DOI:
10.1039/D3NR04774A
(Paper)
Nanoscale, 2024,
16, 3498-3509
Crosstalk-free graphene–liquid elastomer based printed sensors for unobtrusive respiratory monitoring†
Received
21st September 2023
, Accepted 9th January 2024
First published on 9th January 2024
Abstract
Flexible strain sensors have garnered attraction in the human healthcare domain. However, caveats like crosstalk and noise associated with the output signal of such a sensor often limit the accuracy. Hence, developing a strain sensor via frugal engineering is critical, thereby warranting its mass utility. A stencil printable graphene/liquid elastomeric crosstalk-free strain sensor for unobtrusive respiratory monitoring is reported herein. Printing supports the frugality of the process and avoids complex fabrication. The sensor was mounted on a wearable mask, and the sensor console was fabricated. The console demonstrated the capability to detect the respiratory profile at room and low temperature (−26 °C) with an SNR of −12.85 dB. Developed sensors could nullify the impact of temperature and humidity and generate respiratory signals due to strain induced by breathing. A model experiment was conducted to support the fidelity of the strain mechanism. The console demonstrated excellent stability (over 500 cycles) with a sensitivity of −196.56 (0–0.17% strain) and 117.49 (0.17–0.34% strain). The console could accurately determine conditions like eupnea, tachypnoea, etc., and transmit the data wirelessly via Bluetooth. These findings solve major caveats in flexible sensor development by focusing on selectivity, sensitivity, and stability.
1. Introduction
In the era of Industry 4.0, flexible and printable sensors have become an intriguing area of research due to their ease of fabrication, functional benefits, and application potential in varying sectors, like healthcare, aerospace, etc. While various materials and technologies are in exploration to manifest the best possible sensing capabilities that can deliver the finest performance; research on the investigation of the stability, selectivity, and sensitivity of the sensors remains a challenge to explore.1 From the material's perspective, the exploration of a new class of flexible and printable sensors that are easy to manufacture is on the rise and has the potential to cater to the needs of the masses for healthcare monitoring. A major caveat associated with the usage of this new class of sensors is that they are responsive to multiple stimuli. Therefore, to use them in appropriate applications, it becomes necessary to work on the selectivity of the sensors towards the requisite stimuli. From the application purview, one of the potential areas to explore these sensors is unobtrusive respiration monitoring. This is largely guided by the fact that respiratory disorders can compromise a person's well-being.2–5 High-end testing procedures like computed tomography, angiography, etc. are leveraged. However, such tests require significant medical intervention and facilities.6,7 This results in a potential red flag for the progress of point-of-care diagnostics.8
Some of the common sensing mechanisms utilized for respiratory monitoring9–14 include the utilization of sensors that can decipher the signals due to changes in humidity,9,15–19 temperature,10,20–22 and pressure,23,24 triboelectrification,25–27 or their combination thereof.28,29 However, one major disadvantage of humidity and temperature based respiratory sensors is that they can become less accurate in high-humidity or high temperature environments. This is because the sensors rely on measuring changes in capacitance or resistance caused by moisture or an increase in temperature from exhaled breath, and high ambient moisture/temperature levels can interfere with this measurement. Therefore, the need for developing sensors with different sensing mechanisms other than humidity and temperature increased.
The other plausible way is via leveraging strain based sensors, however, reports on such sensors are far and few. The strain sensors have majorly found usage in monitoring breathing via the assessment of expansion and contraction in the abdomen and ribcage region.11 The most possible caveat that might be associated with such bending strain sensors is that they are prone to have signal cross-talks due to the impact of temperature on the strain sensors. Cross-talk or cross-sensitivity is the interference of external stimuli on the sensor's output signal, such as the influence of temperature and humidity on the output of the strain sensor. Crosstalk efficiency is the efficiency of the system to minimise the interference between various signals. Additionally, marginal movement of the body can also trigger false signals. This will result in cross-talk of the signals happening due to the influence of bending strain, body movement, and temperature simultaneously on the sensors. Studies on signal decoupling for similar purposes are far and few.12,13 Albeit such effects can be addressed using a mask-based strain sensor, however, reports on such a strain sensor over the mask to determine the respiratory profile are missing. It is also critical to mention that most such respiratory sensors are reported to work at ambient temperature and not at sub-ambient temperature. However, such measurement is vital for mountaineers under sub-ambient temperature conditions and defence persons under higher altitude and sub-ambient temperature conditions. Thus, developing a respiratory sensor mask that can work at ambient and sub-ambient temperatures is also important. Furthermore, to support mass utility and frugality, it is important to deploy a simplistic approach to fabricate the sensor and avoid the multi-step cumbersome processes. A simplistic strategy could be the printing technique.30 Even though multiple printing techniques are available, stencil mask-based printing seems the preferred choice owing to its inexpensive fabrication route and lower carbon footprint.
In an attempt to address the aforementioned challenges, in this article, we have demonstrated the development of an “on mask” bending strain sensor capable of detecting and exhibiting a cross-talk-free signal from the respiratory profile. Due to the removal of cross talks, the respiratory parameters obtained using the sensor console demonstrated a high confidence value compared to the standard values in the literature. Liquid polyisoprene rubber and graphene (at different volume fractions) were used as the model elastomer and the functional filler. Liquid elastomer facilitated printing. The developed sensors demonstrated a tensile strength of 0.6 MPa and an elongation at break of 109%. The developed sensors showcased gauge factors of −196.56 and 117.49 in the bending strain ranges of (0–0.17) % and (0.17–0.35) %. When subjected to a sub-ambient temperature of −26 °C (sensor's temperature), the developed sensors can also detect the respiratory profile. The causality for detection at lower temperatures was probed via differential scanning calorimetry studies. The sensor console could accurately determine various conditions like eupnea, tachypnoea, etc. and transmit the data wirelessly via Bluetooth. A model experiment using a pneumatic air cylinder and the sensor console was conducted to support the fidelity of the strain-based mechanistic pathway. These findings open up a new dimension in the point-of-care diagnostic tool for pulmonary profiling that offers portability, continuous monitoring, and ease of use. The developed on-mask sensor promises to identify potential respiratory problems before they become serious.
2. Experimental section
2.1. Materials used
Liquid polyisoprene rubber (LIR) with a molecular weight of 28
000 and a viscosity of 70 Pa s has been procured from Kuraray, Japan. Graphene was obtained from Adnano Technologies Private Limited (Karnataka, India) with an average thickness of 0.8–1.6 nm, surface area of 200 m2 g−1, and bulk density of 0.02 g cm−3, and dicumyl peroxide was locally procured. All chemicals were used without any further purification. A locally procured PET sheet with the name “PlusPrint”, India, has been used as a substrate with a thickness of 100 μm. Conductive silver paste has been procured from Ted Pella, California, which is used for electrode development. Conductive copper tape and copper wire have been procured locally (India) and used to connect to the sensor electrodes for further using it on an N95 facemask.
