DOI:
10.1039/D2LC00235C
(Paper)
Lab Chip, 2022,
22, 2343-2351
Laser particle activated cell sorting in microfluidics†
Received
13th March 2022
, Accepted 22nd April 2022
First published on 27th May 2022
Abstract
Laser particles providing bright, spectrally narrowband emission renders them suitable for use as cellular barcodes. Here, we demonstrate a microfluidic platform integrated with a high-speed spectrometer, capable of reading the emission from laser particles in fluidic channels and routing cells based on their optical barcodes. The sub-nanometer spectral emission of each laser particle enables us to distinguish individual cells labeled with hundreds of different laser colors in the near infrared. Furthermore, cells tagged with laser particles are sorted based on their spectral barcodes at a kilohertz rate by using a real-time field programmable gate array and 2-way electric field switch. We demonstrate several different flavors of sorting, including isolation of barcoded cells, and cells tagged with a specific laser color. We term this novel sorting technique laser particle activated cell sorting (LACS). This flow reading and sorting technology adds to the arsenal of single-cell analysis tools using laser particles.
Introduction
The biological behavior of a tissue, organ or organism arises from the complex interactions of numerous distinguishable cell subpopulations. To discern each subpopulation's different role, it is desirable to tag each group in a manner that renders each distinguishable. In the most ideal and extreme case, each tagged subpopulation comprises of just a single cell thereby allowing the relevant biological dynamics to be analyzed at a single cell level. Tags in the form of optical barcodes offer several advantages allowing real-time, nondestructive, and repeatable readout using a relatively simple optical reader. Recently a new form of optical barcode termed laser particles (LPs) has been developed that enables a high degree of multiplexing due to the large number of unique emission signatures LPs can form.1–3 Upon optical excitation, each LP emits coherent radiation of sub-nanometer spectral linewidth providing an ultra-pure, distinguishable laser color. With a wavelength interval of 1 nm, about 400 colors from 1200 to 1600 nm have been achieved,1,2 which could in principle be scaled to millions of barcodes by using combinations of LPs to tag each cell. The scalability of LPs offers distinct advantages over conventional fluorescence-based tagging techniques, such as multi-color fluorophores,4 nanoparticles,5 and microbeads,6 which typically permit only a small number of cells or cell-groups (<10 to 100) to be labelled. Laser-based optical barcoding promises to make it possible to tag single cells at the scales (>10
000) commonly used in single-cell analysis through imaging, flow cytometry, and sequencing. Data acquired at different times and in different instruments can be aligned to individual cells based on their unique optical barcodes allowing for multi-dimensional analysis of the cell population with single cell resolution.7 In our previous work, we demonstrated tracking of LP tagged cells both in 2D and 3D cell cultures and in vivo1 by integrating a high-resolution optical spectrometer capable of reading LP barcodes into a conventional fluorescence microscope.
In this paper, we extend the ability to read LP barcodes to LPs flowing in microfluidic channels and demonstrate a flow sorter capable of routing specific LPs and LP-tagged cells. Fluidics represents a versatile platform for analyzing and processing single cells at high throughput. Flow cytometers and fluorescence-activated cell sorters (FACS) are widely used instruments for various biological and biomedical applications.4,8,9 FACS is commonly used to isolate cells in a high throughput manner for downstream utilities for adoptive cell transfer, cell therapy development, drug screening, and single-cell sequencing. Identification of the specific cells to sort and decision making are made by measuring fluorescence signals from fluorochrome-tagged antibodies, dye-based sensor probes, and protein reporters, as well as fluorescence-tagged barcodes.10,11 For this, FACS typically employs several to a few dozens of photodetector channels.
Our microfluidic instrument employs a 2048-pixel line-scan spectrometer (30 μs exposure, 25–30 kHz readout) with nm-scale resolution and a field programmable gate array (FPGA) to enable real-time identification of the optical barcode of each flowing cell and permit sorting based on the barcode with specific gating strategies. Deflection of cells is achieved via a high-voltage dielectrophoretic force.12,13 The resultant force is applied to aqueous droplets encapsulating LP-tagged cells, allowing for the enrichment of certain LP tags at sorting rates of greater than 1000 events per second. For example, using LACS, we separate cells containing LP tags from those which do not. We also demonstrate isolation of cells containing LPs that emit within a 5 nm window, an order of magnitude narrower than the material's corresponding fluorescence, thereby creating a subpopulation of cells tagged using a color of exceptional spectral purity. We envision that this technology will be useful to purify LP-tagged cells prior to optical microscopy experiments or isolate cells after imaging for further downstream analysis such as single-cell sequencing. Since the pumping and emission wavelengths of LPs are all in the near infrared above 1000 nm, this technology is compatible with fluorescence-based flow cytometry which typically uses dyes emitting in the visible range. We will discuss how this technology may enable novel single-cell analysis workflows.
