DOI:
10.1039/D1EN01014J
(Perspective)
Environ. Sci.: Nano, 2022,
9, 1913-1925
In vitro-based human toxicity effect factors: challenges and opportunities for nanomaterial impact assessment
Received
5th November 2021
, Accepted 29th March 2022
First published on 6th May 2022
Abstract
The growing number of nanomaterials being produced represents a challenge for the assessment of their toxicity impacts in life cycle assessment (LCA). The human toxicity effect factor, indicating the population incidence risk caused by chemical exposure, is traditionally estimated from in vivo animal test data; however, this kind of study is being reduced in favor of in vitro testing. In this perspective, we identify the peculiarities of nanomaterials compared to chemicals, and how this affects, or should affect, the LCA toxicity characterization methodology within the life cycle impact assessment (LCIA) step. Then, we also discuss both the challenges and the opportunities of integrating in vitro data into LCIA, such as the scarcity of chronic in vitro experiments and avoiding inter-species extrapolation. Moreover, we show the acceptable uncertainty space for in vitro-derived toxicity effect factors for nanomaterials, based on the range of uncertainty of toxicity effect factors for chemicals. Last, we advocate that using in vivo data as a benchmark for the accuracy of derived human toxicity effect factors may in certain cases be misleading. While the adaptation of the LCIA toxicity characterization methodology for nanomaterials and in vitro data is not yet achieved, cross-discipline discussions are a fundamental step towards a successful integration of both new data sources and new substance types into LCIA.
Environmental significance
Life cycle assessment studies of nanomaterials often disregard the potential toxicity impacts caused by nanomaterial emissions, due to a lack of respective characterization factors. Among the causes, there is the difficulty of calculating human effect factors, not only due to the scarcity of data but also due to the uncertainty on whether the standard toxicological impact assessment methodology developed for organic chemicals and metal ions can be applied to nanomaterials as well. This perspective gives a new interdisciplinary overview of the critical issues for the adaptation of the human toxicity effect factor calculation to nanomaterials, and investigates the challenges and opportunities connected to the use of in vitro toxicity data instead of animal toxicity data.
|
1 Introduction
The increasing number of nanomaterials that are being developed requires a careful assessment before entering the market, to make sure their use is safe for humans.1 Besides, such materials could provide additional functionalities and enhanced performances compared to existing technologies and chemicals, thus representing a more sustainable alternative.2,3 Two methodologies address these issues: human health risk assessment (HRA) aims at evaluating whether the health risks posed by nanomaterials to humans in specific exposure situations are acceptable or not,4 while life cycle assessment (LCA) aims at comparing products or processes based on the environmental impacts that they generate along their life cycle, including their (negative) effects on human health.5 Despite differences in goals, procedures and boundary conditions, these two methodologies rely on the same kind of data to provide information about nanomaterial toxicity, i.e. human toxicological data or data from animal studies.6 While human toxicological data are rare and can only be obtained after the population has been already exposed, animal data are becoming scarcer as well, as the toxicology field moves from a phenomenological approach to a mechanistic approach, where in vitro testing is preferred to investigate if and how toxicity arises.7
Over the past few years, different approaches have been investigated and developed in the HRA and nanotoxicology fields to accelerate the evaluation of nanomaterial toxicity and to derive human-relevant information from in vitro data instead of animal data.8 Among many, the development of more advanced in vitro models has brought these experiments closer to realistic conditions, both in terms of exposure and dose–response;9 the development of adverse outcome pathways (AOPs) provides insights on the link between initial events that can be observed in vitro and the progression of toxicity up to human pathology;10 grouping approaches are more and more used to infer toxicity based on the similarity in properties of untested and tested nanomaterials.11,12
USEtox, the UNEP/SETAC global scientific consensus model for the characterization of human toxicological and ecotoxicological impacts of chemicals,13 is a widely applied life cycle impact assessment (LCIA) model.14,15 It defines the methodological steps for the calculation of toxicity-related characterization factors (CFs), which represent the potential toxicity-related impacts on human health and on ecosystem quality caused by the emission of substances into the environment. Other impact assessment methods, such as Recipe 2016, use similar approaches.16 For human toxicity impacts, a CF is obtained by the combination of a fate factor (FF), indicating the distribution of a substance in the environmental compartments, an exposure factor (XF), indicating the intake of a substance by humans from an environmental compartment and through different exposure pathways, and a toxicity effect factor (EF), which indicates the disease incidence in the human population linked to the intake of a substance.13,17 The toxicity effect factor is calculated either from human data or by extrapolating to humans the information from animal studies, separating cancer and non-cancer effects. As non-cancer effects are the ones more easily tested in vitro, hereafter the term “toxicity effect factor” will be used to indicate non-cancer toxicity effect factors only.
Considering that the market for nanomaterials and nanomaterial-containing products is now in a phase of fast growth,18 calculating human toxicity effect factors for nanomaterials becomes a much-needed as well as tedious task. In this perspective, we explore and discuss the challenges and opportunities of integrating in vitro data into human toxicity characterization of nanomaterials in LCIA. In the frame of these activities, we identified two main types of hurdles that currently hinder the development of toxicity effect factors for nanomaterials: a) the lack of nano-specific LCIA toxicity characterization methods, and b) the scarcity of animal toxicity studies with respect to the number of existing nanomaterials. For the first point, we describe consecutively the strategies that have been proposed to adapt to nanomaterials the toxicity effect factor calculation procedure originally developed for organic chemicals and metal ions, pointing out which challenges remain today yet to be solved. As a potential solution to the second point, we discuss the challenges as well as the advantages of using in vitro toxicity data in place of in vivo toxicity data.
2 Toxicity effect factor calculations
2.1 The USEtox 2.0 methodology
The human toxicity effect factor “relates human health effects to the mass taken in by humans via different exposure pathways”,13 discriminating between the inhalation and ingestion routes. The toxicity effect factors for each route are derived from the lifetime human ED50 (hED50), i.e. the lifetime dose inducing non-cancer diseases in 50% of the population, considering 70 years of lifetime, a 70 kg body weight for ingestion and a 13 m3 d−1 inhalation rate for inhalation,19 with the formula:
In the absence of human toxicological data, a human-equivalent ED50 is calculated from animal data, by applying the following extrapolation and correction factors as needed:
• Interspecies extrapolation factor: divide by the factor 1 for inhalation or varying from 1.1 for pigs to 7.3 for mice for oral exposure;
• Route-to-route: multiply by 1;
• Discontinuous to continuous exposure correction factor: multiply by
;
• Sub-chronic or sub-acute to chronic extrapolation factors: divide respectively by 2 or 5;
• Acute LD50 to chronic ED50: divide by 26;
• NOAEL to ED50 extrapolation factor: multiply by 9;
• LOAEL to NOAEL extrapolation factor: divide by 4.
2.2 Proposed changes to the methodology
In 2002, Pennington et al.20 suggested that ED10 would be a better reference point in dose–response curves compared to ED50, since this measure better represents the marginal toxicity slope at environmentally-relevant exposure levels.
A recent publication21 proposes an update of the methodology for the calculation of toxicity EFs for non-cancer endpoints. The new human toxicity dose–response framework is based on probabilistic dose–response assessment; a probabilistic approach is applied as well for the extrapolation between toxicological dose descriptors (e.g. NOAEL to LOAEL) and the related uncertainty.22 The new method adopts the benchmark dose (BMD) approach to estimate a human lifetime ED10, and even though multiple dose descriptors can be used (e.g. NOAEL, LOAEL), they are all converted to a BMD value via extrapolation factors.23 The choice of the BMD approach aligns the LCIA methodology with the current consensus on risk assessment (RA).24,25 Compared to NOAEL, which was widely used in RA in the past,26 BMD presents multiple advantages, such as: 1) the full non-linear dose–response curve is used for its calculation, as described in Chiu et al.;22 2) BMD is less dependent on the number and spacing of the selected doses; 3) the uncertainty of BMD is quantifiable and can be reported as confidence intervals.27,28
3 Why we cannot treat nanomaterials as chemicals
Fundamental differences between chemicals and nanomaterials entail that the approaches developed for the former cannot be simply applied to the latter.
