DOI:
10.1039/D4MH01158A
(Review Article)
Mater. Horiz., 2025, Advance Article
DNA nanotechnology-based strategies for minimising hybridisation-dependent off-target effects in oligonucleotide therapies
Received
27th August 2024
, Accepted 5th December 2024
First published on 7th December 2024
Abstract
Targeted therapy has emerged as a transformative breakthrough in modern medicine. Oligonucleotide drugs, such as antisense oligonucleotides (ASOs) and small interfering RNAs (siRNAs), have made significant advancements in targeted therapy. Other oligonucleotide-based therapeutics like clustered regularly interspaced short palindromic repeat (CRISPR)/CRISPR-associated protein (Cas) systems are also leading a revolution in targeted gene therapy. However, hybridisation-dependent off-target effects, arising from imperfect base pairing, remain a significant and growing concern for the clinical translation of oligonucleotide-based therapeutics. These mismatches in base pairing can lead to unintended steric blocking or cleavage events in non-pathological genes, affecting the efficacy and safety of the oligonucleotide drugs. In this review, we examine recent developments in oligonucleotide-based targeted therapeutics, explore the factors influencing sequence-dependent targeting specificity, and discuss the current approaches employed to reduce the off-target side effects. The existing strategies, such as chemical modifications and oligonucleotide length optimisation, often require a trade-off between specificity and binding affinity. To further address the challenge of hybridisation-dependent off-target effects, we discuss DNA nanotechnology-based strategies that leverage the collaborative effects of nucleic acid assembly in the design of oligonucleotide-based therapies. In DNA nanotechnology, collaborative effects refer to the cooperative interactions between individual strands or nanostructures, where multiple bindings result in more stable and specific hybridisation behaviour. By requiring multiple complementary interactions to occur simultaneously, the likelihood of unintended partially complementary binding events in nucleic acid hybridisation should be reduced. And thus, with the aid of collaborative effects, DNA nanotechnology has great promise in achieving both high binding affinity and high specificity to minimise the hybridisation-dependent off-target effects of oligonucleotide-based therapeutics.
Wider impact
Oligonucleotides, which follow the Watson–Crick base-pairing rule, can be used as therapeutic materials to treat a wide range of diseases, from cancer to the “undruggable” diseases. One primary challenge to be addressed is the hybridisation-dependent off-target effects, caused by unintended binding due to thermodynamic overlaps of the correct and mismatched complexes. Mismatches can lead to mutations in nature and off-target side effects in gene therapy. The current off-target mitigation method is a balance between binding affinity and specificity. Over 40 years ago, the concept of DNA nanotechnology was introduced. It takes advantage of the programmability of DNA to collaboratively assemble materials at nanoscale. This self-assembly process is robust, with high precision. The mechanism involved in such hierarchical assembly can be extended to the field of oligonucleotide-based therapeutic materials. In this review, we discuss the sources of hybridization-dependent off-target effects and strategies to mitigate them. Beyond conventional methods, we focus on DNA nanotechnology-derived collaborative effects to reduce these off-target effects. Moreover, DNA nanotechnology enables the functionalisation of multivalent nanostructures with polymers, metals, proteins, and oligonucleotides, creating therapeutic materials with both enhanced targeting affinity and specificity. This advancement will further expand the potential of oligonucleotide materials in targeted gene therapy.
|
1. Introduction
Over the last 20 years, targeted drugs, due to their advantages in efficacy and safety, have been in significantly growing demand for both common and rare diseases. Targeted therapeutics can be simply categorised into two classes: traditional small molecules and macromolecules which include monoclonal antibodies, oligonucleotides, polypeptides, and X-drug conjugates (XDCs).1,2 Although small molecules remain the mainstream drug modality in targeted therapeutics, they face several challenges including drug resistance, low response rate, and limited drug targets.3–5 Recent developments in molecular biology, proteomics, and human genomics have advanced targeted drugs from small molecules to protein-based drugs, and more recently, to a new era of oligonucleotide-based gene therapy.6 Compared to protein-based drugs, gene therapy works by manipulating gene expressions and thus has long-lasting therapeutic or potentially curative effects on a wide range of targets, even including the previously “undruggable” targets.7–9 Gene therapy has great potential in diverse domains of disease treatment, ranging from well-studied applications in cancer to many other genetic diseases and complex acquired disorders, such as infectious diseases, cardiovascular diseases, neurological disorders, and various other rare diseases.10–16
1.1 Oligonucleotide-based targeted therapy
Oligonucleotide-based targeted therapies use short synthetic nucleic acid polymers, including DNA, RNA, and their chemical analogues. They are employed to selectively target and modulate specific pathological RNA or DNA sequences for therapeutic purposes. The field of oligonucleotide therapeutics covers a diverse range of agents, including ASOs, siRNAs, microRNA (miRNA) mimics, plasmid DNAs, small activating RNAs (saRNAs), aptamers, and CRISPR/Cas systems.7,17 Most oligonucleotide therapeutics, as shown in Fig. 1, work by hybridisation to their target sequences via complementary Watson–Crick base pairing. By precisely matching the target sequences, the oligonucleotide therapeutics operate via manipulation of gene expression through a range of mechanisms of action, including gene inhibition, augment, activation, alteration, and replacement.18–20
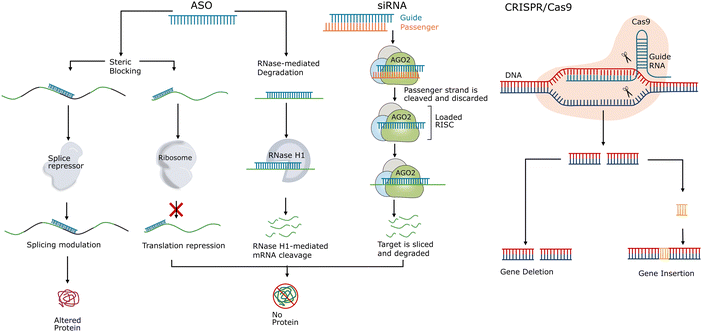 |
| Fig. 1 Molecular mechanisms of the common oligonucleotide-based targeted therapies, including ASO, siRNA, and CRISPR/Cas9. AGO2 refers to argonaute 2. RISC refers to the RNA-induced silencing complex. | |
The majority of oligonucleotide therapeutics function by binding to the target sequence via Watson–Crick base pairing. This straightforward mechanism offers several advantages compared to the conventional treatments. Oligonucleotides are able to target specific genetic sequences with high precision and favourable safety profile.21–23 This allows tailored treatment based on the individual genetic profiles of the patients, providing a versatile platform for personalised medicine.14,24–26 In addition, oligonucleotide therapeutics enable rapid screening of candidate drugs based on knowledge of the sequence of the target gene, and thus oligonucleotide drugs have shorter research and development time spans compared to conventional small-molecule drugs.7,27 Furthermore, oligonucleotide therapeutics target the underlying causes of the diseases and thus may have long-lasting therapeutic effects or even curative effects. Their long-lasting effects also make reduction in dosage or administration frequency feasible, improving patient adherence.28,29 Furthermore, the sequence-specific oligonucleotides do not require complicated conformational recognition and thus are able to offer therapeutic options for the diseases traditionally deemed “undruggable”.18,30–32 These previously undruggable targets lack well-defined binding sites or pockets for small molecule or protein drugs.
1.2 Development of oligonucleotide-based therapeutics
The concept of gene therapy emerged more than half a century ago in the 1960s. The first human gene therapy protocol was not approved until a few decades later in 1990.33,34 Ever since this proof-of-concept trial, gene therapy has experienced its ups and downs, with efficacy and safety being the main concerns.35 In 1998, Vitravene (fomivirsen), a phosphorothioate (PS)-modified ASO developed for the treatment of cytomegalovirus (CMV) retinitis, became the first ASO drug approved by the United States Food and Drug Administration (FDA).36 But in 2006, Vitravene was discontinued in the US because this PS-ASO drug had limited potency and higher risk of inflammatory effects compared to other more recently available treatments.37–39 Much later in 2013, Kynamro (mipomersen sodium), a second generation ASO, was approved by the FDA for the treatment of homozygous familial hypercholesterolemia (HoFH).40,41 However, due to concerns regarding its safety profile and commercial failure, the FDA withdrew Kynamro from the market in 2019.39,42 Without the established technologies of nucleotide modifications and delivery vectors, the reputation of oligonucleotide therapeutics was not optimistic because of the high dosage requirements and low clinical efficacy.7,27
Fortunately, oligonucleotide therapy has captured the attention of the scientific community. Several related studies have been awarded a Nobel Prize, including Andrew Fire's and Craig Mello's research on the process of RNA interference (RNAi) for gene expression regulation by double-stranded RNA,43,44 Emmanuelle Charpentier's and Jennifer Doudna's pioneering work on developing gene-editing technology for CRISPR/Cas9,45,46 and Katalin Karikó's and Drew Weissman's work on nucleoside modifications which were able to prevent unwanted immune responses.47 The modification methods provided the groundwork for the development of effective messenger RNA (mRNA) vaccines against COVID-19. The successful development of SARS-CoV-2 mRNA vaccines contributed another vital piece: lipid nanoparticles (LNPs) which served as the delivery platform for the mRNAs.48–51 Thanks to the development of modification strategies52–54 and the expansion in gene delivery vectors,27,50,55,56 increased binding affinity, better internalisation ability, enhanced stability against nuclease degradation, as well as longer circulation time were achieved.8,11 Thus, the efficacy and safety of gene therapy have been improved, leading a new wave of gene therapy in clinical translation.
In 2016, Spinraza (nusinersen), an ASO drug, was the first drug approved by the FDA for the treatment of spinal muscular atrophy (SMA).57 SMA is a chronic, severe, and rare genetic disorder related to the central nervous system (CNS). Currently, most of the approved oligonucleotide therapeutics are for rare diseases, providing choices for patients with few treatment options.6,8,25 But researchers are also expanding the applications of oligonucleotides beyond rare diseases and are exploring their usage in common chronic diseases, aimed at a larger patient population. In 2021, the FDA approved Leqvio (inclisiran), a first-in-class siRNA conjugated to triantennary N-acetylgalactosamine carbohydrates (GalNAc), to lower cholesterol in cardiovascular disease treatment.58,59 This siRNA therapeutic agent, which only requires two doses annually, signified the advancement of oligonucleotides in common chronic disease treatment.9 As shown in Table 1, by July 2024, 21 oligonucleotide drugs were approved by the FDA, including milasen (not listed in Table 1) which is the first personalised ASO developed for patients with Batten disease.60–64 14 gene therapies including the first CRISPR/Cas9-based gene therapy, Casgevy, have been approved by the FDA to date.65 The future of gene therapy has great potential to revolutionise biomedical therapeutics, ushering a new era of precision medicine.
Table 1 Current oligonucleotide-based targeted therapy approved by the FDAa
Trade name (generic name) |
Sponsor |
Initial approval |
Indications |
Route of administration |
Type |
The information regarding the oligonucleotide drugs, including ASOs, siRNAs, aptamers, and other DNA-based drugs was found in the database of Drugs@FDA: FDA-Approved Drugs.66 The information regarding oligonucleotide-based gene therapy was found on the FDA webpage of Approved Cellular and Gene Therapy Products.65 |
Vitravene (formivirsen sodium) |
Isis Pharmaceuticals |
1998 |
Cytomegalovirus retinitis (CMVR) in AIDS patients |
Intravitreal injection |
ASO |
Kynamro (mipomersen sodium) |
Genzyme |
2013 |
Homozygous familial hypercholesterolemia (HoFH) |
Subcutaneous injection |
ASO |
Exondys 51 (eteplirsen) |
Sarepta Therapeutics |
2016 |
Duchenne muscular dystrophy (DMD) |
Intravenous injection |
ASO |
Spinraza (nusinersen) |
Biogen |
2016 |
Spinal muscular atrophy (SMA) |
Intrathecal injection |
ASO |
Tegsedi (inotersen) |
Akcea Therapeutics |
2018 |
Polyneuropathy of hereditary transthyretin-mediated amyloidosis (hATTR) |
Subcutaneous injection |
ASO |
Vyondys 53 (golodirsen) |
Sarepta Therapeutics |
2019 |
Duchenne muscular dystrophy (DMD) |
Intravenous injection |
ASO |
Viltepso (viltolarsen) |
NS Pharma |
2020 |
Duchenne muscular dystrophy (DMD) |
Intravenous injection |
ASO |
Amondys 45 (casimersen) |
Sarepta Therapeutics |
2021 |
Duchenne muscular dystrophy (DMD) |
Intravenous injection |
ASO |
Qalsody (tofersen) |
Biogen, Ionis Pharmaceuticals |
2023 |
Amyotrophic lateral sclerosis (ALS) |
Intrathecal injection |
ASO |
Wainua (eplontersen) |
AstraZeneca, Ionis |
2023 |
Polyneuropathy of hereditary transthyretin-mediated amyloidosis (hATTR) |
Subcutaneous injection |
siRNA |
Onpattro (patisiran) |
Alnylam |
2018 |
Polyneuropathy of hereditary transthyretin-mediated amyloidosis (hATTR) |
Intravenous injection |
siRNA |
Givlaari (givosiran) |
Alnylam |
2019 |
Acute hepatic porphyria (AHP) |
Subcutaneous injection |
siRNA |
Oxlumo (lumasiran) |
Alnylam |
2020 |
Primary hyperoxaluria type 1 (PH1) |
Subcutaneous injection |
siRNA |
Leqvio (inclisiran) |
Novartis |
2021 |
Heterozygous familial hypercholesterolemia (HeFH) or clinical atherosclerotic cardiovascular disease (ASCVD) |
Subcutaneous injection |
siRNA |
Amvuttra (vutrisiran) |
Alnylam |
2022 |
Polyneuropathy of hereditary transthyretin-mediated amyloidosis (hATTR) |
Subcutaneous injection |
siRNA |
Rivfloza (nedosiran) |
Novo Nordisk |
2023 |
Primary hyperoxaluria type 1 (PH1) |
Subcutaneous injection |
siRNA |
Macugen (pepaptanib sodium) |
Eyetech |
2004 |
Neovaxcular (wet) age-related macular degradation |
Intravitreal injection |
RNA aptamer |
Izervay (avacincaptad pegol) |
Iveric bio |
2023 |
Geographic atrophy (GA) secondary to age-related macular degeneration (AMD) |
Intravitreal injection |
RNA aptamer |
Defitelio (defibrotide sodium) |
Gentium S.r.L. |
2016 |
Hepatic veno-occlusive disease (VOD) following hematopoietic stem-cell transplantation (HSCT) |
Intravenous injection |
ssDNA and dsDNA |
Rytelo (imetelstat) |
Geron |
2024 |
Myelodysplastic syndromes (MDS) |
Intravenous injection |
DNA telomerase inhibitor |
Luxturna (voretigene neparvovec-rzyl) |
Spark Therapeutics |
2017 |
Retinal dystrophy (Biallelic RPE65 mutation-associated) |
Subretinal injection |
Adeno-associated virus (AAV) vector-based gene therapy |
Zolgensma (onasemnogene abeparvovec-xioi) |
Novartis Gene Therapies |
2019 |
Spinal muscular atrophy (SMA) |
Intravenous infusion |
AAV vector-based gene therapy |
Adstiladrin (nadofaragene firadenovec-vncg) |
Ferring Pharmaceuticals |
2022 |
Bacillus Calmette Guérin (BCG), non-muscle invasive bladder cancer (NMIBC) |
Intravesical instillation |
Adenoviral vector-based gene therapy |
Hemgenix (etranacogene dezaparvovec-drlb) |
CSL Behring LLC |
2022 |
Hemophilia B (congenital factor IX deficiency) |
Intravenous infusion |
AAV vector-based gene therapy |
Skysona (elivaldogene autotemcel) |
Bluebird bio |
2022 |
Cerebral adrenoleukodystrophy (CALD) |
Intravenous infusion |
Stem cell-based gene therapy |
Zynteglo (betibeglogene autotemcel) |
Bluebird bio |
2022 |
β-Thalassemia requiring regular red blood cell (RBC) transfusions |
Intravenous infusion |
Stem cell-based gene therapy |
Casgevy (exagamglogene autotemcel (exa-cel)) |
Vertex Pharmaceuticals |
2023 |
Sickle cell disease (SCD) |
Intravenous infusion |
Stem cell-base gene therapy (CRISPR/Cas9) |
Elevidys (delandistrogene moxeparvovec-rokl) |
Sarepta Therapeutics |
2023 |
Duchenne muscular dystrophy (DMD) |
Intravenous infusion |
AAV vector-based gene therapy |
Lyfgenia (lovotibeglogene autotemcel (lovo-cel)) |
Bluebird bio |
2023 |
Sickle cell disease (SCD) and a history of vaso-occlusive events |
Intravenous infusion |
Stem cell-based gene therapy |
Roctavian (valoctocogene roxaparvovec-rvox) |
BioMarin Pharmaceutical |
2023 |
Hemophilia A (congenital factor VIII deficiency) |
Intravenous infusion |
AAV vector-based gene therapy |
Vyjuvek (beremagene geperpavec) |
Krystal Biotech |
2023 |
Dystrophic epidermolysis bullosa |
Topical application |
Herpes-simplex virus type 1 (HSV-1) vector-based gene therapy |
Beqvez (fidanacogene elaparvovec-dzkt) |
Pfizer |
2024 |
Hemophilia B (congenital factor IX deficiency) |
Intravenous infusion |
AAV vector-based gene therapy |
Kebilidi (eladocagene exuparvovec-tneq) |
PTC Therapeutics |
2024 |
Aromatic L amino acid decarboxylase (AADC) deficiency |
Intraputaminal infusion |
AAV vector-based gene therapy |
Lenmeldy (atidarsagene autotemcel) |
Orchard Therapeutics |
2024 |
Pre-symptomatic late infantile (PSLI), pre-symptomatic early juvenile (PSEJ), or early symptomatic early juvenile (ESEJ) metachromatic leukodystrophy (MLD) |
Intravenous infusion |
Stem cell-based gene therapy |
1.3 Current bottlenecks of oligonucleotides in clinical applications
While oligonucleotides offer a versatile platform for targeted gene therapy, this new drug modality still faces several challenges. The issues to be addressed include low stability, immunogenicity, limited cellular uptake, and manufacturing hurdles. Furthermore, the concerns of off-target effects have raised substantial safety concerns and can hinder the clinical translation of oligonucleotide-based drugs.
