DOI:
10.1039/D2EA00033D
(Critical Review)
Environ. Sci.: Atmos., 2022,
2, 563-573
Impacts of crop type, management and soil quality indicators on background nitrous oxide emissions (BNE) from Chinese croplands: a quantitative review and analysis†
Received
30th March 2022
, Accepted 21st May 2022
First published on 27th May 2022
Abstract
N2O emissions (BNE) represents emissions from unfertilised soils during the current growing season or year and these contribute to total N2O emissions. In this quantitative review and analysis, we collected 146 papers reporting BNE from 378 arable crop sites across China. The main aims were to estimate the BNE from Chinese cropland soils and to assess impacts of crop type, management and soil quality indicators on BNE. Data on sites, crops, management and soil quality indicators were collected. The data were segregated into cereal crops, rice and vegetables, and yield- and area-scaled BNE were calculated. The daily and annual BNE values (mean ± standard deviation) for cereals, rice and vegetables are 1.32 ± 1.16, 3.64 ± 3.84 and 10.68 ± 16.22 g N2O–N ha−1 and 0.98 ± 0.83, 1.11 ± 0.49 and 2.86 ± 2.33 kg N–N2O ha−1, respectively. Crop types have significant impacts (p < 0.05) on the BNE values from soils. The overall mean annual BNE for China, considering the proportion of planting area for each crop type, is 0.69 ± 1.52 kg N–N2O ha−1. The total national mean annual BNE for China is 146 ± 109 Gg N2O–N. The BNE from different crop types is variably controlled by interactions of many factors but primarily by soil quality indicators (i.e. pH, soil N, soil C), climate and management. To reduce uncertainty in the total N2O emissions from Chinese croplands, we suggest estimating the BNE at a national level, rather than using the default value of the Intergovernmental Panel on Climate Change (IPCC) of 1 kg N2O–N ha−1.
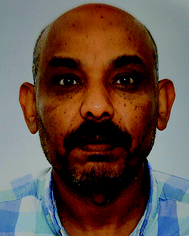 Mohamed Abdalla | Mohamed Abdalla is an Environmental Modeller at the University of Aberdeen since 2012. He got his PhD in Plant Ecophysiology, in 2006, from University of Dublin, and a postdoctoral position until 2012 thereafter. He have been working/collaborating on different national, European and international projects to conduct interdisciplinary research studies/modelling on greenhouse gas emissions from soil, carbon and N biogeochemistry, land-use change, crop production, sustainability and climate change mitigation and adaptation. |
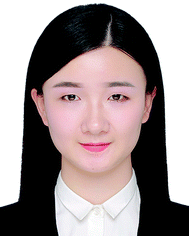 Ziyin Shang | Ziyin Shang is an assistant researcher at Institute of Crop Sciences, Chinese Academy of Agricultural Sciences. She got her master degree from Peking University, and PhD degree from the University of Aberdeen with Prof Pete Smith. She is interested in the regional estimation and mitigation of cropland greenhouse gas emissions. |
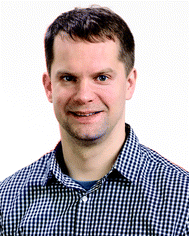 Mikk Espenberg | Mikk Espenberg is a microbiologist and environmental scientist whose main research interests include environmental microbiology, bioinformatics and greenhouse gases. Mikk completed his PhD at the University of Tartu in 2017. He works at the University of Tartu as an Associate Professor in environmental microbiology and at the University of Aberdeen as an Honorary Research Fellow in biological sciences. Mikk leads the Environmental Microbiology and Biotechnology Workgroup at the Department of Geography, Institute of Ecology and Earth Sciences of the University of Tartu. |
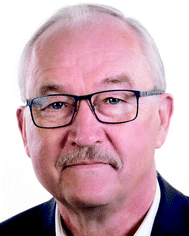 Ülo Mander | Ülo Mander (PhD in 1983 at the University of Tartu (UT), Estonia) is Professor of Physical Geography and Landscape Ecology at the Institute of Ecology & Earth Sciences, UT, Estonia. Main research interest: biogeosciences (carbon and nutrient cycling in wetlands and forests), landscape ecology, and ecological engineering (constructed wetlands). |
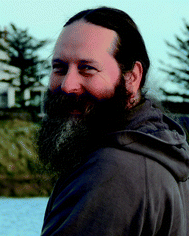 Pete Smith | Pete Smith is Professor of Soils and Global Change at the Institute of Biological and Environmental Sciences at the University of Aberdeen (Scotland, UK) and Science Director of the Scottish Climate Change Centre of Expertise (ClimateXChange). His interests include climate change mitigation, soils, agriculture, food systems, ecosystem services and modelling. He is a Fellow of the Royal Society of Biology, a Fellow of the Institute of Soil Scientists, a Fellow of the Royal Society of Edinburgh, a Foreign Fellow of the Indian National Science Academy, a Fellow of the European Science Academy, and a Fellow of the Royal Society (London). |
Environmental significance
Nitrous oxide (N2O) is a potent greenhouse gas that causes climate change and depletes ozone layer. It emits, mainly, from fertilised soils however, emission from unfertilised soils (background nitrous oxide emission; BNE) is significantly contributing to the total N2O emissions. In this study, the impacts of many factors including crop types, management and soil quality indicators on the BNE from Chinese cropland were investigated. This is essential to reduce uncertainty in the total N2O emissions, support decision-makers to advise on the best possible management strategies for mitigating N2O emissions and reducing the impact of croplands on climate change.
|
1. Introduction
Background N2O emissions (BNE) are emissions from soils that have received no N fertiliser during the current growing season or year.1,2 BNE contribute to the total N2O emissions and are vital for accurately quantifying N2O emission factors from agricultural and natural ecosystems.3–5 A review study highlighted the importance of including the non-growing season when compiling national N2O inventories and selecting mitigation strategies.6 Therefore, it is essential to quantify the BNE throughout the year. Previous studies reported annual BNE values for Chinese croplands range from 0.93 to 1.43 kg N–N2O ha−1.7–9 Moreover, other studies found that BNE from some arable crops in China accounted for 5–41% and 26–30% of total fertilised treatments N2O flux, respectively.10,11 Aliyu et al.12 reported that climate change during this century is gradually increasing total BNE for Chinese croplands from 120.59 ± 0.69 to 142.42 ± 0.78 Gg N year−1. Furthermore, the BNE played an important role in estimating total N2O emissions, especially for dry land.9 However, globally, a BNE value of 1.1 kg N2O–N ha−1 for croplands was reported by.13 The IPCC set an overall annual default value of 1 kg N2O–N ha−1 for BNE.1,14 However, many countries, e.g. Japan, Denmark, the Netherlands, Australia, Canada, Sweden and Germany, have BNE values for croplands, which are higher/lower than the IPCC default value.8,15 This could lead to an under/over-estimation of the total N2O emissions from croplands. Further, to avoid uncertainty, the BNE from Chinese croplands should be estimated for each crop type separately and proportion of planting areas should be considered when BNE is calculated nationally.