First of all, nanomaterials cannot be defined solely by their chemical composition, as the same material can exist in multiple forms, i.e. have different sizes and size distributions, crystalline structures, coatings, shapes, etc.29 The combination of these characteristics determines then the individual material properties, which differ from those of their bulk counterparts.30,31 This requires additional effort in terms of material characterization, and represents a challenge in terms of reproducibility and comparability.31
During storage, use, and disposal of nanomaterials and nano-enabled products, these properties can change due to transformation processes such as oxidation, aggregation, and dissolution;32 in the case of smart nanomaterials, the change in properties and/or activation of specific functions is designed to occur in reaction to specific stimuli, thus adding an additional level of complexity to the characterization and toxicity assessment.33 When released into the environment, nanomaterials can undergo chemical, physical, and biological transformations, as well as interact with macro-molecules.34–36 Upon entering biological systems, they can again be subject to bio-transformations that modify their properties and behavior, such as the formation of a protein corona.37
Not only the behavior, but also the toxicity of nanomaterials is delineated by the combination of these properties, while for chemicals, the biological effects are governed by the chemical identity only.38 For this reason, nanoparticle toxicity is better expressed as a function of the property/ies driving it; for example, surface area can be a better reference dose than mass for inhaled low-toxicity low-solubility particles.39 However, understanding which and how properties affect toxicity is not a trivial task, especially when considering that a nanomaterial reaching a biological target is not anymore as homogeneous as the pristine material, but consists of a population of different materials with different physico-chemical properties.37
This complexity in the structure and properties of nanomaterials distinguishes them from chemicals, and calls for ad hoc approaches.
4 Nano-specific challenges
Compared to chemicals, the development and use of toxicity effect factors for nanomaterials presents some intrinsic and some methodological challenges.
As stipulated in section 3, nanomaterials exist in a potentially endless number of nanoforms, determined by their physico-chemical properties, and are often heterogeneous mixtures once they reach and enter the human body. How should this vastness of property combinations be managed in LCIA? At which point do we consider two nanomaterials/nanoforms as different enough to require for each of them a specific toxicity effect factor? It is obviously not possible to develop toxicity effect factors for each single form; instead, a more realistic approach could be to group nanomaterials based on their toxicity, and use a single toxicity effect factor for each group. However, to classify nanomaterials without testing each one of them, we need to understand how their physico-chemical characteristics affect their toxicity.40 At which point does the change in properties determine a significant shift in toxicity, as for example the fiber paradigm identifying nanofibers as carcinogenic only if they are at the same time stiff, long, and biopersistent?41 In this direction, multiple grouping strategies have been developed, in which nanomaterials are classified based on their intrinsic and extrinsic properties, their behaviour, or their mode of action (see ref. 42 for a comprehensive overview). Establishing groups of nanomaterials is though made difficult by the scarcity of data, the lack of harmonized experimental methods, and concerns about the quality of the data.42
Walser et al.43 faced a similar challenge when developing a derivation strategy for the calculation of EFs for nanomaterials. In their procedure, the critical first step is the assignment of a clear chemical identity to the substance, which would be representative of nanomaterials with similar toxic effects, thus allowing the reduction of the need for new toxicity effect factors for materials that are not comparable with those already existing. However, developing such a scientifically-justifiable hierarchy for grouping is not an easy task because of the large number of combinations of physical and chemical properties of nanomaterials, and requires a consensus among a variety of specialists such as risk assessors and LCA scientists. If a nanomaterial requires a new EF, Walser et al.43 suggests a tiered approach to manage data scarcity, where, in the absence of animal data, the EF is extrapolated based on classification into either poorly soluble, low-toxicity nanoparticles, persistent high aspect ratio nanofibers, or soluble metals and metal oxides. While not yet included in such a strategy, in vitro data could play an important role in LCIA as a basis of comparison of the potency of nanomaterials with a similar mode of toxicity action.43 Building on the work from Walser et al.,43 Fransman et al.44 defined a step-by-step procedure to calculate EFs for inhaled nanomaterials. For the determination of ED50, the dose should be expressed in the most relevant dose metric, based on the recognition of the impact that surface area and particle number may have on toxicity. Normalizing the EF by a unit specific surface area or a specific number of particles would then allow covering the whole spectrum of these two properties, whereas a mass-based EF would be unique to each nanomaterial with e.g. a different specific surface area.
Despite the fact that such initial frameworks have been developed in order to calculate EFs for nanomaterials, it is clear that the challenges connected to the peculiarities of the broad variety of nanomaterials cannot be answered by the LCA field and scientists alone. But LCA practitioners need to be aware of all this, for example in order to define the applicability range of the toxicity effect factors they develop, or to calculate the toxicity effect factor as a function of the most relevant properties, similar to how Laurent et al.45 calculated NOAEL values for titanium dioxide as a function of its primary size.
From a methodological point of view, the extrapolation factors used to convert different dose descriptors (e.g. LOAEL, NOAEL) to ED50 and non-chronic to chronic exposures have been obtained from the analysis of organic chemical toxicity data.46–48 The suitability of these factors for nanomaterials is yet unknown, but they have been used up to now in the absence of better options.45 To verify existing factors as well as to develop nano-specific ones, we would need in vivo toxicity data reporting pairs of, for example, NOAEL and ED50 values, or effects under sub-acute and chronic exposure conditions. Hence, a good number of data points covering different types of nanomaterials would be actually needed; for organic chemicals, the number of pairs used has ranged from 21, for the NOAEL–ED50 comparison of non-cancer effects,47 to more than 200 pairs for sub-chronic to chronic NOAEL values.46
Animal toxicity studies about nanomaterials are quite scarce, especially chronic ones. Moreover, combining results from different studies is not trivial: on one side, a transparent and comprehensive reporting of the nanomaterial physico-chemical properties is often lacking; on the other side, the nanomaterial properties (when reported) are often not the same between studies. For these reasons, calculating nano-specific extrapolation factors seems a remote possibility.
5 Existing toxicity effect factors for nanomaterials
Most LCA studies overlook the potential impacts caused by nanomaterial release, often because of the lack of CFs for such materials.56 The few existing toxicity effect factors for nanomaterials have been calculated by applying the USEtox approach for bulk chemicals, with slight adaptations in some cases (Table 1). The main differences pertained to the dose used in the calculation of the toxicological dose descriptors (e.g. ED50 or ED10), which in some cases was expressed in deposited dose instead of intake dose, or in surface area instead of mass. In the former case, the EF was then calculated in cases per intake dose by converting the dose descriptor from deposited to intake dose using size-specific deposition fractions calculated via a lung dosimetry model;50,57 in this case, while the EF calculation deviates from the consensus model, the obtained EF is expressed in the same unit as USEtox EFs, thus allowing its use for the calculation of characterization factors without further adaptations. In contrast, when the toxic effects were proportional to the surface area of the particle rather than the mass, i.e. the relevant dose metric was the surface area, it affected not only the dose descriptor calculation but also the EF, which was normalized by the specific surface area of the nanoparticle. In this way, the EF could be applied to nanoforms with different surface areas. Only in two studies were the human toxicity effect factors calculated from in vitro toxicity data: in one case the EF was calculated by assuming the in vitro endpoint (reactive oxygen species production) to be predictive of the incidence of inflammation in humans, therefore considering the in vitro ED50 in mg per million neutrophils as corresponding to the human ED50, and requiring only extrapolation from cellular dose to intake dose.54 The other study instead used a comparative approach, as suggested also by Walser et al.;43 the EF was estimated via a relative potency approach, by multiplying the EF of the corresponding ion (e.g. copper ions and copper oxide nanoparticles) by the difference in potency between ions and nanoparticles, measured in vitro.55