2.2. Preparation of graphene–liquid elastomer printable composition
A solvent-free process has been utilized to fabricate sensors based on a liquid polyisoprene rubber (LIR) matrix (Fig. S1a†) and few-layer graphene was used as a filler material. Peroxide-mediated crosslinking of the matrix was done by using dicumyl peroxide (DCP) as the cross-linker. DCP was first added to LIR, followed by the incorporation of the requisite amount of graphene. The composition was then subjected to hand blending for ten minutes, followed by mixing in the planetary centrifugal mixture (Model AR100, by Thinky, Japan) as shown in Fig. 1a. The three-dimensional flow of the material during mixing is achieved by revolution and rotation of the composite at 45° for a cycle of 5 minutes each. After ink preparation, the stencil printing technique is leveraged to prepare sensors. Polyethylene terephthalate was used as a substrate, and the sensor dimension printed was 1.0 × 1.0 cm2. A commercially available silicone encapsulant used for encapsulating electronic components named the SYLGARD™ 517 gel kit was used. This two-part encapsulant system was mixed in a ratio of 1
:
1 and was potted onto the sensor. Thus, encapsulated samples were cured at 110 °C for 45 minutes.
 |
| Fig. 1 (a) Schematic representation of sensor fabrication. The first step involves mixing the requisite recipe (liquid polyisoprene rubber, dicumyl peroxide, and graphene) with the aid of a planetary centrifugal mixture working on the principle of rotation followed by revolution to develop bubble-free ink. Developed inks were then stencil printed on polyethylene terephthalate with the aid of a squeegee. Printed sensors were then dried, and electrodes were applied. Thus the sensor was attached to a mask. Rheological studies on the developed sensor samples. (b) Impact of the curing temperature on the storage modulus of sensor compositions (log scale in the Y-axis). (c) Impact of different loadings of DCP on the storage modulus cured at 185 °C (log scale in the Y-axis), (d) Log electrical conductivity of different compositions as a function of various volume fractions of fillers and (e) Power-law model fitting of the obtained electrical conductivity. | |
2.3. Crosslinking optimization of the graphene–liquid elastomer printable composition
Initially, the cure characteristics of the formulation were determined by introducing the liquid polyisoprene elastomer with varying dosages of dicumyl peroxide (1, 3, 5 and 8) phr (parts per hundred). The changes in the storage modulus value have been probed at varying temperature ranges from 120 °C to 190 °C with the aid of a Modular Compact Rheometer (MCR92). LIRG6 has also been tested for analyzing the filler dispersion by measuring the complex viscosity of the system upon application of strain at a constant frequency of 1 Hz.
2.4. Stencil printing of graphene–liquid elastomer composition-based sensors
Stencil printing was leveraged to develop sensors on a commercially available PET substrate. 1 × 1 cm2 size sensors were developed on the PET which are then cured at 185 °C for 8 minutes followed by the application of silver paste, Ted Pella, product code: 16062, as electrodes with a film thickness of 100 μm.
2.5. Electrical characterization of graphene–liquid elastomer composition-based sensors
A Keithley 2450 sourcemeter has been used for electrically characterizing the sensors. A sensor size of 1 × 1 cm2 was prepared followed by the development of silver electrodes. Also, copper tape was applied on the extended silver paste followed by the attachment of copper wires with the aid of solder.
2.6. Dyanamic mechanical analysis of graphene–liquid elastomer composition-based sensors
A modular compact rheometer (MCR 702e) was used to determine the change in the elastic modulus of the thin films upon application of strain. A solid rectangular film/coating of width 9.7 mm and 0.5 mm thickness was loaded onto twin drive rotating drums in a dynamic mechanical analyzer with a linear drive. The measurements were carried out at 37 °C using a Convection Temperature Device (CTD 600 Multidrive Ready). The extensional stress vs. strain curves were obtained via the setup used for elastomers. Initially during measurement execution, an initially constant stress of 0.01 MPa was applied for 90 seconds to induce stress to avoid any sagging characteristics (under such constant applied stress). An extension rate of 0.1 s−1 has been applied for 1200 s to measure the extent of stress generated under the applied strain rate. Furthermore, thin films have been characterized for dynamic mechanical analysis in the bending mode. The storage modulus of the sample has been studied upon application of strain to probe the structural changes happening upon application of bending strain. A frequency sweep has been utilized to study the time-dependent behavior of the sample on the application of 0.1% bending strain.
2.7. Low-temperature and humidity sensing testing
For low-temperature testing, liquid nitrogen was poured on the sensor and allowed to vaporize naturally. The entire test was monitored using the thermal imaging camera to determine the temperature on the sensor before and after the breathing test. For humidity testing, the humidity of the enclosure in which the test was conducted was regulated using a humidifier, and the relative humidity was monitored using a hygrometer. In that enclosure, the samples were kept, and the samples were tested for their electrical resistance using a sourcemeter under variable relative humidity conditions.
2.8. Breath monitoring using the developed sensors
For breath monitoring, developed sensors were attached to the exhalation valve of a commercially available N95 mask. Protruding electrode wires from the sensors were attached to the two terminals of a Keithley 2450 sourcemeter and the mask was worn by a volunteer aged 28 with normal health conditions. Breathing was performed, and signals were analyzed on a Keithley 2450 sourcemeter.
3. Results and discussion
3.1. Optimization of curing through rheological studies
The printed sensor material as shown in Fig. 1a does contain dicumyl peroxide (DCP) as a crosslinker. Hence, it is important to determine the optimum dosage of DCP, curing temperature and the optimum cure time required to crosslink the formulation effectively. It is worth mentioning that formulation containing DCP as the cross-linker in solid rubber (viz synthetic or natural) is crosslinked in the temperature window of 160–170 °C.