In China, farmers often overuse synthetic N fertiliser by 30 to 40%,16 to maximise crop yields, and sometimes apply low-efficiency N fertilisers.17–19 Such activities may result in higher BNE values in subsequent seasons/years. However, this overuse of N fertilizer has recently declined due to the government policy of zero growth in N fertilizer and pesticide and the widespread use of nationwide technological applications.20,21 Additionally, human activities that disturb soils and change their properties (e.g. bulk density (BD) and soil microclimate (e.g. soil temperature and moisture)) can stimulate the BNE.22 Moreover, application of animal manure in croplands can significantly increase BNE. In a long-term experiment using compost, Ding et al.2 observed an increase of 106% in the BNE values from croplands.
Soil characteristics, climate and management parameters that impact soil nitrification and denitrification rates play an important role in determining the BNE values (Smith et al., 2008).13 found that the main drivers for BNE were soil pH, soil N, atmospheric N deposition, soil volumetric water content, and soil temperature. However, an important factor underpinning these processes is the mineralization of soil organic matter (SOM), which contributes to the microbially available N pool through the release of NH3/NH4+.3 Abdalla et al.23 found that peak N2O emission from control plots (i.e. zero N fertiliser) occurred when high air temperature and precipitation were observed. Under continuously anaerobic conditions, BNE are reduced to N2 before being released to the atmosphere.24 Emissions of BNE vary across sites and seasons, because they are strongly dependent on soil properties, management practices and climatic conditions.7,25
Previous studies found that crop types have great impacts on BNE due to different crop management, quantities and qualities of yield and crop residues.26 Higher annual BNE rates from Chinese field vegetables, compared to other crop systems, were reported by many studies (e.g. 7, 9). However, Min et al.27 reported seven times greater N2O emissions from greenhouse vegetables compared to a rice-wheat system in the same area and on the same soils. Moreover, Powlson et al.20 and Wang et al.28 noted the need for detailed high resolution N2O emission data by crop type, land use and technology development to clarify the change in the emissions and inform the progress towards realistic and cost-effective mitigation pathways. Investigating the impacts of crop type, management and soil quality indicators on BNE from Chinese croplands is crucial to reduce uncertainty and have accurate national and regional inventories for total N2O emissions. The main aim of this quantitative review and analysis is to estimate annual and total BNE and investigate the impacts of crop type for the Chinese cropland soils. Likewise, we assess the effects of soil quality indicators and management on BNE from soils.
2. Materials and methods
2.1. Data collection
This review covered the main arable crops in China (i.e. wheat, maize, rice and vegetables). We performed a comprehensive search on the Web of Science database (accessed between November 2019 and February 2022) using the keywords: background N2O, nitrous oxide, croplands, greenhouse gas emissions and China. To assess publication bias we also searched for any unpublished or grey literature data. To have the best possible coverage, we also checked all references in the papers found in the Web of Science search. The BNE data collected covered all studies with annual, seasonal and rotational cumulative fluxes. Two types of data were excluded from this quantitative review and analysis: (i) observations without replicated measurements of BNE, (ii) data from laboratory, pot or greenhouse experiments. Our searches resulted in 146 published papers investigating BNE and the influence of soil properties and environmental factors on emissions but no un-published or grey literature data were found. We divided the studies into three categories depending on crop type: cereals (wheat and maize), rice and vegetables. Although rice is a cereal, it was put in a separate category due to the differences in its growth and management practices. These studies were carried out at 378 sites (119 on cereals, 94 on rice and 61 on vegetables) from different crops, management systems and agricultural regions across China (Fig. 1 and Table S1†). The typical growing seasons for maize, wheat and rice are 114, 245 and 121 days. The studies with 330 days or more durations were considered full years. We calculated the annual flux for each crop type when not given by the original papers. Here, the average daily BNE from studies that have annual flux26 was multiplied by the length of the fallow period and added to the seasonal flux. We considered the minor errors acceptable, as no N fertilisers were involved. The environmental (average temperature and precipitation) and management (previous N fertiliser rate, tillage, irrigation, liming and drainage) factors for each crop were also analysed separately. Coordinates, annual mean climatic conditions as well as soil characteristics, cumulative BNE (kg N ha−1), study duration, replication and grain yield (t ha−1) are shown in Table S1†.
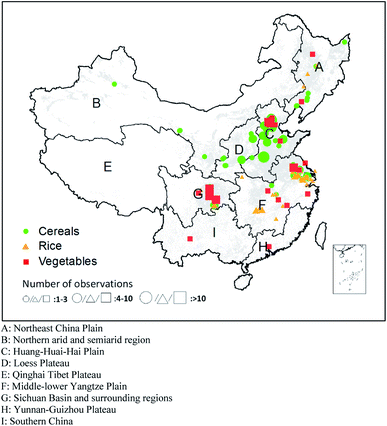 |
| Fig. 1 Map showing the main agroecosystem regions of China with locations of experimental sites of cereal, rice and vegetables considered in this paper. | |
2.2 Estimation of total BNE
We used the annual average area of croplands in China, 2000–2019 of approximately 110 million hectares (62, 30 and 18 million ha for cereals, rice and vegetables, respectively) to extrapolate the overall annual BNE and the total national annual BNE.29 We used the proportional planting area of each crop type to calculate an overall national annual BNE for China.
2.3 Methods for measuring BNE, microclimate and soil properties
All BNE data were derived using the closed static chamber method. Static chambers of different sizes (range from 0.02 to 0.8 m3), shapes (square, round) and materials (plastic, steel), were applied. Measurement periods of BNE ranged from one season to one year with 3 to 5 replications. Microclimate data (i.e. temperature, precipitation) were collected from weather stations at the field sites or sites nearby. Different methods were applied to quantify soil pH (e.g. pH probe or meter in deionized water or 0.01 M CaCl2 in 1
:
1 and 1
:
2 or 1
:
5 (v/v)) soils: solution ratios. For the different studies, different methods were used to measure soil pH, for example, using a pH probe or meter in deionised water or 0.01 M CaCl2 in 1
:
1 and 1
:
2 or 1
:
5 v/v soil:solution ratios. We made no adjustment for analytical methods used for soil pH, and where a range of values were reported, we took the arithmetic mean.
