DOI:
10.1039/D2MH01482C
(Communication)
Mater. Horiz., 2023,
10, 1264-1273
Dynamic competitive strains enabled self-supporting Janus nanostructured films for high-performance airflow perception†
Received
2nd December 2022
, Accepted 6th February 2023
First published on 7th February 2023
Abstract
Recently, piezoresistive airflow sensing systems have shown extensive potential applications in aerospace, weather forecasting, mineral enterprises, and wearable electronics. However, the achievement of both an ultralow detection limit and broad monitoring range still remains challenging. Here, we propose a self-supported Janus film based on a graphene/carbon sphere-elastomer hybrid, which allows us to sensitively and efficiently perceive tiny and strong airflows via responding with opposite current variations enabled by the dynamic competition of transverse and longitudinal strains. The achieved film enables an ultralow detection limit of ∼0.0087 m s−1, a wide detection range of 0.0087–23 m s−1, favorable response speed as fast as ∼0.1 s, and signal stability for 1150 cycles. Furthermore, an artificial smart spiderweb array system is delicately designed to efficiently distinguish the position and intensity of the applied airflow for efficient non-contact manipulation, enabling significant potential in the development of advanced soft electronics and smart biomimetic systems.
New concepts
Piezoresistive airflow sensors have aroused extensive research interest due to their simple configuration, readable signals, and high sensitivity. Efforts have been made to construct delicate structures with diverse materials to lower the detection limits and/or extend the sensing range for airflow sensors. However, there is a significant trade-off between ultralow detection limit and ultrawide sensing performance, as the electrical contact between active materials tends to saturate during airflow loading. Therefore, it is urgent to develop an efficient and alternative approach to balance these two performances. Inspired by the airflow perception of natural spiderwebs, we have recently demonstrated a highly sensitive airflow sensor based on graphene/carbon spheres-Ecoflex Janus film. The combination of hybrid carbon nanomaterials and self-supporting structures endows the airflow sensors with both an ultralow detection limit (∼0.0087 m s−1) and an ultrawide sensing range (up to 23 m s−1), which is attributed to the dynamic competition between transverse and longitudinal strains. Benefiting from the exceptional airflow-responsiveness, an artificial smart spiderweb array is designed to monitor airflow velocity and direction in real time for efficient non-contact manipulation. This opens up a novel avenue for developing high-performance airflow sensor systems for applications in smart biomimetic systems and highly-efficient human–machine interaction.
|
Introduction
Flexible airflow sensor systems based on mechanical deformation principles (e.g., piezoresistive,1–3 piezoelectric,4 and capacitive5 mechanisms) have been actively researched due to their miniaturization, simplicity, and mild operation conditions, demonstrating significant potential in aerospace,6 weather forecasting,7 small unmanned aerial vehicles,8 biomedical engineering,9 mineral exploration,10 and wearable devices.11,12 Among them, piezoresistive airflow sensors have aroused great research interest due to their typical advantages of facile fabrication, easy signal acquisition, and high sensitivity. Nowadays, diverse materials and structure designs have been developed to achieve low detection limits and/or wide detection ranges for piezoresistive airflow sensors. For example, Zhang et al. developed a fabric-based airflow sensor composed of in situ grown fluffy-like carbon nanotubes (CNTs), which enabled a detection limit of 0.05 m s−1.13 More recently, a suspended CNT network was proposed with the combination of ultralong CNTs and short CNTs for reaching a wide detection range from 0.11 to 5.51 m s−1.14 Although these piezoresistive airflow sensors consisting of micro/nanoscale conductive materials could respond to weak airflow stimuli through microscopic deformation, they often failed to achieve a wide range of airflow detection due to the phenomenon of easily saturated electrical contact sites. Conversely, Fu et al. designed an electrical hair sensor composed of nylon fibers and piezo-resistive carbonized papers, in which the macroscopic deformation of fibers compressed the adjacent carbonized paper, endowing the sensor with an airflow detection capability of up to 43.7 m s−1 but the detection limit was as high as 11.5 m s−1.2 Hence, despite the important advances achieved, challenges still exist in the realization of both ultralow detection limit and wide detection range in a simple and efficient way. In particular, it is noted that there is a remarkable trade-off in sensing performance between detection limit and range. Therefore, it is highly desirable to develop soft airflow sensors with the combined performance of ultralow limit and wider range.
