DOI:
10.1039/D4FD00076E
(Paper)
Faraday Discuss., 2025, Advance Article
Crystal structure validation of verinurad via proton-detected ultra-fast MAS NMR and machine learning†
Received
15th April 2024
, Accepted 7th May 2024
First published on 17th July 2024
Abstract
The recent development of ultra-fast magic-angle spinning (MAS) (>100 kHz) provides new opportunities for structural characterization in solids. Here, we use NMR crystallography to validate the structure of verinurad, a microcrystalline active pharmaceutical ingredient. To do this, we take advantage of 1H resolution improvement at ultra-fast MAS and use solely 1H-detected experiments and machine learning methods to assign all the experimental proton and carbon chemical shifts. This framework provides a new tool for elucidating chemical information from crystalline samples with limited sample volume and yields remarkably faster acquisition times compared to 13C-detected experiments, without the need to employ dynamic nuclear polarization.
Introduction
It is impossible to imagine any new drug development pipeline nowadays without a rigorous structure characterization step. This is partly due to the need for structure–activity relationships, partly due to concerns around stability and/or polymorphism, and partly due to regulatory pressure. Many active pharmaceutical ingredients (APIs) and drug formulations are powdered solids in their final form, so structural investigation in the native state requires well-established solid-state techniques capable of providing atomic-level resolution.
To this end, single-crystal X-ray diffraction (SXRD) is the method of choice.1–7 However, in the case of powders, SXRD is not appropriate, and powder X-ray diffraction (PXRD),8–10 electron diffraction11–16 and/or solid-state NMR spectroscopy17–30 are typically used instead. However, PXRD may suffer from peak broadening and spectral overlap due to low crystallinity,31 to the extent that the structure cannot be determined. Consequently, NMR can be used either to determine the crystal structure de novo,32–37 or to refine38–45 or validate46–48 a structure determined by X-ray diffraction methods.
The applicability of NMR crystallography to solve structures at the atomic level originates from the fact that the chemical shift is highly sensitive to the local atomic environment and can therefore report on the neighboring atoms and their spatial distribution.26,33,37,46,49–55 Using NMR crystallography, it is possible to investigate a broad range of solids extending from small organic molecules,32,33,37 to biomolecules,56,57 inorganic materials58–66 and even amorphous drugs.49,50 Atomic-level structures have been determined de novo from NMR chemical shifts in crystalline compounds for molecular solids,32–34,37 enzyme active sites,41,67,68 photovoltaic materials,59,60,63 and cementitious materials.61,64,66 NMR crystallography is extensively used not only to validate or determine the structure, but also to distinguish between multiple polymorphs of the same molecule.69–77
In chemical-shift-based NMR crystallography, the experimentally determined chemical shifts are compared to those calculated for (a set of) candidate crystal structures, and the candidate structure(s) with the lowest root-mean-square chemical-shift deviation (RMSD) is (are) then determined to be the true experimental structure. Confidence in the structure and positional uncertainties can then be determined using statistical methods.78,79 For validation, the candidate structure would typically have been determined by SXRD. For de novo determination, candidate structures are typically generated using crystal structure prediction (CSP)80–84 or molecular dynamics (MD)85,86 with a subsequent selection step of candidates having the lowest predicted lattice energy. For each of the candidates, chemical shifts are computed using density functional theory (DFT) methods, such as plane-wave gauge-including projector augmented wave (GIPAW) methods.87–89
Much progress has been made in solid-state NMR in the last 80 years that aims not only at extending its applications, but also at speeding up the NMR crystallography workflow. Tremendous advances have been achieved using machine-learning approaches that provide rapid chemical-shift predictions for molecular solids with DFT accuracy (dubbed ShiftML and ShiftML2).90,91 By combining ShiftML with the Cambridge Structural Database of three-dimensional structures, Cordova et al. developed a method for automated probabilistic assignment of the experimental chemical shifts for organic solids directly from their two-dimensional molecular structures.92 Due to hardware advances, we are now able to reach magic-angle spinning (MAS) rates of above 100 kHz. In combination with 1H resolution improvement techniques,93–98 ultra-fast MAS results in sufficient 1H line narrowing99,100 for the acquisition of high-resolution 1D and 2D 1H-detected spectra for typical molecular solids. For such materials, transitioning to 1H detection instead of 13C detection translates to faster acquisition due to the high sensitivity of protons, and no need for costly isotopic labelling or for hyperpolarization by dynamic nuclear polarization (DNP), which remain inaccessible for many NMR platforms around the world.
Here we demonstrate an NMR chemical-shift-led approach based on 1H detection at fast-MAS, in combination with the probabilistic assignment method, and machine-learned chemical shifts, to validate crystal structures. The method is illustrated with the validation of the structure of verinurad (RDEA3170) shown in Fig. 1A, a powerful inhibitor of the URAT1 uric acid transporter with potential pharmaceutical applications against hyperuricemia and gout.101,102
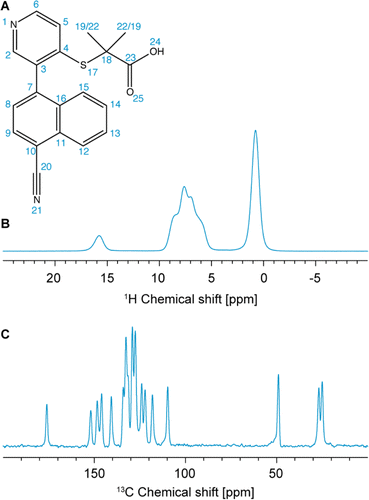 |
| Fig. 1 (A) Chemical structure of verinurad and labels corresponding to each atomic site. (B) 1D 1H spectrum at 160 kHz MAS. (C) 13C CP spectrum at 100 kHz MAS. | |
Previously, 18 solvates were found by a polymorph screen, of which anhydrous form A is the most stable.103 The latter exists as a racemic mixture of atropisomers due to restricted rotation about the cyano-naphthalene and pyridine rings (Fig. 1A). The crystal structure of form A was determined using SXRD, and it was found that it belongs to the P21/n centrosymmetric space group with one molecule in the asymmetric unit.103 Importantly, for the assignment of the experimental chemical shifts, we rely solely on the 1H-detected 1D spectrum, and 1H-detected 2D hCH and 1H–1H DQ/SQ experiments at 160 kHz MAS, and on the probabilistic assignment of 1H and 13C shifts. The acquisition time needed for these experiments is roughly three times shorter than that needed to acquire a 13C DNP-refocused INADEQUATE. The information contained in the INADEQUATE spectrum that we would otherwise need to guide the assignment is replaced by the probabilistic assignment, which is sufficient to unambiguously assign 18 out of 20 13C chemical shifts and 10 out of 12 1H chemical shifts. The remaining carbons and protons corresponding to the two methyl groups have very similar local bonding environments, which makes their corresponding statistical distributions of chemical shifts very similar. Therefore, they are left ambiguously assigned. The experimental shifts are then compared to those predicted for the SXRD structure using ShiftML2 to validate the crystal structure. We find that the measured chemical shifts are in good agreement with calculated shifts from the SXRD structure.