Pressure plays a critical role in crosslinking and is reported to accelerate the crosslinking.31 However, such application of pressure during the curing process of liquid rubber based systems is difficult to achieve. Hence, in the current case of LIR based formulations, a temperature of 185 °C was selected to do the crosslinking. Beyond 185 °C, that is at 190 °C, the sample became brittle. Further to obtain the optimal dosage of DCP, the concentration of the cross-linker was varied. An increase in the crosslink density is bound to impact the storage modulus of the crosslinked composition. In line with the expectation, when the DCP dosage was varied between 1, 3, 5, and 8 phr (phr: parts per hundred grams of rubber), an increase in the storage modulus was noted (as shown in Fig. 1c). An increase in the DCP dosage from 1 phr to 3 phr resulted in an increase in the storage modulus value by 103 order magnitude. Similarly, a four times increase in the storage modulus was noted when the DCP concentration was increased from 3 phr to 5 phr, However, as the dosage was increased from 5 phr to 8 phr, there was a marginal increase in the modulus value. Thus, 5 phr DCP was selected as the optimum dosage of DCP. The experimental temperature selected for the above experiment was 185 °C. Thus, 5 phr DCP was selected as the optimum dosage of DCP. The optimum cure time was determined by recording the storage modulus maxima value for a particular temperature. This was approximated to be the maximum crosslink achievable. The time required to achieve the maximum storage modulus value (corresponding to the maximum crosslink achievable) was 7.9 and 5.1 minutes, respectively. The decrease in the cure time for samples studied at 190 °C was due to the faster availability of peroxy radicals. Samples cured at 190 °C demonstrated a higher fluctuation in the dataset, and the corresponding storage modulus was also lower. This was conjectured to be the sample's breakage due to brittleness (over-curing). Based on the above findings, the optimum cure temperature and time were selected as 185 °C and 7.9 minutes, respectively. An approximation in the curing studies was made that adding graphene will marginally impact the optimum time. Various samples with different graphene loading were prepared based on the optimum curing temperature and time obtained, and their different properties were evaluated. The graphene loadings (φ, vol%) were 0.21, 0.44, 0.56, 0.61, 0.64, and 0.72, respectively. The samples were named LIRG-X, where X corresponds to the volume fraction of the filler used.
3.2. Evaluation of the electrical properties of the sensor
Since the working principle behind the functioning of the developed sensors is the change in electrical resistance upon application of external stimuli, the assessment of electrical conductivity becomes crucial. It was observed from the graph that as the volume fraction (φ) of fillers changes from 0.20 to 0.76, there is a potential change in the electrical conductivity value, as shown in Fig. 1d and Table S1.† At φ = 0.76, the value of electrical conductivity is 1.73 × 10−4 S m−1. It is worth mentioning that a 109 times increase in the electrical conductivity value was noted for the sample at φ = 0.76 compared to the LIR sample with a filler loading of φ = 0.20 (electrical conductivity: 2.41 × 10−13 S m−1). This was due to the formation of percolating networks with an increase in the filler loading. Further investigation of the conductivity plot showed that the percolation threshold occurred at the volume fraction (φc) of 0.56. On a similar note, as can be seen in Fig. S1b† the resistivity plot showed a decreased trend as the filler concentration increased. The phenomena of the exponential increase in the value of one quantity as a dependent variable on another are governed by power law using the following equation (eqn (1)):where φ, φc, and n are the volume fraction, volume fraction at percolation, and critical exponent, respectively. The plot of log conductivity vs. log (φ − φc) shows a linear trend, depicting that the data followed the power law. The value of the critical exponent was 6.24, as shown in the log–log graph in Fig. 1e. Thus, the obtained value of the critical exponent suggests the formation of a 3-dimensional network formation.2 Based on the literature reports, it is worth mentioning that electrically resistive sensors are primarily selected from the percolation regime32 This is guided mainly by the fact that the impact of any external stimuli will cause significant perturbation in the filler network; as a result, the magnitude of relative resistance changes noted for the samples under the influence of external stimuli will be more pronounced. Hence, compositions with φ (vol%) = 0.56, 0.61, and 0.64 were selected, and their efficacy as sensors was studied.
3.3. Impact of bending strain on different compositions and breath monitoring
The developed sensors are intended to be used as small strain sensors capable of detecting the human breathing pattern, mostly via the bending strain. Hence, it is imperative to evaluate the small bending strain-sensing performance. The sensitivity of the strain sensor is defined in terms of the gauge factor (GF), and the calculation is done as per the literature report.35 To determine the response of the sensor to the applied small bending strain, sensors were subjected to bending motion with the help of an in-house fabricated device wherein the sensor was bent to a particular angle (Fig. S2†), and bending strain was calculated as per the following equation (eqn (2)): |  | (2) |
where ε is the obtained bending strain, t is the thickness of the sample, and r is the bending radius. A bending strain of up to 0.35% was applied to the developed sensors, and their electrical response was measured concomitantly. As shown in Fig. 2a, for LIRG 0.56, up to a lower strain value (0–0.17) %, a decrease in the relative resistance with a GF of −161.41 was noted. However, as the bending strain was increased (0.17–0.35) %, the GF decreased to −26.44, indicating lower sensitivity of samples at moderately increased bending strain. Similarly, in the case of LIRG 0.61 (as shown in Fig. 2b), a negative linear change in relative resistance was observed at a strain range of 0–0.17%. The GF value noted for the region was −196.56. At a higher strain range (0.17–0.35%), a linear relative resistance change was observed with a GF of 117.49. This may be attributed to the higher filler loading of LIRG 0.61, which results in a more connected network of fillers in the matrix. A trend of the relative resistance change with bending strain was found to be holding true for LIRG 0.64, wherein the sample demonstrated a linear trend in the change in relative resistance as shown in Fig. 2c, but with a lower GF of −75.36 in the strain regime (0–0.17%) and a GF of 23.42 in the strain regime (0.17–0.35%). The differentiated response of sensors with varying filler loadings is attributed to the distribution of the filler in the matrix, which corresponds to the regions at the start of percolation, in the middle of the percolating sample, and at the end of percolation. The changes in the filler network upon application of strain are, therefore, different in the three regions. In the lower strain region (0–0.17%), all the samples demonstrated a decrease in resistance due to a marginal change in the dimension of the sample, thereby coercing filler particles to touch each other, with different GFs depending upon the amount of disturbance caused in the system. However, when the strain value is increased up to 0.35%, LIRG 0.61 and LIRG 0.64 showed a linear increase in resistance due to structural changes that led to an increase in inter-particle distances. Hence, from this experiment, it could be unequivocally suggested that LIRG 0.61 demonstrated higher bending sensitivity than the other samples.