Nature has given us endless inspiration for bionics. It is well known that spiders can sensitively perceive vibration differences transmitted by the spider web to accurately orient their preys.15 In general, the radial threads, featuring excellent elasticity and stretchability, are responsible for generating and transmitting vibrations.16–18 More interestingly, recent research has reported that the radial lines can also capture vibrations caused by weak or violent airflow generated by insects flapping their wings or large predators running and further transmit the information of the surroundings to the spider in a non-contact manner, which resembles outsourcing hearing to inform spiders in advance of potential prey or predators in their vicinity, thus improving survival (Fig. 1a).16,19,20
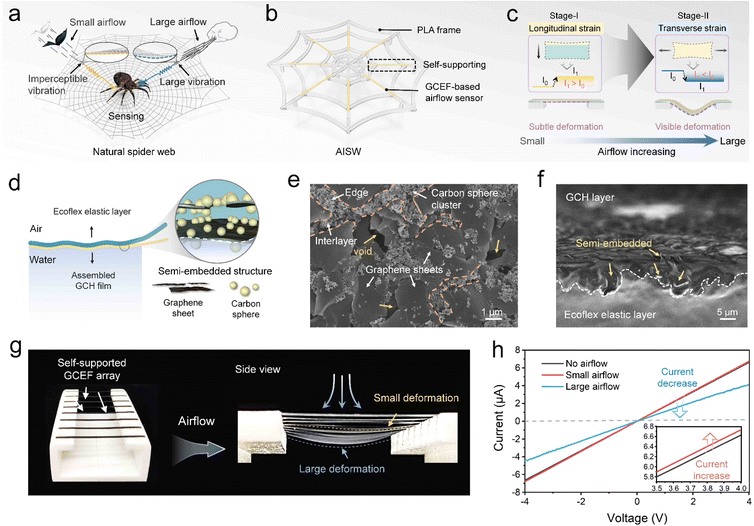 |
| Fig. 1 The construction and characterization of the AISW based on GCEF with an interfacial strategy. (a) Schematic of a spider perceiving its surroundings by distinguishing vibrations captured and transmitted by the radial threads of the spiderweb. Diagram of the structure of the AISW (b) and the two-stage current behaviors and dynamic strain competition under the action of a small and large airflow (c). (d) Schematic of the structure of GCEF fabricated at the water/air interface. (e) Surface morphology of the GCH film. (f) The cross-sectional SEM image of the GCEF. (g) Picture of the deformation behavior of the zither-like airflow sensor array under airflow blowing. (h) The I–V curves of the individual sensor under the action of airflow of different intensities. Inset: The partially magnified I–V curve at small airflow. | |
With inspiration drawn from the capability of spiderwebs that respond mechanically to small and large airflows, here, a biomimetic smart spiderweb enabled by a self-supported elastic and conductive thin film array was rationally designed (Fig. 1b). Typically, the graphene/carbon sphere-Ecoflex elastic film (GCEF) was employed to construct the artificial radial line, which could sensitively capture the airflow, generate vibration, and respond in the form of an electrical signal resulting from the self-supported configuration-induced competitive transverse and longitudinal strains. As a result, the achieved GCEF-based airflow sensor had an ultralow airflow detection limit (∼0.0087 m s−1), ultrawide sensing range of up to 23 m s−1, fast response time of ∼0.1 s, and stability for 1150 cycles. As a proof-of-concept, an artificial intelligent spiderweb (AISW) array system with eight individual sensors was developed to precisely distinguish the intensity, direction, and location of the applied airflow for active warning and non-contact control. This work is expected to provide an alternative approach for achieving both ultralow detection limit and wide sensing range of airflow sensors, demonstrating significant potential in soft electronics and smart biomimetic systems.
Results and discussion
Construction and characterization of the AISW
For the realization of both an ultralow detection limit and a broad range, the self-supported thin GCEF enables a two-stage working mechanism. As illustrated in Fig. 1c, when the weak airflow below the threshold was applied on the GCEF, the stripe displayed subtle deformation with predominantly longitudinal strain, resulting in a positive variation tendency of current at stage-I. As the velocity increased above the threshold, an obvious deformation dominated by the transverse strain appeared, leading to a decrease in current at stage-II. This specific sensing performance of GCEF was achieved by an asymmetric integration of the assembled graphene/carbon sphere hybrid (GCH) film and Ecoflex elastomer at the air/water interface (Fig. 1d and Fig. S1, ESI†).21–24 Note that the alternative combination of two-dimensional graphene and zero-dimensional carbon spheres for the assembled film could result in a relatively loosely packed surface morphology with randomly distributed voids, which may be derived from the fact that the carbon spheres clustered at the edge and interlayer of the graphene nanosheets (Fig. 1e and Fig. S2, ESI†). More importantly, this hybrid structure would provide a higher resistance change for stage-I as the cross-sectional microstructure directly indicated that the support of the interlayered carbon spheres could confer more space in the longitudinal direction to the graphene sheets, thus drastically changing the electrical contact area under airflow stimulation (Fig. S3, ESI†). When the self-assembled GCH film was stabilized at the water/air interface, it was partially wetted by water. Owing to the incompatibility of Ecoflex solution and water, the GCEF with asymmetric structures was formed (Fig. 1d and Fig. S4, ESI†). The cross-sectional scanning electronic microscopy (SEM) images clearly illustrated that the GCH layer was partially embedded into the Ecoflex matrix (Fig. 1f) with an entire thickness of ∼105 μm (Fig. S5, ESI†). It is noted that the existing interlocked interfacial structure between the GCH layer and Ecoflex can enable good mechanical stability to endure cyclic airflow stimuli (Fig. S6, ESI†). Furthermore, the elemental distribution along the thickness direction showed that the contents of C and Si elements at the interface experienced a sharp increase and decrease process, individually (Fig. S7, ESI†), which indicated that the GCH region was partially covered by a thin layer of elastomer, providing the favorable conductivity for the GCH side. In addition, the Raman spectrum was characterized in Fig. S8 (ESI†), which demonstrated that the GCH side exhibited typical characteristic peaks of pure GCH and Ecoflex film.