Experimental section
Materials
Verinurad (2-[3-(4-cyanonaphthalen-1-yl)pyridin-4-yl]sulfanyl-2-methylpropanoic acid) was purchased from Selleckchem and was used without further recrystallization.
NMR experiments
1H 1D, long-range hCH and 1H 2D DQ/SQ BABA spectra were acquired at 160 kHz MAS on a 18.8 T Bruker Avance Neo spectrometer corresponding to a 1H frequency of 800 MHz, using a Bruker 0.4 mm HCN CP-MAS probe. The sample temperature was regulated to 295 K using VT flow at 280 K.
The 1H-detected 2D long-range hCH and the 13C cross polarization (CP)104,105 at 100 kHz MAS and the VMAS 1H 1D dataset spectra for PIPNet at 40–100 kHz MAS were acquired on a Bruker Avance Neo spectrometer operating at 21.14 T (1H and 13C frequency of 900 MHz and 225 MHz, respectively) equipped with a 0.7 mm room temperature HCN CP-MAS probe. All the spectra at 100 kHz MAS were acquired at a constant VT temperature of 280 K to compensate for the frictional heating, resulting in a sample temperature of 295 K.
The one-dimensional 1H spectrum was recorded using a rotor-synchronized spin echo for background suppression. The echo delay was equal to two rotor periods. 2D short-range and long-range hCH106 NMR spectra were recorded with 250 us and 4 ms contact times, respectively, for the direct 1H–13C CP and 125 us and 2 ms contact times, respectively, for the back CP. WALTZ-16 (ref. 107) decoupling at a 1H and 13C nutation frequency of 10 kHz was applied during t1 and t2, respectively. In the short-range hCH, 512 increments of 200 scans each with a recycle delay of 1.5 s were acquired, resulting in 10.2 ms of evolution in the direct dimension and 5.12 ms of evolution in the indirect dimension. In the long-range hCH, 1024 increments of 144 scans each with a 1.5 s recycle delay were acquired. In the 1H 2D DQ/SQ BABA108 spectrum, one DQ excitation and reconversion period of one rotor period each was used. In total, 512 increments with 64 scans each were acquired with a repetition delay of 1.5 s. The acquisition times were 22.5 ms and 6.4 ms in t2 and t1, respectively.
States-TPPI is used for quadrature detection in the indirect dimension for all 2D experiments. In the 2D hCH spectra, 100 Hz exponential line broadening was used in the direct dimension.
Proton chemical shifts were referenced externally with respect to the adamantane CH2 group at 1.87 ppm. Carbon chemical shifts were referenced externally with respect to the adamantane CH group at 38.48 ppm.109
All the acquisition parameters and raw NMR data are available as described in the ESI (see Table S1†).
SXRD structure relaxation
The SXRD structure was taken from ref. 103. The proton positions of the SXRD structure were optimized using the plane-wave DFT software Quantum ESPRESSO version 6.5 in order to correct any systematic error in the X-ray determinations of proton positions. The constrained optimizations were performed at the PBE level of theory using Grimme D3 dispersion correction and projector augmented wave scalar relativistic pseudopotentials with GIPAW reconstruction, S.pbe-nl-kjpaw_psl.1.0.0.UPF, H.pbe-kjpaw_psl.1.0.0.UPF, O.pbe-nl-kjpaw_psl.1.0.0.UPF, C.pbe-n-kjpaw_psl.1.0.0.UPF, N.pbe-n-kjpaw_psl.1.0.0.UPF. The wavefunction and charge density energy cutoffs were set to 120 and 960 Ry, respectively, and the relaxations were carried out without k-points.
Chemical-shift predictions
Chemical shieldings were predicted for all 1H and 13C atomic sites using ShiftML2.90,91 In the training set of ShiftML2, all the training structures were relaxed using DFT-fixed cell geometry optimizations using the Quantum ESPRESSO (QE) electronic structure package with the PBE density functional, a Grimme D2 dispersion correction, wavefunction and charge density energy cut-offs of 60 and 240 Ry, respectively, and ultrasoft pseudopotentials with GIPAW reconstruction. The GIPAW NMR calculations were performed using the QE code with the same DFT parameters as for the structure relaxation, but using refined plane wave and charge density energy cut-offs of 100 and 400 Ry, respectively, a Monkhorst–Pack k-point grid with a maximum spacing of 0.06 Å−1, and the ultrasoft pseudopotentials with GIPAW reconstruction from the USSP pseudopotential database v1.0.0. Further details are given in ref. 90. The shieldings were converted to shifts using offsets of 30.78 and 170.04 ppm for 1H and 13C, respectively. Chemical shifts were also calculated using DFT (Tables S3 and S4 and other details are given in the ESI†).
Results and discussion
Assignment of NMR spectra
The one-dimensional 1H and 13C spectra are shown in Fig. 1B and C. The short- and long-range 1H–13C hCH correlation spectra are shown in Fig. 2. The methyl groups C19 and C22 are immediately recognized due to their 1H and 13C chemical shifts, typical for methyl groups (1.01 and 1.12 ppm for 1H, 27.0 and 25.1 ppm for 13C). The quaternary carbon C18 was assigned to 49.1 ppm due to its correlation with methyl groups in the long-range hCH spectrum (Fig. 2).
