Use of remote sensing to assess vegetative stress as a proxy for soil contamination†
Received
30th October 2023
, Accepted 16th November 2023
First published on 23rd November 2023
Abstract
We report, for the first time, a multimodal investigation of current crude oil reprocessing and storage sites to assess their impact on the environment after 50 years of continuous operation. We have adopted a dual approach to investigate potential soil contamination. The first approach uses conventional analytical techniques i.e. energy dispersive X-ray fluorescence (ED-XRF) for metal analysis, and a complementary metabolomic investigation using hydrophilic liquid interaction chromatography hi-resolution mass spectrometry (HILIC-MS) for organic contaminants. Secondly, the deployment of an unmanned aerial vehicle (UAV) with a multispectral image (MSI) camera, for the remote sensing of vegetation stress, as a proxy for sub-surface soil contamination. The results identified high concentrations of barium (mean 21
017 ± 5950 μg g−1, n = 36) as well as metabolites derived from crude oil (polycyclic aromatic hydrocarbons), cleaning processes (surfactants) and other organic pollutants (e.g. pesticides, plasticizers and pharmaceuticals) in the reprocessing site. This data has then been correlated, with post-flight data analysis derived vegetation indices (NDVI, GNDVI, SAVI and Cl green VI), to assess the potential to identify soil contamination because of vegetation stress. It was found that strong correlations exist (an average R2 of >0.68) between the level of soil contamination and the ground cover vegetation. The potential to deploy aerial remote sensing techniques to provide an initial survey, to inform decision-making, on suspected contaminated land sites can have global implications.
Environmental significance
We report, for the first time, a multimodal investigation of a current crude oil reprocessing and a related storage site with respect to their impact on the environment after 50 years of continuous operation. Using both conventional elemental analysis and metabolomic profiling has enabled identification of some key pollutant markers. This laboratory-based activity has been corroborated using an unmanned aerial vehicle with multispectral image camera to generate various vegetation indices. The potential of deploying a UAV with MSI camera to contribute useful diagnostics on potential sub-surface contamination from former historical industrial or brown-field sites as part of an initial site risk assessment, and prior to ground truth chemical analyses, is presented.
|
Introduction
There are various pathways by which human exposure to contaminants usually takes place; these include ingestion, inhalation, and dermal route.1,2 Inhalation is the primary route of human exposure to contaminants, with occupational exposure being the main source.2 Although, the severity of the impact is dependent upon the concentration of the contaminant, the duration of exposure and the susceptibility of the receptor are also important. The assessment of potential health risks to people living or working on or around contaminated land sites is usually regulated by national governments. In England, the Environmental Agency is the regulating body with the responsibility of controlling the activities which can harm the environment.3 The Environmental Protection Act 1990 (Part 2A) was put in place to effectively manage the risk from contaminated land and thus guarantee safe human health, property, and ecosystem. The Statutory Guidance (SG) for Part 2A of the Environmental Protection Act 1990 has been reviewed to a four-category system for classifying land under Part 2A, ranging from Category 4, where the risk level is acceptably low, to Category 1, where the level of risk is not acceptable.2
Investigation of pollutants (metals/organics) from industrial activities, whether historic or contemporary often focuses on the negative aspects. Often the focus is on ways in which organisations working with legislators can remediate so-called historic or legacy brown field sites as part of a regeneration of the geographical area. It is well known that anthropogenic activities significantly contribute to global environmental issues.4 The accumulation of so-called heavy metals and organic pollutants resulting from former industrial activities can cause issues in various environmental compartments i.e. soil, water, and the atmosphere. The presence of heavy metals and organic pollutants in soil and water has become a significant concern worldwide due to their potentially harmful effects on human health and the environment.5,6 However, few published studies can investigate extant sites that have been solely developed and occupied by one owner (organisation) for the past 50 years.
Research on oil-contaminated sites has shown the potential for long-term persistence of pollutants and the need for comprehensive monitoring and remediation approaches. The assessment of heavy metals and organic pollutants in soil from an oil-contaminated site is essential to determine the potential risks and develop remediation strategies.7,8 The production, processing, transportation, and utilization of oil can cause serious soil pollution.9,10 The extraction and processing of crude oil can lead to accidental spills or leaks, which can contaminate the soil and ultimately the environment with heavy metals (e.g. As, Cd, Cr, Cu, Hg, Ni, Pb and Zn), as well as organic contaminants (e.g. petroleum alkane hydrocarbons, polycyclic aromatic hydrocarbons) and other important emerging pollutants.11–13
In addition to conventional analytical techniques for soil analyses,14 that routinely use sampling, sample storage, and sample preparation for metals e.g. energy dispersive X-ray fluorescence spectrometry (ED-XRF) and organic pollutants e.g. gas or liquid chromatography coupled to mass spectrometry, other non-invasive approaches based on remote sensing are possible. Effective monitoring of vegetation can be performed using remote sensing techniques i.e. unmanned aerial vehicles (UAVs) and spaceborne sensors, and be used to determine plant health and hence potential stressors from pollution.15 In this situation, the detection of vegetation stress, because of underlying soil contamination, can be used as a bio-indicator of the soil conditions. Previous reported research15,16 has investigated the spectral signatures of vegetation grown on contaminated soils and highlighted the importance of reflected radiation in the visible and near-infrared regions of the electromagnetic spectrum. The suitability of these approaches relies on the fact that the plant pigment chlorophyll is spectrally active between 500 and 900 nm and its presence correlate with vegetative vigour and health and potentially signals contamination by crude oil compounds.17 Hence, remotely sensed imaging provides the ability to detect oil spill-contaminated lands through changes to vegetation. For instance, satellite imagery data has been used to map the spectral reflectance of vegetation stress in oil spillage on land in New Mexico.18 Further, using Landsat 8 imagery data, multiple vegetation spectral indices have been tested to monitor the impact of oil spills on vegetation.19 The results of the study outlined a statistically significant difference on the vegetation index between the land which had suffered an oil spill and land that had not. In addition, a machine learning model, based on a random forest classification was used based on satellite imagery data to differentiate oil contaminated and non-contaminated vegetation in Niger Delta.20 However, most of the remote sensing studies monitoring vegetation health in oil contaminated sites use satellite platforms where the spatial resolution is not suitable for analysing at the level of a single industrial site. In addition, coarse spatial resolution obtained from satellites sensors is not suitable for monitoring dense vegetation and not adapted to monitoring of low-lying vegetation. The available multispectral data is often interrogated using simple, but effective, algorithms that allow analysis of vegetation. Some of the developed vegetation indices, VI's,15,21,22 can be used to assess vegetative stress, as a proxy of soil contamination. The availability of commercially available UAVs with a multispectral image (MSI) camera, that cover the visible and near-infrared regions, provides opportunities for low cost and rapid vegetation health monitoring. The deployment of UAVs as remote sensing platforms offers temporal, cost-effective and high spatial resolution (at the cm scale) to monitor vegetation affected by oil spills.23 No studies, to date, have been performed using a UAV with a multispectral image camera to monitor low lying vegetated areas in oil contaminated sites with correlated ground truth data.
This research sets out to investigate two different, but connected sites, within the ConocoPhillips Teesside Operations facility for refining of crude oil and storage that have been developed and used by them exclusively over the past 50 years. ConocoPhillips is a multinational energy company involved in the exploration and production of oil and gas across various regions of the world. It operates several oil and gas production sites worldwide, and the contamination of soil due to oil spills and leaks is a constant concern relating to environmental contamination as well as health and safety issues. The first aim is to assess the composition of the soil environment with respect to heavy metals and organic compound metabolites. The second aim is to use derived VI's from a remote sensing UAV with a multispectral image camera to examine vegetative stress, as a proxy for the underlying soil environment. Finally, this work assesses the long-term chemical impact on the environment from sites used for crude oil reprocessing and storage.
Experimental
Field site and sampling
The Teesside Terminal, the 500-acre Main Site is located at Seal Sands, on the River Tees estuary, in north east England. It comprises a large oil and gas processing terminal facility and nearby storage site, Fig. 1. The original site was development in 1969, Fig. S1(a),† as part of a major land reclamation programme to create deepwater loading berths for sea going tankers. The production site itself was commissioned in 1975 to receive and process unstabilised crude oil and natural gas liquids (NGLs) from the Ekofisk field development in the Norwegian sector of the North Sea. The crude and NGL blend are delivered from the Ekofisk field via a 355 km (220 mile) long 34′′ diameter pipeline. The Main Site at the Teesside Terminal receives crude oil continuously, ‘stabilises’ it by removing volatile natural gas liquids, processes the NGLs into volatile alkane products e.g. propane and butane. It then pipes the crude oil and NGL products 2 miles away to a connected storage tank facility (375 acre), Site B at Greatham, Fig. S1(b).† From this site these products go into sea going tankers for global distribution to refineries and petrochemical plants.
