DOI:
10.1039/D3LC00691C
(Perspective)
Lab Chip, 2023,
23, 5068-5080
Towards skin-on-a-chip for screening the dermal absorption of cosmetics
Received
10th August 2023
, Accepted 23rd October 2023
First published on 1st November 2023
Abstract
Over the past few decades, there have been increasing global efforts to limit or ban the use of animals for testing cosmetic products. This ambition has been at the heart of international endeavours to develop new in vitro and animal-free approaches for assessing the safety of cosmetics. While several of these new approach methodologies (NAMs) have been approved for assessing different toxicological endpoints in the UK and across the EU, there remains an absence of animal-free methods for screening for dermal absorption; a measure that assesses the degree to which chemical substances can become systemically available through contact with human skin. Here, we identify some of the major technical barriers that have impacted regulatory recognition of an in vitro skin model for this purpose and propose how these could be overcome on-chip using artificial cells engineered from the bottom-up. As part of our future perspective, we suggest how this could be realised using a digital biomanufacturing pipeline that connects the design, microfluidic generation and 3D printing of artificial cells into user-crafted synthetic tissues. We highlight milestone achievements towards this goal, identify future challenges, and suggest how the ability to engineer animal-free skin models could have significant long-term consequences for dermal absorption screening, as well as for other applications.
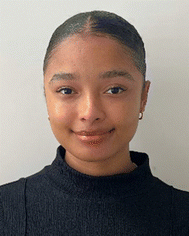 Jessica Govey-Scotland | Jessica Govey-Scotland is an associate toxicologist who works for Reckitt. She developed her expertise in the area of New Approach Methodologies while pursuing her MRes in Molecular Engineering from the Institute for Molecular Science and Engineering (IMSE) at Imperial College London in 2021. She won the IMSE prize for excellence in her year. Jess currently serves as a REACH (Registration, Evaluation, Authorisation and Restriction of Chemicals) Safety Associate in the Regulatory and Safety Department of Reckitt as a member of the Technical Consumer Safety team. She graduated from the University of Kent with a BSc in Chemistry in 2020. |
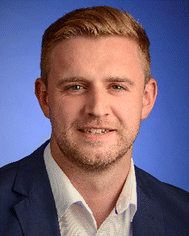 Liam Johnstone | Liam Johnstone is a toxicologist with expertise in the safety of medicines, consumer products and agrochemicals, developing the knowledge of these areas whilst working for several different UK national regulators. He has experience of commissioning a range of different research projects including the efficacy of UV light in killing SAR-COV-2, and the chemical risks from the use of recycled materials; the findings of which have been used to help inform Government policy. Liam holds a MSc in Addictions from Kings College London, a MSc in Human Disease and Therapy from the University of Leeds, and a BSc in Biomedical Sciences from Northumbria University. |
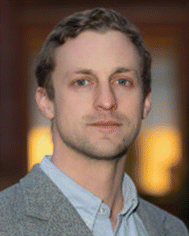 Connor Myant | Connor Myant is a Senior Lecturer (Assistant Professor) and group lead of the Advanced Manufacturing Group at the Dyson School of Design Engineering. His research interests include design for additive manufacture, mass customisation, development of 3D printing technology and software, and tribology. He previously worked in the ICL Tribology Group, where he held a Junior Research Fellowship studying synovial fluid lubrication of artificial articular joints. Connor gained his PhD from Imperial College London in 2010 on the development of experimental techniques for investigating lubricated, compliant, bearing contacts. He graduated from the University of Exeter in 2006 with a Bachelors (BEng) in Mechanical Engineering. |
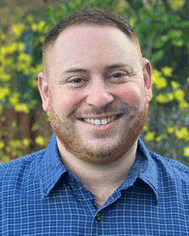 Mark Friddin | Mark Friddin is an Imperial College Research Fellow and co-lead of the Advanced Manufacturing Group at the Dyson School of Design Engineering. His group works in the areas of bioprinting, artificial cell engineering, and rapid prototyped microfluidics. He previously worked as a PDRA in the Membrane Biophysics Group, where he developed novel strategies to generate, functionalise and manipulate artificial cells. Mark obtained his PhD from the University of Southampton where he explored the coupled expression and characterisation of ion channels in droplet interface bilayers. Mark also holds an MSc in Micro- and Nano-Technology and a BSc in Biochemistry. |
1. Introduction
Cosmetic products are mixtures of synthetic or naturally derived chemical compounds that are typically applied to the human body to modify or maintain appearance, rather than to alter biological structure or function. While the precise definition of a cosmetic product, and the way in which they are regulated varies between locations, the common goal is to ensure that products entering the market are safe for consumer use. The UK market for cosmetics, for example, is the fourth largest in Europe and is estimated to be worth over £8bn. Prior to cosmetic products being placed on the UK market, it is essential that they meet the requirements set out in Schedule 34 of the Product Safety and Metrology Statutory Instrument,1 or Regulation (EC) No. 1223/2009 for NI.2 One of the key obligations outlined in these regulations is that the cosmetic product made available shall be safe for human health when used under normal or foreseeable conditions of use. To ensure that this condition is met, it is necessary for the ‘responsible person’ (normally the manufacturer, the importer or the distributor) to conduct a Cosmetic Risk Assessment to evaluate the safety of the product. This process has historically relied on animal testing, which was banned in the UK and EU for cosmetic testing in 2013, and is either restricted or prohibited in 40 other locations worldwide. The development and rollout of new approach methodologies (NAMs) that can assess different toxicological endpoints without the use of animals has therefore been a strategic priority for the scientific community, for the cosmetics industry, and for regulators for some time. To meet this demand, there has been great progress in the development of new in vitro methods, several of which have been approved by the Organisation for Economic Co-operation and Development (OECD) for use in Cosmetic Risk Assessments.3 Regulatory recognition of NAMs in the UK and EU has also increased significantly in recent years with the acceptance of Defined Approaches (DAs), which rely on specific combinations of in silico, in chemico and in vitro methods to compensate for the limitations of individual testing methods when modelling human exposure. Major milestones in this space include OECD approval of Defined Approaches on Skin Sensitisation (Guideline No. 497)4 and Defined Approaches for Serious Eye Damage and Eye Irritation (Test No. 467).5 Similarly, the adoption of Test No. 442E: In vitro Skin Sensitisation addresses the Key Events (KE) in the Adverse Outcome Pathway (AOP) for skin sensitisation using platforms such as SenzaGen's GARDskin.6 This pioneering approach combines gene expression analysis with machine learning to create a Genomic Allergen Rapid Detection (GARD) system where users can identify biomarker signatures in a human dendritic-like cell line and feed them into an in silico prediction model for producing classifications.7 Recent reports have also described using the assay as an in vitro platform for assessing skin sensitising potency.8
Yet despite significant progress in the development of NAMs for use in cosmetic safety assessments, there remains an absence of OECD-approved animal-free alternatives for assessing dermal absorption; a measure of how a chemical substance can become systemically available by penetrating or permeating the skin. This is assessed using OECD Test Guideline No. 428 for Dermal Absorption but remains reliant on ex vivo human and animal tissues.9 This owes largely to the fact that in vivo human studies are usually not appropriate during the early development of a novel pharmaceutical or cosmetic, and a lack of OECD-approved alternatives. Here, we seek to understand the technical barriers that have led to the current status-quo, and propose how they might be addressed using synthetic tissues engineered from artificial cells.