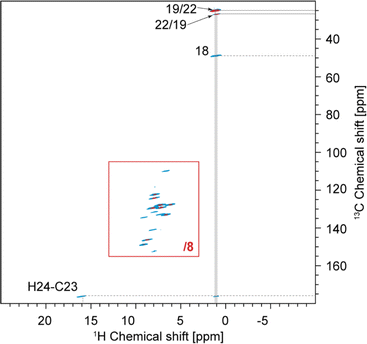 |
| Fig. 2 Complete 2D hCH long-range spectrum in blue (4 ms direct CP contact time) acquired at 160 kHz MAS overlaid with 2D hCH short-range spectrum in red (250 μs direct CP contact time) acquired at 100 kHz MAS. In the red spectrum, the contour levels were decreased by a factor 8 in the (13–3; 155–105) ppm region. This region is also given separately in Fig. 5. The label(s) corresponding to each assigned cross-peak are indicated on the spectrum. The solid lines are used for the protonated carbons and the dashed lines for the quaternary ones. | |
Similarly, H24 was unambiguously assigned at 15.91 ppm due to its characteristic chemical shift, and because it does not show any correlations in the short-range hCH (Fig. 2) and shows only one correlation with a carbon at 175.9 ppm in the long-range hCH (Fig. 2), which was then assigned to C23.
The assignment of the remaining seven quaternary carbons and nine CH groups, all of which are aromatic and resonate in the range from 100 to 160 ppm in the 13C dimension and 6 to 9 ppm in the 1H dimension, with linewidths of roughly 1 ppm for 13C and 0.5 ppm for 1H, requires more advanced methods.
First, to maximize 1H resolution, the 1D 1H spectrum of verinurad was acquired at variable MAS rates between 40 and 100 kHz MAS and this dataset was then used as input to predict the pure isotropic spectrum (free of any residual dipolar coupling) using the PIPNet approach.93 The pure isotropic spectrum is shown in Fig. 3, in comparison to the 100 kHz MAS spectrum, where we see a very significant improvement in resolution. Interestingly, the PIPNet prediction for the carboxylic proton showed significant line narrowing, proving that this 1H site is subject to significant residual dipolar coupling even at 100 kHz MAS.
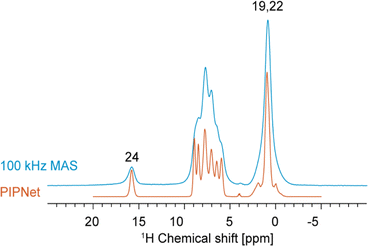 |
| Fig. 3 1D 1H spectrum acquired at 100 kHz MAS in blue overlaid with the PIPNet-predicted isotropic 1D spectrum in orange, obtained from 40 to 100 kHz MAS 1D 1H spectra. | |
The next step in the process is to apply the Bayesian probabilistic assignment method.92 This approach uses a database of chemical shifts predicted using the ShiftML2 machine-learnt model for over 338
000 structures of molecular solids contained in the Cambridge Structural Database. For each nucleus in the molecule, a predicted distribution of chemical shifts is constructed from all the similar local molecular fragments present in the database. The ensemble of individual distributions for all the atoms in the molecule is then compared with the experimental peak lists to determine the most probable complete assignment. Details are given in ref. 92. Using the publicly available scripts at https://github.com/manucordova/ProbAsn, we obtained the results shown in Fig. 4.
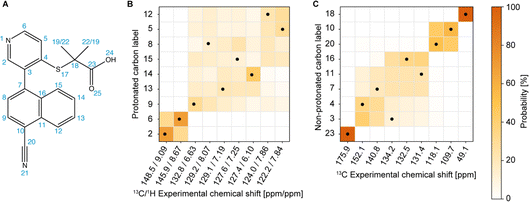 |
| Fig. 4 (A) Chemical structure of verinurad and labels corresponding to each atomic site. (B) and (C) Marginal individual assignment probabilities of the 13C (and 1H) chemical shifts after Bayesian inference of the global assignments of the (B) aromatic CH groups and (C) quaternary carbons. The dots indicate the experimentally determined correct assignment. | |
Based on the probabilistic assignment of CH groups simultaneously using 13C and 1H experimental shifts from short-range hCH, C9 and H9 are assigned at 132.8 ppm and 6.63 ppm, respectively. From the long-range hCH (Fig. 5), H9 has a correlation with a quaternary carbon at 109.7 ppm, which is therefore assigned to C10. C20 is then assigned at 118.1 ppm, based on the probabilistic assignment of the quaternary carbons. Using the BABA spectrum (Fig. 7), it is found that C9 has a correlation with a proton at 8.07 ppm, which is therefore assigned to H8 attached to C8 at 129.2 ppm.
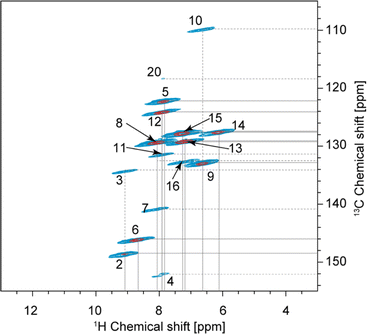 |
| Fig. 5 The aromatic region of the 2D hCH long-range spectrum in blue (4 ms direct CP contact time) at 160 kHz MAS overlaid with 2D hCH short-range spectrum in red (250 μs direct CP contact time) at 100 kHz MAS. The label(s) corresponding to each assigned cross-peak are indicated on the spectrum. The solid lines are used for the protonated carbons and the dashed lines for the quaternary ones. | |
From the probabilistic assignment of CH groups, C14 and H14 are confidently assigned at 127.4 and 6.10 ppm, respectively (43% from the probabilistic assignment, compared to 17% for the next most probable assignment).
According to the probabilistic assignment, (H2; C2) and (H6; C6) are assigned to (9.09; 148.5) ppm and (8.67; 145.9) ppm, though with some ambiguity between them. Then, in the long-range hCH, the proton at 9.09 ppm has a correlation with a quaternary carbon at 134.2 ppm, whereas the proton at 8.67 ppm does not have any correlations with quaternary carbons. H2 and C2 are therefore confidently assigned at 9.09 ppm and 148.5 ppm, and H6 and C6 are assigned at 8.67 ppm and 145.9 ppm, respectively, which is in agreement with the most probable assignment. The quaternary carbon at 134.2 ppm is therefore assigned to C3.
