DOI:
10.1039/D3LC00729D
(Critical Review)
Lab Chip, 2024,
24, 1135-1153
Development and future of droplet microfluidics
Received
25th August 2023
, Accepted 4th October 2023
First published on 2nd January 2024
Abstract
Over the past two decades, advances in droplet-based microfluidics have facilitated new approaches to process and analyze samples with unprecedented levels of precision and throughput. A wide variety of applications has been inspired across multiple disciplines ranging from materials science to biology. Understanding the dynamics of droplets enables optimization of microfluidic operations and design of new techniques tailored to emerging demands. In this review, we discuss the underlying physics behind high-throughput generation and manipulation of droplets. We also summarize the applications in droplet-derived materials and droplet-based lab-on-a-chip biotechnology. In addition, we offer perspectives on future directions to realize wider use of droplet microfluidics in industrial production and biomedical analyses.
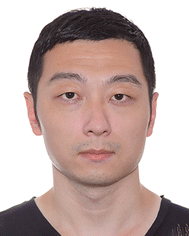 Lang Nan | Lang Nan received his PhD in Mechanical Engineering from the University of Hong Kong in 2021. He currently works as a postdoctoral researcher in Advanced Biomedical Instrumentation Centre in Hong Kong. His research interests include droplet-based microfluidics, point-of-care testing, and biomedical engineering. |
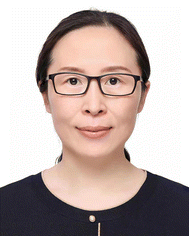 Huidan Zhang | Huidan Zhang received her B.S. degree from the Department of Clinical Medicine at China Medical University in 2001, and M.S. degree from the Department of Gynecology and Obstetrics at Shengjing Hospital of China Medical University in 2004. After that she joined the Department of Cell Biology at China Medical University, where she received her PhD in Cell Biology in 2009 and become an Associate Professor in 2011. Since then, she has been doing research in Weitz group at Harvard John A. Paulson School of Engineering and Applied Sciences. Her research interests focus on droplet-based lab-on-a-chip applications in biotechnology, such as ddPCR, single-cell & pathogen sequencing and high-throughput enzyme evolution. She is also an entrepreneur to apply these techniques to the fields of tumor-targeted therapy and diagnosis of infectious diseases. |
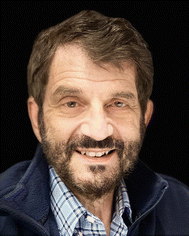 David A. Weitz | Weitz received his PhD in physics from Harvard University and then joined Exxon Research and Engineering Company, where he worked for nearly 18 years. He then became a Professor of physics at the University of Pennsylvania and moved to Harvard at the end of the last millennium as professor of physics and applied physics. He leads a group studying soft matter science with a focus on materials science, biophysics and microfluidics. Several startup companies have come from his lab to commercialize research concepts. |
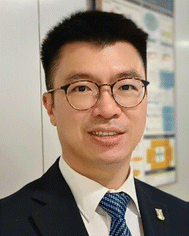 Ho Cheung Shum | Anderson Shum received his PhD in Applied Physics from Harvard University. He is currently a Professor in the Department of Mechanical Engineering at the University of Hong Kong and a core member in the Biomedical Engineering Programme at the University of Hong Kong. He is also the Director of the Advanced Biomedical Instrumentation Centre in Hong Kong. He leads a group studying soft matter and microfluidics. His research interests include liquid–liquid phase separation, emulsions, microfluidics, emulsion-templated materials and soft matter. |
1. Introduction
Microfluidics is a technology of manipulating and controlling fluids in integrated networks of micron-scale channels.1 It has gained extensive impact in a wide range of fields ranging from electrical engineering to biochemical analysis.2,3 Droplet-based microfluidics is a branch of microfluidics focusing on the creation and manipulation of discrete volumes of liquids through adoption of immiscible fluid flows.4,5 Compared with continuous-flow systems, droplet microfluidic systems enable isolation and manipulation of samples in separate compartments. The droplets generated with uniform size and shape provide a stable microenvironment for biochemical reactions.6,7 Processing of a large number of droplets in parallel facilitates to achieve ultrahigh throughput.8 Confinement of individual targets within the droplets and independent processing of each of them allow high-precision analyses.9,10
In the past two decades, there have been significant advances in the design and fabrication of microfluidic devices, which has led to production of ever more sophisticated droplets, and enabled precise control and analysis of their contents.11 Droplet microfluidics is extensively used to produce new materials: droplets serve as advanced templates to synthesize functional materials that enable high-efficiency encapsulation and controlled release of active ingredients.12,13 Droplet microfluidics is also extensively used for lab-on-a-chip applications in biotechnology: droplets function as isolated reactors enabling confinement of individual targets; on-demand manipulation of the droplets allows high-precision and high-throughput analyses of a large number of samples.14,15 However, challenges still remain: democratizing droplet microfluidics to economically produce large quantities of lower-value-added materials has yet to be achieved; precisely labelling the contents of each droplet and retaining the label through multiple manipulation steps remain difficult in lab-on-a-chip applications.
In this review, we discuss the fluidic interactions and physical mechanisms of droplet formation. We describe the synthesis of droplet-derived materials and discuss relevant applications. We also describe the functional manipulation techniques and the enabled lab-on-a-chip applications. Finally, we discuss future directions of droplet microfluidics for its wider use in industrial production and biomedical analyses.
2. Physics of droplet generation
There are two types of microfluidic devices commonly used to produce droplets: capillary devices and devices made from polydimethylsiloxane (PDMS). Both types of devices have the advantages that they can be easily fabricated and can produce highly controllable and uniformly sized droplets (Box 1). In each case, the droplet size can be adjusted by tuning the flow rates and channel geometries. In addition, combining individual droplet formation steps enables generating multiple emulsions.
Box 1. Device fabrication
The fabrication of microfluidic devices is important for the stability of droplet generation and the precision of droplet manipulations. The devices should be fabricated with high precision, efficient sealing, and good transparency. Microfluidic chips fabricated from PDMS using soft lithography are the most commonly used in droplet microfluidics.16 Predesigned patterns are first etched onto a photoresist layer coated on a silicon substrate using photolithography. The PDMS is then cast and cured to form the micropatterned channels. Finally, the channels are sealed by bonding the device with a glass slide. Multiple PDMS chips can be repeatedly fabricated from a single mold, and sophisticated networks can be fabricated for precise droplet manipulations.17 In addition, 3D printing can also be used to fabricate microfluidic chips with custom-designed channels, although the precision of such devices is significantly lower than those made with soft lithography.18 Capillary devices are also commonly used in droplet microfluidics. A series of cylindrical and square glass capillaries are coaxially aligned to generate single and multiple emulsions.19 The capillary devices are compatible with most organic solvents and are thus widely used in materials synthesis.20
For stable and continuous generation of droplets in microfluidic devices, the wettability of the fluids on the channel walls needs to be controlled. The channels should be preferentially wet by the continuous fluid; thus, for forming water-in-oil droplets, the oil should wet the channel walls, which are often rendered hydrophobic through silanization or siliconization; for forming oil-in-water droplets, the channel walls are often pre-treated by oxygen plasma or with a hydrophilic coating.21
|
2.1 Fluid dynamics of droplet formation
When one liquid flows through a nozzle immersed in a second, flowing immiscible liquid, the first liquid forms droplets.22 Each growing drop experiences two competing forces: the viscous force of the second, flowing fluid that pulls it downstream and the surface tension force that holds it to the nozzle.23 At low flow rates, the time required for the drop to be pulled away from the nozzle due to viscous forces is longer than the time it takes to pinch off and form the drop. Thus, the droplets are formed at or near the nozzle, which is a result of an absolute instability and is called the dripping regime.24 The pinch-off occurs at a fixed location in space with an intrinsic frequency; hence the droplet breakup is insensitive to external noise, leading to the formation of monodisperse droplets. When the continuous-phase flow rate increases, the dispersed phase is pulled away from the nozzle before pinch-off. As a result, the dispersed phase forms a jet which becomes thinner as it flows downstream. The jet subsequently breaks up into droplets due to a convective instability, the Rayleigh–Plateau instability.25 Droplet formation is driven by the perturbations in the width of the jet; these perturbations are unstable and grow in amplitude, leading to the formation of droplets with a polydispersity typically higher than that in the dripping regime. In this narrowing jetting regime, the droplets are formed due to the viscous forces imposed by the flow of the continuous phase, which must overcome the surface tension forces. To compare the relative importance of these two forces, the capillary number, Ca, is introduced; it is the ratio of the viscous force to the surface tension force. A different jetting regime is observed when the flow rate of the dispersed phase increases, opposing droplet pinch-off and widening the jet.26 Here, the droplets are formed due to the inertial forces imposed by the flow of the dispersed phase, which must overcome the surface tension forces. To compare the relative importance of these two forces, the Weber number, We, is introduced; it is the ratio of the inertial force to the surface tension force. In each regime, the transition between dripping and jetting occurs when the appropriate dimensionless number is of order one; more generally, the transition occurs when the sum of capillary and Weber numbers is approximately equal to one.27
2.2 Droplet generation in capillary devices
Droplets with uniform size and shape can be generated in capillary devices. The capillary devices are fabricated by coaxial alignment of a series of glass capillaries. The three-dimensional configuration improves droplet formation by ensuring that the continuous phase completely surrounds the dispersed phase as the droplet forms. Furthermore, as glass is compatible with a wide range of solvents, these devices can generate emulsions with almost any liquid phases. Moreover, the wettability of these glass devices can be easily controlled, thus facilitating the generation of multiple emulsions. Commonly used geometries include co-flow and flow-focusing configurations, as well as their combinations (Table 1).
Table 1 Characterization of the droplet size in capillary devices
Generation configuration |
Flow regime |
Droplet size |
Specification |
Schematic representation |
Co-flow |
Dripping |
|
μ
c and uc are the viscosity and velocity of the continuous phase, and γ is the interfacial tension28 |
|
Narrowing jetting |
|
k* is the wave number corresponding to the maximum growth rate of perturbations, and μd is the viscosity of the dispersed phase23 |
|
Widening jetting |
|
u
d is the velocity of the dispersed phase, ωmax is the maximum growth rate of capillary perturbations, Oh is the Ohnesorge number, and uj is the velocity of jet at the most downstream position23 |
|
Flow-focusing |
Dripping |
|
ref. 29 |
|
Jetting |
|
ref. 30 |
|
Combination |
Dripping |
d ∝ Qo−1 |
ref. 31 |
|
Jetting |
|
μ
o is the viscosity of the outermost phase31 |
|
Co-flow devices are constructed by inserting a cylindrical capillary with a tapered tip into a square capillary. Coaxial alignment is ensured by matching the outer diameter of the cylindrical capillaries to the inner diameter of the square capillary. The dispersed phase flows into the inner cylindrical capillary, while the continuous phase flows through the interstitial space between the inner and outer capillaries in the same direction (Fig. 1a).32 When the inner fluid flows out of the orifice, it is spontaneously broken into discrete droplets. Flow-focusing devices are constructed in the same way as the co-flow devices; however, the two phases are introduced from two ends of the square capillary in opposite directions (Fig. 1b).30 The inner fluid is hydrodynamically focused and pinched off by the outer fluid when they flow through the narrow orifice of the inner capillary.29 In both co-flow and flow-focusing, the droplet size can be controlled by the diameter of the orifice and the flow rates.28
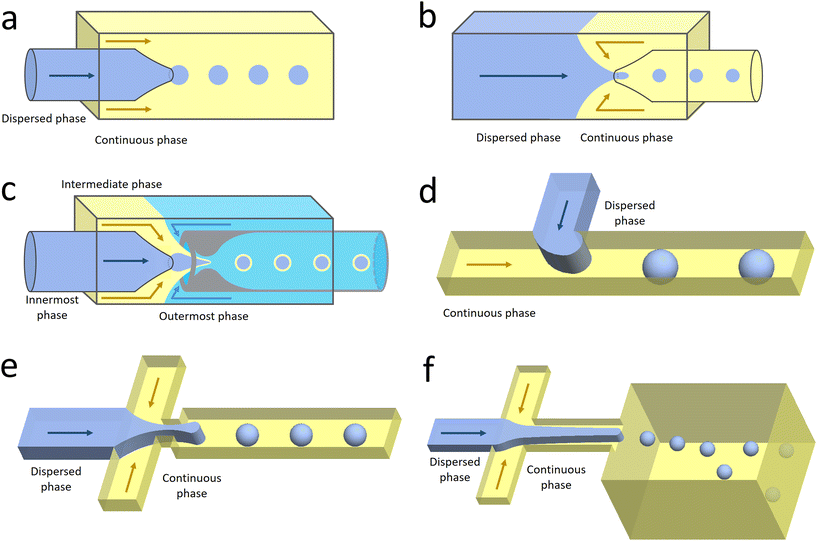 |
| Fig. 1 Configurations for droplet generation. a. Capillary co-flow: two immiscible liquids are introduced from inner and outer capillaries in the same direction. The droplets are formed when the co-flowing fluids meet at the orifice.32 b. Capillary flow-focusing: the two liquids are both introduced from the outer capillaries in opposite directions. The droplets are formed when the two fluids are focused through a narrow orifice.30 c. Combination of capillary co-flow and flow-focusing: when the coaxially flowing innermost and intermediate phases are focused by the outermost phase into the narrow orifice, double-emulsion droplets are formed in a single step.31 d. Cross-flow: the two liquids are introduced from orthogonal channels that form a T-shaped junction. The droplets are formed when the two liquids meet at the T junction.33 e. Planar flow-focusing: the continuous phase is introduced from channels on both sides of the dispersed phase. The droplets are formed when the two fluids are focused through the constriction.34 f. Step-emulsification: the diameter of the flowing dispersed fluid increases as it enters the wider channel, leading to droplet formation at the step.35 | |
The co-flow and flow-focusing configurations can be combined to generate double-emulsion droplets (Fig. 1c).31 Two cylindrical capillaries are aligned end-to-end within a square capillary. The innermost phase flows through the inner cylindrical capillary, while the intermediate phase flows through the interstitial space between the inner and outer capillaries in the same direction. The outermost phase flows through the interstitial space from the opposite end in the opposite direction and hydrodynamically focuses the two co-flowing liquid phases into double-emulsion droplets as they pass through the orifice. Double emulsions with ultrathin fluid shells can be further generated by using this device.36 The innermost and intermediate phases are both introduced from the inner capillary, while the outermost phase is introduced through the interstitial space in the same direction. The intermediate phase that has higher affinity for the capillary flows along its wall and forms a thin layer, surrounding the innermost phase. A compound jet consisting of these two concentric phases is thus formed at the orifice of the capillary. The jet is then broken up into double-emulsion droplets in a single step.