Artificial cells are synthetic constructs that aim to replicate the function and behaviour of natural biological cells. In contrast to top-down synthetic biology, where users engineer synthetic phenotypes from wild-type cells, bottom-up synthetic biology requires the complete assembly of artificial cells from fundamental building blocks such as soft matter, proteins and biomolecules. While these assemblies lack the natural complexity associated with their biological counterparts, one of the major advantages is that they can be precisely engineered at high-frequency under fine user-control using microfluidics.10 There are also existing strategies for sensitising artificial cells to different chemical11,12 and mechanical13 stimuli, as well as for assembling them into extended 2D and 3D networks, which are becoming increasingly regarded as powerful minimal tissue models.14 Here, we discuss how these features could be leveraged to engineer an animal-free model for screening for dermal absorption, and explore how this might be achieved using a digital manufacturing pipeline that connects the design, manufacturing, and 3D printing of artificial cells and tissues.
2. Dermal absorption
The skin is the largest organ of the human body and consists of up to seven biologically distinct layers which exhibit separate absorption characteristics in response to different chemical classes.15 The process of dermal absorption (Fig. 1) begins with penetrating the stratum corneum, a 10–20 μm thick layer comprised of vertical columns of interlocked corneocytes. These terminally differentiated keratinocytes do not contain nuclei or cytoplasmic organelles, are encapsulated by a highly insoluble cornified envelope and are embedded within a matrix of specialised non-polar lipids which serve as the main barrier to block dermal absorption.16,17 Penetration of the stratum corneum therefore requires materials to pass through the intercellular spaces via a tortuous pathway or by diffusion (as indicated in Fig. 1a),16,18,19 before they can migrate down to the viable epidermis, the dermis and eventually the vascular network where they enter systemic circulation. It is here that other pharmacokinetic properties of the material become significant for determining its safety and efficacy, including how it is distributed, metabolised and excreted by the body. An assessment for dermal absorption therefore forms a critical part of the standard battery of studies completed as part of determining and classifying chemical hazards, particularly in the cosmetic, pharmaceutical and agrochemical industries. In the context of cosmetics, this process relies heavily on ex vivo human tissue and more commonly ex vivo animal tissue, due to the lack of availability of the former.9 This creates a chasm between results obtained from ex vivo animal experiments and dermal absorption data generated from ex vivo and in vivo human studies.20 While several studies have shown some correlation between porcine (and to a lesser extent rodent skin) and human permeation, there are still open questions regarding the accuracy of dermato-pharmacokinetic (DPK) profiles extrapolated from animal data, and limited options for studying different skin types, which can vary significantly between subjects of different age, ethnicity and disease states (Fig. 1b).21 A generic route to systemic absorption is outlined in Fig. 1c.
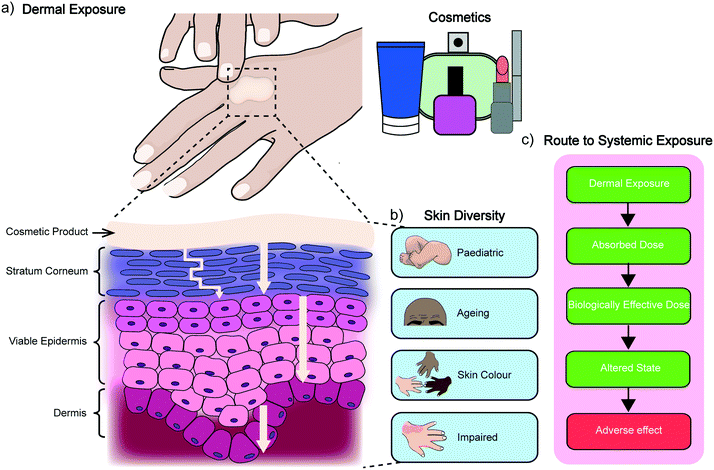 |
| Fig. 1 Dermal absorption. a) Illustration of human skin exposure to a cosmetic product. The turning arrow depicts the tortuous pathway of permeation through the stratum corneum, while the straight arrows relate to the effective diffusion coefficients of each layer. b) Representation of the diversity of skin types. c) An outline of a typical route to systemic exposure. | |
3.
In vitro dermal absorption methods
The Franz diffusion cell (Fig. 2a) is the classic gold standard for assessing dermal absorption in vitro. Depending on the application, the method usually consists of a synthetic (e.g. lipid or polymer model membrane) or ex vivo (e.g. human epidermis) membrane sandwiched between a fluid-filled donor and receptor compartment, where the receptor fluid is used to mimic microcirculation in the dermis and the donor compartment is used to introduce the test material. Typically, where ex vivo human tissue is available, the method is used for assessing permeation whereas synthetic membranes tend to be employed for determining release due to their different material properties. However, despite the wide adoption of the technique, its ability to accurately estimate permeation kinetics is understood to be limited by the presence of the unstirred water layer.22 It is also known to suffer from other drawbacks including its low throughput, lack of standardisation (despite OECD test guideline (No. 428)),23 and inability to replicate the metabolic action that is typically associated with the dermis and sub-dermis. These factors have motivated the development of high-throughput approaches that are based on the parallel artificial membrane permeability assay technique (PAMPA), (Fig. 2b). Ottaviani and co-workers reported the adaptation of this technique to create the first skin PAMPA, which leveraged a mixture of silicone and isopropyl myristate to engineer a ‘skin-like’ synthetic membrane with reduced permeability.24 Efforts have sought to establish the efficacy of such approaches by comparing them against in vivo methods,15 yet despite promising results regulatory recognition of this is varied and is not universally accepted.
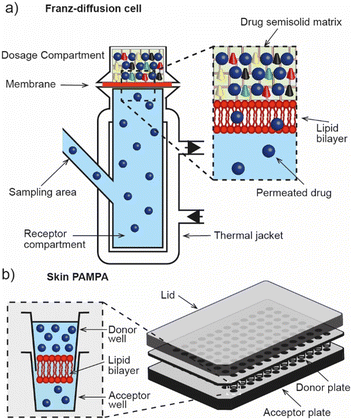 |
| Fig. 2
In vitro dermal absorption methods. a) Franz-diffusion cell for screening the dermal absorption of chemical species across an ex vivo or synthetic membrane. b) Skin PAMPA assay for high-throughput in vitro dermal absorption screening. | |
4. Challenges in engineering skin models for screening for dermal absorption
In recent years, efforts to improve biomimicry and endpoint determination of in vitro skin models has driven the shift away from traditional 2D cell studies and toward 3D culture systems that leverage approaches such as 3D bioprinting25 and organ-on-a-chip (OoC) technology.26,27 Here, 3D bioprinting offers users the ability to create skin models layer-by-layer by precisely depositing cell-laden gels (often designed to mimic native extracellular matrices, ECMs) from a user-generated design, while OoC uses microfluidics to imitate vascularisation, to provide dynamic perfusion and to achieve precise control of culture conditions in efforts to engineer a microenvironment that is more physiologically representative of their origin. When elastomeric substrates are used to engineer devices, investigators also have the option to bolster this effort by imparting biomechanical stimuli that can replicate breathing,28 peristaltic movements,29 and stretching.30 Moreover, there is increasing promise that multiple OoC devices can be connected together to model the impact of systemic exposure on other biologically relevant organs.31 Although these technologies are already yielding new approaches for high-throughput toxicity screening,32 skin irritation,33 and inflammation,34 to our knowledge, the challenge of engineering an animal-free platform for dermal absorption screening remains outstanding due to problems with replicating barrier function in vitro, difficulties in reconstituting metabolism, and the tendency to compare results to data obtained from animal testing.