From long-range hCH, H8 is correlated with three quaternary carbons at 140.8, 134.2, and 132.5 ppm. These three quaternary carbons most likely correspond to C3, C7, and C16 because they are the closest to C8 in the molecular structure of verinurad. The 13C peak at 134.2 ppm was previously assigned to C3. The 13C peak at 140.8 ppm does not show any other long-range correlations in hCH, as opposed to that at 132.5 ppm. As a result, C7 is assigned to 140.8 ppm because it is the only quaternary carbon that is not connected directly to any CH groups except C8, and therefore is the least expected to form long-range hCH correlations. C16 is therefore assigned at 132.5 ppm, which is in agreement with the probabilistic assignment of the quaternary carbons. Consequently, having a long-range hCH correlation with C16, H15 is assigned at 7.25 ppm and C15 at 127.6 ppm.
Three CH groups remain unassigned at this point, C5, C12, and C13. Their 13C and 1H experimental shifts in the short-range hCH spectrum are (122.2; 7.84), (124.0; 7.86), and (129.1; 7.19) ppm. C13 is assigned at 129.1 ppm because it has very low probability to be at either 122.2 or 124.0 ppm according to the probabilistic assignment of the CH groups, whereas C5 and C12 have extremely low probability to be at 129.1 ppm.
C4 and C11 are assigned at 152.1 ppm and 131.4 ppm, respectively. The only hCH correlations for quaternary carbons left unassigned are 152.1 and 131.4 ppm, but based on the probabilistic assignment, C4 has extremely low probability to be at 131.4 ppm and vice versa.
C11 has a long-range hCH correlation with a proton at 7.86 ppm that is therefore assigned to H12, with C12 being assigned at 124.0 ppm. The last CH group left unassigned is C5–H5 which is therefore attributed the 13C and 1H chemical shifts of 122.2 and 7.84 ppm, respectively. Such an assignment of C5 and C12 protonated aromatic groups is in agreement with the ShiftML2 predicted shifts of 122.0 and 123.5 ppm for C5 and C12, respectively, which are very close to the experimental values.
Validation of the SXRD structure
With the assigned chemical shifts in hand, we can now compare the measured chemical shifts with those predicted by ShiftML2 for the crystal structure obtained via SXRD with DFT-relaxed proton positions. Fig. 6 shows the comparison between the measured and predicted shifts. The RMSD between experiment and calculation is 3.1 ppm for 13C and 0.46 ppm for 1H. These deviations are within the expected errors for the ShiftML2 method used here, which have been estimated at 4.53 ppm for 13C and 0.47 ppm for 1H.90 When looking in detail at the correlation between experiment and calculation, there are no significant outliers. We conclude that the NMR results validate the single-crystal X-ray structure.
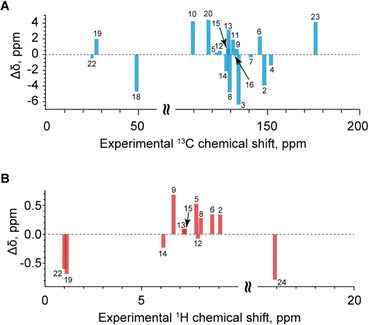 |
| Fig. 6 (A) Difference between the experimental and predicted 13C chemical shifts Δδ per atomic site as a function of experimental 13C chemical shift. (B) Difference between the experimental and predicted 1H chemical shifts Δδ per atomic site as a function of experimental 1H chemical shift. The horizontal gray dashed lines in (A) and (B) represent Δδ = 0 ppm. | |
Intermolecular correlations observed in the BABA spectrum
In the 2D DQ/SQ BABA experiment, the correlations originate from dipolar coupling between nearby protons instead of scalar couplings. As a result, intermolecular correlations can be observed in BABA spectra if the distance between the coupled spins is small enough.
In the BABA spectrum of verinurad (Fig. 7), H2 has a correlation with H24, pointing towards an intermolecular H-bond of which H24 is the donor and N1 is the acceptor. H9 presents an autocorrelation peak that originates from the intermolecular contact between equivalent H9 atomic sites separated by 2.55 Å in the SXRD structure where the proton positions were relaxed via DFT. H6 shows a BABA correlation with either H13 or H15, but given the small chemical-shift difference between H13 and H15 of only 0.06 ppm, this correlation cannot be assigned further. However, from the SXRD structure, this correlation is confidently assigned to H13 where the intermolecular distance from H6 is 2.4 Å and the smallest distance between H6 and H15 is 5.1 Å. For chemical-shift-driven structure prediction, such intermolecular BABA correlations can serve as constraints when no XRD structure is available.
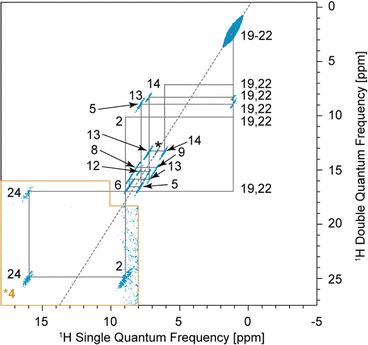 |
| Fig. 7 2D 1H–1H BABA spectrum of verinurad obtained at 160 kHz MAS. The contour levels were increased by a factor 4 in the (18–8; 27.5–16) ppm region. The dotted grey line indicates the spectral 2 : 1 “diagonal”. The solid grey lines indicate the connectivities between cross peaks. The asterisk indicates the intermolecular correlation between equivalent H9 atomic sites separated by 2.55 Å. | |
As for the chemical shifts, we conclude that all the observed inter-molecular correlations are in line with expectations from the SXRD structure.
Conclusions
We have demonstrated an NMR chemical-shift-led approach based on 1H detection at fast-MAS, in combination with the probabilistic assignment method, and machine-learned chemical shifts, to validate the crystal structure of verinurad.