Oligonucleotides are vulnerable to degradation by nucleases, are prone to activate immune responses, and their cellular uptake can be hindered by their physiochemical properties such as relatively large molecular weight and negative charge.6,8,20 To address these issues, chemical modification technologies were introduced to increase their stability against nuclease degradation,67–69 to reduce the immune responses and cytotoxicity,70–72 to improve cellular uptake,73–75 and to enhance their binding affinity.76–78 Furthermore, a growing number of delivery vehicles were developed to address the issue of inefficient delivery through biological barriers. Both viral-vector-based (adenovirus,79 adeno-associated virus,80 and lentivirus81) and non-viral-based delivery vehicles (polymer-based82 and lipid-based83) were developed. These delivery vectors aim to maximise delivery efficiency despite physiological barriers, to reduce off-target cellular intake, to protect the nucleic acid polymers from nuclease degradation, and to allow efficient endosomal escape. The FDA approved LNP-delivered mRNA vaccines demonstrated a safe and efficient way to address the delivery issue. Additionally, the increasing market demands and the emergence of various modification requirements have posed new manufacturing hurdles, including problems of scalability, sustainability, and purification of stereoisomers.9,84 Last but not least, costs of oligonucleotide gene therapy may limit the accessibility of the treatment to patients. New cost-effect, scalable, and sustainable manufacturing alternatives of both synthesis and purification processes are under development to meet the growing market demand for therapeutic oligonucleotides.85–90
Another significant issue that needs to be addressed in the clinical translation of oligonucleotide drugs is the off-target effects. Similar to all therapeutic modalities, oligonucleotides have the risk of triggering off-target effects. These off-target effects arise from unintended interactions between oligonucleotide drugs and biomolecules in the body and may change drug distribution, alter mechanisms of action, and induce unintended short- and long-term toxicities or side effects. Such effects are also correlated to chemical modifications. In the context of oligonucleotide therapeutics, off-target effects can be subdivided into two categories: hybridisation-independent off-target effects and hybridisation-dependent off-target effects.38,91–93
Hybridisation-independent off-target effects are caused by non-specific interactions with a wide range of biomacromolecules, especially plasma and cellular proteins, and these off-target effects can cause immune activation and protein malfunctioning.94 The unintended interactions usually arise from the PS backbone or nucleotide sequence of the oligonucleotides.95–98 Hybridisation-independent off-target effects are not related to oligonucleotide hybridisation via Watson–Crick base paring. As mentioned above, chemical modifications are often used to enhance the stability of oligonucleotides against nuclease degradation. However, it is important to note that these modifications can have impacts on the binding affinity of oligonucleotides to proteins.94,97,98 An increased binding affinity to proteins may pose a risk of hybridisation-independent off-target toxicities.94,99,100 To reduce such hybridisation-independent off-target toxicities, researchers showed that optimisation of the chemical modifications was required to fine-tune the balance between the efficacy and the binding affinity to cellular proteins.97,101 Other researchers suggested that hybridisation-independent toxicities could be eliminated with in silico approaches at the design stage.100 Additionally, these off-target effects can be dose-dependent. And thus, cooperative strategies were developed to reduce the hybridisation-independent off-target effects by using a combination of lower dose oligonucleotide therapeutics which may regulate the gene expression synergistically.102,103
The hybridisation-dependent off-target effect refers to the unintended hybridisation to partially complementary sequences via Watson–Crick base-paring, followed by RNase H1-mediated degradation, AGO2-mediated cleavage, or transcription inhibition via steric blocking.30,92,96,104,105 Though more observations previously reported focused on hybridisation-independent toxicities, with the development of various nucleic acid modification strategies and diverse cellular delivery vehicles, oligonucleotide therapeutic agents with enhanced potency require increased attention to the clinical safety concerns arising from hybridisation-dependent off-target toxicities.30,38,91,93,106
In the next section, this review will explain in detail the nature of hybridisation-dependent off-target effects of oligonucleotide-based therapeutics, focusing on the relationship between mismatch intolerance, binding affinity, and hybridisation specificity. In Section 3, we will discuss the current strategies to mitigate these off-target effects. These conventional approaches include improvement of specificity using chemical modifications, length optimisation, and computer-aided sequence selection, as these conventional methods often require a trade-off between specificity and binding affinity. In Section 4 of this review, in addition to the conventional methods employed to reduce the hybridisation-dependent off-target effects, we propose using strategies inspired by DNA nanotechnology-derived collaborative effects, which may have both high overall binding affinity and high targeting specificity, to minimise the off-target issues.
2. Hybridisation-dependent off-target effects of oligonucleotide therapeutics
Hybridisation-dependent off-target effects of oligonucleotide therapeutics occur when the drug binds to unintended RNA or DNA sequences which are only partially complementary.107 Such off-target effects have a sequence-dependent manner and are caused by the formation of a mismatched complex due to the limited specificity of the oligonucleotide drugs to the target RNAs or DNAs. These non-specific interactions could influence the stability of mRNAs and the translation of proteins, leading to a series of side effects which exhibit clinical safety risk and liability.102,108 And thus, to mitigate hybridisation-dependent off-target effects of oligonucleotide therapeutics, improved specificity is required to reduce the possibility of mismatches between oligonucleotide drugs and partially complementary sequences.
2.1 DNA mismatch tolerance and hybridisation specificity
Watson–Crick base pairing serves as the basis of DNA and RNA structures and the fundamentals of their functions. This process entails the specific hydrogen bonding between complementary nucleotide bases: guanine (G) with cytosine (C) and adenine (A) with thymine (T) in DNA or with uracil (U) in RNA.109 In addition to hydrogen bonding, several other interactions also contribute to the binding affinity and specificity of oligonucleotides, including stacking interactions, hydrophobic interactions, and geometric complementarity.110 These interactions are crucial for maintaining the accuracy of DNA replication, transcription, and translation processes. DNA mismatch, errors in DNA base paring, can act as precursors to mutations.111–114 Mutations play a substantial role in the natural process of evolution by introducing genetic diversity for natural selection, adaption and speciation. However, mutations can disrupt normal cellular functions and contribute to the onset of diseases such as cancer. And thus, mismatches are also closely associated with disease development.
Mismatches within DNA sequences have a significant influence on the stability and dynamics of DNA/RNA duplex formation.115,116 Mismatches can disrupt the ordered structure and the hydrogen bonding of the double helix, leading to increased entropy and decreased overall stability of the DNA–DNA or DNA–RNA duplex. Moreover, mismatches can disrupt the base stacking interactions between adjacent base pairs and thus further reduce the stability. In addition, the reduced stability of the duplex will be reflected in an altered melting temperature (Tm).117 Typically, each mismatch reduces the Tm of a DNA duplex by 1–5 °C.118 The extent of this reduction depends on the type of the mismatch, the surrounding sequences, and the length of the molecule. Mismatches located in the middle of a DNA sequence have a greater impact on the Tm compared to those at the ends.117 Moreover, due to the cumulative effect of disrupted base pairing and stacking interactions, the presence of multiple mismatches leads to a greater reduction in the Tm than the sum of individual reductions from each mismatch. Furthermore, during the annealing process, mismatches can slow down the formation of a double-stranded duplex. This is because the presence of mismatches results in unstable base pairs which are less likely to form a stable duplex structure and thereby reduce the rate of efficient assembly of the duplex.
Mismatch tolerance also affects the authenticity of the DNA self-replication. Binding affinity and specificity are two key concepts characterising the interactions between the polymerases, an enzyme responsible for DNA synthesis, and nucleotides in this process. The binding affinity of a polymerase refers to the strength of the interactions with the nucleotides and the specificity refers to the selectivity of a polymerase in incorporating the correct nucleotides which are complementary to the template strand. Low fidelity polymerases, which exhibit high mismatch tolerance, have lower binding affinity and specificity. And this allows them to bypass mismatches and continue replication processes. In contrast, high fidelity polymerases, exhibiting high binding affinity and specificity, are responsible for precise replication and may stall when encountering a mismatch.119 Maintaining the delicate balance between binding affinity and specificity is crucial for genome integrity and genetic fidelity, with high fidelity polymerases vital for accurate replication and mismatch tolerance essential for coping with damaged DNA in the sophisticated processes of DNA replication and repair.
In the design of oligonucleotide therapeutics, mismatch tolerance is not desirable as it may lead to hybridisation-dependent off-target effects. Jackson et al. reported that, with the matching of as few as 11 contiguous nucleotides, siRNAs were able to induce off-target silencing of the non-target transcripts which possessed sequence homology to the target sequence.120 In the case of RNase H-mediated ASO gapmer, 12 consecutive matched nucleotides would be sufficient to induce degradation with or without mismatches.91 A later study, using a single-molecule fluorescence method to characterise the kinetic processes of annealing and melting, showed that only 7 contiguous matched nucleotides were required to induce gene silencing.115 It was also reported that 2-base mismatches in the central domain led to complete loss of activity while 2-base mismatches in the 5′ and 3′ ends of the sequence resulted in partial loss of activity.121 In general, the on-target efficacy of siRNAs and RNase H-dependent ASOs is dependent on the location of the mismatches. And the tolerance for the imperfect match, which may lead to off-target degradation of unintended genes, depends on the sequence and chemistry of the oligonucleotides.
High hybridisation specificity is required to reduce mismatch tolerance in order to reduce the hybridisation-dependent off-target effects of oligonucleotide-base drugs. In addition to the reduction of hybridisation-dependent off-target effects, specificity related to therapeutic ASOs and siRNAs also involves allele specificity. The allele specificity of oligonucleotides plays a crucial rule in disease treatment related to the selective degradation of mutated mRNA where the mutated genes need to be knocked down while maintaining the wild-type gene.122–124 In such allele-specific oligonucleotide drugs, mismatch discrimination via identification of single nucleotide polymorphism (SNP) is a fundamental characteristic allowing selective binding to the mutated genes while limiting off-target knockdown of the wild-type genes.
Therefore, understanding the thermodynamics of mismatches related to the hybridisation specificity of oligonucleotides is critical for the applications of oligonucleotides in a variety of biotechnologies, such as in biosensor design with single mismatch discrimination ability and in oligonucleotide drug design to diminish hybridisation-dependent off-target effects. The balance between binding affinity and specificity of oligonucleotide-based drugs must be optimised to ensure the effective on-target activity while minimising the off-target side effects.
2.2 Binding affinity and specificity of oligonucleotides
Affinity and specificity are the two decisive factors for the successful design of oligonucleotides in diagnosis and therapy. Enhanced binding affinity leads to higher potency of the drug. Both small molecule and oligonucleotide therapeutics require high affinity to increase the therapeutic index.91 However, a high binding affinity may increase the possibility of mismatches and result in reduced specificity. Nevertheless, in most cases except for aptamers, there is a negative correlation between sequence-based hybridisation specificity and binding affinity.125,126
Unlike in DNA replication processes where binding is based on steric recognition between the DNA strands and the enzymes, the hybridisation specificity of oligonucleotide drugs has to confront the affinity–specificity dilemma.126 In the case of shape-complementarity recognition in DNA replication processes, primarily due to the absence of local interactions along the contact area, enzymes or nucleic acids can bind to closely-related but mismatched target surfaces and result in significant energy penalties.126 However, in complementary Watson–Crick base-paring, the enhanced binding affinity does not only increase the potency to the intended target but also to the unintended off-target sequences.30
As shown in Fig. 2A, unlike steric fit in shape-complementarity recognition, nucleic acid hybridisation via complementary base paring follows the two-step 1D nucleation-zipping mechanism.126–134 The DNA–DNA, DNA–RNA, or RNA–RNA duplex hybridises through the initial nucleation of a short segment of the strand, which involves only a small number of bases, and this process is followed by realignment and zipping of the remaining strands. The interactions between the two flexible linear strands consist of 4 basic units (A, G, C and T or U) in only 2 types of preferred pairs. And thus a strong zip can be formed while tolerating a single or a few mismatches and only resulting in a relatively small energy penalty in the range of 3–5 kcal mol−1.126 Additionally, the increase in binding affinity does not increase this difference between the free energy of the perfect matched duplex and that of the partially matched duplex significantly enough, and thus the mismatched complex may remain stable even with a higher binding affinity.91
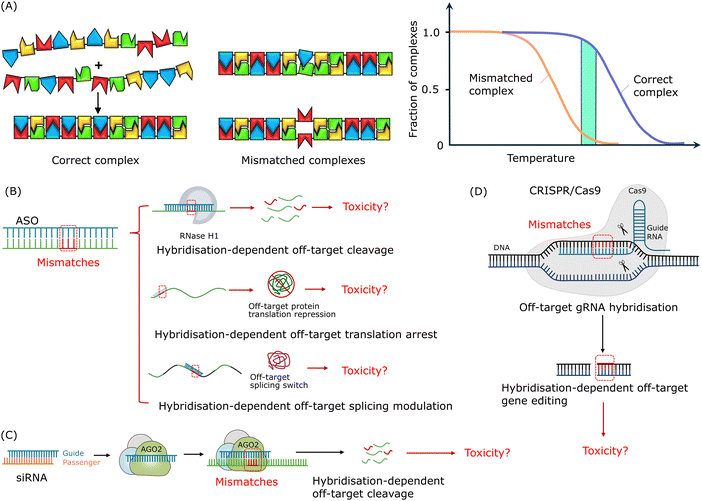 |
| Fig. 2 Mismatch tolerance and hybridisation-dependent off-target effects of oligonucleotide drugs. (A) The formation of a nucleic acid duplex is based on complementary base-paring via a 1D nucleation-zipping mechanism. Reproduced with permission from ref. 126, copyright 2004 Elsevier. (B) Potential hybridisation-dependent off-target effects of ASOs. (C) Potential hybridisation-dependent off-target effects of siRNAs. (D) Potential hybridisation-dependent off-target effects in the CRISPR/Cas9 system. | |
In general, while numerous research studies focus on improving the binding affinity of oligonucleotide drugs, further efforts are required to formulate strategies to enhance the sequence-specificity of oligonucleotide therapeutics to diminish the potential hybridisation-dependent off-target effects. Oligonucleotides with high specificity but low binding affinity may not provide satisfying therapeutic efficacy. Oligonucleotides with high binding affinity but low specificity may lead to unintended off-target activities and potential toxicities. Therefore, a balance between binding affinity and specificity must be optimised in the design of oligonucleotide therapeutics. Strategies such as sequence selection using computational tools and chemical modifications should be employed to enhance the specificity of oligonucleotide therapeutics to improve their efficacy and safety profiles for clinical translations.
2.3 Mismatch induced hybridisation-dependent off-target effects of oligonucleotide therapeutics
As shown in Fig. 2B, depending on the mechanism of action, hybridisation-dependent off-target effects of ASOs may lead to degradation of unintended RNAs, transcription repression, and alteration of splicing events. More than 30 years ago, it was demonstrated using Xenopus oocytes as a model system that ASOs could cause off-target RNase H-mediated degradation of unintended RNAs with partially complementary target sites.135 As mentioned in the introduction section, chemical modifications were introduced to increase the resistance of nucleotides to nuclease degradation as well as to improve the binding affinity to target sequences. And this development in binding affinity raised the awareness of toxicities related to the hybridisation-dependent off-target effects. Locked nucleic acid (LNA)-ASO gapmers, with high binding affinity, were reported to have a higher potency but caused hepatoxicity as a result of unintended off-target RNA cleavage via RNase H1-mediated degradation.106,136–138
As shown in Fig. 2C, hybridisation-dependent off-target effects of siRNAs can lead to downregulation of non-target genes. Researchers reported a case where off-target gene silencing of siRNA against hif-1 alpha was induced by 7-nucleotide (nt) complementarity, and the potency depended on the position of the complementary region and the sequence adjacent to the complementary region.139 It was found that siRNAs could induce miRNA-like off-target effects causing sequence-specific unintended gene silencing via the seed regions of siRNAs.102,140,141 The siRNA seed region commonly consists of about 8 nucleotides at the 5′ end of the siRNA guide strand and targets the 3′ end untranslated region (UTR) of mRNA.142–144 Off-target transcript silencing of targets with limited sequence similarity was observed, and later, it was revealed that this off-target effect was caused by base-pairing mismatches within the siRNA seed region.120,145 Microarray profiling analysis showed that, comparing completely matched targets and seed region-matched targets, the extent of off-target effects depended on the concentration of siRNAs and no off-target effects were detected at the siRNA concentration of 0.05 nM.144 Additionally, Jackson et al. suggested that the on-target activity followed the same dose–response curve as the off-target activity.120,145 Hence, it is not feasible to eliminate these off-target effects by reducing the siRNA concentration as sufficient on-target activity is required for the treatment.
For the CRISPR/Cas9 system, as shown in Fig. 2D, hybridisation-dependent off-target effects usually lead to unintended editing of genes.146 Studies showed that Cas9 protein-mediated off-target cleavage might result in chromosomal losses and hemizygous indels.147,148 Researchers have reported that Cas9 protein tolerated mismatches between sgRNA and DNA sequences in a position-specific and sequence-dependent manner, and this mismatch tolerance lead to off-target cleavage of unintended genes and mutagenesis.149 It was found that up to 5 mismatched base pairs could be tolerated in this case, depending on the location of the mismatches along the sgRNA–DNA duplex and off-target cleavage.
In summary, addressing the issues of hybridisation-dependent off-target effects should be a pivotal consideration in the development of oligonucleotide therapeutics. Limited observations of hybridisation-dependent toxicities in clinical trials are reported, and more systematic studies are required to establish the relationships between the hybridisation-dependent off-target effects and the adverse symptoms of oligonucleotide drugs, including hypotension, fever, asthenia and thrombocytopenia.92 Many efforts have been made to develop approaches to balance between binding affinity and specificity and to reduce the hybridisation-dependent off-target effects. We will review the current strategies employed for hybridisation-dependent off-target effect reduction in Section 3, mainly focusing on conventional methods involving a trade-off between binding affinity and specificity. Furthermore, with the aid of DNA nanotechnology and collaborative effects, both enhanced binding affinity and improved specificity could be achieved. We will review the DNA nanotechnology-based strategies used to mitigate off-targets effects of oligonucleotide-based therapeutics in Section 4.