4.1 Reconstituting barrier function
Percutaneous absorption is determined by the stratum corneum (Fig. 1) which consists of key lipid classes; ceramides (CER), cholesterol, cholesterol sulphate, and free fatty acids (FFA). These lipids form lamellar membranes that are templated by the patterned corneocyte wall35 and extend in a horizontal direction parallel to the corneocyte.16 In addition to lipids, the ECM of the stratum corneum is also known to consist of enzymes, structural proteins, and antimicrobial peptides – many of which have been shown to contribute to barrier function.36 The ECM of the stratum corneum is therefore metabolically active, and understood to dynamically change in structure and function during transit to the skin surface, where epidermal lipids can become incorporated into a mixture of sebaceous lipids (from sebum oil present on the skin surface) though interactions with sebum triglycerides.37 Together, these components form the skin's barrier function, which has proven challenging to replicate using engineered cell lines due to the difficulty of replicating the ratio of the key lipid classes and associated components in vitro.38
In native human tissue CER 1 and 4 are particularly important in aiding the skins barrier function through the formation of long-range lipid bilayer phases (13 nm) and short-range lipid bilayer phases (6 nm) in the stratum corneum.19,39 A poorer barrier function in vitro has been linked to deviations in the amount of these CERs which causes an increase in short range lipid bilayer phases, due to linoleic acid and ω-hydroxy fatty acid.39 Analysis of EpiSkin, EpiDerm, and SkinEthic reconstructed models, however, suggests that the periodicity of these bilayer phases is not the governing factor for the skins permeability.38 The packing arrangement of these lipid bilayer phases can be hexagonal or orthorhombic, with the latter increasing the lipid packing density and resulting barrier function.19 Limited long chained FFA can prevent the formation of an orthorhombic lipid bilayer arrangement and may be linked to increasing the permeability in current reconstructed tissue models.40 Consequently, engineered skin models do not exhibit a barrier function that is comparable to human or animal skin, which prohibits their application as part of an in vitro assay for dermal absorption. In order to make in vitro models more biorepresentative, efforts have been made to incorporate the dermis to achieve epidermal homeostasis, to improve cell differentiation and resulting lipid ratio, and to develop full-thickness tissue. However, these methods also suffer from their own drawbacks. For example, collagen matrices used in the fabrication of full thickness skin equivalents are known to contract in the culture chamber, impacting their barrier function by compromising adhesion with the chamber walls. Consequently, none of these approaches have been approved for use.41,42 Further details of the biophysical roles of ceramides in the stratum corneum can be obtained elsewhere.43
4.2 Replicating metabolism
Another challenge in creating skin models for screening for dermal absorption is replicating metabolism, a process where the activity, toxicity, and water solubility of a penetrating substance can be significantly modified by the skin, potentially leading to different physiological responses as well as routes for excretion and elimination.44 This process is typically achieved in two consecutive phases; a functionalisation phase, where a polar group is either generated or unmasked by oxidative, reductive or hydrolytic reaction; and a conjugation phase, where small hydrophilic endogenous molecules (e.g., glucuronic acid, sulphate or glycerine) are attached by covalent bonds. These steps can significantly alter the dermal absorption profile of a chemical formulation, making it an important factor to consider when determining permeation potentials. Moreover, fully reconstituting this activity from frozen tissue samples is also a challenge in ex vivo studies (both from human and from animal tissue) and is another factor that must be considered when determining metabolic profiles.
4.3 Comparison to animal studies
In addition to difficulties in replicating barrier function and reconstituting metabolic activity in vitro, another challenge that faces the development of animal-free models for screening for dermal absorption is the tendency to compare findings to data obtained from ex vivo animal tissue or live animal testing. This is problematic due to the open questions surrounding the physiological relevance of these samples, and the high inter- and intra-laboratory variability reported by users. However despite these concerns, the performance of engineered skin models continues to rely on historical animal data, which can be misleading when validating the performance of new in vitro models and subsequently impact their approval for use.45,46
In addition to the recently introduced route of Defined Approaches, another approach that avoids reliance on animal reference data while also building confidence in the use of new data for human applications could be the use of Adverse Outcome Pathways (AOPs).47 These were introduced by the OECD in 2012 and link existing knowledge in a linear way along one or a series of causally connected Key Events (KE) between a Molecular Initiating Event (MIE) and an Adverse Outcome (AO), where the linkage between events are described by Key Event Relationships (KER), which detail the relationships between the KEs. To this end, engineering skin models based on KEs in an AOP can ensure they are scientifically valid and based on a mechanistic understanding of human biology; leveraging the ability to generate human-relevant data, rather than relying on existing animal data.47
Collectively, the issues of replicating barrier function and metabolism in vitro, combined with the availability of ex vivo human tissue and the problems of using ex vivo animal data, motivates the exploration of alternative materials in the construction of skin models for screening for dermal absorption.
5. Opportunities for constructing skin models for dermal absorption using artificial cells
Artificial lipid bilayers have been used for decades to probe the biophysical properties of membranes, to study the behaviour of membrane proteins, and to screen for membrane and protein interactions with drugs. While classical methods have traditionally relied on Langmuir–Blodgett films, black/bilayer lipid membranes (BLMs) and supported lipid bilayers (SLBs), contemporary approaches employ single- or multicompartment architectures that have become widely known as artificial cells (Fig. 3). Popular manifolds in this context include the use of droplet interface bilayers (DIBs), which are lipid bilayers formed at the interface of two lipid monolayer-coated water droplets in oil (Fig. 3, left); vesicles (also known as liposomes), which are lipid bilayer encased water droplets; and multicompartment vesicles, which consist of multiple interlinked vesicles (Fig. 3, right). The distinct advantages and drawbacks of each method as well as details of other approaches (including polymer and hybrid architectures) are discussed elsewhere.48 There are also multiple strategies for generating them on-chip,10 including approaches for mitigating the effects of the unstirred water layer.22,49 Moreover, they all share features and present opportunities that could be leveraged as part of a bottom-up skin model for screening for dermal absorption. This includes fine control of the lipid and protein composition of the membrane (Fig. 4a), the option to encapsulate chemical components and biomolecular machinery (Fig. 4b), and the freedom to construct 3D tissue-like assemblies from thousands of individual subunits (Fig. 4c).14
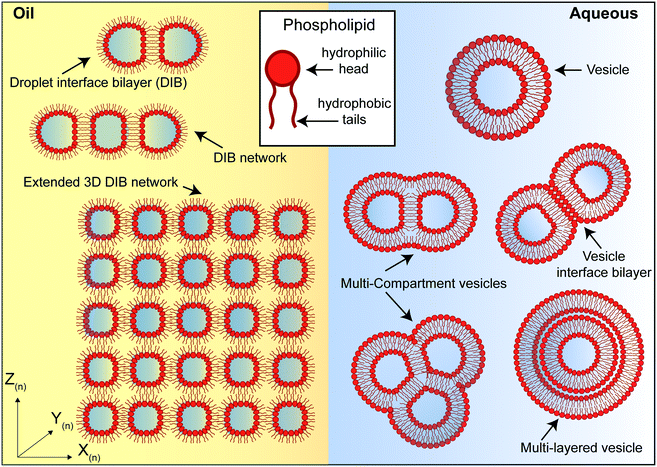 |