2.4 Yield- and area-scaled total emissions of BNE
We have calculated the yield- and area-scaled of BNE. The yield-scaled BNE are the N2O emissions as a function of crop yield (i.e. BNE produced per unit of grain yield) whilst the area-scaled BNE are the N2O emissions as a function of area (i.e. BNE produced per ha). To estimate the yield-scaled emissions for cereals, rice and vegetables we divided the average annual BNE by the average annual grain yield in each case. The yield- and area-scaled total emissions, in kg CO2 eq. Mg−1 and or kg CO2 eq. ha−1, respectively, of BNE were calculated using the global warming potential (GWP) over a 100 year time horizon of 273.30
2.5 Data analysis
We used R, version 4.1.2, to explore, harmonise, analyse and visualise the data.31 Missing data patterns (i.e. 11% of the data; Table S2 and Fig. S1†) were identified and explored using the packages “naniar” version 0.6.132 and “VIM” version 6.1.1.33 The package “missRanger” version 2.1.3 algorithm was used to impute missing values34 and it was repeated 99 times to account for uncertainties in predictions. Further, we performed 100 replications of the random forest analysis using the package “Boruta” version 7.0.035 to determine which of the climate, soil, plant and management parameters best predicted the change in BNE. A linear mixed-effects model (LMM) was applied to test differences in daily and annual and national BNE, yield- and area-scaled emissions between crop categories using the “lmer” method (version 1.1-27.1),36 while p-values were calculated in order to confirm the significance of the relationships using the “lmerTest” package version 3.1-3.37 We performed LMMs of different variables against the logarithm of annual BNE. Only comparable studies were selected through the comprehensive literature research described in Sections 2.1 and 2.2. In addition, different scaling methods were used to normalise data (see Section 2.3). LMM approach was applied for the meta-analysis of the collected data following the principles of ref. 38. To evaluate the relationships between annual background nitrous oxide emissions and environmental parameters, the maximal information-based nonparametric exploration (MINE) approach was applied and the maximal information coefficient (MIC) was calculated39 using the package “minerva” version 1.5 × 10.40 MIC captures relationship strength, and the higher MIC-R2 is, the more non-linear is the relationship.
3. Results and discussion
3.1 Daily, annual and total national BNE from Chinese croplands
Unlike previous studies which compared the BNE of different land use systems, in this quantitative review and analysis, we investigated the impacts of crop type, management and soil quality indicators on the BNE. As shown in Fig. 1, most of the data collected were from the central and eastern parts of China, where most of the arable lands are located. However, although soil can act as a source or a sink for BNE,41 all data collected in this study represent a source (Table S1†). This reflects the importance of croplands as a source of BNE. Most daily BNE values were ≤5 g N2O–N ha−1 with few ≥10 g N2O–N ha−1 or ≤1 g N2O–N ha−1 (Fig. 2). The calculated daily average BNE values (mean ± standard deviation; g N2O–N ha−1 day−1) were 1.32 ± 1.16 for cereals, 3.64 ± 3.84 for rice and 10.68 ± 16.22 for vegetables. The annual BNE for cereals, rice and vegetables were 0.98 ± 0.83, 1.11 ± 0.49 and 2.86 ± 2.33 kg N–N2O ha−1, respectively (Table 1). In both cases (daily and annual), the BNE values from vegetables were statistically significantly higher (p < 0.05) compared to those from cereals and rice crops. Thus, using the IPCC default BNE value of 1 kg N2O–N can result in significant underestimations of total N2O from vegetables in China. The overall average annual BNE value from Chinese croplands without considering the proportion of planting area was 1.6 ± 1.2 kg N–N2O ha−1. However, considering the proportion of annual planting areas of 62, 30 and 18 million ha for cereals, rice and vegetables, respectively, resulted in a significant lower overall annual BNE value of 0.69 ± 0.52 kg N–N2O ha−1. This highlights the importance of considering crop type and the proportion of planting area when calculating national BNE. Previous studies reported annual BNE values for Chinese agriculture range from 0.93 to 1.43 kg N–N2O ha−1.7,8 Higher annual BNE values of 4.7 and 5.89 kg N2O–N ha−1 from Chinese rice paddy and dry land, respectively, were also reported.9 Additionally, Aliyu et al.12 calculated a BNE value of 0.53
kg N ha−1 year−1 for the cold temperate zone, and Gu et al.42 reported a value of 1.35 kg N–N2O ha−1 for the cultivated mineral soils in China. However, global annual BNE values (kg N–N2O ha−1) of 1.42 for vegetable/fruits crops, 1.06 for cereal fields and 0.82 for rice paddy were reported.13 The differences between these studies were due to the data, approaches and methods used.11 Most of these estimated annual BNE rates are greater than the IPCC annual default value of 1 kg N2O–N ha−1 (https://www.sciencedirect.com/science/article/pii/S1352231012002154). However, our calculated annual BNE rate from Chinese croplands is lower than the IPCC default value and than those calculated for croplands in Japan (2.20), Denmark (2.95) and the Netherlands (4.56), Australia (1.25), Canada (1.15), Sweden (1.03) and Germany (1.27).8,15 The BNE is a sensitive factor and an essential source for creating an inventory of cropland N2O emissions.3 Sapkota et al.10 and Gu et al.11 reported that the total BNE represent 5–41% and 26–30% of total fertilised treatments N2O flux from arable crops in China, respectively. The total national annual BNE for Chinese croplands is estimated to be 146 ± 109 Gg N2O–N. This falls within the previously estimated range of 92 to 184 Gg N2O–N.12,13,42,43 Albeit, national annual BNE is expected to gradually increase during this century due to the impacts of climate change12 unless an efficient strategy to mitigate the flux is introduced.
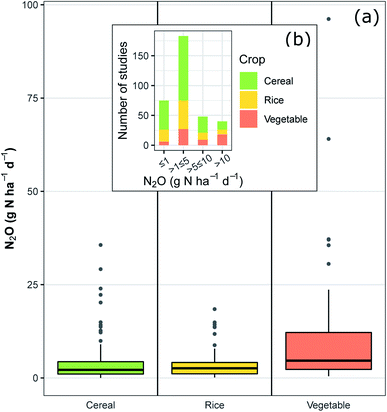 |
| Fig. 2 (a) Boxplot of daily background nitrous oxide emissions (BNE) (g N2O–N) from different crop categories (cereals, rice and vegetables) and (b) histogram of distribution of the daily BNE at these crop categories. | |
Table 1 Statistical comparisons (LMM) of background nitrous oxide emissions (BNE) from the cereal, rice and vegetable crops. N is the number of studies; stdev is the standard deviation. Horizontal values with different letters are statistically significantly different (p < 0.05)
BNE |
Cereals |
Rice |
Vegetables |
Mean |
stdev |
N
|
Mean |
stdev |
N |
Mean |
stdev |
N
|
Daily BNE (g N2O–N) |
3.82b |
4.98 |
198 |
3.64b |
3.84 |
88 |
10.68a |
16.22 |
60 |
Annual BNE (kg N2O–N) |
0.98b |
0.83 |
197 |
1.11b |
0.49 |
94 |
2.86a |
2.34 |
61 |
National BNE (Gg N2O–N) |
60.94a |
51.62 |
197 |
32.82c |
14.48 |
94 |
52.52b |
42.94 |
61 |
Area-scaled BNE (kg CO2 eq. ha−1) |
246.6c |
154.9 |
94 |
275.3b |
131.2 |
46 |
784.4a |
710.9 |
19 |
Yield-scaled BNE (kg CO2 eq. Mg−1) |
79.10b |
88.79 |
94 |
60.41c |
102.1 |
46 |
83.46a |
208.2 |
19 |
3.2 Impacts of crop type on the BNE
In this study, significantly higher (p < 0.05) BNE values were observed from vegetables compared to other crop types (Table 1). Management of frequent irrigation and higher amounts of applied previous N fertiliser rates in vegetable crops result in higher N residues in the soils and consequently, higher BNE.7