The assignment of the experimental chemical shifts relied solely on the 1H-detected experiments at 160 kHz MAS, together with the probabilistic assignment of 1H and 13C shifts. The chemical shifts predicted for the SXRD structure using ShiftML2 are found to be the same, to within error, as those measured experimentally, thereby validating the SXRD structure.
The 1H-based assignment approach outlined here should be applicable to crystal structure validation or refinement for other small organic molecule APIs and is faster than the current standard NMR crystallography methodology.
Author contributions
Manuel Cordova: software (lead); investigation (equal); review and editing (equal). Lyndon Emsley: conceptualization (equal); project administration (lead); formal analysis (equal); supervision; writing – original draft (supporting); writing – review and editing (lead). Kristof Grohe: investigation (supporting); resources; writing – review and editing (equal). Jacob B. Holmes: formal analysis (equal); investigation (equal); software; visualization; writing – review and editing (equal). Sten Nilsson Lill: software; writing – review and editing (equal). Pinelopi Moutzouri: formal analysis (equal); investigation (equal); validation; writing – review and editing (equal). Stefan Norberg: supervision; writing – review and editing (equal). Arthur C. Pinon: formal analysis (equal); investigation (equal); validation; writing – review and editing (equal). Okky Dwichandra Putra: supervision; writing – review and editing (equal). Staffan Schantz: conceptualization (equal); supervision; writing – review and editing (equal). Daria Torodii: data curation; formal analysis; investigation (equal); visualization (lead); writing – original draft preparation (lead); writing – review and editing (equal). Sebastian Wegner: investigation (supporting); resources; writing – review and editing (equal). Anette Welinder: supervision; writing – review and editing (equal).
Conflicts of interest
There are no conflicts to declare. AstraZeneca authors will disclose that they are employees of AstraZeneca and that they have ownership, options, or interests in AstraZeneca stock.
Acknowledgements
This work has been supported by the Swiss National Science Foundation Grant No. 200020_212046, the European Union's Horizon 2020 Research and Innovation Programme under Grant Agreement No. 101008500 (PANACEA) and the NCCR MARVEL.
References
- A. Alex, D. S. Millan, M. Perez, F. Wakenhut and G. A. Whitlock, MedChemComm, 2011, 2, 669–674 RSC.
- T. Asai, S. Morita, N. Shirata, T. Taniguchi, K. Monde, H. Sakurai, T. Ozeki and Y. Oshima, Org. Lett., 2012, 14, 5456–5459 CrossRef CAS.
-
A. D. Bond, Analytical Techniques in the Pharmaceutical Sciences, 2016, pp. 315–337 Search PubMed.
- F. A. Martin, M. M. Pop, G. Borodi, X. Filip and I. Kacso, Cryst. Growth Des., 2013, 13, 4295–4304 CrossRef CAS.
- M. Sorrenti, L. Catenacci, D. L. Cruickshank and M. R. Caira, J. Pharm. Sci., 2013, 102, 3596–3603 CrossRef CAS.
- D. Trzybiński, P. Niedziałkowski, T. Ossowski, A. Trynda and A. Sikorski, Forensic Sci. Int., 2013, 232, e28–e32 CrossRef.
- H. Yu, L. Zhang, M. Liu, D. Yang, G. He, B. Zhang, N. Gong, Y. Lu and G. Du, Pharmaceuticals, 2023, 16, 1349 CrossRef CAS PubMed.
-
K. D. Harris, Advanced X-ray Crystallography, 2012, pp. 133–177 Search PubMed.
- C. E. Hughes, I. Boughdiri, C. Bouakkaz, P. A. Williams and K. D. M. Harris, Cryst. Growth Des., 2018, 18, 42–46 CrossRef CAS.
- H. M. Rietveld, J. Appl. Crystallogr., 1969, 2, 65–71 CrossRef CAS.
- M. Gemmi, E. Mugnaioli, T. E. Gorelik, U. Kolb, L. Palatinus, P. Boullay, S. Hovmöller and J. P. Abrahams, ACS Cent. Sci., 2019, 5, 1315–1329 CrossRef CAS.
- T. Gruene, J. J. Holstein, G. H. Clever and B. Keppler, Nat. Rev. Chem, 2021, 5, 660–668 CrossRef CAS.
- T. Gruene, J. T. Wennmacher, C. Zaubitzer, J. J. Holstein, J. Heidler, A. Fecteau-Lefebvre, S. De Carlo, E. Müller, K. N. Goldie and I. Regeni, Angew. Chem., Int. Ed., 2018, 57, 16313–16317 CrossRef CAS PubMed.
- Z. Huang, E. S. Grape, J. Li, A. K. Inge and X. Zou, Coord. Chem. Rev., 2021, 427, 213583 CrossRef CAS.
- C. G. Jones, M. W. Martynowycz, J. Hattne, T. J. Fulton, B. M. Stoltz, J. A. Rodriguez, H. M. Nelson and T. Gonen, ACS Cent. Sci., 2018, 4, 1587–1592 CrossRef CAS PubMed.
- B. L. Nannenga and T. Gonen, Nat. Methods, 2019, 16, 369–379 CrossRef CAS PubMed.
- S. E. Ashbrook and D. McKay, Chem. Commun., 2016, 52, 7186–7204 RSC.
- S. P. Brown and H. W. Spiess, Chem. Rev., 2001, 101, 4125–4156 CrossRef CAS.
- M. Geppi, G. Mollica, S. Borsacchi and C. A. Veracini, Appl. Spectrosc. Rev., 2008, 43, 202–302 CrossRef CAS.
- P. Hodgkinson, Prog. Nucl. Magn. Reson. Spectrosc., 2020, 118–119, 10–53 CrossRef CAS PubMed.
- N. U. Islam, M. N. Umar, E. Khan, F. A. Al-Joufi, S. N. Abed, M. Said, H. Ullah, M. Iftikhar, M. Zahoor and F. A. Khan, Antibiotics, 2022, 11, 797 CrossRef CAS.
- T. W. Jarrells and E. J. Munson, J. Pharm. Sci., 2022, 111, 2765–2778 CrossRef CAS PubMed.
- J. W. Lubach, D. Xu, B. E. Segmuller and E. J. Munson, J. Pharm. Sci., 2007, 96, 777–787 CrossRef CAS.