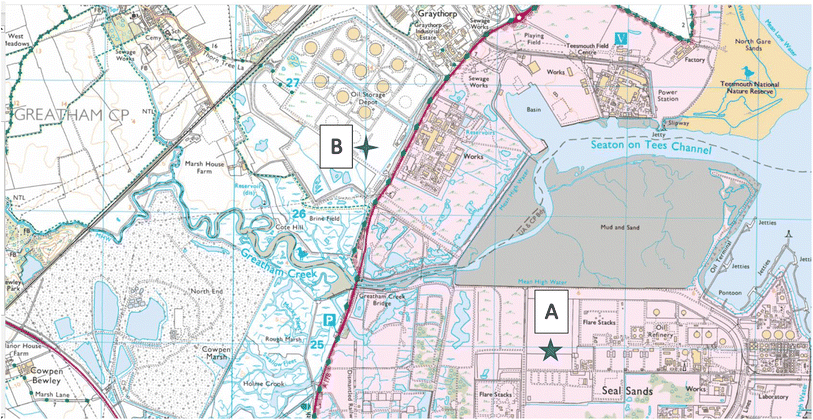 |
| Fig. 1 Sampling locations of Site A (Main Site Teesside Refinery) and Site B (Greatham Storage Depot). | |
Soil sampling was done on the 13 April 2022 in two areas: the former “washing” facility on the Main Site, Site A, Fig. S1(c) and (e)† and the “unused” land reclaimed area of the Storage Depot, Site B, Fig. S1(d) and (f).† Thirty six individual grab samples were collected from bed 3, on the “washing” facility, on an approximate 6 × 6 sampling grid from the Main Site, Site A, Fig. 1. For comparison 9 individual grab samples were collected on the “unused” Storage Depot site, Site B, Fig. 1. All sub-surface soil samples, between 2–10 cm depth, were collected using a stainless-steel trowel, and the sampling coordinates noted by a handheld GPS unit. To avoid cross-contamination, the trowel was cleaned with a new sterile wipe, between each sample. The collected soil samples were placed in labelled collection bags i.e. Kraft sample bags, and transported back to the laboratory for subsequent soil analysis. In the laboratory, all soil samples were air-dried to minimise loss of organic compounds (48 hours), then ground with a mortar and pestle, and finally sieved through a 250 μm nylon mesh.24 Each of the sieved soil samples were subsequently sub-divided into two: one portion was immediately frozen to reduce soil-microbial activity (−18 °C) for subsequent chromatographic analysis while the other portion was stored for analysis by energy dispersive XRF.
Metal analysis by X-ray fluorescence spectrometry
Accurately weighed ground soil sub-samples (approximately 4 g) were thoroughly mixed with Hoeschwax hydrocarbon binder (approximately 0.7 g) using a Retsch mixer mill MM 400 (Retsch, Germany) before being pressed at 10-tonne pressure, using a Specac (Specac Ltd, Kent, UK) manual hydraulic press, into pellets for subsequent analysis. Care was taken to eliminate any cross-contamination between each soil sample. The benchtop ED-XRF instrument, Spectro Xepos (Spectro Analytical Instruments, Kleve, Germany), is equipped with a detector that is comprised of a microprocessor-controlled drift detector with Peltier cooling. An ED-XRF carousel which can accommodate 8 samples was used for sequential analysis of the pressed pellets.
Data processing was done using the Geochemistry Traces method, as supplied by the ED-XRF manufacturer (Spectro Analytical Instruments). Quality control of the generated data for the 10 elements of choice (As, Ba, Ni, Pb, Rb, Sr, V, Y, Zn, Zr) was performed by analysis of six certified reference materials (CRMs). The six selected CRMs were: GBW 07403 (a yellow-brown soil); GBW 07405 (yellow-red soil, polymetallic ore area); GBW 07406 (red soil, polymetallic ore area); GBW 07411 (soil); GBW 07313 (marine sediment) and SRM 2710 (Montana I soil) were obtained from LGC-Promochem Ltd, London. The GBW CRMs are produced by the National Research Center for Certified Reference Materials (NRCCRM), China and the SRM CRM was produced by the National Institute of Standards and Technology, NIST, USA. Data from the CRMs was used to correct, by use of the generated linear regression equations, the sample element concentrations. In addition, determination of the lower limit of detection (LLOD) and limit of quantitation (LOQ) was done using replicate preparation of the calibration curve (n = 6) using the following equations: LLOD = 3.3σ/s and LOQ = 10σ/s, where σ is the standard deviation of the intercept and s is the slope of the calibration graph.
Metabolomic analysis by hydrophilic liquid interaction chromatography hi-resolution mass spectrometry (data dependent analysis)
Metabolite sample extraction.
Defrosted soil sub-samples (approximately 20 mg) were extracted using methanol (1 ml) by sonication (20 min) in an ice water bath using a previously optimised method.25 The supernatant was then recovered, centrifuged (15
000 rpm, for 15 min at 4 °C), and then evaporated to dryness, in a vacuum concentrator (Concentrator plus, Eppendorf UK Ltd, Stevenage, UK) under reduced pressure (20 mbar) and elevated temperature (45 °C) for 2 hours, prior to analysis. When analysis was to be performed extracts were re-suspended in 100 μl of acetonitrile: water (95
:
5% v/v) and sonicated for 15 min. Finally, the supernatant was filtered (0.22 μm, micro costar spin column) at 10
000 rpm for 10 min at 4 °C, prior to analysis. Hydrophilic liquid interaction chromatography (HILIC) metabolite profiling of selected soil extracts from Site A (n = 5) and Site B (n = 5) was performed on a Vanquish Liquid Chromatography chromatographic separation system connected to an ID-X High Resolution Mass Spectrometer (Thermo Scientific, Hemel Hempstead, UK). See ESI† for further details. The metabolomic ESI+ and ESI− data sets were processed via Compound Discoverer 3.2 using the following settings: untargeted metabolomic workflow, parent ion mass tolerance 10 ppm, alignment model adaptive curve, minimum intensity 3 signal to noise ratio (S/N) threshold 0.3 min, compound consolidation, retention time tolerance 0.3 min. Database matching were performed at MS2 level using Thermo Scientific online mzCloud databases (Hemel Hempstead, UK) with a similarity index of 70% or higher. The pooled quality control (QC) data was used to assess for instrumental draft and feature selection, the sum of the QC RSD variation across positive (ESI+) ionization mode and negative (ESI−) ionization mode were 2.34% and 0.81%, respectively. Each MS/MS ID metabolite which had a RSD variation of 25% was retained for multivariate analysis.26,27
Metabolomics data sets for Site A (Main Site) and Site B (Storage Depot) in both positive (ESI+) and negative (ESI−) ionization modes were merged and normalised together. The data was auto scaled and log10 transformed prior to Principal Component Analysis (PCA) visualisation for trend assessment. All data visualisation tools i.e. hierarchical cluster analysis, supervised partial least squares-discriminant analysis (PLS-DA) and unsupervised PCA were generated using metaboanalyst.28
Unmanned aerial vehicle.
A multirotor UAV (DJI Phantom 4, supplied by Coptrz Ltd, Leeds, UK) was used with a multispectral camera, stabilized with a 3-axis gimbal, with a 5 camera-array covering the blue (450 ± 16 nm), green (560 ± 16 nm), red (650 ± 16 nm), red edge (730 ± 16 nm) and near-infrared (840 ± 26 nm) spectra, with an additional camera that can also provide live images in RGB (visible) mode. The camera lenses had a field of view of 62.7°, a focal length of 5.74 mm, with the autofocus set at ∝, and an aperture of f/2.2. In all cases, the camera was angled perpendicular to the ground, with data capture occurring in the “hover and capture” mode. At the Main Site (Site A), 426 image files were gathered over 72 waypoints, as 16-bit TIF files corrected for ambient radiance values. The UAV speed was 5.0 m s−1 and had an average height of 38.2 m for the 605 m flight distance. Whereas at the Storage Depot (Site B), 588 image files were gathered over 172 waypoints, as 16-bit TIF files corrected for ambient radiance values. The UAV speed was 5.0 m s−1 and had an average height of 37.8 m for the 1415 m flight distance. All flights were recorded with a resolution of 2.0 cm per px, a front overlap ratio of 75%, a side overlap ratio of 60% and a course angle of 90°. Specific weather conditions, relating to daytime temperature during flight, wind speed and direction were recorded using a handheld anemometer (Benetech® GM816, Amazon UK), and noted as 8–12 °C, 0–2 mph and in a northerly direction.