Since the GCEF was fabricated at the air/water interface, it could be easily and intactly transferred onto a polyethylene terephthalate (PET) substrate, which was further tailored into targeted stripes. Following a second-transferring procedure, the GCEF stripes were combined with a hollow polylactic acid (PLA) frame for a self-supported airflow sensor array system (Fig. S9, ESI†). As displayed in Fig. 1g, a zither-like airflow sensor array consisting of eight parallel GCEF stripes was constructed, which exhibited obvious deformation differences at different positions. Moreover, the current–voltage (I–V) characteristic curves in Fig. 1h showed distinctly linear response behaviors, illustrating that ohmic conduction dominated the electron transport.25 More importantly, when exposed to airflow with small and large velocities, the GCEF could experience a current increase and decrease tendency, respectively. Therefore, it is essential to balance these two stages to achieve a wide range of airflow sensing.
Competitive deformation behaviors of the GCEF-based airflow sensors
The mechanical deformation characteristics of the GCEF stripe were investigated in Fig. 2. The airflow was vertically applied on the middle of the GCEF stripe about 5 mm above the GCEF surface (Fig. S10, ESI†). As a result, the middle position experienced the largest vertical displacement, which was defined as Δd to represent the largest deformation degree (Fig. 2a). As shown in Fig. 2b, the deformation of the GCEF increased with airflow velocity, which was highly consistent with the finite element simulation results (Fig. 2c). The quantitative correlation between Δd and airflow velocity in Fig. 2d demonstrated the linear positive correlation with a high overlap, indicating that the finite element simulation could be used to predict vertical displacements at an extremely weak airflow. For example, Δd was only 1.5 μm when the flow rate was as low as 0.0087 m s−1, in which the displacement was not easily and accurately measured by experiments. This means that the GCEF stripe deforms in the vertical direction once airflow is applied. To further understand the deformation degree of the GCEF, the displacement of the upper and lower surfaces in the middle section was studied (Fig. 2e). As displayed in Fig. 2f, the upper surface deformed more in the vertical direction, which caused the upper surface to be compressed vertically compared to the lower surface. Therefore, the downward bending not only stretched the GCEF laterally but also compressed it longitudinally. We also calculated the transverse and longitudinal strains according to the Poisson's ratio equation and simulation results (Fig. S11 and Table S1, ESI†). As depicted in Fig. 2g, the transverse and longitudinal strains increased with the airflow velocity. Typically, both the transverse and longitudinal strains induced the GCEF to be stretched in the transverse direction and compressed in the longitudinal one, respectively. Therefore, the vertical deformation resulted in the competition between the transverse and longitudinal strains during the whole airflow loading process (Fig. 2h).
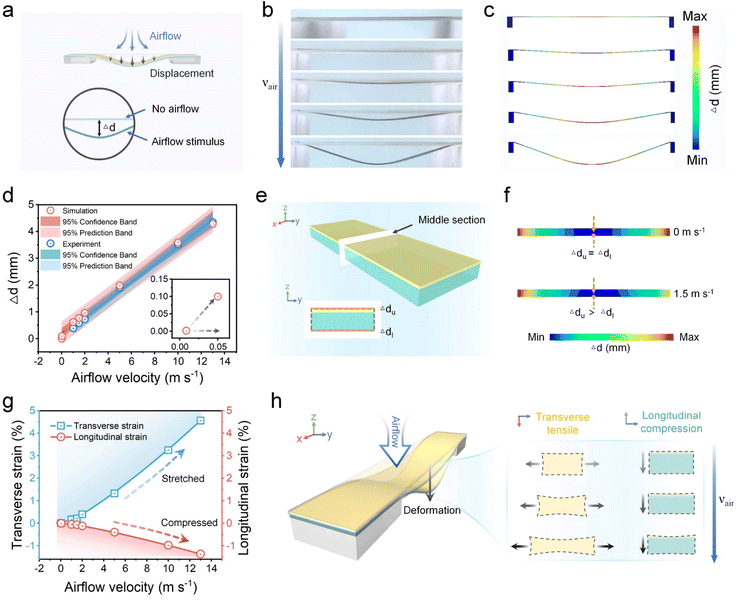 |
| Fig. 2 Deformation behavior characterization of the self-supported GCEF under the action of airflow. (a) Schematic of different degrees of vertical deformation for the GCEF stripe under airflow action and the definition of the vertical displacement. Specific deformation behavior of the stripe in experiments (b) and finite element simulation (c) with increasing flow rates of 0.0087, 1, 2, 5, and 13 m s−1. (d) The relationship between the vertical displacement (Δd) and airflow velocity of the experimental and simulated results. Inset: Obvious deformation occurs at the extremely weak airflow in the simulation result. (e) Schematic of the position of the middle section and the defined displacement of the upper (Δdu) and lower (Δdl) surfaces. (f) Simulation result of the vertical displacement in the middle section at the airflow velocity of 1.5 m s−1. (g) The transverse and longitudinal strains versus the airflow velocity curves. (h) Schematic illustration of the competitive strain behaviors in the transverse and longitudinal directions as airflow increases. | |
Opposite current response behaviors of the GCEF-based airflow sensors
Significantly, the vertical deformation induced three types of current curves (Fig. 3a). The curve of type-i first occurred at the small airflow range (e.g., 0.0087, 0.05, and 1 m s−1), in which the current increased with airflow velocity. When the airflow velocity reached the intermediate range (e.g., 2 and 2.5 m s−1), it was found that the current presented a characteristic of first decreasing and subsequently increasing in the curve of type-ii. Further increasing the velocity (e.g., 5, 10, and 13 m s−1) could result in a negative correlation between current change and velocity in the curve of type-iii. The maximum current variation in Fig. 3b intuitively indicated that there was competition between the current with positive and negative variations, with the end result being that the increased current gradually disappeared, while the decreased current became stronger and stronger. In particular, we tested