Results and discussion
High-speed reading of LP spectra under flow
We used disk-shaped InGaAsP LPs with a diameter of ∼2 μm and thickness of 0.2 μm.1 The diameter of each particle determines the emission wavelength of its lasing peak with a tuning coefficient of ∼1 nm nm−1 in wavelength to disk radius changes. This wavelength is remarkably stable over a range of typical excitation pump intensities (Fig. S1†). LPs of different diameter can each emit their own narrowband radiation within a 75–100 nm full width at half maximum (FWHM) spectral gain bandwidth determined by the semiconductor alloy composition. We used 9 different compositions of In1−xGaxAsyP1−y ranging from (x = 0.26, y = 0.55) to (x = 0.44, y = 0.95) to produce LPs over 1150 nm to 1650 nm.14
To demonstrate the concept of an LP-based flow reader, we first built a straight 1 cm-long channel (width x depth = 50 μm × 25 μm) microfluidic chip constructed using standard polydimethylsiloxane (PDMS)-on-glass technology. The microfluidic chip was positioned atop a microscope stage, with a 1064 nm pulsed pump laser (40 mW, 10 ns pulse width, 2 MHz repetition rate) focused within the channel center. By introducing a cylindrical lens before the objective lens, the pump laser focus was shaped into a line (10 μm × 50 μm, FWHM), covering the entire 50 μm channel width. These parameters were chosen to ensure reliable LP detection across the entire wavelength bandwidth based on our previous threshold and lasing measurements.14 The optical emission from the pumping zone was directed into a home-built grating-based spectrometer (resolution 0.5–1.0 nm) equipped with a 2048-pixel InGaAs linear camera typically operated at a speed of approximately 25
000 lines per s. The flow rate was set to approximately 40 μL min−1, corresponding to a mean flow speed of ∼0.5 m s−1 within the flow channel. Given the parabolic profile of laminar flow, cells travelled at slightly different speeds depending on their location in the channel. Each cell traversed the pump's excitation spot in 20 to 40 μs. We set the spectrometer camera exposure time to 35 μs that allow the lasing event to be captured within 1–2 frames.
Co-culture of LPs with various cell types results in their spontaneous uptake in a manner consistent with Poisson statistics.1,15 Efficient LP uptake has been demonstrated in numerous cell types, including primary cells.1,2 We tagged HeLa cells by overnight incubation with LPs with a low LP to cell ratio so that cells are tagged with an average one LP per cell. 500
000 cells suspended in 1 mL cell media were injected into the input end of the microfluidic chip. Fig. 1a shows high-speed video images (14 μs exposure, 10 k frames per second) of an LP-tagged cell that is traversing the pump excitation. As it flows through this readout region, a distinct signal is clearly observed on a few pixels of the spectrometer's linescan camera corresponding to a wavelength, in this case, of 1387 nm (Fig. 1b). The recorded linewidth δλ was 0.72 nm FWHM, limited by the spectrometer resolution. Importantly, this narrow linewidth means that the detection system can easily identify and resolve large numbers of laser colors. Fig. 1c shows 256 representative recorded spectra from 256 LPs across a bandwidth Δλ of ∼400 nm from 1200 nm to 1600 nm, the detection range of our spectrometer. Fig. 1d shows the histogram of over 460
000 LPs across the bandwidth. The distribution itself reflects the diameters of the recorded LPs. The relatively fewer lasing events at wavelengths greater than 1450 nm is likely due to a relatively lower number of lasing LPs in the spectral range.
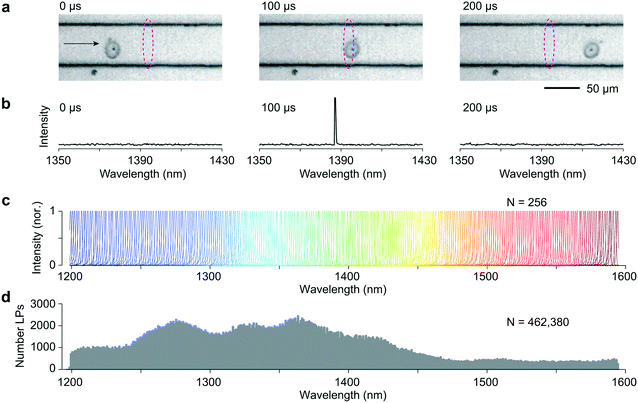 |
| Fig. 1 Readout of LP emission in the flow. a, Image frames showing a cell containing a single LP (arrow), as it traverses the pump laser focus (dashed ellipse). b, Recorded spectra at the corresponding time. A narrow lasing peak is observed on the spectrometer at the frame corresponding to the cell traversing this point. c, Collection of lasing spectra observed during a single experimental run. d, Histogram showing recordings of lasing wavelengths from a near half million LPs measured in a single flow experiment. | |
Unlike organic fluorophores, semiconductor LPs of different emission wavelengths can be excited with a single laser pump source because of the characteristic band-induced absorption of semiconductor gain media. We estimated that each LP emitted ∼107 photons per pump pulse, >1% of which is detectable by the spectrometer. These bright signals from LPs above lasing threshold are critical to the high-speed spectral readout. The identity, or color, of an LP is represented by the peak wavelength, independent of its recorded intensity (Fig. S2†). This spectral information is in general far more robust than intensity-based measurement and particularly critical for the LPs that typically have direction-dependent emission intensity.16 In theory, the flow LP reader presented here can resolve up to 550 colors (Δλ/δλ). We note that many more cells can be uniquely tagged if they are tagged with more than a single LP. For example, with 3 or more LPs per cell, the number of unique identifiers could be as many as 550C3 = 25 million. This set would allow 1 million cells to be uniquely tagged with a low duplicate error of ∼4%.
