DOI:
10.1039/D4FD00210E
(Paper)
Faraday Discuss., 2025, Advance Article
Lipid–GPCR interactions in an asymmetric plasma membrane model†
Received
26th December 2024
, Accepted 23rd January 2025
First published on 30th January 2025
Abstract
We report simulations and analysis of the A2A adenosine receptor in its fully active state, in two different membrane environments. The first is a model in which the lipids are distributed asymmetrically according to recent lipidomics, simulations, and biophysical measurements, which together establish the distribution of lipids and cholesterol between the two leaflets. The second is the symmetrized version, which captures the membrane state following loss of lipid asymmetry. By comparing lipid–protein interactions between these two cases we show that solvation by phosphatidyl serine (PS) is insensitive to the loss of asymmetry—an abundance of positively charged sidechains around the cytoplasmic side of the receptor enriches solvation by PS in both membrane states. Cholesterol interactions are sensitive to the loss of asymmetry, with the abundance of cholesterol in the exoplasmic leaflet driving long-lived cholesterol interactions in the asymmetric state. However, one cholesterol interaction site on helix 6 is observed in both cases, and was also observed in earlier work with different membrane models, supporting its identification as a bona fide cholesterol binding site.
Introduction
The first direct observations of lipid–protein interactions were reported in 1999 by Mitsuoka et al., using electron crystallography of naturally occurring two-dimensional crystalline arrays of bacteriorhodopsin from purple membranes.1 Using protein reconstituted into a single component phospholipid bilayer, Gonen et al. reported (in 2005) high resolution structures of lipids surrounding an aquaporin, reconstituted into a single component phospholipid membrane.2 Around the same time, several publications reported lipids (including cholesterol) bound to membrane proteins observed by X-ray crystallography,3–6 but these were either crystallized from concentrated detergent solutions or from the lipidic cubic phase—in either case, a not-very-native membrane environment. Thus, in these early years of lipid–protein interactions the work of Mitsuoka et al. stands apart as the most representative of the protein in its native environment.
Very recently, two groups have reported high resolution structures of membrane proteins in their native membranes, with stunning results. Tao et al. reported the structure of a K+ channel in two different native membrane vesicle preparations, observing dozens of lipids including cholesterol, and finding that the native membrane environment orders previously unresolvable regions of the protein.7 The cholesterols are observed around the receptor in the exoplasmic leaflet. Coupland et al. obtained the structure of a V-ATPase in native synaptic vesicles, finding regularly spaced cholesterols arrayed on the lumenal side of the transmembrane domain, which becomes the exoplasmic leaflet upon fusing with the plasma membrane.8 Thus, in both cases the sterols are observed in the exoplasmic plasma membrane leaflet.
The lipid interactions observed in these two structures support an emerging model of plasma membrane asymmetry. By combining headgroup specific phospholipases with shotgun lipidomics, Lorent et al. reported the asymmetric distribution of glycero-phospholipids and sphingolipids in the plasma membrane of human red blood cells.9 The results were consistent with prior reports obtained using lower chemical resolution methods.10,11 The exoplasmic leaflet is more saturated in the hydrocarbon region, and contains essentially all of the sphingolipids, while the cytoplasmic leaflet contains all of the negatively charged headgroups (mostly phosphatidyl serine, abbreviated PS), nearly all of the ethanolamine headgroups (including plasmalogens with ether linked chains), and is significantly more unsaturated in the hydrocarbon region than the exoplasmic leaflet. Combining extensive simulations and leaflet-resolved biophysical measurements, Doktorova et al. reported recently that phospholipid asymmetry drives an asymmetric distribution of cholesterol, about two-thirds of which is located in the exoplasmic leaflet—more than half of all of the lipids in the exoplasmic leaflet are cholesterol.12
