle straightforward by comparison of the predicted shifts with the experimentally determined values.39,40
Comparison with experiment is usually done for isotropic 1H and 13C shifts, but shifts from any other nuclei that are available can be straightforwardly included, most commonly today being 15N or 29Si, as well as quadrupolar parameters measured from for example, 35Cl spectra.127 Chemical shift anisotropies (e.g.13C or 15N) can also be compared, when available, although these are usually more laborious to obtain experimentally.
As an example, a comparison of predicted and measured 1H shifts for two candidate structures of cocaine are shown in Fig. 3. The agreement between experiment and prediction is usually consolidated into a single value of the average deviation for all the measured chemical shifts, typically expressed as the root mean-squared deviation (RMSD) in ppm,39,40 or as a χ2 value.214 In Fig. 3 we can see that the predicted shifts of Candidate A contain some obvious outliers that are not in agreement with the data, leading to an overall 1H RMSD of 1.02 ppm. In contrast Candidate B is clearly in better agreement and yields an overall 1H RMSD of 0.28 ppm. However, in order to determine if the predicted shifts of either structure are in agreement with the data, there is need to define a benchmark. For molecular solids this was first done for GIPAW PBE calculations using 15 organic compounds where the average RMSD to experiment was found to be 0.33 ppm (±0.16 ppm) for 1H and 1.9 ppm (±0.4 ppm) for 13C (where the number in brackets corresponds to one standard deviation of the RMSD).39 Using this scale, we can see that Candidate A does not agree with the data, whereas Candidate B is agreement with the data to within error.
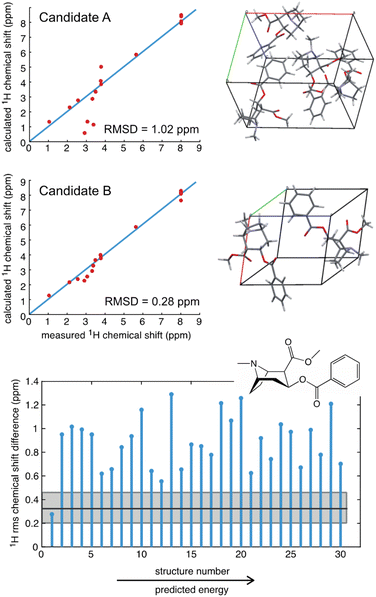 |
| Fig. 3 A comparison of the measured 1H chemical shifts with predicted values (from GIPAW PBE) for two different candidate structures of cocaine free base (upper), and (lower) a plot of the RMSD for 30 candidate structures.40 The solid black horizontal line shows the benchmark mean RMSD error between experimental and predicted shifts, and the horizontal grey shaded zone indicates one standard deviation of the RMSD, such that candidate structures that have an RMSD that falls within the grey zone are in good agreement with the data. | |
Fig. 3 also shows the RMSD between prediction and experiment plotted for a series of 30 candidate structures generated using a comprehensive CSP protocol, and where the candidates correspond to the set of predictions that have computed energies that are within 10 kJ mol−1 of the most stable structure.40 It can immediately be seen that of all 30 structures, only one structure is in good agreement with the data. The full crystal structure of cocaine determined in this way from the powder sample is shown in Fig. 4, where it is overlaid with the known structure of cocaine that had been previously determined by X-ray diffraction from a single crystal sample, where they are seen to be essentially identical.
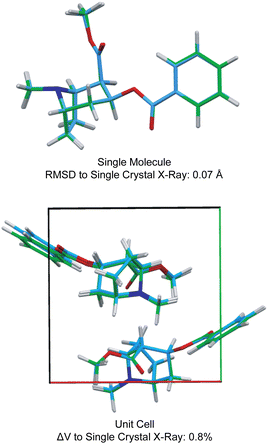 |
| Fig. 4 A comparison of the structure of cocaine free base determined by powder 1H NMR and the structure determined by single crystal X-ray diffraction. Adapted from ref. 40. | |
To provide a complete structural picture, two key elements of structure determination are the level of confidence associated with the determined structure, and the estimation of the errors on the positions. To address confidence, Bayesian approaches have been introduced that assign a probabilistic value that quantifies the likelihood that a given candidate corresponds to the experimental structure.55,214,215 Using the approach of Engel et al.,55 the structure of cocaine determined in Fig. 3 using 1H shifts has a confidence of 95 or 100%, depending on if predicted shifts from ShiftML1 or from GIPAW are used. The article by Mueller214 in this volume provides an excellent overview of the determination of confidence.
We do note that, in general, there is a threshold number of chemical shifts below which there is generally poor confidence in the structure.55 This can be explained in the sense that if the molecule only has, e.g., three different types of 1H/13C, then many candidate structures may accidently agree with the data. However, once the molecule has, e.g., 10 or more shifts, accidental agreement becomes much less likely. A good example of this is theophylline, where 11 out of 45 candidate structures were found to have 1H RMSDs <0.5 ppm.40 Interestingly, we see that chemical shift driven structure determination will typically work better in larger, more complex, molecules.
Positional uncertainties for the structures obtained by chemical shift driven NMR crystallography can be quantified by estimating the correlation between the chemical shift RMSD and the variances of atomic positions of individual atoms,57 thereby making NMR structures directly comparable to structures determined by other methods. This is conveniently achieved by calculating chemical shifts for an ensemble of slightly perturbed crystal structures obtained by MD simulations at finite temperatures, and characterizing the deviation in position that is required to exceed the uncertainty in the chemical shifts.57 The positional distributions obtained in this manner are then converted into anisotropic displacement parameters (ADPs), which can be represented by ellipsoids on the determined structure. For the structure of cocaine, this leads to an average positional RMSD <rav> of 0.169 Å, corresponding to an average equivalent displacement parameter of 0.0095 Å2.57
It is interesting to note that the positional uncertainties obtained for powder NMR structures are similar to those obtained for single crystal X-ray diffraction structures. Further we note that since the chemical shifts are not reliant on long range order, the positional uncertainty should not change significantly with molecular size. Indeed, Holmes et al. have reported average positional RMSD of 0.17 Å for the 1H atoms for structures of the co-factor and substrates for the α-aminoacrylate intermediate of tryptophan synthase in the enzyme active sites.216
Assembling all the elements described above, Fig. 5 shows the NMR structure of the form 4 of the drug (4-[4-(2-adamantylcarbamoyl)-5-tert-butyl-pyrazol-1-yl] benzoic acid) (AZD8329). This was the first example of NMR structure determination for a molecular compound of previously unknown structure. The structure is determined with 100% confidence, and with a positional RMSD of 0.17 Å (corresponding to an average equivalent displacement parameter of 0.0095 Å2).
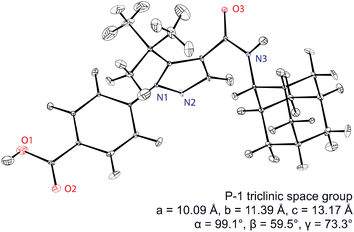 |
| Fig. 5 The de novo structure of AZD8329 determined by 1H chemical shift driven NMR powder crystallography.55,57,217 Adapted from ref. 57. | |
There are today a large and growing number of compounds with structures that have been determined by chemical shift driven powder crystallography using the protocols described above. Fig. 6 shows a series of examples from the work of our group. In addition, some other illustrative examples are the structures of a methanol solvate-hydrate of decitabine,219 catechin,151 furazidine polymorphs,220 aspirin,154 teriflunomide,153 mebendazole,155 linezolid cocrystals,152 three pyridine dicarboxylic acids,221 leucopterin,150 and the series of structures from actives sites in tryptophan synthase.59,96,163
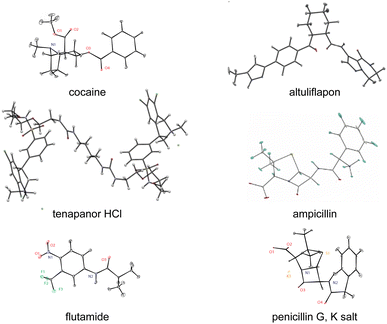 |
| Fig. 6 A set of illustrative structures determined from microcrystalline powder samples by chemical shift driven NMR crystallography.40,54,57,94,218 | |
Systems lacking long-range order
Because the chemical shift is only typically sensitive to the environment up to a range of 5–8 Å from a given nucleus, chemical shift driven NMR crystallography does not require longer range order in the sample to determine structure. This is particularly striking in the case of amorphous solids discussed below, but in particular it allows the straightforward study of hierarchical, composite or slightly disordered materials. One example of this would be the determination of the structure of an active pharmaceutical ingredient inside a tablet formulation.162
Another illustrative example is the determination of the structure of organic spacer layers contained in layered hybrid lead-halide perovskites,160,222 where the inorganic layers are slightly disordered, and where the thickness of the inorganic layers varies from layer to layer. In such systems, although the organic spacer layers as determined by NMR can be highly ordered, as shown in Fig. 7, with two different but well-defined structures forming nano-domains,160 these structures would be essentially invisible to diffraction methods due to the overall longer-range disorder in the hierarchy of the structure.
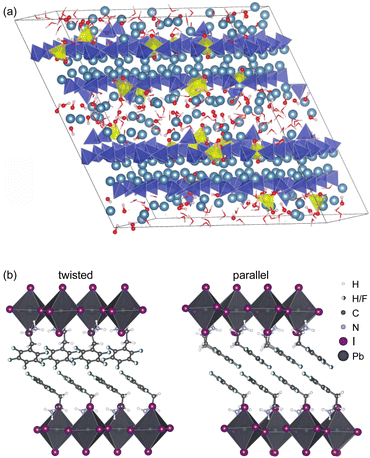 |
| Fig. 7 (a) Representative atomic-level structure of zinc-modified C-S-H as determined with a Zn : Si ratio of 0.15. Silicate tetrahedra are depicted in blue; zincate tetrahedra are depicted in yellow; and calcium ions are depicted in light blue,159 and (b) the structure of S2PbI4 layered perovskites with twisted (left) and parallel (right) relative orientations of the aromatic rings in adjacent layers.160 Adapted from ref. 159 and 160. | |
Notably, by providing this atomic-level window on the details of these complex hierarchical and locally disordered structures, the structural information obtained from NMR has guided new strategies for the design and production of new perovskite formulations that yield better performance in terms of both photovoltaic efficiency12 and environmental stability.223
Another illustration is the determination of the complete atomic-level structures of various calcium silicate hydrates (C-S-H),7,159,224 which are the main components of Portland cement. The structure of pure C-S-H determined by NMR is found to correspond to an ensemble of defective tobermorite unit cells including calcium sites in the interlayer that bridge chain-terminating silicate Q(1) sites.224 This site is associated with an environment of strong hydrogen bonding, which stabilizes the structure and, consequently, promotes high Ca
:
Si ratios in C-S-H. The NMR structure establishes a clear relation between the atomic-level defect structure and the high Ca
:
Si ratio in C-S-H. Similar structures have also been determined for aluminium7 and zinc containing C-S-H, with an illustrative Zn–C-S-H structure shown in Fig. 7.159 The knowledge of these structures is a prerequisite for overcoming the self-limiting growth of C-S-H and to better understand growth mechanisms and kinetics. Once again, despite the lack of long-range order due to the presence of defects, chemical shifts contain all the information needed to fully characterize the structure.
Amorphous solids
Another important category of materials lacking long-range order that can be accessed by NMR crystallography is the area of amorphous solids. Indeed there is a long history of the application of NMR spectroscopy to study amorphous inorganic glasses or polymers.5 Amorphous molecular solids are becoming increasingly important especially in view of the development of amorphous drug formulations in the pharmaceutical industry.225–228 However, while NMR has been used to study some aspects of amorphous compounds,22 complete atomic-level structures are required to rationalize the factors that lead to the stabilization of amorphous forms.