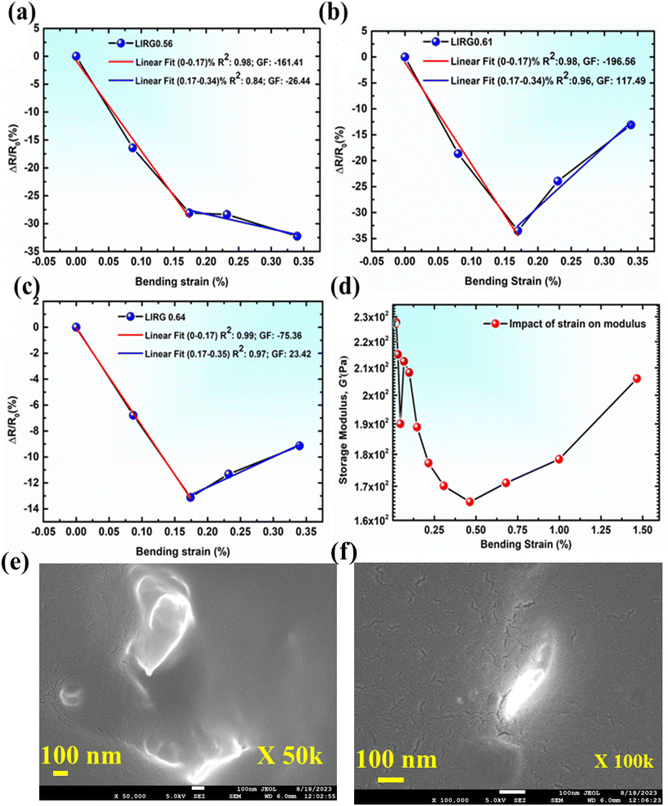 |
| Fig. 2 (a–c) Bending strain sensitivity of the different compositions prepared. (d) Relative change in the storage modulus of the sample as a function of different strains (LIRG 0.61). SEM micrograph of LIRG 0.61 at (e) 50 000 and (f) 100 000 magnifications. | |
In order to second the observation made in the electro-mechanical tests, and further understand the impact of strain on the mechanical properties of the developed nanocomposite films, dynamic mechanical analysis was carried out in the bending mode for LIRG 0.61 (peeled off sensor from the substrate). As shown in Fig. 2d, there is an initial decrease in the storage modulus value, which further increases after a particular strain value. It can be attributed to the filler orientation changes in the structure on applying strain. Poisson's ratio of rubber is close to 0.49, indicating that upon application of lateral strain, there will be a decrease in the dimensions of the sample in the transverse direction. This attribute of rubber will also change the graphene orientation inside the rubber matrix when the strain is applied. The mechanical property of the LIRG 0.61 sensor was also determined, and the developed sensors demonstrated a tensile strength of 0.6 MPa and an elongation at break of 109% (Fig. S3†). From the morphological perspective, the unencapsulated sensors were investigated using scanning electron microscopy. From the SEM micrographs, at 50
000 magnification, it was observed that the sensors demonstrated a uniform surface (Fig. 2e), while at 100
000 magnification, nano-cracks were observed. The process of sensor development involves the usage of DCP as the crosslinker. As a result, a marginal liberation of acetophenone might occur due to the reaction of DCP induced crosslinking. Such a liberation of the gaseous material is bound to leave discontinuous nano-cracks on the surface and is also evident from Fig. 2f.
Furthermore, to check the robustness of the developed sensor, the cycling stability of the LIRG 0.61 sample was tested up to 500 cycles, and it showed a repeatable pattern over 2500 seconds (Fig. 3a). However, it is worth mentioning that the samples demonstrated electrical hysteresis coupled with a negative baseline. The LIR is a viscoelastic material, so it will release heat under cyclic loading and unloading. The heat is predicted to trigger the material to demonstrate negative temperature coefficient (NTC) resistance behavior due to the presence of graphene. NTC behavior of graphene-containing composites is reported in the literature. To check the efficacy of the sample in acting as a breath monitoring sensor, we attached the printed LIRG 0.61 sensor inside an N95 mask (Fig. S4†). After wearing the mask, the volunteer performed regular breathing. Fig. 3b shows that the sensor can detect the breath waveform. The respiratory rate noted was 12 breaths per minute, indicating the sensor's capability to respond under breathing conditions. Although the sensor could decipher the respiratory rate accurately, it was observed that a steep negative baseline was associated with the measurement. This was conjectured to happen due to the presence of different influencing stimuli during the breathing process. These stimuli could be temperature, humidity, strain or a mixture of all. Considering the above two observations, it is critical to evaluate the thermal response of the sensor. To further support the fidelity of the claim, a model experiment was performed, wherein liquid nitrogen was leveraged to cool the sensor. The sensor exposed to liquid nitrogen was attached to the mask, and thus the breathing profile was recorded (a time lag was there between the sensor's exposure to the liquid nitrogen and measurement). As shown in Fig. 3c, the cooled sensor could effectively decipher the respiratory pattern, despite being under sub-zero conditions. The only caveat associated with the measurement was the observation of an exponential decay in the breathing profile. The sensor's temperature for the measurement was analyzed using a thermal infrared camera. The temperature was traced at the sensor surface (Line P1) in the thermal images. The corresponding temperature profile of the respective thermal images for the sensor after the application of liquid nitrogen is shown in the inset of Fig. 3c and the temperature mapping across the sensor used is shown in Fig. 3d. It can be seen that after the application of liquid nitrogen onto the sensor, the minimum temperature observed was −26 °C. It is also worth mentioning that the adjacent area of the sensors registered a temperature in the vicinity of −32 °C, during the measurement time. The low-temperature workability of the sensor is attributed to the low glass transition temperature of the composite (−56 °C; Fig. S5†), above which the mobility of the polymer chains and filler network is not arrested. This indicated that the sensor developed could be used to monitor the respiratory profile even at sub-zero temperatures, which is considered an unmet need in various segments, especially in the military and defense applications pertaining to high altitude and low-temperature conditions for monitoring hypoxia and determining other respiratory parameters.
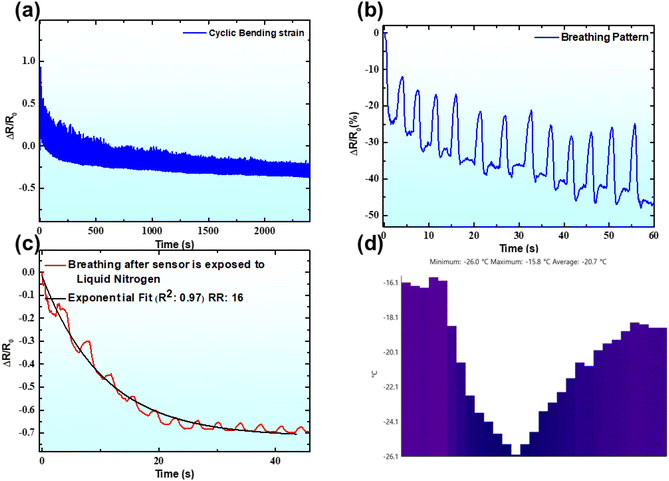 |
| Fig. 3 (a) Cycling stability of the developed LIRG 0.61 sensor, and (b) breathing pattern as obtained from the LIRG 0.61 sensor attached to a mask. (c) Breathing pattern recorded at low temperature (inset showing the thermal mapping of the sensor), and (d) thermal profile across the sensor area. | |
3.4. Impact of temperature and humidity on LIRG 0.61
Upon cyclic loading and unloading and during the breathing experiment, the samples demonstrated a negative baseline. This indicated an NTC-like behavior, so it is critical to assess the thermal response of the sensor. Also, it is worth mentioning that the developed composition is intended to be used as a strain sensor to monitor the breathing pattern. Hence, the study of thermal behavior is also needed. This is so because, during breathing, an average human being can generate a temperature of around 34.5 °C with their breath. To study the same, a heating stage was leveraged to probe the temperature sensitivity of the developed sensors. The samples were subjected to a temperature window of 30 °C to 40 °C to simulate the temperature response of breathing. As shown in Fig. S6,† LIRG 0.61 demonstrated a prominent NTC behavior with a temperature coefficient of resistance (TCR) value of −0.56%/°C. This is due to the formation of a percolating network inside the LIR matrix, which is further attributed to graphene's more prominent phonon transport behavior. This warrants a comprehensive understanding of the impact of humidity on the proposed sensor. As shown in Fig. S7,† it can be seen that the developed sensors respond well to humidity. The humidity sensitivity of the sensor is −0.96%/% RH, which is consistent with the previously reported study.33 Hitherto, it could be noted that the developed sensor is responsive not only to bending strain but also to temperature and humidity. As a result, a cross-talk of the signal will happen due to the influence of multiple stimuli working simultaneously. This warrants the development of a process to effectively decouple the breathing signals due to the bending strain and compensate for the effect of humidity and temperature on the output signal.