UAV photogrammetric processing.
The multispectral UAV images were used to create an orthomosaic image, Fig. 3(a) and (b) (Agisoft Metashape Professional (64 bit) software v.1.7.1, Agisoft LLC, St. Petersburg, Russia). Initially, the aerial images were first merged, and aligned to create a sparse point cloud, by matching similar image attributes. Then, the images were precisely positioned to create a 3D point cloud based on the GPS coordinates of each image, and formed into a solid mesh model. Finally, an orthomosaic image was created using the WGS 1984 Web Mercator coordinate system.
Vegetation index calculation.
ArcGIS Pro software was used for calculating the four green vegetation indices using multispectral images from Site A (Main Site) and Site B (Storage Depot). The most common VI is the normalised difference vegetation index (NDVI) which has a sensitive response to green vegetation but can be affected by soil brightness and soil colour.29 It can be derived by calculating the reflectance of the NIR and red orthomosaic images (eqn (1)). The green normalised difference vegetation index (GNDVI), was developed as an indicator of photosynthetic activity (or “greenness”)22,30 and hence is more sensitive to chlorophyll variation. It can be calculated by using the reflectance of NIR and green VI's.22,31 It can be calculated by using the reflectance of NIR and red orthomosaic images and a soil brightness factor (L) defined as 0.5 (eqn (3)). Finally, the chlorophyll green vegetation index (CI green VI) was developed to enhance the estimation of chlorophyll.32,33 It can be calculated by using the reflectance of NIR and green orthomosaic images (eqn (4)). In addition, ArcGIS Pro was used to create raster profiles for barium, C12-AS and tetradecylsulfate distributions on Site A and Site B using the sampled map coordinates. | 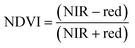 | (1) |
| 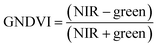 | (2) |
| 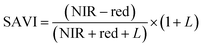 | (3) |
| 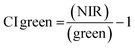 | (4) |
Statistical analysis
For statistical analysis 108 vegetation index values from NDVI, GNDVI, SAVI and CI green were extracted within a 2 m radius from the GPS sampling point from Site A (Main Site), Fig. 2(a) and Site B (Storage Depot), Fig. 2(b). Afterwards, to compare the vegetation indices (NDVI, GNDVI, SAVI and CI green) from Site A (Main Site) and Site B (Storage Depot) statistical t-test was performed. If p < 0.05 the VI are statistically significant indicating there is a difference between the vegetation index from Site A (Main Site) and Site B (Storage Depot). Likewise, if p > 0.05 the VI means are statistically insignificant indicating there is no difference between the vegetation index from Site A (Main Site) and Site B (Storage Depot).
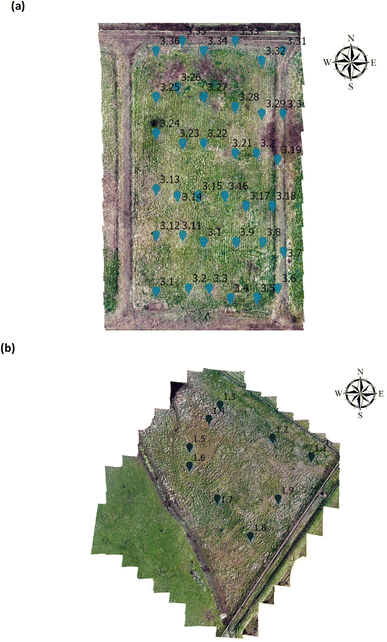 |
| Fig. 2 Arcgis orthomosaic image for analysis on the (a) Main Site Teesside Refinery (Site A) and (b) Greatham Storage Depot (Site B). | |
Results and discussion
ED-XRF analysis of soil samples
Soil samples were analysed for the elements As, Ba, Ni, Pb, Rb, Sr, V, Y, Zn, and Zr from both sites (Site A, Main Site and Site B, Storage Depot) using EDXRF. Quality control of EDXRF analyses was done by analysis of the six CRMs. Excellent correlation between certified and measured concentrations (typically >0.99) were reported (Table S1†), while typical LLODs varied between 0.5 μg g−1 for Sr and Y, and 24.3 μg g−1 for Zn, and LOQs varied between 1.4 μg g−1 for Sr and 73.6 μg g−1 for Zn. Intra-precision data was completed at the lower and higher concentration, per element with typical data varying between 0.2% RSD and 2.4% RSD (n = 6). The full results for the 10 elements in the soil samples are reported in Table S2,† as the mean concentration (and two replicates). The replicate values were determined independently by different individuals on different days. The results were compared with data from the UK Soil Observatory database34 to the nearest publicly accessible point to Site A and Site B (Table 1). Analysis of the determined element concentration and the UKSO database concentration indicated stepwise differences of generally <10× for all elements across both sites, except for Ba on the main site (Site A) which had a difference of >×100.
Table 1 A summary of the concentration (μg g−1) of elements on the Main Site Teesside Terminal (Site A) and Greatham Oil Storage Depot (Site B), compared to the nearest UKSO data for Site A and Site Ba
Site |
Information |
V |
Ni |
Zn |
As |
Rb |
Sr |
Y |
Zr |
Ba |
Pb |
Site A: Conoco Phillips, Main Site Teesside Terminal, Seal Sands. A 285-acre site investigating the middle (number 3) of 5 available beds. Site B: Greatham Oil Storage Depot, Greatham. A 375-acre site investigating the periphery of the accessible area. + Determined using wavelength dispersive X-ray fluorescence spectrometry using a 1 km grid resolution. UKSO data was reported outside the restricted and controlled areas sampled within this study (https://www.ukso.org/).
|
A |
Mean concentration ± SD (n = 36) |
327 ± 21 |
53.7 ± 4.2 |
142 ± 196 |
12.4 ± 1.3 |
60.8 ± 3.7 |
295 ± 69 |
20.9 ± 2.4 |
221 ± 15 |
21 017 ± 5950 |
91.3 ± 7.8 |
UKRO34 |
Concentration |
51.4 |
12.8 |
86.9 |
14.4 |
36.4 |
109.3 |
11.3 |
96.8 |
206.1 |
68.5 |
|
x Difference |
7.0 |
4.3 |
1.6 |
0.9 |
1.7 |
2.7 |
1.9 |
2.3 |
101 |
1.4 |
B |
Mean concentration ± SD (n = 9) |
378 ± 52 |
59.4 ± 3.7 |
255 ± 216 |
21.4 ± 13.2 |
81.9 ± 9.1 |
103 ± 13 |
24.1 ± 1.6 |
193 ± 23 |
3796 ± 672 |
152 ± 95 |
UKRO34 |
Concentration |
112.4 |
35.7 |
233.6 |
29.7 |
90.9 |
126.7 |
24.9 |
219.3 |
394.7 |
198.0 |
|
x Difference |
3.5 |
1.7 |
1.1 |
0.7 |
0.9 |
0.8 |
1.0 |
0.9 |
9.5 |
0.8 |
UKRO data34 |
LLoD+ |
2.7 |
1.3 |
1.1 |
2.4 |
0.7 |
0.8 |
2.4 |
0.8 |
0.25 |
1.2 |
Precision+ |
2% |
3% |
3% |
5% |
1.5% |
1% |
12% |
2% |
8% |
1.5% |
The significantly higher barium concentrations in Site A (Main Site) compared to Site B (Storage Depot) is noted. The elevated concentration of barium in the soil samples obtained from Site A, can be attributed to the presence of barium sulfate, an essential component that is extensively utilized in the oil and gas industry. Barium sulfate is commonly found in drilling mud, as a fluid that is injected into oil or gas wells to facilitate various operations. This includes lubrication of the drilling equipment, removal of rock debris, preventing well wall collapse, and mitigating the risk of blowouts in the event of encountering over-pressured strata.35 The prevalence of barium ions in the vicinity of oil and gas processing sites is well-documented, particularly in treated wastewaters generated during drilling activities.36 These traces are known to persist and contribute to the overall barium levels in the surrounding environment, including the soil samples collected from Site A. Additionally it is known that the soil samples' origin on Site A was formerly used for the cleaning of components linked to the facilities operations.