3. Conventional strategies for hybridisation-dependent off-target effects reduction
It is essential to develop strategies to mitigate the hybridisation-dependent off-target effects of oligonucleotide therapeutics to ensure their efficacy and safety. The specificity needs to be improved to minimise the unintended hybridisation to the non-target RNA or DNA sequences and sufficient binding affinity should be achieved to retain the on-target activity. Researchers have established various approaches to fine-tune the balance between binding affinity and specificity of oligonucleotide drugs. The current strategies developed include sequence length optimisation, chemical modifications, as well as sequence selection using computational tools.
Compared to ASO and siRNA, the CRISPR/Cas system is more complex because of its reliance on the use of programmable Cas nucleases. And thus, in addition to the above-mentioned strategies which focus on the optimisation of the oligonucleotide strands, the mitigation of off-target effects in the CRISPR/Cas system also involves optimisation of delivery methods, optimisation of Cas protein variants and so on.150–158 For example, it was found that the employment of paired Cas9 nickases could reduce the off-target activity of the CRISPR/Cas9 system.159 Researchers designed a small molecule activated Cas9 protein which was able to increase the specificity up to 25 fold compared to wild-type Cas9.160 Scientists also demonstrated that enhanced Streptococcus pyogenes Cas9 (eSpCas9), which was designed to neutralise the positive charges in the non-target strand groove, was able to reduce the off-target cleavage events while maintaining the on-target activity.161
3.1 Improving specificity via chemical modification
Chemical modifications are employed to manipulate the global properties of oligonucleotides and to enhance the pharmacological and therapeutic behaviours. Fig. 3A shows examples of common chemical modifications of DNAs and RNAs, including modifications of their backbone, nucleobase, and sugar. These chemical modification methods are developed to increase nuclease resistance and pharmacokinetics, to improve binding affinity.56,75 And these modifications can provide different levels of target binding affinity for the oligonucleotide drugs.77,162 As mentioned in the previous sections, the risk of off-target binding may increase with improved binding affinity.56 And thus attention must be paid to chemical modifications as the altered binding affinity may induce off-target toxicities.
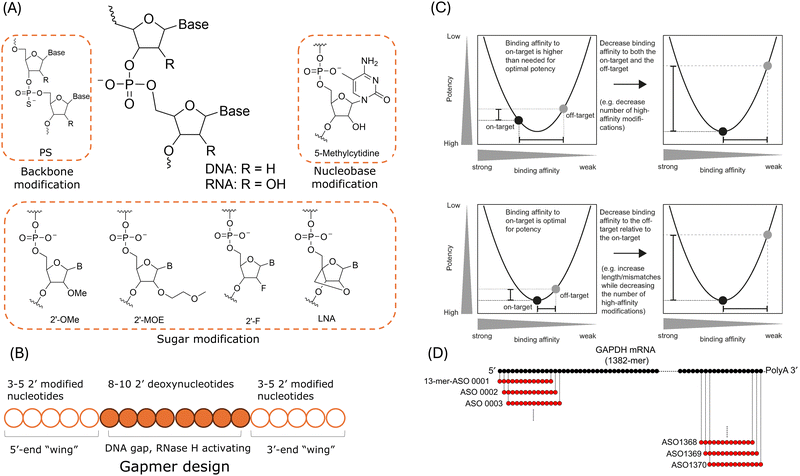 |
| Fig. 3 Current strategies for hybridisation-dependent off-target effects reduction. (A) Common chemical modification methods. (B) Schematic illustration of a gapmer ASO design. (C) The relationship between binding affinity, potency, and specificity of gapmer ASOs. Upper: Specificity optimisation by reducing the number of modifications which might lead to high binding affinity. Lower: Specificity optimisation by gapmer length extension to reduce the off-target binding affinity while maintaining the on-target binding affinity. Reproduced with permissions from ref. 163, copyright 2018 Oxford University Press. (D) An example of in silico search for ASO candidates targeting human GAPH mRNA. Reproduced with permission from ref. 164, copyright 2018 John Wiley and Sons. | |
As shown in Fig. 3B, in addition to the above mentioned modification methods, “gapmer” design is employed in the new generation of RNase H-mediated ASOs.27,77 A gapmer consists of “wings” at both 5′- and 3′-ends of the ASO and a 8- to 10-nt central gap region. The “wings” are 2′-chemically modified flanks designed to improve its stability against nucleases and binding affinity. And the gap region is designed to support RNase H1-mediated cleavage of the target RNA as at least 5 contiguous nts are required for the enzyme degradation.38 As mentioned in Section 2, researchers have demonstrated that LNA gapmers could induce off-target hepatoxicity as a result of RNase H-mediated degradation of unintended transcripts.30,106,137 Typically, the off-target effects are influenced by the binding affinity which governs the amounts of duplex between the ASO and the target RNA and the off-target effects are also affected by how well RNase H handles the structural changes induced by mismatches and bulges in the duplex.163 As suggested by a study of 236 distinctive LNA gapmer ASOs in mice, the hybridisation-dependent toxicity was strongly correlated to the binding affinity.138 In addition, the analysis reported that a melting temperature of 55 °C was the threshold to separate hepatoxic gapmers and non-hepatoxic gapmers. Hence, the off-target effects can be reduced by controlling the binding affinity between the gapmer ASO and the target RNA.101
The off-target effects of siRNAs are derived from mismatch sites of the guide strand or the passenger strand with non-target mRNAs.165 These effects can be reduced by siRNA redundancy,166 siRNA pooling,102 as well as chemical modifications.167 For example, researchers have proved that asymmetric 5′-O-methylation (5′-OMe) modification of siRNA duplexes was able to reduce off-target gene silencing events.168 Studies also suggested that the degree of off-target effect reduction was dependent on the positions of the modifications.169 For example, a position-specific 2′-O-methylation (2′-OMe) modification in the guide strand of siRNA was proved to reduce off-target gene silencing events while maintaining the on-target activity of siRNA.170 Another study reported that unlocked nucleic acid (UNA) modification of siRNAs reduced off-target effects up to more than 10-fold compared to unmodified siRNAs, and the extent of the reduction depended on the modification position in the strands.171,172 Additionally, LNA substitution could also reduce incorrect loading into the RISC when it was applied at the 5′-end of the passenger strand.173 Similarly, position-specific methoxyethyl (MOE) modification of the siRNA scissile site could improve the specificity while enhancing the on-target silencing activity of siRNAs via controlling the oriented RISC loading.165 Furthermore, a combined modification strategy, which involved site-specific MOE modifications of both guide- and passenger-strands of siRNAs, has been proved to be effective in eliminating off-target effects.165 In addition, researchers modified the seed regions of siRNAs via replacement of the RNA in this region by cognate DNA and this modification of siRNAs was able to maintain their gene knockdown activity without any off-target effect observed.174
Various chemical modifications can also be applied in CRISPR/Cas systems to improve the specificity in DNA cleavage.175 For example, a 29-nt synthetic CRISPR-RNA (crRNA) was designed with PS backbone, 2′-fluoro (2′-F), 2′-OMe, and S-constrained ethyl (cEt) modifications, and an obvious reduction in off-target cleavage was observed.176 Implementation of chemical modifications at selected positions in crRNA, including LNA and bridged nucleic acid, led to an evident reduction in off-target cleavage both in vitro and in cells.177 Modified sgRNA with a 2′-hydroxyl group and PS backbone also showed improved specificity while the on-target activity was maintained or even enhanced.178
In summary, various chemical modifications have been demonstrated to be effective in improving hybridisation specificity. And in most cases, the effectiveness of reductions in hybridisation-dependent off-target effects via chemical modifications followed a position-specific manner. When chemical modifications are applied, thorough evaluation is needed to balance the potency, stability, and the risks of off-target effects of the oligonucleotide drugs.
3.2 Improving specificity via sequence length optimisation
The length of oligonucleotide drugs offers sufficient affinity for the unwinding of the target RNA secondary structure and the formation of stable DNA–RNA complexes under physiological conditions.89 Most oligonucleotide drugs contain around 18–30 nucleotides.27,125,166 However, as mentioned above in Section 2, 7 or more contiguous base pairs are adequate to form a stable complex for the following gene silencing.115 And this length is also sufficient for the binding with unintended mRNAs which are partially complementary to the oligonucleotide drugs. And thus, with the relatively short sequence length of oligonucleotide drugs, the specificity can be compromised and lead to hybridisation-dependent off-target effects. In addition, the tolerance for mismatches was lower for shorter ASOs and longer ASOs would have lower specificity.179 On the other hand, as shown in Fig. 3C, besides chemical modification, extension of oligonucleotides may also lower the chance of off-target hybridisation events. With a longer sequence length, though the oligonucleotide drugs may have increased mismatch tolerance, they may also have a lower probability of complementary sequences within the genome. To balance between the on-target activity and the risks of hybridisation-dependent off-target effects, optimisation of sequence length is needed.
A computational study suggested that 21-nt was the optimal length for siRNA and this length provided an optimised balance between high hybridisation specificity and low off-target probability within the genome.180 Researchers reported that, for a CRISPR/Cas9 system, truncating the sgRNA length from 20 nts to 17–18 nts led to reduced hybridisation-dependent off-target effects by at least 5000-fold, while retaining the on-target gene editing efficiency.181 Further truncation of sgRNA to 14–16 nts also showed no detectable off-target effects whilst efficiently modulating the gene expression in Drosophila loci.182 It should be noticed that additional optimisation is required because reduced off-target effects can be accompanied by compromised gene modulation efficiency in some cases. For example, a study of CRISPR-mediated gene knockdown in 293T cells showed that decreased mismatch tolerance and reduced off-target cleavage were achieved when sgRNA was truncated from 20 nts to 17 nts.183 However, compared to the truncated sgRNA, the 20-nt sgRNA had a higher potency. In contrast to sequence length truncation, length extension may also lower the chance of hybridisation with the unintended targets. For instance, a microarray analysis showed that decreased off-target gene expression was achieved by oligonucleotide extension of a 14-mer gapmer ASO to a 18-mer gapmer ASO with the same core region.96 In this case, the binding affinity increased with extension of the sequence length and led to higher mismatch tolerance. However, the reduction of off-target gene expression was achieved because the longer ASO had a lower probability of perfectly complementary candidate genes.
In summary, the length of oligonucleotides can impact their tolerance for mismatches and thus influence their hybridisation specificity. Hence, sequence length optimisation is necessary to balance between the probability of off-target hybridisation within the genome, the specificity, and the on-target activity.
3.3 Computational tools for sequence selection
Computer-aided tools also play a crucial role in mitigating the off-target effects of oligonucleotide drugs by leveraging the predictability of Watson–Crick base-paring. As shown in Fig. 3D, screening of oligonucleotide candidates targeting specific mRNA sequences can be assisted by in silico analysis.164 The predictable Watson–Crick base-pairing enables the exploration of sequence databases to speculate the potential binding sites to non-target sequences and thus to minimise off-target effects by assessment of sequence alignment.91 In addition, certain sequences motifs are more prone to off-target hybridisation.100 And these motifs could be analysed from databases and removed at the early phase of sequence selection. The Oligonucleotide Safety Working Group (OSWG) recommended that, to minimise off-target effects of oligonucleotide drugs, in silico sequence database examination prior to in vitro microarray analysis and in vivo toxicity studies should be applied in the early drug screening phase.91 By integrating computational tools, the design of oligonucleotide drugs can transit from labour-intensive and time-consuming empirical observations to a more cost-effective, systematic, and rational design approach.
Bioinformatic tools are particularly useful in predicting promising lead sequences for oligonucleotides via applying prior knowledge of the target gene. A set of bioinformatic tools for in silico analysis have been developed to assist primary target sequence selection and experimental validation in order to reduce off-target effects.184–190 The probable off-target effects of oligonucleotide drugs can be assessed based on analysis using sequence databases, such as Basic Local Alignment Search Tool (BLAST) and Fast-All (FASTA). For example, researchers have developed a web-based software, AsiDesigner, which supports the design of siRNAs.191 It was based on a scoring scheme which analysed the known key factors in siRNA design and engaged off-target searching with BLAST and FASTA algorithms. BLAST analysis was also employed to evaluate the possible off-target genes of LNA gapmers.136 In addition, libraries of sgRNAs were also established to maximise the on-target activity of the CRISPR/Cas systems and to predict the off-target binding sites based on large-scale empirical data.185
Furthermore, computational tools aid in addressing the complexity associated with chemical modifications of oligonucleotide drugs. For example, the on-target knockdown activity and cytotoxicity of gapmers can be influenced by a range of variables, including the sequence length, the length of the gap region, the sequence motifs in the gap region, and the chemical modifications of nucleotides.93,192 And thus, machine-learning models are desirable to optimise the design of oligonucleotide drugs to balance the on-target activity and off-target toxicity. Platforms and web servers based on machine learning are under development to facilitate the rational design of ASOs.193,194 For example, researchers developed ASOptimizer, a comprehensive platform based on a deep-learning framework.194 This platform combines both sequence selection and chemical modification optimisation, which takes binding to off-target genes and binding affinity into consideration. The authors also showed in vitro experiments on the ASOs recommended by the in silico results on ASOptimizer, targeting indoleamine 2,3-dioxygenase 1 (IDO1) mRNA for potential cancer treatment. By integrating machine learning technology into the design of oligonucleotides, researchers can boost the accuracy and efficiency of sequence selection and modification optimisation, leading to enhanced overall efficacy and safety profiles of the oligonucleotide therapeutics.
In conclusion, in silico analysis and other computational tools enable faster, more efficient, and more rational approaches to minimise the off-target effects. These computer-aided methods can be applied in the sequence selection processes and in the optimisation phases to balance the binding affinity and specificity. Further reduction in the off-target effects can be facilitated by conducting additional in vitro and in vivo validation experiments to confirm the impacts of off-target hybridisation. The combination of in silico, in vitro, and in vivo methods has the potential to mitigate the risks of off-target effects associated with oligonucleotide drugs, enhancing the safety and efficacy of this new therapeutic modality.
4. DNA nanotechnology for oligonucleotide-based targeted therapy with improved specificity
4.1 DNA nanotechnology
4.1.1 Introduction of DNA nanotechnology. DNA is not merely a genetic information carrier but also an ideal material for constructing structured materials and devices with nanoscale precision. In the early 1980s, Nadrian Seeman proposed the concept of fabricating structured nanomaterials via self-assembly of DNA molecules.195 Later in 2006, Paul Rothemund introduced the concept of DNA origami which enables the assembly of tailored DNA nanomaterials and further advancement in DNA nanotechnology.196 The characteristic properties of DNA nanostructure, such as its programmability, structural versatility, biocompatibility, and capability to respond to environmental stimuli, make it a promising platform for nanodevice construction and applications in theranostics fields, such as biosensors,197–199 precise drug delivery,200–202 and targeted gene therapy.202–204 Moreover, given the chemical similarity between DNA and RNA, the principles of DNA nanotechnology are applicable not only to DNA-based therapeutic agents but also to RNA-based therapeutics, as both benefit from the predictable and programmable Watson–Crick base pairing.
4.1.2 Applications of DNA nanostructures in drug delivery. DNA nanostructures, which are precisely assembled with specific sizes and shapes, can be programmed to deliver payloads to specific tissues or cells.205–207 Nanostructures such as nanocages and DNA boxes exhibit enhanced resistance to nuclease degradation and cellular uptake.208–210 In addition, their modifiable surfaces enable sequence-response site-specific delivery. As shown in Fig. 4A, Anderson et al. designed a 3D DNA box structure with a lid opened by strand displacement with a specific oligonucleotide with no significant response to unrelated oligonucleotides.211 This DNA box possessed the potential for the controlled release of payloads. As shown in Fig. 4B, researchers examined biodistribution of radiolabelled DNA origami nanostructures (DONs) with various shapes in the kidneys of mice and showed rectangular DONs had lower off-target potential in acute kidney injury treatment.212 Fig. 4C showed that framework nucleic acids with programmable sizes worked as a promising platform for the transdermal drug delivery because the skin penetration capacity was size-dependent.213 These DNA frameworks can also be applied in cancer treatment. For example, as shown in Fig. 4D, the triangle-shaped DNA frameworks showed their ability in tumour targeting and long-lasting controlled release of the loaded drugs.200 In addition, as shown in Fig. 4E, DNA nanostructures also enabled real-time in situ detection of gene expressions in living cells.214 The DNA tetrahedron structure provided protection for molecular beacons from nuclease-degradation and enabled delivery of the nano-sensor into the target cells.