the response performance of the GCEF-based airflow sensor with the constraint of the transverse strain (Fig. S12a, ESI†). This result showed that the supported airflow sensor has a more sensitive positive current variation and a wider response range for stage-I (Fig. S12b, ESI†), indicating that there is competition between transverse and longitudinal strains whenever the airflow was loaded in the self-supporting configuration, in which the longitudinal compression and transverse stretching increased and decreased the interlayer and horizontal electrical contacts in the hybrid sensing structure, respectively, resulting in two opposite trends of current variation (Fig. 3c). The specific competing sensing mechanisms are explained in detail in Fig. 3d. Specifically, in the stage for type-i curve, the longitudinal strain dominated the variation of the total resistance based on the “microspring effect”,26 increasing the interlayered contact area in the vertical direction. The transverse strain gradually increased when the airflow velocity reached the stage with type-ii curve, which facilitated the partial sliding of the graphene sheets to reduce the transverse electron flow. However, the longitudinal compression continued to increase the interlayered electrical contact, allowing the most intense competition of the current at this stage. Finally, in the final stage of type-iii curve, the electrical contacts in the longitudinal direction were almost saturated. The large airflow impact caused the considerable separation of the graphene sheets and the carbon spheres at the edges owing to the dominant transverse strain, resulting in the sharp blockage of electron flow, which is similar to the sensing behavior of the ordinary resistive-type strain sensors.27–29
 |
| Fig. 3 The characterization of the current response behavior. (a) Three types of current curves corresponding to different airflow velocities. (b) The trend of maximum current variation with the increase of airflow. (c) Schematic of the microscopic mechanism responsible for the increase and decrease of current. (d) Macroscopic and microscopic behaviors/mechanisms corresponding to the three types of current curves. | |
Airflow sensing performance characterization of the GCEF-based airflow sensors
The normalized current versus airflow velocity curve is displayed in Fig. S13 (ESI†), which is divided into three stages corresponding to the three types of current curves discussed above. However, the double solution phenomenon with opposite current response resulting from the conflicting sensing mechanisms of the competitive strains in the longitudinal and transverse directions exists in the stages with type-i and type-ii curves, which may lead to a non-monotonic response behavior.30 Therefore, a compromise was made on the competition to avoid this contradiction. The updated normalized current curve with only two stages is displayed in Fig. 4a. One of them still corresponded to the curve of type-i, while the other included curves of type-ii and iii. Here, the classification for stage-II is based on the feature of the remarkable valley in the current curve. In this way, the normalized current maintained monotonic variation in both stages without the double solution problem. The sensitivity (S) of the airflow sensor can be calculated from the slope of the curve, which is defined by the following formula (1): |  | (1) |
where ΔI = I − I0, and I0 and I represent the initial current and the current value at a given airflow velocity (ν), respectively. As shown in Fig. S14 (ESI†), the sensitivity declined from 44.59% s m−1 to 0.82% s m−1 in stage-I (0.0087–1.5 m s−1), which resulted from the saturation of the interlayer contact points. In stage-II, the relative current variation was almost linear with the sensitivity of −4.3% s m−1 in the range of 2–13 m s−1.
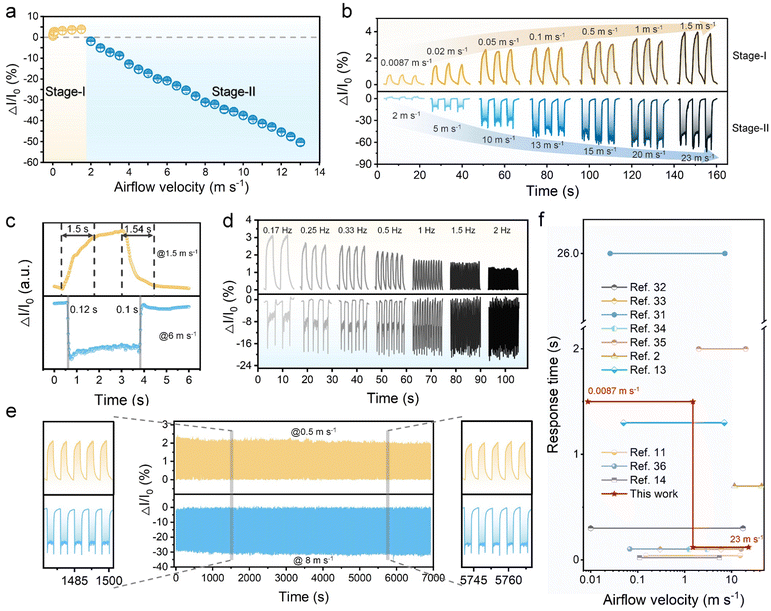 |
| Fig. 4 Airflow sensing performance characterization. (a) The normalized current versus airflow velocity curve with a two-stage current variation trend (test number, n = 3). (b) Cyclic switching characteristics of the self-supported airflow sensor in stage-I and II. (c) The response and recovery times in stage-I and stage-II. (d) Frequency response behaviors at 1 m s−1 and 6 m s−1, respectively. (e) The relative current variation under 1150 airflow on–off cycles in stages-I and II, respectively. (f) Comparison of the detectable airflow velocity range and response time between our GCEF-based airflow sensor and other reported airflow sensors based on various sensing principles in ref. 2, 11, 13, 14 and 31–36. | |
In addition, we also investigated the effects of the concentration and the hybridization ratio in the GCH layer on the sensing performance (Fig. S15, ESI†). As shown in Fig. S15a (ESI†), the optimal performance was observed when the concentration of GCH was 1 mg mL−1, which was due to the fact that either too low or too high concentrations resulted in less variation in the longitudinal contact sites. For stage-II, higher concentrations lead to a more dense stacking of the GCH layer, resulting in more difficult transverse separation of the conductive pathways. By optimizing the mass ratio of graphene and carbon spheres, the airflow sensor exhibited the optimum signal response to small airflow at a hybrid ratio of 1