The structure determination process for amorphous molecular solids follows the same outline as for crystalline materials in Fig. 1, but with some important differences.54,164,165
For amorphous solids, structure generation is achieved using molecular dynamics simulations. As an example, for the drug molecule AZD4625 (with the chemical structure shown in Scheme 1), eight MD simulations were carried out with cells containing 128 molecules of AZD4625, randomly initialized in order to model an amorphous system. Chemical shift predictions were then performed using ShiftML2 for 8000 snapshots taken from the MD trajectories, corresponding to more than 1 million molecules. The predicted shifts were then compared with the experimental values obtained for 1H and 13C for all the molecular environments extracted from the MD snapshots, where each environment comprises a central molecule and all molecules with at least one atom within 7 Å from any atom of the central molecule. For each atomic site, the probability that the predicted shift is drawn from the corresponding experimental chemical shift distribution was calculated and combined into a global probability that the molecular environment matches the NMR experiments. Then the top 1% of local molecular environments in best agreement with experiment were taken as a set to describe the experimental structure, referred to as the NMR set. More details are given in ref. 165. A similar approach was used for the drug Atuliflapon.54,164 It is worth pointing out that care should be taken to make sure that the range of conformations generated as candidates by the molecular dynamics simulations is larger than those present in the sample, and to assess the presence of any actual molecular dynamics that might be present in the samples which could affect the observed lines’ shapes.229 This latter point is common to any NMR crystallography investigation, whether on crystalline or disordered samples.5
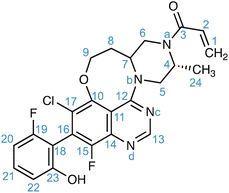 |
| Scheme 1 The chemical structure and numbering of AZD4625. | |
Once the NMR set has been obtained, it can be analysed in terms of the distributions of structural features present in the amorphous structure and correlated to predicted energies.54,164,165 For example, Fig. 8b shows the occurrence of hydrogen bonding patterns observed in the NMR set of AZD4625 as compared to the background MD set.165 Notably, over 25% of environments in the MD set have no hydrogen bond to the OH proton, whereas almost all the environments are H-bonded in the NMR structure, with a particularly strong promotion of H-bonding to O3. This can be directly correlated to the computed energies shown in Fig. 8c, where a clear stabilisation of the structure by the O3 H-bonding interaction is observed. Conversely, it is interesting to note that H-bonding to Nd is predicted to be strongly stabilising, but that this is not significantly promoted in the experimental structure. Similarly, the structure can be analysed in terms of preferred conformations. Fig. 8d shows the population of conformations of the aliphatic ring in AZD4625, which shows a clear preference for the chair conformation in the experimental structure. A similar analysis has been carried out for Atuliflapon.164
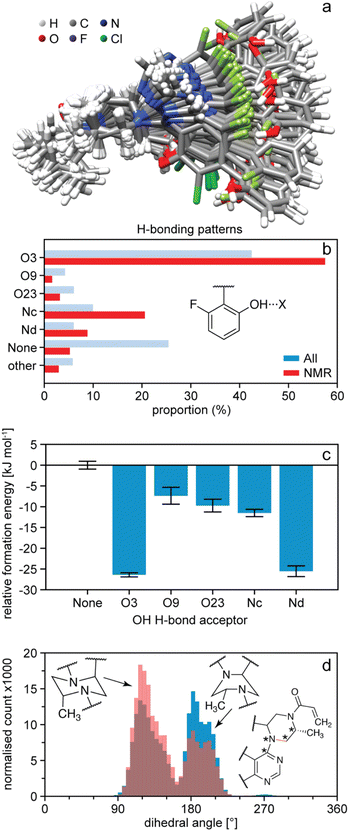 |
| Fig. 8 The structure of amorphous AZD4625.165 (a) Superposition of 100 conformations randomly sampled from the NMR set, and aligned on atoms Nb–C5–C4–Na. (b) Proportions of different hydrogen bond acceptors bonded to the OH group of AZD4625 in all local molecular environments (blue) and in the NMR set (red). (c) Computed relative formation energies of the local molecular environments in the NMR set for different hydrogen bond acceptors bonded to the OH proton. The zero is set to be the mean formation energy of intermolecular complexes where no hydrogen bonding acceptor is bonded to the OH proton of the central molecule. (d) Histogram of dihedral angles for the aliphatic ring in all molecules (blue) and in the NMR set (pink). The rotatable bond associated with the dihedral angle is drawn in orange. Stars indicate the atoms used for the computation of the dihedral angle. Adapted from ref. 165. | |
Direct structure optimization
Before moving on to discuss experimental approaches to measuring and assigning chemical shifts, we note that there is ample room for improvement in the structure generation and shift prediction steps for NMR crystallography, and this is an active area of research. For example, while powerful, CSP is a time-consuming approach whose efficiency could be greatly improved by making use of chemical shifts at an earlier stage of the process. Moreover, if the set of candidates does not contain the correct structure, then the process fails, and in these cases requires the addition of some knowledge of internuclear distances to constrain or direct the CSP process.56,81,92 In contrast, more established approaches to de novo structure determination, for example by single-crystal X-ray diffraction of large molecules or by solution NMR, usually involve an iterative process where a (often random) starting structure is optimized under the combined effect of an (usually empirical) energetic potential and a penalty term that compares the computed observables with the measured values at every step of the optimization.230 This is a very powerful approach to finding the correct structure, and is enabled by the fact that the calculation of observables from any trial structure is rapid. Until recently, this had not been possible in chemical shift based NMR crystallography, except for a few exceptions where shifts could be derived from force-fields,231,232 since it would have required the DFT calculations discussed above. We have recently shown how the structure of microcrystalline molecular solids can be determined by integrating on-the-fly shift calculations using ShiftML into a simulated annealing optimization protocol, as illustrated in Fig. 9.218 The approach was demonstrated to successfully determine five crystal structures, and notably for two different polymorphs of the drug molecule AZD8329.218 While this proof-of-concept implementation is exciting, we note that this approach is not as straightforward as it seems, since it requires high accuracy in shift predictions and because optimizing crystal structures is very different from optimizing isolated molecules.
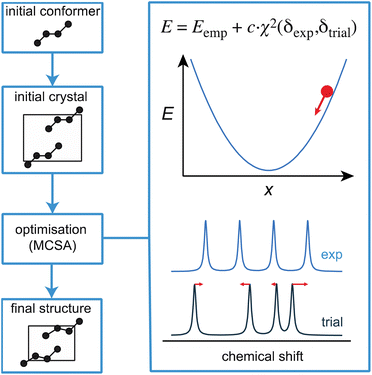 |
| Fig. 9 A schematic outline of one approach to direct structural optimisation using on-the-fly chemical shifts. The driving force for optimisation is an empirical energy term that is the sum of a computed internal energy term E that ensures physically realistic structures, and a term that drives agreement between experimental and predicted chemical shifts.218 | |
Measuring and assigning chemical shifts
We now turn our attention to the experimental measurement and assignment of chemical shifts in powdered molecular solids at natural isotopic abundance. This is a prerequisite for any NMR crystallography study, and has been the subject of intense ongoing research for the last 80 years, with hundreds of different NMR pulse sequences having been developed and tested.5 We will not review this large body of work here, but rather propose to illustrate the two main approaches used to assign 1H, 13C and 15N spectra in molecular solids at natural isotopic abundance today, with illustrations from our own group.
First, in general, all of the state-of-the-art assignment methods are based on using magic angle spinning (MAS)233,234 to obtain high-resolution NMR spectra. Spectral resolution is then further increased by acquisition of two (or higher) dimensional correlation spectra, and the nature of the correlations is then used to determine site-specific assignments of the observed resonance frequencies to each of the nuclei in the molecule/material. Correlations are most often obtained using cross polarization235 to transfer magnetization from one nuclei to another via the through-space dipolar coupling.
There are two main approaches to implement this, which are based on (i) carbon-13 (or 15N, 29Si, 31P…), or (ii) proton detected experiments, and the schematic workflows are illustrated in Fig. 10.
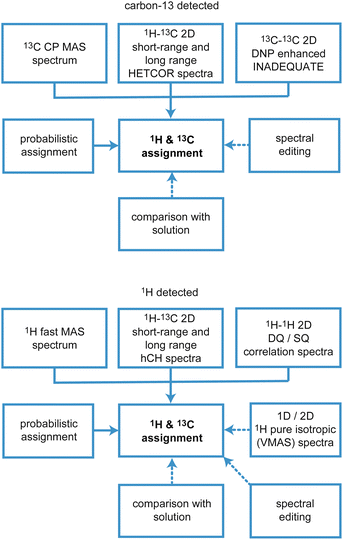 |
| Fig. 10 Schematic workflows for assignment using 13C (upper) and 1H (lower) detection strategies. Solid arrows indicate core steps in the assignment process that are always used, while dotted arrows indicate the most typical supplementary experiments and approaches that are used in cases where the core techniques leave some ambiguity. | |
Assignment using carbon-13 detected experiments
Typically, as shown in Fig. 10, all assignment strategies start with the acquisition of one-dimensional 1H directly detected and 13C CPMAS spectra. Examples of these are shown in Fig. 11 for the example of a sample of micro-crystalline Atuliflapon,54 together with the one-dimensional 15N CPMAS spectrum.
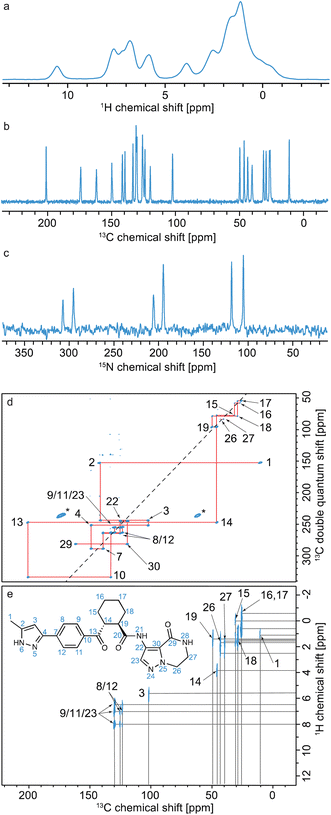 |
| Fig. 11 Spectra used for the carbon-13 detection based assignment of Atuliflapon (chemical structure shown inset).54 One dimensional (a) 1H (21.1 T, 111 kHz MAS rate, 298 K), (b) 13C CP (11.7 T, 22 kHz MAS rate, 298 K) and (c) 15N CP (9.4 T, 12 kHz MAS rate, 298 K) NMR spectra. (d) 13C–13C DNP enhanced solvent suppressed INADEQUATE (9.4 T, 12.5 kHz MAS rate, 100 K), and (e), 1H–13C HETCOR spectra (11.7 T, 22 kHz MAS rate, with eDUMBO-122 homonuclear decoupling236 in t1). In (d), the 13C peaks denoted by an asterisk at 60 and 170 ppm are attributed to impurities introduced during sample preparation. Adapted from ref. 54. | |
In both strategies, the core methods for assignment involve two-dimensional correlation spectra, and the approaches diverge in the set of spectra that are used. For 13C detected experiments, the two workhorse correlation experiments used for assignment are 13C detected 1H–13C HETCOR5,237,238 and 13C–13C refocused INADEQUATE239 spectra. Spectra are usually acquired using 3–4 mm rotors, with MAS rates of 8–12 kHz.
HETCOR spectra correlate 1H and 13C spectra through cross-polarization from 1H to 13C, followed by detection of the 13C signal. This yields dipolar mediated (i.e. through-space) correlations between the isotropic chemical shifts of protons in ω1, and isotropic chemical shifts of 13C in ω2. Typically, two HETCOR spectra are recorded; one with a short (e.g. 100 μs for the spectrum shown in Fig. 11e) CP contact time that yields correlations primarily between directly bonded nuclei, and a second with a longer (e.g. 4 ms) CP contact time that yields correlations with nuclei that are up to around 3–5 Å apart.
Refocused INADEQUATE spectra yield J-mediated (i.e. through-bond) correlations between directly-bonded pairs of carbon-13 nuclei, as illustrated in the spectrum of Fig. 11d.
Armed with these two spectra, complete 1H and 13C assignment can often be directly achieved by first mapping out all the connections between bonded 13C seen in the refocused INADEQUATE spectrum, and then connecting each of the assigned 13C resonances to their attached proton. This is the case for Atuliflapon using the spectra shown in Fig. 11.
However, in many cases, spectral overlap or missing correlations may render parts of the assignment ambiguous, and these two correlation spectra alone may not be sufficient. In that case, there are two straightforward additions that are used. First, spectral editing techniques240–243 can be used to simplify the one-dimensional 13C spectra and/or the HETCOR spectra and assign a multiplicity to each of the 13C resonances.
Second, Cordova et al.244 recently developed a method for automated probabilistic assignment of experimental chemical shifts for molecular solids directly from their two-dimensional molecular structure using a statistical analysis of a shift database that was constructed by computing shifts for over 200
000 compounds in the Cambridge Structural Database using ShiftML. An example of this applied to Atuliflapon is shown in Fig. 12 where we see that most of the chemical shifts are directly assigned with high levels of confidence, typically leaving only a few permutations of pairs or triples that would need to be resolved by analysis of the two-dimensional connectivities.244 This probabilistic method has no extra cost in terms of experimental acquisition, and when applied to 2D X–H correlation spectra, with or without spectral editing, proves to be very robust. It is now a core guide in all our assignment workflows.
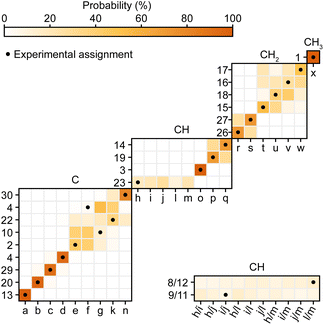 |
| Fig. 12 Marginal individual assignment probabilities of 13C chemical shifts of Atuliflapon obtained using correlated 1H–13C chemical shift distributions and spectral editing.244 For the probability map, labels along the vertical axis indicate nuclei (according to the inset in Fig. 11), and labels along the horizontal axis denote experimental shifts labelled alphabetically in order of decreasing 13C shift. The carbon multiplicity is indicated above each marginal assignment probability map. Adapted from ref. 244. | |
The 1H and 13C assignment of Ritonavir provides a particularly complete recent example of carbon-13 based assignment using HETCOR, refocused-INADEQUATE, spectral editing and the probabilistic method.244
There is one key Achilles’ heel to the carbon-13 based methods, which is sensitivity. The refocused-INADEQUATE experiment correlates pairs of directly bonded carbon-13 nuclei, which only occur every 1 in 10
000 at natural isotopic abundance. While De Paëpe et al. showed 20 years ago that it is possible to obtain refocused-INADEQUATE spectra from natural products with up to 30 carbon atoms in favorable cases, if the experiment is carefully optimized and long (∼1 week) acquisition times are used,245 in most practical cases for molecules with more than 10 carbon atoms this is not possible.