An alternative design to generate double-emulsion droplets is by combining two co-flow formation steps in series.37 The innermost phase is first emulsified into the intermediate phase to form single emulsions, followed by emulsification into the outermost phase to form double emulsions. Higher-order multiple emulsions can be further produced by adding additional sequential emulsification steps. The number and size of the droplets at each level can be precisely controlled. Moreover, multiple emulsions with different components can be generated by merging multiple streams of inner droplets.38
2.3 Droplet generation in PDMS devices
Microfluidic chips fabricated from PDMS using soft lithography are commonly used in biochemical analyses. This type of device can be custom-designed for upstream adjustments of fluid streams and downstream manipulations of droplets. Commonly used geometries include cross-flow, flow-focusing, and step-emulsification (Table 2).
Table 2 Characterization of the droplet size in PDMS devices
Generation configuration |
Flow regime |
Droplet size |
Specification |
Schematic representation |
Cross-flow |
Squeezing |
|
α is one-order constant39 |
|
Dripping |
|
d
i is the hydraulic diameter of the channel40 |
|
Jetting |
|
σ is the interfacial tension coefficient, Cd is the drag coefficient, ρc is the density of the continuous phase, is the velocity of the continuous phase at the center of droplet41 |
|
Flow-focusing |
Squeezing |
d ∝ Ca−1/3 |
ref. 42 |
|
Thread formation |
|
Dripping |
|
Jetting |
|
ref. 43 |
|
Step emulsification |
— |
|
ref. 35 |
|
In the cross-flow geometry, the droplets are typically formed when the two phases meet at a T-shaped junction (Fig. 1d).33 The fluid introduced from the main channel forms the continuous phase, while the other fluid introduced from the branch channel forms the dispersed phase. At low flow rates of the continuous phase, the dispersed phase occupies almost the entire channel before formation of the droplets.40 As the incipient drop grows and gradually obstructs the channel, the gap through which the continuous phase could flow decreases in size. This results in an increasing dynamic pressure imposed on the growing drop. Once the pressure is sufficiently large to overcome the interfacial tension, the interface is squeezed and pinched off to form a droplet.44 This is known as the squeezing regime. Due to channel confinement, the resulting droplets are uniform in size, which is proportional to the flow rate ratio of dispersed phase to continuous phase and the width of main channel.39
In the flow-focusing geometry, the droplets are formed when both the dispersed phase and continuous phase are focused through a constriction (Fig. 1e).34 The resulting viscous shear force focuses the dispersed stream into a narrow thread that eventually breaks up into droplets.45 With increasing Ca, the flow regime transits from squeezing to dripping and to jetting. Occasionally, an array of satellite droplets is formed between the primary droplets in a regime called “thread formation”.46 In the squeezing and dripping regimes, the droplet size is inversely proportional to one-third power of Ca.42 Compared with cross-flow, the flow-focusing allows more stable formation of droplets due to the symmetric shearing effect.47 In addition, multiple emulsions can be generated by combining a series of flow-focusing formation steps.48
In step-emulsification geometry, the droplets are formed as the channel thickness abruptly increases across a step (Fig. 1f).35 The dispersed phase forms a tongue-shaped thread in the shallow channel. When the thread flows through the step, it expands into the reservoir, forming a spherical bulb. The internal Laplace pressure decreases due to the decreased curvature. The pressure difference between the thread and bulb thus sucks the dispersed fluid into the bulb, triggering droplet growth and formation.49 As the curvatures of the thread and bulb are determined by the channel geometries and the contact angle between the dispersed phase and the channel wall, the droplet size is not affected by variations in flow rates.50 This makes the operations of multiple integrated step-emulsification droplet makers very robust, which can facilitate high-volume production of monodisperse droplets.51
As PDMS is deformable, the channel of a microfluidic device can be periodically compressed by applying external forces. The resulting periodic changes in channel width allows active control over the droplet formation. This method can be used to break up liquid jets with low interfacial tension and high viscosity, such as the aqueous two-phase system (ATPS).52 When only hydrodynamic pressure is applied, the ATPS droplets are generated in a jetting regime with high polydispersity and at low frequencies.53 When pneumatic valves are actuated to compress the channel, additional perturbations can be provided to force the jetting regime into the dripping regime, leading to stable and efficient droplet generation.54
3. Droplet-derived materials
Microfluidic droplets can serve as monodisperse templates to synthesize microparticles and microcapsules. Well-defined structures can be fabricated for high-efficiency encapsulation and controlled release of actives. The droplets can also function as controlled reactors to synthesize high-quality nanomaterials. The materials synthesis can be scaled up by parallelizing the droplet generation.
3.1 Materials templated from droplets
Monodisperse microparticles can be fabricated through solidification of the entire droplets.55,56 Chemical polymerization is a common method to solidify the droplets and has been applied to a variety of materials, including gelatin methacrylate (GelMA)57 and poly(ethylene glycol)diacrylate (PEGDA).58 Upon ultraviolet (UV) irradiation, heating, or redox reaction, covalent linkages can be formed within the matrices to solidify the droplets.59 Polymerization provides a fast response time, and the resultant particles are mechanically durable. Physical gelation triggered by ionic cross-linking or freezing is another commonly used method to solidify the droplets. It is often applied to some natural materials that have high levels of biocompatibility, such as alginate60 and agarose.61 However, the reactions between precursors and cross-linking agents are normally conducted in an ultrafast manner, which may instantaneously block the channel and stop droplet generation. To prevent the premature reaction and channel blocking, delicate control over the reactions is required. For instance, in the synthesis of alginate particles, the gelation is slowed by controlled release of calcium ions (Ca2+) from calcium carbonate (CaCO3) or calcium–ethylenediaminetetraacetic acid (calcium–EDTA) nanoparticles (Fig. 2a).62,63 Solidification of droplets through temperature-induced gelation and freezing relies on phase transition of some hydrocarbon and lipid: these molecules possess melting point at or above room temperature, which can be simply solidified by changing the temperature. This type of particle is more biocompatible and thermo-responsive, but the pores formed are relatively large, which may cause undesired leakage of some encapsulants.64 In addition, the droplets can also be solidified through evaporation or diffusion of the solvent.65 Although this approach can be applied to a variety of polymers and compounds dissolvable in a volatile solvent, the response time is significantly slower than that of the polymerization and cross-linking methods, limiting its widespread use. Microcapsules with well-defined core-shell structures can also be templated from microfluidic droplets. They can be produced from single emulsions through phase separation66 or interfacial assembly.67 More improved method of fabrication focuses on the use of double-emulsion templates by solidifying the shell components.68,69 The thickness of the shell as well as the size and number of the cores can be precisely controlled by tuning the flow rates.70
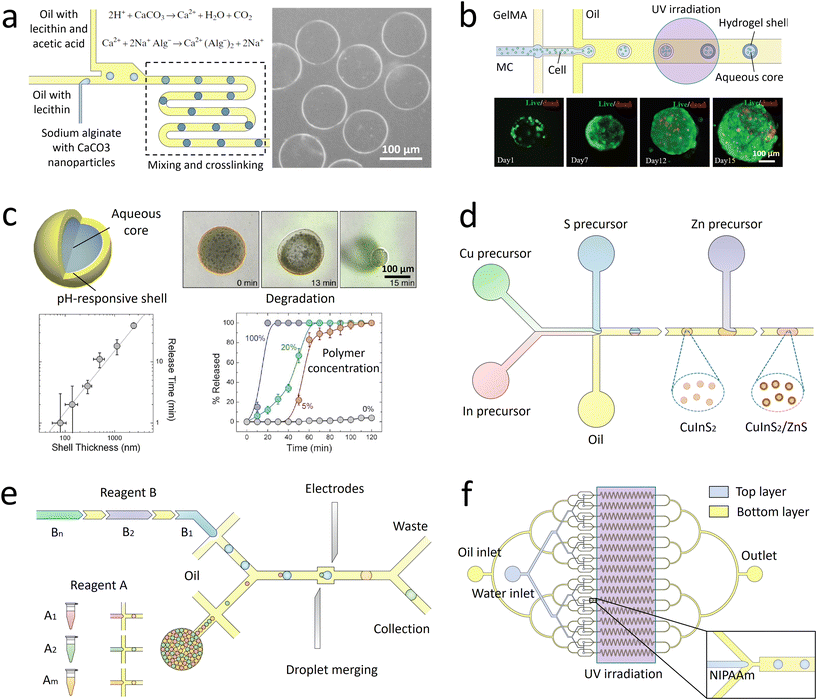 |
| Fig. 2 Droplet-derived materials. a. When the droplets flow with acidic oil, Ca2+ ions are gradually released from CaCO3 nanoparticles to cross-link the sodium alginate.62 b. Microparticles with a core of methyl cellulose (MC) and a shell of GelMA are fabricated to encapsulate cells. After transfer into culture medium for further incubation, spherical cell aggregates are formed with high cell viability.71 c. When a microcapsule is exposed to a trigger pH, its solid shell composed of pH-responsive polymers gradually degrades to release encapsulants. The release kinetics can be precisely controlled by the shell thickness, polymer concentration, and pH value.72 d. Precursors of Cu, In, and S are compartmentalized into droplets and reacted to form CuInS2 nanoparticles as the core. Then Zn precursors are injected into the droplets to form ZnS shells on the core particles.73 e. A library of reagent A is compartmentalized into droplets and reinjected into the channel. Another library of reagent B, spaced by an oil phase, is successively introduced into the channel and compartmentalized into droplets. The two sets of droplets are merged in pairs, producing a library of different combinations. The synthesized products are driven into the collection channel by negative pressure, while the spacing oil between reagent B flows into the waste channel.74 f. Droplets of N-isopropylacrylamide (NIPAAm) are generated and polymerized in 16 channels in parallel to achieve mass production of microparticles.75 Panel A is adapted with permission from ref. 62. Copyright (2007) WILEY-VCH Verlag GmbH & Co. KGaA, Weinheim. Panel B is adapted with permission from ref. 71. Copyright (2019) WILEY-VCH Verlag GmbH & Co. KGaA, Weinheim. Panel C is adapted with permission from ref. 72. Copyright (2013) American Chemical Society. | |
The droplet-templated particles can encapsulate target samples with high efficiencies. Conventional bulk-volume emulsification that is often conducted by turbulent mixing results in a significant sample loss during stirring or agitation.76 By contrast, in microfluidic emulsification, the dispersed phase is stably injected into the continuous phase under laminar-flow conditions, thus achieving high-efficiency encapsulation of samples with a low loss.77 By adjusting the sample concentration and flow rates, the doses of ingredients in each particle can be precisely controlled.78 The high loading efficiency and effective protection facilitate encapsulation of pharmaceutical, food, and cosmetic additives to enhance their functions.79 More delicate materials, such as cells, can be encapsulated in the capsules with well-defined core–shell structures.80 The solid shell isolates the samples from outer environment, while the liquid core supports cell growth and development (Fig. 2b).71 Moreover, the flexible choice of shell materials allows fabrication of more complex capsules for enhanced encapsulation. For example, phospholipids or amphiphilic diblock copolymers can be dissolved into a solvent that forms the intermediate phase of water–oil–water double emulsions. Upon evaporation of the solvent, vesicles of liposomes81 or polymersomes82 can be fabricated. Their shells resemble natural bilayer membranes and can facilitate the encapsulation of bioactive samples, such as enzymes.83