Laser particle activated sorting
Traditional FACS uses a gating strategy based on fluorescence biomarker intensities to sort cells in order to enrich for certain, desired subpopulations. To realize single-cell sorting based on their LP barcoding signals, we adopted a previously established water-in-oil droplet based sorting technique17 and fabricated a 2-way microdroplet sorting device (Fig. 2a and S3†). This device is comprised of three inlets and two outlets. In one of the inlets, cells suspended in cell media were flowed. A fluorinated oil, immiscible with the aqueous cell media was flowed into the other two inlets. The first of these inlets pinched the aqueous flow, generating 50 μm diameter aqueous droplets. The second inlet was used to space the droplets at intervals of approximately 500 μm to ensure that each droplet was independently sorted. The central sorting zone had a channel size (width x depth) of ∼55 μm × 25 μm and a mean flow speed of ∼0.5 m s−1. Droplets entered the flow channel at a rate of approximately 1.5 kHz.
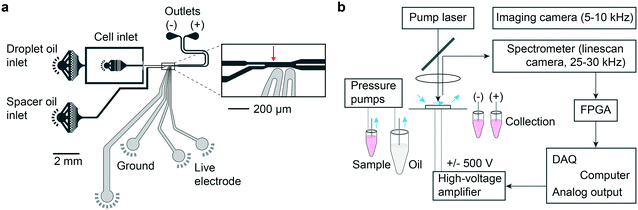 |
| Fig. 2 The microfluidic system. a, Schematic of a microfluidic chip. Pump laser focus location shown in inset (red arrow). b, Schematic of the cell sorting setup. A spectrometer reads LP laser emission. Decision hardware in a field programmable gate array (FPGA) triggers the computer to send high voltage pulses to electrodes to deflect cell-containing droplets into the (+) outlet. | |
Fig. 2b shows a schematic of the experimental setup. The pump laser is focused just upstream of the sorting junction, enabling the possible presence of an LP emission signal to be registered. Data from the spectrometer is streamed in real time to an FPGA that processes the spectra for sorting. The FPGA first determines whether the intensity value of any pixel exceeds the threshold defined by the camera noise floor and applies pre-programmed gating criteria to decide between ‘sort (+)’ and ‘no-sort (−)’. In the event that the criteria are met, the FPGA immediately sends a TTL pulse to a data acquisition (DAQ) card triggering it to output a 30 kHz square wave pulse train for a 400 μs duration. Once again, the flow rates are set such that the traversal time of the LP through the detection zone was approximately comparable to the 30 μs exposure time of the camera such that individual LP spectra are read in only one or two frames. The square wave pulse train is fed to a high voltage amplifier, which sends a 1 kV pk–pk voltage to a pair of microelectrodes offset by 15 μm from the proximal sidewall of the flow channel. The electric field applies a bound charge to the aqueous droplet since it has a higher permittivity than the surrounding oil. The droplet is then attracted by the electrode via Coulomb interaction, deflecting it into the desired outflow channel connected to the (+) outlet. This mechanism is known as dielectrophoresis12,13 (ESI† Note 1). The no-sort flow channel is ∼5% shorter in length, offering lower resistance pressure than the sorting channel. As a result, when the electrodes remain off, cells passively flow in the lower-pressure path and are collected in the (−) outlet. The specific sorting junction geometry we use includes a gapped divider that occupies part of the channel height and acts to further push the droplets toward either the (+) or (−) outlet.17
We tested the simplest scenario of binary LP sorting in which LP-containing cells are separated from un-barcoded cells that do not contain LPs. HeLa cells were mixed with LPs and cultured overnight. The next day, approximately 35
000 cells (containing about 25
000 LPs) were taken out of the cell culture and suspended in cell media. The cell sample was fed to the setup for sorting with a gating criterion that sends a high-voltage pulse when any single lasing peak is detected above the noise floor (Fig. 3, and see Videos S1 and S2†).