Our dataset showed that the average annual previous N fertiliser (kg N ha−1) amounts applied in vegetables were 405 compared to 230 and 220 for cereals and rice crops, respectively. This increases NO3− concentration in the soil, which positively correlated with BNE.44 Moreover, higher annual BNE rates from Chinese vegetables, compared to other crop systems, were reported by many studies.7,9,12 However, Zhang et al.45 reported an extremely high annual BNE rate of 5.1 kg N2O–N ha−1 for Chinese vegetables. They argued that this was due to management especially higher amount of previous N fertiliser. Wang et al.7 found the BNE accounted for about 29.3% of the total direct N2O emissions from vegetable fields in China. These higher BNE in combination with negligible amounts of soil organic carbon addition to soil by vegetable, as most of their biomass is removed from the fields, could have serious impacts on climate change.46 Nonetheless, the BNE from vegetables could be mitigated by optimising irrigation and N fertiliser rate and by using high-efficiency N fertilisers. The BNE values from cereals and rice are different but not statistically significantly different. However, in some regions, e.g. north China plain, where N fertiliser is overused or a low-efficiency fertiliser is applied in wheat and maize, significant N can accumulate in soils and result in higher BNE in the subsequent years.47,48 The BNE from flooded rice are sometimes lower and can be even negative because of complete denitrification.49
The total national annual BNE from cereals, rice and vegetables of 60.9 ± 51.6, 32.8 ± 14.5 and 52.5 ± 42.9 Gg N2O–N, respectively, were statistically significantly different (p < 0.05) (Table 1). This is mainly due to the differences in the annual BNE value and area covered by each crop type. The contributions of the three crop categories to the total national BNE are 42%, 22% and 36% for the cereals, rice and vegetables, respectively. Thus for better estimation of total N2O emissions the BNE should be calculated for each crop type separately. The BNE yield- and area-scaled emissions (kg CO2 eq. ha−1 or kg CO2 eq. Mg−1, respectively) for the cereals, rice and vegetables are shown in Table 1. The yield-scaled BNE of 84 ± 208 kg CO2 eq. Mg−1 for vegetables was significantly different (p < 0.05) from the value of 60 ± 102 kg CO2 eq, Mg−1 for rice but not significantly different from the value of 79 ± 88 kg CO2 eq, Mg−1for cereals. Here, as already mentioned, each crop has different management, quantities and qualities of yield and crop residues, which affected the BNE differently.26 The significantly higher area-scaled BNE of 784 ± 711 kg CO2 eq. ha−1 was from vegetables compared to 247 ± 155 and 275 ± 131 kg CO2 eq. ha−1 for cereals and rice, respectively. Similar results were reported by others (e.g. 7, 9, 12). Moreover, although not investigated in this study, it is crucial to quantify and include BNE in the calculations of the emission factor (EF) even for the period of the non-growing season, as an accurate estimation of BNE is essential for these calculations. In a review study, Shang et al.6 reported increases of 19% and 11% in the EF of vegetables and rice, respectively, when they included the non-growing season period in their calculations. Furthermore, Wang et al.28 re-assessed global fertilised cropland-N2O emissions from 1961 to 2014 using EFs upscaled from field measurements. Their results agreed with the IPCC Tier 1 default EFs for upland crops in 1990–2014, but gave ∼15% lower EF for the period1961-1989. However, they calculated a ∼67% larger EF for paddy rice over the period 1961–2014. The global crop-specific N2O mitigation potential from the decrease in N application rate without compromising crop production of maize, wheat, rice or other crops was investigated.50 They found that the N2O mitigation potential for maize, wheat, rice and other crops were 54%, 43%, 69% and 65%, respectively.
3.3 Impacts of environmental and management factors on BNE
The BNE are primarily the results of biological processes in soils and are controlled by complex interactions between many parameters (climate, soil, crop and management). The importance of these parameters in estimating BNE were investigated in this study. The ranking of each parameter shows how important it is for predicting the BNE from soils under each crop category (Fig. 3). Additionally, the statistically significant relationships (p < 0.05) represented by MIC and measure of the MIC-R2 values between annual BNE and environmental parameters for each crop category were also presented in Table 2. Depending on the data availability, varying degrees of significant associations between the different parameters e.g. pH, BD, soil texture, soil organic carbon, soil nitrogen, previous N fertiliser, yield and air temperature, management and BNE from each crop category were found. Yin et al.13 reported the importance of soil pH, N mineralization and soil temperature in driving BNE from soils. Moreover, Conrad51 found the degree of spatial and temporal variation in N2O emissions depends on interactions between many factors, e.g. soil type, land use and climatic factors. The amounts of N and C in the soils have a significant influence on the value of BNE. Availability of larger amounts of soil N increase nitrification/denitrification processes and results in higher BNE. The soil N also interacts with other soil properties like soil organic carbon, pH and BD and positively affects the BNE.8 The field historical N fertiliser application influences the BNE amounts due to residual N effects. LaHue et al.52 found a strong relationship between historical N fertiliser use and N2O emissions. They reported that higher extractable and potentially mineralizable N was positively correlated with N2O emissions. Abdalla et al.53 and Zhao et al.54 found that Chinese optimized and alternative management cropping systems in which less N fertiliser is used, can decrease total N2O emissions and increase grain yield compared to the conventional cropping management.
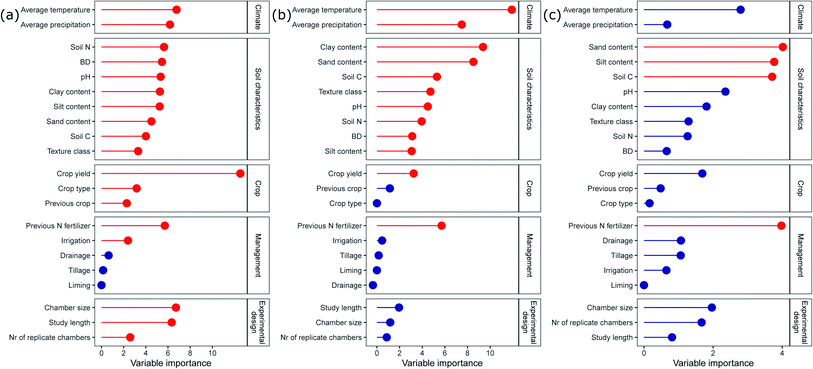 |
| Fig. 3 Random forest analysis results for cereals (a) (n = 119), rice (b) (n = 49), and vegetables (c) (n = 39) by different variable's categories (climate, soil characteristics, crop, management, experimental design) describing the importance of variables for changes in annual background nitrous oxide emissions (BNE). The resulting median of importance was used to order parameters from highest to lowest. The variables in red have statistically significant (p < 0.05) contributions. | |
Table 2 Statistically significant relationships (p < 0.05) represented by maximal information coefficient (MIC) and measure of the non-linearity of the relationship (MIC-R2) values between annual background nitrous oxide emissions (BNE) and environmental parameters for the cereal, rice and vegetable crops. MIC captures relationship strength, and the higher MIC-R2 is, the more non-linear the relationship is. N is the number of studies
Parameters |
Cereal |
Rice |
Vegetable |
MIC |
MIC-R2 |
N
|
MIC |
MIC-R2 |
N |
MIC |
MIC-R2 |
N
|
Air temperature |
0.48 |
0.37 |
167 |
|
|
|
|
|
|
pH |
0.37 |
0.19 |
195 |
|
|
|
0.50 |
−0.39 |
59 |
BD |
0.33 |
0.30 |
176 |
0.40 |
0.37 |
68 |
0.50 |
0.34 |
56 |
Silt content |
0.42 |
0.26 |
115 |
|
|
|
|
|
|
Sand content |
0.39 |
0.38 |
116 |
|
|
|
|
|
|
Clay content |
0.30 |
0.19 |
176 |
|
|
|
|
|
|
Soil C |
0.32 |
0.07 |
192 |
0.40 |
0.23 |
81 |
|
|
|
Soil N |
0.38 |
0.18 |
161 |
|
|
|
|
|
|
Previous N fertilizer |
0.31 |
0.29 |
163 |
|
|
|
0.59 |
0.51 |
54 |
Crop yield |
0.47 |
0.31 |
94 |
|
|
|
0.59 |
0.18 |
19 |
Study length |
0.61 |
0.58 |
197 |
0.39 |
−0.02 |
78 |
|
|
|
Chamber size |
0.34 |
0.10 |
152 |
|
|
|
0.50 |
−0.39 |
43 |
The disturbance of BD and soil temperature by human activities like tillage can result in higher BNE.22 A simulation study found that both measured and simulated BNE from the control plots increased with increasing BD.55 According to Abbasi and Adams,56 high BD reduces macro pore spaces and increases water filled pore space thereby, stimulating soil microbial activity. Additionally, increasing soil C stimulates N2O emissions.55 Soils with higher clay content usually preserve high soil moisture, which is favourable for higher BNE production by nitrification, or denitrification.42,57 In contrast, Xu et al.58 found higher clay content reduced BNE diffusion from micro-sites to the atmosphere.