Metabolomic analysis using HILIC-MS
Metabolomic profiling of soil samples (n = 10) from both sites identified 91 metabolites; 33 in positive mode (ESI+) and 58 in negative mode (ESI−), with a relative standard deviation of <25% within the 3 quality control (QC) samples. The PCA visualization of the 10 soil samples, five from Site A (sample number 3.7, 3.14, 3.16, 3.27 and 3.31) and 5 from Site B (sample number 1.3, 1.4, 1.5, 1.6 and 1.7), identified two distinct clusters that were explained by 48.1% of the total variance, Fig. 3(a). The two clusters display a distinct phenotype, which are separated via a planar separation across the PC1 space. The hierarchical clustering dendrogram, shown in Fig. 3(b) further illustrates the distinct separation of the two groups (Site A and Site B) with the positioning of the pooled QC sample appropriately identified between the two groups.
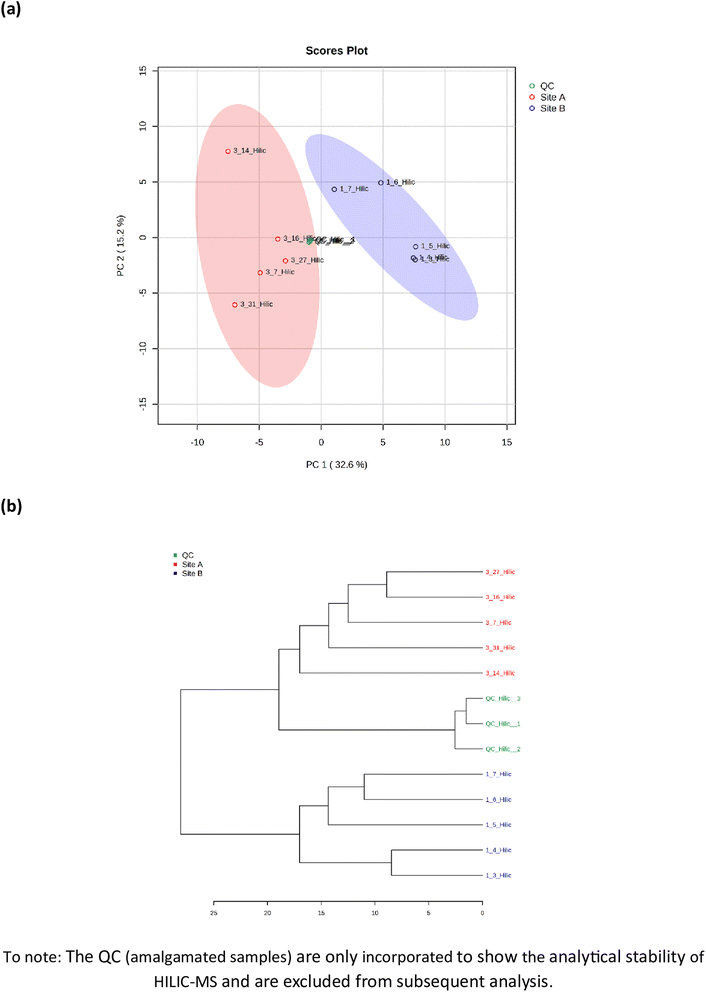 |
| Fig. 3 Metabolomic analysis by hydrophilic liquid interaction chromatography mass spectrometry of soil samples from Site A (Main Site) and Site B (Storage Depot). (a) Principal component analysis of the 91 identified metabolites, and (b) the hierarchical clustering dendrogram showing a measure of sample similarity. | |
The variable importance in projection (VIP) scores for the 25 most abundant metabolites which are statistically significant (x > 1.2), have been calculated and is shown in Fig. 4. The metabolites with a high VIP score (x > 1.2), have a higher discriminating contribution, compared to those with the lower VIP scores (x < 1.2). It is noted that Site A (Main site), has 16 important metabolites while Site B (Storage Depot) has 9 important metabolites (all highlighted in red), and no coincident metabolites. The major metabolites identified in Site A (Main Site) include surfactants i.e. long chain alkyl ethoxylates, such as, C12-AE1S and C12-AS, as well as dimethyl(tetradecyl)amine and tetradecylsulfate; pesticides, a cereal crop fungicide, fenpropidin and a herbicide, atraton; environmental persistent pharmaceutical pollutants, dibucaine and mianserin; and a global ‘forever’ pollutant, perfluorooctanesulfonic acid. The major metabolites identified in Site B (Storage Depot) are more aligned with naturally occurring compounds from plants, animals and microorganisms and include a diverse range of fatty acids, such as, lignoceric acid and oleic acid, phospholipids, such as, PG 32
:
1 and PC (16
:
O/18
:
1)(9Z), as well as a surfactant, dodecylbenzenesulfonic acid, and mannitol which is used as a sweetener in the formulation of oral medication.
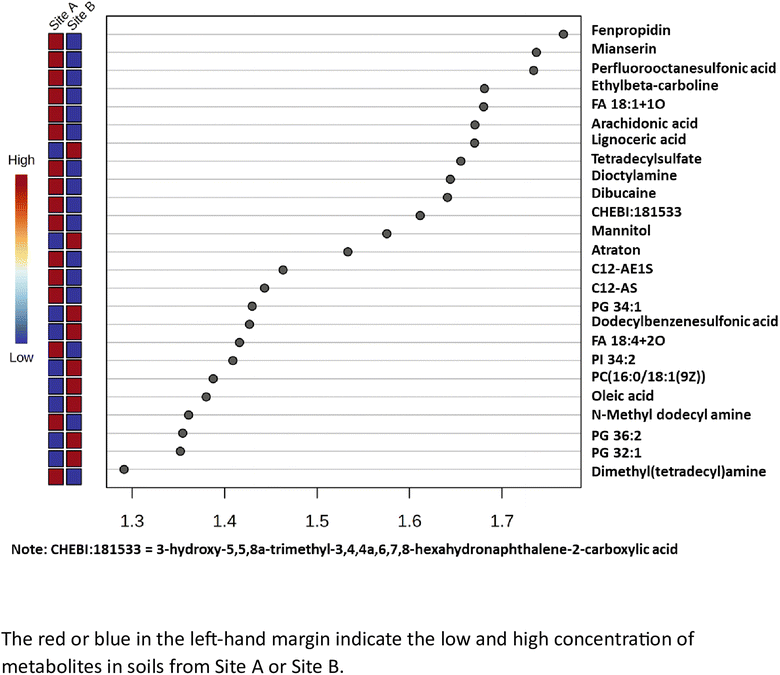 |
| Fig. 4 The partial least squares – discriminant analysis (PLS-DA) variable importance projection (VIP) scores for the top 25 metabolites. | |
The average heat map response for all 91 metabolites, across both Site A and Site B, is shown in Fig. 5. The distinctiveness of the metabolites from both sites is clear, with no overlap, irrespective of whether using ESI+ or ESI−. Other significant metabolites which predominate across the sites include a range of polycyclic aromatic hydrocarbons or their metabolites. This included, 10-azabenzo[a]pyrene, a known metabolic transformation product of benzo[a]pyrene (BaP), a known environmental carcinogen; dibenzo[a,j]acridine, formed from the incomplete burning of organic matter; and benz[c]acridine, which is released from stack effluents from residential coal-burning furnaces and incineration effluents from oil refineries. Another metabolite identified was dibutyl sebacate, a di butyl ester of sebacic acid, which is used as a plasticizer in the production of plastics and many synthetic rubbers, especially nitrile rubber and neoprene, which have been industrially important in the Tees Valley chemical zone. Finally, box and whisker plots (Fig. 6) show the relative abundance profiles for four key metabolites which are more abundant in Site A (Main Site). These include two surfactants i.e. C12-AS, a metabolite of the non-ionic surfactant, alkylethoxylate, and tetradecylsulfate, as its sodium salt, an anionic surfactant. In addition, plots are shown for a ‘forever’ pollutant i.e. perfluorooctanesulfonic acid, and a fungicide i.e. fenpropidin. All plots clearly show the significant concentration differences between Site A (in red) and Site B (in blue).