The droplet-templated capsules can also be used to control the release of encapsulated ingredients.84 The target samples can be passively released through diffusion across the pores in the shells, leading to release over an extended period of time. In this case, the release profile is mainly controlled by the capsule structure; the release rate decreases with increasing shell thickness and decreasing pore size.85 The passive diffusion is widely used in sustained release of drugs, where the dose of therapeutic ingredients can be optimized according to the needs of treatment.86 The encapsulated samples can also be actively released through degradation of the capsule matrix. By incorporating stimuli-responsive polymers into the shells, their matrices can be broken upon changes in temperature,87 osmotic pressure,88 or pH (Fig. 2c).72 Moreover, release can be triggered on demand by exposing the capsules to specific molecules. For instance, protein-based capsules can be degraded when digestive enzymes are introduced.89 The active release can facilitate targeted delivery of drug components, where the release profile can be manipulated by varying the capsule size and polymer concentration.90
3.2 Materials synthesized from in-drop reactions
Monodisperse droplets provide a controlled environment for the synthesis of new materials. Nanoparticles with narrow size distributions and optimal formulations can be produced by using the droplets as microreactors. In conventional synthesis, nanoparticles are produced in bulk batch reactors. The length scale of the reactors sets the mixing timescale. If the mixing timescale is significantly longer than the reaction timescale, the reaction occurs before the solution becomes well-mixed, resulting in polydisperse particles with nonuniform size and properties. In droplet-based synthesis, the mixing timescale can be significantly reduced due to the small length scale of droplets. Moreover, the viscous drag at the channel wall induces internal recirculation within the droplets, facilitating the mass transfer and further shortening the mixing timescale.91 As a result, the reactants within the droplets are better mixed, yielding more uniform particle size and properties. In addition, by adjusting the flow rates of each stream, the doses of reagents encapsulated into the droplets can be precisely controlled.92 The well-controlled reactions enable synthesis of monodisperse particles loaded with optimal amounts of DNA and proteins, and the surface can be linked with multiple ligands with a predefined ratio.93 These nanoparticles allow simultaneous delivery of genes and transcription factors for synergistic therapeutic effects.
The droplet reactors also permit controlled multi-step reactions to synthesize more sophisticated nanostructures. Controlled volume of new reagents are repeatedly added to the droplets, enabling multiple rounds of reactions. The reagents can be added by fusing two sets of droplets: the new reagents are encapsulated into a separate set of droplets, which are merged with the target droplets.94 Alternatively, the new reagents can be directly injected into the droplets: the reagent stream is introduced from a channel meeting another channel in a T-shaped junction; when the droplets pass through the junction, the droplet interface is destabilized to allow injection of the reagents.95 Monodisperse core–shell nanoparticles with tailored structures can be fabricated through the multi-step reactions. The core of the nanoparticles is first formed in the droplet reactors, followed by addition of new reactants to form the shell (Fig. 2d).73 This type of nanoparticles could have superior optical and sensing properties, which can function as tracer particles in cell biology for specific molecular binding and labelling.96
Besides fabricating identical products, combinatorial synthesis allows to fabricate different materials in each droplet reactor.97 A library of reagents A, including A1, A2, …, Am, is compartmentalized into one set of droplets; a second library of reagents B, including B1, B2, …, Bn, is compartmentalized into another set of droplets. By merging the two sets of droplets in pairs, m × n different types of materials can be synthesized (Fig. 2e).74 The library of combinatorial products can be further screened to discover new materials. The original reagents are encoded with different concentrations of fluorescent dyes, so that the composition of droplets can be identified by measuring the fluorescence intensity.98
3.3 Scale up
Scale up of the droplet generation enables increased production of the microparticles and microcapsules, as well as the nanomaterials. In a single device, the droplet generation rate is normally below 10 mL h−1, limiting further industrial applications.99 One approach to increase the generation rate is to operate multiple droplet makers in separate devices in parallel. In this case, each device functions independently; any fluctuation of flow rates in one device will not affect the operation of other devices. Thus, the droplets can be generated in a robust manner. However, each device has its own inlets, which require separate pumps for flow control. As the number of devices and inlets increases, the number of pumps required also increases, complicating the operation and limiting the achievable production rate.
To eliminate the requirement of multiple pumps, strategies to reduce the number of devices and inlets have been developed. The most commonly used method focuses on integrating multiple droplet makers into one device with only one set of inlets.100 The liquid is introduced from one inlet and then equally distributed to all the droplet makers. To facilitate smooth operation, crossing of the two-phase flows that perturbs the whole system must be prevented. Thus, two-layer devices are often used: the dispersed phase and the continuous phase flow in upper and lower layers separately without crossing (Fig. 2f).75 As many as 1000 droplet makers can be integrated into a single device, which results in an ultrahigh production rate of 1.5 L h−1.101 In such integrated droplet makers, fluctuations in one droplet maker need to be isolated to enhance the stability of the overall droplet generation and uniformity of droplet generated.102,103
4. Droplet-based lab-on-a-chip applications in biotechnology
Droplet microfluidics enables numerous lab-on-a-chip applications in biotechnology that take advantages of the small scale and precise control of the droplets. The droplets serve as reactor vessels to separate and isolate target samples. The confinement of samples decouples the in-drop reactions from the flow that is controlled through the continuous phase. Independent manipulation of each droplet enables further processing and analysis of the encapsulated targets with high levels of precision and throughput.
4.1 Droplet manipulation
4.1.1 Mixing.
Mixing of reagents within the droplets is important to initiate and control in-drop reactions. When a droplet flows through a straight channel, symmetric recirculating flows are generated within the droplet. The reagent within each region of recirculating flow can be well mixed, but the mixing across the different regions is restricted.104 To accelerate the mixing, unsteady fluid flow is introduced to perturb the uniform recirculation. By guiding the droplets through a winding channel, chaotic advection induces asymmetric recirculating flows within the droplets that accelerate the mixing (Fig. 3a).105 Moreover, protrusions along the channel walls can induce oscillatory flows to further enhance the mixing.106 In addition to these passive methods, thermal actuation can be actively applied to accelerate the mixing. Laser107 or microwave108 heating are normally used to induce time-varying Marangoni stresses at the interface, thus creating chaotic flows within the droplets for mixing acceleration. However, the heat generated may perturb the activity of some biomolecules, thus making it less preferable for processing of some biological samples. Besides accelerating in-drop reactions, the enhanced mixing can also facilitate mass transfer between the dispersed phase and continuous phase.109 Some analytical compounds can be controllably transferred in or out of droplets for efficient liquid–liquid extraction and sample analysis.110
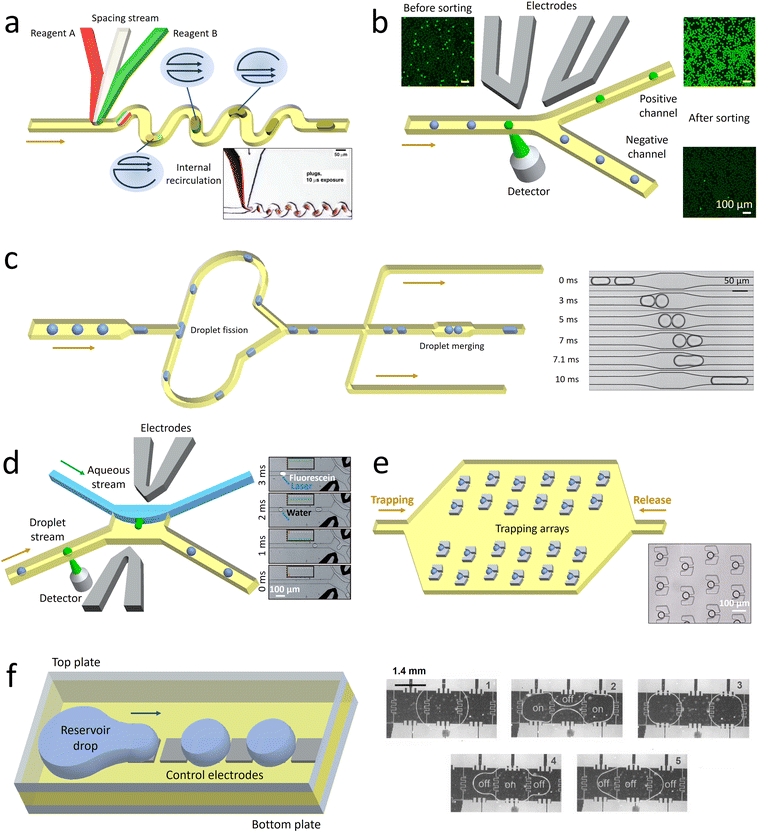 |
| Fig. 3 Schematic illustrating some droplet manipulation modules. a. Mixing: when the droplets flow through the winding channel, the motion of two counter-rotating vortices is induced to accelerate the mixing. The inset shows the rapid mixing inside the droplets.105 b. Selection: the droplets of interest are detected and sorted into the collection channel by dielectrophoretic forces, while the undesired candidates flow into the waste channel. The droplets before and after sorting are shown in the insets.111 c. Splitting and merging: the mother droplets are split into pairs of daughter droplets when they collide onto the channel wall at the T-junction. After extracting the oil downstream, the two synchronized droplets contact each other and coalesce in the expansion chamber. The time sequence images show the process of droplet merging in the expansion chamber.112 d. Extraction: when a desired droplet is detected, an electric field is triggered. Consequently, the droplet is forced across the water–oil interface to merge into a lateral aqueous stream. The time sequence images illustrate the process of droplet extraction activated by fluorescence.113 e. Monitoring: an oil flow is introduced from left to right to immobilize the droplets in traps. After a period of monitoring, the droplets are released by introducing another oil flow in the opposite direction. The inset shows a set of single droplets immobilized in an array of traps.114 f. Manipulation through electrowetting: by regulating the spatial wettability through on-demand voltage switching, the reservoir drop can be broken up into droplets and transported in target directions. The time sequence images show the process of droplet splitting and merging.115 Panel A is adapted with permission from ref. 105. Copyright (2003) WILEY-VCH Verlag GmbH & Co. KGaA, Weinheim. Panel B is adapted with permission from ref. 116. Copyright (2015) Royal Society of Chemistry. Panel C is adapted with permission from ref. 112. Copyright (2008) American Physical Society. Panel D is adapted with permission from ref. 113. Copyright (2008) WILEY-VCH Verlag GmbH & Co. KGaA, Weinheim. Panel E is adapted with permission from ref. 114. Copyright (2009) Royal Society of Chemistry. Panel E is adapted with permission from ref. 115. Copyright (2003) IEEE-INST ELECTRICAL ELECTRONICS ENGINEERS INC. | |