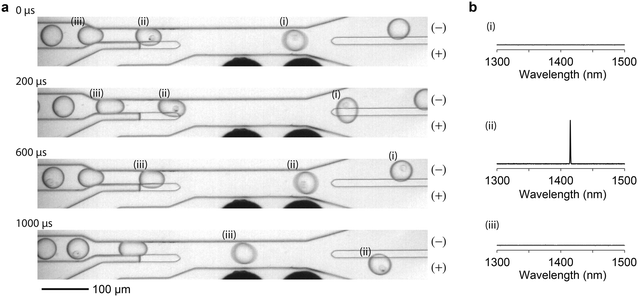 |
| Fig. 3 Binary cell sorting to divide cells in populations with and without LPs. The cells collected from the (+) outlet were actively sorted. The cells collected from the (−) outlet were allowed to flow through the sorting junction without the application of a dielectrophoretic force. a, Image frames of multiple droplets flowing through the sorting junction. Three successive droplets (i)–(iii) are labelled of which (ii) contains an LP-tagged cell and is thus deflected into the (+) outlet. b, Recorded spectra of the three droplets as they traverse the detection zone. | |
The collected cell samples in the (−) and (+) outlets were replated separately and imaged 4 h after sorting (Fig. 4a and b). For comparison, prior to taking the cell sample from the original culture, the relative distribution of LPs within cells roughly followed a Poisson distribution (Fig. 4c). Binary sorting produced a stark difference in the relative distributions of LPs within cells (Fig. 4d and e). In the (+) outlet sample, 94.1% of cells contained 1 or more LPs. By contrast, the (−) outlet sample was almost disk free, with 99.3% of cells containing no disks. Erroneous events were documented and classified using a high-speed video camera (Fig. S5a, Videos S3–S5†). If we define the accuracy in terms of the sensitivity and specificity of our sorting apparatus, we identify that 99.8% of cells containing at least 1 LP were correctly sorted (see ESI† Note 2). 85.2% of cells without an LP correctly arrive at the (−) outlet, and the rest 14.8% of the non-barcoded cells ended up in the (+) channel. From failure mode analysis based on high-speed imaging, we found that the high false positive ratio was not due to barcode identification error. One failure mode was two droplets packing abnormally close together, causing deflection into the incorrect outlet (Fig. S5b, Video S4†). The occasional abnormal droplet spacing is due to flow pump instabilities caused by cell aggregates in the sample. Another failure mode was a droplet occupied by two cells. When only one cell contains an LP, this leads to the other non-tagged cells in the (+) outlet (Fig. S5c, Video S5†).
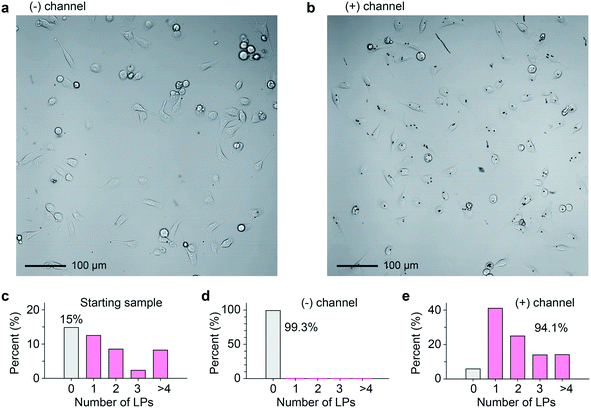 |
| Fig. 4 a and b, Representative bright-field images of HeLa cells after sorting as collected from the (−) and (+) outlets. c–e, Statistics of the fraction of cells that contain a specified number of disks, analyzed from >500 cells in all acquired images. | |
In the future, binary sorting based on the presence or absence of an LP could be helpful to isolate an LP-tagged subpopulation of cells for downstream experiments such as cell-tracking imaging.1,2 Alternatively, LP tags have been proposed as single cell sequencing probes, in which the emission signatures allow individual cells to be tracked through an RNA sequencing workflow to enable knowledge of each cell's spatial location to be tied to information regarding its genetic expression.7 In some single cell sequencing protocols, cells are commonly sorted prior to sequencing by traditional FACS to target a desired subpopulation to be sequenced.18,19 Similarly, binary LACS would be able to enrich for cells suitable for sequencing by selecting tagged cells.
Spectrally selective LP activated cell sorting
We then explored sorting based on spectral barcodes. First, we tested a gating strategy for short-pass or long-pass wavelength sorting. The spectrometer-linked FPGA was programmed to identify, in real time, the pixel of highest intensity each time a lasing event was registered. If this pixel corresponded to a wavelength within the sorting window, a high-voltage electric field was applied to the electrodes to deflect the traversing droplet toward the appropriate outflow channel. In cases in which more than one lasing peak emanated from a droplet, possibly because of the presence of two cells or a single cell with two LPs in the droplet, the gating condition was set such that the sorting signal is fired only if all the emission peaks fell within the sorting window. In the experiment, we used a short-wavelength gating condition with a cutoff wavelength of 1450 nm. Exemplary events are shown in Fig. 5 and Videos S6–S8.†
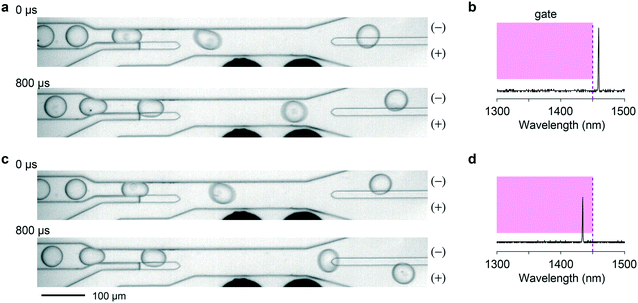 |
| Fig. 5 Short-wavelength sorting. a, A negative cell (λ > 1450 nm) flowing into the (−) channel. b, The LP emission spectrum of the negative cell. The magenta box indicates the gating condition used. c, A positive cell (λ < 1450 nm) directed into the (+) channel. d, The LP spectrum of the positive cell. | |
As a more sophisticated demonstration, we implemented band-pass sorting. A 5 nm-wide sorting window centered around 1285 nm was applied as the selection criterion. We used LPs obtained from a single wafer with In0.75Ga0.25As0.54P0.46 as active material, which covers a spectral range from 1245 to 1340 nm. The LPs were co-cultured with HeLa cells and flowed through the sorting chip. Cells collected from each outlet were re-plated on a culture well plate, and the LP emission from the cells were measured using the previously modified confocal microscope employing a high-resolution spectrometer.1,2Fig. 6 shows representative images and LP emission spectra obtained from both channels. The cells harvested from the (+) outlet contained LPs that predominantly lased at wavelengths within the predefined 5 nm window close to 1285 nm (Fig. S7†). The routing accuracy was consistent with that obtained in the above wavelength-independent binary sorting (ESI† Note 3).