The sensitivity problem in MAS NMR has in principle been alleviated with the introduction of high-field dynamic nuclear polarization (DNP),246,247 which can increase NMR signal intensity by up to two orders of magnitude. MAS DNP has been extensively developed with the introduction of impregnation methods for materials,76,248,249 and can today yield signal enhancements of up to a factor 100 for powdered molecular solids. This has had a transformative effect, and in particular with the demonstration that efficient natural abundance 13C–13C correlation spectra can be obtained, DNP76–78 has made the carbon-13 based assignment strategy outlined above practical. Indeed, the refocused-INADEQUATE spectrum shown in Fig. 11d was only accessible with the use of DNP.
That said, enabling DNP requires formulating the sample with a non-solvent containing a polarizing agent, and finding a formulation that yields significant DNP enhancements on the target substrate is often not simple. If DNP enhancements more than a factor 10 cannot be achieved, natural abundance 13C–13C correlations are often inaccessible. In turn, the absence of a 13C–13C correlation makes carbon-13 based assignment extremely challenging in all but the most straightforward cases.
Assignment using 1H detected experiments
Another route to sensitivity enhancement in solid-state NMR would be to adopt 1H detection strategies. Indeed solution-state NMR has been driven by 1H based detection strategies, primarily enabled by the high spectral resolution obtained in solution-state 1H NMR. The more limited use of these methods in solids is because 1H NMR spectra of solids are typically two-orders of magnitude less well resolved.5 However, in cases where the resolution in the proton spectrum is sufficient, the advantage provided by 1H detection in solids is clearly established both in biological applications5,66,250–257 and in materials samples,42,258–276 including in combination with DNP.277–282
The advent of faster MAS, which usually leads to better resolved 1H spectra, has been a key factor in enabling 1H detection in a broader range of systems.42,256,275,283–288 Due to hardware advances, we are now able to reach magic-angle spinning (MAS) rates above 100 kHz. At such spinning rates MAS results in sufficient 1H line narrowing for the acquisition of high-resolution 1D and 2D 1H-detected spectra for typical molecular solids. For such materials, transitioning to 1H detection instead of 13C detection translates to faster acquisition due to the high sensitivity of protons, and avoids the need for hyperpolarization by DNP.
For 1H detected experiments, the two workhorse correlation experiments used for assignment are 1H detected hCH66,268,270,289 and 1H–1H double-quantum–single-quantum (DQ/SQ) experiments,42,290,291 as illustrated in Fig. 13 for the case of Verinurad.128 Spectra are usually acquired using sub 2 mm rotors, with MAS rates >50 kHz.
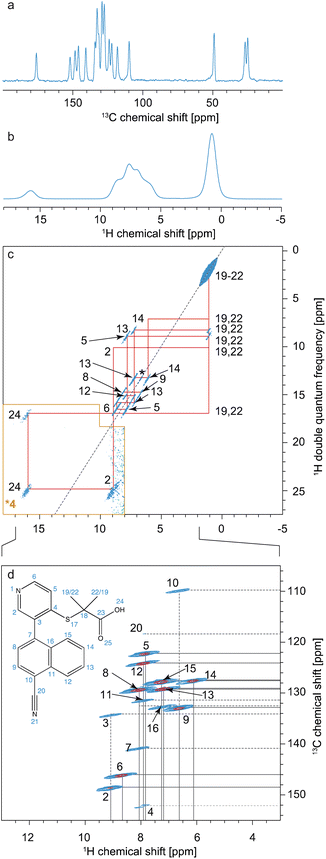 |
| Fig. 13 Spectra used for the 1H detection based assignment of Verinurad (chemical structure shown inset).128 (a) 1D 1H spectrum at 160 kHz MAS. (b) 13C CP spectrum at 100 kHz MAS. (c) 2D 1H–1H BABA spectrum obtained at 160 kHz MAS. Red lines indicate the connectivities between cross peaks. (d) The aromatic region of the 2D hCH long-range spectrum in blue (4 ms direct CP contact time) overlaid with a 2D hCH short-range spectrum in red. The assigned cross-peaks are indicated by labels, with solid lines for protonated carbons and dashed lines for quaternary carbons. Adapted from ref. 128. | |
hCH spectra correlate 1H and 13C spectra through cross-polarization from 1H to 13C, followed by evolution of 13C magnetization and back CP transfer to 1H for detection. This yields dipolar mediated (i.e. through-space) correlations between the isotropic chemical shifts of carbon-13 in ω1, and isotropic chemical shifts of protons in ω2. Typically, two hCH spectra are recorded; one with a short (e.g. 250 μs for the red spectrum shown in Fig. 13d) back CP contact time that yields correlations primarily between directly bonded nuclei, and a second with a longer (e.g. 4 ms for the blue spectrum in Fig. 13d) back CP contact time that yields correlations with nuclei that are up to around 3–5 Å apart.
1H–1H DQ/SQ spectra yield dipolar-mediated (i.e. through-space) correlations between pairs of 1H nuclei, typically separated by distances up to 5 Å depending on the mixing times used for double-quantum excitation and reconversion, as illustrated in the spectrum of Fig. 13c.
In analogy to the use of the carbon-13 detected spectra above, complete 1H and 13C assignment can then be directly achieved by first mapping out all the connections between proximal 1H seen in the DQ/SQ spectrum, and then connecting each of the assigned 1H resonances to their attached carbon.
There is usually likely to be more spectral overlap in the 1H–1H DQ/SQ spectrum than in an analogous 13C–13C refocused INADEQUATE spectrum, and these two correlation spectra alone are often not sufficient. As a result the probabilistic assignment approach244 described above is used by default, and spectral editing methods can also be used as needed.
This 1H detected approach has recently been described in detail, for example, for the assignment of the 1H and 13C resonances in Verinurad.128 Currently, the 1H approach allows complete assignment in molecular solids with up to about 30 carbon atoms. The main limit of the 1H detected approach in molecular solids is poor 1H resolution. Due to the dense network of 1H–1H dipolar couplings, 1H spectra respond differently to MAS than dilute nuclei such as 13C or 15N, and 1H spectra get progressively narrower as spinning gets faster.292–294 At spinning rates up to around 60 kHz, additional narrowing can be obtained by using dipolar-decoupling pulse sequences in CRAMPS type approaches236,295–298 (and indeed this is what is usually done in t1 of the carbon-13 detected HETCOR experiments discussed above (Fig. 11e)). However, so far, no additional narrowing has been observed above 60–65 kHz MAS from CRAMPS type approaches, and the best 1H resolution obtained today by coherent averaging is from MAS spectra spinning at the fastest MAS rates available (∼160–180 kHz) in the highest magnetic fields,254 with good representative contemporary examples for molecular solids being the 800 MHz spectra of Verinurad at 160 kHz MAS in Fig. 13,128 or the 1 GHz spectra of Ritlectinib tosylate at 60 kHz MAS obtained by Rehman et al.129 Even with recent advances, typical 1H linewidths obtained at 100 kHz MAS still comprise 100–400 Hz of dipolar broadening.293
An alternative approach to 1H line narrowing was recently introduced where instead of trying to optimize and perfect a coherent averaging scheme to minimize errors that cause residual dipolar broadening, the errors are mapped into a second dimension of a 2D correlation experiment.299 For example, in a dataset of 1H spectra acquired at different MAS rates, the isotropic shifts do not change as a function of MAS rate, but the dipolar contribution (broadening and shift) scales with the rate. These 2D datasets can be transformed using deep learning models to yield a one-dimensional pure-isotropic proton (PIP) spectrum.300 These new approaches provide the highest 1H NMR resolution available today in rigid solids. In analogy, a 3D dataset of (for example) 1H–1H DQ/SQ spectra acquired at different MAS rates can be transformed to obtain a 2D pure-isotropic 1H–1H DQ/SQ correlation spectrum.301 These methods were used in the assignment of Verinurad,128 and an example of the increased resolution this provides for the case of L-tyrosine hydrochloride is shown in Fig. 14.300,301 These new methods enable assignment of more crowded 1H spectra and open up new routes to 1H detected strategies.
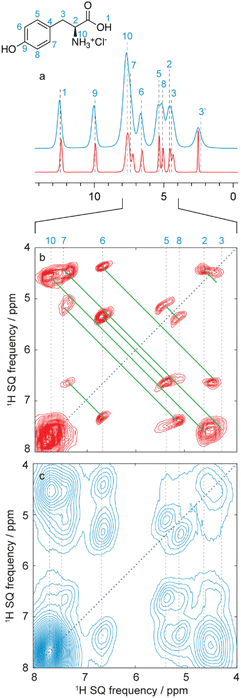 |
| Fig. 14 (a) Pure isotropic 1H spectrum of L-tyrosine hydrochloride obtained using a 2D dataset of 36 MAS spectra recorded at spinning rates from 30 to 100 kHz (red), as compared to the 100 kHz MAS spectrum (blue). The variable rate dataset was transformed using the PIPNet deep learning model.300 (b and c) Expansions of 100 kHz MAS 2D 1H–1H DQ/SQ BABA spectra (blue) and pure isotropic 2D 1H–1H DQ/SQ BABA spectra (red). The isotropic spectrum was inferred with the PINet2D model from a 3D VMAS dataset of 11 2D spectra recorded at MAS rates between 50 and 100 kHz.301 Both spectra are shown after shearing to an SQ/SQ representation. Vertical lines indicate the previously assigned proton shifts at 100 kHz MAS,299,300 and the green lines the observed double quantum correlations. Adapted from ref. 300 and 301. | |
Conclusions
In summary, the development of new NMR methods, in combination with advanced computational methods, over the last 20 years has led to robust and broadly applicable methods for atomic-level structure determination in materials where structure and dynamics were previously the most inaccessible. These range from powdered micro-crystalline compounds to complex hierarchical hybrid materials.
The advent of these advanced NMR crystallography methods has been enabled in particular: by the introduction of new strategies for structure determination from chemical shifts through first principles calculations, augmented by machine learning and large scale data mining approaches; by new strategies for dynamic nuclear polarization enhanced NMR through innovative approaches to generating hyperpolarization; and by developments in multi-dimensional super-resolution 1H NMR experiments, through ultra-fast magic angle spinning and new approaches to error mapping.
We note that here we have centred the discussion on molecular solids, but that analogous chemical shift led structure determination methods are also broadly developed and implemented for inorganic materials, and detailed discussions and reviews can be found elsewhere.5,23,34
Also, in this article the focus has been exclusively on the NMR methods. As mentioned briefly in the introduction, it should go without saying that the NMR led methods here will always be used in combination with any other structural information available, whether from other methods (such as STM, TEM, PXRD, micro-ED…), or from other NMR probes.
The impact of these advances cannot be understated, as they provide the possibility for rational design of better properties in all the application areas discussed above.
Data availability
There is no primary data associated with this article.
Conflicts of interest
There are no conflicts of interest to declare.
Acknowledgements
This work has been supported by the Swiss National Science Foundation Grant No. 200020_212046. I am grateful to Daria Torodii and Jacob Holmes for their comments on the manuscript, and for their daily stimulus in all the areas of development discussed above.
References
- R. Wang, M. Mujahid, Y. Duan, Z. K. Wang, J. J. Xue and Y. Yang, Adv. Funct. Mater., 2019, 29, 1808843 CrossRef CAS
.
- A. K. Jena, A. Kulkarni and T. Miyasaka, Chem. Rev., 2019, 119, 3036–3103 CrossRef CAS PubMed
.
- S. I. Seok, M. Gratzel and N. G. Park, Small, 2018, 14, 1704177 CrossRef
.
- Y. G. Rong, Y. Hu, A. Y. Mei, H. R. Tan, M. I. Saidaminov, S. I. Seok, M. D. McGehee, E. H. Sargent and H. W. Han, Science, 2018, 361, eaat8235 CrossRef
.
- B. Reif, S. E. Ashbrook, L. Emsley and M. Hong, Nat. Rev. Methods Primers, 2021, 1, 2 CrossRef CAS
.
- E. J. Kim, R. L. Siegelman, H. Z. H. Jiang, A. C. Forse, J. H. Lee, J. D. Martell, P. J. Milner, J. M. Falkowski, J. B. Neaton, J. A. Reimer, S. C. Weston and J. R. Long, Science, 2020, 369, 392 CrossRef CAS
.