We found higher crop yield and the addition of higher amount of biomass to soils by the crop significantly increases BNE. This is due to the regulating process of soil C, N availability, and microbial activities.59 A review study noticed that crop residue decreased N2O emissions by 17.3% in the flooded rice but increased them by 21.5% for upland crops.60 In contrast, other studies, e.g. Ma et al.,61 reported some inhibitory effects of crop residues. High temperature is also an important parameter that increases the BNE by increasing the emission rate of microbial activity in the soils or because of thawing/freezing events.8,62 Temperature also increases the decomposition of soil organic matter and microbial responses to other management and environmental parameters (e.g. rainfall).63,64 Under higher temperatures the respiratory sink for O2 increases, leading to a higher anaerobic fraction and, thereby, higher BNE.65 This suggests that global warming will have strong and clear positive feedback on the BNE from Chinese croplands.12 However, the effects of temperature on N2O emissions in a fertilised field can be masked by the applied N fertiliser.66 Further, the experimental parameters like study length and number of replicates increase the precision of measurements and play an important role in the accuracy of the BNE values. In a N2O study, Flechard et al.67 compared longer N2O measurements with shorter duration ones and found that spatial and temporal variability may contribute to uncertainties of ±50% in the annual flux estimates. Thus, it is important to understand all these factors to develop efficient mitigation strategies and select an appropriate model for accounting and reporting of the BNE and total N2O from Chinese croplands.68
This study findings confirm that to mitigate BNE from Chinese croplands, it is important to follow the nutrients management guidelines (4Rs: right source, right rate, right time, and right place). Adoption by farmers of a suitable fertiliser source and amount that matches crop needs and soil properties would increase nutrient use efficiency and decrease the total N2O emissions. Moreover, application of fertilisers at the right time in the crop root zone and considering crop demands, weather and seasonal conditions would allow the crop to successfully access the nutrients, improve yields and benefit the environment.69
3.4 Limitations
Estimation of uncertainty is of crucial importance since it has a large impact on management decisions. It needs to be considered by policy makers to develop strategies and policies for emission reductions and to track the progress of these policies. One limitation that may increase the uncertainty in this analysis is that the majority of the N2O studies included were short-term experiments (1–2 years), as long-term experiments for a single crop in Chinese croplands are scarce in the literature. The multiple year-studies included had more than one crop. Some of the control treatments received N fertiliser in previous season/year and only not fertilised in the present measurement year, which may overestimated BNE. Moreover, estimation of annual BNE when not given in the original papers and the different experimental conditions and common limitation of unbalanced sampling and geographical distribution of sites might also increase the uncertainty. Only a few studies from the Northwest of the country fulfilled our search criteria. However, pooling observations from different conditions and geographical distributions to obtain a general understanding has been widely used in meta-analysis studies70,71. Further, no publication bias had been assessed. Here, we found it challenging to access unpublished or grey literature data due to scarcity of these data. However, unlike other research discipline such as medicine, there is no evidence that publication bias is a significant issue in climate change science,72 although, Michaels73 and Reckova and Irsova74 reported some publication bias when using a small set of pre-defined Journals or a small sample size of only 16 studies, respectively. Additionally, we have used data from a range of Journals some of them have low impact factors such as Journal of Plant Nutrition and Soil Science (2.083) whilst others have high impact factors such as National Science Review (17.275). To reduce uncertainty in the total N2O emissions from the Chinese croplands, accurate estimates of both direct N2O emissions from N fertiliser and BNE are required. We have conservatively estimated the uncertainty, in this analysis, due to these limitations and other approximations and assumptions incorporated in the methods by calculating the standard deviation for all values.
4. Conclusions
This quantitative review and analysis show that crop types have a significant impact (p < 0.05) on the values of BNE from cropland soils. Daily, annual, yield- and area-scaled BNE from vegetables are all significantly higher (p < 0.05) than those from other crop types. This could be mitigated by reducing the amounts of previous N fertiliser and irrigation. Accordingly, it is important to estimate BNE for each crop type separately and consider the proportion of planting area for national BNE calculations. This is essential to correctly estimate BNE for croplands and reduce uncertainty in the total N2O emissions. It will also support decision-makers to advise on the best possible management strategies for mitigating N2O emissions and reducing the impact of croplands on climate change. The BNE is controlled by interactions of different parameters but mostly by soil quality indicators (i.e. pH, soil N, soil C), climate and management. To mitigate BNE, we suggest that all these factors should be well considered.
Author contributions
Mohamed Abdalla: conceptualization, methodology, investigation, resources, formal analysis, visualization, writing – original draft. Ziyin Shang: resources, visualization, writing – review & editing. Mikk Espenberg: methodology, formal analysis, visualization, writing – review & editing. Ulo Mander: writing – review & editing. Xiaoqing Cui: resources. Pete Smith: writing – review & editing.
Conflicts of interest
There are no conflicts to declare.
Acknowledgements
This work contributes to the N-Circle project (grant number BB/N013484/1) and SuperG (funded under EU Horizon 2020 programme).
References
- A. F. Bouwman, Direct emission of nitrous oxide from agricultural soils, Nutr. Cycling Agroecosyst., 1996, 46, 53–70 CrossRef CAS.
- W. Ding, J. Luo, J. Li, H. Yu, J. Fan and D. Liu, Effect of long-term compost and inorganic fertilizer application on background N2O and fertilizer-induced N2O emissions from an intensively cultivated soil, Sci. Total Environ., 2013, 465, 115–124 CrossRef CAS PubMed.