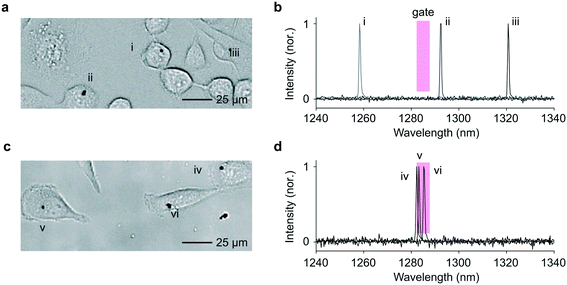 |
| Fig. 6 Band-pass sorting. a, A bright-field image of replated cells collected from the (−) outlet. b, The LP lasing emissions from the LPs labelled i, ii and ii. c and d, Image and emission spectra of cells collected from the (+) outlet, showing three LPs (iv–vi). | |
Wavelength-based LP sorting allows specific cells identified from an imaging experiment to be isolated for further analysis. Since our LPs emit at infrared wavelengths, they do not exhibit crosstalk with common fluorophores, making LPs compatible with established fluorescence-based technologies. For example, LACS could be used to isolate cells based on their LP barcodes that have been previously identified by techniques such as microscopy or flow cytometry, that can associate individual cells with phenotypic features, such as protein-tagged fluorescence expression. By reading LP signatures at each step of a workflow, comprehensive cell profiles could ultimately be built and isolated as desired.
Conclusions
Cell sorters are indispensable instruments used in biological research and medical research for isolating specific cellular subpopulations with specific phenotypes. FACS are the amongst the most common types, which divert and collect cells into different vials based on the fluorescence signals related to immuno-stained biomarkers, viability, and reporter-gene expressions.8 Light scattering-based sorting is also used for label-free purification of cell subpopulations such as lymphocytes and pancreatic islet cells.20,21 Magnetic-activated cell sorters (MACS) are also increasingly used to purify engineered cells for cell therapy.22 Fluorescence image-based cell sorting has recently been developed to enable selective isolation of single cells with unique spatial and morphological traits.23 In all these techniques, the sorting signals or routing decision are related to the cellular phenotypes measured in situ. Here we have demonstrated a new type of cell sorter based on laser-emitting cellular barcodes, which we term LP activated cell sorting (LACS).
Besides cell sorting, the technology may be used to sort LPs, either with or without cells, inside droplets. Currently, LPs are fabricated such that each particle has a random wavelength, distributed over the gain bandwidth of the semiconductor. However, for some experiments it would be useful to be able to produce a batch of LPs with an identical wavelength, just like fluorophores with identical fluorescence spectrum. By repeated wavelength-selective sorting, batches of LPs at different colors could be created for various applications such as highly multiplexed cell-type labeling for in vivo imaging or drug screening, which is currently performed using fluorescent cell barcoding.24,25
Future improvements in sorting accuracy should also be emphasized and can likely be realized most easily by using alternative sorting mechanisms26–28 that can deflect droplets in the air and into multiple channels, such as those used in commercial machines (2- to 6-way sorting). Increased sorting device sophistication could enable sorting rates as high as tens of thousands of cells per second.
In conclusion, the demonstrated flow technology enabling cell barcode identification and sorting based on laser emission spectra will prove useful in the emerging single-cell applications of LPs.
Materials and methods
Fabrication and transfer of LPs
Epitaxially grown wafers were used to produce LPs. The wafers were based on an InP substrate, with a 300 nm thick undoped InP buffer layer. On top of this layer, one or more 200 nm thick InGaAsP layers were grown with 300 nm separation between them. 2 μm thick SU8-2002 (MicroChem) photoresist was spun on the wafer surface followed by soft bakes for 1 min at 65 °C and 2 min at 95 °C. The wafer was exposed through a chrome on quartz mask comprised of a hexagonal array of circles, using an i- and h-line mercury arc lamp and with a dose of 60 mJ cm−2 (Karl Suss MJB4 mask aligner). After this, further 1 min bakes at 65 °C and 95 °C were performed followed by development in SU8 developer (MicroChem). A 10 min hard bake on a 190 °C hotplate was then performed after which the wafer was descummed using an O2 plasma (Anatech Barrel SCE 160). The remaining circular photoresist features were then used as a mask to dry etch columns into the wafer with ICP-RIE using chlorine chemistry (Oxford Instruments PlasmaPro 100 Cobra 300). The remaining SU8 was then removed using a cleaning sequence comprised of a 3 min plasma clean using CF4 and O2 (Oxford Instruments PlasmaPro 100 Cobra 300), a 30 s dip in 1
:
1 H2SO4
:
H2O and a final O2 plasma clean (Matrix 105). Microdisk LPs were then released from the substrate by dissolving the InP supports using 3
:
1 HCl
:
H2O for 30 s. The LPs were then washed at least 3 times in deionized water through repeated rounds of centrifugation and removal of the supernatant. LPs suspended in water were then added to cells followed by the appropriate amount of 10× PBS to maintain an isosmotic solution.