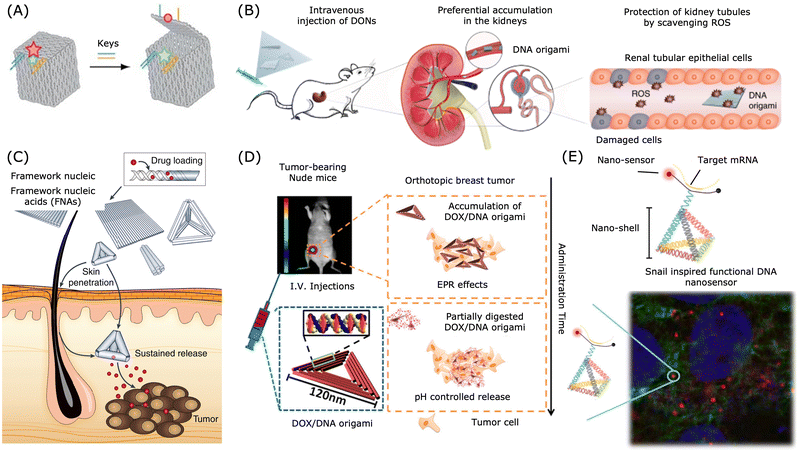 |
| Fig. 4 Applications of DNA nanostructures in drug delivery. (A) A DNA box with a programmable lid for controlled release. Reproduced from ref. 211, copyright 2009 Spring Nature. (B) Preferential accumulation of DNA origami nanostructures in the kidneys of mice. Reproduced from ref. 212, copyright 2018 Spring Nature. (C) DNA nanostructures for transdermal drug delivery. Reproduced from ref. 213, copyright 2019 Spring Nature. (D) Breast tumour treatment using drug-loaded DNA origami in a nude mice model. Reproduced from ref. 200, copyright 2014 American Chemical Society. (E) Snail inspired functional DNA biosensor. Reproduced from ref. 214, copyright 2015 American Chemical Society. | |
4.1.3 Moving from 1D to 3D DNA nanostructures. In hierarchal assembly, branched DNA motifs and sticky ends are required to realise more complexed structures (Fig. 5A).195,215 DNA motifs are stringently designed to minimise symmetry to reduce the chances of unintended Watson–Crick associations and to promote the desired conformation for the assemblies. Sticky end cohesion is a key mechanism employed in DNA nanostructure construction to promote the assembly of complex structures with high precision.216,217 In addition to the commonly used sticky end cohesion self-assembly mechanism, geometry also provides promising potential as a strategy to program DNA nanostructure self-assembly with high specificity and binding affinity.216,218–220 In general, both sequence-based and geometry-based hierarchical assembly mechanisms can facilitate the precise assembly of DNA into one-, two-, and three-dimensional (1D, 2D, and 3D) complex nanostructures.215,221–227
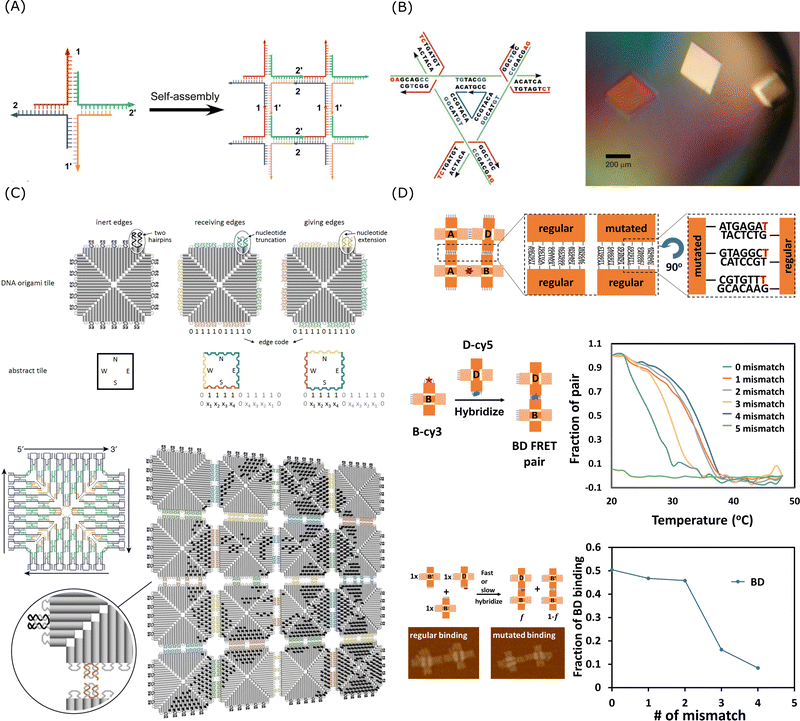 |
| Fig. 5 Sticky end cohesion and hierarchical assembly of DNA. (A) Self-assembly of branched DNA via sticky end cohesion. Reproduced from ref. 228, copyright 2009 ACS Publications. (B) Schematic design of the DNA tensegrity triangle and optical image of the 3D DNA crystals. Reproduced from ref. 223, copyright 2009 Springer Nature. (C) Fractal assembly of DNA origami arrays and the example pattern of Mona Lisa formed by this method. Reproduced from ref. 229, copyright 2017 Springer Nature. (D) Schematic design and the effect of mismatch for mutated self-assembly processes. Reproduced from ref. 230, copyright 2021 National Academy of Sciences. | |
Additionally, the principles governing hierarchical DNA assembly have been successfully extended to RNA-based systems. For example, researchers have designed various RNA origami which are nanostructures folded from a single continuous RNA strand.231,232 Similarly, DNA and RNA strands can assemble into hybrid nanostructures. Researchers have designed RNA–DNA hybrid fibres and polygons for theranostics applications.233 Furthermore, a hybrid nucleic acid origami was introduced, using RNA as the scaffold strand and DNA as staples.234
As shown in Fig. 5B, Seeman's group reported a crystal structure at 4 Å resolution and this engineered 3D DNA crystal with the dimensions of 250 micrometres was self-assembled via 2-nt sticky end cohesion of branched DNA tensegrity triangles.223 Though the binding affinity of a single 2-nt sticky end might be weak, through the cooperative interactions of multiple 2-nt sticky ends, a stable and highly specific arrangement of DNA molecules can be achieved in a confined periodic array. Qian's group demonstrated that multistage hierarchical assembly of simple DNA tiles with edge codes could enable scalable and programmable fabrication of complicated patterning using a simple algorithm.229 As shown in Fig. 5C, the multiple interactions of 2-nt sticky ends and stacking bonds cooperatively formed unique edge codes for each array and the edge codes made the DNA tiles addressable for the fractal assembly. As the self-assembly was a dynamic process with on- and off-motions of the strands, the short staples, compared to long DNA strands, were able to provide sufficiently high specificity for preferred interactions while their binding energy was weak enough for re-arrangement to happen in order to prevent being kinetically trapped in off-target configurations. As shown in Fig. 5D, using an artificial system of cross-tile DNA origami dimers, Chaikin's group showed that the self-replicating rate could be controlled by the introduction of mismatches in the sticky end of the tiles as mutations.230 The thermodynamic properties such as melting temperature of the system would change in the presence of mismatch and thus influence the hybridisation selectivity.
4.2 Collaborative effects in DNA nanotechnology
Collaborative effects in DNA nanotechnology refer to the synergetic interactions that occur between individual DNA strands or nanostructures. These effects arise when the hybridisation behaviour of one DNA strand is influenced by the presence and proximity of other strands or structures. This collaborative behaviour offers several key benefits for oligonucleotide therapeutic design, including enhanced overall binding affinity, increased local concentration of targeting ligands, possibility of conditional activation, and multivalent targeting capabilities. Collaborative effects involving Watson–Crick base-pairing are employed in hierarchical assembly and in dynamic DNA nanotechnology to improve both the binding affinity and specificity, including in the fields of 3D DNA crystals,235 spherical nucleic acid (SNA),236 colloidal crystal engineering with DNA,237 cooperative hybridisation,238 and more. Similar to the collaborative effects observed in DNA nanotechnology, cooperative self-assembly represents a well-established mechanism for enhancing targeting specificity in natural biological processes, notably in gene regulation.239–242 For example, in the gene regulatory network mediated by the binding of zinc-finger-based transcription factors to the target gene, cooperative assembly with weak multivalent interactions showed enhanced specificity over the lock-and-key mode with strong binary interactions.243 And this cooperative assembly strategy could be applied to mitigate gene misregulation caused by off-target binding.
Flexible linear oligonucleotides are able to bypass mismatches in base paring because a single mismatch or a few mismatches exhibit relatively small energy penalties. 1D recognition via complementary base pairing would tolerate mismatches to some degree and thus reduce the target specificity. As mentioned in the previous section, sticky end cohesion allows precise self-assembly of higher-ordered DNA nanostructures. Cooperative interactions guarantee that only perfectly complementary sequences bind together by minimising the probability of mismatches in the assembly process. In addition to sequential complementarity, Zhang et al. reported successful geometry-controlled self-assembly of 2D DNA arrays and 3D DNA crystals with the same pair of sticky-end sequences with optimised lengths of motif branches, and self-sorting phase behaviour based on hairpin structure density was also observed.219 Furthermore, Zhang and Wei proposed a highly specific DNA sticky-end recognition strategy using 1-nt sticky ends of a fixed sequence for precise DNA self-assembly based on configurational recognition and successfully generated 2D tessellation and 3D crystallisation based on this strategy.220 In general, moving from the linear molecular structure to a higher-order nanostructure, both sticky end hybridisation and geometry configuration can be involved in mismatch tolerance reduction and hence able to encode DNA patterning with high specificity and make precise assembly of the programmable DNA nanostructures feasible.244
In summary, collaborative effects are driven by cooperative interactions between multiple adjacent nucleotides or strands, leading to a greater reduction in the free energy. The strength of the interactions depends on DNA length, density, complementary fraction, and geometrical configuration.245 These interactions play a crucial role in enhancing the stability, binding affinity, and specificity in the DNA self-assembly process and also enable the design of precise and programmable polyvalent structures with multiple binding sites which can target multiple targets simultaneously. Alongside the hydrogen bonds and van der Waals forces, configuration-specific interactions involving the conformational changes of DNA structures also play a part in collaborative effects.246
4.3 Improved specificity via collaborative effects in DNA nanotechnology
4.3.1 Collaborative effects in spherical nucleic acids. DNA-coated particles, such as spherical nucleic acids and DNA-coated colloidal systems, leverage the collaborative effects of complementary DNA strands to reduce mismatch tolerance. As shown in Fig. 6A, compared to the unhybridised state, the freedom of motion is reduced when the sticky ends are hybridised. The initial binding event can increase the stability of the overall complex and thus make the subsequent binding event easier. In the DNA-coated colloidal system, researchers showed that the balance between hybridisation behaviour and steric repulsion played a crucial role in the control of melting temperature which was an important macroscopic property.245 The study also showed that the hybridisation behaviour was determined by the sequence length, nucleotide composition, and the number of DNA sticky ends. And the steric repulsion was impacted by the polymer layers.
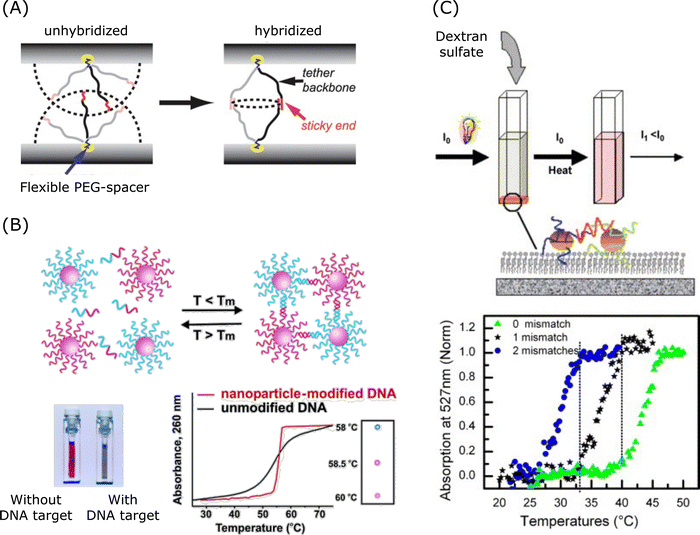 |
| Fig. 6 Collaborative effect and its application in spherical nucleic acids. (A) Mechanism of collaborative effect in the spherical nucleic acid hybridisation process. Reproduced from ref. 247, copyright 2010 American Chemical Society. (B) Aggregation of nanoparticle-labelled DNA. Reproduced from ref. 248, copyright 2005 American Chemical Society. (C) DNA mismatch detection via 2D aggregation and desorption of DNA-coated gold nanoparticles. Reproduced from ref. 249, copyright 2007 Elsevier. | |
Mismatch discrimination, especially the ability to discriminate SNPs, plays a crucial role in both disease diagnosis and targeted gene therapy. DNA-coated nanostructures have a very sharp phase transition for temperature-based mismatch discrimination.250,251 As shown in Fig. 6B and C, DNA-modified gold nanoparticles showed sharp melting transitions, which is useful for DNA detection.248,249 Researchers revealed that sharp melting in the hybrids is attributed to neighbouring-duplex interactions.250 And the study proposed a thermodynamic model which quantitatively assessed these effects, and this model showed that cooperative melting arose from neighbouring-duplex interactions while enhanced stabilisation in aggregates was the result of both neighbouring-duplex interactions and multivalent properties. Researchers also reported a pH-responsive DNA circuitry based on SNA possessing high specificity and it was able to discriminate single single-base mismatch at different positions.252 In addition, DNA-AuNPs were designed to enhance the binding affinity and to amplify the signal via paramagnetic beads for colorimetric assays detecting Kirsten rat sarcoma viral oncogene homologue (KRAS) gene mutation.253 A number of DNA-AuNPs designed for single mismatch discrimination could be used under mild salt conditions and under room temperature conditions, and the design was facilitated by the cooperative interactions of oligonucleotides to enhance the binding affinities and to enable sharp melting transitions.238,253–255
4.3.2 Collaborative effects in dual-targeting strategies. The dual-targeting strategy, which involves simultaneous engagement of two distinct molecular targets, has emerged as a promising approach in therapeutic design. By leveraging collaborative effects, this strategy enhances the targeting specificity and therapeutic efficacy, addressing concerns such as off-target effects and related challenges. In this approach, the therapeutic molecule is designed to recognise two distinct targets, functioning as a molecular AND gate. By requiring dual recognition, the selectivity is improved, and the off-target effects can be minimised. Additionally, a dual-targeting approach can also enhance the overall binding strength and thermodynamic stability. In addition, the concept of dual-targeting strategies has also been broadly applied in the design of various therapeutic modalities, including, but not limited to, bispecific antibodies (BsAbs)256–259 and bispecific aptamer chimeras.260–262 The dual-targeting design may enhance the therapeutic efficacy by disrupting signal cascades or harnessing synergistic effects.263 Furthermore, by targeting multiple molecular pathways simultaneously, the drug resistance regarding mutation may also be reduced.264In the design of oligonucleotide-based therapeutics, the molecule can be engineered to target a pathological gene and a disease-specific biomarker. As shown in Fig. 7A, a marker-activated binary ASO design was proposed to increase the target selectivity based on this concept of dual-targeting.265 This binary ASO combined ASO a which recognised a pathological target mRNA and ASO b which recognised a marker sequence over-expressed by the disease-related cells. In this design, the sensing strands were chemically modified to be protected from undesired cleavage. These two strands were brought into proximity and formed a stable four-way junction upon recognition of the target mRNA and the cancer marker sequence, and then the target mRNA could be cleaved by RNase H.
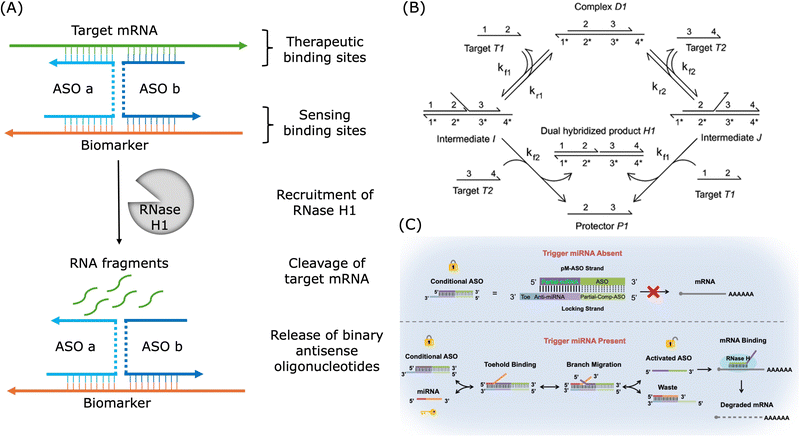 |
| Fig. 7 Examples of applications of dual-targeting strategies in the design of therapeutic oligonucleotides. (A) An example of binary ASO activated by the cancer biomarker. Redrawn from ref. 265. (B) Mechanism of cooperative hybridisation of oligonucleotides. Reproduced from ref. 238, copyright 2010 American Chemical Society. (C) An example of ASO activated by miRNA via toehold exchange. Reproduced from ref. 266, copyright 2021 American Chemical Society. | |
As shown in Fig. 7B, cooperative hybridisation in strand displacement is a mechanism in which two independent target oligonucleotides with different sequences can cooperatively and simultaneously hybridise to a two-stranded DNA complex in a stoichiometric and parallel manner.238 Similar first binding events increase the thermodynamic favourability of the subsequent binding event and thus a cooperative hybridisation mechanism demonstrated improved robustness and specificity over typical nucleic acid hybridisation.238,267 This mechanism enables the creation of networks for gene expression detection and offers practical advantages, such as resilience to impurities and interference from unrelated nucleic acids, which makes it a valuable tool in engineering dynamic DNA nanotechnological devices.238,268 In SNP detection, a target strand with a single mutation has to be discriminated from the wild-type gene without the mutation. For example, a dual-toehold and bulge-loop (DT&BL) probe with a strand displacement catalytic (SDC) cycle was designed to detect the SNP of three genes related to cancer.269 In this study, cooperative hybridisation was employed to facilitate single mismatch discrimination and the secondary structure, bulge loops, was used to adjust the signals. Binary probes, which involve two parts of the probe hybridising cooperatively, are well-established approaches for highly selective nucleic acid analysis.270 For example, researchers established a highly specific dual-recognition toehold-mediated strand displacement method, combining cooperative hybridisation and sequestering agent, for KRAS mutation detection.267 In addition, a highly specific siRNA was designed with an extra hairpin probe and this siRNA was only active upon hybridisation between the hairpin part and a trigger RNA.271 If binary probes are not employed, suitable conditions would be required where a perfectly matched duplex could be formed and a mismatched complex was dissociated. Low ionic strength, denaturing agents, or increased temperatures were usually required to destabilise the duplex.270 And such conditions require massive amounts of screening and might be unique for individual sequences. Moreover, these conditions may not be suitable for in vivo applications. In addition to the field of theranostics, cooperative hybridisation is also employed in allosteric DNA toehold strand displacement for applications in computational logic gate design and DNA data storage.272–274 Though toehold exchange reaction based on cooperative hybridisation is often employed in the design of probes for mismatch discrimination, it can also be applied in conditionally activated oligonucleotide drugs. As shown in Fig. 7C, it was demonstrated that conditional HIF1α ASO activation was feasible by a hepatocyte-specific miR-122 miRNA via toehold exchange and the specificity of the ASO was enhanced.266 This study demonstrated that cooperative hybridisation could offer programmable and highly specific gene regulation for therapeutic applications by enhancing the specificity and minimising the off-target events.