In this submission we use the recently reported asymmetric membrane model of Doktorova et al. for simulations of a G-protein coupled receptor (GPCR) (the A2A adenosine receptor). Because this protein has been studied by many simulation groups to assess lipid–protein interactions,13–26 it is a good test case to identify how such interactions change (or not) when the receptor is in a native-like membrane model. Visually, the receptor with its first shell lipids looks remarkably similar to the two recent native membrane structures mentioned above (Fig. 1), with substantially more cholesterol density observed around the receptor in the exoplasmic leaflet. Because the controlled loss of lipid asymmetry is associated with many different cellular functions,27 we also consider how lipid–protein interactions change following loss of asymmetry by simulating the receptor in the symmetrized version of the asymmetric membrane model. Based on a pair of 10 μs all atom simulations (one in each membrane state) of the fully active receptor, we find that PS headgroups are enriched around the cytoplasmic half of the receptor regardless of membrane state, driven by a corresponding enrichment of positively charged sidechains (19 in all), following the “positive inside rule”.28,29 A recently described motif of conserved positive charge that allosterically favors receptor activation is occupied by PS headgroups in both membrane states.15 We also find that cholesterol binds a previously described motif in the outer leaflet region of helix 6, again in both membrane states.13
 |
| Fig. 1 Simulation snapshot showing the A2A adenosine receptor in an asymmetric plasma membrane model (panel A) and its symmetrized counterpart (panel B). First shell cholesterols are rendered in pink and other first shell lipids in cyan. Wireframe mesh shows the outline of the lipid mass density. Each snapshot is the final configuration of a 10 μs all-atom simulation. | |
Methods
System setup for molecular dynamics simulations
Initial coordinates of the protein were based on a high-resolution structure of the adenosine A2A receptor in complex with the full agonist NECA and an intracellular engineered G protein (PDB: 5G53).30 The engineered G protein was deleted in silico, allowing lipids to access the G protein-binding interface. Modeller 10.5 was used to rebuild residues 147 to 158 and residues 212 to 223 that were not resolved in the receptor PDB structure.31 The protein complex was embedded in two different membrane models. The first is a bilayer with an asymmetric composition containing 12 lipids,12 containing a mixture of sphingolipids (SM), phosphatidylcholine (PC), phosphatidylethanolamine (PE), ether-linked (plasmalogen) PE (abbreviated PLAS), phosphatidylserine (PS), and cholesterol. The other is a symmetrized version of the same membrane, in which the lipid compositions of the two leaflets are identical. The symmetric membrane bilayer has the same overall lipid compositions as the asymmetric model and models the membrane environment following a loss of lipid asymmetry. The composition of both models is given in Table 1 and its caption.
Table 1 Number of lipids in asymmetric and symmetric membrane systems. The value in the parentheses is the mole fraction % in each leaflet. Abbreviations are as follows: PC – phosphatidylcholine; PE – phosphatidyl ethanolamine; PS – phosphatidyl serine; PSM – 18:1,18:0 sphingomyelin; LSM – 18:1,24:0 sphingomyelin; NSM – 18:1,24:1 sphingomyelin; PAPC – 16:0,20:4 PC; SOPC – 18:0,18:1 PC; PLPC – 16:0,18:2 PC; POPC – 16:0,18:1 PC; OAPE – 18:1,20:4 PE; PDPE – 16:0,22:6 PE; PLQS – 18:0,22:4 plasmalogen PE; PAPS – 16:0,20:4 PS; CHOL – cholesterol
Lipid class |
Abbreviation |
Asymmetric |
Symmetric |
Exo |
Cyto |
Exo |
Cyto |
SM |
LSM |
48 (6.6) |
0 |
24 (3.8) |
24 (3.8) |
NSM |
56 (7.7) |
0 |
28 (4.5) |
28 (4.5) |
PSM |
72 (9.9) |
0 |
36 (5.7) |
36 (5.7) |
|
PC |
PAPC |
24 (3.3) |
0 |
12 (1.9) |
12 (1.9) |
SOPC |
40 (5.5) |
0 |
20 (3.2) |
20 (3.2) |
PLPC |
80 (11.0) |
80 (15.0) |
80 (12.7) |
80 (12.7) |
POPC |
0 |
32 (6.0) |
16 (2.5) |
16 (2.5) |
|
PE |
OAPE |
0 |
32 (6.0) |
16 (2.5) |
16 (2.5) |
PDPE |
0 |
72 (13.5) |
36 (5.7) |
36 (5.7) |
|
PLAS |
PLQS |
0 |
80 (15.0) |
40 (6.4) |
40 (6.4) |
|
PS |
PAPS |
0 |
120 (22.6) |
60 (9.6) |
60 (9.6) |
|
CHOL |
CHOL |
404 (55.8) |