4.1.2 Selection.
Desired targets can be selected by diverting specific droplets into designated branch channels. This droplet selection is normally conducted by combining droplet detection and sorting: when a droplet of interest is detected, it is sorted to the collection channel; when no target is detected, the undesired droplets flow into the waste channel with a lower hydrodynamic resistance (Fig. 3b).111 Dielectrophoresis (DEP) is the most commonly used method to sort the droplets. When signals are applied to the electrodes placed on one side of the channel, a nonuniform electric field is generated. Then the passing droplet becomes polarized and is driven into the collection channel.117,118 The droplets can also be sorted by using surface acoustic wave (SAW).119 When the SAW travels into the fluid, an acoustic streaming is induced and propagates perpendicularly to the fluid flow. Then the droplet is forced into the collection channel.120,121 In addition, a membrane valve can also be used to sort the droplets. The valve is pressurized to squeeze the waste channel and increase its resistance. Consequently, the flowing droplets are diverted into the collection channel.122 By using high-precision electronics to control the droplet detection and actuation, these active sorting approaches can be conducted automatically, and in some instances at frequencies as high as 30 kHz.116 The number of sorted droplets can be further controlled for quantitative collection and analysis of the encapsulants.123 By coupling a droplet sorter with a motorized stage, individual droplets containing desired encapsulants can be continuously isolated and collected onto an array of microwells for off-chip analysis.124
Apart from the active methods, droplet sorting can also be triggered passively under the effect of hydrodynamic forces. Droplets with different characteristics, such as size125 or viscosity,126 are driven along the channel with biased lateral displacement and finally guided to different branches. Compared with the active sorting, the passive sorting requires the channel geometries to be matched with the sorting parameters, thus limiting its flexibility and widespread use. Moreover, passive sorting can be conducted only when the desired droplets have sufficiently large differences in properties from others. If the encapsulated targets, such as the cells and DNA molecules, have little effect on the overall droplet properties, passive sorting fails to select the desired candidates.
4.1.3 Splitting and merging.
The formed droplets can be further split into pairs of daughter droplets to number up the reaction vessels and conduct parallel assays.127 The splitting is typically conducted in a channel with bifurcating junctions.128 When the droplet collides onto the junction wall, asymmetric viscous stresses are induced to overcome the interfacial tension and stretch it into two smaller daughter droplets (Fig. 3c).112 The volume of the daughter droplets is inversely proportional to the hydrodynamic resistance of the subchannel.129
Droplet merging enables sequentially adding the reagents after rounds of droplet processing to conduct multi-step reactions. Two sets of synchronized droplets are merged by first bringing them close together and then causing them to coalesce.130 To ensure that pairs of each type of droplets are alternately arranged with constant distances, the flow rates should be precisely controlled.131 Then the pairs of droplets are guided into expansion chambers, with their flow velocities and the distance between them significantly reduced.132 Under the effect of an electric field, the surfactant-coated interfaces are destabilized to trigger droplet fusion.133 Alternatively, pico-liters of fluids can be injected into the droplets at a T-shaped junction.134 By adjusting the droplet velocity and injection pressure, reagents can be precisely injected into the passing droplets.135 Compared with merging droplet pairs, the pico-injection method requires no precise control over the positions of neighboring droplets, but the volume of reagents that can be injected is limited.
4.1.4 Extraction.
Extraction of the droplet contents is necessary for downstream analysis of the samples after droplet processing. Conventionally, the extraction is operated off chip by adding demulsifier to coalesce the droplets and centrifuging the samples to separate the samples from the carrier oil.136 However, this method incurs inevitable sample loss and may pose damage to some biological samples. By comparison, it is more efficient to extract the droplet contents on chip: when the droplets flow past a junction, they are forced to coalesce with an aqueous stream in a parallel channel under the effect of electric field137 or channel wetting.138 By using detection systems to identify the droplets of interest, desired encapsulants can be selectively extracted (Fig. 3d).113 Moreover, precise extraction of single-droplet contents is sometimes needed for analysis of individual targets off chip. This could be achieved by transferring one single droplet into a tube, followed by addition of aqueous medium to merge and extract the contents.139
4.1.5 Monitoring.
Droplets that are immobilized in traps can be stored over extended periods for real-time monitoring of the in-drop dynamics. The most commonly used traps include converging structures140 and microwells.141 When the droplets pass through these traps, the carrier medium flows through bypass paths, leaving the droplets immobilized under the balance of Laplace pressure and hydrostatic pressure. By further actuating a reverse flow114 or increasing the hydrostatic pressure,142 the trapped droplets can be released after a period of monitoring (Fig. 3e). The trapping and release of each droplet can be precisely controlled by an array of pneumatic valves: when the valve is off, the droplet is allowed to enter the trap for immobilization; when the valve is on, an increased hydrostatic pressure is applied to release the droplet from the trap.143 The switching of each valve can be independently controlled, enabling selective retrieval of individual droplets.144
4.1.6 Manipulation through electrowetting.
Apart from manipulation inside microchannels, droplets can also be formed and manipulated between two parallel plates by electrowetting.145 The top plate is fabricated with a continuous ground electrode, while the bottom plate contains an array of control electrodes. When an electric voltage is applied to the electrodes, the dispersed fluid wets on the surface of the plate and spreads out to form a liquid finger. Then after switching off the voltage, the fluid is retracted, with the neck of the finger narrowing and breaking up into a droplet.146 The formed droplet can be transported across an array of programmed electrodes on demand. Activating the electrodes on only one side of the droplet creates imbalanced interfacial tension on two opposite edges of the droplet, thus inducing its asymmetric deformation. The consequent pressure difference across the droplet actuates it to move. Precise control of the motion enables customized manipulations of the droplet (Fig. 3f).115 For instance, two droplets can be merged by moving them towards each other;147 the merged contents can be further oscillated and mixed by moving the droplet back and forth between adjacent electrodes.148 Compared with manipulating droplets in channels, the manipulations on plates can impose independent control over individual droplets, but the throughput is significantly lower.
4.2 Applications
4.2.1 Single-target analyses.
By confining individual biological targets, such as cells, bacteria, and viruses within the droplets, each target can be independently processed in isolated space for high-precision and high-throughput analyses. Typically, target loading follows Poisson statistics: the portion of droplets encapsulating x targets, P(x), can be characterized by P(x) = e−λ[λx/x!], where λ is the average number of targets in each droplet.149 To reduce the portion of droplets encapsulating double or multiple targets, low values of λ below 0.1 are normally used. As a result, the majority of the droplets are empty, and only a small portion contains single targets.150
The genotypes of the encapsulated single targets can be analyzed with high throughput using droplet microfluidics. To identify the genomes from the same target in final analysis, the droplet contents should be labelled with barcodes. Nucleic acid barcodes comprising unique sequences of oligonucleotides can link with target DNA151 and RNA152 molecules, and their identity can be read out through sequencing.153 This type of barcodes is widely used in droplet-based high-throughput sequencing of single biological targets.154,155 A library of barcodes is separately encapsulated into the droplets with single targets, thus labelling the nucleic acids from the same targets with unique primers. Then the processed samples are pooled and sequenced to profile the gene expression at single-target level (Fig. 4a).156 The enabled high-throughput single-cell sequencing allows screening of the cell heterogeneity in chromatin states,157 somatic mutations,158 and transcriptional states,146 thus revealing the cellular functions that are not detectable by standard bulk sequencing. Single bacteria can also be sequenced using this technique, which allows profiling of the distribution of antibiotic resistance in microbial communities.159,160
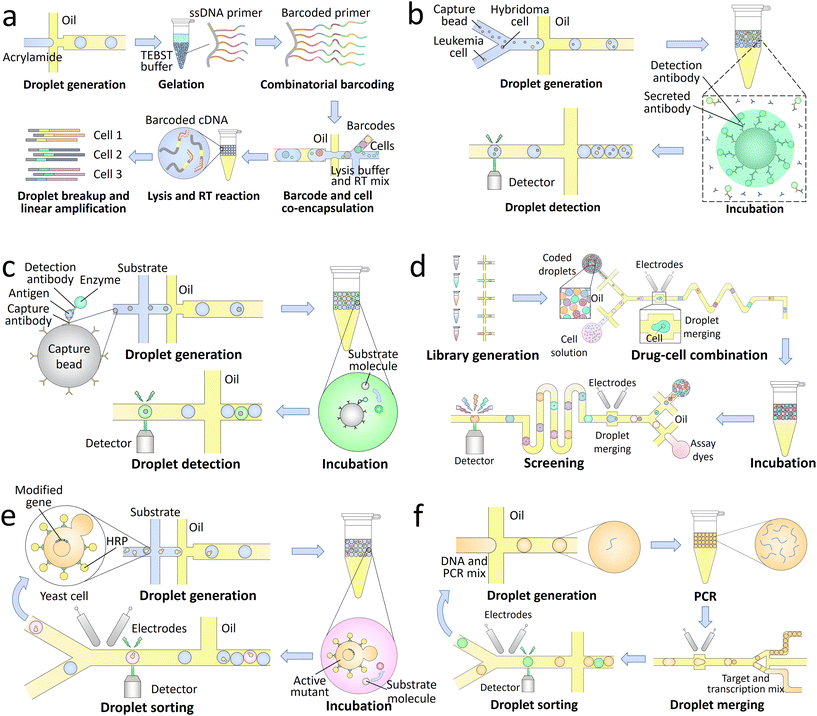 |
| Fig. 4 Droplet-based lab-on-a-chip applications in biotechnology. a. Single-cell sequencing: a library of barcoding hydrogels is synthesized through enzymatic extension reactions, followed by co-encapsulation with single cells, lysis buffer, and RT mix into the droplets. Through successive cell lysis and RT reaction, the mRNA released from individual cells are barcoded with unique oligonucleotide primers. After further breaking the droplets and amplifying the synthesized cDNA, the processed samples are sequenced to achieve a high-throughput transcriptomic analysis of single cells.156 b. Single-cell secretion analysis: single hybridoma and leukemia cells are co-encapsulated with capture beads and fluorescently labelled detection antibodies into the droplets. Upon a period of incubation, the antibodies secreted by the hybridoma cells are concentrated onto the beads together with detection antibodies in a sandwich assay, making the beads to fluoresce. The antibody secretion of single cells can thus be analyzed through detection of the droplet signals.161 c. Droplet digital ELISA: the capture beads sequentially bind with capture antibodies, antigens, detection antibodies, and enzymes, followed by co-encapsulation with substrates into the droplets. The enzymes can convert the substrates to their fluorescent products, thus generating detectable signals for high-sensitivity detection.162 d. Drug screening: a library of optically-coded drugs is encapsulated into the droplets and combined with single cells through pairwise droplet fusion. After a period of incubation, the droplets are reinjected into an assay chip to merge with dye-containing droplets for cell staining. Through further screening of the droplet signals, the cytotoxicity of each candidate can be quantitatively characterized.163 e. Directed evolution: a library of modified genes is transformed into yeast cells to enable surface display of horseradish peroxidase (HRP). After co-encapsulation of the single cells and substrates into the droplets, the actively displayed HRP converts the substrates into fluorescent products. The active mutants are then sorted and harvested in cycles for directed evolution.164 f. Single-aptamer selection: a library of DNA is encapsulated into the droplets and amplified through PCR. The processed droplets are then merged with another set of droplets containing transcription reagents and fluorogenic targets. After a period of incubation to synthesize RNA aptamers binding to the targets, the droplets expressing high fluorescence signals are sorted to select the high-affinity candidates.165 | |
The phenotypes of the encapsulated single targets can also be precisely characterized through detection of the droplet signals. Compared with continuous-flow techniques, such as the flow cytometry, droplet microfluidics shows its unique advantages in analysis of metabolic products and the molecules secreted by the single targets. The target molecules can be separated and confined in pico-littered compartments without cross-contamination, and thus become detectable.166 The signals can come from the whole droplet, for instance, in the characterization of enzymatic activities.167 Fluorogenic substrates are co-encapsulated with single targets and uniformly distributed inside the droplets. The enzymes secreted by the targets can convert the substrates into their fluorescent products, making the whole droplet fluorescent. The signals can also come from only a small part of the droplet, for example, in the analysis of antibody secretion (Fig. 4b).161 Capture beads are encapsulated into the droplets to bind the secreted antibodies, and detection antibodies are added for fluorescent labelling. As a result of the sandwich assay, the fluorophores are concentrated on the capture beads, making the beads more fluorescent than other parts of the droplet to generate detectable signals.