4.3.3 Collaborative effects in multivalent DNA nanostructures for targeted therapy. Polyvalent DNA nanostructures with multiple binding sites and functional groups are able to engage with different targets simultaneously. For example, a multivalent branched DNA nanostructure was designed to bind to three various target miRNAs simultaneously and it showed enhanced synergetic downregulation of FOXO1a expression.275 Following a similar manner to collaborative effects in spherical nucleic acids or cooperative hybridisation in strand displacement, the cooperative binding in multivalent nanostructures can enhance the sequence specificity. In addition, multivalent nanostructures enable highly precise recognition and regulation through multiple parameters. Secondary structures such as aptamers can be introduced to increase the energetic difference between perfect-match and mismatch events in binding.276–278 With the incorporation of pattern-recognition ligands in the designed structure, such as antibodies and aptamers, the targeting specificity of the nanostructures would be improved for the applications in targeted therapy.As shown in Fig. 8A, the multivalent DNA nanostructure showed potent interactions with its target through pattern recognition.279 Based on this mechanism, an antiviral platform of star-shaped DNA nanostructure which could geometrically recognise the antigens on viral particle surfaces was proposed.279,280 And this designer DNA nanostructure with a polyvalent spatial-matching pattern demonstrated improved binding affinity compared to structures with monomeric aptamers. As shown in Fig. 8B, Bathe's group designed a DNA-based virus-like particle (DNA-VLP), which was a 34-nm DNA origami with 30 copies of B cell receptors.281 This multivalent DNA nanostructure exhibited enhanced antigen targeting ability and improved immunogenicity, and thus it could work as an alternative nanoparticulate vaccine. Ding's group proposed a multivalent DNA origami for targeted antimicrobial therapies.282 In this study, by organizing DNAzymes, Levofloxacin, and DNA aptamers on DNA origami, a custom-made nanoplatform was created for combined antibacterial therapy, targeting bacterial membranes and peptidoglycans to treat infectious diseases effectively. Moreover, multivalent nanostructures based on SNAs embedded with aptamers could also provide high specificity for cancer therapy.283 As shown in Fig. 8D, Ding's group designed a branched DNA-based nanostructure, combined with aptamer and peptide which improve cellular uptake and endosomal escape, for the delivery of ASO and CRISPR/Cas9 to silence the tumour-associated gene, PLK1.284 Additionally, DNA-based nanorobots with logic gates were designed to respond to environmental stimuli for controlled delivery and release of the payloads. As shown in Fig. 8C, a robust DNA nanorobot was designed to deliver the payload in response to cell-surface biomarkers and its targeting specificity was enhanced by encoding aptamer-based logic gates in this DNA nanostructure.285 As shown in Fig. 8E, a thrombin-loaded DNA nanorobot was designed for targeted cancer treatment.286 This nanorobot was functionalised with aptamers on the outside and was designed to respond to the trigger of nucleolin, a tumour-associated biomarker.
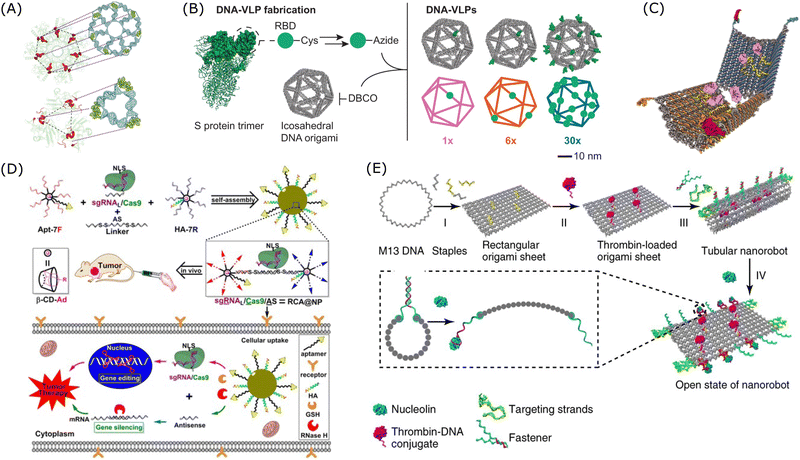 |
| Fig. 8 Examples of applications of multivalent DNA nanostructures in targeted therapy. (A) DNA-based nanostructure with a pattern-recognition property. Reproduced from ref. 279, copyright 2019 Spring Nature. (B) Design of DNA-based virus-like particles. Reproduced from ref. 281, copyright 2024 Spring Nature. (C) Design of a DNA nanorobot with an aptamer-based logic gate and protein payload. Reproduced from ref. 285, copyright 2012 American Association for the Advancement of Science. (D) Design of a multivalent DNA-based delivery platform for a sgRNA/Cas9/antisense complex. Reproduced from ref. 284, copyright 2019 American Chemical Society. (E) Design of a multifunctional DNA nanorobot functionalised with thrombin. Reproduced from ref. 286, copyright 2018 Spring Nature. | |
5. Concluding remarks and future perspectives
DNA is not only a material that stores genetic information but also a promising therapeutic material. Hybridisation-dependent off-target effects are some of the unresolved challenges for the clinical translation of oligonucleotide-based drugs. In this review, we have summarised the causes of such off-target effects and the strategies to mitigate the problems. Conventional strategies, such as chemical modifications and sequence length optimisation, are methods engaging trade-off between binding affinity and specificity. With the development of DNA nanotechnology, both high binding affinity and high specificity may be achieved with the aid of collaborative effects. Collaborative effects play a significant role in the assembly of high-ordered nanostructures, including DNA tiles, DNA tetrahedron, DNA origami, spherical nucleic acid, virus-like framework molecular machines, and so on. Using DNA nanotechnology with the predictability and programmability of Watson–Crick base-pairing, researchers are able to develop precise nanostructures from 1D to 3D with tailored shapes, sizes, and mechanical properties.287 The programmable structural reconfiguration and mobility enabled by DNA nanotechnology could allow top-down manipulations of the target materials, such as surgical robots in nanoscale for gene therapy. In addition, these nucleic acid based nanostructures allow precise control over the spatial arrangement of the functional molecules with nanoscale precision and thus allow tailorable functionalities.287 Hence, DNA nanotechnology can facilitate functionalisation with a wide range of materials to generate artificial enzymes or organelles for multi-functional targeting. These multivalent DNA-based or RNA-based nanostructures with improved targeting affinity and specificity can work as the new generation of oligonucleotide-based therapeutics, expanding the field of gene therapy.
Computer-aided tools have also been employed to reduce the off-target effects of oligonucleotides. Models and algorithms based on databases are used for sequence design. And machine-learning based models can be used for high-throughput screening of oligonucleotide candidates with less off-target effects. Moreover, a patient-specific model may be built to advance personalised medicine. With further advancement in artificial intelligence (AI), we believe that more computational chemistry aided drug design and computationally driven drug discovery will be realised. In recent years, the development of artificial intelligence for molecular folding prediction, such as the AlphaFold database, has already shown its power in understanding the function and evolution of proteins.288–291 Such molecular folding prediction is also essential in oligonucleotide drugs, especially for aptamers and multivalent nanostructures with geometrical recognition requirements. In addition, AI tools may ease the analysis of collaborative effects in DNA nanostructures and aid the rational design of multivalent DNA frameworks with both high affinity and specificity.
In conclusion, with the development of DNA nanotechnology, oligonucleotide-based therapeutics with high binding affinity and specificity can be developed in a more efficient and rational manner. Over the next decade, with the current unresolved challenges addressed, oligonucleotide-based targeted drugs will play a vital role in oncology, cardio, neurodegenerative diseases, and various genetic disorders, offering more precise and effective treatment options for patients.
Data availability
No primary research results, software or code have been included and no new data were generated or analysed as part of this review.
Conflicts of interest
There are no conflicts to declare.
Acknowledgements
This work was supported by the National Key R&D Program of China (2023YFC2706100), the National Natural Science Foundation of China (22307124, 82402141, and U24A20377), the China Postdoctoral Science Foundation (GZB20230788), and the Key Research and Development Project in Ningbo (2024Z186).
References
- K. Trajanoska, C. Bhérer, D. Taliun, S. Zhou, J. B. Richards and V. Mooser, Nature, 2023, 620, 737–745 CrossRef CAS PubMed.
- Y. T. Lee, Y. J. Tan and C. E. Oon, Eur. J. Pharmacol., 2018, 834, 188–196 CrossRef CAS PubMed.
- L. Zhong, Y. Li, L. Xiong, W. Wang, M. Wu, T. Yuan, W. Yang, C. Tian, Z. Miao, T. Wang and S. Yang, Signal Transduction Targeted Ther., 2021, 6, 1732–1779 Search PubMed.
- M. J. Henley and A. N. Koehler, Nat. Rev. Drug Discovery, 2021, 20, 669–688 CrossRef CAS PubMed.
- X. Xie, T. Yu, X. Li, N. Zhang, L. J. Foster, C. Peng, W. Huang and G. He, Signal Transduction Targeted Ther., 2023, 8, 335 CrossRef PubMed.
- E. Tambuyzer, B. Vandendriessche, C. P. Austin, P. J. Brooks, K. Larsson, K. I. Miller Needleman, J. Valentine, K. Davies, S. C. Groft, R. Preti, T. I. Oprea and M. Prunotto, Nat. Rev. Drug Discovery, 2020, 19, 93–111 CrossRef CAS PubMed.
- A. Khvorova and J. K. Watts, Nat. Biotechnol., 2017, 35, 238–248 CrossRef CAS PubMed.
- J. A. Kulkarni, D. Witzigmann, S. B. Thomson, S. Chen, B. R. Leavitt, P. R. Cullis and R. van der Meel, Nat. Nanotechnol., 2021, 16, 630–643 CrossRef CAS PubMed.
- R. Obexer, M. Nassir, E. R. Moody, P. S. Baran and S. L. Lovelock, Science, 2024, 384, eadl4015 CrossRef CAS PubMed.
- O. Khorkova and C. Wahlestedt, Nat. Biotechnol., 2017, 35, 249–263 CrossRef CAS PubMed.
- C. E. Dunbar, K. A. High, J. K. Joung, D. B. Kohn, K. Ozawa and M. Sadelain, Science, 2018, 359, eaan4672 CrossRef PubMed.
- C. Rinaldi and M. J. A. Wood, Nat. Rev. Neurol., 2018, 14, 9–21 CrossRef CAS PubMed.
- X. M. Anguela and K. A. High, Annu. Rev. Med., 2019, 70, 273–288 CrossRef CAS PubMed.
- J. Kim, C. Hu, C. M. E. Achkar, L. E. Black, J. Douville, A. Larson, M. K. Pendergast, S. F. Goldkind, E. A. Lee, A. Kuniholm, A. Soucy, J. Vaze, N. R. Belur, K. Fredriksen, I. Stojkovska, A. Tsytsykova, M. Armant, R. L. DiDonato, J. Choi, L. Cornelissen, L. M. Pereira, E. F. Augustine, C. A. Genetti, K. Dies, B. Barton, L. Williams, B. D. Goodlett, B. L. Riley, A. Pasternak, E. R. Berry, K. A. Pflock, S. Chu, C. Reed, K. Tyndall, P. B. Agrawal, A. H. Beggs, P. E. Grant, D. K. Urion, R. O. Snyder, S. E. Waisbren, A. Poduri, P. J. Park, A. Patterson, A. Biffi, J. R. Mazzulli, O. Bodamer, C. B. Berde and T. W. Yu, N. Engl. J. Med., 2019, 381, 1644–1652 CrossRef CAS PubMed.
- S. Ait Benichou, D. Jauvin, T. De Serres-Bérard, M. Pierre, K. K. Ling, C. F. Bennett, F. Rigo, G. Gourdon, M. Chahine and J. Puymirat, Gene Ther., 2022, 29, 698–709 CrossRef CAS PubMed.
- A. Schambach, C. J. Buchholz, R. Torres-Ruiz, K. Cichutek, M. Morgan, I. Trapani and H. Büning, Lancet, 2024, 403, 568–582 CrossRef CAS PubMed.
- Y. Shi, X. Zhen, Y. Zhang, Y. Li, S. Koo, Q. Saiding, N. Kong, G. Liu, W. Chen and W. Tao, Chem. Rev., 2024, 124, 929–1033 CrossRef CAS PubMed.
- B. Hu, L. Zhong, Y. Weng, L. Peng, Y. Huang, Y. Zhao and X. Liang, Signal Transduction Targeted Ther., 2020, 5, 101 CrossRef CAS PubMed.
- H. Li, Y. Yang, W. Hong, M. Huang, M. Wu and X. Zhao, Signal Transduction Targeted Ther., 2020, 5, 1 CrossRef PubMed.
- Y. Weng, C. Li, T. Yang, B. Hu, M. Zhang, S. Guo, H. Xiao, X. Liang and Y. Huang, Biotechnol. Adv., 2020, 40, 107534 CrossRef CAS PubMed.
- N. J. Sauer, J. Mozoruk, R. B. Miller, Z. J. Warburg, K. A. Walker, P. R. Beetham, C. R. Schöpke and G. F. W. Gocal, Plant Biotechnol. J., 2016, 14, 496–502 CrossRef CAS PubMed.
- D. H. Li, F. L. Mastaglia, S. Fletcher and S. D. Wilton, Trends Pharmacol. Sci., 2018, 39, 982–994 CrossRef CAS PubMed.
- S. Yang, H. Li, L. Xu, Z. Deng, W. Han, Y. Liu, W. Jiang and Y. Zu, Mol. Ther.--Nucleic Acids, 2018, 13, 164–175 CrossRef CAS PubMed.
- I. Fyfe, Nat. Rev. Neurol., 2019, 15, 687 CrossRef PubMed.
- S. T. Crooke, Trends Mol. Med., 2022, 28, 87–96 CrossRef PubMed.
- M. E. Gilles, L. L. Hao, L. Huang, R. Rupaimoole, P. P. Lopez-Casas, E. Pulver, J. C. Jeong, S. K. Muthuswamy, M. Hidalgo, S. N. Bhatia and F. J. Slack, Clin. Cancer Res., 2018, 24, 1734–1747 CrossRef CAS PubMed.
- T. C. Roberts, R. Langer and M. J. A. Wood, Nat. Rev. Drug Discovery, 2020, 19, 673–694 CrossRef CAS PubMed.
- T. H. Baryakova, B. H. Pogostin, R. Langer and K. J. McHugh, Nat. Rev. Drug Discovery, 2023, 22, 387–409 CrossRef CAS PubMed.
- Q. Li, K. Yin, H. P. Ma, H. H. Liu, S. Li, X. Luo, R. Hu, W. W. Zhang, Z. S. Lv, X. L. Niu, M. H. Gu, C. L. Li, Y. S. Liu, Y. J. Liu, H. B. Li, N. Y. Li, C. Li, W. W. Gu and J. J. Li, Mol. Ther., 2024, 32, 637–645 CrossRef CAS PubMed.
- P. J. Kamola, J. D. A. Kitson, G. Turner, K. Maratou, S. Eriksson, A. Panjwani, L. C. Warnock, G. A. D. Guilloux, K. Moores, E. L. Koppe, W. E. Wixted, P. A. Wilson, N. J. Gooderham, T. W. Gant, K. L. Clark, S. A. Hughes, M. R. Edbrooke and J. D. Parry, Nucleic Acids Res., 2015, 43, 8638–8650 CrossRef CAS PubMed.
- T. Khan, H. Weber, J. DiMuzio, A. Matter, B. Dogdas, T. Shah, A. Thankappan, J. Disa, V. Jadhav, L. Lubbers, L. Sepp-Lorenzino, W. R. Strapps and M. Tadin-Strapps, Mol. Ther.--Nucleic Acids, 2016, 5, e342 CrossRef CAS PubMed.
- A. Goga and M. Stoffel, Nat. Rev. Drug Discovery, 2022, 21, 417–439 CrossRef CAS PubMed.
- T. Friedmann, Nat. Genet., 1992, 2, 93–98 CrossRef CAS PubMed.
- W. F. Anderson, Science, 1992, 256, 808–813 CrossRef CAS PubMed.
- D. B. Kohn, Y. Y. Chen and M. J. Spencer, Gene Ther., 2023, 30, 738–746 CrossRef CAS PubMed.
- C. M. Perry and J. A. B. Balfour, Drugs, 1999, 57, 375–380 CrossRef CAS PubMed.
- A. M. Quemener, L. Bachelot, A. Forestier, E. Donnou-Fournet, D. Gilot and M. D. Galibert, Wiley Interdiscip. Rev.: RNA, 2020, 11, e1594 CrossRef PubMed.
- S. T. Crooke, B. F. Baker, R. M. Crooke and X. Liang, Nat. Rev. Drug Discovery, 2021, 20, 427–453 CrossRef CAS PubMed.
- D. R. Corey, Nat. Neurosci., 2017, 20, 497–499 CrossRef CAS PubMed.
- P. Hair, F. Cameron and K. McKeage, Drugs, 2013, 73, 487–493 CrossRef CAS PubMed.
- M. T. Tse, Nat. Rev. Drug Discovery, 2013, 12, 179 CrossRef CAS.
- F. Fogacci, N. Ferri, P. P. Toth, M. Ruscica, A. Corsini and A. F. G. Cicero, Drugs, 2019, 79, 751–766 CrossRef CAS PubMed.
- A. Fire, S. Xu, M. K. Montgomery, S. A. Kostas, S. E. Driver and C. C. Mello, Nature, 1998, 391, 806–811 CrossRef CAS PubMed.
- M. K. Montgomery, Nat. Struct. Mol. Biol., 2006, 13, 1039–1041 CrossRef CAS PubMed.
- M. Jinek, K. Chylinski, I. Fonfara, M. Hauer, J. A. Doudna and E. Charpentier, Science, 2012, 337, 816–821 CrossRef CAS PubMed.
- T. Li, Y. Yang, H. Qi, W. Cui, L. Zhang, X. Fu, X. He, M. Liu, P. Li and T. Yu, Signal Transduction Targeted Ther., 2023, 8, 36 CrossRef PubMed.
- K. Karikó, M. Buckstein, H. Ni and D. Weissman, Immunity, 2005, 23, 165–175 CrossRef PubMed.