116 (21.8) |
260 (41.4) |
260 (41.4) |
Each system was prepared using the membrane builder in CHARMM-GUI.32 The protein complex was embedded in the mixtures of lipids mentioned above, solvated with TIP3P33 water molecules to provide a 30 Å-thick layer above and below the bilayer. Na+ ions were added to neutralize the system, and additional Na+ and Cl− ions were added to maintain 0.15 M ionic concentration. Both systems contained approximately 464
000 atoms and measured 18.5 × 18.5 × 14.3 nm. Protein and lipids were modeled with the CHARMM36 force field,34,35 and the ligand NECA was modeled with the CHARMM general force field.36
Simulation details
The equilibration of the two systems was run with NAMD version 3,37 following CHARMM-GUI’s default six-step protocol. The initial configuration was relaxed by 10
000 steps of steepest descent. The first three simulations had 1 fs timestep for a total of 375 ps (125 ps × 3) of simulation time, and the last three steps had a 2 fs timestep for a total of 1500 ps (500 ps × 3) simulation length. During the six-step equilibration protocol, velocities were reassigned every 500 steps. The heavy atoms of the receptor backbone and the ligand NECA were positionally restrained but with a decreasing force constant from 10.0 to 0.1 kcal mol−1 Å−2 following CHARMM-GUI’s default. The force constant was gradually reduced from 5.0 to 0 kcal mol−1 Å−2 for the heavy atoms of the receptor sidechain. Similarly, the harmonic restraints on the heavy atoms of lipid headgroups were reduced from 5.0 to 0 kcal mol−1 Å−2 in a stepwise manner. The simulation box volume was allowed to change semi-isotropically via a Langevin piston with a barostat damping time scale of 25 fs and an oscillation period of 50 fs.38 The target pressure and temperature were 1.01325 bar and 310 K, respectively. All covalently bonded hydrogens were constrained by SHAKE.39 A cutoff of 1.2 nm was used for the electrostatic and van der Waals interactions, while the switching algorithm was on if the distance between two atoms was between 1.0 nm and 1.2 nm, ensuring the potential was smoothly reduced to 0 at the cutoff distance. The long-range electrostatic interactions were computed using the Particle Mesh Ewald (PME) method40 on a 1 Å grid, with a tolerance of 10−6 and sixth order interpolation. The nonbonded interactions were not computed only if two atoms were connected within 3 covalent bonds. The neighbor list was updated every 10 steps, including all pairs of atoms whose distances were 1.2 nm or less. The sixth equilibration step was run for another 30 ns to ensure the receptor was well equilibrated. An additional 70 ns production run was performed in which all restraints were removed.
The equilibrated binary restart files from NAMD were converted into DMS format for further production simulation on Anton2. Viparr 4.7.49c7 was used to add force field information. Integration was performed under constant pressure (1 atm), temperature (310 K), and particle number with the multigrator41 method with a 2.5 fs time step. Temperature was controlled by a Nosé–Hoover42 thermostat coupled every 24 timesteps and pressure was controlled by the Martyna–Tobias–Klein barostat (semi-isotropic) coupled every 480 timesteps.43 Nonbonded interactions were cut off at 1.2 nm. Long-range electrostatic interactions were computed using the u-series method44 following Anton2’s default. Hydrogens were constrained by M-SHAKE.45 Production simulations for each system were performed for 10 μs, and configurations were stored every 480 ps.
Simulation analysis
Visual Molecular Dynamics (VMD)46 was used for molecular visualization. Data analysis and plotting were performed using in-house python scripts based on publicly hosted python packages: NumPy,47 MDAnalysis,48 and matplotlib.49
We conducted Voronoi analysis with the Voronoi module from the python package SciPy.50 For the entire 10 μs trajectory, the configurations were sampled at 0.48 ns intervals. In each of the 20
834 frames, for each leaflet, the center of mass of each residue from the seven transmembrane domains (above or below the midplane) and the center of mass of each lipid were projected onto the midplane (z = 0) to form a 2D point set. Following Voronoi analysis, lipids sharing an edge with receptor residues were identified as belonging to the first shell.