Table 1 Toxicity effect factors for non-cancer effects of nanomaterials. Differences from the USEtox methodology can be observed in the units of the toxicity effect factor, in the toxicological dose descriptor calculations, and in the source of toxicological information. SWCNTs: single-walled carbon nanotubes; MWCNTs: multi-walled carbon nanotubes
Nanomaterial |
Effect factor |
Units |
Exposure route |
Differences from the USEtox methodology |
Source data |
Ref. |
SWCNTs |
5.3 × 10−2 |
Cases/kgintake |
Inhalation |
— |
In vivo
|
49
|
SWCNTs |
1.1 × 10−3 |
Cases/kgintake |
Ingestion |
— |
In vivo
|
49
|
MWCNTs |
1.4 × 10−2 |
Cases/kgintake |
Inhalation |
— |
In vivo
|
49
|
MWCNTs |
13 |
Cases/kgintake |
Ingestion |
— |
In vivo
|
49
|
MWCNTs |
530 |
Cases/kgintake |
Inhalation |
Dose descriptor calculated in mass deposited in the lungs |
In vivo
|
50
|
MWCNTs |
2.5 × 103 |
Cases/kgintake |
Inhalation |
Dose descriptor calculated in mass deposited in the lungs |
In vivo
|
50
|
Carbon black |
2.9 × 10−2 |
Cases/(m2 g−1 kgintake) |
Inhalation |
Surface area as dose metric |
In vivo
|
50
|
Titanium dioxide |
1.72 × 10−2 |
Cases/kgintake |
Inhalation |
Indoor workplace exposure (45 years, 240 days per year) |
In vivo
|
51
|
Titanium dioxide |
7.26 × 10−3 |
Cases/kgintake |
Inhalation |
— |
In vivo
|
51
|
Titanium dioxide |
1.15 |
Cases/kgintake |
Inhalation |
— |
In vivo
|
52
|
Titanium dioxide |
2.94 × 10−2 |
Cases/kgintake |
Ingestion |
— |
In vivo
|
52
|
Titanium dioxide |
1.21 × 106 |
Cases/(kgdeposited glung−1) |
Inhalation |
Dose descriptor calculated in mass deposited per lung unit mass |
In vivo
|
53
|
Titanium dioxide |
5.6 × 10−2 |
Cases/(m2 g−1 kgintake) |
Inhalation |
Surface area as dose metric |
In vivo
|
50
|
Titanium dioxide |
5.6 × 10−2 |
Cases/(m2 g−1 kgintake) |
Inhalation |
Surface area as dose metric |
In vivo
|
44
|
Copper |
5.96 × 10−1 |
Cases/kgintake |
Ingestion |
Calculated from in vitro experiments |
In vitro
|
54
|
Copper oxide |
4.5 × 10−2 |
Cases/kgintake |
Inhalation |
Dose descriptor calculated via the relative potency approach |
In vitro
|
55
|
Copper oxide |
7.5 × 10−3 |
Cases/kgintake |
Ingestion |
Dose descriptor calculated via the relative potency approach |
In vitro
|
55
|
Silver |
6.5 × 10−1 |
Cases/(m2 g−1 kgintake) |
Inhalation |
Surface area as dose metric |
In vivo
|
50
|
Silver |
1.2 |
Cases/kgintake |
Inhalation |
Dose descriptor calculated via the relative potency approach |
In vitro
|
55
|
Silver |
5.9 × 10−1 |
Cases/kgintake |
Ingestion |
Dose descriptor calculated via the relative potency approach |
In vitro
|
55
|
Zinc oxide |
2.9 × 10−2 |
Cases/kgintake |
Inhalation |
Dose descriptor calculated via the relative potency approach |
In vitro
|
55
|
Zinc oxide |
2.5 × 10−2 |
Cases/kgintake |
Ingestion |
Dose descriptor calculated via the relative potency approach |
In vitro
|
55
|
6 Challenges and advantages of the use of in vitro data
In addition to human and animal toxicological data, in vitro toxicity data are a more recent but already richer source of toxicological information, and could potentially be used to calculate human toxicity effect factors for nanomaterials as well as chemicals (Fig. 1).
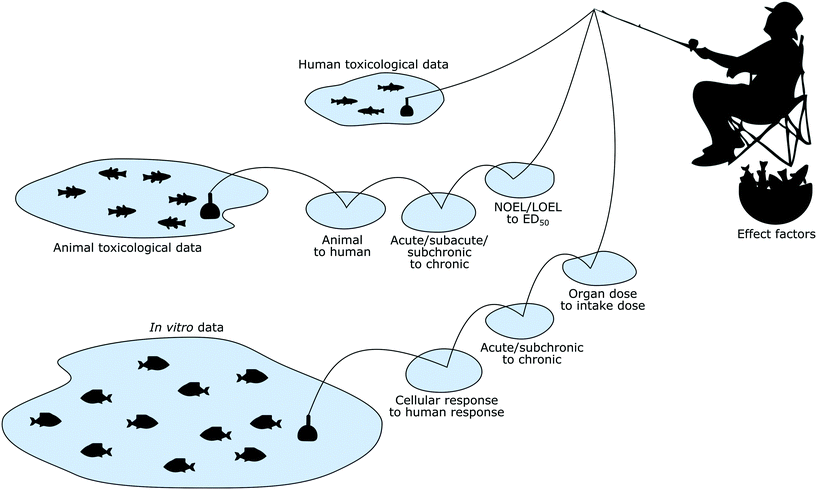 |
| Fig. 1 The landscape of data sources and extrapolation factors needed to calculate toxicity effect factors from each data type. When human toxicological data are available, the EF can be directly calculated. In the case of animal toxicity data, which represent a bigger data pool compared to human data, multiple extrapolation steps may be needed to calculate the EF, respectively accounting for the differences in species, exposure time, and toxicological dose descriptor. In vitro data represent the richest data source, but would require as well additional extrapolation procedures to be used to calculate the EF. In particular, the response at the cellular level would have to be related to a response at the human level (due to the difference between in vitro and human endpoints); shorter exposure times would have to be extrapolated to chronic exposures; organ doses measured in vitro would have to be linked to the corresponding intake doses. | |
Using in vitro data would have many advantages—beyond the simple fact that producing such data is much simpler compared to producing animal data—but this introduces at the same time also new challenges and requires that the respective LCIA methodologies are adapted accordingly. While these advantages and challenges are not necessarily nanomaterial-specific but more generally apply to the use of in vitro data for any kind of substances (e.g. endocrine disruptors58), the nanomaterial perspective is nevertheless required when developing a practical approach to overcome these hurdles for this material category.
First of all, compared to animal-based nanotoxicology, in vitro nanotoxicology is a fast-evolving and very active field, meaning that any consideration we do based on current technologies, practices, and experimental systems should account for the fact that those practices will be further improved over the years, and we therefore can expect more realistic systems and higher-quality results in the future.
A comparison of in vitro and in vivo toxicity screening tests showed that the former, in addition to sparing the life of many animals, was cheaper than the latter (see Meigs et al.59 for figures on specific comparisons of in vivo vs. in vitro experiments). While the costs increase with the complexity of in vitro systems, the results obtained using these systems are also more informative.60 Considering that in vitro tests can be both high-content and high-throughput, their application offers the possibility to test many more nanomaterials and also react faster to the development of new materials than what would be possible using only animals.61,62
Being able to do more tests in less time also means, with respect to the toxicity effect factor calculation, that different cell lines could be used to test both the inhalation and the ingestion exposure routes, avoiding the need for route-to-route extrapolation. Moreover, multiple doses can be used to obtain a dose–response curve and identify resulting BMD or ED50 values, instead of extrapolating from NOAEL or LOAEL values.
Since in vitro tests are based on human cells, we can avoid the need for extrapolating from animals to humans, but we need to extrapolate a cellular response to a human response instead. To do so, we need the in vitro system to mimic as much as possible at least the early events driving the toxic effects that we would observe in humans. Unfortunately, in vitro systems cannot currently capture the complexity of in vivo pathophysiological conditions. However, in vitro technologies are starting to anticipate this complexity by moving from cancer cell lines to primary human cells, from mono-cultures to co-cultures, from 2D to 3D systems, and from static to dynamic conditions, creating novel systems such as organs-on-a-chip.63
While submerged mono-cultures can be considered quite rudimentary systems, co-cultures, where different cell lines are cultured together to represent the complexity of cell–cell interactions, more realistically respond to nanomaterial exposure.63–66 Depending on the case, additional factors have to be considered and integrated in the in vitro system to mimic physiological conditions. Cells exposed to a flow (e.g. blood and lymph) are subjected to sheer stress, which affects the cellular structure and function.67 Microfluidic technologies, thanks to their ability to replicate steady and transient flows, have revolutionized the study of the microenvironment of cells, even though their complexity is still a limit to their wide application.68 The liver is another example: in this organ, CYP450 enzymes are fundamental for the metabolism of substances, but in vitro mono-cultures of hepatocytes lose this function. However, growing the cells on specific extracellular matrices or co-culturing them with other liver cells restores the CYP enzyme activity.69
More and more used, organoids are 3D multicellular in vitro systems in which stem cells organize and differentiate into complex tissue structures, thus mimicking specific organs.60,70,71 Recognized by the World Economic Forum as a top emerging technology in 2016, organs-on-a-chip allow a level of emulation of biological systems never seen before.72 By combining living cell tissues (that can go from simple 2D cultures to complex organoids) with a microfluidic system, the organ-on-a-chip creates a physiological microenvironment where the complex responses to stimuli or substances can be monitored.73,74
There is therefore great potential for in vitro tests to better mimic human responses, even though the current costs and complexity of these advanced systems limit their systematic application,60,74 making the use of these data difficult in the LCIA context. Moreover, additional work is needed to verify the predictivity and reliability of these technologies,63 and until then LCIA should prefer to extrapolate human toxicity from animal data (if available).