- X. Han, H. Zhang, K. Butowska, K. L. Swingle, M. G. Alameh, D. Weissman and M. J. Mitchell, Nat. Commun., 2021, 12, 7233 CrossRef CAS PubMed.
- X. Hou, T. Zaks, R. Langer and Y. Dong, Nat. Rev. Mater., 2021, 6, 1078–1094 CrossRef CAS PubMed.
- M. J. Mitchell, M. M. Billingsley, R. M. Haley, M. E. Wechsler, N. A. Peppas and R. Langer, Nat. Rev. Drug Discovery, 2021, 20, 101–124 CrossRef CAS PubMed.
- X. Han, M. Alameh, K. Butowska, J. J. Knox, K. Lundgreen, M. Ghattas, N. Gong, L. Xue, Y. Xu, M. Lavertu, P. Bates, J. Xu, G. Nie, Y. Zhong, D. Weissman and M. J. Mitchell, Nat. Nanotechnol., 2023, 18, 1105–1114 CrossRef CAS PubMed.
- K. Chen, B. S. Zhao and C. He, Cell Chem. Biol., 2016, 23, 74–85 CrossRef CAS PubMed.
- M. Egli and M. Manoharan, Nucleic Acids Res., 2023, 51, 2529–2573 CrossRef CAS PubMed.
- E. M. Lee, N. A. Setterholm, M. Hajjar, B. Barpuzary and J. C. Chaput, Nucleic Acids Res., 2023, 51, 9542–9551 CrossRef CAS PubMed.
- K. Paunovska, D. Loughrey and J. E. Dahlman, Nat. Rev. Genet., 2022, 23, 265–280 CrossRef CAS PubMed.
- J. P. Bost, H. Barriga, M. N. Holme, A. Gallud, M. Maugeri, D. Gupta, T. Lehto, H. Valadi, E. K. Esbjörner, M. M. Stevens and S. El-Andaloussi, ACS Nano, 2021, 15, 13993–14021 CrossRef CAS PubMed.
- V. Prakash, Gene Ther., 2017, 24, 497 CrossRef CAS PubMed.
- J. M. Migliorati, J. Jin and X. Zhong, Trends Pharmacol. Sci., 2022, 43, 455–456 CrossRef CAS PubMed.
- Y. N. Lamb, Drugs, 2021, 81, 389–395 CrossRef CAS PubMed.
- K. N. Vokinger, C. E. G. Glaus and A. S. Kesselheim, Gene Ther., 2023, 30, 756–760 CrossRef CAS PubMed.
- M. Senior, Nat. Biotechnol., 2024, 42, 355–361 CrossRef CAS PubMed.
- B. P. Vinjamuri, J. Pan and P. Peng, J. Pharm. Sci., 2024, 113, 1749–1768 CrossRef CAS PubMed.
- M. González-Sales, A. L. Lennox, F. Huang, C. Pamulapati, Y. Wan, L. Sun, T. Berry, M. Kelly Behrs, F. Feller and P. N. Morcos, CPT: Pharmacometrics Syst. Pharmacol., 2024, 13, 1264–1277 Search PubMed.
- A. Sang, S. Zhuo, A. Bochanis, J. E. Manautou, R. Bahal, X.-B. Zhong and T. P. Rasmussen, BioDrugs, 2024, 38, 511–526 CrossRef CAS PubMed.
- FDA, Approved Cellular and Gene Therapy Products, https://www.fda.gov/vaccines-blood-biologics/cellular-gene-therapy-products/approved-cellular-and-gene-therapy-products, (accessed 2024 Nov. 22, 2024).
- FDA, Drugs@FDA, https://www.accessdata.fda.gov/scripts/cder/daf/index.cfm, (accessed 2024 Nov. 22, 2024).
- B. P. Monia, J. F. Johnston, H. Sasmor and L. L. Cummins, J. Biol. Chem., 1996, 271, 14533–14540 CrossRef CAS PubMed.
- S. Choung, Y. J. Kim, S. Kim, H. O. Park and Y. C. Choi, Biochem. Biophys. Res. Commun., 2006, 342, 919–927 CrossRef CAS PubMed.
- J. M. Harp, D. C. Guenther, A. Bisbe, L. Perkins, S. Matsuda, G. R. Bommineni, I. Zlatev, D. J. Foster, N. Taneja, K. Charisse, M. A. Maier, K. G. Rajeev, M. Manoharan and M. Egli, Nucleic Acids Res., 2018, 46, 8090–8104 CrossRef CAS PubMed.
- M. Robbins, A. Judge, L. Liang, K. McClintock, E. Yaworski and I. MacLachlan, Mol. Ther., 2007, 15, 1663–1669 CrossRef CAS PubMed.
- B. A. Anderson, G. C. Freestone, A. Low, C. L. De-Hoyos, W. J. Drury, M. E. Ostergaard, M. T. Migawa, M. Fazio, W. B. Wan, A. Berdeja, E. Scandalis, S. A. Burel, T. A. Vickers, S. T. Crooke, E. E. Swayze, X. H. Liang and P. P. Seth, Nucleic Acids Res., 2021, 49, 9026–9041 CrossRef CAS PubMed.
- T. Yoshida, T. Hagihara, Y. Uchida, Y. Horiuchi, K. Sasaki, T. Yamamoto, T. Yamashita, Y. Goda, Y. Saito, T. Yamaguchi, S. Obika, S. Yamamoto and T. Inoue, Sci. Rep., 2024, 14, 11540 CrossRef CAS PubMed.
- T. Ohmichi, M. Kuwahara, N. Sasaki, M. Hasegawa, T. Nishikata, H. Sawai and N. Sugimoto, Angew. Chem., Int. Ed., 2005, 44, 6682–6685 CrossRef CAS PubMed.
- S. Ellipilli and K. N. Ganesh, J. Org. Chem., 2015, 80, 9185–9191 CrossRef CAS PubMed.
- Y. R. Baker, C. Thorpe, J. Chen, L. M. Poller, L. Cox, P. Kumar, W. F. Lim, L. Lie, G. McClorey, S. Epple, D. Singleton, M. A. McDonough, J. S. Hardwick, K. E. Christensen, M. J. A. Wood, J. P. Hall, A. H. El-Sagheer and T. Brown, Nat. Commun., 2022, 13, 4036 CrossRef CAS PubMed.
- A. M. Kawasaki, M. D. Casper, S. M. Freier, E. A. Lesnik, M. C. Zounes, L. L. Cummins, C. Gonzalez and P. D. Cook, J. Med. Chem., 1993, 36, 831–841 CrossRef CAS PubMed.
- B. P. Monia, E. A. Lesnik, C. Gonzalez, W. F. Lima, D. McGee, C. J. Guinosso, A. M. Kawasaki, P. D. Cook and S. M. Freier, J. Biol. Chem., 1993, 268, 14514–14522 CrossRef CAS PubMed.
- T. C. Mou and D. M. Gray, Nucleic Acids Res., 2002, 30, 749–758 CrossRef CAS PubMed.
- T. M. Numnum, S. Makhija, B. Lu, M. Wang, A. Rivera, M. Stoff-Khalili, R. D. Alvarez, Z. B. Zhu and D. T. Curiel, Gynecol. Oncol., 2008, 108, 34–41 CrossRef CAS PubMed.
- J. H. Wang, D. J. Gessler, W. Zhan, T. L. Gallagher and G. Gao, Signal Transduction Targeted Ther., 2024, 9, 78 CrossRef CAS PubMed.
- K. V. Morris and J. J. Rossi, Gene Ther., 2006, 13, 553–558 CrossRef CAS PubMed.
- P. Huang, H. Deng, Y. Zhou and X. Chen, Matter, 2022, 5, 1670–1699 CrossRef CAS.
- J. Buck, P. Grossen, P. R. Cullis, J. Huwyler and D. Witzigmann, ACS Nano, 2019, 13, 3754–3782 CrossRef CAS PubMed.
- B. I. Andrews, F. D. Antia, S. B. Brueggemeier, L. J. Diorazio, S. G. Koenig, M. E. Kopach, H. Lee, M. Olbrich and A. L. Watson, J. Org. Chem., 2021, 86, 49–61 CrossRef CAS PubMed.
- A. L. Featherston, Y. Kwon, M. M. Pompeo, O. D. Engl, D. K. Leahy and S. J. Miller, Science, 2021, 371, 702–707 CrossRef CAS PubMed.
- M. Flamme, S. Hanlon, H. Iding, K. Puentener, F. Sladojevich and M. Hollenstein, Bioorg. Med. Chem. Lett., 2021, 48, 128242 CrossRef CAS PubMed.
- Y. Huang, K. W. Knouse, S. Qiu, W. Hao, N. M. Padial, J. C. Vantourout, B. Zheng, S. E. Mercer, J. Lopez-Ogalla, R. Narayan, R. E. Olson, D. G. Blackmond, M. D. Eastgate, M. A. Schmidt, I. M. McDonald and P. S. Baran, Science, 2021, 373, 1265–1270 CrossRef CAS PubMed.
- X. Zhou, W. F. Kiesman, W. Yan, H. Jiang, F. D. Antia, J. Yang, Y. A. Fillon, L. Xiao and X. Shi, J. Org. Chem., 2022, 87, 2087–2110 CrossRef CAS PubMed.
- E. R. Moody, R. Obexer, F. Nickl, R. Spiess and S. L. Lovelock, Science, 2023, 380, 1150–1154 CrossRef CAS PubMed.
- K. J. D. Van Giesen, M. J. Thompson, Q. Meng and S. L. Lovelock, JACS Au, 2023, 3, 13–24 CrossRef CAS PubMed.
- M. Lindow, H. P. Vornlocher, D. Riley, D. J. Kornbrust, J. Burchard, L. O. Whiteley, J. Kamens, J. D. Thompson, S. Nochur, H. Younis, S. Bartz, J. Parry, N. Ferrari, S. P. Henry and A. A. Levin, Nat. Biotechnol., 2012, 30, 920–923 CrossRef CAS PubMed.
- D. D. Nedorezova, M. V. Dubovichenko, E. P. Belyaeva, E. D. Grigorieva, A. V. Peresadina and D. M. Kolpashchikov, Theranostics, 2022, 12, 7132–7157 CrossRef CAS PubMed.
- C. Terada, K. Oh, R. Tsubaki, B. Chan, N. Aibara, K. Ohyama, M. A. Shibata, T. Wada, M. Harada-Shiba, A. Yamayoshi and T. Yamamoto, Nat. Commun., 2023, 14, 7972 CrossRef CAS PubMed.
- W. Shen, C. L. De Hoyos, H. Sun, T. A. Vickers, X. H. Liang and S. T. Crooke, Nucleic Acids Res., 2018, 46, 2204–2217 CrossRef CAS PubMed.
- S. T. Crooke, T. A. Vickers and X. Liang, Nucleic Acids Res., 2020, 48, 5235–5253 CrossRef PubMed.
- H. Yasuhara, T. Yoshida, K. Sasaki, S. Obika and T. Inoue, Mol. Diagn. Ther., 2022, 26, 117–127 CrossRef CAS PubMed.
- W. Shen, C. L. De Hoyos, M. T. Migawa, T. A. Vickers, H. Sun, A. Low, T. A. Bell, M. Rahdar, S. Mukhopadhyay, C. E. Hart, M. Bell, S. Riney, S. F. Murray, S. Greenlee, R. M. Crooke, X. H. Liang, P. P. Seth and S. T. Crooke, Nat. Biotechnol., 2019, 37, 640–650 CrossRef CAS PubMed.
- X. H. Liang, H. Sun, W. Shen and S. T. Crooke, Nucleic Acids Res., 2015, 43, 2927–2945 CrossRef CAS PubMed.
- T. A. Vickers and S. T. Crooke, PLoS One, 2016, 11, e0161930 CrossRef PubMed.
- A. D. Burdick, S. Sciabola, S. R. Mantena, B. D. Hollingshead, R. Stanton, J. A. Warneke, M. Zeng, E. Martsen, A. Medvedev, S. S. Makarov, L. A. Reed, J. W. Davis, II and L. O. Whiteley, Nucleic Acids Res., 2014, 42, 4882–4891 CrossRef CAS PubMed.
- T. Yoshida, K. Morihiro, Y. Naito, A. Mikami, Y. Kasahara, T. Inoue and S. Obika, Nucleic Acids Res., 2022, 50, 7224–7234 CrossRef CAS PubMed.
- M. Hannus, M. Beitzinger, J. C. Engelmann, M. T. Weickert, R. Spang, S. Hannus and G. Meister, Nucleic Acids Res., 2014, 42, 8049–8061 CrossRef CAS PubMed.
- X. Lai, M. Eberhardt, U. Schmitz and J. Vera, Nucleic Acids Res., 2019, 47, 7753–7766 CrossRef CAS PubMed.
- M. T. Migawa, W. Shen, W. B. Wan, G. Vasquez, M. E. Oestergaard, A. Low, C. L. De Hoyos, R. Gupta, S. Murray, M. Tanowitz, M. Bell, J. G. Nichols, H. Gaus, X. H. Liang, E. E. Swayze, S. T. Crooke and P. P. Seth, Nucleic Acids Res., 2019, 47, 5465–5479 CrossRef CAS PubMed.
- S. T. Crooke, X. H. Liang, R. M. Crooke, B. F. Baker and R. S. Geary, Biochem. Pharmacol., 2021, 189, 114196 CrossRef CAS PubMed.
- S. A. Burel, C. E. Hart, P. Cauntay, J. Hsiao, T. Machemer, M. Katz, A. Watt, H. H. Bui, H. Younis, M. Sabripour, S. M. Freier, G. Hung, A. Dan, T. P. Prakash, P. P. Seth, E. E. Swayze, C. F. Bennett, S. T. Crooke and S. P. Henry, Nucleic Acids Res., 2016, 44, 2093–2109 CrossRef CAS PubMed.
- J. D. A. Kitson, P. J. Kamola and L. Kane, in Oligonucleotide-Based Drugs and Therapeutics, 2018, pp. 191–225 DOI:10.1002/9781119070153.ch7.
- A. L. Jackson and P. S. Linsley, Trends Genet., 2004, 20, 521–524 CrossRef CAS PubMed.
- J. D. Watson and F. H. C. Crick, Nature, 1953, 171, 737–738 CrossRef CAS PubMed.
- H. Karabiyik, R. Sevincek and H. Karabiyik, Phys. Chem. Chem. Phys., 2014, 16, 15527–15538 RSC.
- R. R. Iyer, A. Pluciennik, V. Burdett and P. L. Modrich, Chem. Rev., 2006, 106, 302–323 CrossRef CAS PubMed.
- N. Chatterjee and G. C. Walker, Environ. Mol. Mutagen., 2017, 58, 235–263 CrossRef CAS PubMed.
- H. Echols and M. F. Goodman, Annu. Rev. Biochem., 1991, 60, 477–511 CrossRef CAS PubMed.
- D. E. Barnes and T. Lindahl, Annu. Rev. Genet., 2004, 38, 445–476 CrossRef CAS PubMed.
- I. I. Cisse, H. Kim and T. Ha, Nat. Struct. Mol. Biol., 2012, 19, 623–627 CrossRef CAS PubMed.
- M. Todisco and J. W. Szostak, Nucleic Acids Res., 2022, 50, 9647–9662 CrossRef CAS PubMed.
- D. K. Kim, Y. S. Kwon, Y. Takamura and E. Tamiya, Jpn. J. Appl. Phys., Part 1, 2006, 45, 509–512 CrossRef CAS.
- S. R. Doyle, C. K. Chan and W. N. Grant, BMC Biotechnol., 2011, 11, 83 CrossRef CAS PubMed.
- S. E. Sandler, N. E. Weckman, S. Yorke, A. Das, K. Chen, R. Guitterez and U. F. Keyser, Nat. Biomed. Eng., 2024, 8, 325–334 CrossRef CAS PubMed.
- A. L. Jackson, S. R. Bartz, J. Schelter, S. V. Kobayashi, J. Burchard, M. Mao, B. Li, G. Cavet and P. S. Linsley, Nat. Biotechnol., 2003, 21, 635–637 CrossRef CAS PubMed.
- T. A. Vickers, S. Koo, C. F. Bennett, S. T. Crooke, N. M. Dean and B. F. Baker, J. Biol. Chem., 2003, 278, 7108–7118 CrossRef CAS PubMed.
- S. Aguti, S. Cheng, P. Ala, S. Briggs, F. Muntoni and H. Zhou, Mol. Ther.--Nucleic Acids, 2024, 35, 102237 CrossRef CAS PubMed.
- D. Magner, E. Biala, J. Lisowiec-Wachnicka and R. Kierzek, Sci. Rep., 2017, 7, 12532 CrossRef PubMed.
- F. Conroy, R. Miller, J. F. Alterman, M. R. Hassler, D. Echeverria, B. M. D. C. Godinho, E. G. Knox, E. Sapp, J. Sousa, K. Yamada, F. Mahmood, A. Boudi, K. Kegel-Gleason, M. DiFiglia, N. Aronin, A. Khvorova and E. L. Pfister, Nat. Commun., 2022, 13, 5802 CrossRef CAS PubMed.
- A. Lomakin and M. D. Frank-Kamenetskii, J. Mol. Biol., 1998, 276, 57–70 CrossRef CAS PubMed.
- V. V. Demidov and M. D. Frank-Kamenetskii, Trends Biochem. Sci., 2004, 29, 62–71 CrossRef CAS PubMed.
- M. E. Craig, D. M. Crothers and P. Doty, J. Mol. Biol., 1971, 62, 383–401 CrossRef CAS PubMed.
- J. G. Wetmur, Annu. Rev. Biophys., 1976, 5, 337–361 CAS.
- D. H. Turner, Curr. Opin. Struct. Biol., 1996, 6, 299–304 CrossRef CAS PubMed.
- R. R. Sinden, C. E. Pearson, V. N. Potaman and D. W. Ussery, in Advances in Genome Biology, ed. R. S. Verma, JAI, 1998, vol. 5, pp. 1–141 Search PubMed.
- E. Giudice and R. Lavery, J. Am. Chem. Soc., 2003, 125, 4998–4999 CrossRef CAS PubMed.