Microfluidic device fabrication
Poly(dimethylsiloxane) (PDMS) microfluidic devices were created using an SU8-on-silicon master mold. To create this mold, a two-layer device mold was created. The first layer consisted of the sorting divider and channels separating the sorting outflow tracts that allowed for pressure equalization. To create this layer, SU8-3010 (Kayaku Advanced Materials) was spun at 3000 rpm onto a Si wafer (UniversityWafer) and then soft baked for 5 min at 95 °C. The first layer of the pattern was then exposed using a 2500 mJ cm−2 365 nm wavelength laser writer (Heidelberg Instruments MLA150). A 95 °C post exposure bake was then performed for 5 min followed by a 5 min development in propylene glycol methyl ether acetate. The second layer defined the microfluidic flow channels and the electrode channels. To form this layer, the process was repeated but with SU8-3025. The laser writer was used to write the pattern in a manner that aligned it to the first layer. The master mold was then placed inside a vacuum chamber for 30 min with a drop of 1H,1H,2H,2H-perfluorooctyltrichlorosilane (Oakwood Chemical) and then baked for 1 min at 180 °C. This prevented PDMS from sticking to the mold too firmly. Next, a 10
:
1 base elastomer
:
curing agent PDMS mixture (Sylgard 184 Silicone Elastomer Kit) was poured onto the mold and allowed to cure at 65 °C for 2 h. After this, the molded PDMS was peeled off the wafer. A 1.2 mm diameter biopsy punch (Harris Uni-Core) was then used to create holes in the PDMS for each of the 3 inlets, 2 outlets, and 4 electrode openings. The PDMS was then bonded to a glass slide substrate using O2 plasma bonding (Plasma Etch) and baked at 70 °C for 2 h to further strengthen the bonding. At each flow channel inlet and outlet, 1/16′′ × 0.006′′ OD × ID PTFE tubing (Valco Instruments) was connected. For the tubing linked to the inlets, pressure pumps were connected to the other end (Fluigent Flow EZ), along with an in-line flow meter (Fluigent Flow Unit). Aquapel (Pittsburgh Glass Works) was flowed into the device to render the glass surface hydrophobic. After sitting for 30 s, this was rinsed by flushing the devices with FC-40 and dried using an N2 air gun. To create electrodes, the microfluidic device was placed on a hot plate at 80 °C, and low melting point In51Bi32.5Sn16.5 solder (Indium Corporation Indalloy) was fed into one of the openings into the live electrode channel and one of the openings into the ground electrode channel. Whilst still on the hotplate, gold connects (Digi-Key 87224-1) were placed into each of the two openings. UV-curable adhesive (Loctite 352) was used to strengthen the connection between the PDMS and the gold connect.
Cell culture
HeLa cells (ATCC) were cultured in a solution comprised of Dulbecco's modified Eagle medium, 10% (v/v) fetal bovine serum (FBS) and 1% (v/v) penicillin–streptomycin. For LP imaging experiments, LPs were added directly to the cell culture along with the requisite quantity of 10× PBS to ensure isotonicity. After 24 h, cells now with internalized LPs were removed from the plate by first washing the culture three times in PBS, incubating (Thermo Scientific Heracell 240i) for 5 min in TrypLE Express Enzyme (Fisher Scientific). Next, the now suspended cells were taken from the culture plate and run through a 40 μm strainer (MTC Bio SureStrain Premium Cell Strainers) to remove any cell clumps. An excess of media was then run through the strainer to prevent individual cells from sticking to the mesh. Centrifugation was then used to pellet the cells and the supernatant was aspirated. Cells were then resuspended in a solution of 79% cell media, 17% OptiPrep (Sigma Aldrich), 1.28% DNase I (Thermo Scientific), and 2.55% DNase reaction buffer (Thermo Scientific). The OptiPrep prevented cells from sedimenting during the flow experiment and the DNase minimized cell clumping caused by free DNA strands. Following sorting experiments, cells were recovered from droplets surrounded by oil by adding approximately twice the droplet volume of Pico-Break 1 (Sphere Fluidics) to the collection tube and leaving the mixture to sit on ice for 1 min. The top solution was then gently pipetted up and down. This caused the droplets to lyse, leaving a two-layer liquid with oil on the bottom and cell media containing cells on the top. Next, an excess of cell media containing 20% OptiPrep was added. The collection tube was then centrifuged at 100 g for 5 s to better delineate the interface between the oil and the aqueous suspension of cells. The addition of OptiPrep minimized the likelihood of cells being pinned to the oil–aqueous interface. The top layer was then carefully removed, pipetting this layer into a clean centrifuge tube. An excess of OptiPrep-free cell media was then added to this tube, diluting the OptiPrep and allowing the cells to be pelleted by centrifugation. Following pellet formation, the supernatant was aspirated and replaced with fresh media. The cells could then be re-plated on a glass bottom well for further imaging and characterization.