In addition to the analysis of single targets, droplets can also be used to compartmentalize pairs of individuals, whose interactions can be directly studied. Pairs of single cells can be co-cultured within droplets, enabling identification and studies of the cell–cell interactions in central nervous system.168,169 The host–phage association and microbial interactions can also be analyzed by encapsulation, barcoding, and sequencing of the whole genomes of single bacteria.170 In addition, the co-infection of different viruses on single cells and the reassortment of their genome segments can be analyzed using droplet-based barcoding and sequencing techniques.171
4.2.2 Digital droplet detection.
Ultralow concentrations of targets can be detected by dividing the highly diluted samples into a large number of droplets and accurately screening the signal of each droplet. Although the concentration in the whole sample is low, individual targets can be confined and processed within single droplets, creating a high analyte concentration in these droplets for eligible detection. The number of targets can be further quantified by counting the positive droplets. This digital detection can be used for identification of pathogens and early diagnosis of diseases. Droplet digital polymerase chain reaction (ddPCR) is a commonly used method to detect the nucleic acids of pathogens.172,173 Individual DNA molecules are encapsulated and amplified within the droplets. Upon addition of gene-specific probes, the droplets containing target molecules can generate fluorescence signals after amplification.174 Then through screening of the droplet signals, the copy number of the DNA molecules can thus be quantified at single-molecule level.175 With additional reverse transcription (RT) to synthesize complementary DNA (cDNA), single RNA molecules can also be amplified and detected with high precision.176 Due to its high sensitivity and specificity, ddPCR has been effectively applied in combating the pandemics of 2009 H1N1 influenza177 and corona virus disease 2019 (COVID-19).178 In addition, ddPCR can also be used to identify the contaminants in water, soil, and food samples for environmental monitoring and safety control.179
Droplet digital enzyme-linked immunosorbent assay (ddELISA), which focuses on the identification of antigens, can also be used to detect pathogens.180 After sequentially binding antigens, detection antibodies, and enzymes onto capture beads, the conjugates are co-encapsulated with substrates into the droplets. The substrates are converted into fluorescent products and confined in pico-littered volumes to generate high-intensity signals (Fig. 4c).162 Single molecules of proteins can be detected by using this technique with an ultralow detection limit of 20 aM.181 Compared with ddPCR, ddELISA does not require a long thermal cycling time. However, non-specific binding of some contaminants may interfere the target detection, lowering its specificity.
4.2.3 High-throughput screening.
With parallel assays conducted in a massive number of droplets, a wide variety of candidates can be encapsulated and screened with high throughput. Compared with conventional screening performed in microwell plates, droplet-based screening can significantly reduce the required time, cost, and reagent volume. The increased efficiency allows screening of pharmaceutical candidates for drug discovery. After co-encapsulation into the droplets, various drug candidates and the same type of targets are co-incubated for a specific period of time (Fig. 4d).163 Through further screening of the droplet signals, multiple properties of the drug candidates, such as dose response,182 enzyme inhibition,183 and antibiotic synergy,184 can be quantitatively characterized.
The high-throughput screening also allows accurate selection of single cells with specific phenotypic characteristics.185,186 For instance, the candidates that secrete desired enzymes can be periodically sorted and enriched for directed evolution (Fig. 4e).164 In addition, single molecules of nucleic acids amplified within the droplets can also be accurately selected. The nucleic acid candidates are co-encapsulated with specific targets; the candidates that can bind to the targets and generate high fluorescence signals are sorted. High-affinity-binding aptamers that can act as sensitive probes for biosensing applications can be selected by using this technique (Fig. 4f).165,187 Moreover, through sorting and collection of individual droplets, the encapsulated single targets can be recovered and analyzed using conventional biological assays. For instance, the enzyme catalysis and metabolomic data of single yeast cells can be measured by mass spectrometry,188,189 and the full-length genomes of single proviruses can be sequenced with their paired integration sites.190
The single-cell screening can be further combined with genome sequencing to map the relationships between their genotypes and phenotypes.191 Through sorting and sequencing of a specific set of IgG-secreting primary cells, the immunoglobulin G (IgG) secretion and the sequences of paired VH–VL genes can be coupled.192 Single glial cells can also be sorted and collected by nucleic acid cytometry, thus enabling interrogation of the signaling pathways that regulate the pathogenic activities.193
5. Future directions
With the rapid development of droplet generation and manipulation techniques, the focus of droplet microfluidics is moving from laboratory investigations to technological applications, including the industrial production of lower-value-added materials and wider-ranging analyses of biomedical samples.
5.1 Production of materials
Microfluidic droplets serve as advanced templates to synthesize monodisperse particles and capsules with tailored structures and properties. The enhanced encapsulation and release demonstrate great potential for industrial fabrication of high-quality products.194 However, currently it is only applied to the production of some high-value-added products, such as cosmetics. The limited production rate and high manufacturing cost pose significant challenges for adopting microfluidic processes in producing lower-value-added products. Although parallelization enables scale-up of the production, the rate cannot yet meet the industrial demand and the robustness is still too low. Moreover, the use of syringes and pumps restricts the ease of operations and increases the cost, making droplet microfluidics uncompetitive with other manufacturing methods. To address these limitations, more advanced scale-up strategies must be developed to further increase the production rate, and more cost-effective operation strategies are required to lower the cost. With improvement of the production rate and manufacturing cost, droplet microfluidic techniques can be efficiently applied in the production of high-quality foods and beverages. Product structures can be precisely tailored to enhance the stability of active ingredients and control their release profiles.195 In addition to the food and beverage, agriculture may also be benefited from the microfluidic production. For instance, droplet-templated capsules could regulate the release of fertilizers and pesticides to enhance the growth of crops.
5.2 Biomedical analyses
Droplet microfluidics allows a large set of single-target data to be individually acquired and analyzed. However, in conventional microfluidic systems, the droplets lose their original order after rounds of processing and reinjection. Without effective tracking of the droplet contents, tracing and correlating the detected characteristics to their original targets become impossible. Although DNA barcodes can be added to index the droplets, this technique can only trace genotypic characteristics but fails for phenotypic characterization. Thus, more versatile droplet indexing methods must be developed to uniquely code each droplet, and corresponding decoding techniques should be coupled to identify the code. This promises a precise linking of the genotypic and phenotypic characteristics of single cells, thus revealing the mechanisms of cell heterogeneity and dysfunction. Moreover, conventional analytical assays through droplet screening only allow transient characterization of the targets at the end point, while dynamic information of the droplet assays remains difficult to be probed. Although high-speed imaging method enables acquisition of kinetic data when droplet flows through a captured region, the analysis duration is limited to only within 1 s.196 Long-term kinetic studies may rely on high-throughput trapping and monitoring methods. Such enhanced characterization will allow prolonged monitoring on the encapsulated targets.
High-sensitivity detection can be achieved by dividing a highly diluted sample into large numbers of micron-scale droplets. However, in current droplet testing assays, the detection is usually limited to fluorescence. Despite the fast response time and high signal-to-noise ratio, fluorescence requires high-specificity labelling, thus limiting the targets that can be detected. To address this limitation, more label-free detection methods, such as artificial intelligence (AI)-based ultrafast imaging that enables extraction of subtle features of images and autonomous identification of targets, should be incorporated into the droplet assays.197 The large dataset generated by droplet microfluidics favors AI to train its models, while the constructed models can feed back microfluidic systems to achieve high degrees of accuracy and sensitivity.198 The enhanced detection will allow more pathogens to be accurately identified, thus facilitating the application of droplet microfluidics in a much broader range of medical tests.
With a large number of assays conducted in parallel, droplet microfluidics enables screening and selection of a wide variety of candidates. However, in current microfluidic systems, the droplets are normally detected and sorted one by one, limiting the throughput. Advanced scale-up strategies should be developed to simultaneously screen multiple droplets. Moreover, it is technically challenging for current detection assays to characterize and probe chemical components within droplets, thus preventing the screening of large libraries of drug compounds. The integration of more developed detection techniques, such as machine learning that can recognize complex flow patterns, is needed for sophisticated droplet characterization.199 The increased throughput and promoted detection will facilitate the application of droplet microfluidics in pharmaceutical industry to screen and select high-performance drugs.
Droplet microfluidics promises completion of the whole process from sample preparation to sample analysis in a single device. However, in current microfluidic systems, most droplet assays are still conducted in separate devices and platforms. To achieve integrated operations, the microfluidic devices should be developed into standardized modules for convenient connection. Multiple components, including pumps and detectors, should be miniaturized and assembled into one platform for integrated actuation and control. Such enhanced integration will significantly increase the efficiency, lower the sample loss, and simplify the operations. Moreover, materials that can be more easily manufactured at a large scale should be adopted.200 This will facilitate the development of the integrated systems into commercial instruments, thus allowing their use by some non-experts for wider biomedical applications.
6. Conclusions
Droplet-based microfluidics has inspired new approaches for high-throughput processing and analysis of samples. After two decades of development of generation and manipulation techniques, droplet microfluidics enables synthesis of materials with well-defined structures and novel biotechnologies for lab-on-a-chip applications. However, applying droplet microfluidics in industrial production of lower-value-added materials and wider-ranging analysis of biomedical samples remains challenging. This review introduces the basic principles behind droplet operations and outlines the future directions. With advances in scale-up and integration, droplet microfluidics will be more widely used, facilitating industrial production, promoting clinical diagnoses, and enhancing medical treatments. Overall, droplet microfluidics is destined to remain an important sub-field of microfluidics.
Author contributions
All authors have read, discussed, and contributed to the writing of the manuscript.
Conflicts of interest
The authors declare no competing interests.
Acknowledgements
DAW acknowledges support from the NSF through the Harvard MRSEC (DMR-1420570 and DMR-2011754). HCS acknowledges support from the Croucher Senior Research Fellowship, NSFC Excellent Young Scientists Fund (Hong Kong and Macau) (21922816) as well as Research Grants Council of Hong Kong through the General Research Fund (17304017 and 17307919). In addition, DAW and HCS have benefited from interactions with many current and former members of his group. The work is also supported by the Health@InnoHK Program of the Innovation and Technology Commission of the Hong Kong SAR Government.
References
- T. Thorsen, S. J. Maerkl and S. R. Quake, Science, 2002, 298, 580–584 CrossRef CAS PubMed.
- J. Atencia and D. Beebe, Nature, 2004, 437, 648 CrossRef PubMed.
- A. J. Demello, Nature, 2006, 442, 394–402 CrossRef CAS PubMed.
- L. Shang, Y. Cheng and Y. Zhao, Chem. Rev., 2017, 117, 7964–8040 CrossRef CAS PubMed.
- T. Moragues, D. Arguijo, T. Beneyton, C. Modavi, K. Simutis, A. R. Abate, J.-C. Baret, A. J. deMello, D. Densmore and A. D. Griffiths, Nat. Rev. Methods Primers, 2023, 3, 32 CrossRef CAS.
- M. Joanicot and A. Ajdari, Science, 2005, 309, 887–888 CrossRef CAS PubMed.
- O. Cybulski, P. Garstecki and B. A. Grzybowski, Nat. Phys., 2019, 15, 706–713 Search PubMed.
- A. C. Sun, D. J. Steyer, A. R. Allen, E. M. Payne, R. T. Kennedy and C. R. Stephenson, Nat. Commun., 2020, 11, 6202 Search PubMed.
- E. Z. Macosko, A. Basu, R. Satija, J. Nemesh, K. Shekhar, M. Goldman, I. Tirosh, A. R. Bialas, N. Kamitaki and E. M. Martersteck, Cell, 2015, 161, 1202–1214 CrossRef CAS PubMed.
- I. C. Clark, P. Mudvari, S. Thaploo, S. Smith, M. Abu-Laban, M. Hamouda, M. Theberge, S. Shah, S. H. Ko and L. Pérez, Nature, 2023, 1–8 Search PubMed.
- R. K. Shah, J. W. Kim and D. A. Weitz, Adv. Mater., 2009, 21, 1949–1953 CrossRef CAS.