- A. V. Pinheiro, J. Nangreave, S. Jiang, H. Yan and Y. Liu, ACS Nano, 2012, 6, 5521–5530 CrossRef CAS PubMed.
- T. E. Ouldridge, P. Šulc, F. Romano, J. P. K. Doye and A. A. Louis, Nucleic Acids Res., 2013, 41, 8886–8895 CrossRef CAS PubMed.
- F. Häse and M. Zacharias, Nucleic Acids Res., 2016, 44, 7100–7108 Search PubMed.
- T. M. Woolf, D. A. Melton and C. G. Jennings, Proc. Natl. Acad. Sci. U. S. A., 1992, 89, 7305–7309 CrossRef CAS PubMed.
- S. Kakiuchi-Kiyota, P. H. Koza-Taylor, S. R. Mantena, L. F. Nelms, A. E. Enayetallah, B. D. Hollingshead, A. D. Burdick, L. A. Reed, J. A. Warneke, L. O. Whiteley, A. M. Ryan and N. Mathialagan, Toxicol. Sci., 2014, 138, 234–248 CrossRef CAS PubMed.
- T. Kasuya, S. Hori, A. Watanabe, M. Nakajima, Y. Gahara, M. Rokushima, T. Yanagimoto and A. Kugimiya, Sci. Rep., 2016, 6, 30377 CrossRef CAS PubMed.
- A. Dieckmann, P. H. Hagedorn, Y. Burki, C. Brügmann, M. Berrera, M. Ebeling, T. Singer and F. Schuler, Mol. Ther.--Nucleic Acids, 2018, 10, 45–54 CrossRef CAS PubMed.
- X. Y. Lin, X. Ruan, M. G. Anderson, J. A. McDowell, P. E. Kroeger, S. W. Fesik and Y. Shen, Nucleic Acids Res., 2005, 33, 4527–4535 CrossRef CAS PubMed.
- P. C. Scacheri, O. Rozenblatt-Rosen, N. J. Caplen, T. G. Wolfsberg, L. Umayam, J. C. Lee, C. M. Hughes, K. S. Shanmugam, A. Bhattacharjee, M. Meyerson and F. S. Collins, Proc. Natl. Acad. Sci. U. S. A., 2004, 101, 1892–1897 CrossRef CAS PubMed.
- S. Saxena, Z. O. Jónsson and A. Dutta, J. Biol. Chem., 2003, 278, 44312–44319 CrossRef CAS PubMed.
- T. Cazares, R. E. Higgs, J. Wang and H. G. Ozer, Bioinformatics, 2024, 40, btae011 CrossRef CAS PubMed.
- A. L. Jackson and P. S. Linsley, Nat. Rev. Drug Discovery, 2010, 9, 57–67 CrossRef CAS PubMed.
- K. Ui-Tei, Y. Naito, K. Nishi, A. Juni and K. Saigo, Nucleic Acids Res., 2008, 36, 7100–7109 CrossRef CAS PubMed.
- A. L. Jackson, J. Burchard, J. Schelter, B. N. Chau, M. Cleary, L. Lim and P. S. Linsley, RNA, 2006, 12, 1179–1187 CrossRef CAS PubMed.
- H. Manghwar, B. Li, X. Ding, A. Hussain, K. Lindsey, X. L. Zhang and S. X. Jin, Adv. Sci. Lett., 2020, 7 Search PubMed.
- M. V. Zuccaro, J. Xu, C. Mitchell, D. Marin, R. Zimmerman, B. Rana, E. Weinstein, R. T. King, K. L. Palmerola, M. E. Smith, S. H. Tsang, R. Goland, M. Jasin, R. Lobo, N. Treff and D. Egli, Cell, 2020, 183, 1650–1664 CrossRef CAS PubMed.
- K. Kraft, S. Geuer, A. J. Will, W. L. Chan, C. Paliou, M. Borschiwer, I. Harabula, L. Wittler, M. Franke, D. M. Ibrahim, B. K. Kragesteen, M. Spielmann, S. Mundlos, D. G. Lupiáñez and G. Andrey, Cell Rep., 2015, 10, 833–839 CrossRef CAS PubMed.
- Y. Fu, J. A. Foden, C. Khayter, M. L. Maeder, D. Reyon, J. K. Joung and J. D. Sander, Nat. Biotechnol., 2013, 31, 822–826 CrossRef CAS PubMed.
- X. H. Zhang, L. Y. Tee, X. G. Wang, Q. S. Huang and S. H. Yang, Mol. Ther.--Nucleic Acids, 2015, 4, e264 CrossRef CAS PubMed.
- H. Nishimasu, X. Shi, S. Ishiguro, L. Gao, S. Hirano, S. Okazaki, T. Noda, O. O. Abudayyeh, J. S. Gootenberg, H. Mori, S. Oura, B. Holmes, M. Tanaka, M. Seki, H. Hirano, H. Aburatani, R. Ishitani, M. Ikawa, N. Yachie, F. Zhang and O. Nureki, Science, 2018, 361, 1259–1262 CrossRef CAS PubMed.
- J. K. Lee, E. Jeong, J. Lee, M. Jung, E. Shin, Y.-H. Kim, K. Lee, I. Jung, D. Kim, S. Kim and J.-S. Kim, Nat. Commun., 2018, 9, 3048 CrossRef PubMed.
- A. Casini, M. Olivieri, G. Petris, C. Montagna, G. Reginato, G. Maule, F. Lorenzin, D. Prandi, A. Romanel, F. Demichelis, A. Inga and A. Cereseto, Nat. Biotechnol., 2018, 36, 265–271 CrossRef CAS PubMed.
- H. Manghwar, B. Li, X. Ding, A. Hussain, K. Lindsey, X. Zhang and S. Jin, Adv. Sci. Lett., 2020, 7, 1902312 CAS.
- X. Q. Huang, D. S. Yang, J. F. Zhang, J. Xu and Y. E. Chen, Cells, 2022, 11, 2186 CrossRef CAS PubMed.
- C. T. Guo, X. T. Ma, F. Gao and Y. X. Guo, Front. Bioeng. Biotechnol., 2023, 11, 1143157 CrossRef PubMed.
- M. Asmamaw Mengstie, M. Teshome Azezew, T. Asmamaw Dejenie, A. A. Teshome, F. Tadele Admasu, A. Behaile Teklemariam, A. Tilahun Mulu, M. Mekonnen Agidew, D. G. Adugna, H. Geremew and E. C. Abebe, Biol.: Targets Ther., 2024, 18, 21–28 Search PubMed.
- B. P. Kleinstiver, V. Pattanayak, M. S. Prew, S. Q. Tsai, N. T. Nguyen, Z. Zheng and J. K. Joung, Nature, 2016, 529, 490–495 CrossRef CAS PubMed.
- F. A. Ran, P. D. Hsu, C.-Y. Lin, J. S. Gootenberg, S. Konermann, A. E. Trevino, D. A. Scott, A. Inoue, S. Matoba, Y. Zhang and F. Zhang, Cell, 2013, 154, 1380–1389 CrossRef CAS PubMed.
- K. M. Davis, V. Pattanayak, D. B. Thompson, J. A. Zuris and D. R. Liu, Nat. Chem. Biol., 2015, 11, 316–318 CrossRef CAS PubMed.
- I. M. Slaymaker, L. Gao, B. Zetsche, D. A. Scott, W. X. Yan and F. Zhang, Science, 2016, 351, 84–88 CrossRef CAS PubMed.
- X. J. Piao, H. Z. Wang, D. W. Binzel and P. X. Guo, RNA, 2018, 24, 67–76 CrossRef CAS PubMed.
- P. H. Hagedorn, M. Pontoppidan, T. S. Bisgaard, M. Berrera, A. Dieckmann, M. Ebeling, M. R. Moller, H. Hudlebusch, M. L. Jensen, H. F. Hansen, T. Koch and M. Lindow, Nucleic Acids Res., 2018, 46, 5366–5380 CrossRef CAS PubMed.
- T. Yoshida, Y. Naito, K. Sasaki, E. Uchida, Y. Sato, M. Naito, T. Kawanishi, S. Obika and T. Inoue, Genes Cells, 2018, 23, 448–455 CrossRef CAS PubMed.
- X. Song, X. Wang, Y. Ma, Z. Liang, Z. Yang and H. Cao, Mol. Ther.--Nucleic Acids, 2017, 9, 242–250 CrossRef CAS PubMed.
- E. Fakhr, F. Zare and L. Teimoori-Toolabi, Cancer Gene Ther., 2016, 23, 73–82 CrossRef CAS PubMed.
- H. Iribe, K. Miyamoto, T. Takahashi, Y. Kobayashi, J. Leo, M. Aida and K. Ui-Tei, ACS Omega, 2017, 2, 2055–2064 CrossRef CAS PubMed.
- P. Y. Chen, L. Weinmann, D. Gaidatzis, Y. Pei, M. Zavolan, T. Tuschl and G. Meister, RNA, 2008, 14, 263–274 CrossRef CAS PubMed.
- Y. L. Chiu and T. M. Rana, RNA, 2003, 9, 1034–1048 CrossRef CAS PubMed.
- A. L. Jackson, J. Burchard, D. Leake, A. Reynolds, J. Schelter, J. Guo, J. M. Johnson, L. Lim, J. Karpilow, K. Nichols, W. Marshall, A. Khvorova and P. S. Linsley, RNA, 2006, 12, 1197–1205 CrossRef CAS PubMed.
- N. Vaish, F. Chen, S. Seth, K. Fosnaugh, Y. Liu, R. Adami, T. Brown, Y. Chen, P. Harvie, R. Johns, G. Severson, B. Granger, P. Charmley, M. Houston, M. V. Templin and B. Polisky, Nucleic Acids Res., 2010, 39, 1823–1832 CrossRef PubMed.
- J. B. Bramsen, M. M. Pakula, T. B. Hansen, C. Bus, N. Langkjær, D. Odadzic, R. Smicius, S. L. Wengel, J. Chattopadhyaya, J. W. Engels, P. Herdewijn, J. Wengel and J. Kjems, Nucleic Acids Res., 2010, 38, 5761–5773 CrossRef CAS PubMed.
- J. Elmén, H. Thonberg, K. Ljungberg, M. Frieden, M. Westergaard, Y. Xu, B. Wahren, Z. Liang, H. Ørum, T. Koch and C. Wahlestedt, Nucleic Acids Res., 2005, 33, 439–447 CrossRef PubMed.
- K. Ui-Tei, Y. Naito, S. Zenno, K. Nishi, K. Yamato, F. Takahashi, A. Juni and K. Saigo, Nucleic Acids Res., 2008, 36, 2136–2151 CrossRef CAS PubMed.
- E. Rozners, J. Am. Chem. Soc., 2022, 144, 12584–12594 CrossRef CAS PubMed.
- M. Rahdar, M. A. McMahon, T. P. Prakash, E. E. Swayze, C. F. Bennett and D. W. Cleveland, Proc. Natl. Acad. Sci. U. S. A., 2015, 112, E7110–E7117 CrossRef CAS PubMed.
- C. R. Cromwell, K. Sung, J. Park, A. R. Krysler, J. Jovel, S. K. Kim and B. P. Hubbard, Nat. Commun., 2018, 9, 1448 CrossRef PubMed.
- H. Yin, C. Q. Song, S. Suresh, Q. Wu, S. Walsh, L. H. Rhym, E. Mintzer, M. F. Bolukbasi, L. J. Zhu, K. Kauffman, H. Mou, A. Oberholzer, J. Ding, S. Y. Kwan, R. L. Bogorad, T. Zatsepin, V. Koteliansky, S. A. Wolfe, W. Xue, R. Langer and D. G. Anderson, Nat. Biotechnol., 2017, 35, 1179–1187 CrossRef CAS PubMed.
- J. Scharner, W. K. Ma, Q. Zhang, K. T. Lin, F. Rigo, C. F. Bennett and A. R. Krainer, Nucleic Acids Res., 2020, 48, 802–816 CrossRef CAS PubMed.
- S. Qiu, C. M. Adema and T. Lane, Nucleic Acids Res., 2005, 33, 1834–1847 CrossRef CAS PubMed.
- Y. Fu, J. D. Sander, D. Reyon, V. M. Cascio and J. K. Joung, Nat. Biotechnol., 2014, 32, 279–284 CrossRef CAS PubMed.
- A. Auradkar, A. Guichard, S. Kaduwal, M. Sneider and E. Bier, Nat. Commun., 2023, 14, 5587 CrossRef CAS PubMed.
- J. Zhang, X. Li, A. Neises, W. Chen, L. Hu, G. Ji, J. Yu, J. Xu, W. Yuan, T. Cheng and X. Zhang, Sci. Rep., 2016, 6, 28566 CrossRef CAS PubMed.
- S. Petri and G. Meister, Methods Mol. Biol., 2013, 986, 59–71 CrossRef CAS PubMed.
- J. G. Doench, N. Fusi, M. Sullender, M. Hegde, E. W. Vaimberg, K. F. Donovan, I. Smith, Z. Tothova, C. Wilen, R. Orchard, H. W. Virgin, J. Listgarten and D. E. Root, Nat. Biotechnol., 2016, 34, 184–191 CrossRef CAS PubMed.
- S. Michel, K. Schirduan, Y. M. Shen, R. Klar, J. Tost and F. Jaschinski, Mol. Diagn. Ther., 2021, 25, 77–85 CrossRef CAS PubMed.
- M. Naeem and O. S. Alkhnbashi, Int. J. Mol. Sci., 2023, 24, 6261 CrossRef CAS PubMed.
- G. Q. Liu, Y. Zhang and T. Zhang, Comput. Struct. Biotechnol. J., 2020, 18, 35–44 CrossRef CAS PubMed.
- S. S. Xie, B. Shen, C. B. Zhang, X. X. Huang and Y. L. Zhang, PLoS One, 2014, 9, 100448 CrossRef PubMed.
- T. Yamada and S. Morishita, Bioinformatics, 2005, 21, 1316–1324 CrossRef CAS PubMed.
- Y. K. Park, S. M. Park, Y. C. Choi, D. Lee, M. Won and Y. J. Kim, Nucleic Acids Res., 2008, 36, W97–W103 CrossRef CAS PubMed.
- N. Papargyri, M. Pontoppidan, M. R. Andersen, T. Koch and P. H. Hagedorn, Mol. Ther.--Nucleic Acids, 2020, 19, 706–717 CrossRef CAS PubMed.
- S. Chiba, K. R. Q. Lim, N. Sheri, S. Anwar, E. Erkut, M. N. A. Shah, T. Aslesh, S. Woo, O. Sheikh, R. Maruyama, H. Takano, K. Kunitake, W. Duddy, Y. Okuno, Y. Aoki and T. Yokota, Nucleic Acids Res., 2021, 49, W193–W198 CrossRef CAS PubMed.
- G. Hwang, M. Kwon, D. Seo, D. H. Kim, D. Lee, K. Lee, E. Kim, M. Kang and J. H. Ryu, Mol. Ther.--Nucleic Acids, 2024, 35, 102186 CrossRef CAS PubMed.
- N. C. Seeman, J. Theor. Biol., 1982, 99, 237–247 CrossRef CAS PubMed.
- Y. Zhang, J. Tu, D. Wang, H. Zhu, S. K. Maity, X. Qu, B. Bogaert, H. Pei and H. Zhang, Adv. Mater., 2018, 30, e1703658 CrossRef PubMed.
- P. Zhang, J. Rufo, C. Chen, J. Xia, Z. Tian, L. Zhang, N. Hao, Z. Zhong, Y. Gu, K. Chakrabarty and T. J. Huang, Nat. Commun., 2021, 12, 3844 CrossRef CAS PubMed.
- L. Shen, P. Wang and Y. Ke, Adv. Healthcare Mater., 2021, 10, 2002205 CrossRef CAS PubMed.
- D. Selnihhin, S. M. Sparvath, S. Preus, V. Birkedal and E. S. Andersen, ACS Nano, 2018, 12, 5699–5708 CrossRef CAS PubMed.
- Q. Zhang, Q. Jiang, N. Li, L. Dai, Q. Liu, L. Song, J. Wang, Y. Li, J. Tian, B. Ding and Y. Du, ACS Nano, 2014, 8, 6633–6643 CrossRef CAS PubMed.
- C. Angell, S. Xie, L. Zhang and Y. Chen, Small, 2016, 12, 1117–1132 CrossRef CAS PubMed.
- Q. Hu, H. Li, L. Wang, H. Gu and C. Fan, Chem. Rev., 2019, 119, 6459–6506 CrossRef CAS PubMed.
- X. Wu, C. Yang, H. Wang, X. Lu, Y. Shang, Q. Liu, J. Fan, J. Liu and B. Ding, J. Am. Chem. Soc., 2023, 145, 9343–9353 CrossRef CAS PubMed.
- J. A. Kretzmann, A. Liedl, A. Monferrer, V. Mykhailiuk, S. Beerkens and H. Dietz, Nat. Commun., 2023, 14, 1017 CrossRef CAS PubMed.
- K. E. Bujold, J. Fakhoury, T. G. W. Edwardson, K. M. M. Carneiro, J. N. Briard, A. G. Godin, L. Amrein, G. D. Hamblin, L. C. Panasci, P. W. Wiseman and H. F. Sleiman, Chem. Sci., 2014, 5, 2449–2455 RSC.
- K. E. Bujold, J. C. C. Hsu and H. F. Sleiman, J. Am. Chem. Soc., 2016, 138, 14030–14038 CrossRef CAS PubMed.
- H. H. Fakih, J. J. Fakhoury, D. Bousmail and H. F. Sleiman, ACS Appl. Mater. Interfaces, 2019, 11, 13912–13920 CrossRef CAS PubMed.
- P. Wang, M. A. Rahman, Z. Zhao, K. Weiss, C. Zhang, Z. Chen, S. J. Hurwitz, Z. G. Chen, D. M. Shin and Y. Ke, J. Am. Chem. Soc., 2018, 140, 2478–2484 CrossRef CAS PubMed.
- M. M. C. Bastings, F. M. Anastassacos, N. Ponnuswamy, F. G. Leifer, G. Cuneo, C. Lin, D. E. Ingber, J. H. Ryu and W. M. Shih, Nano Lett., 2018, 18, 3557–3564 CrossRef CAS PubMed.