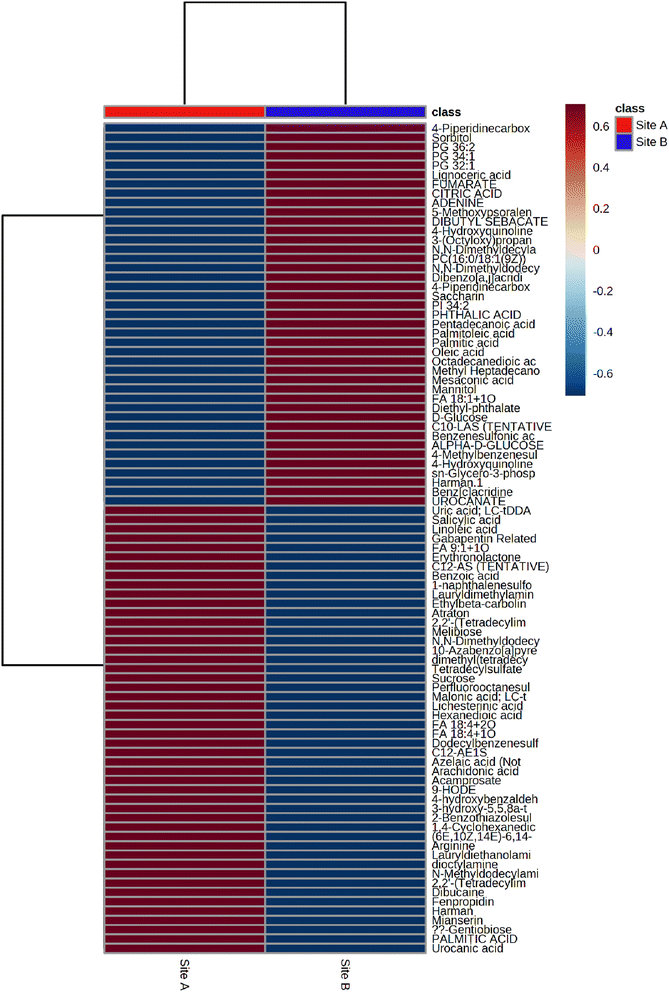 |
| Fig. 5 the average heat map response for all 91 metabolites across Site A and Site B. | |
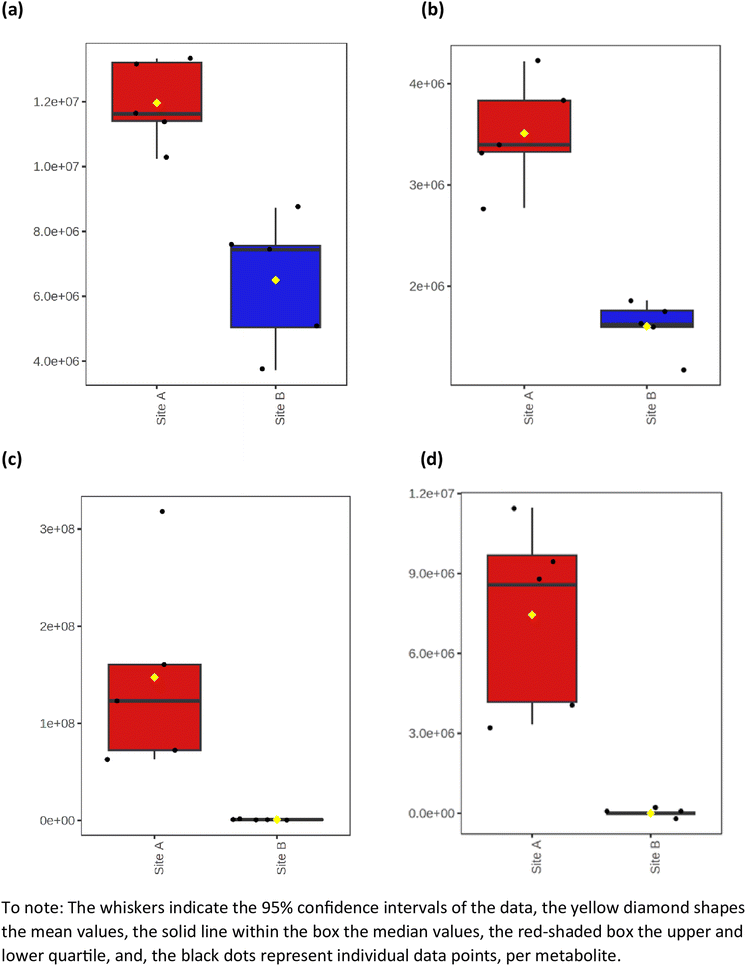 |
| Fig. 6 Box and whisker plots illustrating the relative abundance differences between some key metabolites in Site A and Site B. (a) C12-AS, (b) tetradecylsulfate, (c) perfluorooctanesulfonic acid, and (d) fenpropidin. | |
Consideration of the two contrasting sites, and their current and former uses, is crucial in providing an understanding to what metabolites have been identified in the soil sub-samples. Site A, which is part of the Main Site, has the capacity to receive up to 1 million barrels per day of crude oil. Within the site, processing of the crude oil is done by fractionation. In addition, this site also fractionates NGLs into ethane, propane, and butane. The specific areas sampled within this site, Fig. S1(c),† have a known history for washing of industrial components linked to the facilities operations, it is therefore not surprising that metabolites linked to the surfactants, C12-AS, C12-AE1S, dimethyl(tetradecyl)amine and tetradecylsulfate, have been identified. Other metabolites linked to oil processing and refining have also been identified, specifically, 10-azabenzo[a]pyrene, dibenzo[a,j]acridine, and benz[c]acridine. In addition, analysis has also identified perfluorooctanesulfonic acid (PFOS), an anthropogenic chemical that is identified as a persistent organic pollutant (POP) by the Stockholm Convention on Persistent Organic Pollutants (2001).37 In addition, the pesticides, fenpropidin and atraton, have been determined in Site A. As this is a non-agricultural site, the presence of the pesticides could be due to wind dispersal from local crop spraying. It is not surprising to find ubiquitous pollutants, such as, dibutyl sebacate, a plasticizer. Other metabolites relating to pharmaceutical pollutants, namely, dibucaine and mianserin, are less attributable to site activities, however, and could be linked to airborne, as well as ground and surface water run-off from neighbouring pharmaceutical and fine chemical manufacturing facilities.
In contrast Site B is a storage facility which contains nine storage tanks each of 750
000 barrel capacity, Fig. S1(d).† The specific areas sampled, which are within the boundary of the tank farm, have no known pre-history or current use. The lack of metabolomic ‘signatures’ for surfactants (with one exception), polycyclic aromatic hydrocarbons, and other pollutants recognises the lack of contamination on this site. Indeed, the presence of fatty acids and phospholipids, as metabolites from natural occurring sources, confirm the relatively pristine nature of the site.
Remote sensing using an unmanned aerial vehicle with multispectral image camera
The effect of oil spill on vegetation indices at site A and site B.
The presence of heavy metals and polycyclic aromatic hydrocarbons from crude oil in soil have been reported to have biophysical and chemical impacts on vegetation.38 These biophysical and chemical impacts lead to a reduction in the chlorophyll pigment, which has an adverse effect on the vegetation and ultimately results in deterioration and death, depending upon the concentration of the pollutants. The use of green VI's, as a measure of vegetation quality, could enable differentiation between vegetation on oil contaminated soil and non-contaminated soil. Four green VI's (i.e. NDVI, GNDVI, SAVI, and CI green VI) were evaluated using remote sensing MSI data to assess whether the vegetation was affected by oil contamination (on Site A) versus vegetation from the unaffected site (Site B). The VI's from a selected area within Site A (Main Site) are shown in Fig. 7, while those from a selected area within Site B (Storage Depot), are shown in Fig. 8. The VI maps from Site A (Fig. 7), indicate moderately healthy vegetation values of NDVI (0.42–0.88), GNDVI (0.32–0.81), SAVI (0.62–1.32) and CI green VI (2.27–8.51). Whereas the VI maps from Site B (Fig. 8), indicate healthy vegetation values of NDVI (0.73–1), GNDVI (0.74–1), SAVI (1.2–1.5) and CI green VI (12.3–36.8). Vegetation indices (NDVI, GNDVI, SAVI and CI green VI) maps for the full sites are shown in Fig. S2† for Site A (Main Site) and Fig. S3† for Site B (Storage Depot).