:
1. When the concentration of graphene in the hybrid system is relatively low, excessive carbon spheres occupy the surface and interlayer of the graphene sheets, leading to oversaturated contacts in the longitudinal direction in the absence of airflow action. With the increase of the concentration of graphene to a much higher value, only a few carbon spheres exist in the interlayers, resulting in limited contact under airflow stimulation. However, in stage-II, the response sensitivity experienced an improvement with the increase of the graphene concentration in the hybrid, which was ascribed to the increase in the variation of transverse contact resistance due to the decrease of the carbon spheres intercalated inside the graphene nanosheets with the increase of the graphene concentration (Fig. S15b, ESI†). Finally, after the comprehensive balance of the sensing performance in the two stages, the GCH layer with the concentration of 1 mg mL−1 and the hybridization ratio of 1
:
1 was adopted for the subsequent experiments unless otherwise stated. Furthermore, we investigated the effect of the hybrid composition with the alternative combination of different dimensional materials on the airflow response performance. As shown in Fig. S16 (ESI†), when there are no graphene nanosheets added in the sensing layer, the obtained airflow sensor exhibits remarkably weakened response performance in both phases, especially for the detection of small airflow. Once graphene nanosheets were introduced in the hybrid system, the airflow sensor responded sensitively and exhibited three types of curve characteristics under both small and large airflow stimuli. The above results suggest that two-dimensional (2D) graphene nanosheets play a crucial role in the hybrid system, and they can be used as the basic components to build a laminar stacking structure, while the introduced zero-dimensional (0D) carbon spheres or one-dimensional (1D) carbon tubes can be inserted between the nanosheets to increase the longitudinal electrical contact sites without overly hindering the lateral separation of the graphene sheets, thus ensuring a sensitive response under different levels of airflow. Therefore, this hybrid of coupling 2D sheet structures and 0D or 1D nanostructures is expected to be a general strategy in combination with a self-supporting design to achieve high-performance airflow sensors, enabling both extremely low detection limits and wide sensing ranges. Compared with the effect of the carbon layer composition and structure, the thickness of the hybrid film can also influence the airflow response performance. It is worth noting that the performance in stage-II is strongly affected by the film thickness, as the macroscopic deformation ability of the film depends on the variation of the intrinsic bending stiffness, which is highly related to the film thickness. Since the hybrid film was fabricated asymmetrically at the water/air interface, the structure of the carbon-based sensing layer was not remarkably affected by the thickness of the elastomer layer. As a result, the sensing performance in stage-I hardly changes with the film thickness. As shown in Fig. S17, (ESI†), the normalized current variation in stage-II significantly decreased with the increase of the thickness (∼150 μm). Moreover, the increase of the film thickness may lead to an increase of the threshold flow rate for the appearance of negative current changes and prolong the transition phase of the type-ii current curve.
The self-supported airflow sensor was capable of detecting airflow in the range from 0.0087 to 23 m s−1 with excellent airflow cycling response performance in stages-I and II (Fig. 4b). The GCEF-based sensor responded and recovered quickly in both stages (Fig. 4c). In particular, the response and recovery times were only about 0.1 s at large airflows, which facilitates its application in smart electronics to achieve accurate and rapid signal acquisition. In addition, the response behavior of the GCEF-based sensor at different frequencies was tested to imitate the frequency-dependence of the natural spiderwebs.19 Specifically, the commercial reciprocating stepper motor was used to accurately control the speed of the airflow switch (Fig. S18, ESI†). As can be seen from Fig. 4d, the sensor could respond to the dynamic airflow velocities ranging from 0.17 to 2 Hz. More importantly, the airflow sensor displayed stable response performance with only −0.23% and 2.28% fluctuations in electrical response performance under 1150 cycles of airflow stimulus within the two stages, indicating the significant potential in practical long-term applications (Fig. 4e and Fig. S19, ESI†). Compared with previously reported airflow sensors based on various mechanisms (e.g., thermal, optical, piezoresistive, piezoelectric, electrical, and capacitive principles),2,11,13,14,31–36 the airflow sensor with hybrid nanostructure and self-supporting configuration in this work responded as fast as 0.1 s and was capable of detecting a wide airflow range of up to 23 m s−1 with an extremely low detection limit of 0.0087 m s−1 (Fig. 4f and Table S2, ESI†).