An additional criticality resides in the choice of in vitro endpoints predictive of the effects at the level of the whole organism. Here, rather than focusing on acute toxic responses, the emphasis should be on disrupted cell functions or non-lethal injuries which are seen as suitable indicators of the early phases of a chronic response.75 In multiple cases, the release of cytokines in vitro was shown to correlate well with acute in vivo inflammation in the lungs, indicating the inflammation pathway as promising for predictive purposes.76–80 On the other hand, a large-scale comparison of in vitro and in vivo points of departure of chemicals (i.e. doses at which low effects were observed) showed low predictivity of in vivo adverse effects using in vitro bioactivity data: in 89% of the cases, the in vitro dose descriptor was lower than the in vivo one, but the ratio between the two values ranged several orders of magnitude.81 Hence, more studies are still needed in this issue in order to verify if and which in vitro data might be predictive of in vivo effects.
The exposure length is another critical aspect for the implementation of in vitro data into LCIA. While the methodology requires chronic ED50, either from chronic experiments or extrapolated from shorter exposure times with the corresponding extrapolation factors (developed for organic chemicals), in vitro studies mostly focus on acute effects and exposures. While in vitro tests have been shown to be predictive of acute in vivo effects, especially inflammation,82–84 a correlation with chronic effects is not yet known. However, recent advancements in cell culture methods are making it possible to maintain cells alive for longer periods of time, thus allowing sub-chronic toxicity testing in vitro.85–88
A further challenge for the use of in vitro data is the need to link observed effects on the cells to intake doses instead of the dose delivered to the cells, i.e. combine the toxicodynamics of the material (i.e. the interaction of the toxicant with the target, in this case the cells) with its toxicokinetics (i.e. the fate of the toxicant in the body).89 When considering inhaled nanomaterials and their effect on the lungs, the MPPD dosimetry model57 is widely used in risk assessment to estimate the deposition of particles in the lungs; moreover, such a model has been recommended also for the development of toxicity effect factors via inhalation.50 When the target organ is not the original point of entry of the nanomaterial, the back-calculation of the intake dose from the organ dose is more complex; in this case, physiologically-based pharmaco-kinetic (PBPK) models can be used to model the distribution, excretion, and metabolism of the nanomaterial in the human body. Unfortunately, the existing PBPK models cover only a handful of nanomaterials, and generalizing them to expand their applicability is made difficult by the complexity of the biotransformations nanomaterials are subjected to in biological systems.37,90
All in all, while we cannot afford to ignore the in vitro data pool, its implementation into LCIA is not (yet) straightforward and we still need further, novel procedures that make these data compatible with the methodology, such as the examples presented above. At the same time, the methodology itself requires both adaptations and benefits from the peculiarities of in vitro data.
7 Uncertainty space for the integration of in vitro data in LCIA
Due to the high uncertainty of the EF, the human health impacts calculated in LCA via USEtox or similar methodologies should be used qualitatively to identify the most impacting substances, only comparing the magnitude of the results rather than the precise value.13 The level of uncertainty of the EF depends on the uncertainty of the extrapolation factors used to extrapolate animal data to chronic human data (Table 2).
Table 2 The uncertainty factor k associated to each EF extrapolation factor. Each study calculated the uncertainty factor according to Slob,91i.e. so that 95% of the data used for the determination of the extrapolation factor was within a factor k from the median (eqn (1))
Extrapolation factor |
Uncertainty factor k |
Ref. |
Interspecies |
19 |
46
|
Route-to-route |
50 |
48
|
NOAEL to ED50 |
11 |
47
|
LOAEL to NOAEL |
4 |
47
|
Acute LD50 to chronic |
46 |
48
|
Sub-acute to chronic |
12 |
46
|
Sub-chronic to chronic |
12 |
46
|
Sub-acute to sub-chronic |
15 |
46
|
For log-normally distributed data, the uncertainty factor k is defined based on a 95% confidence interval, so that
| 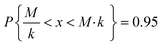 | (1) |
with
P being the probability,
x the variable being calculated and
M the median.
91
The uncertainty factor of the toxicity effect factor is a combination of the uncertainties of each extrapolation factor used; to calculate it, we followed the analytical method of Slob91 which is based on the assumption of log-normal-distributed uncertainties for multiplicative models, as also done in USEtox.13,47 The uncertainty factor of the toxicity effect factor kEF is calculated according to the formula:
|  | (2) |
with
k being the uncertainty factor of each extrapolation factor.
Route-to-route extrapolation is the factor with the highest uncertainty; the possibility to perform specific in vitro experiments for each exposure route would make this extrapolation factor, and its connected uncertainty, unnecessary. The uncertainty in extrapolating from animals to humans and from NOAEL/LOAEL to ED50 is avoided as well given the use of human cells and the possibility to construct a dose–response curve by testing multiple doses. Based on eqn (2), the combined uncertainty of these extrapolation factors, which may be avoided using in vitro data, is of a factor 277. However, as discussed before, the focus of in vitro studies on acute effects can be a challenge for their use, not only for their predictivity but also in terms of uncertainty contribution; as the acute LD50 to chronic extrapolation factor for chemicals has the second highest uncertainty, we may expect a similar impact for in vitro data. Hence, a shift towards sub-acute and sub-chronic in vitro experiments would help reduce this source of uncertainty.
All in all, we could consider in vitro data a good alternative to animal data if the uncertainty of the in vitro toxicity effect factor is equal to or smaller than the one from animal data. As the extrapolation factors for in vitro data do not exist yet, we calculated the uncertainty space into which the in vitro toxicity effect factor should fall, based on the uncertainty of in vivo extrapolation factors and eqn (2).
For example, the toxicity effect factor for inhalation from Pini et al.51 from Table 1 (EF = 7.26 × 10−2 cases per kgintake) was calculated from the NOAEL value obtained from a sub-chronic oral study on mice. The combination of the uncertainties of the NOAEL-to-ED50 extrapolation factor, the sub-chronic to chronic extrapolation factor, the interspecies extrapolation factor, and the route-to-route extrapolation factor results in a toxicity effect factor with an uncertainty of 400. Excluding the route-to-route extrapolation, i.e. if the exposure had been via inhalation, the uncertainty factor would have been 93. If instead of a NOAEL value the study had provided an ED50 value, the uncertainty would have been 47. Assuming the worst case possible, i.e. an acute LD50 value requiring acute-to-chronic extrapolation, route-to-route extrapolation, and interspecies extrapolation, an uncertainty factor of 500 will be obtained. Similar ranges have been reported also using a probabilistic approach, with a 400-fold uncertainty when using sub-chronic LOAEL values.22
The space of uncertainty of the toxicity effect factors calculated from in vivo data can be very wide, but they are still accepted into e.g. USEtox as the best option available, as having no toxicity effect factor would result in completely disregarding the impacts of a substance in each LCA study based on the concerned impact assessment method. The same attitude is needed towards the estimation of toxicity effect factors from in vitro data; uncertain results are inevitable, but they can still be fit for purpose as long as their uncertainty factor is equal to or below 500.
8 On the risks of using animal data as a benchmark
When evaluating the predictivity and accuracy of in vitro data, animal studies are often used as a benchmark.41,92,93 Similarly, the toxicity effect factors calculated from in vitro data may be compared with the ones calculated from in vivo data to verify whether they are in accord, assuming the latter to be the most accurate of the two. This assumption is though not necessarily true, since the reproducibility of in vivo results and their inter-species predictivity have been shown to be poor.94 For example, studies on the effects of inhaled particles on rats have been used to calculate both non-cancer and cancer toxicity effect factors;53,50 however, the rats have been shown to be particularly susceptible to inhaled particles compared to other animals, and the same mechanism causing the emergence of cancer has not been observed in humans.95,96 Even in the same animal family (Muridae, which includes rats and mice), the average interspecies predictive power was around 50% for both long- and short-term effects, based on the analysis of 37 chemicals.97 While detecting toxicity in an animal increases the probability of the substance to be toxic to other species, the opposite was not found to be true: the lack of toxicity in an animal had very little predictive power towards human (lack of) toxicity.98
With the goal of the toxicity effect factors being to represent the potency of the nanomaterial toxicity to humans, an ED50 or ED10 value (from now on called EDx) extrapolated from in vitro data may be more accurate than the one extrapolated from animal data (Fig. 2). However, this depends on how close the extrapolated EDx values are to the real human EDx values, which is unknown. For example, an in vitro-extrapolated EDx value may be close to the real human EDx value, but be very different from the animal-extrapolated EDx value; on the other hand, we could also have in vitro-extrapolated EDx values very similar to the animal values, but less similar to the real human EDx values. Only through human toxicological studies can we benchmark both animal- and in vitro-extrapolated EDx values and verify their accuracy.