- A. K. Mohamed, P. Moutzouri, P. Berruyer, B. J. Walder, J. Siramanont, M. Harris, M. Negroni, S. C. Galmarini, S. C. Parker, K. L. Scrivener, L. Emsley and P. Bowen, J. Am. Chem. Soc., 2020, 142, 11060–11071 CrossRef PubMed
.
- M. Seifrid, G. N. M. Reddy, B. F. Chmelka and G. C. Bazan, Nat. Rev. Mater., 2020, 5, 910–930 CrossRef CAS
.
- A. Kirui, W. Zhao, F. Deligey, H. Yang, X. Kang, F. Mentink-Vigier and T. Wang, Nat. Commun., 2022, 13, 538 CrossRef CAS
.
- T. Liu, M. Leskes, W. Yu, A. J. Moore, L. Zhou, P. M. Bayley, G. Kim and C. P. Grey, Science, 2015, 350, 530–533 CrossRef CAS PubMed
.
- T. Z. H. Gani, Z. J. Berkson, R. Zhu, J. H. Kang, J. R. Di Iorio, K. W. Chan, D. F. Consoli, S. K. Shaikh, C. Coperet and Y. Roman-Leshkov, Nature, 2023, 617, 524–528 CrossRef CAS
.
- J. Jeong, M. Kim, J. Seo, H. Lu, P. Ahlawat, A. Mishra, Y. Yang, M. A. Hope, F. T. Eickemeyer, M. Kim, Y. J. Yoon, I. W. Choi, B. P. Darwich, S. J. Choi, Y. Jo, J. H. Lee, B. Walker, S. M. Zakeeruddin, L. Emsley, U. Rothlisberger, A. Hagfeldt, D. S. Kim, M. Gratzel and J. Y. Kim, Nature, 2021, 592, 381–385 CrossRef CAS PubMed
.
- H. M. Rietveld, J. Appl. Crystallogr., 1969, 2, 65–71 CrossRef CAS
.
- K. D. M. Harris, Top. Curr. Chem., 2012, 315, 133–177 CrossRef CAS
.
- C. E. Hughes, I. Boughdiri, C. Bouakkaz, P. A. Williams and K. D. M. Harris, Cryst. Growth Des., 2018, 18, 42–46 CrossRef CAS
.
- T. Gruene, J. T. C. Wennmacher, C. Zaubitzer, J. J. Holstein, J. Heidler, A. Fecteau-Lefebvre, S. De Carlo, E. Muller, K. N. Goldie, I. Regeni, T. Li, G. Santiso-Quinones, G. Steinfeld, S. Handschin, E. van Genderen, J. A. van Bokhoven, G. H. Clever and R. Pantelic, Angew. Chem., Int. Ed., 2018, 57, 16313–16317 CrossRef CAS
.
- M. Gemmi, E. Mugnaioli, T. E. Gorelik, U. Kolb, L. Palatinus, P. Boullay, S. Hovmoller and J. P. Abrahams, ACS Cent. Sci., 2019, 5, 1315–1329 CrossRef CAS
.
- T. Gruene, J. J. Holstein, G. H. Clever and B. Keppler, Nat. Rev. Chem., 2021, 5, 660–668 CrossRef CAS
.
- Z. H. Huang, E. S. Grape, J. Li, A. K. Inge and X. D. Zou, Coord. Chem. Rev., 2021, 427, 213583 CrossRef CAS
.
- C. G. Jones, M. W. Martynowycz, J. Hattne, T. J. Fulton, B. M. Stoltz, J. A. Rodriguez, H. M. Nelson and T. Gonen, ACS Cent. Sci., 2018, 4, 1587–1592 CrossRef CAS
.
- B. L. Nannenga and T. Gonen, Nat. Methods, 2019, 16, 369–379 CrossRef CAS
.
- P. Hodgkinson, Prog. Nucl. Magn. Reson. Spectrosc., 2020, 118–119, 10–53 CrossRef CAS
.
-
S. A. Southern and D. L. Bryce, in Annual Reports on NMR Spectroscopy, ed. G. A. Webb, 2021, vol. 102, pp. 1–80 Search PubMed
.
- D. J. Kubicki, S. D. Stranks, C. P. Grey and L. Emsley, Nat. Rev. Chem., 2021, 5, 624–645 CrossRef CAS PubMed
.
-
L. P. Hughes, P. M. J. Szell, H. Blade and S. P. Brown, in Magnetic Resonance and its Applications in Drug Formulation and Delivery, ed. M. D. Mantle and L. P. Hughes, Royal Society of Chemistry, 2024, vol. 33 Search PubMed
.
- D. H. Brouwer, J. Am. Chem. Soc., 2008, 130, 6306 CrossRef CAS
.
- D. H. Brouwer, S. Cadars, J. Eckert, Z. Liu, O. Terasaki and B. F. Chmelka, J. Am. Chem. Soc., 2013, 135, 5641–5655 CrossRef CAS
.
- D. H. Brouwer, Solid State Nucl. Magn. Reson., 2013, 51–52, 37–45 CrossRef CAS
.
- D. H. Brouwer, R. J. Darton, R. E. Morris and M. H. Levitt, J. Am. Chem. Soc., 2005, 127, 10365–10370 CrossRef CAS PubMed
.
- S. Cadars, D. H. Brouwer and B. F. Chmelka, Phys. Chem. Chem. Phys., 2009, 11, 1825–1837 RSC
.
- C. A. Fyfe and D. H. Brouwer, J. Am. Chem. Soc., 2006, 128, 11860–11871 CrossRef CAS
.
- T. Loiseau, L. Lecroq, C. Volkringer, J. Marrot, G. Ferey, M. Haouas, F. Taulelle, S. Bourrelly, P. L. Llewellyn and M. Latroche, J. Am. Chem. Soc., 2006, 128, 10223–10230 CrossRef CAS
.
- C. J. Pickard and R. J. Needs, J. Phys.: Condens. Matter, 2011, 23, 053201 CrossRef PubMed
.
- S. E. Ashbrook and D. McKay, Chem. Commun., 2016, 52, 7186–7204 RSC
.
- B. Elena and L. Emsley, J. Am. Chem. Soc., 2005, 127, 9140–9146 CrossRef CAS PubMed
.
- B. Elena, G. Pintacuda, N. Mifsud and L. Emsley, J. Am. Chem. Soc., 2006, 128, 9555–9560 CrossRef CAS PubMed
.
- C. J. Pickard, E. Salager, G. Pintacuda, B. Elena and L. Emsley, J. Am. Chem. Soc., 2007, 129, 8932–8933 CrossRef CAS
.
- E. Salager, R. S. Stein, C. J. Pickard, B. Elena and L. Emsley, Phys. Chem. Chem. Phys., 2009, 11, 2610–2621 RSC
.
- E. Salager, G. M. Day, R. S. Stein, C. J. Pickard, B. Elena and L. Emsley, J. Am. Chem. Soc., 2010, 132, 2564–2566 CrossRef CAS
.
- M. Baias, C. M. Widdifield, J.-N. Dumez, H. P. G. Thompson, T. G. Cooper, E. Salager, S. Bassil, R. S. Stein, A. Lesage, G. M. Day and L. Emsley, Phys. Chem. Chem. Phys., 2013, 15, 8069–8080 RSC
.
- M. Baias, J.-N. Dumez, P. H. Svensson, S. Schantz, G. M. Day and L. Emsley, J. Am. Chem. Soc., 2013, 135, 17501–17507 CrossRef CAS PubMed
.
- S. P. Brown and H. W. Spiess, Chem. Rev., 2001, 101, 4125–4155 CrossRef CAS
.
- S. P. Brown, T. Schaller, U. P. Seelbach, F. Koziol, C. Ochsenfeld, F. G. Klarner and H. W. Spiess, Angew. Chem., Int. Ed., 2001, 40, 717–720 CrossRef CAS
.
- G. R. Goward, D. Sebastiani, I. Schnell, H. W. Spiess, H.-D. Kim and H. Ishida, J. Am. Chem. Soc., 2003, 125, 5792–5800 CrossRef CAS PubMed
.
- A. Rapp, I. Schnell, D. Sebastiani, S. P. Brown, V. Percec and H. W. Spiess, J. Am. Chem. Soc., 2003, 125, 13284–13297 CrossRef CAS PubMed
.
- T. Schaller, U. P. Buechele, F.-G. Klaerner, D. Blaeser, R. Boese, S. P. Brown, H. W. Spiess, F. Koziol, J. Kussmann and C. Ochsenfeld, J. Am. Chem. Soc., 2007, 129, 1293–1303 CrossRef CAS
.
- D. Dudenko, A. Kiersnowski, J. Shu, W. Pisula, D. Sebastiani, H. W. Spiess and M. R. Hansen, Angew. Chem., Int. Ed., 2012, 51, 11068–11072 CrossRef CAS PubMed
.
- L. Mafra, S. M. Santos, R. Siegel, I. Alves, F. A. Almeida Paz, D. Dudenko and H. W. Spiess, J. Am. Chem. Soc., 2012, 134, 71–74 CrossRef CAS PubMed
.
- J. K. Harper, D. H. Barich, E. M. Heider, D. M. Grant, R. R. Franke, J. H. Johnson, Y. G. Zhang, P. L. Lee, R. B. Von Dreele, B. Scott, D. Williams and G. B. Ansell, Cryst. Growth Des., 2005, 5, 1737–1742 CrossRef CAS
.
- J. K. Harper and D. M. Grant, Cryst. Growth Des., 2006, 6, 2315–2321 CrossRef CAS
.
- E. M. Heider, J. K. Harper and D. M. Grant, Phys. Chem. Chem. Phys., 2007, 9, 6083–6097 RSC
.
- J. K. Harper, R. Iuliucci, M. Gruber and K. Kalakewich, CrystEngComm, 2013, 15, 8693–8704 RSC
.
- M. Li, F. Meng, Y. Tsutsumi, J.-P. Amoureux, W. Xu, X. Lu, F. Zhang and Y. Su, Mol. Pharmaceutics, 2020, 17, 2196–2207 CrossRef CAS PubMed
.
- M. Cordova, M. Balodis, A. Hofstetter, F. Paruzzo, S. O. N. Lill, E. S. E. Eriksson, P. Berruyer, B. S. de Almeida, M. J. Quayle, S. T. Norberg, A. S. Ankarberg, S. Schantz and L. Emsley, Nat. Commun., 2021, 12, 2964 CrossRef CAS PubMed
.
- E. A. Engel, A. Anelli, A. Hofstetter, F. Paruzzo, L. Emsley and M. Ceriotti, Phys. Chem. Chem. Phys., 2019, 21, 23385–23400 RSC
.
- A. Hofstetter, M. Balodis, F. M. Paruzzo, C. M. Widdifield, G. Steyanato, A. C. Pinon, P. J. Bygrave, G. M. Day and L. Emsley, J. Am. Chem. Soc., 2019, 141, 16624–16634 CrossRef CAS PubMed
.
- A. Hofstetter and L. Emsley, J. Am. Chem. Soc., 2017, 139, 2573–2576 CrossRef CAS PubMed
.
- F. M. Paruzzo, A. Hofstetter, F. Musil, S. De, M. Ceriotti and L. Emsley, Nat. Commun., 2018, 9, 4501 CrossRef PubMed
.
- A. Klein, P. Rovó, V. V. Sakhrani, Y. Wang, J. B. Holmes, V. Liu, P. Skowronek, L. Kukuk, S. K. Vasa, P. Güntert, L. J. Mueller and R. Linser, Proc. Natl. Acad. Sci. U. S. A., 2022, 119, e2114690119 CrossRef PubMed
.
- K. R. Chalek, X. Dong, F. Tong, R. A. Kudla, L. Zhu, A. D. Gill, W. Xu, C. Yang, J. D. Hartman, A. Magalhaes, R. O. Al-Kaysi, R. C. Hayward, R. J. Hooley, G. J. O. Beran, C. J. Bardeen and L. J. Mueller, Chem. Sci., 2021, 12, 453–463 RSC
.
- F. Castellani, B. van Rossum, A. Diehl, M. Schubert, K. Rehbein and H. Oschkinat, Nature, 2002, 420, 99–102 CrossRef
.
- A. Lange, K. Giller, S. Hornig, M. F. Martin-Eauclaire, O. Pongs, S. Becker and M. Baldus, Nature, 2006, 440, 959–962 CrossRef CAS PubMed
.
- C. Wasmer, A. Lange, H. Van Melckebeke, A. B. Siemer, R. Riek and B. H. Meier, Science, 2008, 319, 1523–1526 CrossRef CAS
.
- M. J. Knight, A. J. Pell, I. Bertini, I. C. Felli, L. Gonnelli, R. Pierattelli, T. Herrmann, L. Emsley and G. Pintacuda, Proc. Natl. Acad. Sci. U. S. A., 2012, 109, 11095–11100 CrossRef CAS
.