- A. F. Bouwman, L. J. M. Boumans and N. H. Batjes, Modeling global annual N2O and NO emissions from fertilized fields, Global Biogeochem. Cycles, 2002, 16(4), 1080 Search PubMed.
-
C. A. M. de Klein, R. J. Eckard and T. J. van der Weerden, 2010. Nitrous oxide emissions from the N cycle in livestock agriculture: Estimation and mitigation, in Nitrous Oxide and Climate Change, Ed.,K. A. Smith. pp. 107–142 Search PubMed.
- D. Fowler, M. Coyle, U. Skiba, M. A. Sutton, J. Neil Cape, S. Reis, L. J. Sheppard, A. Jenkins, B. Grizzetti, J. N. Galloway, P. Vitousek, A. Leach, A. F. Bouwman, K. Butterbach Bahl, F. Dentener, D. Stevenson, M. Amann and M. Voss, The global nitrogen cycle in the twenty-first century, Philos. Trans. R. Soc., B, 2013, 368(1621), 20130164 CrossRef PubMed.
- Z. Y. Shang, M. Abdalla, M. Kuhnert, F. Albanito, F. Zhou, L. L. Xia and P. Smith, Measurement of N2O emissions over the whole year is necessary for estimating reliable emission factors, Environ. Pollut., 2020, 259, 113864 CrossRef CAS PubMed.
- J. Wang, Z. Xiong and X. Yan, Fertilizer-induced emission factors and background emissions of N2O from vegetable fields in China, Atmos. Environ., 2011, 45, 6923–6929 CrossRef CAS.
- D. G. Kim, D. Giltrap and G. Hernandez-Ramirez, Background nitrous oxide emissions in agricultural and natural lands: a meta-analysis, Plant Soil, 2013, 373, 1007–1008 CrossRef CAS.
- J. Chen, W. Cao, Y. Li, D. Cao and F. Wang, Estimating nitrous oxide emission flux from arable lands in China using improved background emission and fertiliser-induced emission factors, Atmos. Pollut. Res., 2015, 6, 343–350 CrossRef CAS.
- T. B. Sapkota, L. K. Singh, A. K. Yadav, A. Khatri-Chhetri, H. S. Jat, P. C. Sharma, M. L. Jat and C. M. Stirling, Identifying optimum rates of fertilizer nitrogen application to maximize economic return and minimize nitrous oxide emission from rice-wheat systems in the Indo-Gangetic Plains of India, Arch. Agron. Soil Sci., 2020, 66(14), 2039–2054 CrossRef CAS.
- J. X. Gu, X. H. Zheng and W. Zhang, Background nitrous oxide emissions from croplands in China in the year 2000, Plant Soil, 2009, 320, 307–320 CrossRef CAS.
- G. Aliyu, A. Sanz-Cobena, C. Müller, M. Zaman, J. Luo, D. Liu, J. Yuan, Z. Chen, Y. Niu, A. Arowolo and W. Ding, A meta-analysis of soil background N2O emissions from croplands in China shows variation among climatic zones, Agric., Ecosyst. Environ., 2018, 267, 63–73 CrossRef CAS.
- Y. Yin, Z. Wang, X. Tian, Y. Wang, J. Cong and Z. Cui, Evaluation of variation in background nitrous oxide emissions: A new global synthesis integrating the impacts of climate, soil and management conditions, Glob. Change Biol., 2022, 28, 480–492 CrossRef PubMed.
-
P. Forster, V. Ramaswamy, P. Artaxo, T. Berntsen, R. Betts, D. Fahey, J. Haywood, J. Lean, D. Lowe and G. Myhre, 2007. Changes in atmospheric constituents and in radiative forcing in, Climate Change 2007: the Physical Science Basis, ed.,Solomon S., Qin D., Manning M., Chen Z., Marquis M., Averyt K. B., Tignor M. and Miller H. L., 129–234 Search PubMed.
- H. F. Jungkunst, A. Freibauer, H. Neufeldt and G. Bareth, Nitrous oxide emissions from agricultural land use in Germany- a synthesis of available annual field data, J. Plant Nutr. Soil Sci., 2006, 169, 341–351 CrossRef CAS.
-
D. Norse, SAIN. Improved Nutrient Management in Agriculture - a Neglected Opportunity for China's Low Carbon Growth Path, Policy Brief No. 1. Sustainable Agricultural Innovation Network, 2011, http://www.eu-china.net/upload/pdf/materialien/11-02-11_PolicyBriefNo1updatedfinal.pdf, Accessed on the 2nd of April 2022 Search PubMed.
- H. Li, J. Qiu, L. Wang, M. Xu, Z. Liu and W. Wang, Estimates of N2O emissions and mitigation potential from a spring maize field based on DNDC model, J. Integr. Agric., 2012, 11(12), 2067–2078 CrossRef.
- J. Li, E. L. Van Bueren, J. Jiggins and C. Leeuwis, ‘Farmers’ adoption of maize (Zea mays L.) hybrids and the persistence of landraces in Southwest China: implications for policy and breeding, Genet. Resour. Crop Evol., 2012, 59, 1147–1160 CrossRef.
- K. Y. Li, Y. Y. Zhao, X. L. Yuan, H. B. Zhao, Z. H. Wang, H. X. Li and S. S. Malhi, Comparison of factors affecting soil nitrate nitrogen and ammonium nitrogen extraction, Commun. Soil Sci. Plant Anal., 2012, 43(3), 571–588 CrossRef CAS.
-
D. Powlson, D. Norse and Y. Lu, SAIN. Agricultural Development in China-Environmental Impacts, Sustainability Issues and Policy Implications Assessed through China-UK Projects under SAIN (UK-China Sustainable Agriculture Innovation Network), 2008 e 2017. https://file:///G:/DNDC%20trail/Corrections%20from%20the%20Chinese/SAIN,%20%202018.pdf, 2018, (Accessed 25 April 2022) Search PubMed.
- Z. Y. Shang, F. Zhou, P. Smith, E. Saikawa, P. Ciais, J. F. Chang, H. Tian, S. J. Del Grosso, A. Ito, M. Chen, Q. Wang, Y. Bo, X. Cui, S. Castaldi, R. Juszczak, Å. Kasimir, V. Magliulo, S. Medinets, V. Medinets and S. Sabbatini, Weakened growth of cropland-N2O emissions in China associated with nationwide policy interventions, Glob. Change Biol., 2019, 25, 3706–3719 CrossRef PubMed.
-
S. Saggar, J. Luo, D.-G. Kim and N. Jha,. 2011. Intensification in pastoral farming: impacts on soil attributes and gaseous emissions. in, Soil Health and Climate Change (Soil Biology Series), ed., B. P. Singh, A. Cowie and Y. Chan, Springer-Verlag. 207–236 Search PubMed.
- M. Abdalla, X. Song, X. Ju, C. F. E. Topp and P. Smith, Calibration and validation of the DNDC model to estimate nitrous oxide emissions and crop productivity for a summer maize-winter wheat double cropping system in Hebei, China, Environ. Pollut., 2020, 262, 114199 CrossRef CAS PubMed.