The GCEF-based AISW for non-contact manipulation
To further improve the accuracy of the sensor for dynamic airflow recognition, we constructed an AISW system to implement non-contact human–machine control as a way to mimic the airflow response behavior of real spiderwebs. The AISW array consisted of eight self-supported GCEFs named from A1 to A8 (Fig. S20, ESI†), which were arranged uniformly and acted as a compass to monitor the intensity and direction of the applied airflow by comparing the values of current change (Fig. S21, ESI†). Fig. 5a shows the composition of the AISW system, including signal generation, signal processing, and real-time display of the results. The complete circuit connection includes (i) a synchronous current data acquisition unit based on eight high-precision ammeters, (ii) Python software for data processing and analysis, and (iii) processing software for animation display (Fig. S22, ESI†). In our system, three types of current curves could be classified into small and large airflows corresponding to stage-I and stage-II based on the analysis of the eigenvalues extracted from the current curves (Fig. S23, ESI†). Moreover, the cyclic experiments with 60 times-tests were performed for these three types of current curves with a high recognition accuracy of 100%, 98.3%, and 98.3%, respectively (Fig. S24 and Movies S1–S3, ESI†), demonstrating the excellent airflow stage recognition performance of the AISW system. After finishing the airflow stage identification, the location corresponding to the largest absolute value of current change was further found and compared with the threshold to guide the virtual spider for the correct addressing behavior. It is worth noting that the spider will move towards or against the position with the maximum current change for the identified small or large airflow state, respectively, mimicking the reaction of a real spider when it senses prey or danger through the spiderweb.
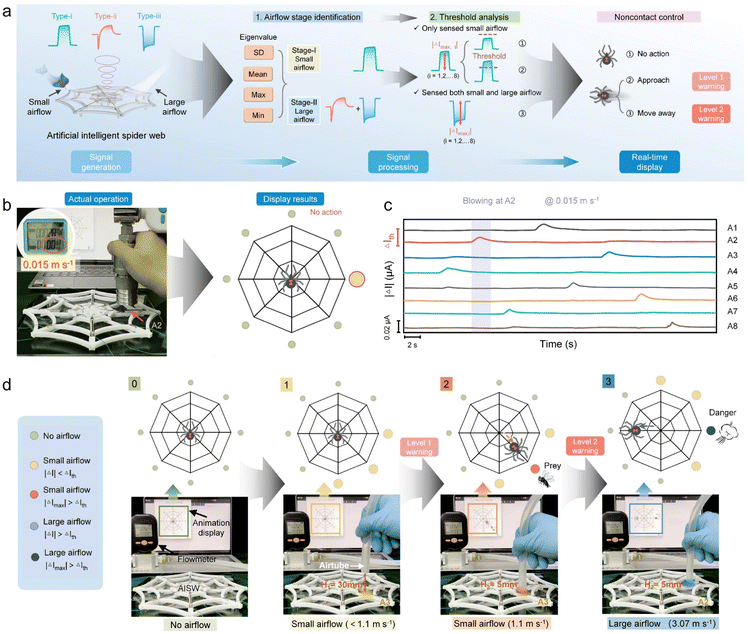 |
| Fig. 5 Application of the AISW system for non-contact manipulation. (a) Schematic illustration of the non-contact control process flow for the AISW system, including signal generation, signal processing, and animation display. (b) Picture of the display results for stimulating the A2 position in the AISW array under extremely weak airflow (0.015 m s−1). (c) Real-time filtered current signals when randomly acting on each sensor in the array with airflow as low as 0.015 m s−1. (d) Photographs of the virtual spider's movement when stimulating different positions in the AISW array with different intensities of airflow. | |
Maintaining the excellent performance of each sensor in the AISW system plays a critical role in achieving accurate non-contact manipulation. Each sensor was first stimulated randomly with an extremely weak airflow to demonstrate high sensitivity and rapid responsiveness (Movie S4, ESI†). Fig. 5b displays that a small airflow state is immediately identified when blowing to the sensor at A2 with the airflow as low as 0.015 m s−1. It is worth noting that the virtual spider did not move due to the current change below the threshold (Fig. 5c). Moreover, the enhanced airflow corresponding to small and large airflow stages was applied to stimulate the array to manipulate the spider's movements, accompanied by level 1 and level 2 warning behaviors, respectively, demonstrating the superior responsiveness and favorable consistency of the sensing performance for the eight sensors in the array (Fig. S25, ESI†).
Finally, the AISW was employed to mimic the behavior of the real spiderweb, which perceives surrounding airflow and guides the spider's movement through the transmission of vibrations. As shown in Fig. 5d, Fig. S26 and Movie S5 (ESI†), the spider initially stayed in the middle of the web waiting for the prey to arrive. Since there was no airflow applied, no remarkable current change was observed (Fig. S26a, ESI†). When a small airflow (<1.1 m s−1) was applied far above A3, the position of A2, A3, and A4 could be successfully identified as a small airflow state (Fig. 5d-1) and respond with upward-increasing signals (Fig. S26a-1, ESI†). It is noteworthy that the spider did not move as the current change was lower than the threshold (Fig. S26b-1, ESI†). Moreover, as the applied airflow was close to the sensor at A3, the three positions A2, A3, and A4 were still recognized as small airflow. However, the spider quickly approached A3 (Fig. 5d-2) as the current variation here exceeded the threshold value for level 1 warning (Fig. S26a, b-2, ESI†). When the large airflow (3.07 m s−1) was applied on A2, the large airflow state was immediately detected for level 2 warning, while the adjacent sensors were small airflow states due to the attenuation of the diffused airflow. The spider moved quickly towards the opposite direction of A2 (Fig. 5d-3) as this position experienced the maximum current variation and was greater than the threshold (Fig. S26a and b-3, ESI†). Therefore, the AISW with self-supported configuration provides a novel platform for sensitively perceiving and capturing the wide range of airflows and serving as soft electronics for smart biomimetic systems and non-contact human–machine interaction.