- A. Paudel, Z. A. Worku, J. Meeus, S. Guns and G. Van den Mooter, Int. J. Pharm., 2013, 453, 253–284 CrossRef CAS PubMed.
- T. N. Pham, S. A. Watson, A. J. Edwards, M. Chavda, J. S. Clawson, M. Strohmeier and F. G. Vogt, Mol. Pharm., 2010, 7, 1667–1691 CrossRef CAS PubMed.
- B. Reif, S. E. Ashbrook, L. Emsley and M. Hong, Nat. Rev. Methods Primers, 2021, 1, 2 CrossRef CAS.
- A. J. Rossini, A. Zagdoun, F. Hegner, M. Schwarzwälder, D. Gajan, C. Copéret, A. Lesage and L. Emsley, J. Am. Chem. Soc., 2012, 134, 16899–16908 CrossRef CAS PubMed.
-
K. Schmidt-Rohr and H. W. Spiess, Multidimensional Solid-State NMR and Polymers, Academic Press, 1994 Search PubMed.
- M. Skotnicki and P. Hodgkinson, Solid State Nucl. Magn. Reson., 2022, 118, 101783 CrossRef CAS.
- R. Youngman, Materials, 2018, 11, 476 CrossRef.
- N. K. Thakral, R. L. Zanon, R. C. Kelly and S. Thakral, J. Pharm. Sci., 2018, 107, 2969–2982 CrossRef CAS PubMed.
- M. Baias, J.-N. Dumez, P. H. Svensson, S. Schantz, G. M. Day and L. Emsley, J. Am. Chem. Soc., 2013, 135, 17501–17507 CrossRef CAS.
- M. Baias, C. M. Widdifield, J.-N. Dumez, H. P. Thompson, T. G. Cooper, E. Salager, S. Bassil, R. S. Stein, A. Lesage and G. M. Day, Phys. Chem. Chem. Phys., 2013, 15, 8069–8080 RSC.
- M. Balodis, M. Cordova, A. Hofstetter, G. M. Day and L. Emsley, J. Am. Chem. Soc., 2022, 144, 7215–7223 CrossRef CAS PubMed.
- F. Bravetti, S. Bordignon, E. Alig, D. Eisenbeil, L. Fink, C. Nervi, R. Gobetto, M. U. Schmidt and M. R. Chierotti, Chem.–Eur. J., 2022, 28, e202103589 CrossRef CAS PubMed.
- M. Khalaji, P. Paluch, M. J. Potrzebowski and M. K. Dudek, Solid State Nucl. Magn. Reson., 2022, 121, 101813 CrossRef CAS PubMed.
- E. Salager, G. M. Day, R. S. Stein, C. J. Pickard, B. Elena and L. Emsley, J. Am. Chem. Soc., 2010, 132, 2564–2566 CrossRef CAS PubMed.
- S. R. Byrn, P. A. Sutton, B. Tobias, J. Frye and P. Main, J. Am. Chem. Soc., 1988, 110, 1609–1614 CrossRef CAS.
- E. Davies, M. J. Duer, S. E. Ashbrook and J. M. Griffin, J. Am. Chem. Soc., 2012, 134, 12508–12515 CrossRef CAS.
- J. C. Facelli and D. M. Grant, Nature, 1993, 365, 325–327 CrossRef CAS.
- J. B. Holmes, V. Liu, B. G. Caulkins, E. Hilario, R. K. Ghosh, V. N. Drago, R. P. Young, J. A. Romero, A. D. Gill and P. M. Bogie, Proc. Natl. Acad. Sci. U. S. A., 2022, 119, e2109235119 CrossRef CAS.
- T. Pawlak and M. J. Potrzebowski, J. Phys. Chem. B, 2014, 118, 3298–3309 CrossRef CAS.
- Z. Rehman, W. T. Franks, B. Nguyen, H. F. Schmidt, G. Scrivens and S. P. Brown, J. Pharm. Sci., 2023, 112, 1915–1928 CrossRef CAS.
- U. Sternberg, F.-T. Koch, W. Prieß and R. Witter, Cellulose, 2003, 10, 189–199 CrossRef CAS.
- C. M. Widdifield and D. L. Bryce, Phys. Chem. Chem. Phys., 2009, 11, 7120–7122 RSC.
- S. O. Nilsson Lill, C. M. Widdifield, A. Pettersen, A. Svensk Ankarberg, M. Lindkvist, P. Aldred, S. Gracin, N. Shankland, K. Shankland, S. Schantz and L. Emsley, Mol. Pharm., 2018, 15, 1476–1487 CrossRef CAS PubMed.
- A. S. Tatton, H. Blade, S. P. Brown, P. Hodgkinson, L. P. Hughes, S. O. N. Lill and J. R. Yates, Cryst. Growth Des., 2018, 18, 3339–3351 CrossRef CAS.
- A. E. Watts, K. Maruyoshi, C. E. Hughes, S. P. Brown and K. D. Harris, Cryst. Growth Des., 2016, 16, 1798–1804 CrossRef CAS.
- M. Cordova, M. Balodis, A. Hofstetter, F. Paruzzo, S. O. Nilsson Lill, E. S. E. Eriksson, P. Berruyer, B. Simões de Almeida, M. J. Quayle, S. T. Norberg, A. Svensk Ankarberg, S. Schantz and L. Emsley, Nat. Commun., 2021, 12, 2964 CrossRef CAS PubMed.
- M. Cordova, P. Moutzouri, S. O. Nilsson Lill, A. Cousen, M. Kearns, S. T. Norberg, A. Svensk Ankarberg, J. McCabe, A. C. Pinon and S. Schantz, Nat. Commun., 2023, 14, 5138 CrossRef CAS.
- J. A. Fernandes, M. Sardo, L. Mafra, D. Choquesillo-Lazarte and N. Masciocchi, Cryst. Growth Des., 2015, 15, 3674–3683 CrossRef CAS.
- R. K. Harris, Solid State Sci., 2004, 6, 1025–1037 CrossRef CAS.
- J. Leclaire, G. Poisson, F. Ziarelli, G. Pepe, F. Fotiadu, F. M. Paruzzo, A. J. Rossini, J.-N. Dumez, B. Elena-Herrmann and L. Emsley, Chem. Sci., 2016, 7, 4379–4390 RSC.