Cell sorting experiments
A microfluidic device was placed atop a microscope stage. The microscope was equipped with a high-speed camera (Integrated Design Tools M3), a pump source to excite LPs and a home-built spectrometer to measure lasing output. The pump laser focus was positioned adjacent to the sorting junction. Of the three input flow channels, one was connected to an aqueous reservoir comprised of LP-tagged cells. The other two were filled with droplet generation oil (Bio-Rad QX200 Droplet Generation Oil). Flow was driven using pressurized sources (Fluigent Flow EZ) and velocity controlled by flow meters (Fluigent Flow Unit) with closed loop feedback. The two outflow channels were each connected to collection tubes. Flow speeds were first set at 35 μL min−1 into the spacer inlet, 5 μL min−1 into the oil generation inlet, and 2 μL min−1 for the aqueous cell solution. This resulted in the generation of droplets containing tagged cells. These droplets were then spaced by oil from the spacer inlet, enabling a single droplet to be sorted at a time. The flow speeds were then tuned to achieve the correct timing by using the high-speed camera to monitor droplet flow patterns. One end of the live electrode and one end of the ground electrode was then connected to a high-voltage amplifier (TREK Model 2210-CE) via crocodile clips. The amplifier amplified a square wave train emanating from a DAQ card (National Instruments PCIe-6321), that was triggered by a pulse sent from an FPGA (National Instruments PCIe-1473R) when the appropriate sorting condition was met.
Optical measurements
LP emission during flow was collected by pumping LPs using a 10 ns pulse width at 1064 nm with 2 MHz repetition rate (CNILaser FL-1064-Nano-LAB). Excitation light was focused using a 10× 0.3 NA objective (Leica HC PL FLUOTAR). Emission light was collected and dispersed using a custom-built grating spectrometer equipped with a linescan camera (Sensors Unlimited 2048 L). This camera was connected to an FPGA (National Instruments PCIe-1473R) that determined whether sorting criteria were met. Sorting accuracy was assessed by comparing the flow device inputs and outputs using a confocal microscope (Olympus Fluoview 3000). This microscope was able to attain brightfield images of cells and LPs and was equipped with a cell incubator (Tokai Hit). Furthermore, the microscope was modified to measure LPs using a 1060–1070 pump laser operating at 2 MHz with 10 ns pulse width (Spectra Physics VGEN-ISP-POD). Spectra were collected by a 20× 0.45NA objective (Olympus IMS LCPLN20XIR) and sent to a spectrometer (Andor Kymera 328i) equipped with a linescan camera (Sensors Unlimited 2048 L). All camera data was saved and analyzed using custom code based on MATLAB and Python.
Author contributions
P. H. D., J. K., and J. Wang fabricated the microfluidic chips and performed flow experiments. A. K. and S. F. performed cell work. P. H. D., N. M., and J. Wu designed and constructed the optical imaging systems and their associated electronics. P. H. D. and N. M. wrote the data analysis code. P. H. D., J. K., N. M., and A. C. L. fabricated the LPs used in the experiments. S. H. Y. supervised the project. P. H. D. and S. H. Y. wrote the manuscript with input from all other authors.
Conflicts of interest
P. H. D., N. M., and S. H. Y. hold patents on laser particle technologies. S. H. Y., A. C. L., N. M., and S. F. have financial interests in LASE Innovation Inc., a company focused on commercializing technologies based on laser particles. The financial interests of S. H. Y. and N. M. were reviewed and are managed by Mass General Brigham in accordance with their conflict-of-interest policies.
Acknowledgements
This work was supported by the US National Institutes of Health grants (DP1-OD022296, R01-EB033155). This research used the resources of the Center for Functional Nanomaterials, which is a US Department of Energy Office of Science User Facility, at Brookhaven National Laboratory under contract number DE-SC0012704 and of the Center for Nanoscale Systems, part of Harvard University, a member of the National Nanotechnology Coordinated Infrastructure Network, which is supported by the NSF under award number 1541959. P. H. D. acknowledges funding from the Harvard University Presidential Scholars Fund, N. M. acknowledges Bullock postdoctoral fellowship, and J. Wu and J. Wang acknowledge funding from the China Scholarship Council. We thank Kwon-Hyeon Kim for his thoughtful reading and comments regarding the manuscript draft. We also thank Adam Abate for sending CAD designs of a microfluidic sorting junction.
References
- N. Martino, S. J. J. Kwok, A. C. Liapis, S. Forward, H. Jang, H. M. Kim, S. J. Wu, J. Wu, P. H. Dannenberg, S. J. Jang, Y. H. Lee and S. H. Yun, Nat. Photonics, 2019, 13, 720–727 CrossRef CAS PubMed.
- A. H. Fikouras, M. Schubert, M. Karl, J. D. Kumar, S. J. Powis, A. Di Falco and M. C. Gather, Nat. Commun., 2018, 9, 4817 CrossRef PubMed.
- V. M. Titze, S. Caixeiro, A. Di Falco, M. Schubert and M. C. Gather, ACS Photonics, 2022, 9, 952–960 CrossRef CAS PubMed.
- J. Brummelman, C. Haftmann, N. G. Núñez, G. Alvisi, E. M. C. Mazza, B. Becher and E. Lugli, Nat. Protoc., 2019, 14, 1946–1969 CrossRef CAS PubMed.