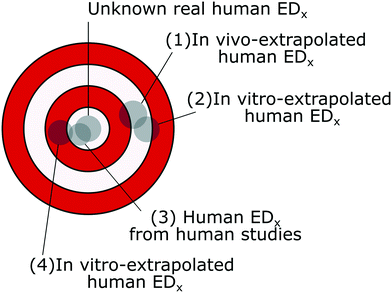 |
| Fig. 2 Using in vivo data as a benchmark to judge the quality of the in vitro-extrapolated EDx values could lead to selecting values (2) that are more similar to the animal-extrapolated EDx values (1), while other in vitro-extrapolated EDx values (4) might be closer to the real, unknown human EDx values. Only through epidemiological studies (3) can we verify the accuracy of the extrapolated values in describing the toxicity of nanomaterials to humans. It should be noted that each EDx value is not a single point, but rather an area representing the variability and uncertainty of the measure. | |
9 Conclusions
A change in the LCIA methodologies is needed if we want to cover the impacts on human health of nanomaterials in LCA, in particular with respect to the toxicity effect factor calculations. First, we need to acknowledge that nanomaterials are not chemicals, meaning that we cannot rely on traditional approaches, and we need to explicitly address the multidimensionality of nanomaterial identity and its implications for LCIA/LCA. Chemical composition cannot be the only distinguishing property reported, but other relevant properties such as the shape and size should be included as well, both in the calculation of characterization factors and in the inventory data.99 For the toxicity effect factor calculation, understanding the relationship between nanoparticle properties and toxicity is needed to develop EFs as a continuous or discrete function of the relevant property/ies.41,45
All in all, implementing in vitro data into LCIA has to become a priority to avoid nanomaterial effects being ignored due to the scarcity of animal toxicity data. However, this adaptation is not an easy task, as it falls midway between LCA and nanotoxicology; while good propositions already exist,54,55,100 additional (new) ideas and comprehensive strategies are still needed. Rather than a single solution, an iterative and collaborative process is needed; a kind of prospective toxicity effect factor calculation strategy where proofs of concept based on the available knowledge go hand in hand with the development of adaptable theoretical structures based on the foresight of future advancements in the nanotoxicology field. Such a strategy will be characterized, especially in the beginning, by a high level of uncertainty, but, as we showed in section 7, this can be the case for animal-based EFs as well. The uncertainty space delimited by the range of uncertainty that a traditional EF can have (k between 19 and 500) provides a reference for comparison for in vitro-based EFs.
In the end, such cross-discipline discussions will assure that, once the nanotoxicology field is ready, in vitro data can be smoothly and efficiently implemented into LCIA. Until then, human data first and in vivo data secondly should be the preferred source of toxicological information.
Notably, while we focused on human toxicity impacts of nanomaterials and the calculation of EFs, the challenges and opportunities we described go beyond this specific case. For example, a similar reasoning could be done for ecotoxicity impacts, as the use of animal cells instead of whole organisms would speed up the toxicity testing of new substances.
Conflicts of interest
There are no conflicts to declare.
Acknowledgements
This research is part of the project NANORIGO, which has received funding from the European Union's Horizon 2020 research and innovation programme under grant agreement no. 814530. This publication reflects only the authors' view and the Commission is not responsible for any use that may be made of the information it contains.
Notes and references
- V. Srivastava, D. Gusain and Y. C. Sharma, Critical Review on the Toxicity of Some Widely Used Engineered Nanoparticles, Ind. Eng. Chem. Res., 2015, 54, 6209–6233 CrossRef CAS.
- I. Corsi, M. Winther-Nielsen, R. Sethi, C. Punta, C. Della Torre, G. Libralato, G. Lofrano, L. Sabatini, M. Aiello, L. Fiordi, F. Cinuzzi, A. Caneschi, D. Pellegrini and I. Buttino, Ecofriendly nanotechnologies and nanomaterials for environmental applications: Key issue and consensus recommendations for sustainable and ecosafe nanoremediation, Ecotoxicol. Environ. Saf., 2018, 154, 237–244 CrossRef CAS PubMed.
- M. M. Falinski, D. L. Plata, S. S. Chopra, T. L. Theis, L. M. Gilbertson and J. B. Zimmerman, A framework for sustainable nanomaterial selection and design based on performance, hazard, and economic considerations, Nat. Nanotechnol., 2018, 13, 708–714 CrossRef CAS PubMed.
- J. S. Tsuji, A. D. Maynard, P. C. Howard, J. T. James, C.-W. Lam, D. B. Warheit and A. B. Santamaria, Research strategies for safety evaluation of nanomaterials, part IV: risk assessment of nanoparticles, Toxicol. Sci., 2006, 89, 42–50 CrossRef CAS PubMed.
- G. Rebitzer, T. Ekvall, R. Frischknecht, D. Hunkeler, G. Norris, T. Rydberg, W.-P. Schmidt, S. Suh, B. Weidema and D. Pennington, Life cycle assessment, Environ. Int., 2004, 30, 701–720 CrossRef CAS PubMed.
- J. B. Guinée, R. Heijungs, M. G. Vijver and W. J. Peijnenburg, Setting the stage for debating the roles of risk assessment and life-cycle assessment of engineered nanomaterials, Nat. Nanotechnol., 2017, 12, 727 CrossRef PubMed.
- M. E. Andersen and D. Krewski, Toxicity Testing in the 21st Century: Bringing the Vision to Life, Toxicol. Sci., 2009, 107, 324–330 CrossRef CAS PubMed.
- V. Stone, H. J. Johnston, D. Balharry, J. M. Gernand and M. Gulumian, Approaches to Develop Alternative Testing Strategies to Inform Human Health Risk Assessment of Nanomaterials, Risk Anal., 2016, 36, 1538–1550 CrossRef PubMed.
- B. Drasler, P. Sayre, K. G. Steinhäuser, A. Petri-Fink and B. Rothen-Rutishauser, In vitro approaches to assess the hazard of nanomaterials, NanoImpact, 2017, 8, 99–116 CrossRef.
- K. Gerloff, B. Landesmann, A. Worth, S. Munn, T. Palosaari and M. Whelan, The Adverse Outcome Pathway approach in nanotoxicology, Comput. Toxicol., 2017, 1, 3–11 CrossRef.
- L. Lamon, D. Asturiol, A. Richarz, E. Joossens, R. Graepel, K. Aschberger and A. Worth, Grouping of nanomaterials to read-across hazard endpoints: from data collection to assessment of the grouping hypothesis by application of chemoinformatic techniques, Part. Fibre Toxicol., 2018, 15, 37 CrossRef CAS PubMed.
- V. Stone, S. Gottardo, E. A. Bleeker, H. Braakhuis, S. Dekkers, T. Fernandes, A. Haase, N. Hunt, D. Hristozov, P. Jantunen, N. Jeliazkova, H. Johnston, L. Lamon, F. Murphy, K. Rasmussen, H. Rauscher, A. S. Jiménez, C. Svendsen, D. Spurgeon, S. Vázquez-Campos, W. Wohlleben and A. G. Oomen, A framework for grouping and read-across of nanomaterials- supporting innovation and risk assessment, Nano Today, 2020, 35, 100941 CrossRef CAS.
- R. K. Rosenbaum, T. M. Bachmann, L. S. Gold, M. A. Huijbregts, O. Jolliet, R. Juraske, A. Koehler, H. F. Larsen, M. MacLeod, M. Margni, T. E. McKone, J. Payet, M. Schuhmacher, D. Van De Meent and M. Z. Hauschild, USEtox - The UNEP-SETAC toxicity model: Recommended characterisation factors for human toxicity and freshwater ecotoxicity in life cycle impact assessment, Int. J. Life Cycle Assess., 2008, 13, 532–546 CrossRef CAS.
- T. B. Westh, M. Z. Hauschild, M. Birkved, M. S. Jørgensen, R. K. Rosenbaum and P. Fantke, The USEtox story: a survey of model developer visions and user requirements, Int. J. Life Cycle Assess., 2015, 20, 299–310 CrossRef.
- P. Fantke, L. Aylward, J. Bare, W. A. Chiu, R. Dodson, R. Dwyer, A. Ernstoff, B. Howard, M. Jantunen and O. Jolliet,
et al., Advancements in life cycle human exposure and toxicity characterization, Environ. Health Perspect., 2018, 126, 125001 CrossRef CAS PubMed.