3.5. Decoupling humidity and temperature signal from the strain signal for breath monitoring
The humidity-sensitive behavior of the developed sensor can create cross-talk when humidity-containing breath has to be analyzed. Due to the baseline shift in the respiration signal, it can be observed that the number of peaks is getting shifted. Moreover, it also becomes difficult to predict the peak height. These two factors are correlated with a respiration signal as the respiration rate (RR) and depth of respiration (DR). Therefore, in order to get accurate respiration vitals, decoupling of humidity and temperature from strain response seems essential. Therefore, a silicone-based encapsulant was leveraged to eliminate the impact of humidity on the developed sensors. Fig. 4a showcases the impact of humidity on the relative resistance of the encapsulated versus non-encapsulated sensors. The non-encapsulated sample demonstrated a linear decrease in the relative resistance value upon increasing the humidity. There was a 38% decrease in the relative resistance value as the humidity changed from 40% to 80%. However, in the case of the encapsulated sensor, the relative resistance remained largely constant, indicating the silicone encapsulant's efficacy in creating a humidity barrier over the sensor and successfully eliminating the sensor's response to humidity.
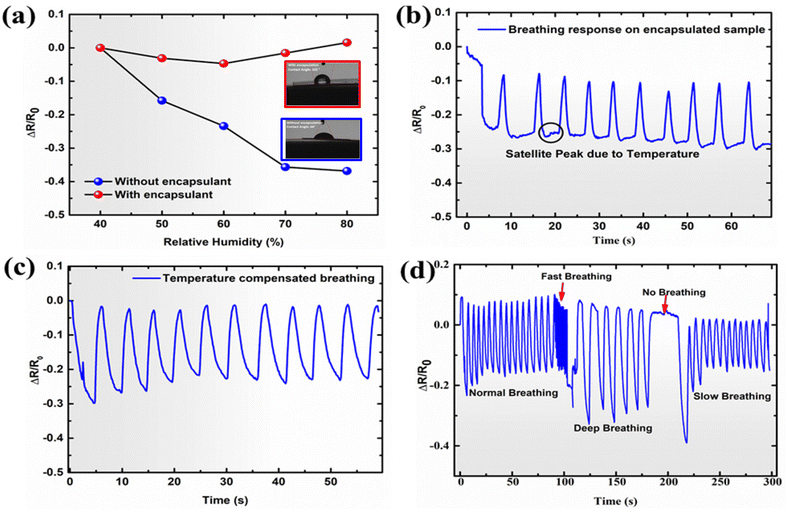 |
| Fig. 4 (a) Comparison of the change in the relative resistance to the relative humidity in the non-encapsulated and encapsulated samples. The inset shows the contact angle measurements on encapsulated (red inset, a contact angle of 102°) and non-encapsulated sample (blue inset, a contact angle of 68°), (b) Sensor's response to breathing with an encapsulant, (c) temperature compensated breathing signal on the encapsulated sample and (d) breathing signal corresponding to normal, fast, deep, no breathing, and slow breathing as a function of the change in relative resistance. | |
3.6. Crosstalk removal and breath monitoring
To demonstrate the efficacy of the adopted process, an encapsulated LIRG 0.61 sensor was attached to an N95 mask. A breath-sensing experiment was thus carried out. As shown in Fig. 4b, the breath waveform can be detected nicely, with a marginal decrease in the baseline value of the breathing signal as compared to the breathing waveform shown in Fig. 3b. The relative change in the baseline (for the non-encapsulated sample, by considering the relative resistance change) as observed in Fig. 3b is in good coherence with the relative resistance changes due to humidity (Fig. 4a). It can be unequivocally suggested that the encapsulant was successful in removing the impact of humidity on the sample. However, it is worth mentioning that the output signal did demonstrate a marginal negative baseline. It is also worth mentioning that, apart from the negative baseline, there was also an observation of a small satellite peak alongside the main peak (Fig. 4b). The observation of a marginal negative baseline change and the small satellite peak was predicted due to the influence of temperature during the breathing process.
In order to negate the influence of temperature (during breathing, the temperature increases locally around the sensor, Fig. S8†) we adopted a temperature compensation technique based on the Wheatstone bridge application, as depicted in Fig. S9.† It illustrates the Wheatstone bridge arrangement PQRS for temperature compensation where R1 and R2 are the active and dummy sensors, respectively, and R3 and R4 are the known resistors. The bridge was powered by a DC battery source of 9 V, and the A and B nodes were connected to the source meter. Similarly, a corresponding Wheatstone setup on a breadboard was done, and it was connected to the active sensor and the dummy sensor, as shown in Fig. S10.† As suggested by Karimov et al., who developed carbon nanotube-based strain sensors, to compensate for the temperature in the strain sensor, the active sensor is required to be placed in the strain region, whereas the dummy sensor is required to be placed in a stress-free zone. However, both the sensors must be placed in the same thermal environment34 Herein, two developed sensors and two resistors of known value were taken and arranged in a half-bridge configuration. Two encapsulated sensors were taken, one attached to the exhalation value area of the commercially available N95 mask (filter region) and the other at the side of the valve. The sensor on the valve was subjected to both temperature and bending strain due to breathing.
In contrast, the dummy sensor was attached to the inside of the mask with the aid of a commercially available double-sided tape, thereby keeping the sensor in place and restricting the motion of the sensors. This warrants the active sensor to be only subjected to temperature and strain, while the dummy sensor will be only subjected to the temperature. The two sensors were arranged in the adjacent arms of the Wheatstone bridge. As per the golden rule of the Wheatstone bridge arrangement, the effects arising from the opposite arms were added, whereas those arising from the adjacent arms we subtracted.35 As already noted in Fig. 4b, small satellite peaks were observed in the breathing signal. As anticipated, after utilizing the Wheatstone configuration, we could observe the appearance of sharp peaks alongside the disappearance of these small satellite peaks. This demonstrates that the impact of temperature was compensated and the signal was extracted from the sensor as depicted in Fig. 4c. As a control, we repeated the same experiment with a non-encapsulated sample. When the temperature compensation technique was applied to the non-encapsulated samples, a negative baseline was noted due to the impact of humidity; however, the small satellite peaks disappeared, further confirming that small satellite peaks were observed due to the effects of temperature and also supported that the Wheatstone bridge can eliminate the temperature response (Fig. S11†).