| Fig. 3 Different phospholipid-based artificial cell and tissue manifolds. Left: water droplet in oil assemblies. A lipid monolayer assembles at the water : oil interface and a droplet interface bilayer (DIB) is formed when two droplets are placed into contact. Additional compartments can be added in 2D and 3D to create more complex tissue-like structures. Right: vesicle assemblies. A lipid bilayer encapsulates an aqueous volume ranging from nm (small unilamellar vesicles) to μm (giant unilamellar vesicles, as shown) in diameter. It is also possible to engineer multicompartment vesicle architectures such as multicompartment vesicles, vesicle interface bilayers and multilayered vesicles. | |
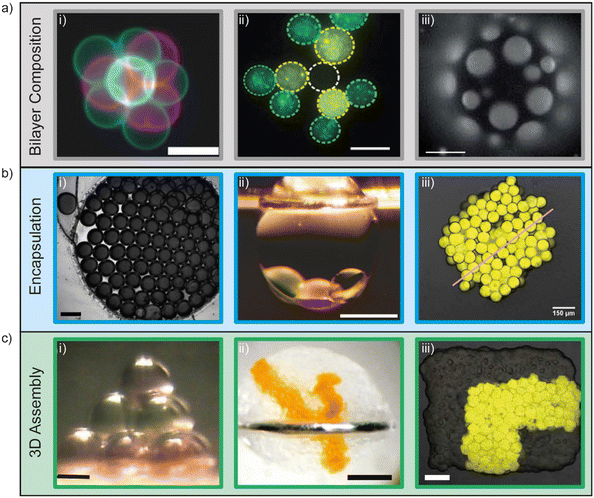 |
| Fig. 4 Demonstrations of fine user control of a) membrane composition, b) encapsulation and c) 3D assembly of artificial cells. a) Control of membrane composition, i) fluorescent lipids used to construct and identify vesicles for 3D assembly using optical traps (scale bar = 10 μm). Image reprinted from ref. 67 under a Creative Commons Attribution 4.0 International License (https://creativecommons.org/licenses/by/4.0/) and was originally published by Nature Publishing Group. ii) Fluorescent lipids used to construct and identify lipid monolayer encased water droplets in oil for 3D assembly using optical traps (scale bar = 15 μm). Image reprinted from ref. 77 under a Creative Commons Attribution 3.0 Unported License (https://creativecommons.org/licenses/by/3.0/) and was originally published by The Royal Society of Chemistry. iii) Ternary vesicles with different lateral membrane structure (scale bar = 5 μm). Image reprinted from ref. 51 under a Creative Commons Attribution 3.0 Unported License (https://creativecommons.org/licenses/by/3.0/) and was originally published by The Royal Society of Chemistry. b) Control of encapsulation i) encapsulation of dye on a rapid prototyped droplet microfluidic device (scale bar = 200 μm). Image reprinted from ref. 81. Copyright 2018 American Chemical Society. ii) Encapsulation of a three droplet network inside an oil in water droplet (scale bar = 400 μm). Image modified from ref. 59 with reprint permission from Nature Publishing Group. iii) Encapsulation of IVTT systems in artificial tissues (scale bar = 150 μm). Image reprinted from ref. 65 under a Creative Commons Attribution-Non Commercial License (https://creativecommons.org/licenses/by-nc/4.0/) and was originally published by the American Association for the Advancement of Science. c) 3D assembly of artificial cells. i) Magnetic manipulation of 10 lipid monolayer coated water droplets in oil into a pyramid (scale bar = 200 μm). Image reprinted from ref. 76. Copyright 2014 American Chemical Society. ii) 3D printing of lipid monolayer coated water droplets in oil into tissue-like assemblies in bulk aqueous solution (scale bar = 400 μm). Image reprinted from ref. 14 with permission from the American Association for the Advancement of Science. iii) Light-activated patterned expression of proteins in a 3D printed synthetic tissue (scale bar = 75 μm). Image reprinted from ref. 65 under a Creative Commons Attribution-Non Commercial License (https://creativecommons.org/licenses/by-nc/4.0/) and was originally published by the American Association for the Advancement of Science. | |
5.1 Control of lipid bilayer composition
All phospholipid-based model membrane and artificial cell architectures require the user to supply lipids, meaning that the exact formulation of the membrane can be precisely designed if only pure components are used (Fig. 4a). This fundamental property has been exploited widely in a number of studies, from probing the sensitivity of ion channels to negatively charged lipids in BLMs,50 to investigating the assembly of lipid domains in vesicles,51 SLBs,52 and in droplet-hydrogel bilayers.53 The ability to construct asymmetric bilayers using DIBs has also been exploited to probe the gating characteristics of OmpG,54 to activate embedded mechanosensitive channels,13 and to explore the role of alamethicin peptides in facilitating the translocation and mixing of lipids (also known as bilayer flip-flop) across bilayer leaflets.55 Efforts to replicate plant membranes have also been attempted using the DIB platform by controlling the amount of phospholipids, sterols and cerebrosides to mimic the membrane compositions of Arabidopsis thaliana, tobacco and oats.56 Further, reports have detailed the use of a microfluidic platform that utilises DIBs composed of L-α-phosphatidylcholine (PC) and L-α-phosphatidylethanolamine (PE) in a minimised version of a PAMPA assay used to mimic the jejunum.57 The same group also investigated the role of temperature in the assembly and stability of DIBs in a move towards the use of naturally derived lipids (instead of synthetic lipids) for engineering human-mimetic artificial cells.58 Together, these reports showcase the potential to precisely engineer membranes according to the needs of users.
5.2 Encapsulation of chemical components and biomolecular machinery
In contrast to traditional BLM setups, where ∼1 mL chambers are separated by an aperture-suspended lipid bilayer, contemporary models that leverage droplet microfluidics often consist of lipid monolayer or lipid bilayer encased subunits that can range in diameter from tens of microns through to millimetres in diameter.10 The scale and volume of these subunits presents opportunities both to encapsulate chemical components and biomolecular machinery (Fig. 4b), and to separate them into discrete internal compartments. This capability has been reported by demonstrating both the intercompartment exchange of calcium ions in water/oil/water double emulsions59 and the parallel processing of dual environmental signals in a three compartment multisome.60 It has also been shown that a three-step enzymatic cascade can be directed in three spatially segregated compartments within a multicompartment vesicle.61 Arguably, one of the most complex biochemical processes that can be achieved in compartmentalised architectures is the cell-free expression of proteins, which involves supplying a DNA or RNA template to a mixture containing the ingredients required to complete transcription and/or translation (i.e. a cell lysate, amino acids, and a metabolic energy supply). In their landmark paper, Noireaux and Libchaber demonstrated that in vitro transcription/translation (IVTT) could be realised within a single vesicle bioreactor and used to express the fluorescent protein eGFP and the α-haemolysin pore protein from Staphylococcus aureus.62 It was later shown that the same protein pore could be expressed and reconstituted in situ within a DIB platform, but issues were highlighted with temporal bilayer stability using an E. coli lysate which prohibited the coupled expression and characterisation of more complex proteins that required longer expression times. The same group also studied the use of the highly engineered Protein Expression using Recombinant Elements (PURE)63 system in the same DIB format, which extended the lifetime of the DIBs by ∼8 h but could not support protein expression at room temperature.64 In order to reinforce DIBs formed in the presence of the PURE system, the use of PEGylated lipids has been reported as part of a novel approach that allowed protein expression embedded in DIB networks to be controlled via light sensitive DNA promoters.65,66 In a different approach, it has also been shown that light can also be used to trigger the expression of proteins by fusing cell-sized vesicles containing IVTT components together using optical tweezers.67 Collectively, these achievements demonstrate the rich potential for embedding functionality into artificial cells, and present scope for engineering metabolism-like activity within synthetic constructs.