- M. A. Huijbregts, Z. J. Steinmann, P. M. Elshout, G. Stam, F. Verones, M. Vieira, M. Zijp, A. Hollander and R. Van Zelm, ReCiPe2016: a harmonised life cycle impact assessment method at midpoint and endpoint level, Int. J. Life Cycle Assess., 2017, 22, 138–147 CrossRef.
- M. Z. Hauschild, M. Huijbregts, O. Jolliet, M. Macleod, M. Margni, D. Van De Meent, R. K. Rosenbaum and T. E. McKone, Building a model based on scientific consensus for life cycle impact assessment of chemicals: The search for harmony and parsimony, Environ. Sci. Technol., 2008, 42, 7032–7037 CrossRef CAS PubMed.
-
E. Inshakova and O. Inshakov, MATEC web of conferences, 2017, p. 02013 Search PubMed.
-
P. Fantke, M. Bijster, C. Guignard, M. Z. Hauschild, M. A. Huijbregts, O. Jolliet, A. Kounina, V. Magaud, M. Margni, T. E. McKone, L. Posthuma, R. K. Rosenbaum, D. van de Meent and R. van Zelm, USEtox 2.0 Documentation (Version 1), USEtox International Center hosted at the Technical University of Denmark, 2017, p. 208 Search PubMed.
- D. Pennington, P. Crettaz, A. Tauxe, L. Rhomberg, K. Brand and O. Jolliet, Assessing human health response in life cycle assessment using ED10s and DALYs: Part 2 - Noncancer effects, Risk Anal., 2002, 22, 947–963 CrossRef PubMed.
- P. Fantke, W. A. Chiu, L. Aylward, R. Judson, L. Huang, S. Jang, T. Gouin, L. Rhomberg, N. Aurisano, T. McKone and O. Jolliet, Exposure and toxicity characterization of chemical emissions and chemicals in products: global recommendations and implementation in USEtox, Int. J. Life Cycle Assess., 2021, 26(5), 899–915 CrossRef CAS PubMed.
- W. A. Chiu, D. A. Axelrad, C. Dalaijamts, C. Dockins, K. Shao, A. J. Shapiro and G. Paoli, Beyond the RfD: broad application of a probabilistic approach to improve chemical dose– response assessments for noncancer effects, Environ. Health Perspect., 2018, 126, 067009 CrossRef PubMed.
- N. Aurisano, L. Huang, S. Jang, W. Chiu, R. S. Judson, O. Jolliet and P. Fantke, Broadening the chemical coverage to derive human toxicity dose-response factors for non-cancer endpoints, Toxicol. Lett., 2021, 1, S276 Search PubMed.
-
World Health Organization, Guidance document on evaluating and expressing uncertainty in hazard characterization, 2nd edn, 2018 Search PubMed.
- L. T. Haber, M. L. Dourson, B. C. Allen, R. C. Hertzberg, A. Parker, M. J. Vincent, A. Maier and A. R. Boobis, Benchmark dose (BMD) modeling: current practice, issues, and challenges, Crit. Rev. Toxicol., 2018, 48, 387–415 CrossRef PubMed.
- K. Z. Travis, I. Pate and Z. K. Welsh, The role of the benchmark dose in a regulatory context, Regul. Toxicol. Pharmacol., 2005, 43, 280–291 CrossRef CAS PubMed.
- A. F. Filipsson, S. Sand, J. Nilsson and K. Victorin, The Benchmark Dose Method—Review of Available Models, and Recommendations for Application in Health Risk Assessment, Crit. Rev. Toxicol., 2010, 33, 505–542, DOI:10.1080/10408440390242360.
- W. Slob, Benchmark dose and the three Rs. Part I. Getting more information from the same number of animals, Crit. Rev. Toxicol., 2014, 44, 557–567, DOI:10.3109/10408444.2014.925423.
- M. Sajid, Nanomaterials: types, properties, recent advances, and toxicity concerns, Curr. Opin. Environ. Sci. Health, 2022, 25, 100319 CrossRef.
- A. Dhawan, V. Sharma and D. Parmar, Nanomaterials: A challenge for toxicologists, Nanotoxicology, 2009, 3, 1–9 CrossRef CAS.
- K. Schwirn, L. Tietjen and I. Beer, Why are nanomaterials different and how can they be appropriately regulated under REACH?, Environ. Sci. Eur., 2014, 26(1), 1–9 CrossRef.
- D. M. Mitrano, S. Motellier, S. Clavaguera and B. Nowack, Review of nanomaterial aging and transformations through the life cycle of nano-enhanced products, Environ. Int., 2015, 77, 132–147 CrossRef CAS PubMed.
- S. Gottardo, A. Mech, J. Drbohlavová, A. Małyska, S. Bøwadt, J. Riego Sintes and H. Rauscher, Towards safe +PVSOBM/BNF 1–12 | 9 and sustainable innovation in nanotechnology: State-of-play for smart nanomaterials, NanoImpact, 2021, 21, 100297 CrossRef PubMed.
- A. D. López, M. Fabiani, V. L. Lassalle, C. V. Spetter and M. D. Severini, Critical review of the characteristics, interactions, and toxicity of micro/nanomaterials pollutants in aquatic environments, Mar. Pollut. Bull., 2022, 174, 113276 CrossRef PubMed.
- S. A. Mazari, E. Ali, R. Abro, F. S. A. Khan, I. Ahmed, M. Ahmed, S. Nizamuddin, T. H. Siddiqui, N. Hossain, N. M. Mubarak and A. Shah, Nanomaterials: Applications, wastehandling, environmental toxicities, and future challenges – A review, J. Environ. Chem. Eng., 2021, 9, 105028 CrossRef CAS.
- G. V. Lowry, K. B. Gregory, S. C. Apte and J. R. Lead, Transformations of nanomaterials in the environment, Environ. Sci. Technol., 2012, 46, 6893–6899 CrossRef CAS PubMed.
- A. Milosevic, D. Romeo and P. Wick, Understanding Nanomaterial Biotransformation: An Unmet Challenge to Achieving Predictive Nanotoxicology, Small, 2020, 16, 1907650 CrossRef CAS PubMed.
- A. G. Oomen, P. M. Bos, T. F. Fernandes, K. Hund-Rinke, D. Boraschi, H. J. Byrne, K. Aschberger, S. Gottardo, F. Von Der Kammer, D. Kühnel, D. Hristozov, A. Marcomini, L. Migliore, J. Scott-Fordsmand, P. Wick and R. Landsiedel, Concern-driven integrated approaches to nanomaterial testing and assessment-report of the NanoSafety Cluster Working Group 10, Nanotoxicology, 2014, 8, 334–348 CrossRef PubMed.
- O. Schmid and T. Stoeger, Surface area is the biologically most effective dose metric for acute nanoparticle toxicity in the lung, J. Aerosol Sci., 2016, 99, 133–143 CrossRef CAS.
- E. Burello and A. P. Worth, A theoretical framework for predicting the oxidative stress potential of oxide nanoparticles, Nanotoxicology, 2011, 5, 228–235 CrossRef CAS PubMed.
- K. Donaldson and C. L. Tran, An introduction to the shortterm toxicology of respirable industrial fibre, Mutat. Res., Fundam. Mol. Mech. Mutagen., 2004, 553, 5–9 CrossRef CAS PubMed.
- A. Giusti, R. Atluri, R. Tsekovska, A. Gajewicz, M. D. Apostolova, C. L. Battistelli, E. A. Bleeker, C. Bossa, J. Bouillard, M. Dusinska, P. Gómez-Fernández, R. Grafström, M. Gromelski, Y. Handzhiyski, N. R. Jacobsen, P. Jantunen, K. A. Jensen, A. Mech, J. M. Navas, P. Nymark, A. G. Oomen, T. Puzyn, K. Rasmussen, C. Riebeling, I. Rodriguez-Llopis, S. Sabella, J. R. Sintes, B. Suarez-Merino, S. Tanasescu, H. Wallin and A. Haase, Nanomaterial grouping: Existing approaches and future recommendations, NanoImpact, 2019, 16, 100182 CrossRef.
- T. Walser, D. Meyer, W. Fransman, H. Buist, E. Kuijpers and D. Brouwer, Life-cycle assessment framework for indoor emissions of synthetic nanoparticles, J. Nanopart. Res., 2015, 17(6), 1–18 CrossRef CAS.