3.7. Mechanistic pathway for probing the working mechanism of the sensor
To confirm the sensing mechanism of the developed sensor towards small strains induced by air flow, a simulated breathing experiment has been carried out with the aid of an air pump to analyze the response of the sensor to the air flow. As shown in Fig. S12,† the sensor was attached to the mask and the two terminals of the sensor were connected to a sourcemeter. The mask has been mounted on a cardboard having a hole for the insertion of an air pump pipe. Furthermore, with the aid of Scotch tape, the sensor has been adhered tightly to the cardboard so that air does not leak out. Air was blown in and sucked out in every cycle to simulate the human respiration pattern. As seen in Fig. S13,† it can be seen that while the air was pumped in and sucked out, there has been an increase and decrease in the resistance value, respectively, which is analogous to the response obtained from both the strain sensing as well as the breath monitoring experiment. The experiment can be visually seen in ESI Movie S1† where it can be seen that as the air is blown in and sucked out, there is a corresponding motion in the sensing film confirming the occurrence of small strains on the sensor which is further reflected in the changes in the resistance values in a Keithley 2450. Hence, it can be unequivocally suggested that the developed sensor worked on the principle of strain due to the inhalation/exhalation of air.
3.8. Respiratory parameter determination
Monitoring of breathing and its corresponding parameters is important as it can provide critical information related to the pulmonary function of an incumbent. For instance, for normal breathing, the average inhalation and exhalation times are (1–1.5) s and (1.5–2.0) s, corresponding to an average normal respiration rate of 12–20 breaths per minute. Higher or lower respiration rates than this are called tachypnea and bradypnea, respectively. Tachypnea can occur due to underlying conditions such as sepsis, COPD, and asthma, whereas bradypnea can happen due to various underlying reasons such as alcohol, stress, and hypothyroidism. Moreover, a respiration rate higher than 25 has been a broad indicator of predicting heart attack.36 Hence, it is critical to assess these parameters using the developed sensor. As shown in Fig. 4d, the sensor can respond to various types of breathing, including normal, fast, deep, no-breathing, and slow-breathing patterns. The sensor could generate different signal patterns corresponding to different kinds of breathing, further signifying the respiration rate, depth of breathing, inhalation and exhalation time, and total breathing time. The respiratory rate was calculated based on the breathing signals’ Fast Fourier transform (FFT). Based on the FFT calculation, the characteristic frequencies for normal, fast, deep, and slow breathing were noted to be 0.197, 1.77, 0.098, and 0.181 Hz, respectively (Fig. S14(a–d)†).37–39 Using the frequency data, the corresponding respiratory rates for the normal, fast, deep, and slow breathing were calculated, and they were found to be 12, 106, 6, and 11, respectively.
As shown in Fig. 5(a, b) and Fig. S15(a–c),† the expanded signal (refer to Fig. 4d for the main signal output) shows the inhalation and exhalation times recorded for different breathing signals. The inhalation times for normal, fast, deep, and slow breathing were found to be 0.72 s, 0.5 s, 1.09 s, and 1.45 s, respectively, whereas the exhalation times were 2.48 s, 0.50 s, 3.81 s and 2.35 s, respectively. The lowest breathing time was observed for fast breathing (1 s), and the highest breathing time was observed for deep breathing (4.90 s). Moreover, the peak width and depth depend on the breathing time and the depth of breathing also varies with the breathing pattern. Also, no peak was observed when no breathing was done. Moreover, when the normal breathing data obtained after the application of the encapsulant and Wheatstone bridge, depicted in Fig. 5a, is compared with the normal breathing data obtained without the application of the encapsulant and Wheatstone bridge, as depicted in Fig. 5b, also summarized in Table S2,† it can be unambiguously concluded that the cross-talk removal helps in accurate assessment of respiration monitoring. The normal inspiratory to expiratory ratio (I
:
E) is usually in the range of 1
:
3 to 1
:
5, meaning that exhalation takes 3 to 5 times more time than the inhalation process.40 It can be noticed from the table that the inspiratory ratio (I
:
E) obtained without the removal of the impact of breath humidity and temperature is 1.5
:
1, which is an inaccurate value for normal breath. However, as the impact of humidity and temperature has been removed after the application of the encapsulant and Wheatstone bridge, the value for I
:
E came out to be 1
:
3.4. It clearly suggests that the developed sensors are capable of accurate measurement of the respiration signal opening new avenues in the domain of printed elastomeric strain sensors for respiration sensing. A comprehensive literature review suggests that most of the research articles on respiration sensors have not reported inhalation and exhalation times. However, a comparison of the reported sensors for normal breathing is compiled in Table S3.† The calculation of I
:
E values from thus reported articles suggests that due improvement is needed to extract accurate respiration monitoring parameters. Moreover, it has been observed that there is a decrease in the signal to noise ratio (SNR) from −21.48 dB to −12.85 dB upon application of the encapsulant and Wheatstone bridge which was expected due to the addition of more components to the system.
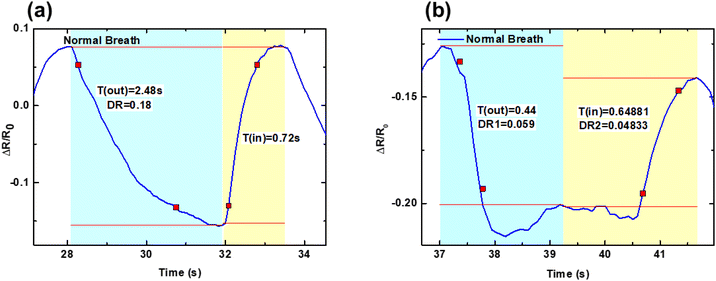 |
| Fig. 5 Expanded breathing signal obtained (a) after and (b) before the application of the encapsulant and Wheatstone bridge. | |
Apart from the determination of the respiratory rate, inhalation and exhalation times, and the I
:
E ratio it is also critical to assess the depth of respiration (DR). DR corresponds to the tidal volume of human breath, i.e., the air exchanged in one breath. An increase in the DR is known as hyperpnea and can occur due to various underlying causes such as anxiety states, lung infection, and exercise. Moreover, the increase in the DR is known as hyperventilation and can happen due to anxiety, exercise, and pathological disorders such as diabetic ketoacidosis. On the other end, hypoventilation can also occur due to decreased DR and RR. It generally happens due to sedation, obesity, hypoventilation syndrome, and metabolic alkalosis.41 Using the developed sensor, we could probe the DR effectively, and in the window of the study, the DR was noted to be the highest for deep breathing and the lowest for fast breathing. Hence, it has unequivocally suggested that the developed sensors can sense major parameters such as the depth of respiration, breathing pattern, respiration rate, and inhalation and exhalation times.