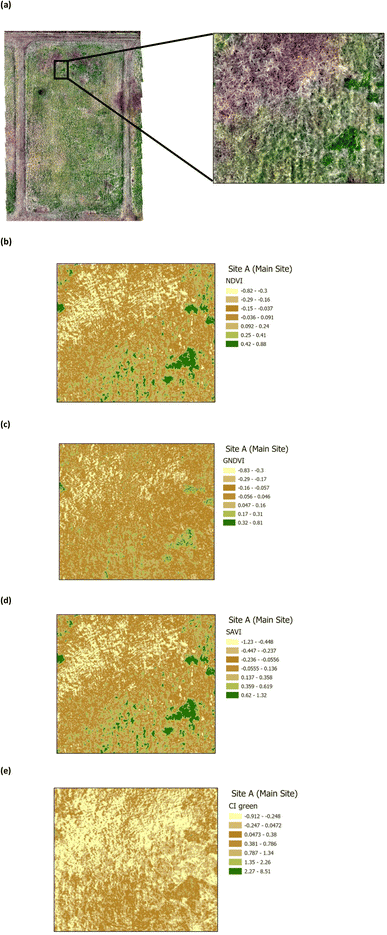 |
| Fig. 7 Vegetation index maps for an area of Site A (Main Site) (a) RGB image indicating the area chosen from Site A (b) NDVI (c) GNDVI (d) SAVI and (e) CI green. | |
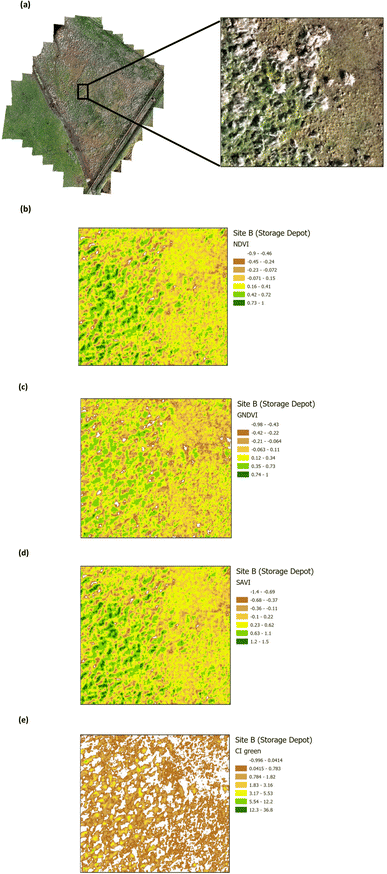 |
| Fig. 8 Vegetation index maps for an area of Site B (Storage Depot) (a) RGB image indicating the area chosen from Site B (b) NDVI (c) GNDVI (d) SAVI and (e) CI green VI. | |
A box and whisker plot generated using the extracted VI's (n = 108 data points) from Site A and Site B is shown in Fig. 9. The results indicate lower VI values for Site A across all investigated VI's, in comparison to Site B. In addition, a t-test was performed to determine if there is a statistical difference between the VI's from Site A and Site B. According to the t-test results (Table 2), the p-value across all VI's is found to be <0.05 and hence statistically significant. This concludes that there is a difference between the VI from Site A and Site B.
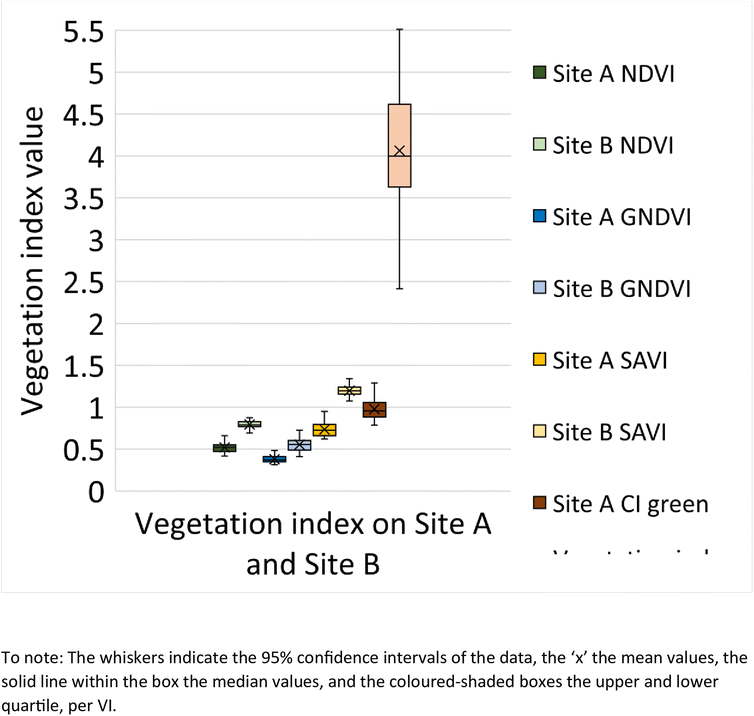 |
| Fig. 9 Box and whisker plot representing the vegetation index value for NDVI, GNDVI, SAVI and CI green on Site A (Main Site) and Site B (Storage Depot). | |
Table 2 Vegetation index statistical parameters for Site A (Main Site) and Site B (Storage Depot)a
Vegetation index |
Mean ± SD |
Min |
Max |
N
|
P-Value |
p-Value <0.05 statistically significant.
|
NDVI (Site A) |
0.52 ± 0.059 |
0.41 |
0.67 |
108 |
0.0000 |
NDVI (Site B) |
0.79 ± 0.039 |
0.69 |
0.88 |
108 |
GNDVI (Site A) |
0.38 ± 0.040 |
0.31 |
0.48 |
108 |
0.0000 |
GNDVI (Site B) |
0.55 ± 0.079 |
0.41 |
0.73 |
108 |
SAVI (Site A) |
0.73 ± 0.085 |
0.62 |
0.95 |
108 |
0.0000 |
SAVI (Site B) |
1.19 ± 0.057 |
1.08 |
1.34 |
108 |
CI green (Site A) |
0.98 ± 0.13 |
0.79 |
1.46 |
108 |
0.0000 |
CI green (Site B) |
4.06 ± 0.75 |
1.98 |
5.51 |
108 |
Links between soil contaminants and vegetation indices at site A and site B.