- A. Loquet, N. G. Sgourakis, R. Gupta, K. Giller, D. Riedel, C. Goosmann, C. Griesinger, M. Kolbe, D. Baker, S. Becker and A. Lange, Nature, 2012, 486, 276–279 CrossRef CAS
.
- L. B. Andreas, K. Jaudzems, J. Stanek, D. Lalli, A. Bertarello, T. Le Marchand, D. C. D. Paepe, S. Kotelovica, I. Akopjana, B. Knott, S. Wegner, F. Engelke, A. Lesage, L. Emsley, K. Tars, T. Herrmann and G. Pintacuda, Proc. Natl. Acad. Sci. U. S. A., 2016, 113, 9187–9192 CrossRef CAS PubMed
.
- M. D. Tuttle, G. Comellas, A. J. Nieuwkoop, D. J. Covell, D. A. Berthold, K. D. Kloepper, J. M. Courtney, J. K. Kim, A. M. Barclay, A. Kendall, W. Wan, G. Stubbs, C. D. Schwieters, V. M. Y. Lee, J. M. George and C. M. Rienstra, Nat. Struct. Mol. Biol., 2016, 23, 409–415 CrossRef CAS
.
- M. A. Walti, F. Ravotti, H. Arai, C. G. Glabe, J. S. Wall, A. Bockmann, P. Guntert, B. H. Meier and R. Riek, Proc. Natl. Acad. Sci. U. S. A., 2016, 113, E4976–E4984 CrossRef CAS PubMed
.
- Z. J. Berkson, R. J. Messinger, K. Na, Y. Seo, R. Ryoo and B. F. Chmelka, Angew. Chem., Int. Ed., 2017, 56, 5164–5169 CrossRef CAS PubMed
.
- R. J. Messinger, K. Na, Y. Seo, R. Ryoo and B. F. Chmelka, Angew. Chem., Int. Ed., 2015, 54, 927–931 CrossRef CAS PubMed
.
- R. P. Sangodkar, B. J. Smith, D. Gajan, A. J. Rossini, L. R. Roberts, G. P. Funkhouser, A. Lesage, L. Emsley and B. F. Chmelka, J. Am. Chem. Soc., 2015, 137, 8096–8112 CrossRef CAS PubMed
.
- P. Berruyer, M. Lelli, M. P. Conley, D. L. Silverio, C. M. Widdifield, G. Siddiqi, D. Gajan, A. Lesage, C. Coperet and L. Emsley, J. Am. Chem. Soc., 2017, 139, 849–855 CrossRef CAS PubMed
.
- F. A. Perras, U. Kanbur, A. L. Paterson, P. Chatterjee, I. I. Slowing and A. D. Sadow, Inorg. Chem., 2022, 61, 1067–1078 CrossRef CAS
.
- C. Ochsenfeld, S. P. Brown, I. Schnell, J. Gauss and H. W. Spiess, J. Am. Chem. Soc., 2001, 123, 2597–2606 CrossRef CAS
.
- G. N. M. Reddy, A. Marsh, J. T. Davis, S. Masiero and S. P. Brown, Cryst. Growth Des., 2015, 15, 5945–5954 CrossRef CAS
.
- A. J. Rossini, A. Zagdoun, F. Hegner, M. Schwarzwälder, D. Gajan, C. Copéret, A. Lesage and L. Emsley, J. Am. Chem. Soc., 2012, 134, 16899–16908 CrossRef CAS
.
- H. Takahashi, D. Lee, L. Dubois, M. Bardet, S. Hediger and G. De Paepe, Angew. Chem., Int. Ed., 2012, 51, 11766–11769 CrossRef CAS PubMed
.
- K. Maerker, M. Pingret, J.-M. Mouesca, D. Gasparutto, S. Hediger and G. De Paepe, J. Am. Chem. Soc., 2015, 137, 13796–13799 CrossRef
.
- M. Dekhil, G. Mollica, T. T. Bonniot, F. Ziarelli, P. Thureau and S. Viel, Chem. Commun., 2016, 52, 8565–8568 RSC
.
- G. Mollica, M. Dekhil, F. Ziarelli, P. Thureau and S. Viel, Angew. Chem., Int. Ed., 2015, 54, 6028–6031 CrossRef CAS PubMed
.
- P. Thureau, S. Sturniolo, M. Zilka, F. Ziarelli, S. Viel, J. R. Yates and G. Mollica, Magn. Reson. Chem., 2019, 57, 256–264 CrossRef CAS PubMed
.
- K. Märker, S. Paul, C. Fernández-de-Alba, D. Lee, J.-M. Mouesca, S. Hediger and G. De Paëpe, Chem. Sci., 2017, 8, 974–987 RSC
.
- A. C. Dedios, J. G. Pearson and E. Oldfield, Science, 1993, 260, 1491–1496 CrossRef CAS PubMed
.
- J. C. Facelli and D. M. Grant, Nature, 1993, 365, 325–327 CrossRef CAS
.
- D. Sebastiani and M. Parrinello, J. Phys. Chem. A, 2001, 105, 1951–1958 CrossRef CAS
.
- P. E. Blochl, Phys. Rev. B: Condens. Matter Mater. Phys., 1994, 50, 17953–17979 CrossRef PubMed
.
- C. J. Pickard and F. Mauri, Phys. Rev. B: Condens. Matter Mater. Phys., 2001, 63, 245101 CrossRef
.
- J. R. Yates, C. J. Pickard and F. Mauri, Phys. Rev. B, 2007, 76, 024401 CrossRef
.
- G. J. O. Beran, eMagRes, 2019, 8, 215–226 CAS
.
- S. A. Ramos, L. J. Mueller and G. J. O. Beran, Faraday Discuss., 2024 10.1039/D4FD00072B
.
- J. A. Fernandes, M. Sardo, L. Mafra, D. Choquesillo-Lazarte and N. Masciocchi, Cryst. Growth Des., 2015, 15, 3674–3683 CrossRef CAS
.
- J. Leclaire, G. Poisson, F. Ziarelli, G. Pepe, F. Fotiadu, F. M. Paruzzo, A. J. Rossini, J.-N. Dumez, B. Elena-Herrmann and L. Emsley, Chem. Sci., 2016, 7, 4379–4390 RSC
.
- M. Selent, J. Nyman, J. Roukala, M. Ilczyszyn, R. Oilunkaniemi, P. J. Bygrave, R. Laitinen, J. Jokisaari, G. M. Day and P. Lantto, Chem. – Eur. J., 2017, 23, 5258–5269 CrossRef CAS PubMed
.
- S. O. Nilsson Lill, C. M. Widdifield, A. Pettersen, A. Svensk Ankarberg, M. Lindkvist, P. Aldred, S. Gracin, N. Shankland, K. Shankland, S. Schantz and L. Emsley, Mol. Pharmaceutics, 2018, 15, 1476–1487 CrossRef CAS
.
- H. Al-Johani, E. Abou-Hamad, A. Jedidi, C. M. Widdifield, J. Viger-Gravel, S. S. Sangaru, D. Gajan, D. H. Anjum, S. Ould-Chikh, M. N. Hedhili, A. Gurinov, M. J. Kelly, M. El Eter, L. Cavallo, L. Emsley and J.-M. Basset, Nat. Chem., 2017, 9, 890–895 CrossRef CAS PubMed
.
- L. J. Mueller and M. F. Dunn, Acc. Chem. Res., 2013, 46, 2008–2017 CrossRef CAS
.
- J. D. Hartman, R. A. Kudla, G. M. Day, L. J. Mueller and G. J. Beran, Phys. Chem. Chem. Phys., 2016, 18, 21686–21709 RSC
.
- J. D. Hartman, G. M. Day and G. J. O. Beran, Cryst. Growth Des., 2016, 16, 6479–6493 CrossRef CAS
.
- M. Dracinsky, J. Vicha, K. Bartova and P. Hodgkinson, ChemPhysChem, 2020, 21, 2075–2083 CrossRef CAS PubMed
.
- R. J. Iuliucci, J. D. Hartman and G. J. O. Beran, J. Phys. Chem. A, 2023, 127, 2846–2858 CrossRef CAS
.
- J. R. Yates and A. P. Bartók, Faraday Discuss., 2024 10.1039/D4FD00142G
.
- J. R. Yates, T. N. Pham, C. J. Pickard, F. Mauri, A. M. Amado, A. M. Gil and S. P. Brown, J. Am. Chem. Soc., 2005, 127, 10216–10220 CrossRef CAS PubMed
.
- A. L. Webber, L. Emsley, R. M. Claramunt and S. P. Brown, J. Phys. Chem. A, 2010, 114, 10435–10442 CrossRef CAS PubMed
.
- R. K. Harris, S. A. Joyce, C. J. Pickard, S. Cadars and L. Emsley, Phys. Chem. Chem. Phys., 2006, 8, 137–143 RSC
.
- A. Abraham, D. C. Apperley, T. Gelbrich, R. K. Harris and U. J. Griesser, Can. J. Chem., 2011, 89, 770–778 CrossRef CAS
.
- R. K. Harris, P. Hodgkinson, V. Zorin, J.-N. Dumez, B. Elena-Herrmann, L. Emsley, E. Salager and R. S. Stein, Magn. Reson. Chem., 2010, 48, S103–S112 CrossRef CAS
.
- A. L. Webber, S. Masiero, S. Pieraccini, J. C. Burey, A. S. Tatton, D. Iuga, T. N. Pham, G. P. Spada and S. P. Brown, J. Am. Chem. Soc., 2011, 133, 19777–19795 CrossRef CAS PubMed
.
- R. Harris, Analyst, 2006, 131, 351–373 RSC
.
- A. Othman, J. S. Evans, I. R. Evans, R. K. Harris and P. Hodgkinson, J. Pharm. Sci., 2007, 96, 1380–1397 CrossRef CAS
.
- N. Mifsud, B. Elena, C. J. Pickard, A. Lesage and L. Emsley, Phys. Chem. Chem. Phys., 2006, 8, 3418–3422 RSC
.
- D. H. Brouwer, K. P. Langendoen and Q. Ferrant, Can. J. Chem., 2011, 89, 737–744 CrossRef
.
- J. Brus, J. Czernek, M. Urbanova and C. Cervinka, Molecules, 2022, 27, 679 CrossRef CAS PubMed
.
- J. Czernek and J. Brus, Int. J. Mol. Sci., 2020, 21, 4907 CrossRef CAS
.
- X. Y. Lu, W. Xu, M. Hanada, S. V. Jermain, R. O. Williams and Y. C. Su, J. Pharm. Biomed. Anal., 2019, 165, 47–55 CrossRef CAS
.
- B. R. Luginbuhl, P. Raval, T. Pawlak, Z. F. Du, T. H. Wang, G. Kupgan, N. Schopp, S. Chae, S. Yoon, A. Yi, H. J. Kim, V. Coropceanu, J. L. Bredas, T. Q. Nguyen and G. N. M. Reddy, Adv. Mater., 2022, 34, 2105943 CrossRef PubMed
.
- R. Mathew, B. Stevensson and M. Eden, J. Phys. Chem. B, 2021, 125, 10985–11004 CrossRef CAS PubMed
.
- R. K. Harris, J. Pharm. Pharmacol., 2007, 59, 225–239 CrossRef CAS
.
- A. L. Webber, B. Elena, J. M. Griffin, J. R. Yates, T. N. Pham, F. Mauri, C. J. Pickard, A. M. Gil, R. Stein, A. Lesage, L. Emsley and S. P. Brown, Phys. Chem. Chem. Phys., 2010, 12, 6970–6983 RSC
.
- J. Yates, S. Dobbins, C. Pickard, F. Mauri, P. Ghi and R. Harris, Phys. Chem. Chem. Phys., 2005, 7, 1402–1407 RSC
.
- A.-C. Uldry, J. M. Griffin, J. R. Yates, M. Perez-Torralba, M. D. S. Maria, A. L. Webber, M. L. L. Beaumont, A. Samoson, R. M. Claramunt, C. J. Pickard and S. P. Brown, J. Am. Chem. Soc., 2008, 130, 945–954 CrossRef CAS
.
- J. P. Bradley, S. P. Velaga, O. N. Antzutkin and S. P. Brown, Cryst. Growth Des., 2011, 11, 3463–3471 CrossRef CAS
.
- J. P. Bradley, C. J. Pickard, J. C. Burley, D. R. Martin, L. P. Hughes, S. D. Cosgrove and S. P. Brown, J. Pharm. Sci., 2012, 101, 1821–1830 CrossRef CAS
.
- E. Carignani, S. Borsacchi, J. P. Bradley, S. P. Brown and M. Geppi, J. Phys. Chem. C, 2013, 117, 17731–17740 CrossRef CAS
.
- C. Bonhomme, C. Gervais, F. Babonneau, C. Coelho, F. Pourpoint, T. Azais, S. E. Ashbrook, J. M. Griffin, J. R. Yates, F. Mauri and C. J. Pickard, Chem. Rev., 2012, 112, 5733–5779 CrossRef CAS
.