3.9. Wireless monitoring of data
With the aid of the Internet of Things (IoT), wireless monitoring of vitals has become an interwoven part of the elevated lifestyle worldwide. With the increased health awareness, the millennials of the century look forward to a change for additional ease in monitoring health. Wireless monitoring of critical parameters such as breathing is an important step in this direction. The developed flexible strain sensors can be connected to create hybrid electronics for monitoring the respiration of patients suffering from chronic and life-threatening diseases for real-data breath data acquisition. The wireless monitoring ability of the developed sensor has been demonstrated by using Arduino UNO. A Bluetooth module has been leveraged to transmit the data to the smartphone using an open-source Serial Bluetooth Terminal application. ESI Movie S2† depicts the wireless monitoring where the volunteer has been wearing the mask and breathing normally. The relative resistance has been measured using the device for 60 seconds after the completion of 100 seconds when the process starts for the calculation of RR. The measured RR value is 10 in this case. Furthermore, data transferred by the Bluetooth module are also plotted in Fig. S16.† Since the encapsulated sensors have been connected via. a Wheatstone bridge for the elimination of the humidity and temperature response. Wirelessly transferred breathing signal data are similar to that established from the experimental protocol, as seen in Fig. 4c. This showcases the applicability of the developed sensors in real-time healthcare monitoring.
4. Concluding remarks
A solvent-free, stencil printable formulation based on graphene/liquid polyisoprene rubber has been developed for respiration sensing. Developed compositions have been rheologically studied for their curing characteristics to decipher the optimum cure time. Thus, prepared inks with varying volume fractions of the functional filler were stencil printed on a polyethylene terephthalate substrate. A comprehensive study has been carried out to identify the sensors’ strain, temperature, and humidity sensing response because breathing can cause air movement leading to strain along with breath humidity and temperature. It has been deciphered from the strain sensing experiment that there is a decrease in the resistance value of the sensor in the strain region of 0–0.17% followed by an increase in the region of 0.17–0.35% upon application of strain. The dynamic mechanical analysis study seconded the strain sensing experiment showcasing a similar trend in elastic modulus values upon application of strain. The temperature coefficient of the sensor has been found to be −0.56%/°C and the humidity sensitivity has been found to be −0.96%/% suggesting that the sensor is fairly temperature- and humidity-sensitive. Additionally, both temperature and humidity affect the sensor's response by decreasing the resistance to the increase in their values. Thus, as predicted, a negative shift in the baseline has been observed in the breath-sensing experiment. Moreover, the sensor was tested at sub-ambient temperature wherein a similar behavior was observed in the baseline value. Therefore, the decoupling of the temperature and humidity response from the strain response has been done by leveraging the Wheatstone bridge configuration and the encapsulant. By successfully removing unwanted signals such as temperature and humidity, the sensors were tested to measure the vital respiration parameters, including RR, DR, inhalation time, exhalation time, and total breathing time for various breath patterns. In summary, we report the development of a smart mask that can effectively decipher the lung profile faster and easier than the state of the facilities available (a detailed comparison is shown in Table S4†). These findings open up a new dimension in the point-of-care diagnostic tool for pulmonary profiling that offers portability, continuous monitoring, and also report about the utilization of such flexible electronic sensors for strategic applications.
Author contributions
S. Sharma: experimentation, ideation, data interpretation, and writing the original draft, A. Thapa: experimentation related to wireless monitoring and air pumping for simulated breathing. S. Singh: experimentation related to modular compact rheometry analysis. T. Mondal: conceiving the idea, supervising the experimental part, acquiring funds, reviewing and writing, and editing the original draft.
Data availability
The data for all the experimental work is available from the corresponding author based on reasonable requests.
Conflicts of interest
The authors have no conflicts to disclose.
Acknowledgements
T. Mondal acknowledges the funding received from the Science and Engineering Research Board (SERB), India, through project no. EEQ/2020/000139. S. S. acknowledges the SERB for funding her fellowship.
References
- L. Xie, Z. Zhang, Q. Wu, Z. Gao, G. Mi, R. Wang, H.-B. Sun, Y. Zhao and Y. Du, Nanoscale, 2023, 15, 405 RSC.
- M. Selvan T, S. Sharma, S. Naskar, S. Mondal, M. Kaushal and T. Mondal, ACS Appl. Mater. Interfaces, 2022, 14, 45921 CrossRef CAS PubMed.
- S. Sharma, M. T. Selvan, S. Naskar, S. Mondal, T. Mukhopadhyay and T. Mondal, ACS Appl. Mater. Interfaces, 2022, 14, 57265 CrossRef CAS.
- M. Chu, T. Nguyen, V. Pandey, Y. Zhou, H. N. Pham, R. Bar-Yoseph, S. Radom-Aizik, R. Jain, D. M. Cooper and M. Khine, NPJ Digit. Med., 2019, 2, 8 CrossRef PubMed.
- Z. Liu, Z. Li, H. Zhai, L. Jin, K. Chen, Y. Yi, Y. Gao, L. Xu, Y. Zheng, S. Yao, Z. Liu, G. Li, Q. Song, P. Yue, S. Xie, Y. Li and Z. Zheng, Chem. Eng. J., 2021, 426, 130869 CrossRef CAS.
- F. Güder, A. Ainla, J. Redston, B. Mosadegh, A. Glavan, T. J. Martin and G. M. Whitesides, Angew. Chem., 2016, 128, 5821 CrossRef.
- W. Y. Kim and S. B. Hong, Tuberc. Respir. Dis., 2016, 79, 53 CrossRef.
- R. He, H. Liu, Y. Niu, H. Zhang, G. M. Genin and F. Xu, npj Flexible Electron., 2022, 6, 20 CrossRef.
- L. Ma, R. Wu, A. Patil, S. Zhu, Z. Meng, H. Meng, C. Hou, Y. Zhang, Q. Liu, R. Yu, J. Wang, N. Lin and X. Y. Liu, Adv. Funct. Mater., 2019, 29, 1904549 CrossRef CAS.
- Q. Liu, H. Tai, Z. Yuan, Y. Zhou, Y. Su and Y. Jiang, Adv. Mater. Technol., 2019, 4, 1800594 CrossRef.
- X. Peng, K. Dong, C. Ning, R. Cheng, J. Yi, Y. Zhang, F. Sheng, Z. Wu and Z. L. Wang, Adv. Funct. Mater., 2021, 31, 34 Search PubMed.
- H. Wang, Z. Xiang, P. Zhao, J. Wan, L. Miao, H. Guo, C. Xu, W. Zhao, M. Han and H. Zhang, ACS Nano, 2022, 16, 14679 CrossRef CAS PubMed.