- M. Selent, J. Nyman, J. Roukala, M. Ilczyszyn, R. Oilunkaniemi, P. J. Bygrave, R. Laitinen, J. Jokisaari, G. M. Day and P. Lantto, Chem.–Eur. J., 2017, 23, 5258–5269 CrossRef CAS.
- C. M. Widdifield, S. O. Nilsson Lill, A. Broo, M. Lindkvist, A. Pettersen, A. Svensk Ankarberg, P. Aldred, S. Schantz and L. Emsley, Phys. Chem. Chem. Phys., 2017, 19, 16650–16661 RSC.
- R. K. Harris, P. Hodgkinson, V. Zorin, J.-N. Dumez, B. Elena-Herrmann, L. Emsley, E. Salager and R. S. Stein, Magn. Reson. Chem., 2010, 48, S103–S112 CrossRef CAS.
- J. R. Yates, T. N. Pham, C. J. Pickard, F. Mauri, A. M. Amado, A. M. Gil and S. P. Brown, J. Am. Chem. Soc., 2005, 127, 10216–10220 CrossRef CAS PubMed.
- P. Gras, A. Baker, C. Combes, C. Rey, S. Sarda, A. J. Wright, M. E. Smith, J. V. Hanna, C. Gervais and D. Laurencin, Acta Biomater., 2016, 31, 348–357 CrossRef CAS.
- M. A. Hope, T. Nakamura, P. Ahlawat, A. Mishra, M. Cordova, F. Jahanbakhshi, M. Mladenovic, R. Runjhun, L. Merten and A. Hinderhofer, J. Am. Chem. Soc., 2021, 143, 1529–1538 CrossRef CAS.
- D. J. Kubicki, S. D. Stranks, C. P. Grey and L. Emsley, Nat. Rev. Chem, 2021, 5, 624–645 CrossRef CAS.
- A. Kunhi Mohamed, P. Moutzouri, P. Berruyer, B. J. Walder, J. Siramanont, M. Harris, M. Negroni, S. C. Galmarini, S. C. Parker and K. L. Scrivener, J. Am. Chem. Soc., 2020, 142, 11060–11071 CrossRef CAS PubMed.
- S. K. Lee, S. Y. Park, Y. S. Yi and J. Moon, J. Phys. Chem. C, 2010, 114, 13890–13894 CrossRef CAS.
- A. Mishra, M. A. Hope, M. Almalki, L. Pfeifer, S. M. Zakeeruddin, M. Grätzel and L. Emsley, J. Am. Chem. Soc., 2022, 144, 15175–15184 CrossRef CAS.
- A. Morales-Melgares, Z. Casar, P. Moutzouri, A. Venkatesh, M. Cordova, A. Kunhi Mohamed, K. L. Scrivener, P. Bowen and L. Emsley, J. Am. Chem. Soc., 2022, 144, 22915–22924 CrossRef CAS PubMed.
- J. Senker, J. Sehnert and S. Correll, J. Am. Chem. Soc., 2005, 127, 337–349 CrossRef CAS PubMed.
- B. Walkley and J. Provis, Mater. Today Adv., 2019, 1, 100007 CrossRef.
- A. Bertarello, L. Benda, K. J. Sanders, A. J. Pell, M. J. Knight, V. Pelmenschikov, L. Gonnelli, I. C. Felli, M. Kaupp, L. Emsley, R. Pierattelli and G. Pintacuda, J. Am. Chem. Soc., 2020, 142, 16757–16765 CrossRef CAS.
- H. Singh, S. K. Vasa, H. Jangra, P. Rovó, C. Päslack, C. K. Das, H. Zipse, L. V. Schäfer and R. Linser, J. Am. Chem. Soc., 2019, 141, 19276–19288 CrossRef CAS PubMed.
- Y. Dai, V. Terskikh, A. Brinmkmann and G. Wu, Cryst. Growth Des., 2020, 20, 7484–7491 CrossRef CAS.
- R. K. Harris, S. A. Joyce, C. J. Pickard, S. Cadars and L. Emsley, Phys. Chem. Chem. Phys., 2006, 8, 137–143 RSC.
- D. A. Middleton, C. S. Le Duff, X. Peng, D. G. Reid and D. Saunders, J. Am. Chem. Soc., 2000, 122, 1161–1170 CrossRef CAS.
- E. Pindelska, L. Szeleszczuk, D. M. Pisklak, A. Mazurek and W. Kolodziejski, J. Pharm. Sci., 2015, 104, 106–113 CrossRef CAS.
- A. C. Pinon, A. J. Rossini, C. M. Widdifield, D. Gajan and L. Emsley, Mol. Pharm., 2015, 12, 4146–4153 CrossRef CAS.
- C. A. Rezende, R. A. San Gil, L. B. Borré, J. R. Pires, V. S. Vaiss, J. A. Resende, A. A. Leitão, R. B. De Alencastro and K. Z. Leal, J. Pharm. Sci., 2016, 105, 2648–2655 CrossRef CAS PubMed.
-
S. A. Southern and D. L. Bryce, in Annual Reports on NMR Spectroscopy, ed. G. A. Webb, Academic Press, 2021, vol. 102, pp. 1–80 Search PubMed.
- Ł. Szeleszczuk, D. M. Pisklak, T. Gubica, K. Matjakowska, S. Kaźmierski and M. Zielińska-Pisklak, Solid State Nucl. Magn. Reson., 2019, 97, 17–24 CrossRef.
- Y. Tian, W. D. Wang, W.-B. Zou, J.-Q. Qian and C.-Q. Hu, Front. Chem., 2018, 6, 113 CrossRef.
- E. A. Engel, A. Anelli, A. Hofstetter, F. Paruzzo, L. Emsley and M. Ceriotti, Phys. Chem. Chem. Phys., 2019, 21, 23385–23400 RSC.
- A. Hofstetter and L. Emsley, J. Am. Chem. Soc., 2017, 139, 2573–2576 CrossRef CAS.
- V. E. Bazterra, M. B. Ferraro and J. C. Facelli, J. Chem. Phys., 2002, 116, 5984–5991 CrossRef CAS.