- J. Zhang, R. J. Coulston, S. T. Jones, J. Geng, O. A. Scherman and C. Abell, Science, 2012, 335, 690–694 CrossRef CAS PubMed.
- P. Zhang and A. R. Abate, Adv. Mater., 2020, 32, 2005346 CrossRef PubMed.
- H. M. Kang, M. Subramaniam, S. Targ, M. Nguyen, L. Maliskova, E. McCarthy, E. Wan, S. Wong, L. Byrnes and C. M. Lanata, Nat. Biotechnol., 2018, 36, 89 CrossRef CAS PubMed.
- M. Niu, W. Cao, Y. Wang, Q. Zhu, J. Luo, B. Wang, H. Zheng, D. A. Weitz and C. Zong, Nat. Biotechnol., 2023, 1–13 Search PubMed.
- D. Qin, Y. Xia and G. M. Whitesides, Nat. Protoc., 2010, 5, 491–502 CrossRef CAS PubMed.
- B. L. Wang, A. Ghaderi, H. Zhou, J. Agresti, D. A. Weitz, G. R. Fink and G. Stephanopoulos, Nat. Biotechnol., 2014, 32, 473 CrossRef CAS PubMed.
- K. C. Bhargava, B. Thompson and N. Malmstadt, Proc. Natl. Acad. Sci. U. S. A., 2014, 111, 15013–15018 CrossRef CAS PubMed.
- H. C. Shum, Y. J. Zhao, S. H. Kim and D. A. Weitz, Angew. Chem., Int. Ed., 2011, 50, 1648–1651 CrossRef CAS PubMed.
- S.-H. Kim, J.-G. Park, T. M. Choi, V. N. Manoharan and D. A. Weitz, Nat. Commun., 2014, 5, 1–8 Search PubMed.
- W.-A. C. Bauer, M. Fischlechner, C. Abell and W. T. Huck, Lab Chip, 2010, 10, 1814–1819 RSC.
- P. Umbanhowar, V. Prasad and D. A. Weitz, Langmuir, 2000, 16, 347–351 CrossRef CAS.
- E. Castro-Hernandez, V. Gundabala, A. Fernández-Nieves and J. M. Gordillo, New J. Phys., 2009, 11, 075021 CrossRef.
- A. S. Utada, A. Fernandez-Nieves, J. M. Gordillo and D. A. Weitz, Phys. Rev. Lett., 2008, 100, 014502 CrossRef PubMed.
- P. Guillot, A. Colin, A. S. Utada and A. Ajdari, Phys. Rev. Lett., 2007, 99, 104502 CrossRef PubMed.
- A. S. Utada, A. Fernandez-Nieves, H. A. Stone and D. A. Weitz, Phys. Rev. Lett., 2007, 99, 094502 CrossRef PubMed.
- A. Utada, L.-Y. Chu, A. Fernandez-Nieves, D. Link, C. Holtze and D. Weitz, MRS Bull., 2007, 32, 702–708 CrossRef CAS.
- G. F. Christopher and S. L. Anna, J. Phys. D: Appl. Phys., 2007, 40, R319 CrossRef CAS.
- L. Yobas, S. Martens, W.-L. Ong and N. Ranganathan, Lab Chip, 2006, 6, 1073–1079 RSC.
- A. M. Ganán-Calvo and J. M. Gordillo, Phys. Rev. Lett., 2001, 87, 274501 CrossRef PubMed.
- A. Utada, E. Lorenceau, D. Link, P. Kaplan, H. A. Stone and D. Weitz, Science, 2005, 308, 537–541 CrossRef CAS PubMed.
- C. Cramer, P. Fischer and E. J. Windhab, Chem. Eng. Sci., 2004, 59, 3045–3058 CrossRef CAS.
- T. Thorsen, R. W. Roberts, F. H. Arnold and S. R. Quake, Phys. Rev. Lett., 2001, 86, 4163 CrossRef CAS PubMed.
- S. L. Anna, N. Bontoux and H. A. Stone, Appl. Phys. Lett., 2003, 82, 364–366 CrossRef CAS.
- Z. Li, A. Leshansky, L. Pismen and P. Tabeling, Lab Chip, 2015, 15, 1023–1031 RSC.
- S.-H. Kim, J. W. Kim, J.-C. Cho and D. A. Weitz, Lab Chip, 2011, 11, 3162–3166 RSC.
- L. Y. Chu, A. S. Utada, R. K. Shah, J. W. Kim and D. A. Weitz, Angew. Chem., Int. Ed., 2007, 46, 8970–8974 CrossRef CAS PubMed.
- W. Wang, R. Xie, X.-J. Ju, T. Luo, L. Liu, D. A. Weitz and L.-Y. Chu, Lab Chip, 2011, 11, 1587–1592 RSC.
- P. Garstecki, M. J. Fuerstman, H. A. Stone and G. M. Whitesides, Lab Chip, 2006, 6, 437–446 RSC.
- N. Tarchichi, F. Chollet and J.-F. Manceau, Microfluid. Nanofluid., 2013, 14, 45–51 CrossRef CAS.
- M. Pathak, J. Membr. Sci., 2011, 382, 166–176 CrossRef CAS.
- W. Lee, L. M. Walker and S. L. Anna, Phys. Fluids, 2009, 21, 032103 CrossRef.
- T. Fu, Y. Wu, Y. Ma and H. Z. Li, Chem. Eng. Sci., 2012, 84, 207–217 CrossRef CAS.
- M. De Menech, P. Garstecki, F. Jousse and H. Stone, J. Fluid Mech., 2008, 595, 141–161 CrossRef.
- B. Dollet, W. Van Hoeve, J.-P. Raven, P. Marmottant and M. Versluis, Phys.
Rev. Lett., 2008, 100, 034504 CrossRef PubMed.
- S. L. Anna and H. C. Mayer, Phys. Fluids, 2006, 18, 121512 CrossRef.
- P. Garstecki, H. A. Stone and G. M. Whitesides, Phys. Rev. Lett., 2005, 94, 164501 CrossRef PubMed.
- A. Abate and D. Weitz, Small, 2009, 5, 2030–2032 CrossRef CAS PubMed.
- M. L. Eggersdorfer, H. Seybold, A. Ofner, D. A. Weitz and A. R. Studart, Proc. Natl. Acad. Sci. U. S. A., 2018, 115, 9479–9484 CrossRef CAS PubMed.
- A. Ofner, D. G. Moore, P. A. Rühs, P. Schwendimann, M. Eggersdorfer, E. Amstad, D. A. Weitz and A. R. Studart, Macromol. Chem. Phys., 2017, 218, 1600472 CrossRef.
- E. Amstad, M. Chemama, M. Eggersdorfer, L. R. Arriaga, M. P. Brenner and D. A. Weitz, Lab Chip, 2016, 16, 4163–4172 RSC.
- Y. Chao and H. C. Shum, Chem. Soc. Rev., 2020, 49, 114–142 RSC.
- L. Nan, Y. Cao, S. Yuan and H. C. Shum, Microsyst. Nanoeng., 2020, 6, 1–10 CrossRef PubMed.
- H. T. Liu, H. Wang, W. B. Wei, H. Liu, L. Jiang and J. H. Qin, Small, 2018, 14, 1801095 CrossRef PubMed.
- S. Xu, Z. Nie, M. Seo, P. Lewis, E. Kumacheva, H. A. Stone, P. Garstecki, D. B. Weibel, I. Gitlin and G. M. Whitesides, Angew. Chem., Int. Ed., 2005, 44, 724–728 CrossRef CAS PubMed.
- Y. Liu, Y. Cheng, C. Zhao, H. Wang and Y. Zhao, Adv. Sci., 2022, 9, 2104272 CrossRef CAS PubMed.
- X. Zhao, S. Liu, L. Yildirimer, H. Zhao, R. Ding, H. Wang, W. Cui and D. Weitz, Adv. Funct. Mater., 2016, 26, 2809–2819 CrossRef CAS.
- S. Ma, J. Thiele, X. Liu, Y. Bai, C. Abell and W. T. Huck, Small, 2012, 8, 2356–2360 CrossRef CAS PubMed.
- C. Zhou, P. Zhu, Y. Tian, M. Xu and L. Wang, ACS Nano, 2019, 13, 6319–6329 CrossRef CAS PubMed.
- A. S. Mao, J.-W. Shin, S. Utech, H. Wang, O. Uzun, W. Li, M. Cooper, Y. Hu, L. Zhang and D. A. Weitz, Nat. Mater., 2017, 16, 236–243 CrossRef CAS PubMed.
- R. Novak, Y. Zeng, J. Shuga, G. Venugopalan, D. A. Fletcher, M. T. Smith and R. A. Mathies, Angew. Chem., Int. Ed., 2011, 50, 390–395 CrossRef CAS PubMed.
- W. H. Tan and S. Takeuchi, Adv. Mater., 2007, 19, 2696–2701 CrossRef CAS.
- S. Utech, R. Prodanovic, A. S. Mao, R. Ostafe, D. J. Mooney and D. A. Weitz, Adv. Healthcare Mater., 2015, 4, 1628–1633 CrossRef CAS PubMed.
- X. Yin, B. Chen, M. He and B. Hu, Anal. Chem., 2022, 94, 6582–6590 CrossRef CAS PubMed.
- Q. Xu, M. Hashimoto, T. T. Dang, T. Hoare, D. S. Kohane, G. M. Whitesides, R. Langer and D. G. Anderson, Small, 2009, 5, 1575–1581 CrossRef CAS PubMed.
- M. F. Haase and J. Brujic, Angew. Chem., Int. Ed., 2014, 53, 11793–11797 CrossRef CAS PubMed.
- R. Ameloot, F. Vermoortele, W. Vanhove, M. B. Roeffaers, B. F. Sels and D. E. De Vos, Nat. Chem., 2011, 3, 382–387 CrossRef CAS PubMed.
- S. Seiffert, J. Thiele, A. R. Abate and D. A. Weitz, J. Am. Chem. Soc., 2010, 132, 6606–6609 CrossRef CAS PubMed.
- J. Wang, S. Hahn, E. Amstad and N. Vogel, Adv. Mater., 2022, 34, 2107338 CrossRef CAS PubMed.
- S. H. Kim and D. A. Weitz, Angew. Chem., 2011, 123, 8890–8893 CrossRef.
- H. Wang, H. Liu, H. Liu, W. Su, W. Chen and J. Qin, Adv. Mater. Technol., 2019, 4, 1800632 CrossRef.
- A. Abbaspourrad, S. S. Datta and D. A. Weitz, Langmuir, 2013, 29, 12697–12702 CrossRef CAS PubMed.
- A. Yashina, I. Lignos, S. Stavrakis, J. Choo and A. J. deMello, J. Mater. Chem. C, 2016, 4, 6401–6408 RSC.
- A. B. Theberge, E. Mayot, A. El Harrak, F. Kleinschmidt, W. T. Huck and A. D. Griffiths, Lab Chip, 2012, 12, 1320–1326 RSC.
- W. Li, J. Greener, D. Voicu and E. Kumacheva, Lab Chip, 2009, 9, 2715–2721 RSC.
- S. Tcholakova, Z. Valkova, D. Cholakova, Z. Vinarov, I. Lesov, N. Denkov and S. K. Smoukov, Nat. Commun., 2017, 8, 1–11 CrossRef PubMed.
- R. Vasiliauskas, D. Liu, S. Cito, H. Zhang, M.-A. Shahbazi, T. Sikanen, L. Mazutis and H. L. A. Santos, ACS Appl. Mater. Interfaces, 2015, 7, 14822–14832 CrossRef CAS PubMed.
- L. Nan, Z. Yang, H. Lyu, K. Y. Y. Lau and H. C. Shum, Adv. Biosyst., 2019, 1900076 CrossRef PubMed.
- H. Zhang, D. Liu, M. A. Shahbazi, E. Mäkilä, B. Herranz-Blanco, J. Salonen, J. Hirvonen and H. A. Santos, Adv. Mater., 2014, 26, 4497–4503 CrossRef CAS PubMed.
- Y. Wang, M. Liu, Y. Zhang, H. Liu and L. Han, Lab Chip, 2023, 23, 1080–1096 RSC.