- R. Yang, W. Zhang, N. Tiwari, H. Yan, T. Li and H. Cheng, Adv. Sci., 2022, 9, 2202470 CrossRef PubMed.
- L. Cao, Z. Zhang, J. Li, Z. Wang, Y. Ren, Q. Wang, D. Huang and Z. Li, Micromachines, 2022, 13, 1743 CrossRef PubMed.
- X. Huang, B. Li, L. Wang, X. Lai, H. Xue and J. Gao, ACS Appl. Mater. Interfaces, 2019, 11, 24533 CrossRef CAS PubMed.
- R. Xie, Q. Du, B. Zou, Y. Chen, K. Zhang, Y. Liu, J. Liang, B. Zheng, S. Li, W. Zhang, J. Wu and F. Huo, ACS Appl. Bio Mater., 2019, 2, 1427 CrossRef CAS PubMed.
- J. Luo, Y. Yao, X. Duan and T. Liu, J. Mater. Chem. C, 2018, 6, 4727 RSC.
- H. Guo, C. Lan, Z. Zhou, P. Sun, D. Wei and C. Li, Nanoscale, 2017, 9, 6246 RSC.
- H. Zhao, T. Zhang, R. Qi, J. Dai, S. Liu and T. Fei, ACS Appl. Mater. Interfaces, 2017, 9, 28002 CrossRef CAS PubMed.
- T. Jiang, L. Deng, W. Qiu, J. Liang, Y. Wu, Z. Shao, D. Wang, M. Zhang, X. Qian, J. Zhong, X. Wang and L. Lin, Biosens. Bioelectron., 2020, 163, 112288 CrossRef CAS PubMed.
- Y. Liu, L. Zhao, R. Avila, C. Yiu, T. Wong, Y. Chan, K. Yao, D. Li, Y. Zhang, W. Li, Z. Xie and X. Yu, Mater. Today Phys., 2020, 13, 100199 CrossRef.
- S. Veeralingam, S. Khandelwal, R. Sha and S. Badhulika, Mater. Sci. Semicond. Process., 2020, 108, 104910 CrossRef CAS.
- X. Chen, J. Shao, N. An, X. Li, H. Tian, C. Xu and Y. Ding, J. Mater. Chem. C, 2015, 3, 11806 RSC.
- L.-Q. Tao, K.-N. Zhang, H. Tian, Y. Liu, D.-Y. Wang, Y.-Q. Chen, Y. Yang and T.-L. Ren, ACS Nano, 2017, 11, 8790 CrossRef CAS PubMed.
- T. Tat, A. Libanori, C. Au, A. Yau and J. Chen, Biosens. Bioelectron., 2021, 171, 112714 CrossRef CAS.
- Y. Su, G. Chen, C. Chen, Q. Gong, G. Xie, M. Yao, H. Tai, Y. Jiang and J. Chen, Adv. Mater., 2021, 33, 2101262 CrossRef CAS PubMed.
- M. Wang, J. Zhang, Y. Tang, J. Li, B. Zhang, E. Liang, Y. Mao and X. Wang, ACS Nano, 2018, 12, 6156 CrossRef CAS PubMed.
- H. Jung, C. Park, H. Lee, S. Hong, H. Kim and S. J. Cho, Sensors, 2019, 19, 2834 CrossRef CAS PubMed.
- N. N. Jason, M. D. Ho and W. Cheng, J. Mater. Chem. C, 2017, 5, 5845 RSC.
-
S. Sharma and T. Mondal, in Progress in Polymer Research for Biomedical, Energy and Specialty Applications, ed. A. Srinivasan, S. Murugesan and A. R. Mahendran, CRC Press, Boca Raton, 2022, ch. 11, pp. 251–273 Search PubMed.
- I. S. Okhrimenko, Rubber Chem. Technol., 1960, 33, 1019 CrossRef.
- A. Haridas, S. Sharma, K. Naskar and T. Mondal, ACS Appl. Mater. Interfaces, 2023, 15, 17279 CrossRef.
- S. Honda, H. Hara, T. Arie, S. Akita and K. A. Takei, iScience, 2022, 25, 104163 CrossRef CAS PubMed.
- K. S. Karimov, F. A. Khalid and M. T. S. Chani, Measurement, 2012, 45, 918 CrossRef.
-
D. M. Ştefănescu, Handbook of Force Transducers Principles and Components, Springer Berlin, Heildelberg, 2011 Search PubMed.
- M. A. Cretikos, R. Bellomo, K. Hillman, J. Chen, S. Finfer and A. Flabouris, Med. J. Aust., 2008, 188, 657 CrossRef PubMed.
- Y. Pang, S. Chen, Y. Cao, Z. Huang, X. Xu, Y. Fang and C. Cao, Adv. Mater. Interfaces, 2022, 9, 2201202 CrossRef.
- Z. Zhang, J. Zhang, H. Zhang, H. Wang, Z. Hu, W. Xuan, S. Dong and J. Luo, Nanoscale Res. Lett., 2019, 14, 354 CrossRef PubMed.
-
F. Adib, H. Mao, Z. Kabelac, D. Katabi and R. C. Miller, Proceedings of the 33rd Annual ACM Conference on Human Factors in Computing Systems, Seoul, 2015.
- Basic Principles of Mechanical Ventilation, https://ecampusontario.pressbooks.pub/mechanicalventilators/ (Assessed 2023-09-03).
-
Clinical Methods: The History, Physical, and Laboratory Examination, ed. H. K. Walker, W. D. Hall and J. W. Hurst, Butterworths, Boston, 3rd edn, 1990 Search PubMed.
Footnote |
† Electronic supplementary information (ESI) available: Electrical resistivity plot for different compositions against filler volume fractions; bending of the samples at different angles using the in-house fabricated device; tensile strength and elongation at break of LIRG 0.61; an N95 mask attached to the LIRG 0.61 sample; simulated breathing on the mask setup with the aid of an air pump; the relative resistance change with the simulated breathing experiment; thermal sensitivity of the LIRG 0.61 sensor; the impact of the humidity on the LIRG 0.61 sample; the infrared image of the mask during (a) inhalation, and (b) exhalation demonstrating an increase in temperature during breathing; a Wheatstone bridge arrangement for the sensors; a Wheatstone bridge arrangement for the sensors over the mask; the temperature-compensated signal output for non-encapsulated samples; Fast Fourier Transform (FFT) image of the transformed signals, respectively, for (a) normal, (b) fast, (c) deep and (d) fast breathing; an expanded breathing signal obtained after the application of the encapsulant and Wheatstone bridge for fast, deep and slow breathing. ESI Movie S1: the simulated breathing experiment with the aid of an air pump. ESI Movie S2: wireless respiration monitoring on a volunteer. See DOI: https://doi.org/10.1039/d3nr04774a |
|
This journal is © The Royal Society of Chemistry 2024 |
Click here to see how this site uses Cookies. View our privacy policy here.