- E. A. Davidson, M. Keller, H. E. Erickson, L. V. Verchot and E. Veldkamp, Testing a conceptual model of soil emissions of nitrous and nitric oxides, Bioscience, 2000, 50, 667–680 CrossRef.
- C. Wagner-Riddle, A. Furon, N. L. Mclaughlin, I. Lee, J. Barbeau, S. Jayasundara, G. Parkin, P. Von Bertoldi and J. Warland, Intensive measurement of nitrous oxide emissions from a corn-soybean-wheat rotation under two contrasting management systems over 5 years, Glob. Change Biol., 2007, 13, 1722–1736 CrossRef.
- K. E. Dobbie, I. P. McTaggart and K. A. Smith, Nitrous oxide emissions from intensive agricultural systems: Variations between crops and seasons, key driving variables, and mean emission factors, J. Geophys. Res.: Atmos., 1999, 104, 26891–26899 CrossRef CAS.
- J. Min, W. Shi, G. Xing, D. Powlson and Z. Zhu, Nitrous oxide emissions from vegetables grown in a polytunnel treated with high rates of applied nitrogen fertilizers in Southern China, Soil Use Manage., 2012, 28, 70–77 CrossRef.
- Q. Wang, F. Zhou, Z. Shang, P. Ciais, W. Winiwarter, R. B. Jackson, F. N. Tubiello, G. Janssens-Maenhout, H. Tian, X. Cui, J. G. Canadell, S. Piao and S. Tao, Data-driven estimates of global nitrous oxide emissions from croplands, Natl. Sci. Rev., 2020, 7, 441–452 CrossRef CAS PubMed.
-
National Bureau of Statistics, 2021. Acess at: National Bureau of Statistics of China (stats.gov.cn).
-
IPCC, The Physical Science Basis. Contribution of Working Group I to the Sixth Assessment Report of the Intergovernmental Panel on Climate Change, Cambridge University Press, 2021 Search PubMed.
-
R Core Team, R: A Language and Environment for Statistical Computing, R Foundation for Statistical Computing, Vienna, Austria, 2021 Search PubMed.
-
N. Tierney, D. Cook, M. McBain, C. Fay, M. O’Hara-Wild, J. Hester and L. Smith, Naniar: Data structures, Summaries, and Visualizations for Missing DataVersion 0.6.0, R package, 2020, https://cran.r-project.org/web/packages/naniar/index.html, accessed on 1 January 2022 Search PubMed.
- A. Kowarik and M. Templ, Imputation with the R Package VIM, J. Stat. Softw., 2016, 74(7), 1–16 Search PubMed.
-
M. Mayer, missRanger: Fast Imputation of Missing Values, 2019, https://CRAN.R-project.org/package=missRanger, accessed on 1 January 2022 Search PubMed.
- M. B. Kursa and W. R. Rudnicki, Feature Selection with the Boruta Package, J. Stat. Softw., 2010, 36, 1–13 Search PubMed.
- D. Bates, M. Machler, B. Bolker and S. Walker, Fitting Linear Mixed-Effects Models Using lme4, J. Stat. Softw., 2015, 67, 1–48 Search PubMed.
- A. Kuznetsova, P. B. Brockhoff and R. H. B. Christensen, lmerTest Package: Tests in Linear Mixed Effects Models, J. Stat. Softw., 2017, 82(13), 1–26 Search PubMed.
-
Handbook of Meta-Analysis in Ecology and evolution, ed., Koricheva, J., Gurevitch, J. and Mengersen, K., Princeton University Press, 2013 Search PubMed.
- D. N. Reshef, Y. A. Reshef, H. K. Finucane, S. R. Grossman, G. McVean, P. J. Turnbaugh, E. S. Lander, M. Mitzenmacher and P. C. Sabeti, Detecting novel associations in large data sets, Sci, 2011, 334(6062), 1518–1524 CrossRef CAS PubMed.
- D. Albanese, M. Filosi, R. Visintainer, S. Riccadonna, G. Jurman and C. Furlanello, Minerva and minepy: a C engine for the MINE suite and its R, Python and MATLAB wrappers, Bioinform, 2013, 29(3), 407–408 CrossRef CAS PubMed.
-
A. Syakila and C. Kroeze, 2011. The Global Nitrous Oxide Budget Revisited. Greenhouse Gas Measurement and Management 1, pp. 17–26. Accessed on 6/5/2022 at https://doi.org/10.3763/ghgmm.2010.0007 Search PubMed.
- J. X. Gu, X. H. Zheng, Y. H. Wang, W. X. Ding, B. Zhu, X. Chen, Y. Y. Wang, Z. C. Zhao, Y. Shi and J. Zhu, Regulatory effects of soil properties on background N2O emissions from agricultural soils in China, Plant Soil, 2007, 295, 53–65 CrossRef CAS.
- Y. Lu, Y. Huang, J. Zou and X. Zheng, An inventory of N2O emissions from agriculture in China using precipitation-rectified emission factor and background emission, Chemosph, 2006, 65, 1915–1924 CrossRef CAS PubMed.
- G. Benckiser, R. Eilts, A. Linn, H. J. Lorch, E. Sumer, A. Weiske and F. Wenzhofer, N2O emissions from different cropping systems and from aerated, nitrifying and denitrifying tanks of a municipal waste water treatment plant, Biol. Fertil. Soils, 1996, 23, 257–265 CrossRef CAS.
- Y. J. Zhang, F. Lin, Y. G. Jin, X. F. Wang, S. W. Liu and J. W. Zou, Response of nitric and nitrous oxide fluxes to N fertilizer application in greenhouse vegetable cropping systems in southeast China, Sci. Rep., 2016, 6, 20700 CrossRef CAS PubMed.
- X. Y. Yan, Z. C. Cai, S. W. Wang and P. Smith, Direct measurement of soil organic carbon content change in the croplands of China, Glob. Change Biol., 2011, 17(3), 1487e1496 CrossRef.
- M. Sebilo, B. Mayer, B. Nicolardot, G. Pinay and A. Mariotti, Long-term fate of nitrate fertilizer in agricultural soils, Proc. Natl. Acad. Sci. U.S.A, 2013, 110, 18185–18189 CrossRef CAS PubMed.
- J. Y. Zhou, B. J. Gu, W. H. Schlesinger and X. T. Ju, Significant accumulation of nitrate in Chinese semi-humid croplands, Sci. Rep., 2016, 6, 25088 CrossRef CAS PubMed.
- D. Majumdar, Biogeochemistry of N2O uptake and consumption in submerged soils and rice fields and implications in climate change, Crit. Rev. Environ. Sci. Technol., 2013, 43, 2653–2684 CrossRef CAS.
- X. Cui, F. Zhou, P. Ciais, E. A. Davidson, F. N. Tubiello, X. Niu, X. Ju, J. G. Canadell, A. F. Bouwman, R. B. Jackson, N. D. Mueller, X. Zheng, D. R. Kanter, H. Tian, W. Adalibieke, Y. Bo, Q. Wang, X. Zhan and D. Zhu, Global mapping of crop-specific emission factors highlights hotspots of nitrous oxide mitigation, Nat. Food, 2021, 2, 886–893 CrossRef CAS.