- W. Fransman, H. Buist, E. Kuijpers, T. Walser, D. Meyer, E. Zondervan-van den Beuken, J. Westerhout, R. H. Klein Entink and D. H. Brouwer, Comparative human health impact assessment of engineered nanomaterials in the framework of life cycle assessment, Risk Anal., 2017, 37, 1358–1374 CrossRef PubMed.
- A. Laurent, J. R. Harkema, E. W. Andersen, M. Owsianiak, E. B. Vea and O. Jolliet, Human health no-effect levels of TiO2 nanoparticles as a function of their primary size, J. Nanopart. Res., 2017, 19, 130 CrossRef.
-
T. Vermeire, M. Pieters, M. Rennen and P. Bos, Probabilistic assessment factors for human health risk assessment, RIVM Technical Report March, Rijksinstituut voor Volksgezondheid en Milieu RIVM, 2001.
- M. A. J. Huijbregts, L. J. A. Rombouts, A. M. J. Ragas and D. van de Meent, Human-toxicological effect and damage factors of carcinogenic and noncarcinogenic chemicals for life cycle impact assessment, Integr. Environ. Assess. Manage., 2005, 1, 181–244 CrossRef CAS PubMed.
- R. K. Rosenbaum, M. A. J. Huijbregts, A. D. Henderson, M. Margni, T. E. McKone, D. van de Meent, M. Z. Hauschild, S. Shaked, D. S. Li, L. S. Gold and O. Jolliet, USEtox human exposure and toxicity factors for comparative assessment of toxic emissions in life cycle analysis: sensitivity to key chemical properties, Int. J. Life Cycle Assess., 2011, 16, 710–727 CrossRef CAS.
- G. Rodriguez-Garcia, B. Zimmermann and M. Weil, Nanotoxicity and Life Cycle Assessment: First attempt towards the determination of characterization factors for carbon nanotubes, IOP Conf. Ser.: Mater. Sci. Eng., 2014, 64, 012029 CAS.
- H. Buist, R. Hischier, J. Westerhout and D. Brouwer, Derivation of health effect factors for nanoparticles to be used in LCIA, NanoImpact, 2017, 7, 41–53 CrossRef.
- M. Pini, B. Salieri, A. M. Ferrari, B. Nowack and R. Hischier, Human health characterization factors of nano-TiO2 for indoor and outdoor environment, Int. J. Life Cycle Assess., 2016, 21, 1452–1462 CrossRef CAS.
- K. Ettrup, A. Kounina, S. F. Hansen, J. A. J. Meesters, E. B. Vea and A. Laurent, Development of Comparative Toxicity Potentials of TiO2 Nanoparticles for Use in Life Cycle Assessment, Environ. Sci. Technol., 2017, 51, 4027–4037 CrossRef CAS PubMed.
- M. P. Tsang, D. Li, K. L. Garner, A. A. Keller, S. Suh and G. W. Sonnemann, Modeling human health characterization factors for indoor nanomaterial emissions in life cycle assessment: a case-study of titanium dioxid, Environ. Sci.: Nano, 2017, 4, 1705–1721 RSC.
- Y. Pu, B. Laratte, R. S. Marks and R. E. Ionescu, Impact of copper nanoparticles on porcine neutrophils: ultrasensitive characterization factor combining chemiluminescence information and USEtox assessment model, Mater. Today Commun., 2017, 11, 68–75 CrossRef CAS.
- B. Salieri, J.-P. Kaiser, M. Rösslein, B. Nowack, R. Hischier and P. Wick, Relative potency factor approach enables the use of in vitro information for estimation of human effect factors for nanoparticle toxicity in life-cycle impact assessment, Nanotoxicology, 2020, 1–12 Search PubMed.
- B. Salieri, D. A. Turner, B. Nowack and R. Hischier, Life cycle assessment of manufactured nanomaterials: Where are we?, NanoImpact, 2018, 10, 108–120 CrossRef.
- F. J. Miller, B. Asgharian, J. D. Schroeter and O. Price, Improvements and additions to the Multiple Path Particle Dosimetry model, J. Aerosol Sci., 2016, 99, 14–26 CrossRef CAS.
- Y. Emara, P. Fantke, R. Judson, X. Chang, P. Pradeep, A. Lehmann, M. W. Siegert and M. Finkbeiner, Integrating endocrine-related health effects into comparative human toxicity characterization, Sci. Total Environ., 2021, 762, 143874 CrossRef CAS PubMed.
- L. Meigs, L. Smirnova, C. Rovida, M. Leist and T. Hartung, Animal testing and its alternatives – the most important omics is economics, Altex, 2018, 35, 275–305 CrossRef PubMed.
-
W. Pimtong, P. Samutrtai, R. Wongwanakul and S. Aueviriyavit, Predictive models for nanotoxicology: in vitro, in vivo, and computational models, Handbook of Nanotechnology Applications, 2021, pp. 683–710 Search PubMed.
- J. Y. Choi, G. Ramachandran and M. Kandlikar, The impact of toxicity testing costs on nanomaterial regulation, Environ. Sci. Technol., 2009, 43, 3030–3034 CrossRef CAS PubMed.
- O. Bondarenko, M. Mortimer, A. Kahru, N. Feliu, I. Javed, A. Kakinen, S. Lin, T. Xia, Y. Song, T. P. Davis, I. Lynch, W. J. Parak, D. T. Leong, P. C. Ke, C. Chen and Y. Zhao, Nanotoxicology and nanomedicine: The Yin and Yang of nanobio interactions for the new decade, Nano Today, 2021, 39, 101184 CrossRef CAS.
- P. Wick, S. Grafmueller, A. Petri-Fink and B. Rothen-Rutishauser, Advanced human in vitro models to assess metal oxide nanoparticle-cell interactions, MRS Bull., 2014, 39, 984–989 CrossRef CAS.
- C. Hempt, C. Hirsch, Y. Hannig, A. Rippl, P. Wick and T. Buerki-Thurnherr, Investigating the effects of differently produced synthetic amorphous silica (E 551) on the integrity and functionality of the human intestinal barrier using an advanced in vitro co-culture model, Arch. Toxicol., 2021, 95, 837–852 CrossRef CAS PubMed.
- J. Kasper, M. I. Hermanns, C. Bantz, M. Maskos, R. Stauber, C. Pohl, R. E. Unger and J. C. Kirkpatrick, Inflammatory and cytotoxic responses of an alveolar-capillary coculture model to silica nanoparticles: Comparison with conventional monocultures, Part. Fibre Toxicol., 2011, 8, 1–16 CrossRef PubMed.
- Y. Wang, A. Adamcakova-Dodd, B. R. Steines, X. Jing, A. K. Salem and P. S. Thorne, Comparison of in vitro toxicity of aerosolized engineered nanomaterials using air-liquid interface mono-culture and co-culture model, NanoImpact, 2020, 18, 100215 CrossRef PubMed.
- L. C. Delon, Z. Guo, A. Oszmiana, C. C. Chien, R. Gibson, C. Prestidge and B. Thierry, A systematic investigation of the effect of the fluid shear stress on Caco-2cells towards the optimization of epithelial organ-on-chip model, Biomaterials, 2019, 225, 119521 CrossRef CAS PubMed.
- H. Chen, Z. Yu, S. Bai, H. Lu, D. Xu, C. Chen, D. Liu and Y. Zhu, Microfluidic models of physiological or pathological flow shear stress for cell biology, disease modeling and drug development, TrAC, Trends Anal. Chem., 2019, 117, 186–199 CrossRef CAS.
- E. Frohlich, Comparison of conventional and advanced in vitro models in the toxicity testing of nanoparticles, Artif. Cells, Nanomed., Biotechnol., 2018, 46, 1091–1107 CrossRef PubMed.
- N. De Souza, Organoids, Nat. Methods, 2018, 15, 23 CrossRef CAS.
- B. Hu, Z. Cheng and S. Liang, Advantages and prospects of stem cells in nanotoxicology, Chemosphere, 2022, 291, 132861 CrossRef CAS PubMed.
-
World Economic
Forum, These are the top 10 emerging technologies of 2016 | World Economic Forum, 2016, https://www.weforum.org/agenda/2016/06/top-10-emerging-technologies-2016/ Search PubMed.
- P. Wick, S. Chortarea, O. T. Guenat, M. Roesslein, J. D. Stucki, S. Hirn, A. Petri-Fink and B. Rothen-Rutishauser, In vitro-ex vivo model systems for nanosafety assessment, Eur. J. Nanomed., 2015, 7, 169–179 Search PubMed.