5.3 Construction of artificial cells into synthetic tissues
Perhaps one of the most unique properties of artificial cells is the ability to place successive subunits into contact to form networked architectures (Fig. 4c). This was first achieved using DIBs through the manual formation of a 6-droplet biobattery using an anion selective α-haemolysin pore, and 5-droplet light sensing network that leveraged bacteriorhodopsin.68 Contactless assembly of microlitre droplets into a three droplet network has also been reported using droplet dielectrophoresis/electrowetting-on-dielectric (EWOD),69 while the first high-throughput approach allowed 3D DIB networks to be created in linear sequences of alternating composition using a combination of polyethylene tubing and glass capillary tubes.70 This method was later extended and scaled-up to build DIB networks consisting of thousands of droplets around the contours of a microfluidic chip, with precise control over droplet size, position and packing density.71 In other approaches, microfluidic devices have been used to assemble DIB networks in traps,72,73 on rails,74 and within alginate shells.75 There are also examples of ‘drag and drop’ strategies for the construction of 3D assemblies, including the use of magnetic beads (Fig. 4c(i)),76 and optical tweezers, which have been used to successfully assemble 3D networks of cell-sized DIBs77 and vesicles.67 These additive methods offer the added advantage of readdressing individual subunits after they have been deposited, providing the user with scope to reconfigure or reposition the network after it has been assembled. In their landmark paper, the Bayley group also reported the 3D printing of ‘tissue-like’ DIB networks from thousands of individual subunits using two piezoelectric actuators to dispense different droplets on-demand (Fig. 4c(ii)).14 The technique was used to construct extended networks with user-defined conduction pathways, self-folding networks that can leverage different osmotic pressures to drive reconfiguration, and formed the basis for assembling light-sensitive DIB networks (Fig. 4c(iii)).65,66 A similar 3D printing approach was also reported where DIB networks consisting of thousands of droplets were encapsulated into a thermoreversible organogel,78 and more recently, the precise spotting of 1D and 2D DIB networks using a microfluidic T-junction to facilitate tailored translocation and reaction cascades has also been achieved.79 The construction, manipulation, and functionalisation of DIB networks is discussed in detail elsewhere.80 In summary, there has been great progress over the past 15–20 years in the development of new droplet microfluidic methods for assembling artificial cells into 3D ‘tissue-like’ constructs. This fuels the notion that these biomimetic fabrics can be used to engineer synthetic skin models that can be deployed to tackle real-world problems, such as the animal-free screening for dermal absorption.
6. Discussion and future perspective
The development of new in vitro models for screening for dermal absorption are needed for alleviating and removing the reliance on ex vivo animal tissues used currently as part of cosmetic risk assessments. However, before any new model can be implemented as part of the assessment process, it should ideally be sufficiently rigorous, robust, and reliable enough to meet the gold-standard of OECD approval, although regulatory validation is not a formal requirement.43 While this has been realised for several of the toxicological endpoints that comprise the risk assessment process for cosmetics, to our knowledge, it remains to be achieved for dermal absorption. Considering the drive towards the development of NAMs, the 10-year timeframe since the ban on animal testing for cosmetics, and the flexibility offered through the introduction of Defined Approaches, we first set out to identify some of the technical roadblocks that have impacted on progress in this space. To this end, we highlighted and unpacked the challenges associated with replicating barrier function and reconstituting metabolic behaviour in vitro. We then suggested how these roadblocks might be overcome using artificial cells by outlining how the lipid composition of membranes can be precisely controlled by the user, how a synthetic metabolism may be imparted by encapsulating chemical reagents or IVTT systems, and how individual subunits can be constructed in 3D to create tissue-like assemblies.
While this union between bottom-up synthetic biology and in vitro dermal absorption methods appears promising in principle, it seems clear from the current state-of-the-art that the technology has yet to arrive at a readiness level where it can be used to engineer approvable synthetic skin models for this application. This leads us to consider the advances that would need to be made and challenges that would need to be overcome in order for this to become possible in the future.
Given that a synthetic skin model engineered from the bottom-up would be expected to consist of tens of thousands of artificial cells that are precisely assembled in 3D, it seems logical to envisage that any future implementation would involve a pipeline that connects design with manufacturing and 3D assembly (Fig. 5). We therefore explore these areas as part of our future perspective and discuss how they could combine to create a digital manufacturing pipeline for engineering a skin-on-a-chip for screening the dermal absorption of cosmetics.
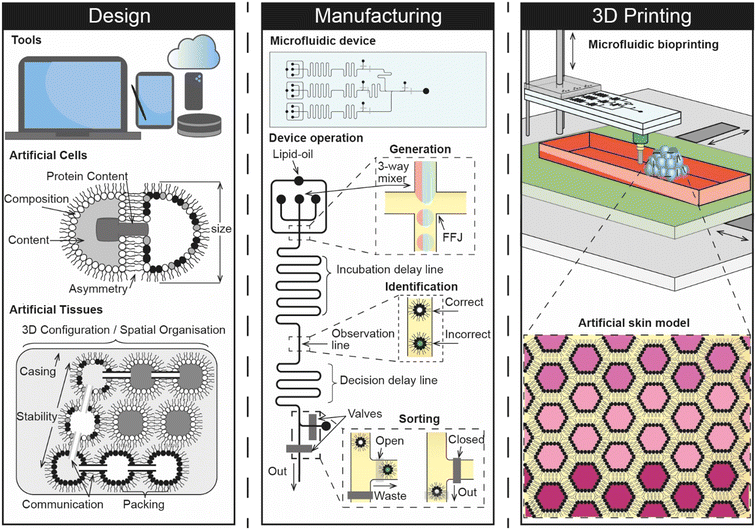 |
| Fig. 5 Concept vision of a digital manufacturing pipeline that connects the design, manufacturing and 3D printing of artificial cells for engineering synthetic skin models. The figure highlights some of the nano-, micro-, and macroscopic design considerations for the construction of artificial cells and tissues, including the composition of membranes, the contents of internal compartments and the 3D configuration/interconnectivity of artificial tissues. The design phase drives the on-chip manufacturing of artificial cells, which can be composed of multiple different ingredients and sorted in-situ before they are 3D printed. This could be achieved by connecting a 3D bioprinting needle to the outlet of the device (underside) and by controlling the position of the build platform relative to the height of the device. | |
6.1 Computer aided design of artificial cells and tissues
Computer aided design (CAD) is central to most engineering and manufacturing processes. Over the past 30 years, the capability of software has increased drastically to a point where users can now construct assemblies from an almost unlimited number of parts composed of a number of different materials and perform detailed mechanical analysis within the same digital environment. Alongside progress in the areas of artificial intelligence and machine learning,82 there have also been advances in parametric design, where 3D CAD models can be rapidly optimised based on algorithmic control of multiple different parameters. As highlighted by recent reports, these trends support the notion that the landscape is steadily shifting towards a point where CAD processes can be fully automated or derived from a simple sketch, scan or photograph supplied by the user.83 This highlights a growing challenge in design engineering where future users cannot rely on graphical interfaces but must instead be proficient in computer programming to drive automation. Current CAD packages would also require users to spend an unrealistic amount of time to accurately model the multi-scale heterogeneity and complexity of artificial cells, especially if nanofeatures such as lipids, proteins, and nucleic acids were to be included.
It therefore seems likely that any 3D CAD representation of artificial cells will be driven by sophisticated parametric code which can be linked to their manufacturing by yielding a recipe in the form of a series of commands that can either be enacted by the user or, more likely, fed directly to a fluid handling platform that is under full software control.
The need for new CAD methodologies is highlighted by the requirement to standardise as part of an approved platform, and the expectation that artificial tissues will consist of tens of thousands of multiple different types of interconnected artificial cells. Indeed, the Bayley group have previously engaged with this issue by developing their own in-house software that leverages a colour coded system to generate a printing pattern for assembling two different types of artificial cells in 3D.14 This elegant solution could be a significant milestone towards the development of a more comprehensive digital environment for designing artificial tissues, where users can craft multiple different types of artificial cell and produce plans for assembling them in 3D. Further, software of this nature could also be used to suggest designs for microfluidic devices and predict the mechanical stability of the overall 3D assembly (i.e. by highlighting regions that are structurally vulnerable, and providing recommendations for different configurations). If the physiochemical properties of the artificial tissue can be predicted as part of this process, it may even open the door to the development of an in silico model.