- R. Conrad, Soil microorganisms as controllers of atmospheric trace gases (H2, CO, CH4, OCS, N2O, and NO), Microbiol. Rev., 1996, 60, 609 CrossRef CAS PubMed.
- G. T. Lahue, C. van Kessel, B. A. Linquist, M. A. Adviento-Borbe and S. J. Fonte, Residual Effects of Fertilization History Increase Nitrous Oxide Emissions from Zero-N Controls: Implications for Estimating Fertilizer-Induced Emission Factors, J. Environ. Qual., 2016, 45, 1501–1508 CrossRef CAS PubMed.
- M. Abdalla, X. Song, X. Ju and P. Smith, Evaluation of the DNDC model to estimate soil parameters, crop yield and nitrous oxide emissions for alternative long-term multi-cropping systems in the north China plain, Agron, 2022, 12, 109 CrossRef CAS.
- Z. Zhao, L. Cao, Z. Sha, J. Deng, C. Chu, D. Zhou, S. Wu and W. Lv, Impacts of fertilization optimization on N loss from paddy fields: Observations and DNDC modeling case study in Shanghai, China, Soil Tillage Res., 2020, 199, 104587 CrossRef.
- M. Abdalla, M. Wattenbach, P. Smith, P. Ambus, M. Jones and M. Williams, Application of the DNDC model to predict emissions of N2O from Irish agriculture, Geoderma, 2009, 151, 327–337 CrossRef CAS.
- M. K. Abbasi and W. A. Adams, Gaseous N emission during simultaneous nitrification-denitrification associated with mineral N fertilization to a grassland soil under field conditions, Soil Biol. Biochem., 2000, 32, 1251–1259 CrossRef CAS.
- X. Zheng, M. Wang, Y. Wang, R. Shen, J. Gou, J. Li, J. Jin and L. Li, Impacts of soil moisture on nitrous oxide emission from croplands: a case study on the rice-based agro-ecosystem in Southeast China, Chemosphere: Global Change Sci., 2000, 2, 207–224 CrossRef CAS.
- H. Xu, G. Xing, Z. Cai and H. Tsoruta, Effect of soil texture on N2O emissions from winter wheat and cotton fields (in Chinese), Agro. Environ. Prot., 2000, 19, 1–3 Search PubMed.
- V. C. Gregorutti and O. P. Caviglia, Nitrous oxide emission after the addition of organic residues on soil surface, Agric., Ecosyst. Environ., 2017, 246, 234–242 CrossRef CAS.
- L. L. Xia, S. K. Lam, B. Wolf, R. Kiese, D. L. Chen and K. Butterbach-Bahl, Trade-offs between soil carbon sequestration and reactive nitrogen losses under straw return in global agroecosystems, Glob. Change Biol., 2018, 24, 5919–5932 CrossRef PubMed.
- E. D. Ma, G. B. Zhang, J. Ma, H. Xu, Z. C. Cai and K. Yagi, Effects of rice straw returning methods on N2O emission during wheat-growing season, Nutr. Cycling Agroecosyst., 2010, 88, 463–469 CrossRef CAS.
- E. G. Gregorich, P. Rochette, D. W. Hopkins, U. F. Mckim and P. St-Georges, Tillage-induced environmental conditions in soil and substrate limitation determine biogenic gas production, Soil Biol. Biochem., 2006, 38, 2614–2628 CrossRef CAS.
- V. Z. Antonopoulos, Comparison of different models to simulate soil temperature and moisture effects on nitrogen mineralization in the soil, J. Plant Nutr. Soil Sci., 1999, 162, 667–675 CrossRef CAS.
- P. Wennman and T. Katterer, Effects of moisture and temperature on carbon and nitrogen mineralization in mine tailings mixed with sewage sludge, J. Environ. Qual., 2006, 35, 1135–1141 CrossRef CAS PubMed.
- K. A. Smith, T. Ball, F. Conen, K. E. Dobbie, J. Massheder and A. Rey, Exchange of greenhouse gases between soil and atmosphere: interactions of soil physical factors and biological processes, Eur. J. Soil Sci., 2003, 54, 779–791 CrossRef.
- M. Abdalla, M. Jones, P. Ambus and M. Williams, Emissions of nitrous oxide from Irish arable soils: effects of tillage and reduced N input, Nutr. Cycling Agroecosyst., 2010, 86, 53–65 CrossRef CAS.
- C. R. Flechard, P. Ambus, U. Skiba, R. M. Rees, A. Hensen, A. van Amstel, A. V. Pol-van Dasselaar, J. F. Soussana, M. Jones, J. Clifton-Brown, A. Raschi, L. Horvath, A. Neftel, M. Jocher, C. Ammann, J. Leifeld, J. Fuhrer, P. Calanca, E. Thalman, K. Pilegaard, C. Di Marco, C. Campbell, E. Nemitz, K. J. Hargreaves, P. E. Levy, B. C. Ball, S. K. Jones, W. C. M. van de Bulk, T. Groot, M. Blom, R. Domingues, G. Kasper, V. Allard, E. Ceschia, P. Cellier, P. Laville, C. Henault, F. Bizouard, M. Abdalla, M. Williams, S. Baronti, F. Berretti and B. Grosz, Effects of climate and management intensity on nitrous oxide emissions in grassland systems across Europe, Agric., Ecosyst. Environ., 2007, 121, 135–152 CrossRef CAS.
-
O. Oenema, A. Bannink, S. G. Sommer and G. L. Velthof, 2001Gaseous Nitrogen Emissions form Livestock Farming Systems, ed., Follett, R.F. and Hatfield, J.L., In, Nitrogen in the Environment: Sources, Problems, and Management Ch. 10, Elsevier, Amsterdam, pp. 255–289 Search PubMed.
- P. He, X. Xu, W. Zhou, W. Smith, W. He, B. Grant, W. Ding, S. Qiu and S. Zhao, Ensuring future agricultural sustainability in China utilizing an observationally validated nutrient recommendation approach, Eur. J. Agron., 2022, 132, 126409 CrossRef CAS.
- J. Gurevitch and L. V. Hedges, Statistical issues in ecological meta-analyses, Ecol, 1999, 80(4), 1142–1149 CrossRef.
- Z. Shang, M. Abdalla, L. Xia, F. Zhou, W. Sun and P. Smith, Can cropland management practices lower net greenhouse emissions without compromising yield?, Glob. Change Biol., 2021, 27(2), 1–14 Search PubMed.
- C. Harlos, T. C. Edgell and J. Hollander, No evidence of publication bias in climate-change science, Clim. Change, 2017, 140, 375–385 CrossRef.
- P. J. Michaels, Evidence for Publication bias concerning global warming in Science and Nature, Energy Environ., 2008, 19, 287–301 CrossRef.
- D. Reckova and Z. Irsova, Publication bias in measuring anthropogenic climate change, Energy Environ., 2015, 26, 853–862 CrossRef.
|
This journal is © The Royal Society of Chemistry 2022 |
Click here to see how this site uses Cookies. View our privacy policy here.