- Y. M. Zhang, X. C. Tian, Z. J. Wang, H. C. Wang, F. Liu, Q. P. Long and S. L. Jiang, Front. Mol. Biosci., 2023, 10, 1239952 CrossRef CAS PubMed.
- E. S. Andersen, M. Dong, M. M. Nielsen, K. Jahn, R. Subramani, W. Mamdouh, M. M. Golas, B. Sander, H. Stark, C. L. Oliveira, J. S. Pedersen, V. Birkedal, F. Besenbacher, K. V. Gothelf and J. Kjems, Nature, 2009, 459, 73–76 CrossRef CAS PubMed.
- D. Jiang, Z. Ge, H. J. Im, C. G. England, D. Ni, J. Hou, L. Zhang, C. J. Kutyreff, Y. Yan, Y. Liu, S. Y. Cho, J. W. Engle, J. Shi, P. Huang, C. Fan, H. Yan and W. Cai, Nat. Biomed. Eng., 2018, 2, 865–877 CrossRef CAS PubMed.
- C. Wiraja, Y. Zhu, D. C. S. Lio, D. C. Yeo, M. Xie, W. Fang, Q. Li, M. Zheng, M. Van Steensel, L. Wang, C. Fan and C. Xu, Nat. Commun., 2019, 10, 1147 CrossRef PubMed.
- C. Y. Tay, L. Yuan and D. T. Leong, ACS Nano, 2015, 9, 5609–5617 CrossRef CAS PubMed.
- H. Qiu, J. C. Dewan and N. C. Seeman, J. Mol. Biol., 1997, 267, 881–898 CrossRef CAS PubMed.
- A. R. Chandrasekaran and R. Zhuo, Appl. Mater. Today, 2016, 2, 7–16 CrossRef.
- C. G. Evans and E. Winfree, Chem. Soc. Rev., 2017, 46, 3808–3829 RSC.
- D. Hayakawa, T. E. Videbaek, D. M. Hall, H. Fang, C. Sigl, E. Feigl, H. Dietz, S. Fraden, M. F. Hagan, G. M. Grason and W. B. Rogers, Proc. Natl. Acad. Sci. U. S. A., 2022, 119, e2207902119 CrossRef CAS PubMed.
- C. Zhang, M. Zheng, Y. P. Ohayon, S. Vecchioni, R. Sha, N. C. Seeman, N. Jonoska and C. Mao, J. Am. Chem. Soc., 2022, 144, 8741–8745 CrossRef CAS PubMed.
- T. Zhang and B. Wei, J. Am. Chem. Soc., 2022, 144, 18479–18484 CrossRef CAS PubMed.
- R. Chen, S. Feng, J. Ren, H. Kang, Y. Yang, N. Xia, F. Fang and B. Wei, J. Am. Chem. Soc., 2023, 145, 9176–9181 CrossRef CAS PubMed.
- J. Zhao, C. Zhang, B. Lu, R. Sha, N. Noinaj and C. Mao, J. Am. Chem. Soc., 2023, 145, 10475–10479 CrossRef CAS PubMed.
- J. P. Zheng, J. J. Birktoft, Y. Chen, T. Wang, R. J. Sha, P. E. Constantinou, S. L. Ginell, C. D. Mao and N. C. Seeman, Nature, 2009, 461, 74–77 CrossRef CAS PubMed.
- Y. P. Ohayon, C. Hernandez, A. R. Chandrasekaran, X. Wang, H. O. Abdallah, M. A. Jong, M. G. Mohsen, R. Sha, J. J. Birktoft, P. S. Lukeman, P. M. Chaikin, S. L. Ginell, C. Mao and N. C. Seeman, ACS Nano, 2019, 13, 7957–7965 CrossRef CAS PubMed.
- K. Woloszyn, S. Vecchioni, Y. P. Ohayon, B. Lu, Y. Ma, Q. Huang, E. Zhu, D. Chernovolenko, T. Markus, N. Jonoska, C. Mao, N. C. Seeman and R. Sha, Adv. Mater., 2022, 34, 2206876 CrossRef CAS PubMed.
- E. Torelli, M. Marini, S. Palmano, L. Piantanida, C. Polano, A. Scarpellini, M. Lazzarino and G. Firrao, Small, 2014, 10, 2918–2926 CrossRef CAS PubMed.
- F. Zhou, H. Ni, G. L. Zhu, L. Bershadsky, R. J. Sha, N. C. Seeman and P. M. Chaikin, Sci. Rob., 2023, 8, eadf1274 CrossRef PubMed.
- C. Lin, Y. Liu and H. Yan, Biochemistry, 2009, 48, 1663–1674 CrossRef CAS PubMed.
- G. Tikhomirov, P. Petersen and L. Qian, Nature, 2017, 552, 67–71 CrossRef CAS PubMed.
- F. Zhou, R. J. Sha, H. Ni, N. Seeman and P. Chaikin, Proc. Natl. Acad. Sci. U. S. A., 2021, 118, e2111193118 CrossRef PubMed.
- C. Geary, P. W. K. Rothemund and E. S. Andersen, Science, 2014, 345, 799–804 CrossRef CAS PubMed.
- C. Geary, G. Grossi, E. K. S. McRae, P. W. K. Rothemund and E. S. Andersen, Nat. Chem., 2021, 13, 549–558 CrossRef CAS PubMed.
- W. Ke, E. Hong, R. F. Saito, M. C. Rangel, J. Wang, M. Viard, M. Richardson, E. F. Khisamutdinov, M. Panigaj, N. V. Dokholyan, R. Chammas, M. A. Dobrovolskaia and K. A. Afonin, Nucleic Acids Res., 2018, 47, 1350–1361 CrossRef PubMed.
- M. F. Parsons, M. F. Allan, S. Li, T. R. Shepherd, S. Ratanalert, K. Zhang, K. M. Pullen, W. Chiu, S. Rouskin and M. Bathe, Nat. Commun., 2023, 14, 382 CrossRef CAS PubMed.
- H. Kong, B. Sun, F. Yu, Q. Wang, K. Xia and D. Jiang, Adv. Sci., 2023, 10, 2302021 CrossRef CAS PubMed.
- J. I. Cutler, E. Auyeung and C. A. Mirkin, J. Am. Chem. Soc., 2012, 134, 1376–1391 CrossRef CAS PubMed.
- C. R. Laramy, M. N. O’Brien and C. A. Mirkin, Nat. Rev. Mater., 2019, 4, 201–224 CrossRef CAS.
- D. Y. Zhang, J. Am. Chem. Soc., 2011, 133, 1077–1086 CrossRef CAS PubMed.
- D. Panne, Curr. Opin. Struct. Biol., 2008, 18, 236–242 CrossRef CAS PubMed.
- I. L. Tong and R. A. Young, Cell, 2013, 152, 1237–1251 CrossRef PubMed.
- S. P. Bell, R. M. Learned, H.-M. Jantzen and R. Tjian, Science, 1988, 241, 1192–1197 CrossRef CAS PubMed.
- A. Gao, K. Shrinivas, P. Lepeudry, H. I. Suzuki, P. A. Sharp and A. K. Chakraborty, Proc. Natl. Acad. Sci. U. S. A., 2018, 115, E11053–E11060 CAS.
- M. D. J. Bragdon, N. Patel, J. Chuang, E. Levien, C. J. Bashor and A. S. Khalil, Cell, 2023, 186, 3810–3825 CrossRef CAS PubMed.
- G. Tikhomirov, P. Petersen and L. Qian, Nat. Nanotechnol., 2017, 12, 251–259 CrossRef CAS PubMed.
- F. Cui, S. Marbach, J. A. Zheng, M. Holmes-Cerfon and D. J. Pine, Nat. Commun., 2022, 13, 2304 CrossRef CAS PubMed.
- C. Xie, Z. Chen, K. Chen, Y. Hu and L. Pan, ACS Nano, 2023, 17, 1505–1510 CrossRef CAS PubMed.
- M. E. Leunissen, R. Dreyfus, R. Sha, N. C. Seeman and P. M. Chaikin, J. Am. Chem. Soc., 2010, 132, 1903–1913 CrossRef CAS PubMed.
- N. L. Rosi and C. A. Mirkin, Chem. Rev., 2005, 105, 1547–1562 CrossRef CAS PubMed.
- A. Charrier, N. Candoni, N. Liachenko and F. Thibaudau, Biosens. Bioelectron., 2007, 22, 1881–1886 CrossRef CAS PubMed.
- J. M. Gibbs-Davis, G. C. Schatz and S. T. Nguyen, J. Am. Chem. Soc., 2007, 129, 15535–15540 CrossRef CAS PubMed.
- A. K. R. Lytton-Jean, J. M. Gibbs-Davis, H. Long, G. C. Schatz, C. A. Mirkin and S. T. Nguyen, Adv. Mater., 2009, 21, 706–709 CrossRef CAS PubMed.
- Y. Guo, D. Yao, B. Zheng, X. Sun, X. Zhou, B. Wei, S. Xiao, M. He, C. Li and H. Liang, ACS Nano, 2020, 14, 8317–8327 CrossRef CAS PubMed.
- P. Valentini, R. Fiammengo, S. Sabella, M. Gariboldi, G. Maiorano, R. Cingolani and P. P. Pompa, ACS Nano, 2013, 7, 5530–5538 CrossRef CAS PubMed.
- R. Jin, G. Wu, Z. Li, C. A. Mirkin and G. C. Schatz, J. Am. Chem. Soc., 2003, 125, 1643–1654 CrossRef CAS PubMed.
- T. Song, S. Xiao, D. Yao, F. Huang, M. Hu and H. Liang, Adv. Mater., 2014, 26, 6181–6185 CrossRef CAS PubMed.
- U. Brinkmann and R. E. Kontermann, Science, 2021, 372, 916–917 CrossRef CAS PubMed.
- C. Klein, U. Brinkmann, J. M. Reichert and R. E. Kontermann, Nat. Rev. Drug Discovery, 2024, 23, 301–319 CrossRef CAS PubMed.
- D. Slaga, D. Ellerman, T. N. Lombana, R. Vij, J. Li, M. Hristopoulos, R. Clark, J. Johnston, A. Shelton, E. Mai, K. Gadkar, A. A. Lo, J. T. Koerber, K. Totpal, R. Prell, G. Lee, C. Spiess and T. T. Junttila, Sci. Transl. Med., 2018, 10, eaat5775 CrossRef PubMed.
- J. Chavarria-Smith, C. P. C. Chiu, J. K. Jackman, J. Yin, J. Zhang, J. A. Hackney, W.-Y. Lin, T. Tyagi, Y. Sun, J. Tao, D. Dunlap, W. D. Morton, S. V. Ghodge, H. R. Maun, H. Li, H. Hernandez-Barry, K. M. Loyet, E. Chen, J. Liu, C. Tam, B. L. Yaspan, H. Cai, M. Balazs, J. R. Arron, J. Li, A. J. Wittwer, R. Pappu, C. D. Austin, W. P. Lee, R. A. Lazarus, J. Sudhamsu, J. T. Koerber and T. Yi, Sci. Transl. Med., 2022, 14, eabp9159 CrossRef CAS PubMed.
- L. Wang, H. Liang, J. Sun, Y. Liu, J. Li, J. Li, J. Li and H. Yang, J. Am. Chem. Soc., 2019, 141, 12673–12681 CrossRef CAS PubMed.
- Y. Miao, Q. Gao, M. Mao, C. Zhang, L. Yang, Y. Yang and D. Han, Angew. Chem., Int. Ed., 2021, 60, 11267–11271 CrossRef CAS PubMed.
- Y. Sun, L. Mo, X. Hu, D. Yu, S. Xie, J. Li, Z. Zhao, X. Fang, M. Ye, L. Qiu, W. Tan and Y. Yang, ACS Nano, 2022, 16, 21129–21138 CrossRef CAS PubMed.
- B. Cheng, W. Wang, T. Liu, H. Cao, W. Pan, Y. Xiao, S. Liu and J. Chen, Signal Transduction Targeted Ther., 2023, 8, 91 CrossRef CAS PubMed.
- J. Ye, J. Wu and B. Liu, Biochim. Biophys. Acta, 1878, 2023, 188866 Search PubMed.
- V. S. Drozd, A. A. Eldeeb, D. M. Kolpashchikov and D. D. Nedorezova, Nucleic Acid Ther., 2022, 32, 412–420 CrossRef CAS PubMed.
- J. Zhang, R. Sharma, K. Ryu, P. Shen, K. Salaita and H. Jo, ACS Chem. Biol., 2021, 16, 2255–2267 CrossRef CAS PubMed.
- S. C. Hu, N. Li and F. Liu, Chem. Commun., 2018, 54, 3223–3226 RSC.
- Y. Li, Y. Wang, G. Huang and J. Gao, Chem. Rev., 2018, 118, 5359–5391 CrossRef CAS PubMed.
- D. Bai, X. Zhou, W. Luo, H. Yu, S. Bai, Y. Wu, L. Song, K. Chen, Y. Xie, X. Chen, J. Zhao, Y. Fu, Y. Yang, J. Li and G. Xie, Biosens. Bioelectron., 2022, 216, 114677 CrossRef CAS PubMed.
- D. M. Kolpashchikov, Chem. Rev., 2010, 110, 4709–4723 CrossRef CAS PubMed.
- H. Masu, A. Narita, T. Tokunaga, M. Ohashi, Y. Aoyama and S. Sando, Angew. Chem., Int. Ed., 2009, 48, 9481–9483 CrossRef CAS PubMed.
- X. Yang, Y. Tang, S. M. Traynor and F. Li, J. Am. Chem. Soc., 2016, 138, 14076–14082 CrossRef CAS PubMed.
- D. N. Taylor, S. R. Davidson and L. Qian, J. Am. Chem. Soc., 2021, 143, 15567–15571 CrossRef CAS PubMed.
- T. Zhang and B. Wei, J. Am. Chem. Soc., 2021, 143, 16693–16699 CrossRef CAS PubMed.
- S. Nahar, A. K. Nayak, A. Ghosh, U. Subudhi and S. Maiti, Nanoscale, 2018, 10, 195–202 RSC.
- Z. Guo, Q. Liu and L. M. Smith, Nat. Biotechnol., 1997, 15, 331–335 CrossRef CAS PubMed.
- D. Y. Zhang, S. X. Chen and P. Yin, Nat. Chem., 2012, 4, 208–214 CrossRef CAS PubMed.
- S. X. Chen, D. Y. Zhang and G. Seelig, Nat. Chem., 2013, 5, 782–789 CrossRef CAS PubMed.
- P. S. Kwon, S. Ren, S. J. Kwon, M. E. Kizer, L. Kuo, M. Xie, D. Zhu, F. Zhou, F. Zhang, D. Kim, K. Fraser, L. D. Kramer, N. C. Seeman, J. S. Dordick, R. J. Linhardt, J. Chao and X. Wang, Nat. Chem., 2020, 12, 26–35 CrossRef CAS PubMed.
- S. Ren, K. Fraser, L. Kuo, N. Chauhan, A. T. Adrian, F. Zhang, R. J. Linhardt, P. S. Kwon and X. Wang, Nat. Protoc., 2022, 17, 282–326 CrossRef CAS PubMed.
- E. C. Wamhoff, L. Ronsard, J. Feldman, G. A. Knappe, B. M. Hauser, A. Romanov, J. B. Case, S. Sanapala, E. C. Lam, K. J. S. Denis, J. Boucau, A. K. Barczak, A. B. Balazs, M. S. Diamond, A. G. Schmidt, D. Lingwood and M. Bathe, Nat. Commun., 2024, 15, 795 CrossRef CAS PubMed.
- T. Wu, H. Wang, R. Tian, S. Guo, Y. Liao, J. Liu and B. Ding, Angew. Chem., Int. Ed., 2023, 62, e202311698 CrossRef CAS PubMed.
- J. Zheng, G. Zhu, Y. Li, C. Li, M. You, T. Chen, E. Song, R. Yang and W. Tan, ACS Nano, 2013, 7, 6545–6554 CrossRef CAS PubMed.
- J. Liu, T. Wu, X. Lu, X. Wu, S. Liu, S. Zhao, X. Xu and B. Ding, J. Am. Chem. Soc., 2019, 141, 19032–19037 CrossRef CAS PubMed.
- S. M. Douglas, I. Bachelet and G. M. Church, Science, 2012, 335, 831–834 CrossRef CAS PubMed.
- S. Li, Q. Jiang, S. Liu, Y. Zhang, Y. Tian, C. Song, J. Wang, Y. Zou, G. J. Anderson, J. Han, Y. Chang, Y. Liu, C. Zhang, L. Chen, G. Zhou, G. Nie, H. Yan, B. Ding and Y. Zhao, Nat. Biotechnol., 2018, 36, 258–264 CrossRef CAS PubMed.
- Z. Ge, H. Gu, Q. Li and C. Fan, J. Am. Chem. Soc., 2018, 140, 17808–17819 CrossRef CAS PubMed.
- I. Barrio-Hernandez, J. Yeo, J. Jänes, M. Mirdita, C. L. M. Gilchrist, T. Wein, M. Varadi, S. Velankar, P. Beltrao and M. Steinegger, Nature, 2023, 622, 637–645 CrossRef CAS PubMed.
- P. H. Yoon, Z. Zhang, K. J. Loi, B. A. Adler, A. Lahiri, K. Vohra, H. Shi, D. B. Rabelo, M. Trinidad, R. S. Boger, M. J. Al-Shimary and J. A. Doudna, Science, 2024, 385, 538–543 CrossRef CAS PubMed.
- M. Duran-Frigola, M. Cigler and G. E. Winter, J. Am. Chem. Soc., 2023, 145, 2711–2732 CrossRef CAS PubMed.
- A. Pillai, A. Idris, A. Philomin, C. Weidle, R. Skotheim, P. J. Y. Leung, A. Broerman, C. Demakis, A. J. Borst, F. Praetorius and D. Baker, Nature, 2024, 632, 911–920 CrossRef CAS PubMed.
|
This journal is © The Royal Society of Chemistry 2025 |
Click here to see how this site uses Cookies. View our privacy policy here.