Conclusions
In this work, with the inspiration drawn from the airflow perception ability of natural spiderwebs, a bioinspired AISW composed of an eight GCEF-based airflow sensor array was proposed. The self-supported configuration responds sensitively to airflows with an opposite tendency of current variation based on the competition between the transverse and longitudinal strains, resulting in high-performance airflow sensing, including an ultralow airflow detection limit (∼0.0087 m s−1), ultrawide airflow sensing range (∼0.0087–23 m s−1), fast response speed (∼0.1 s) and good cycling stability (1150 times airflow on–off). As a proof of concept, an AISW system integrated with the current signal processing function was developed to recognize the location and intensity of the applied airflow for real-time monitoring and danger warning. The design of the self-supported airflow sensor shows significant potential in the field of soft electronics and smart biomimetic systems.
Author contributions
P. X. and T. C. proposed the idea and supervised the project. W. Z., P. X., and T. C. designed the experiments. W. Z. and C. Z. performed the fabrication, characterizations, and data analysis. W. Z., P. X., Q. Y., and T. C. wrote the manuscript. All the authors discussed the results and commented on the manuscript. The manuscript was written with the contributions of all authors.
Conflicts of interest
The authors declare no competing financial interests.
Acknowledgements
This work was supported by the Natural Science Foundation of China (52073295), Youth Innovation Promotion Association of Chinese Academy of Sciences (No.2023313), the National Key Research and Development Program of China (2022YFC2805204, 2022YFC2805202), Sino-German Mobility Program (M-0424), Open Research Projects of Zhejiang Lab (No. 2022MG0AB01), Key Research Program of Frontier Sciences, Chinese Academy of Sciences (QYZDB-SSWSLH036), Bureau of International Cooperation, Chinese Academy of Sciences (174433KYSB20170061) and K. C. Wong Education Foundation (GJTD-2019-13).
Notes and references
- J. Park, Y. Lee, J. Hong, M. Ha, Y.-D. Jung, H. Lim, S. Y. Kim and H. Ko, ACS Nano, 2014, 8, 4689–4697 CrossRef CAS PubMed
.
- Y.-F. Liu, P. Huang, Y.-Q. Li, Q. Liu, J.-K. Tao, D.-J. Xiong, N. Hu, C. Yan, H. Wang and S.-Y. Fu, J. Mater. Chem. A, 2019, 7, 1889–1896 RSC
.
- J. Chen, P. Liu, J. Hu, J. Yang and C. Chen, Rev. Sci. Instrum., 2022, 93, 025001 CrossRef CAS PubMed
.
- Y. Bian, R. Liu and S. Hui, Funct. Mater. Lett., 2016, 9, 1650001 CrossRef CAS
.
- N. Izadi, M. J. de Boer, J. W. Berenschot and G. J. M. Krijnen, J. Micromech. Microeng., 2010, 20, 085041 CrossRef
.
-
A. Panahi, M. H. Sabour and E. Ghafar-Zadeh, in 2017 IEEE 30th Canadian Conference on Electrical and Computer Engineering (CCECE), 2017, 1–4.
- R.-H. Ma, Y.-H. Wang and C.-Y. Lee, Sensors, 2011, 11, 2715–2727 CrossRef PubMed
.
- Z. Dong, D. Shen, Z. Gong, D. Zhang and Y. Jiang, IEEE Sens. J., 2022, 22, 15884–15892 CAS
.
- T. Jiang, L. Deng, W. Qiu, J. Liang, Y. Wu, Z. Shao, D. Wang, M. Zhang, X. Qian, J. Zhong, X. Wang and L. Lin, Biosens. Bioelectron., 2020, 163, 112288 CrossRef CAS PubMed
.
- M. Shriwas and C. Pritchard, Min. Metall. Explor., 2020, 37, 1015–1021 Search PubMed
.
- S. Huang, B. Zhang, Y. Lin, C.-S. Lee and X. Zhang, Nano Lett., 2021, 21, 4684–4691 CrossRef CAS PubMed
.
- M. Venkatesan, W.-C. Chen, C.-J. Cho, L. Veeramuthu, L.-G. Chen, K.-Y. Li, M.-L. Tsai, Y.-C. Lai, W.-Y. Lee, W.-C. Chen and C.-C. Kuo, Chem. Eng. J., 2022, 433, 133620 CrossRef CAS
.
- H. Wang, S. Li, Y. Wang, H. Wang, X. Shen, M. Zhang, H. Lu, M. He and Y. Zhang, Adv. Mater., 2020, 32, 1908214 CrossRef CAS PubMed
.
- Q. Jiang, R. Li, F. Wang, X. Shi, F. Chen, Y. Huang, B. Wang, W. Zhang, X. Wu, F. Wei and R. Zhang, Adv. Mater., 2022, 34, 2107062 CrossRef CAS PubMed
.