6.2 Manufacturing of artificial cells
Considering that manual approaches such as pipetting are unlikely to yield the quantities, sizes, or types of artificial cells required for engineering artificial tissues, it seems safe to assume that a high-throughput process for manufacturing will be at the heart of any future pipeline dedicated to engineering artificial tissues. Indeed, trends in the literature are already steering away from the use of sessile microdroplets and towards the use of microfluidic platforms which could be used for this purpose due to their ability to offer fine fluid handling capabilities at seemingly unrivalled throughput. However, one key drawback is that engineering microfluidic devices can be time consuming and expensive, often requiring the use of dedicated fabrication facilities. Recent efforts have been made to overcome this using rapid prototyping methods, including 3D printing, however the technology has yet to reach a maturity level where it can be used reliably in place of soft lithography, the existing gold-standard.84 The challenges associated with device fabrication are compounded further in the context of creating a biomanufacturing pipeline for engineering artificial tissues by the need to deliver greater functionality than what exists currently. For example, most microfluidic platforms are only able to generate one or two different types of artificial cells, whereas an artificial skin model would be expected to require many more while also offering fine control of membrane compositions and cargos. This might be achieved to some degree using on-chip mixing to control the relative abundance of two or three components present in either the membrane or within the cell interior, however a core of individually addressable droplet generators is still likely to be needed to deliver the number of different subunits required. The emerging use of hydrogels85 in artificial cell and tissue models also points towards the unmet need for platforms to be capable of handling multiple different materials with varying rheological properties. This feature may be important for helping to control the permeability of synthetic tissue models, as well as enhancing their mechanical and temporal stability. Further, it is reasonable to expect that precise on-chip control will be needed to select and switch between different artificial cells and materials used to construct synthetic tissues, and to identify and remove non-functional or erroneous subunits that would otherwise be incorporated as part of the assembly process. There is also a fundamental need for the system to be reliable and repeatable with low breakdown for the purposes of standardisation, while scale-up and uptake are also likely to depend on the degree to which the system can be automated.
Although the engineering challenges are significant, it is promising that many of these capabilities have already been demonstrated in some form. For example, there are already a number of different microfluidic strategies for engineering artificial cells; microfluidic sorting has been well documented using a variety of active or passive means; automated platforms have been reported, and robotic microfluidic systems are on the horizon.86 The task at hand is therefore to develop new and accessible strategies that can bring these capabilities together. Achieving this would pave the way toward the development of embedded lab-on-a-chip devices, particularly if fabrication can be fully digitised and if features can be engineered across multiple interconnected fluidic layers.
6.3 Construction of an on-chip artificial skin model
Although there are several different strategies available for manipulating individual artificial cells in 3D, the scale of assembling tens of thousands of subunits when constructing synthetic tissues is a challenge that must be addressed. If for example, a single drag and drop operation takes 1 s to complete, then the total build time for an assembly of 3 × 104 subunits would take over 8 h. This suggests that indirect deposition methods (i.e. where artificial cells are selected from an array of prepared subunits) would need to be quick enough to complete each individual assembly step in less than 250 ms for the build time to be meaningful. This time constraint is likely to become even more stringent as the size of the assembly grows. One potential solution could be to conduct multiple construction steps simultaneously (e.g. using several optical traps, magnetic fields, or modular assembly processes), otherwise this bottleneck points towards the use of direct deposition methods where the 3D assembly of artificial cells can be directly coupled with their generation. Indeed, Bayley and co-workers reported that the piezo-controlled droplet generators used in their 3D printing approach were used to directly dispense droplets ∼50 μm in diameter with an XY spacing of 50 μm every 200 ms and a 2 s time delay between rows.14 This elegant way of coupling the throughput of the system to the 3D assembly of the artificial tissue directly addresses the issue of build time when fabricating such a large construction, however the platform remains limited to two droplet types that are extruded from two separate nozzles. It is in this sense that a more elaborate microfluidic process holds promise for advancing the technology further, particularly if multiple different types of artificial cells can be directly 3D printed from a single printhead. Combining microfluidics and 3D printing in this way is an emerging concept that has not been widely explored,87 but seems a natural fit for coupling the high-throughput generation of artificial cells with their 3D assembly. Considering that the technology for manipulating build platforms in 3-axis is already well developed, with open-source options available, it appears that the challenge is more focused around precise control over the design, generation and selection of different subunits, which highlights the importance of establishing a robust additive biomanufacturing pipeline.
6.4 Future opportunities and challenges
The development of an additive biomanufacturing pipeline that can construct artificial tissues holds great promise for a myriad of applications beyond the creation of synthetic skin models for dermal absorption screening. For instance, the same process could be used to generate personalised skin disease models, skin grafts, and drug screening platforms. In addition, there is potential to engineer artificial plant models for use in agricultural settings, such as for screening pesticides, biocides, and herbicides, as well as for synthetic food production. Furthermore, the ability to engineer functionalised and interconnected membranes at scale opens up possibilities for constructing electronic devices that could offer a more sustainable alternative to conventional approaches, especially if the assemblies can be easily disassembled and reassembled.
While the development of fluid handling capabilities appears to be the most significant engineering challenge facing the development of this pipeline for these purposes at the present time, overcoming this obstacle will likely expose underlying challenges in design. Indeed, different applications will rely on different design features of the final tissue model, from permeability and metabolism for artificial skin models, to electrical properties and taste/nutritional features for electronic devices and synthetic meat. The complexity of these assemblies coupled with the attraction of tailoring them to individual requirements also opens the door to AI and parametric design, which may also be applied to the development of microfluidic devices as well as artificial cells and tissues.
The regulatory requirements for different products of an additive biomanufacturing pipeline will vary depending on the end-user's location. In the UK, for example, medicines, medical devices, agricultural products, and food substances are all regulated differently from an in vitro dermal absorption platform for screening cosmetics. Furthermore, there are regulatory challenges associated with automated and/or parametric design processes. In some locations, every single iteration of the process will need to be certified by the relevant regulator before it can be placed on the market. These factors are essential considerations for the research community, especially as various applications developed in the same laboratory may lead to different regulatory challenges.
Conclusions
Animal-free methods for screening the dermal absorption of cosmetics are needed to remove the reliance on ex vivo tissues used as part of cosmetic risk assessments, but at the present time there are no approved alternatives. This is largely due to difficulties in reconstituting barrier function and metabolism in vitro, and open questions regarding the relevance of historical animal data, which is often used as a primary source of reference when validating new approaches. In principle, several of these obstacles could be addressed using synthetic tissue models engineered from artificial cells, however this will require capabilities in the design, synthesis, functionalisation and 3D assembly of artificial cells that extends beyond what can be achieved currently using microfluidics and additive manufacturing. Progress in these areas could unlock the potential for constructing an additive biomanufacturing pipeline for engineering a host of different products, including artificial skin models for screening for dermal absorption.
Author contributions
MSF conceived the study. MSF, LJ and CM supervised JGS. MSF, LJ, CM and JGS wrote the manuscript. MF and JGS produced the figures/illustrations.
Conflicts of interest
There are no conflicts to declare.
Acknowledgements
This work was supported by an Imperial College Research Fellowship awarded to MSF.
References
- The Product Safety and Metrology etc. (Amendment etc.) (EU Exit) Regulations 2019, Statute Law Database.
-
https://webarchive.nationalarchives.gov.uk/eu-exit/, https://eur-lex.europa.eu/legal-content/EN/TXT/?uri=CELEX:02009R1223-20201203.
- R. J. Silva and S. Tamburic, Cosmetics, 2022, 9, 90 CrossRef CAS.
-
Guideline No. 497: Defined Approaches on Skin Sensitisation, OECD, 2021 Search PubMed.
-
Test No. 467: Defined Approaches for Serious Eye Damage and Eye Irritation, OECD, 2022 Search PubMed.
-
Test No. 442E: In Vitro Skin Sensitisation, OECD, 2022 Search PubMed.