Raster profiles showing the geographical distribution of barium in the Main Site (Site A) and Storage Depot (Site B) are shown in Fig. 10(a) and (b), respectively. Fig. 10(a) displays the barium concentration on Site A, which ranges between 8493 μg g−1 and 38
430 μg g−1, while on Site B (Fig. 10(b)) the barium concentration ranges between 3242 μg g−1 and 5078 μg g−1. In addition, the relative abundances of the surfactant C12-AS range between 10
255
800 and 13
328
600 on Site A (Fig. 11(a)) and from 3
772
900 and 8
697
660 on Site B (Fig. 11(b)). Whereas for tetradecylsulfate values on Site A (Fig. 11(a)) range between 2
777
540 and 4
222
500 while on-Site B (Fig. 11(b)) the values range between 1
189
550 and 1
859
400. The results demonstrate that the concentration of barium, and the relative abundances of C12-AS and tetradecylsulfate are comparatively higher in Site A than in Site B, and it could be theorized that these elevated levels might lead to vegetative stress. Conversely, the lower levels in Site B, have no detrimental effect on the vegetation. It could therefore now be hypothesized, based on the barium data in Table 1 and the metabolites data in Fig. 6, from Site A, that this could be aligned with the lower VI values shown in Fig. 9, which have disrupted the vegetation (and vice versa for Site B). Table 3 summarises the correlation (R2) between the four VI's (NDVI, GNDVI, SAVI and Cl green VI) against barium concentration and the two metabolites, C12-AS and tetradecylsulfate (Fig. S4–S6†). In all cases a good (>0.60) to excellent (>0.79) correlation coefficient (R2) was noted irrespective of the VI selected. A similar effect has been reported,19 in terms of a VI linked to the presence of compounds in crude oil contaminated soil. While a barium study, reported that the high levels of barium in contaminated soil negatively impact plant growth.39,40
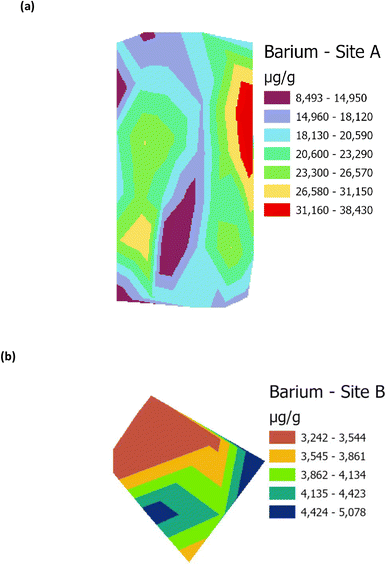 |
| Fig. 10 Raster profile for analysis of barium on (a) Main Site Teesside Refinery (Site A), and (b) Greatham Storage Depot (Site B). | |
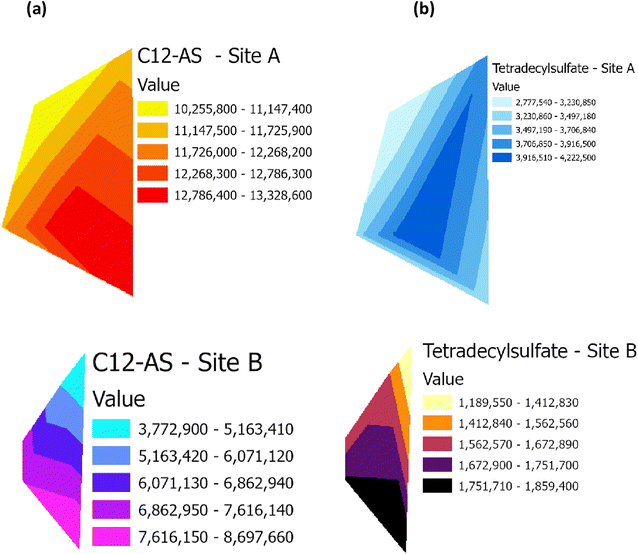 |
| Fig. 11 Raster profile for the surfactant metabolites, C12-AS and tetradecylsulfate, on (a) the Main Site Teesside Refinery (Site A) and (b) Greatham Storage Depot (Site B). | |
Table 3 Correlation between vegetation index and (a) barium concentration (mg kg−1), (b) C12-AS metabolite peak area, and (c) tetradecylsulfate metabolite peak area for Site A (Main Site) and Site B (Storage Depot)
(a) |
VI |
Barium concentration range (mg kg−1) |
Number of data points from Site A |
Number of data points from Site B |
Y = mx + c |
Correlation R2 |
NDVI |
3261–38 808 |
36 |
9 |
−0.00001x + 0.7558 |
0.6093 |
GNDVI |
−0.000007x + 0.5313 |
0.6090 |
SAVI |
−0.00002x + 1.133 |
0.6056 |
CI green VI |
−0.0001x + 3.499 |
0.5779 |
(b) |
VI |
C12-AS metabolite range (peak area) |
Number of data points from Site A |
Number of data points from Site B |
Y = mx + c |
Correlation R2 |
NDVI |
3 727 851–13 332 319 |
5 |
5 |
−0.00000003x + 0.9744 |
0.6151 |
GNDVI |
−0.00000003x + 0.7176 |
0.7347 |
SAVI |
−0.00000006x + 1.5312 |
0.6497 |
CI green VI |
−0.0000005x + 6.9469 |
0.7695 |
(c) |
VI |
Tetradecylsulfate metabolite range (peak area) |
Number of data points from Site A |
Number of data points from Site B |
Y = mx + c |
Correlation R2 |
NDVI |
1 177 073–4 224 172 |
5 |
5 |
−0.0000001x + 0.9548 |
0.7592 |
GNDVI |
−0.00000008x + 0.6699 |
0.6765 |
SAVI |
−0.0000002x + 1.4549 |
0.6835 |
CI green VI |
−0.000002x + 6.4859 |
0.8626 |
It is noted that the impact on the site is minimal despite its 50 years of use. The contaminants identified on Site A (Main Site) were identified due to the former legacy of it being using as a washing facility for containers of crude oil, hence the identification of the components of crude oil and washing materials i.e. surfactants. The presence of the metabolites from other compounds, notably pesticides, plasticizers, and pharmaceuticals, could have impacted this site from surrounding industries. The ‘pristine’ condition of Site B, the Storage Site, is testimony to the lack of any industrial activity taking place here, on what was originally reclaimed land from the sea.
Conclusion
The application of VI's, derived from data gathered by remote sensing, has been shown to correlate with ground truth chemical analyses. The deployment of a remote sensing UAV with MSI camera provides readily accessible data that is indicative of vegetative stress. The data can be interpreted using a variety of derived VI's. These VI's can be used to assess the environmental impact of soil-based contaminants. The use of remote sensing approaches can provide wide geographical coverage of potential contaminated sites in a rapid and cost-effective manner. This remote sensing approach can be effectively deployed to complement initial desk-top surveys, prior to full scale laboratory-based soil analyses. This approach has a considerable cost–benefit analysis, to guide decision-making, as part of any initial environmental risk assessment, and prior to any high-cost invasive soil chemical analyses.
Author contributions
Conceptualization: J. R. D., C. E. N., J. J. P. Data curation: J. R. D., S. A., W. C., I. S., M. R., S. L. B., C. E. N., J. J. P. Formal analysis: J. R. D., S. A., W. C., I. S., M. R., S. L. B. Funding acquisition: J. R. D., J. J. P. Investigation: J. R. D., S. A., C. E. N. Methodology: J. R. D., S. A., W. C., I. S., M. R., S. L. B. Project administration: J. R. D., C. E. N., J. J. P. Resources: J. R. D., C. E. N., J. J. P. Supervision: J. R. D., C. E. N., J. J. P. Writing – original draft: J. R. D., S. A., W. C., I. S. Writing – review & editing: J. R. D., S. A., W. C., C. E. N., J. J. P.
Conflicts of interest
The authors have no conflicts of interest to declare.
Acknowledgements
We acknowledge Mr Tony J. Finn, Environment Engineer, Conoco Phillips, Teesside Operations, Seal Sands, Middlesbrough for providing accompanied access to the sites.
References
- A. A. Sanford, B. A. Manuel, M. A. Romero-Reyes and J. M. Heemstra, Combating small molecule environmental contaminants: detection and sequestration using functional nucleic acids, Chem. Sci., 2022, 13, 7670–7684, 10.1039/D2SC00117A.
-
CL: AIRE, Category 4 Screening Levels: Vinyl Chloride, 2021, available online: https://www.claire.co.uk/c4sl Search PubMed.
-
EA, The Future for Environmental Regulation: Green Growth Not Red Tap: A Speech Delivered at the Westminster Energy and Environment Forum, GOV.UK, 2021, available online, The future for environmental regulation: Green growth not red tape, https://www.gov.uk/ Search PubMed.
-
I. Melendez-Pastor, J. Navarro-Pedreno, I. Gomez and M. B. Almendro Candel, The use of remote sensing to locate heavy metal as a source of pollution, in Advances in Environ. Res., 2011, vol. 7, pp. 225–233, ISBN: 978-1-61728-774-9 Search PubMed.
- J. Briffa, E. Sinagra and R. Blundell, Heavy metal pollution in the environment and their toxicological effects on humans, Heliyon, 2020, 6(9), e04691, DOI:10.1016/j.heliyon.2020.e04691.
- Z. Asif, Z. Chen, C. An and J. Dong, Environmental impacts and challenges associated with oil spills on shorelines, J. Mar. Sci. Eng., 2022, 10(6), 762, DOI:10.3390/jmse10060762.
- S. Adipah, Introduction to petroleum hydrocarbons contaminants and its human effects, Int. J. Environ. Res. Publ. Health, 2019, 3(1), 001–009, DOI:10.26502/jesph.96120043.
- O. A. Aigberua, An assessment of trace metal pollution indicators in soils around oil well clusters, Pet. Res., 2022, 7, 275–285, DOI:10.1016/j.ptlrs.2021.09.001.
- M. S. Abu-Khasan and Y. I. Makarov, Analysis of soil contamination with oil and petroleum products, IOP Conf. Ser.: Earth Environ. Sci., 2021, 937, 022046, DOI:10.1088/1755-1315/937/2/022046.
- M. D. Yuniati, Bioremediation of petroleum-contaminated soil: A review, IOP Conf. Series, Earth Environ. Sci., 2018, 118, 012063, DOI:10.1088/1755-1315/118/1/012063.