- Q. Wu, J. Liu, X. Wang, L. Feng, J. Wu, X. Zhu, W. Wen and X. Gong, Organ-on-a-chip: recent breakthroughs and future prospects, J. Geophys. Res. Planets, 2020, 19(1), 1–19 Search PubMed.
- K. K. Comfort, L. K. Braydich-Stolle, E. I. Maurer and S. M. Hussain, Less Is More: Long-Term in Vitro Exposure to Low Levels of Silver Nanoparticles Provides New Insights for Nanomaterial Evaluation, ACS Nano, 2014, 8, 3260–3271 CrossRef CAS PubMed.
- C. Monteiller, L. Tran, W. MacNee, S. Faux, A. Jones, B. Miller and K. Donaldson, The pro-inflammatory effects of low-toxicity low-solubility particles, nanoparticles and fine particles, on epithelial cells in vitro: the role of surface area, Occup. Environ. Med., 2007, 64, 609–615 CrossRef CAS PubMed.
- R. Duffin, L. Tran, D. Brown, V. Stone and K. Donaldson, Proinflammogenic Effects of Low-Toxicity and Metal Nanoparticles In Vivo and In Vitro: Highlighting the Role of Particle Surface Area and Surface Reactivity, Inhalation Toxicol., 2007, 19, 849–856 CrossRef CAS PubMed.
- M. A. Dobrovolskaia and S. E. McNeil, Understanding the correlation between in vitro and in vivo immunotoxicity tests for nanomedicines, J. Controlled Release, 2013, 172, 456–466 CrossRef CAS PubMed.
- J. G. Teeguarden, V. B. Mikheev, K. R. Minard, W. C. Forsythe, W. Wang, G. Sharma, N. Karin, S. C. Tilton, K. M. Waters, B. Asgharian, O. R. Price, J. G. Pounds and B. D. Thrall, Comparative iron oxide nanoparticle cellular dosimetry and response in mice by the inhalation and liquid cell culture exposure routes, Part. Fibre Toxicol., 2014, 11, 46 CrossRef PubMed.
- E. K. Rushton, J. Jiang, S. S. Leonard, S. Eberly, V. Castranova, P. Biswas, A. Elder, X. Han, R. Gelein, J. Finkelstein and G. Oberdörster, Concept of Assessing Nanoparticle Hazards Considering Nanoparticle Dosemetric and Chemical/Biological Response Metrics, J. Toxicol. Environ. Health, Part A, 2010, 73, 445–461 CrossRef CAS PubMed.
- K. Paul Friedman, M. Gagne, L.-H. Loo, P. Karamertzanis, T. Netzeva, T. Sobanski, J. A. Franzosa, A. M. Richard, R. R. Lougee and A. Gissi,
et al., Utility of in vitro bioactivity as a lower bound estimate of in vivo adverse effect levels and in risk based prioritization, Toxicol. Sci., 2020, 173, 202–225 CrossRef PubMed.
- T. Loret, F. Rogerieux, B. Trouiller, A. Braun, C. Egles and G. Lacroix, Predicting the in vivo pulmonary toxicity induced by acute exposure to poorly soluble nanomaterials by using advanced in vitro method, Part. Fibre Toxicol., 2018, 15, 25 CrossRef PubMed.
- K. Donaldson, P. J. A. Borm, G. Oberdorster, K. E. Pinkerton, V. Stone and C. L. Tran, Concordance Between In Vitro and In Vivo Dosimetry in the Proinflammatory Effects of Low Toxicity, Low-Solubility Particles: The Key Role of the Proximal Alveolar Region, Inhalation Toxicol., 2008, 20, 53–62 CrossRef CAS PubMed.
- X. Han, N. Corson, P. Wade-Mercer, R. Gelein, J. Jiang, M. Sahu, P. Biswas, J. N. Finkelstein, A. Elder and G. Oberdörster, Assessing the relevance of in vitro studies in nanotoxicology by examining correlations between in vitro and in vivo data, Toxicology, 2012, 297, 1–9 CrossRef CAS PubMed.
- C. D. Guglielmo, J. D. Lapuente, C. Porredon, D. Ramos-López, J. Sendra and M. Borrás, In Vitro Safety Toxicology Data for Evaluation of Gold Nanoparticles–Chronic Cytotoxicity, Genotoxicity and Uptake, J. Nanosci. Nanotechnol., 2012, 12, 6185–6191 CrossRef PubMed.
- S. Phuyal, M. Kasem, L. Rubio, H. L. Karlsson, R. Marcos, V. Skaug and S. Zienolddiny, Effects on human bronchial epithelial cells following low-dose chronic exposure to nanomaterials: A 6-month transformation study, Toxicol. In Vitro, 2017, 44, 230–240 CrossRef CAS PubMed.
- T. Thurnherr, C. Brandenberger, K. Fischer, L. Diener, P. Manser, X. Maeder-Althaus, J. P. Kaiser, H. F. Krug, B. Rothen-Rutishauser and P. Wick, A comparison of acute and long-term effects of industrial multiwalled carbon nanotubes on human lung and immune cells in vitro, Toxicol. Lett., 2011, 200, 176–186 CrossRef CAS PubMed.
- S. Chortarea, H. Barosova, M. J. D. Clift, P. Wick, A. Petri-Fink and B. Rothen-Rutishauser, Human Asthmatic Bronchial Cells Are More Susceptible to Subchronic Repeated Exposures of Aerosolized Carbon Nanotubes At Occupationally Relevant Doses Than Healthy Cells, ACS Nano, 2017, 11, 7615–7625 CrossRef CAS PubMed.
- P. G. Welling, Differences between pharmacokinetics and toxicokinetics, Toxicol. Pathol., 1995, 23, 143–147 CrossRef CAS PubMed.
- L. Lamon, D. Asturiol, A. Vilchez, J. Cabellos, J. Damásio, G. Janer, A. Richarz and A. Worth, Physiologically based mathematical models of nanomaterials for regulatory toxicology: A review, Comput. Toxicol., 2019, 9, 133–142 CrossRef CAS PubMed.
- W. Slob, Uncertainty Analysis in Multiplicative Models, Risk Anal., 1994, 14, 571–576 CrossRef.
- W.-S. Cho, R. Duffin, C. A. Poland, A. Duschl, G. J. Oostingh, W. MacNee, M. Bradley, I. L. Megson and K. Donaldson, Differential pro-inflammatory effects of metal oxide nanoparticles and their soluble ions in vitro and in vivo ; zinc and copper nanoparticles, but not their ions, recruit eosinophils to the lungs, Nanotoxicology, 2012, 6, 22–35 CrossRef CAS PubMed.
- B. A. Weldon, W. C. Griffith, T. Workman, D. K. Scoville, T. J. Kavanagh and E. M. Faustman, In vitro to in vivo benchmark dose comparisons to inform risk assessment of quantum dot nanomaterials, Wiley Interdiscip. Rev.: Nanomed. Nanobiotechnol., 2018, 10, e1507 Search PubMed.
- T. Hartung, Opinion versus evidence for the need to move away from animal testing, Altex, 2017, 34, 193–200 CrossRef PubMed.
- D. B. Warheit, Pulmonary Bioassay Methods for Evaluating Hazards Following Exposures to Nanoscale or Fine Particulate Materials, Assess. Nanopart. Risks Hum. Health, 2011, 99–108 CAS.
- D. B. Warheit, R. Kreiling and L. S. Levy, Relevance of the rat lung tumor response to particle overload for human risk assessment—Update and interpretation of new data since ILSI 2000, Toxicology, 2016, 374, 42–59 CrossRef CAS PubMed.
- B. Wang and G. Gray, Concordance of Noncarcinogenic Endpoints in Rodent Chemical Bioass, Risk Anal., 2015, 35, 1154–1166 CrossRef PubMed.
- G. A. Van Norman, Limitations of Animal Studies for Predicting Toxicity in Clinical Trials: Is it Time to Rethink Our Current Approach?, JACC, 2019, 4, 845–854 Search PubMed.
- R. Hischier, Framework for LCI modelling of releases of manufactured nanomaterials along their life cycle, Int. J. Life Cycle Assess., 2014, 19(4), 838–849 CrossRef CAS.
- D. Romeo, B. Salieri, R. Hischier, B. Nowack and P. Wick, An integrated pathway based on in vitro data for the human hazard assessment of nanomaterials, Environ. Int., 2020, 137, 105505 CrossRef CAS PubMed.
|
This journal is © The Royal Society of Chemistry 2022 |
Click here to see how this site uses Cookies. View our privacy policy here.