- H. Johansson, R. Gradin, A. Johansson, E. Adriaens, A. Edwards, V. Zuckerstätter, A. Jerre, F. Burleson, H. Gehrke and E. L. Roggen, Toxicol. Sci., 2019, 170, 374–381 CrossRef CAS PubMed.
- R. Gradin, A. Forreryd, U. Mattson, A. Jerre and H. Johansson, Sci. Rep., 2021, 11, 18904 CrossRef CAS PubMed.
-
Test No. 428: Skin Absorption: In Vitro Method, OECD, 2004 Search PubMed.
- T. Trantidou, M. S. Friddin, A. Salehi-Reyhani, O. Ces and Y. Elani, Lab Chip, 2018, 18, 2488–2509 RSC.
-
T. Yamada, H. Sugiura, H. Mimura, K. Kamiya, T. Osaki and S. Takeuchi, Proceedings of the IEEE International Conference on Micro Electro Mechanical Systems (MEMS), 2020, 92–93, DOI:10.1126/sciadv.abd2013.
- S. Haylock, M. S. Friddin, J. W. Hindley, E. Rodriguez, K. Charalambous, P. J. Booth, L. M. C. Barter and O. Ces, Commun. Chem., 2020, 3, 77 CrossRef CAS PubMed.
- R. Strutt, J. W. Hindley, J. Gregg, P. J. Booth, J. D. Harling, R. V. Law, M. S. Friddin and O. Ces, Chem. Sci., 2021, 12, 2138–2145 RSC.
- G. Villar, A. D. Graham and H. Bayley, Science, 2013, 340, 48–52 CrossRef CAS PubMed.
-
F. Rodrigues and M. B. P. P. Oliveira, Concepts and Models for Drug Permeability Studies: Cell and Tissue based In Vitro Culture Models, 2016, pp. 155–167 Search PubMed.
- J. A. Bouwstra, P. L. Honeywell-Nguyen, G. S. Gooris and M. Ponec, Prog. Lipid Res., 2003, 42, 1–36 CrossRef CAS PubMed.
- Y. Zhang, M. E. Lane, J. Hadgraft, M. Heinrich, T. Chen, G. Lian and B. Sinko, Int. J. Pharm., 2019, 556, 142–149 CrossRef CAS PubMed.
- Dermal absorption, https://apps.who.int/iris/handle/10665/43542, (accessed 4 January 2023).
- G. S. Gooris and J. A. Bouwstra, Biophys. J., 2007, 92, 2785–2795 CrossRef CAS.
- E. Abd, S. A. Yousef, M. N. Pastore, K. Telaprolu, Y. H. Mohammed, S. Namjoshi, J. E. Grice and M. S. Roberts, Clin. Pharmacol.: Adv. Appl., 2016, 8, 163–176 CAS.
- H. Todo, Pharmaceutics, 2017, 9, 33 CrossRef PubMed.
- M. Alberti, Y. Dancik, G. Sriram, B. Wu, Y. L. Teo, Z. Feng, M. Bigliardi-Qi, R. G. Wu, Z. P. Wang and P. L. Bigliardi, Lab Chip, 2017, 17, 1625–1634 RSC.
- B. Sinkó, T. M. Garrigues, G. T. Balogh, Z. K. Nagy, O. Tsinman, A. Avdeef and K. Takács-Novák, Eur. J. Pharm. Sci., 2012, 45, 698–707 CrossRef PubMed.
- G. Ottaviani, S. Martel and P.-A. Carrupt, J. Med. Chem., 2006, 49(13), 3948–3954 CrossRef CAS PubMed.
- A. Olejnik, J. A. Semba, A. Kulpa, A. Dańczak-Pazdrowska, J. D. Rybka and J. Gornowicz-Porowska, ACS Synth. Biol., 2022, 11, 26–38 CrossRef CAS PubMed.
- E. Sutterby, P. Thurgood, S. Baratchi, K. Khoshmanesh, E. Pirogova, E. Sutterby, P. Thurgood, K. Khoshmanesh, E. Pirogova and S. Baratchi, Small, 2020, 16, 2002515 CrossRef CAS PubMed.
- T. Moniz, S. A. Costa Lima and S. Reis, Br. J. Pharmacol., 2020, 177, 4314–4329 CrossRef CAS PubMed.
- D. Huh, B. D. Matthews, A. Mammoto, M. Montoya-Zavala, H. Y. Hsin and D. E. Ingber, Science, 2010, 328, 1662–1668 CrossRef CAS PubMed.
- H. J. Kim, D. Huh, G. Hamilton and D. E. Ingber, Lab Chip, 2012, 12, 2165–2174 RSC.
- S. Jeong, J. Kim, H. M. Jeon, K. Kim and G. Y. Sung, Int. J. Mol. Sci., 2021, 22, 12788 CrossRef CAS PubMed.
- D. E. Ingber, Nat. Rev. Genet., 2022, 23, 467–491 CrossRef CAS PubMed.
- A. T. O'Neill, N. A. Monteiro-Riviere and G. M. Walker, Cytotechnology, 2008, 56, 197–207 CrossRef PubMed.
- N. Jusoh, J. Ko and N. L. Jeon, APL Bioeng., 2019, 3, 36101 CrossRef PubMed.
- M. Wufuer, G. H. Lee, W. Hur, B. Jeon, B. J. Kim, T. H. Choi and S. H. Lee, Sci. Rep., 2016, 6, 37471 CrossRef CAS PubMed.
- C. Das, M. G. Noro and P. D. Olmsted, Phys. Rev. Lett., 2013, 111, 148101 CrossRef PubMed.
- P. M. Elias, J. Invest. Dermatol., 2012, 132, 2131–2133 CrossRef CAS PubMed.
- A. S. Tascini, M. G. Noro, J. M. Seddon, R. Chen and F. Bresme, Phys. Chem. Chem. Phys., 2019, 21, 1471–1477 RSC.
- M. Ponec, E. Boelsma, A. Weerheim, A. Mulder, J. Bouwstra and M. Mommaas, Int. J. Pharm., 2000, 203, 211–225 CrossRef CAS PubMed.
- J. A. Bouwstra, G. S. Gooris, F. E. R. Dubbelaar and M. Ponec, J. Lipid Res., 2001, 42, 1759–1770 CrossRef CAS.
- J. A. Bouwstra, G. S. Gooris, K. Cheng, A. Weerheim, W. Bras and M. Ponec, J. Lipid Res., 1996, 37, 999–1011 CrossRef CAS.
- G. Sriram, M. Alberti, Y. Dancik, B. Wu, R. Wu, Z. Feng, S. Ramasamy, P. L. Bigliardi, M. Bigliardi-Qi and Z. Wang, Mater. Today, 2018, 21, 326–340 CrossRef CAS.
- N. Mori, Y. Morimoto and S. Takeuchi, Biomaterials, 2017, 116, 48–56 CrossRef CAS PubMed.
- T. Schmitt and R. H. H. Neubert, Chem. Phys. Lipids, 2018, 216, 91–103 CrossRef CAS PubMed.
- S. M. Pyo and H. I. Maibach, Skin Pharmacol. Physiol., 2019, 4, 283–294 CrossRef PubMed.
- L. L. Pham, T. Y. Sheffield, P. Pradeep, J. Brown, D. E. Haggard, J. Wambaugh, R. S. Judson and K. P. Friedman, Curr. Opin. Toxicol., 2019, 15, 40–47 CrossRef.
- M. Dent, R. T. Amaral, P. A. Da Silva, J. Ansell, F. Boisleve, M. Hatao, A. Hirose, Y. Kasai, P. Kern, R. Kreiling, S. Milstein, B. Montemayor, J. Oliveira, A. Richarz, R. Taalman, E. Vaillancourt, R. Verma, N. V. O. R. C. Posada, C. Weiss and H. Kojima, Comput. Toxicol., 2018, 7, 20–26 CrossRef.