- H. R. Karfunkel and R. J. Gdanitz, J. Comput. Chem., 1992, 13, 1171–1183 CrossRef CAS.
- S. Yang and G. M. Day, J. Chem. Theory Comput., 2021, 17, 1988–1999 CrossRef CAS.
- Q. Zhu, A. R. Oganov, C. W. Glass and H. T. Stokes, Acta Crystallogr., Sect. B: Struct. Sci., 2012, 68, 215–226 CrossRef CAS PubMed.
- M. Zilka, D. V. Dudenko, C. E. Hughes, P. A. Williams, S. Sturniolo, W. T. Franks, C. J. Pickard, J. R. Yates, K. D. M. Harris and S. P. Brown, Phys. Chem. Chem. Phys., 2017, 19, 25949–25960 RSC.
- T.-X. Xiang and B. D. Anderson, J. Pharm. Sci., 2013, 102, 876–891 CrossRef CAS.
- X. Yuan, T.-X. Xiang, B. D. Anderson and E. J. Munson, Mol. Pharm., 2015, 12, 4518–4528 CrossRef CAS.
- C. Bonhomme, C. Gervais, F. Babonneau, C. Coelho, F. Pourpoint, T. Azaïs, S. E. Ashbrook, J. M. Griffin, J. R. Yates, F. Mauri and C. J. Pickard, Chem. Rev., 2012, 112, 5733–5779 CrossRef CAS PubMed.
- T. Charpentier, Solid State Nucl. Magn. Reson., 2011, 40, 1–20 CrossRef CAS PubMed.
- C. J. Pickard and F. Mauri, Phys. Rev. B: Condens. Matter Mater. Phys., 2001, 63, 245101 CrossRef.
- M. Cordova, E. A. Engel, A. Stefaniuk, F. Paruzzo, A. Hofstetter, M. Ceriotti and L. Emsley, J. Phys. Chem. C, 2022, 126, 16710–16720 CrossRef CAS.
- F. M. Paruzzo, A. Hofstetter, F. Musil, S. De, M. Ceriotti and L. Emsley, Nat. Commun., 2018, 9, 4501 CrossRef PubMed.
- M. Cordova, M. Balodis, B. Simões de Almeida, M. Ceriotti and L. Emsley, Sci. Adv., 2021, 7, eabk2341 CrossRef CAS PubMed.
- M. Cordova, P. Moutzouri, B. Simões de Almeida, D. Torodii and L. Emsley, Angew. Chem., Int. Ed., 2023, 62, e202216607 CrossRef CAS PubMed.
- P. Moutzouri, M. Cordova, B. Simões de Almeida, D. Torodii and L. Emsley, Angew. Chem., Int. Ed., 2023, 62, e202301963 CrossRef CAS.
- P. Moutzouri, B. S. de Almeida and L. Emsley, J. Magn. Reson., 2020, 321, 106856 CrossRef CAS.
- P. Moutzouri, F. M. Paruzzo, B. Simões de Almeida, G. Stevanato and L. Emsley, Angew. Chem., 2020, 132, 6294–6297 CrossRef.
- P. Moutzouri, B. Simões de Almeida, D. Torodii and L. Emsley, J. Am. Chem. Soc., 2021, 143, 9834–9841 CrossRef CAS PubMed.
- F. M. Paruzzo, G. Stevanato, M. E. Halse, J. Schlagnitweit, D. Mammoli, A. Lesage and L. Emsley, J. Magn. Reson., 2018, 293, 41–46 CrossRef CAS PubMed.
- B. Simões de Almeida, D. Torodii, P. Moutzouri and L. Emsley, J. Magn. Reson., 2023, 355, 107557 CrossRef PubMed.
- V. E. Zorin, S. P. Brown and P. Hodgkinson, J. Chem. Phys., 2006, 125, 144508 CrossRef PubMed.
- C. A. Lee, C. Yang, V. Shah, Z. Shen, D. M. Wilson, T. M. Ostertag, J.-L. Girardet, J. Hall and M. Gillen, Drug Metab. Dispos., 2018, 46, 532 CrossRef CAS PubMed.
- P. K. Tan, S. Liu, E. Gunic and J. N. Miner, Sci. Rep., 2017, 7, 665 CrossRef.
- O. T. Ring, B. R. Hayter, T. O. Ronson, L. R. Agnew, I. W. Ashworth, J. Cherryman, M. A. Y. Gall, P. R. Hamilton, P. A. Inglesby, M. F. Jones, A. L. Lamacraft, A. J. Leahy, D. McKinney, L. Miller-Potucka, L. Powell, O. D. Putra, A. J. Robbins, S. Tomasi and R. A. Wordsworth, Org. Process Res. Dev., 2022, 26, 936–948 CrossRef CAS.
- A. Pines, M. G. Gibby and J. Waugh, J. Chem. Phys., 1973, 59, 569–590 CrossRef CAS.
- E. O. Stejskal, J. Schaefer and J. S. Waugh, J. Magn. Reson., 1977, 28, 105–112 CAS.
- L. B. Andreas, K. Jaudzems, J. Stanek, D. Lalli, A. Bertarello, T. Le Marchand, D. Cala-De Paepe, S. Kotelovica, I. Akopjana, B. Knott, S. Wegner, F. Engelke, A. Lesage, L. Emsley, K. Tars, T. Herrmann and G. Pintacuda, Proc. Natl. Acad. Sci. U. S. A., 2016, 113, 9187–9192 CrossRef CAS.
- A. Shaka, J. Keeler and R. Freeman, J. Magn. Reson., 1983, 53, 313–340 CAS.
- M. Feike, D. E. Demco, R. Graf, J. Gottwald, S. Hafner and H. W. Spiess, J. Magn. Reson., Ser. A, 1996, 122, 214–221 CrossRef CAS.
- J. D. Hartman, R. A. Kudla, G. M. Day, L. J. Mueller and G. J. O. Beran, Phys. Chem. Chem. Phys., 2016, 18, 21686–21709 RSC.
|
This journal is © The Royal Society of Chemistry 2025 |
Click here to see how this site uses Cookies. View our privacy policy here.