- G. Schwartz, E. Ben-Dor and G. Eshel, Quantitative assessment of hydrocarbon contamination in soil using reflectance spectroscopy: A “multipath” approach, Appl. Spectrosc., 2013, 67(11), 1323–1331, DOI:10.1366/13-07053.
- C. Kumunda, A. S. Adekunle, B. B. Mamba, N. W. Hlongwa and T. T. I. Nkambule, Electrochemical detection of environmental pollutants based on graphene derivatives: a review, Front. Mater., 2021, 7, 606787, DOI:10.3389/fmats.2020.616787.
- S. Sattar, R. Hussain, S. M. Shah, S. Bibi, S. R. Ahmad, A. Shahzad, A. Zamir, Z. Rauf, A. Noshad and L. Ahmad, Composition, impacts, and removal of liquid petroleum waste through bioremediation as an alternate clean-up technology: a review, Heliyon, 2022, 8(10), e11101, DOI:10.1016/j.heliyon.2022.e11101.
-
J. R. Dean, Extraction Techniques for Environmental Analysis, John Wiley & Sons, 2022, pp. 1–384 Search PubMed.
- A. Gholizadeh and V. Kopačková, Detecting vegetation stress as a soil contamination proxy: a review of optimal proximal and remote sensing techniques, Int. J. Environ. Sci. Technol., 2019, 16, 2511–2524, DOI:10.1007/s13762-019-02310-w.
- T. Shi, H. Liu, Y. Chen, J. Wang and G. Wu, Estimation of arsenic in agricultural soils using hyperspectral vegetation indices of rice, J. Hazard. Mater., 2016, 308, 243–252, DOI:10.1016/j.jhazmat.2016.01.022.
- S. Asadzadeh, W. J. de Oliveira and C. R. Souza Filho, UAV-based remote sensing for the petroleum industry and environmental monitoring: State-of-the-art and perspectives, J. Pet. Sci. Eng., 2022, 208, 109633, DOI:10.1016/j.petrol.2021.109633.
- L. Li, S. L. Ustin and M. Lay, Application of AVIRIS data in detection of oil-induced vegetation stress and cover change at Jornada, New Mexico, Remote Sens. Environ., 2005, 94(1), 1–16, DOI:10.1016/j.rse.2004.08.010.
- B. Adamu, K. Tansey and B. Ogutu, Remote sensing for detection and monitoring of vegetation affected by oil spills, Int. J. Rem. Sens., 2018, 39(11), 3628–3645, DOI:10.1080/01431161.2018.1448483.
- M. S. Ozigis, J. D. Kaduk, C. H. Jarvis, P. da Conceição Bispo and H. Balzter, Environ. Pollut., 2020, 256, 113360, DOI:10.1016/j.envpol.2019.113360.
- J. L. Hatfield, J. H. Prueger, T. J. Sauer, C. Dold, P. O'Brien and K. Wacha, Applications of vegetative indices from remote sensing to agriculture: past and future, Inventions, 2019, 4(4), 71, DOI:10.3390/inventions4040071.
- J. Xue and B. Su, Significant remote sensing vegetation indices: a review of developments and applications, J. Sens., 2017, 1353691, DOI:10.1155/2017/1353691.
- G. Lassalle, S. Fabre, A. Credoz, D. Dubucq and A. Elger, Monitoring oil contamination in vegetated areas with optical remote sensing: a comprehensive review, J. Hazard. Mater., 2020, 393, 122427, DOI:10.1016/j.jhazmat.2020.122427.
- J. R. Dean, P. M. Amaibi, A. Okorie and J. A. Entwistle, A critical evaluation of the use and ‘misuse’ of As and Pb bioaccessibility data in human health risk assessments, Environ. Res., 2020, 189, 109915, DOI:10.1016/j.envres.2020.109915.
- H. Gould, W. Cheung, J. D. Finnigan, J. Muñoz-Muñoz, S. J. Charnock and G. W. Black, In search of complementary extraction methods for comprehensive coverage of the Escherichia coli metabolome, Metabolites, 2023, 13, 1010, DOI:10.3390/metabo13091010.
- W. B. Dunn, W. Lin, D. Broadhurst, P. Begley, M. Brown and E. Zelena,
et al., Molecular phenotyping of a UK population: defining the human serum metabolome, Metabolomics, 2015, 11, 9–26, DOI:10.1007/s11306-014-0707-1.
- O. Fiehn, D. Robertson, J. Griffin, M. van der Werf, B. Nikolau and N. Morrison,
et al., The metabolomics standard initiative (MSI), Metabolomics, 2007, 3, 175–178, DOI:10.1007/s11306-007-0070-6.
-
MetaboAnalyst 5.0, 2023, available at, https://www.metaboanalyst.ca/ Search PubMed.
- J. W. Rouse, R. H. Haas, J. A. Schell and D. W. Deering, Monitoring vegetative systems in the great plains with ERTS, NASA [Spec. Publ.] SP, 1974, 351(1), 309–317 Search PubMed.
- A. A. Gitelson, J. Y. Kaufman and M. N. Merzlyak, Use of a green channel in remote sensing of global vegetation from EOS-MODIS, Remote Sens. Environ., 1996, 58(3), 289–298, DOI:10.1016/S0034-4257(96)00072-7.
- A. R. Huete, A soil-adjusted vegetation index (SAVI), Remote Sens. Environ., 1988, 25(3), 295–309, DOI:10.1016/0034-4257(88)90106-X.
- L. Zhang, Z. Zhang, Y. Luo, J. Cao, R. Xie and S. Li, Integrating satellite-derived climatic and vegetation indices to predict smallholder maize yield using deep learning, Agric. For. Meteorol., 2021, 311, 108666, DOI:10.1016/j.agrformet.2021.108666.
- A. A. Gitelson, A. Viña, T. J. Arkebauer, D. C. Rundquist, G. Keydan and B. Leavitt, Remote estimation of leaf area index and green leaf biomass in maize canopies, Geophys. Res. Lett., 2003, 30(5), 1248, DOI:10.1029/2002GL016450.
-
UK Soil Observatory (UKSO), British Geological Survey, https://mapapps2.bgs.ac.uk/ukso/home.html?layers=%26extent=-144633,7284935,-126833,7294193%26basemap=topo, accessed 26/04/2023 Search PubMed.
-
C. A. Johnson, N. M. Piatak and M. M. Miller, Critical mineral resources of the United States – Economic and environmental geology and prospects for future supply, Barite (Barium), ed. D of K.J. Schulz, J.H. DeYoung Jr, R.R. Seal II, and D.C. Bradley, U.S. Geological Survey, Professional Paper 1802, 2017, pp. D1–D18, DOI:10.3133/pp1802D.
- M. D. Ahmaruzzaman, MXenes based advanced next generation materials for sequestration of metals and radionuclides from aqueous stream, J. Environ. Chem. Eng., 2022, 10(5), 108374, DOI:10.1016/j.jece.2022.108371.
-
Stockholm Convention on Persistent Organic Pollutants, 2001, available at, http://chm.pops.int/Portals/0/Repository/convention_text/UNEP-POPS-COP-CONVTEXT-FULL.English.PDF Search PubMed.
- C. B. Obida, G. A. Blackburn, J. D. Whyatt and K. T. Semple, Counting the cost of the Niger Delta's largest oil spills: Satellite remote sensing reveals extensive environmental damage with >1 million people in the impact zone, Sci. Total Environ., 2021, 775, 145854, DOI:10.1016/j.scitotenv.2021.145854.
- N. Sleimi, R. Kouki, M. Hadj Ammar, R. Ferreira and R. Perez-Clemente, barium effect on germination, plant growth, and antioxidant enzymes in Cucumis sativus L. plants, Food Sci. Nutr., 2021, 9(4), 2086–2094, DOI:10.1002/fsn3.2177.
- A. A. de Souza Cardoso, A. P. P. Nunes, E. R. Batista, L. Nataren, M. Nunes, F. T. L. Gomes and F. M. L. S. Silva, Sulfate supply decreases barium availability, uptake, and toxicity in lettuce plants grown in a tropical Ba-contaminated soil, Environ. Sci. Pollut. Res. Int., 2023, 30(18), 53938–53947, DOI:10.1007/s11356-023-25960-3.
|
This journal is © The Royal Society of Chemistry 2024 |
Click here to see how this site uses Cookies. View our privacy policy here.