- A. J. van der Zalm, J. Barroso, P. Browne, W. Casey, J. Gordon, T. R. Henry, N. C. Kleinstreuer, A. B. Lowit, M. Perron and A. J. Clippinger, Arch. Toxicol., 2022, 96, 2865–2879 CrossRef CAS PubMed.
- T. Trantidou, M. Friddin, Y. Elani, N. J. Brooks, R. V. Law, J. M. Seddon and O. Ces, ACS Nano, 2017, 11, 6549–6565 CrossRef CAS PubMed.
- N. E. Barlow, G. Bolognesi, S. Haylock, A. J. Flemming, N. J. Brooks, L. M. C. Barter and O. Ces, Sci. Rep., 2017, 7, 17551 CrossRef PubMed.
- P. Marius, M. R. R. de Planque and P. T. F. Williamson, Biochim. Biophys. Acta, Biomembr., 2012, 1818, 90–96 CrossRef CAS PubMed.
- K. Karamdad, J. W. Hindley, G. Bolognesi, M. S. Friddin, R. V. Law, N. J. Brooks, O. Ces and Y. Elani, Chem. Sci., 2018, 9, 4851–4858 RSC.
- M. S. Friddin, G. Bolognesi, A. Salehi-Reyhani, O. Ces and Y. Elani, Commun. Chem., 2019, 2, 1–7 CrossRef.
- J. S. H. Danial, B. Cronin, C. Mallik and M. I. Walllace, Soft Matter, 2017, 13, 1788–1793 RSC.
- W. L. Hwang, M. Chen, B. Cronin, M. A. Holden and H. Bayley, J. Am. Chem. Soc., 2008, 130, 5878–5879 CrossRef CAS PubMed.
- G. Taylor, M. A. Nguyen, S. Koner, E. Freeman, C. P. Collier and S. A. Sarles, Biochim. Biophys. Acta, Biomembr., 2019, 1861, 335–343 CrossRef CAS PubMed.
- N. E. Barlow, E. Smpokou, M. S. Friddin, R. Macey, I. R. Gould, C. Turnbull, A. J. Flemming, N. J. Brooks, O. Ces and L. M. C. Barter, Biomicrofluidics, 2017, 11, 024107 CrossRef CAS PubMed.
- J. L. Korner, E. B. Stephenson and K. S. Elvira, Lab Chip, 2020, 20, 1898–1906 RSC.
- J. L. Korner and K. S. Elvira, Soft Matter, 2021, 17, 8891–8901 RSC.
- G. Villar, A. J. Heron and H. Bayley, Nat. Nanotechnol., 2011, 6, 803–808 CrossRef CAS PubMed.
- I. Cazimoglu, M. J. Booth and H. Bayley, ACS Nano, 2021, 15, 20214–20224 CrossRef CAS PubMed.
- Y. Elani, R. V. Law and O. Ces, Nat. Commun., 2014, 5, 5305 CrossRef CAS PubMed.
- V. Noireaux and A. Libchaber, Proc. Natl. Acad. Sci. U. S. A., 2004, 101, 17669–17674 CrossRef CAS PubMed.
- Y. Shimizu, A. Inoue, Y. Tomari, T. Suzuki, T. Yokogawa, K. Nishikawa and T. Ueda, Nat. Biotechnol., 2001, 19, 751–755 CrossRef CAS PubMed.
- R. Syeda, M. A. Holden, W. L. Hwang and H. Bayley, J. Am. Chem. Soc., 2008, 130, 15543–15548 CrossRef CAS PubMed.
- M. J. Booth, V. R. Schild, A. D. Graham, S. N. Olof and H. Bayley, Sci. Adv., 2016, 2, e1600056 CrossRef PubMed.
- M. J. Booth, V. Restrepo Schild, S. J. Box and H. Bayley, Sci. Rep., 2017, 7, 9315 CrossRef PubMed.
- G. Bolognesi, M. S. Friddin, A. Salehi-Reyhani, N. E. Barlow, N. J. Brooks, O. Ces and Y. Elani, Nat. Commun., 2018, 9, 1882 CrossRef PubMed.
- M. A. Holden, D. Needham and H. Bayley, J. Am. Chem. Soc., 2007, 129, 8650–8655 CrossRef CAS PubMed.
- S. Aghdaei, M. E. Sandison, M. Zagnoni, N. G. Green and H. Morgan, Lab Chip, 2008, 8, 1617–1620 RSC.
- C. E. Stanley, K. S. Elvira, X. Z. Niu, A. D. Gee, O. Ces, J. B. Edel and A. J. Demello, Chem. Commun., 2010, 46, 1620–1622 RSC.
- Y. Elani, A. J. Demello, X. Niu and O. Ces, Lab Chip, 2012, 12, 3514–3520 RSC.
- B. Schlicht and M. Zagnoni, Sci. Rep., 2015, 5, 9951 CrossRef CAS PubMed.
- P. H. King, G. Jones, H. Morgan, M. R. R. de Planque and K. P. Zauner, Lab Chip, 2014, 14, 722–729 RSC.
- P. Carreras, Y. Elani, R. V. Law, N. J. Brooks, J. M. Seddon and O. Ces, Biomicrofluidics, 2015, 9, 064121 CrossRef CAS PubMed.
- D. K. Baxani, A. J. L. Morgan, W. D. Jamieson, C. J. Allender, D. A. Barrow and O. K. Castell, Angew. Chem., Int. Ed., 2016, 55, 14240–14245 CrossRef CAS PubMed.
- T. Wauer, H. Gerlach, S. Mantri, J. Hill, H. Bayley and K. T. Sapra, ACS Nano, 2013, 8, 771–779 CrossRef PubMed.
- M. S. Friddin, G. Bolognesi, Y. Elani, N. J. Brooks, R. V. Law, J. M. Seddon, M. A. A. Neil and O. Ces, Soft Matter, 2016, 12, 7731–7734 RSC.
- E. J. Challita, J. S. Najem, R. Monroe, D. J. Leo and E. C. Freeman, Sci. Rep., 2018, 8, 6494 CrossRef PubMed.
- S. Bachler, D. Haidas, M. Ort, T. A. Duncombe and P. S. Dittrich, Commun. Biol., 2020, 3, 769 CrossRef CAS PubMed.
- M. M. Makhoul-Mansour and E. C. Freeman, Langmuir, 2021, 37, 3231–3247 CrossRef CAS PubMed.
- T. Trantidou, M. S. Friddin, K. B. Gan, L. Han, G. Bolognesi, N. J. Brooks and O. Ces, Anal. Chem., 2018, 90, 13915–13921 CrossRef CAS PubMed.
- B. R. Hunde and A. D. Woldeyohannes, Results Eng., 2022, 14, 100478 CrossRef.
- T. A. Burge, J. R. T. Jeffers and C. W. Myant, Sci. Rep., 2023, 13, 3317 CrossRef CAS PubMed.
- M. S. Friddin, Y. Elani, T. Trantidou and O. Ces, Anal. Chem., 2019, 91, 4921–4928 CrossRef CAS PubMed.
- M. E. Allen, J. W. Hindley, D. K. Baxani, O. Ces and Y. Elani, Nat. Rev. Chem., 2022, 6, 562–578 CrossRef CAS PubMed.
- J. Zhong, J. Riordon, T. C. Wu, H. Edwards, A. R. Wheeler, K. Pardee, A. Aspuru-Guzik and D. Sinton, Lab Chip, 2020, 20, 709–716 RSC.
- C. Richard, C. Richard, A. Neild, V. J. Cadarso and V. J. Cadarso, Lab Chip, 2020, 20, 2044–2056 RSC.
|
This journal is © The Royal Society of Chemistry 2023 |
Click here to see how this site uses Cookies. View our privacy policy here.