- H. C. Shum, D. Lee, I. Yoon, T. Kodger and D. A. Weitz, Langmuir, 2008, 24, 7651–7653 CrossRef CAS PubMed.
- H. C. Shum, J.-W. Kim and D. A. Weitz, J. Am. Chem. Soc., 2008, 130, 9543–9549 CrossRef CAS PubMed.
- N. N. Deng and W. T. Huck, Angew. Chem., Int. Ed., 2017, 56, 9736–9740 CrossRef CAS PubMed.
- A. Abbaspourrad, N. J. Carroll, S.-H. Kim and D. A. Weitz, J. Am. Chem. Soc., 2013, 135, 7744–7750 CrossRef CAS PubMed.
- T. Watanabe, C. G. Lopez, J. F. Douglas, T. Ono and J. O. T. Cabral, Langmuir, 2014, 30, 2470–2479 CrossRef CAS PubMed.
- S. Guo, T. Yao, X. Ji, C. Zeng, C. Wang and L. Zhang, Angew. Chem., 2014, 126, 7634–7639 CrossRef.
- M. Windbergs, Y. Zhao, J. Heyman and D. A. Weitz, J. Am. Chem. Soc., 2013, 135, 7933–7937 CrossRef CAS PubMed.
- W. Zhang, A. Abbaspourrad, D. Chen, E. Campbell, H. Zhao, Y. Li, Q. Li and D. A. Weitz, Adv. Funct. Mater., 2017, 27, 1700975 CrossRef.
- J. Zhou, D. C. Hyun, H. Liu, H. Wu and Y. Xia, Macromol. Rapid Commun., 2014, 35, 1436–1442 CrossRef CAS PubMed.
- E. Amstad, S. H. Kim and D. A. Weitz, Angew. Chem., Int. Ed., 2012, 51, 12499–12503 CrossRef CAS PubMed.
- B. K. Yen, A. Günther, M. A. Schmidt, K. F. Jensen and M. G. Bawendi, Angew. Chem., 2005, 117, 5583–5587 CrossRef.
- N. Visaveliya and J. M. Köhler, Small, 2015, 11, 6435–6443 CrossRef CAS PubMed.
- Y. Liu, J. Du, J. S. Choi, K. J. Chen, S. Hou, M. Yan, W. Y. Lin, K. S. Chen, T. Ro and G. S. Lipshutz, Angew. Chem., 2016, 128, 177–181 CrossRef.
- L. Frenz, A. El Harrak, M. Pauly, S. Bégin-Colin, A. D. Griffiths and J. C. Baret, Angew. Chem., Int. Ed., 2008, 47, 6817–6820 CrossRef CAS PubMed.
- A. M. Nightingale, T. W. Phillips, J. H. Bannock and J. C. De Mello, Nat. Commun., 2014, 5, 1–8 Search PubMed.
- A. Knauer, A. Thete, S. Li, H. Romanus, A. Csaki, W. Fritzsche and J. Köhler, Chem. Eng. J., 2011, 166, 1164–1169 CrossRef CAS.
- T. Moragues, S. Mitchell, D. Faust Akl, J. Pérez-Ramírez and A. deMello, Small Struct., 2023, 4, 2200284 CrossRef CAS.
- J. Clausell-Tormos, A. D. Griffiths and C. A. Merten, Lab Chip, 2010, 10, 1302–1307 RSC.
- T. Nisisako, T. Ando and T. Hatsuzawa, Lab Chip, 2012, 12, 3426–3435 RSC.
- C. T. Riche, E. J. Roberts, M. Gupta, R. L. Brutchey and N. Malmstadt, Nat. Commun., 2016, 7, 1–7 Search PubMed.
- H.-H. Jeong, V. R. Yelleswarapu, S. Yadavali, D. Issadore and D. Lee, Lab Chip, 2015, 15, 4387–4392 RSC.
- A. Vian, B. Reuse and E. Amstad, Lab Chip, 2018, 18, 1936–1942 RSC.
- H. Yi, S. Lu, J. Wu, Y. Wang and G. Luo, Particuology, 2022, 62, 47–54 CrossRef CAS.
- M. R. Bringer, C. J. Gerdts, H. Song, J. D. Tice and R. F. Ismagilov, Philos. Trans. R. Soc., A, 2004, 362, 1087–1104 CrossRef CAS PubMed.
- H. Song, J. D. Tice and R. F. Ismagilov, Angew. Chem., Int. Ed., 2003, 42, 768–772 CrossRef CAS PubMed.
- A. Liau, R. Karnik, A. Majumdar and J. H. D. Cate, Anal. Chem., 2005, 77, 7618–7625 CrossRef CAS PubMed.
- M. L. Cordero, H. O. Rolfsnes, D. R. Burnham, P. A. Campbell, D. McGloin and C. N. Baroud, New J. Phys., 2009, 11, 075033 CrossRef.
- G. Yesiloz, M. S. Boybay and C. L. Ren, Anal. Chem., 2017, 89, 1978–1984 CrossRef CAS PubMed.
- P. Mary, V. Studer and P. Tabeling, Anal. Chem., 2008, 80, 2680–2687 CrossRef CAS PubMed.
- P. Wägli, Y.-C. Chang, A. Homsy, L. Hvozdara, H. P. Herzig and N. F. De Rooij, Anal. Chem., 2013, 85, 7558–7565 CrossRef PubMed.
- J.-C. Baret, O. J. Miller, V. Taly, M. Ryckelynck, A. El-Harrak, L. Frenz, C. Rick, M. L. Samuels, J. B. Hutchison and J. J. Agresti, Lab Chip, 2009, 9, 1850–1858 RSC.
- N. Bremond, A. R. Thiam and J. Bibette, Phys. Rev. Lett., 2008, 100, 024501 CrossRef PubMed.
- L. M. Fidalgo, G. Whyte, D. Bratton, C. F. Kaminski, C. Abell and W. T. Huck, Angew. Chem., 2008, 120, 2072–2075 CrossRef.
- A. Huebner, D. Bratton, G. Whyte, M. Yang, C. Abell and F. Hollfelder, Lab Chip, 2009, 9, 692–698 RSC.
- S. K. Cho, H. Moon and C.-J. Kim, J. Microelectromech. Syst., 2003, 12, 70–80 CrossRef.
- A. Sciambi and A. R. Abate, Lab Chip, 2015, 15, 47–51 RSC.
- K. Ahn, C. Kerbage, T. P. Hunt, R. Westervelt, D. R. Link and D. A. Weitz, Appl. Phys. Lett., 2006, 88, 024104 CrossRef.
- J. Panwar, A. Autour and C. A. Merten, Nat. Protoc., 2023, 18, 1090–1136 CrossRef CAS PubMed.
- L. Schmid, D. A. Weitz and T. Franke, Lab Chip, 2014, 14, 3710–3718 RSC.
- T. Franke, A. R. Abate, D. A. Weitz and A. Wixforth, Lab Chip, 2009, 9, 2625–2627 RSC.
- E. S. Richter, A. Link, J. S. McGrath, R. W. Sparrow, M. Gantz, E. J. Medcalf, F. Hollfelder and T. Franke, Lab Chip, 2023, 23, 195–202 RSC.
- A. R. Abate, J. J. Agresti and D. A. Weitz, Appl. Phys. Lett., 2010, 96, 203509 CrossRef.
- L. Nan, M. Y. A. Lai, M. Y. H. Tang, Y. K. Chan, L. L. M. Poon and H. C. Shum, Small, 2020, 16, 1902889 CrossRef CAS PubMed.
- R. H. Cole, S.-Y. Tang, C. A. Siltanen, P. Shahi, J. Q. Zhang, S. Poust, Z. J. Gartner and A. R. Abate, Proc. Natl. Acad. Sci. U. S. A., 2017, 114, 8728–8733 CrossRef CAS PubMed.
- M. Chabert and J.-L. Viovy, Proc. Natl. Acad. Sci. U. S. A., 2008, 105, 3191–3196 CrossRef CAS PubMed.
- A. C. Hatch, A. Patel, N. R. Beer and A. P. Lee, Lab Chip, 2013, 13, 1308–1315 RSC.
- D. Link, S. L. Anna, D. Weitz and H. A. Stone, Phys. Rev. Lett., 2004, 92, 054503 CrossRef CAS PubMed.
- X. Niu, F. Gielen, J. B. Edel and A. J. Demello, Nat. Chem., 2011, 3, 437 CrossRef CAS PubMed.
- Y.-C. Tan, J. S. Fisher, A. I. Lee, V. Cristini and A. P. Lee, Lab Chip, 2004, 4, 292–298 RSC.
- L. Mazutis, J.-C. Baret, P. Treacy, Y. Skhiri, A. F. Araghi, M. Ryckelynck, V. Taly and A. D. Griffiths, Lab Chip, 2009, 9, 2902–2908 RSC.
- L. Nan, T. Mao and H. C. Shum, Microsyst. Nanoeng., 2023, 9, 24 CrossRef CAS PubMed.
- H. Babahosseini, T. Misteli and D. L. DeVoe, Lab Chip, 2019, 19, 493–502 RSC.
- H. S. Kim, A. R. Guzman, H. R. Thapa, T. P. Devarenne and A. Han, Biotechnol. Bioeng., 2016, 113, 1691–1701 CrossRef CAS PubMed.
- A. R. Abate, T. Hung, P. Mary, J. J. Agresti and D. A. Weitz, Proc. Natl. Acad. Sci. U. S. A., 2010, 107, 19163–19166 CrossRef CAS PubMed.
- H. Yuan, Y. Chao, S. Li, M. Y. Tang, Y. Huang, Y. Che, A. S. Wong, T. Zhang and H. C. Shum, Anal. Chem., 2018, 90, 13173–13177 CrossRef CAS PubMed.
- I. C. Clark, C. Gutiérrez-Vázquez, M. A. Wheeler, Z. Li, V. Rothhammer, M. Linnerbauer, L. M. Sanmarco, L. Guo, M. Blain and S. E. Zandee, Science, 2021, 372(6540), eabf1230 CrossRef CAS PubMed.
- G. T. Roman, M. Wang, K. N. Shultz, C. Jennings and R. T. Kennedy, Anal. Chem., 2008, 80, 8231–8238 CrossRef CAS PubMed.
- Y. Zhu and Q. Fang, Anal. Chem., 2010, 82, 8361–8366 CrossRef CAS PubMed.
- T. Xu, Y. Gong, X. Su, P. Zhu, J. Dai, J. Xu and B. Ma, Small, 2020, 16, 2001172 CrossRef CAS PubMed.
- W. Wang, C. Yang and C. M. Li, Lab Chip, 2009, 9, 1504–1506 RSC.
- S. H. Han, Y. Choi, J. Kim and D. Lee, ACS Appl. Mater. Interfaces, 2020, 12, 3936–3944 CrossRef CAS PubMed.
- M. G. Simon, R. Lin, J. S. Fisher and A. P. Lee, Biomicrofluidics, 2012, 6, 014110 CrossRef PubMed.
- H.-H. Jeong, B. Lee, S. H. Jin, S.-G. Jeong and C.-S. Lee, Lab Chip, 2016, 16, 1698–1707 RSC.
- K. Leung, H. Zahn, T. Leaver, K. M. Konwar, N. W. Hanson, A. P. Pagé, C.-C. Lo, P. S. Chain, S. J. Hallam and C. L. Hansen, Proc. Natl. Acad. Sci. U. S. A., 2012, 109, 7665–7670 CrossRef CAS PubMed.
- J. Li, N. S. Ha and R. M. van Dam, Nature, 2019, 572, 507–510 CrossRef CAS PubMed.
- M. G. Pollack, A. D. Shenderov and R. B. Fair, Lab Chip, 2002, 2, 96–101 RSC.
- P. Paik, V. K. Pamula and R. B. Fair, Lab Chip, 2003, 3, 253–259 RSC.
- P. Paik, V. K. Pamula, M. G. Pollack and R. B. Fair, Lab Chip, 2003, 3, 28–33 RSC.
- J. Clausell-Tormos, D. Lieber, J.-C. Baret, A. El-Harrak, O. J. Miller, L. Frenz, J. Blouwolff, K. J. Humphry, S. Köster and H. Duan, Chem. Biol., 2008, 15, 427–437 CrossRef CAS PubMed.