- A. Z. Tier, K. M. Wust, J. C. B. Vieira, M. Sardo, T. Cendak, L. Mafra, J. Rocha, I. M. Gindri, M. Horner and C. P. Frizzo, CrystEngComm, 2020, 22, 708–719 RSC
.
- L. Wang, F. J. Uribe-Romo, L. J. Mueller and J. K. Harper, Phys. Chem. Chem. Phys., 2018, 20, 8475–8487 RSC
.
- C. Fleischer, S. T. Holmes, K. Levin, S. L. Veinberg and R. Schurko, Faraday Discuss., 2024 10.1039/D4FD00089G
.
- D. Torodii, J. Holmes, P. Moutzouri, S. O. Nilsson Lill, M. Cordova, A. C. Pinon, K. Grohe, S. Wegner, O. D. Putra, S. T. Norberg, A. Welinder, S. Schantz and L. Emsley, Faraday Discuss., 2024 10.1039/D4FD00076E
.
- Z. Rehman, J. Lubay, W. T. T. Franks, E. Corlett, B. Nguyen, G. Scrivens, B. Samas, H. Frericks-Schmidt and S. P. Brown, Faraday Discuss., 2024 10.1039/D4FD00088A
.
- C. Gervais, M. Profeta, V. Lafond, C. Bonhomme, T. Azais, H. Mutin, C. J. Pickard, F. Mauri and F. Babonneau, Magn. Reson. Chem., 2004, 42, 445–452 CrossRef CAS PubMed
.
- A. Scarperi, G. Barcaro, A. Pajzderska, F. Martini, E. Carignani and M. Geppi, Molecules, 2021, 26, 4577 CrossRef CAS PubMed
.
- D. H. Brouwer and J. G. Mikolajewski, Solid State Nucl. Magn. Reson., 2023, 123, 101848 Search PubMed
.
- D. M. Dawson, I. A. Smellie and S. E. Ashbrook, Phys. Chem. Chem. Phys., 2024, 26, 14256–14264 RSC
.
- Y. Shi and D. L. Bryce, Can. J. Chem., 2023, 102, 144–151 CrossRef
.
- P. Hodgkinson and C. M. Widdifield, Chem. Eng. News, 2016, 94, 30–31 Search PubMed
.
- A. C. Poppler, E. K. Corlett, H. Pearce, M. P. Seymour, M. Reid, M. G. Montgomery and S. P. Brown, Acta Crystallogr., Sect. C: Struct. Chem., 2017, 73, 149–156 CrossRef CAS
.
- A. C. Poppler, D. Walker and S. P. Brown, CrystEngComm, 2017, 19, 224–236 RSC
.
- A. E. Watts, K. Maruyoshi, C. E. Hughes, S. P. Brown and K. D. M. Harris, Cryst. Growth Des., 2016, 16, 1798–1804 CrossRef CAS
.
- C. M. Widdifield, H. Robson and P. Hodgkinson, Chem. Commun., 2016, 52, 6685–6688 RSC
.
- O. Al Rahal, G. E. Hughes, P. A. Williams, A. J. Logsdail, Y. Diskin-Posner and K. D. M. Harris, Angew. Chem., Int. Ed., 2019, 58, 18788–18792 CrossRef CAS PubMed
.
- O. Al Rahal, P. A. Williams, C. E. Hughes, B. M. Kariuki and K. D. M. Harris, Cryst. Growth Des., 2021, 21, 2498–2507 CrossRef
.
- E. K. Corlett, H. Blade, L. P. Hughes, P. J. Sidebottom, D. Walker, R. I. Walton and S. P. Brown, CrystEngComm, 2019, 21, 3502–3516 RSC
.
- M. Husak, A. Jegorov, J. Czernek, J. Rohlicek, S. Zizkova, P. Vraspir, P. Kolesa, A. Fitch and J. Brus, Cryst. Growth Des., 2019, 19, 4625–4631 CrossRef CAS
.
- T. Pawlak, M. Szczesio and M. J. Potrzebowski, Materials, 2021, 14, 7175 CrossRef CAS PubMed
.
- B. Peric, G. Szalontai, M. Borovina, D. Vikic-Topic and S. I. Kirin, J. Mol. Struct., 2020, 1221, 128834 CrossRef CAS
.
- F. Rossi, P. Cerreia Vioglio, S. Bordignon, V. Giorgio, C. Nervi, E. Priola, R. Gobetto, K. Yazawa and M. R. Chierotti, Cryst. Growth Des., 2018, 18, 2225–2233 CrossRef CAS
.
- I. C. B. Martins, M. Sardo, E. Alig, L. Fink, M. U. Schmidt, L. Mafra and M. T. Duarte, Cryst. Growth Des., 2019, 19, 1860–1873 CrossRef CAS
.
- T. L. E. Pereira, J. Serrano-Sevillano, B. D. Moreno, J. Reid, D. Carlier and G. Goward, Faraday Discuss., 2024 10.1039/D4FD00077C
.
- C. Guzman-Afonso, Y. L. Hong, H. Colaux, H. Iijima, A. Saitow, T. Fukumura, Y. Aoyama, S. Motoki, T. Oikawa, T. Yamazaki, K. Yonekura and Y. Nishiyama, Nat. Commun., 2019, 10, 3537 CrossRef
.
- F. Bravetti, L. Tapmeyer, K. Skorodumov, E. Alig, S. Habermehl, R. Huhn, S. Bordignon, A. Gallo, C. Nervi, M. R. Chierotti and M. U. Schmidt, IUCrJ, 2023, 10, 448–463 CrossRef CAS PubMed
.
- M. K. Dudek, P. Paluch, J. Sniechowska, K. P. Nartowski, G. M. Day and M. J. Potrzebowski, CrystEngComm, 2020, 22, 4969–4981 RSC
.
- M. Khalaji, P. Paluch, M. J. Potrzebowski and M. K. Dudek, Solid State Nucl. Magn. Reson., 2022, 121, 101813 CrossRef CAS PubMed
.
- T. Pawlak, I. Sudgen, G. Bujacz, D. Iuga, S. P. Brown and M. J. Potrzebowski, Cryst. Growth Des., 2021, 21, 3328–3343 CrossRef CAS PubMed
.
- R. Mathew, K. A. Uchman, L. Gkoura, C. J. Pickard and M. Baias, Magn. Reson. Chem., 2020, 58, 1018–1025 CrossRef CAS
.
- F. Bravetti, S. Bordignon, E. Alig, D. Eisenbeil, L. Fink, C. Nervi, R. Gobetto, M. U. Schmidt and M. R. Chierotti, Chem. – Eur. J., 2022, 28, e202103589 CrossRef CAS PubMed
.
- C. M. Widdifield, S. O. N. Lill, A. Broo, M. Lindkvist, A. Pettersen, A. S. Ankarberg, P. Aldred, S. Schantz and L. Emsley, Phys. Chem. Chem. Phys., 2017, 19, 16650–16661 RSC
.
- A. Bertarello, L. Benda, K. J. Sanders, A. J. Pell, M. J. Knight, V. Pelmenschikov, L. Gonnelli, I. C. Felli, M. Kaupp, L. Emsley, R. Pierattelli and G. Pintacuda, J. Am. Chem. Soc., 2020, 142, 16757–16765 CrossRef CAS
.
- A. Kumar, B. J. Walder, A. K. Mohamed, A. Hofstetter, B. Srinivasan, A. J. Rossini, K. Scrivener, L. Emsley and P. Bowen, J. Phys. Chem. C, 2017, 121, 17188–17196 CrossRef CAS
.
- A. Morales-Melgares, Z. Casar, P. Moutzouri, A. Venkatesh, M. Cordova, A. Kunhi Mohamed, K. L. Scrivener, P. Bowen and L. Emsley, J. Am. Chem. Soc., 2022, 144, 22915–22924 CrossRef CAS
.
- M. A. Hope, T. Nakamura, P. Ahlawat, A. Mishra, M. Cordova, F. Jahanbakhshi, M. Mladenović, R. Runjhun, L. Merten, A. Hinderhofer, B. I. Carlsen, D. J. Kubicki, R. Gershoni-Poranne, T. Schneeberger, L. C. Carbone, Y. Liu, S. M. Zakeeruddin, J. Lewinski, A. Hagfeldt, F. Schreiber, U. Rothlisberger, M. Grätzel, J. V. Milić and L. Emsley, J. Am. Chem. Soc., 2021, 143, 1529–1538 CrossRef CAS
.
- Q. Z. Ni, F. Yang, T. V. Can, I. V. Sergeyev, S. M. D’Addio, S. K. Jawla, Y. Li, M. P. Lipert, W. Xu, R. T. Williamson, A. Leone, R. G. Griffin and Y. Su, J. Phys. Chem. B, 2017, 121, 8132–8141 CrossRef CAS PubMed
.
- J. Brus, J. Czernek, M. Hruby, P. Svec, L. Kobera, S. Abbrent and M. Urbanova, Macromolecules, 2018, 51, 5364–5374 CrossRef CAS
.
-
R. K. Ghosh, J. B. Holmes, M. F. Dunn and L. J. Mueller, in Integrated Structural Biology, ed. T. Polenova, C. M. Quinn and A. M. Gronenborn, Royal Society of Chemistry, 2023, vol. 30 Search PubMed
.
- J. Holmes, D. Torodii, M. Balodis, M. Cordova, A. Hofstetter, F. Paruzzo, S. O. Nilsson Lill, E. Eriksson, P. Berruyer, B. Simões de Almeida, M. J. Quayle, S. T. Norberg, A. Svensk-Ankarberg, S. Schantz and L. Emsley, Faraday Discuss., 2024 10.1039/D4FD00078A
.
- M. Cordova, P. Moutzouri, S. O. Nilsson Lill, A. Cousen, M. Kearns, S. T. Norberg, A. Svensk Ankarberg, J. McCabe, A. C. Pinon, S. Schantz and L. Emsley, Nat. Commun., 2023, 14, 5138 CrossRef CAS
.
- S. Yang and G. M. Day, J. Chem. Theory Comput., 2021, 17, 1988–1999 CrossRef CAS PubMed
.
- H. R. Karfunkel and R. J. Gdanitz, J. Comput. Chem., 1992, 13, 1171–1183 CrossRef CAS
.
- M. Zilka, D. V. Dudenko, C. E. Hughes, P. A. Williams, S. Sturniolo, W. T. Franks, C. J. Pickard, J. R. Yates, K. D. M. Harris and S. P. Brown, Phys. Chem. Chem. Phys., 2017, 19, 25949–25960 RSC
.
- V. E. Bazterra, M. B. Ferraro and J. C. Facelli, J. Chem. Phys., 2002, 116, 5984–5991 CrossRef CAS
.
- Q. Zhu, A. R. Oganov, C. W. Glass and H. T. Stokes, Acta Crystallogr., Sect. B: Struct. Sci., 2012, 68, 215–226 CrossRef CAS
.
- G. J. O. Beran, Chem. Sci., 2023, 14, 13290–13312 RSC
.
- D. Firaha, Y. M. Liu, J. van de Streek, K. Sasikumar, H. Dietrich, J. Helfferich, L. Aerts, D. E. Braun, A. Broo, A. G. DiPasquale, A. Y. Lee, S. Le Meur, S. O. Nilsson Lill, W. J. Lunsmann, A. Mattei, P. Muglia, O. D. Putra, M. Raoui, S. M. Reutzel-Edens, S. Rome, A. Y. Sheikh, A. Tkatchenko, G. R. Woollam and M. A. Neumann, Nature, 2023, 623, 324–328 CrossRef CAS
.
- A. M. Reilly, R. I. Cooper, C. S. Adjiman, S. Bhattacharya, A. D. Boese, J. G. Brandenburg, P. J. Bygrave, R. Bylsma, J. E. Campbell, R. Car, D. H. Case, R. Chadha, J. C. Cole, K. Cosburn, H. M. Cuppen, F. Curtis, G. M. Day, R. A. DiStasio Jr, A. Dzyabchenko, B. P. van Eijck, D. M. Elking, J. A. van den Ende, J. C. Facelli, M. B. Ferraro, L. Fusti-Molnar, C. A. Gatsiou, T. S. Gee, R. de Gelder, L. M. Ghiringhelli, H. Goto, S. Grimme, R. Guo, D. W. Hofmann, J. Hoja, R. K. Hylton, L. Iuzzolino, W. Jankiewicz, D. T. de Jong, J. Kendrick, N. J. de Klerk, H. Y. Ko, L. N. Kuleshova, X. Li, S. Lohani, F. J. Leusen, A. M. Lund, J. Lv, Y. Ma, N. Marom, A. E. Masunov, P. McCabe, D. P. McMahon, H. Meekes, M. P. Metz, A. J. Misquitta, S. Mohamed, B. Monserrat, R. J. Needs, M. A. Neumann, J. Nyman, S. Obata, H. Oberhofer, A. R. Oganov, A. M. Orendt, G. I. Pagola, C. C. Pantelides, C. J. Pickard, R. Podeszwa, L. S. Price, S. L. Price, A. Pulido, M. G. Read, K. Reuter, E. Schneider, C. Schober, G. P. Shields, P. Singh, I. J. Sugden, K. Szalewicz, C. R. Taylor, A. Tkatchenko, M. E. Tuckerman, F. Vacarro, M. Vasileiadis, A. Vazquez-Mayagoitia, L. Vogt, Y. Wang, R. E. Watson, G. A. de Wijs, J. Yang, Q. Zhu and C. R. Groom, Acta Crystallogr., Sect. B: Struct. Sci., Cryst. Eng. Mater., 2016, 72, 439–459 CrossRef CAS
.