- P. K. Chattopadhyay, D. A. Price, T. F. Harper, M. R. Betts, J. Yu, E. Gostick, S. P. Perfetto, P. Goepfert, R. A. Koup, S. C. De Rosa, M. P. Bruchez and M. Roederer, Nat. Med., 2006, 12(128), 972–977 CrossRef CAS PubMed.
- M. Humar, A. Upadhya and S. H. Yun, Lab Chip, 2017, 17, 2777–2784 RSC.
- S. J. J. Kwok, N. Martino, P. H. Dannenberg and S. H. Yun, Light: Sci. Appl., 2019, 8, 74 CrossRef PubMed.
- A. Adan, G. Alizada, Y. Kiraz, Y. Baran and A. Nalbant, Crit. Rev. Biotechnol., 2017, 37, 163–176 CrossRef CAS PubMed.
- J. P. Nolan, D. Condello, E. Duggan, M. Naivar and D. Novo, Cytometry, Part A, 2013, 83, 253–264 CrossRef PubMed.
- W. A. Bonner, H. R. Hulett, R. G. Sweet and L. A. Herzenberg, Rev. Sci. Instrum., 2003, 43, 404 CrossRef PubMed.
- J. Krüger, K. Singh, A. O'Neill, C. Jackson, A. Morrison and P. O'Brein, J. Micromech. Microeng., 2002, 12, 486 CrossRef.
- K. Ahn, C. Kerbage, T. P. Hunt, R. M. Westervelt, D. R. Link and D. A. Weitz, Appl. Phys. Lett., 2006, 88, 1–3 Search PubMed.
-
H. Bruus, Theoretical Microfluidics, Oxford University Press, 2007 Search PubMed.
- P. H. Dannenberg, A. C. Liapis, N. Martino, J. Kang, Y. Wu, A. Kashiparekh and S.-H. Yun, ACS Photonics, 2021, 8, 1301–1306 CrossRef CAS.
- M. Schubert, K. Volckaert, M. Karl, A. Morton, P. Liehm, G. B. Miles, S. J. Powis and M. C. Gather, Sci. Rep., 2017, 7, 40877 CrossRef CAS PubMed.
- S.-J. Tang, P. H. Dannenberg, A. C. Liapis, N. Martino, Y. Zhuo, Y.-F. Xiao and S.-H. Yun, Light: Sci. Appl., 2021, 10, 23 CrossRef CAS PubMed.
- A. Sciambi and A. R. Abate, Lab Chip, 2015, 15, 47–51 RSC.
- H. Keren-Shaul, E. Kenigsberg, D. A. Jaitin, E. David, F. Paul, A. Tanay and I. Amit, Nat. Protoc., 2019, 14, 1841–1862 CrossRef CAS PubMed.
- J. Cao, J. S. Packer, V. Ramani, D. A. Cusanovich, C. Huynh, R. Daza, X. Qiu, C. Lee, S. N. Furlan, F. J. Steemers, A. Adey, R. H. Waterston, C. Trapnell and J. Shendure, Science, 2017, 357, 661–667 CrossRef CAS PubMed.
- A. Cossarizza, H. D. Chang, A. Radbruch, M. Akdis, I. Andrä, F. Annunziato, P. Bacher, V. Barnaba, L. Battistini and W. M. Bauer,
et al.
, Eur. J. Immunol., 2017, 47, 1584–1797 CrossRef CAS PubMed.
- D. A. Nielsen, A. Lernmark, M. Berelowitz, G. D. Bloom and D. F. Steiner, Diabetes, 1982, 31, 299–306 CrossRef CAS PubMed.
- A. Grützkau and A. Radbruch, Cytometry, Part A, 2010, 77, 643–647 CrossRef PubMed.
- D. Schraivogel, T. M. Kuhn, B. Rauscher, M. Rodríguez-Martínez, M. Paulsen, K. Owsley, A. Middlebrook, C. Tischer, B. Ramasz, D. Ordoñez-Rueda, M. Dees, S. Cuylen-Haering, E. Diebold and L. M. Steinmetz, Science, 2022, 375, 315–320 CrossRef CAS PubMed.
- S. Song, M. Manook, J. Kwun, A. M. Jackson, S. J. Knechtle and G. Kelsoe, Commun. Biol., 2021, 4, 1338 CrossRef CAS PubMed.
- P. O. Krutzik and G. P. Nolan, Nat. Methods, 2006, 3(5), 361–368 CrossRef CAS PubMed.
- H. D. Xi, H. Zheng, W. Guo, A. M. Gañán-Calvo, Y. Ai, C. W. Tsao, J. Zhou, W. Li, Y. Huang, N. T. Nguyen and S. H. Tan, Lab Chip, 2017, 17, 751–771 RSC.
- S. Li, X. Ding, F. Guo, Y. Chen, M. I. Lapsley, S. C. S. Lin, L. Wang, J. P. McCoy, C. E. Cameron and T. J. Huang, Anal. Chem., 2013, 85, 5468–5474 CrossRef CAS PubMed.
- A. Adan, G. Alizada, Y. Kiraz, Y. Baran and A. Nalbant, Crit. Rev. Biotechnol., 2017, 37, 163–176 CrossRef CAS PubMed.
|
This journal is © The Royal Society of Chemistry 2022 |
Click here to see how this site uses Cookies. View our privacy policy here.