- S. Köster, F. E. Angile, H. Duan, J. J. Agresti, A. Wintner, C. Schmitz, A. C. Rowat, C. A. Merten, D. Pisignano and A. D. Griffiths, Lab Chip, 2008, 8, 1110–1115 RSC.
- A. Rotem, O. Ram, N. Shoresh, R. A. Sperling, A. Goren, D. A. Weitz and B. E. Bernstein, Nat. Biotechnol., 2015, 33, 1165–1172 CrossRef CAS PubMed.
- R. Zilionis, J. Nainys, A. Veres, V. Savova, D. Zemmour, A. M. Klein and L. Mazutis, Nat. Protoc., 2017, 12, 44 CrossRef CAS PubMed.
- G. X. Zheng, J. M. Terry, P. Belgrader, P. Ryvkin, Z. W. Bent, R. Wilson, S. B. Ziraldo, T. D. Wheeler, G. P. McDermott and J. Zhu, Nat. Commun., 2017, 8, 1–12 CrossRef PubMed.
- I. Setliff, A. R. Shiakolas, K. A. Pilewski, A. A. Murji, R. E. Mapengo, K. Janowska, S. Richardson, C. Oosthuysen, N. Raju and L. Ronsard, Cell, 2019, 179, 1636–1646.e1615 CrossRef CAS PubMed.
- C. Sun, L. Liu, L. Pérez, X. Li, Y. Liu, P. Xu, E. A. Boritz, J. I. Mullins and A. R. Abate, Nat. Biomed. Eng., 2022, 1–9 Search PubMed.
- A. M. Klein, L. Mazutis, I. Akartuna, N. Tallapragada, A. Veres, V. Li, L. Peshkin, D. A. Weitz and M. W. Kirschner, Cell, 2015, 161, 1187–1201 CrossRef CAS PubMed.
- K. Grosselin, A. Durand, J. Marsolier, A. Poitou, E. Marangoni, F. Nemati, A. Dahmani, S. Lameiras, F. Reyal and O. Frenoy, Nat. Genet., 2019, 51, 1060–1066 CrossRef CAS PubMed.
- A. S. Nam, K.-T. Kim, R. Chaligne, F. Izzo, C. Ang, J. Taylor, R. M. Myers, G. Abu-Zeinah, R. Brand and N. D. Omans, Nature, 2019, 571, 355–360 CrossRef CAS PubMed.
- F. Lan, B. Demaree, N. Ahmed and A. R. Abate, Nat. Biotechnol., 2017, 35, 640–646 CrossRef CAS PubMed.
- P. Ma, H. M. Amemiya, L. L. He, S. J. Gandhi, R. Nicol, R. P. Bhattacharyya, C. S. Smillie and D. T. Hung, Cell, 2023, 186, 877–891.e814 CrossRef CAS PubMed.
- L. Mazutis, J. Gilbert, W. L. Ung, D. A. Weitz, A. D. Griffiths and J. A. Heyman, Nat. Protoc., 2013, 8, 870–891 CrossRef CAS PubMed.
- V. Yelleswarapu, J. R. Buser, M. Haber, J. Baron, E. Inapuri and D. Issadore, Proc. Natl. Acad. Sci. U. S. A., 2019, 116, 4489–4495 CrossRef CAS PubMed.
- E. Brouzes, M. Medkova, N. Savenelli, D. Marran, M. Twardowski, J. B. Hutchison, J. M. Rothberg, D. R. Link, N. Perrimon and M. L. Samuels, Proc. Natl. Acad. Sci. U. S. A., 2009, 106, 14195–14200 CrossRef CAS PubMed.
- J. J. Agresti, E. Antipov, A. R. Abate, K. Ahn, A. C. Rowat, J.-C. Baret, M. Marquez, A. M. Klibanov, A. D. Griffiths and D. A. Weitz, Proc. Natl. Acad. Sci. U. S. A., 2010, 107, 4004–4009 CrossRef CAS PubMed.
- A. Autour, E. Westhof and M. Ryckelynck, Nucleic Acids Res., 2016, 44, 2491–2500 CrossRef PubMed.
- R. Rosenthal, X. Zhang, K. Ilic Durdic, J. J. Collins and D. A. Weitz, Angew. Chem., 2023, e202303112 CAS.
- S. S. Terekhov, I. V. Smirnov, A. V. Stepanova, T. V. Bobik, Y. A. Mokrushina, N. A. Ponomarenko, A. A. Belogurov, M. P. Rubtsova, O. V. Kartseva and M. O. Gomzikova, Proc. Natl. Acad. Sci. U. S. A., 2017, 114, 2550–2555 CrossRef CAS PubMed.
- J. L. Madrigal, N. G. Schoepp, L. Xu, C. S. Powell, C. L. Delley, C. A. Siltanen, J. Danao, M. Srinivasan, R. H. Cole and A. R. Abate, Proc. Natl. Acad. Sci. U. S. A., 2022, 119, e2110867119 CrossRef CAS PubMed.
- M. A. Wheeler, I. C. Clark, H.-G. Lee, Z. Li, M. Linnerbauer, J. M. Rone, M. Blain, C. F. Akl, G. Piester and F. Giovannoni, Science, 2023, 379, 1023–1030 CrossRef CAS PubMed.
- W. Zheng, S. Zhao, Y. Yin, H. Zhang, D. M. Needham, E. D. Evans, C. L. Dai, P. J. Lu, E. J. Alm and D. A. Weitz, Science, 2022, 376, eabm1483 CrossRef CAS PubMed.
- K.-Y. Chen, J. Karuppusamy, M. B. O'Neill, V. Opuu, M. Bahin, S. Foulon, P. Ibanez, L. Quintana-Murci, T. Ozawa and S. van der Werf, Proc. Natl. Acad. Sci. U. S. A., 2023, 120, e2211098120 CrossRef CAS PubMed.
- G. Sun, L. Qu, F. Azi, Y. Liu, J. Li, X. Lv, G. Du, J. Chen, C.-H. Chen and L. Liu, Biosens. Bioelectron., 2023, 115107 CrossRef CAS PubMed.
- D. Xu, W. Zhang, H. Li, N. Li and J.-M. Lin, Lab Chip, 2023, 23, 1258–1278 RSC.
- R. Tewhey, J. B. Warner, M. Nakano, B. Libby, M. Medkova, P. H. David, S. K. Kotsopoulos, M. L. Samuels, J. B. Hutchison and J. W. Larson, Nat. Biotechnol., 2009, 27, 1025–1031 CrossRef CAS PubMed.
- B. J. Hindson, K. D. Ness, D. A. Masquelier, P. Belgrader, N. J. Heredia, A. J. Makarewicz, I. J. Bright, M. Y. Lucero, A. L. Hiddessen and T. C. Legler, Anal. Chem., 2011, 83, 8604–8610 CrossRef CAS PubMed.
- C. M. Hindson, J. R. Chevillet, H. A. Briggs, E. N. Gallichotte, I. K. Ruf, B. J. Hindson, R. L. Vessella and M. Tewari, Nat. Methods, 2013, 10, 1003 CrossRef CAS PubMed.
- S. C. Taylor, J. Carbonneau, D. N. Shelton and G. Boivin, J. Virol. Methods, 2015, 224, 58–66 CrossRef CAS PubMed.
- T. Suo, X. Liu, J. Feng, M. Guo, W. Hu, D. Guo, H. Ullah, Y. Yang, Q. Zhang and X. Wang, Emerging Microbes Infect., 2020, 9, 1259–1268 CrossRef CAS PubMed.
- N. Rački, T. Dreo, I. Gutierrez-Aguirre, A. Blejec and M. Ravnikar, Plant Methods, 2014, 10, 1–10 CrossRef PubMed.
- M. Y. Tang and H. C. Shum, Lab Chip, 2016, 16, 4359–4365 RSC.
- L. Cohen, N. Cui, Y. Cai, P. M. Garden, X. Li, D. A. Weitz and D. R. Walt, ACS Nano, 2020, 14(8), 9491–9501 CrossRef CAS PubMed.
- O. J. Miller, A. El Harrak, T. Mangeat, J.-C. Baret, L. Frenz, B. El Debs, E. Mayot, M. L. Samuels, E. K. Rooney and P. Dieu, Proc. Natl. Acad. Sci. U. S. A., 2012, 109, 378–383 CrossRef CAS PubMed.
- B. El Debs, R. Utharala, I. V. Balyasnikova, A. D. Griffiths and C. A. Merten, Proc. Natl. Acad. Sci. U. S. A., 2012, 109, 11570–11575 CrossRef CAS PubMed.
- A. Kulesa, J. Kehe, J. E. Hurtado, P. Tawde and P. C. Blainey, Proc. Natl. Acad. Sci. U. S. A., 2018, 115, 6685–6690 CrossRef PubMed.
- M. Huang, Y. Bai, S. L. Sjostrom, B. M. Hallström, Z. Liu, D. Petranovic, M. Uhlén, H. N. Joensson, H. Andersson-Svahn and J. Nielsen, Proc. Natl. Acad. Sci. U. S. A., 2015, 112, E4689–E4696 CAS.
- W. Postek and P. Garstecki, Acc. Chem. Res., 2022, 55, 605–615 CrossRef CAS PubMed.
- B. Wang, C. Zhao, Z. Wang, K.-A. Yang, X. Cheng, W. Liu, W. Yu, S. Lin, Y. Zhao and K. M. Cheung, Sci. Adv., 2022, 8, eabk0967 CrossRef CAS PubMed.
- L. Xu, K.-C. Chang, E. M. Payne, C. Modavi, L. Liu, C. M. Palmer, N. Tao, H. S. Alper, R. T. Kennedy and D. S. Cornett, Nat. Commun., 2021, 12, 6803 CrossRef CAS PubMed.
- L. Xu, X. Li, W. Li, K. C. Chang, H. Yang, N. Tao, P. Zhang, E. Payne, C. Modavi and J. Humphries, Adv. Mater., 2021, 2108194 Search PubMed.
- C. Sun, L. Liu, L. Pérez, X. Li, Y. Liu, P. Xu, E. A. Boritz, J. I. Mullins and A. R. Abate, Nat. Biomed. Eng., 2022, 6, 1004 CrossRef CAS PubMed.
- J. De Jonghe, T. S. Kaminski, D. B. Morse, M. Tabaka, A. L. Ellermann, T. N. Kohler, G. Amadei, C. E. Handford, G. M. Findlay and M. Zernicka-Goetz, Nat. Commun., 2023, 14, 4788 CrossRef CAS PubMed.
- A. Gérard, A. Woolfe, G. Mottet, M. Reichen, C. Castrillon, V. Menrath, S. Ellouze, A. Poitou, R. Doineau and L. Briseno-Roa, Nat. Biotechnol., 2020, 38, 715–721 CrossRef PubMed.
- I. C. Clark, M. A. Wheeler, H.-G. Lee, Z. Li, L. M. Sanmarco, S. Thaploo, C. M. Polonio, S. W. Shin, G. Scalisi and A. R. Henry, Nature, 2023, 1–3 Search PubMed.
- Y. Wang, J. Li, L. Sun, H. Chen, F. Ye, Y. Zhao and L. Shang, Adv. Mater., 2023, 35, 2211731 CrossRef CAS PubMed.
- X. Li, B. You, H. C. Shum and C.-H. Chen, Biomaterials, 2022, 287, 121631 CrossRef CAS PubMed.
- D. Hess, V. Dockalova, P. Kokkonen, D. Bednar, J. Damborsky, A. DeMello, Z. Prokop and S. Stavrakis, Chem, 2021, 7, 1066–1079 CAS.
- K. Gardner, M. M. Uddin, L. Tran, T. Pham, S. Vanapalli and W. Li, Lab Chip, 2022, 22, 4067–4080 RSC.
- H. Liu, L. Nan, F. Chen, Y. Zhao and Y. Zhao, Lab Chip, 2023, 23, 2497–2513 RSC.
- R. Vinuesa and S. L. Brunton, Nat. Comput. Sci., 2022, 2, 358–366 CrossRef.
- J. Wu, D. A. Issadore and D. Lee, ACS Appl. Mater. Interfaces, 2023, 15, 10212–10218 CrossRef CAS PubMed.
|
This journal is © The Royal Society of Chemistry 2024 |
Click here to see how this site uses Cookies. View our privacy policy here.