- N. F. Ramsey, Phys. Rev., 1950, 78, 699–703 CrossRef CAS
.
- N. F. Ramsey, Phys. Rev., 1950, 77, 567 CrossRef CAS
.
- N. F. Ramsey, Phys. Rev., 1953, 91, 303–307 CrossRef CAS
.
- P. E. Blöchl, Phys. Rev. B: Condens. Matter Mater. Phys., 1994, 50, 17953–17979 CrossRef
.
- J. P. Perdew, K. Burke and M. Ernzerhof, Phys. Rev. Lett., 1996, 77, 3865–3868 CrossRef CAS
.
- S. Grimme, J. Antony, S. Ehrlich and H. Krieg, J. Chem. Phys., 2010, 132, 154104 CrossRef PubMed
.
- G. J. Beran, Chem. Rev., 2016, 116, 5567–5613 CrossRef CAS PubMed
.
- S. T. Holmes, O. G. Engl, M. N. Srnec, J. D. Madura, R. Quinones, J. K. Harper, R. W. Schurko and R. J. Iuliucci, J. Phys. Chem. A, 2020, 124, 3109–3119 CrossRef CAS PubMed
.
- S. T. Holmes, C. M. Boley, A. Dewicki, Z. T. Gardner, C. S. Vojvodin, R. J. Iuliucci and R. W. Schurko, Magn. Reson. Chem., 2024, 62, 179–189 CrossRef CAS PubMed
.
- E. K. Corlett, H. Blade, L. P. Hughes, P. J. Sidebottom, D. Walker, R. I. Walton and S. P. Brown, Solid State Nucl. Magn. Reson., 2020, 108, 101662 CrossRef CAS PubMed
.
- R. K. Harris, P. Hodgkinson, C. J. Pickard, J. R. Yates and V. Zorin, Magn. Reson. Chem., 2007, 45(Suppl 1), S174–S186 CrossRef CAS
.
- S. T. Holmes, C. S. Vojvodin and R. W. Schurko, J. Phys. Chem. A, 2020, 124, 10312–10323 CrossRef CAS PubMed
.
- J. N. Dumez and C. J. Pickard, J. Chem. Phys., 2009, 130, 104701 CrossRef
.
- J. Blahut, J. R. Stocek, M. Sala and M. Dracinsky, J. Magn. Reson., 2022, 345, 107334 CrossRef CAS PubMed
.
- J. R. Stocek, O. Socha, I. Cisarova, T. Slanina and M. Dracinsky, J. Am. Chem. Soc., 2022, 144, 7111–7116 CrossRef CAS
.
- E. A. Engel, V. Kapil and M. Ceriotti, J. Phys. Chem. Lett., 2021, 12, 7701–7707 CrossRef CAS PubMed
.
- M. Wern Huay, N. Nadzran Hafiy Ahmad, Y. Wei Sheung, F. Farah Hasyeena, F. Ibrahima and W. Cecilia Devi, C. R. Chim., 2022, 25, 83–95 Search PubMed
.
- C. Cobas, Magn. Reson. Chem., 2020, 58, 512–519 CrossRef CAS PubMed
.
- J. Meiler, J. Biomol. NMR, 2003, 26, 25–37 CrossRef CAS PubMed
.
- B. Han, Y. Liu, S. W. Ginzinger and D. S. Wishart, J. Biomol. NMR, 2011, 50, 43–57 CrossRef CAS
.
- Y. Shen and A. Bax, J. Biomol. NMR, 2010, 48, 13–22 CrossRef CAS PubMed
.
- Z. Yang, M. Chakraborty and A. D. White, Chem. Sci., 2021, 12, 10802–10809 RSC
.
- J. Aires-de-Sousa, M. C. Hemmer and J. Gasteiger, Anal. Chem., 2002, 74, 80–90 CrossRef CAS
.
- K. A. Blinov, Y. D. Smurnyy, M. E. Elyashberg, T. S. Churanova, M. Kvasha, C. Steinbeck, B. A. Lefebvre and A. J. Williams, J. Chem. Inf. Model., 2008, 48, 550–555 CrossRef CAS PubMed
.
- Y. D. Smurnyy, K. A. Blinov, T. S. Churanova, M. E. Elyashberg and A. J. Williams, J. Chem. Inf. Model., 2008, 48, 128–134 CrossRef CAS PubMed
.
- S. Kuhn, B. Egert, S. Neumann and C. Steinbeck, BMC Bioinf., 2008, 9, 400 CrossRef PubMed
.
- M. Rupp, R. Ramakrishnan and O. A. von Lilienfeld, J. Phys. Chem. Lett., 2015, 6, 3309–3313 CrossRef CAS
.
- A. P. Bartók, S. De, C. Poelking, N. Bernstein, J. R. Kermode, G. Csányi and M. Ceriotti, Sci. Adv., 2017, 3, e1701816 CrossRef PubMed
.
- A. Gupta, S. Chakraborty and R. Ramakrishnan, Machine Learning: Science and Technology, 2021, 2, 035010 Search PubMed
.
- W. Gerrard, L. A. Bratholm, M. J. Packer, A. J. Mulholland, D. R. Glowacki and C. P. Butts, Chem. Sci., 2020, 11, 508–515 RSC
.
- L. A. Bratholm, W. Gerrard, B. Anderson, S. Bai, S. Choi, L. Dang, P. Hanchar, A. Howard, S. Kim, Z. Kolter, R. Kondor, M. Kornbluth, Y. Lee, Y. Lee, J. P. Mailoa, T. T. Nguyen, M. Popovic, G. Rakocevic, W. Reade, W. Song, L. Stojanovic, E. H. Thiede, N. Tijanic, A. Torrubia, D. Willmott, C. P. Butts and D. R. Glowacki, PLoS One, 2021, 16, e0253612 CrossRef CAS
.
- Y. Guan, S. V. Shree Sowndarya, L. C. Gallegos, P. C. St. John and R. S. Paton, Chem. Sci., 2021, 12, 12012–12026 RSC
.
- W. Gerrard, C. Yiu and C. P. Butts, Magn. Reson. Chem., 2022, 60, 1087–1092 CrossRef CAS
.
- J. Cuny, Y. Xie, C. J. Pickard and A. A. Hassanali, J. Chem. Theory Comput., 2016, 12, 765–773 CrossRef CAS PubMed
.
- M. Cordova, E. A. Engel, A. Stefaniuk, F. Paruzzo, A. Hofstetter, M. Ceriotti and L. Emsley, J. Phys. Chem. C, 2022, 126, 16710–16720 CrossRef CAS PubMed
.
- S. Liu, J. Li, K. C. Bennett, B. Ganoe, T. Stauch, M. Head-Gordon, A. Hexemer, D. Ushizima and T. Head-Gordon, J. Phys. Chem. Lett., 2019, 10, 4558–4565 CrossRef CAS
.
- P. A. Unzueta, C. S. Greenwell and G. J. O. Beran, J. Chem. Theory Comput., 2021, 17, 826–840 CrossRef CAS
.
- Z. Chaker, M. Salanne, J.-M. Delaye and T. Charpentier, Phys. Chem. Chem. Phys., 2019, 21, 21709–21725 RSC
.
- Z. Li, B. Zhao, H. Zhang and Y. Zhang, Faraday Discuss., 2024 10.1039/D4FD00128A
.
- C. R. Groom, I. J. Bruno, M. P. Lightfoot and S. C. Ward, Acta Crystallogr., Sect. B: Struct. Sci., Cryst. Eng. Mater., 2016, 72, 171–179 CrossRef CAS
.
- L. J. Mueller, Faraday Discuss., 2024 10.1039/D4FD00114A
.
- G. A. Valdivia-Berroeta, K. Sarpal and N. C. Gonnella, Cryst. Growth Des., 2021, 21, 5904–5913 CrossRef CAS
.
- J. B. Holmes, V. Liu, B. G. Caulkins, E. Hilario, R. K. Ghosh, V. N. Drago, R. P. Young, J. A. Romero, A. D. Gill, P. M. Bogie, J. Paulino, X. Wang, G. Riviere, Y. K. Bosken, J. Struppe, A. Hassan, J. Guidoulianov, B. Perrone, F. Mentink-Vigier, C. A. Chang, J. R. Long, R. J. Hooley, T. C. Mueser, M. F. Dunn and L. J. Mueller, Proc. Natl. Acad. Sci. U. S. A., 2022, 119, e2109235119 CrossRef CAS PubMed
.
- M. Baias, J. N. Dumez, P. H. Svensson, S. Schantz, G. M. Day and L. Emsley, J. Am. Chem. Soc., 2013, 135, 17501–17507 CrossRef CAS
.
- M. Balodis, M. Cordova, A. Hofstetter, G. M. Day and L. Emsley, J. Am. Chem. Soc., 2022, 144, 7215–7223 CrossRef CAS
.
- J. Brus, J. Czernek, L. Kobera, M. Urbanova, S. Abbrent and M. Husak, Cryst. Growth Des., 2016, 16, 7102–7111 CrossRef CAS
.
- M. K. Dudek, P. Paluch and E. Pindelska, Acta Crystallogr. B, 2020, 76, 322–335 CrossRef CAS
.
- F. Bravetti, R. E. Russo, S. Bordignon, A. Gallo, F. Rossi, C. Nervi, R. Gobetto and M. R. Chierotti, Molecules, 2023, 28, 7215–7223 CrossRef
.
- A. Q. Alanazi, D. J. Kubicki, D. Prochowicz, E. A. Alharbi, M. E. F. Bouduban, F. Jahanbakhshi, M. Mladenović, J. V. Milić, F. Giordano and D. Ren,
et al.
, J. Am. Chem. Soc., 2019, 141, 17659–17669 CrossRef CAS
.
- H. Lu, Y. Liu, P. Ahlawat, A. Mishra, W. R. Tress, F. T. Eickemeyer, Y. Yang, F. Fu, Z. Wang, C. E. Avalos, B. I. Carlsen, A. Agarwalla, X. Zhang, X. Li, Y. Zhan, S. M. Zakeeruddin, L. Emsley, U. Rothlisberger, L. Zheng, A. Hagfeldt and M. Grätzel, Science, 2020, 370, eabb8985 CrossRef CAS PubMed
.
- A. Kumar, B. J. Walder, A. Kunhi Mohamed, A. Hofstetter, B. Srinivasan, A. J. Rossini, K. Scrivener, L. Emsley and P. Bowen, J. Phys. Chem. C, 2017, 121, 17188–17196 CrossRef CAS
.
- Y. Kawabata, K. Wada, M. Nakatani, S. Yamada and S. Onoue, Int. J. Pharm., 2011, 420, 1–10 CrossRef CAS
.
- N. J. Babu and A. Nangia, Cryst. Growth Des., 2011, 11, 2662–2679 CrossRef CAS
.
- R. Laitinen, K. Löbmann, C. J. Strachan, H. Grohganz and T. Rades, Int. J. Pharm., 2013, 453, 65–79 CrossRef CAS
.
- L. Yu, Adv. Drug Delivery Rev., 2001, 48, 27–42 CrossRef CAS
.
- J. L. Guest, E. A. E. Bourne, M. A. Screen, M. R. Wilson, T. N. Pham and P. Hodgkinson, Faraday Discuss., 2024 10.1039/D4FD00097H
.
- A. Cavalli, X. Salvatella, C. M. Dobson and M. Vendruscolo, Proc. Natl. Acad. Sci. U. S. A., 2007, 104, 9615 CrossRef CAS
.
- S. M. Santos, J. Rocha and L. Mafra, Cryst. Growth Des., 2013, 13, 2390–2395 CrossRef CAS
.
- U. Sternberg, F.-T. Koch, W. Prieß and R. Witter, Cellulose, 2003, 10, 189–199 CrossRef CAS
.
- I. J. Lowe, Phys. Rev. Lett., 1959, 2, 285–287 CrossRef CAS
.
- E. R. Andrew, A. Bradbury and R. G. Eades, Nature, 1959, 183, 1802–1803 CrossRef CAS
.
- A. Pines, M. G. Gibby and J. S. Waugh, J. Chem. Phys., 1973, 59, 569–590, DOI:10.1063/1.1680061
.
- B. Elena, G. de Paëpe and L. Emsley, Chem. Phys. Lett., 2004, 398, 532–538 CrossRef CAS
.
- P. Caravatti, G. Bodenhausen and R. R. Ernst, Chem. Phys. Lett., 1982, 89, 363–367 CrossRef CAS
.