- X.-F. Zhao, X.-H. Wen, P. Sun, C. Zeng, M.-Y. Liu, F. Yang, H. Bi, D. Li, R.-G. Ma, J.-C. Wang, X.-B. Yu, D. W. Zhang and H.-L. Lu, ACS Appl. Mater. Interfaces, 2021, 13, 10428–10436 CrossRef CAS PubMed
.
- Y. Lee, W. J. Song, Y. Jung, H. Yoo, M.-Y. Kim, H.-Y. Kim and J.-Y. Sun, Sci. Rob., 2020, 5, eaaz5405 CrossRef PubMed
.
- L. Liu, Y. Huang, F. Li, Y. Ma, W. Li, M. Su, X. Qian, W. Ren, K. Tang and Y. Song, Chem. Commun., 2018, 54, 4810–4813 RSC
.
- X. Liu, D. Liu, J. Lee, Q. Zheng, X. Du, X. Zhang, H. Xu, Z. Wang, Y. Wu, X. Shen, J. Cui, Y.-W. Mai and J.-K. Kim, ACS Appl. Mater. Interfaces, 2019, 11, 2282–2294 CrossRef CAS PubMed
.
- J. Zhou and R. N. Miles, Proc. Natl. Acad. Sci. U. S. A., 2017, 114, 12120–12125 CrossRef CAS PubMed
.
- J. Zhou, J. Lai, G. Menda, J. A. Stafstrom, C. I. Miles, R. R. Hoy and R. N. Miles, Proc. Natl. Acad. Sci. U. S. A., 2022, 119, e2122789119 CrossRef CAS PubMed
.
- P. Xiao, W. Zhou, Y. Liang, S.-W. Kuo, Q. Yang and T. Chen, Adv. Funct. Mater., 2022, 32, 2201812 CrossRef CAS
.
- J. He, R. Zhou, Y. Zhang, W. Gao, T. Chen, W. Mai and C. Pan, Adv. Funct. Mater., 2022, 32, 2107281 CrossRef CAS
.
- Y. Liang, P. Xiao, F. Ni, L. Zhang, T. Zhang, S. Wang, W. Zhou, W. Lu, S.-W. Kuo and T. Chen, Nano Energy, 2021, 81, 105617 CrossRef CAS
.
- S. Wang, Y. Gao, A. Wei, P. Xiao, Y. Liang, W. Lu, C. Chen, C. Zhang, G. Yang, H. Yao and T. Chen, Nat. Commun., 2020, 11, 4359 CrossRef PubMed
.
- T.-S. Dinh, Le, J. An, Y. Huang, Q. Vo, J. Boonruangkan, T. Tran, S.-W. Kim, G. Sun and Y.-J. Kim, ACS Nano, 2019, 13, 13293–13303 CrossRef PubMed
.
- W. Zhou, P. Xiao, Y. Liang, Q. Wang, D. Liu, Q. Yang, J. Chen, Y. Nie, S.-W. Kuo and T. Chen, Adv. Funct. Mater., 2021, 31, 2105323 CrossRef CAS
.
- L. Liu, S. Niu, J. Zhang, Z. Mu, J. Li, B. Li, X. Meng, C. Zhang, Y. Wang, T. Hou, Z. Han, S. Yang and L. Ren, Adv. Mater., 2022, 34, 2200823 CrossRef CAS PubMed
.
- P. Xiao, Y. Liang, J. He, L. Zhang, S. Wang, J. Gu, J. Zhang, Y. Huang, S.-W. Kuo and T. Chen, ACS Nano, 2019, 13, 4368–4378 CrossRef CAS PubMed
.
- L. Veeramuthu, C.-J. Cho, F.-C. Liang, M. Venkatesan, R. Kumar G, H.-Y. Hsu, R.-J. Chung, C.-H. Lee, W.-Y. Lee and C.-C. Kuo, ACS Appl. Mater. Interfaces, 2022, 14, 30160–30173 CrossRef CAS PubMed
.
- Y. Ma, J. Ouyang, T. Raza, P. Li, A. Jian, Z. Li, H. Liu, M. Chen, X. Zhang, L. Qu, M. Tian and G. Tao, Nano Energy, 2021, 85, 105941 CrossRef CAS
.
- Z. Xu, K. Wu, S. Zhang, Y. Meng, H. Li and L. Li, Mater. Horiz., 2017, 4, 383–388 RSC
.
- M. M. Sadeghi, R. L. Peterson and K. Najafi, J. Micromech. Microeng., 2013, 23, 085017 CrossRef CAS
.
- S. Zhao and R. Zhu, Adv. Mater., 2017, 29, 1606151 CrossRef PubMed
.
- K. Takei, Z. Yu, M. Zheng, H. Ota, T. Takahashi and A. Javey, Proc. Natl. Acad. Sci. U. S. A., 2014, 111, 1703–1707 CrossRef CAS PubMed
.
- H. Takahashi, A. Nakai and I. Shimoyama, Sens. Actuators, A, 2018, 281, 243–249 CrossRef CAS
.
- J. Luo, N. Ji, W. Zhang, P. Ge, Y. Liu, J. Sun, J. Wang, Q. Zhuo, C. Qin and L. Dai, Mater. Horiz., 2022, 9, 1503–1512 RSC
.
|
This journal is © The Royal Society of Chemistry 2023 |
Click here to see how this site uses Cookies. View our privacy policy here.