- P. Caravatti, L. Braunschweiler and R. R. Ernst, Chem. Phys. Lett., 1983, 100, 305–310 CrossRef CAS
.
- A. Lesage, M. Bardet and L. Emsley, J. Am. Chem. Soc., 1999, 121, 10987–10993 CrossRef CAS
.
- S. J. Opella and M. H. Frey, J. Am. Chem. Soc., 1979, 101, 5854–5856 CrossRef CAS
.
- X. L. Wu and K. W. Zilm, J. Magn. Reson., Ser. A, 1993, 102, 205–213 CrossRef CAS
.
- X. L. Wu, S. T. Burns and K. W. Zilm, J. Magn. Reson., Ser. A, 1994, 111, 29–36 Search PubMed
.
- A. Lesage, S. Steuernagel and L. Emsley, J. Am. Chem. Soc., 1998, 120, 7095–7100 Search PubMed
.
- M. Cordova, M. Balodis, B. Simoes de Almeida, M. Ceriotti and L. Emsley, Sci. Adv., 2021, 7, eabk2341 CrossRef CAS PubMed
.
- G. De Paëpe, A. Lesage, S. Steuernagel and L. Emsley, ChemPhysChem, 2004, 5, 869–875 CrossRef PubMed
.
- Q. Z. Ni, E. Daviso, T. V. Can, E. Markhasin, S. K. Jawla, T. M. Swager, R. J. Temkin, J. Herzfeld and R. G. Griffin, Acc. Chem. Res., 2013, 46, 1933–1941 CrossRef CAS PubMed
.
- A. S. Lilly Thankamony, J. J. Wittmann, M. Kaushik and B. Corzilius, Prog. Nucl. Magn. Reson. Spectrosc., 2017, 102–103, 120–195 CrossRef CAS
.
- A. Lesage, M. Lelli, D. Gajan, M. A. Caporini, V. Vitzthum, P. Mieville, J. Alauzun, A. Roussey, C. Thieuleux, A. Mehdi, G. Bodenhausen, C. Coperet and L. Emsley, J. Am. Chem. Soc., 2010, 132, 15459–15461 CrossRef CAS
.
- A. J. Rossini, A. Zagdoun, M. Lelli, A. Lesage, C. Coperet and L. Emsley, Acc. Chem. Res., 2013, 46, 1942–1951 CrossRef CAS
.
- V. Chevelkov, K. Rehbein, A. Diehl and B. Reif, Angew. Chem., Int. Ed., 2006, 45, 3878–3881 CrossRef CAS
.
- A. Daskalov, D. Martinez, V. Coustou, N. El Mammeri, M. Berbon, L. B. Andreas, B. Bardiaux, J. Stanek, A. Noubhani, B. Kauffmann, J. S. Wall, G. Pintacuda, S. J. Saupe, B. Habenstein and A. Loquet, Proc. Natl. Acad. Sci. U. S. A., 2021, 118, e2014085118 CrossRef CAS
.
- K. Jaudzems, A. Kirsteina, T. Schubeis, G. Casano, O. Ouari, J. Bogans, A. Kazaks, K. Tars, A. Lesage and G. Pintacuda, Angew. Chem., Int. Ed., 2021, 60, 12847–12851 CAS
.
- V. Jirasko, A. Lends, N. A. Lakomek, M. L. Fogeron, M. E. Weber, A. A. Malär, S. Penzel, R. Bartenschlager, B. H. Meier and A. Böckmann, Angew. Chem., Int. Ed., 2021, 60, 5339–5347 CAS
.
- M. Schledorn, A. A. Malär, A. Torosyan, S. Penzel, D. Klose, A. Oss, M. L. Org, S. Wang, L. Lecoq, R. Cadalbert, A. Samoson, A. Böckmann and B. H. Meier, ChemBioChem, 2020, 21, 2540–2548 CrossRef CAS PubMed
.
- M. V. Schoenle, Y. Li, M. Yuan, M. W. Clarkson, I. A. Wilson, W. Peti and R. Page, J. Am. Chem. Soc., 2021, 143, 7930–7934 CrossRef CAS PubMed
.
- T. Le Marchand, T. Schubeis, M. Bonaccorsi, P. Paluch, D. Lalli, A. J. Pell, L. B. Andreas, K. Jaudzems, J. Stanek and G. Pintacuda, Chem. Rev., 2022, 122, 9943–10018 CrossRef CAS
.
- Y. Su, L. Andreas and R. G. Griffin, Annu. Rev. Biochem., 2015, 84, 465–497 CrossRef CAS PubMed
.
- S. P. Brown, Solid State Nucl. Magn. Reson., 2012, 41, 1–27 CrossRef CAS
.
- R. W. Dorn, M. J. Ryan, T. H. Kim, T. W. Goh, A. Venkatesh, P. M. Heintz, L. Zhou, W. Y. Huang and A. J. Rossini, Chem. Mater., 2020, 32, 3109–3121 CrossRef CAS
.
-
T. Kobayashi, Y. Nishiyama and M. Pruski, in Modern Methods in Solid-State NMR: A Practitioner’s Guide, ed. P. Hodgkinson, The Royal Society of Chemistry, 2018, pp. 1–38, 10.1039/9781788010467-00001
.
- D. Mance, A. Comas-Vives and C. Coperet, J. Phys. Chem. Lett., 2019, 10, 7898–7904 CrossRef CAS
.
- P. Paluch, N. Potrzebowska, A. M. Ruppert and M. J. Potrzebowski, Solid State Nucl. Magn. Reson., 2017, 84, 111–117 CrossRef CAS PubMed
.
- J. Trebosc, J. W. Wiench, S. Huh, V. S. Y. Lin and M. Pruski, J. Am. Chem. Soc., 2005, 127, 3057–3068 CrossRef CAS
.
- A. Venkatesh, X. Luan, F. A. Perras, I. Hung, W. Huang and A. J. Rossini, Phys. Chem. Chem. Phys., 2020, 22, 20815–20828 RSC
.
- R. Bayzou, J. Trebosc, I. Hung, Z. Gan, O. Lafon and J. P. Amoureux, J. Chem. Phys., 2022, 156, 064202 CAS
.
- D. Carnevale, X. Ji and G. Bodenhausen, J. Chem. Phys., 2017, 147, 184201 CrossRef PubMed
.
- N. T. Duong, F. Rossi, M. Makrinich, A. Goldbourt, M. R. Chierotti, R. Gobetto and Y. Nishiyama, J. Magn. Reson., 2019, 308, 106559 CAS
.
- Y. Ishii and R. Tycko, J. Magn. Reson., 2000, 142, 199–204 CAS
.
- Y. Ishii, N. P. Wickramasinghe and S. Chimon, J. Am. Chem. Soc., 2003, 125, 3438–3439 CrossRef CAS PubMed
.
- Y. Ishii, J. P. Yesinowski and R. Tycko, J. Am. Chem. Soc., 2001, 123, 2921–2922 CrossRef CAS PubMed
.
- K. Mao, T. Kobayashi, J. W. Wiench, H. T. Chen, C. H. Tsai, V. S. Y. Lin and M. Pruski, J. Am. Chem. Soc., 2010, 132, 12452–12457 CrossRef CAS
.
- P. Paluch, A. G. M. Rankin, J. Trebosc, O. Lafon and J. P. Amoureux, Solid State Nucl. Magn. Reson., 2019, 100, 11–25 CrossRef CAS
.
- S. Parthasarathy, Y. Nishiyama and Y. Ishii, Acc. Chem. Res., 2013, 46, 2127–2135 CrossRef CAS PubMed
.
- A. J. Rossini, M. P. Hanrahan and M. Thuo, Phys. Chem. Chem. Phys., 2016, 18, 25284–25295 RSC
.
- A. Venkatesh, A. Lund, L. Rochlitz, R. Jabbour, C. P. Gordon, G. Menzildjian, J. Viger-Gravel, P. Berruyer, D. Gajan, C. Coperet, A. Lesage and A. J. Rossini, J. Am. Chem. Soc., 2020, 142, 18936–18945 CrossRef CAS
.
- N. P. Wickramasinghe, M. Shaibat and Y. Ishii, J. Am. Chem. Soc., 2005, 127, 5796–5797 CrossRef CAS PubMed
.
- P. Berruyer, S. Bjorgvinsdottir, A. Bertarello, G. Stevanato, Y. Rao, G. Karthikeyan, G. Casano, O. Ouari, M. Lelli, C. Reiter, F. Engelke and L. Emsley, J. Phys. Chem. Lett., 2020, 11, 8386–8391 CrossRef CAS
.
- Z. Wang, M. P. Hanrahan, T. Kobayashi, F. A. Perras, Y. Chen, F. Engelke, C. Reiter, A. Purea, A. J. Rossini and M. Pruski, Solid State Nucl. Magn. Reson., 2020, 109, 101685 CrossRef CAS PubMed
.
- S. Bahri, R. Silvers, B. Michael, K. Jaudzems, D. Lalli, G. Casano, O. Ouari, A. Lesage, G. Pintacuda, S. Linse and R. G. Griffin, Proc. Natl. Acad. Sci. U. S. A., 2022, 119, e2114413119 CrossRef CAS
.
- T. Kobayashi, F. A. Perras, U. Chaudhary, I. I. Slowing, W. Huang, A. D. Sadow and M. Pruski, Solid State Nucl. Magn. Reson., 2017, 87, 38–44 CrossRef CAS
.
- J. R. Yarava, S. R. Chaudhari, A. J. Rossini, A. Lesage and L. Emsley, J. Magn. Reson., 2017, 277, 149–153 CrossRef CAS PubMed
.
- P. Berruyer, A. Bertarello, S. Björgvinsdóttir, M. Lelli and L. Emsley, J. Phys. Chem. C, 2022, 126, 7564–7570 CrossRef CAS
.
- I. Hung and Z. H. Gan, J. Phys. Chem. Lett., 2020, 11, 4734–4740 Search PubMed
.
- A. A. Malär, Q. Sun, J. Zehnder, G. Kehr, G. Erker and T. Wiegand, Phys. Chem. Chem. Phys., 2022, 24, 7768–7778, 10.1039/D2CP00616B
.
- L. Rajput, M. Banik, J. R. Yarava, S. Joseph, M. K. Pandey, Y. Nishiyama and G. R. Desiraju, IUCrJ, 2017, 4, 466–475 Search PubMed
.
- I. Schnell, S. P. Brown, H. Y. Low, H. Ishida and H. W. Spiess, J. Am. Chem. Soc., 1998, 120, 11784–11795 CAS
.
- S. P. Brown, I. Schnell, J. D. Brand, K. Mullen and H. W. Spiess, J. Am. Chem. Soc., 1999, 121, 6712–6718 CrossRef CAS
.
- P. J. Sideris, U. G. Nielsen, Z. H. Gan and C. P. Grey, Science, 2008, 321, 113–117 CAS
.
- E. K. Paulson, C. R. Morcombe, V. Gaponenko, B. Dancheck, R. A. Byrd and K. W. Zilm, J. Am. Chem. Soc., 2003, 125, 15831–15836 CAS
.
- I. Schnell and H. W. Spiess, J. Magn. Reson., 2001, 151, 153–227 Search PubMed
.
- H. Geen, J. J. Titman, J. Gottwald and H. W. Spiess, Chem. Phys. Lett., 1994, 227, 79–86 CrossRef CAS
.
- M. M. Maricq and J. S. Waugh, J. Chem. Phys., 1979, 70, 3300–3316 CrossRef CAS
.
- B. S. de Almeida, D. Torodii, P. Moutzouri and L. Emsley, J. Magn. Reson., 2023, 355, 107557 Search PubMed
.
- B. S. de Almeida, P. Moutzouri, G. Stevanato and L. Emsley, J. Chem. Phys., 2021, 155, 084201 Search PubMed
.
- B. C. Gerstein, R. G. Pembleton, R. C. Wilson and L. M. Ryan, J. Chem. Phys., 1977, 66, 361–362 Search PubMed
.
- E. Vinogradov, P. K. Madhu and S. Vega, Chem. Phys. Lett., 1999, 314, 443–450 Search PubMed
.
- D. Sakellariou, A. Lesage, P. Hodgkinson and L. Emsley, Chem. Phys. Lett., 2000, 319, 253–260 CAS
.
- F. M. Paruzzo and L. Emsley, J. Magn. Reson., 2019, 309, 106598 Search PubMed
.
- P. Moutzouri, B. Simoes de Almeida, D. Torodii and L. Emsley, J. Am. Chem. Soc., 2021, 143, 9834–9841 CAS
.
- M. Cordova, P. Moutzouri, B. Simoes de Almeida, D. Torodii and L. Emsley, Angew. Chem., Int. Ed., 2023, 62, e202216607 Search PubMed
.
- P. Moutzouri, M. Cordova, B. Simoes de Almeida, D. Torodii and L. Emsley, Angew. Chem., Int. Ed., 2023, 62, e202301963 CrossRef CAS
.
|
This journal is © The Royal Society of Chemistry 2025 |
Click here to see how this site uses Cookies. View our privacy policy here.