To characterize the interactions between cholesterol and the receptor residues, contacts between protein sidechains and cholesterol were identified for each residue, using a definition of a contact as ≤4.5 Å between the closest heavy atoms of any cholesterol and the protein sidechain. The interaction frequency of cholesterol with each residue was then classified into three tiers: “high” if the residue contacts a cholesterol for more than 80% of the duration of the 10 μs simulation, “medium” greater than 50%, and low if below 50%. Interactions between the receptor and PS were defined by a distance of ≤4.5 Å between the closest heavy atoms of any PS headgroups and the receptor residue sidechain, using the same classification of the interaction frequency.
Results
A PS interaction motif is occupied in both membrane states
Two simulations were performed of the receptor in its fully active state, but in the absence of the G-protein (see Methods for details on how the initial structure was prepared). This state of the receptor was chosen because in a recent publication15 we showed that a motif of positive charge on the intracellular face of the receptor mediates interaction with a PS headgroup, favoring the fully active state of the receptor in the absence of the G-protein. Here, we simulated this receptor state in two different membrane environments—an asymmetric membrane model (in which all of the PS is located on the cytoplasmic leaflet), and its symmetrized counterpart, in which the concentration of PS is reduced by more than a factor of two. By designing the simulations in this way we were able to assess changes in PS interactions following loss of lipid asymmetry.
The lipid compositions of the first solvation shell in both leaflets were obtained by a Voronoi analysis, as described in Methods. To simplify the analysis the lipids were grouped into classes based on headgroup; the lipid classes are defined in Table 1. Most (though not all) lipids that begin the production simulation in the first shell have exchanged out of the first shell by the end of the 10 μs production simulations (Fig. S1†). The average number of first shell lipids in each class was then computed over 1 μs trajectory segments, these are reported as mole fractions in Fig. S2,† and the averages of each class (computed over the final 5 μs of each simulation) are reported as mole fractions in Fig. 2 and as numbers of each lipid class in Table 2.
 |
| Fig. 2 The mole fraction of lipids in each class in the first solvation shell in the exoplasmic and cytoplasmic leaflets, and in the asymmetric membrane simulation (left panel) and in the symmetric membrane simulation (right panel), averaged over the last 5 μs of each trajectory simulations. Gray filled bars are the results for the first solvation shell of the receptor, and the magenta filled bars are the composition of that species averaged over the entire leaflet. An asterisk (*) indicates cases for which the lipid composition of the bulk is outside the 95% confidence interval of its composition in the first shell. The PE class includes plasmalogen. | |
Table 2 The average number of lipids in the first shell of the receptor in asymmetric and symmetric membrane systems over the last 5 μs simulations. The value in the parentheses is the standard deviation
Lipid class |
Asymmetric |
Symmetric |
Exo |
Cyto |
Exo |
Cyto |
SM |
6.9 (1.4) |
0 |
2.7 (1.3) |
0.9 (0.7) |
PC |
5.4 (1.0) |
2.1 (1.4) |
7.0 (1.6) |
3.7 (1.3) |
CHOL |
12.7 (2.1) |
2.2 (1.1) |
8.5 (2.2) |
6.3 (1.8) |
PE + PLAS |
0 |
5.6 (1.5) |
2.5 (1.1) |
4.1 (1.3) |
PS |
0 |
7.7 (1.8) |
2.0 (0.8) |
6.8 (1.8) |
Fig. 2 shows that the composition of the first shell does not differ significantly from the bulk for most lipid classes. This can be seen by comparing the average first shell composition (gray bars with errors) to the bulk composition (pink bars). Cases for which the bulk lipid composition is outside the 95% confidence interval of the first shell composition are indicated by an asterisk. A clear exception is PS, which is enriched in the cytoplasmic first shell in both the asymmetric model and its symmetrized counterpart. In the asymmetric membrane simulation it averages 43.8 ± 10.1 mol%, compared to the average value in the bulk of about 23 mol%. In the symmetrized membrane simulation it is still enriched compared to bulk, at 31.4 ± 8.1 mol% compared to about 10 mol% in the bulk. In the symmetrized model this comes at the expense of sphingolipids, which are depleted by a statistically significant amount, and to a lesser extent PC. In the asymmetric model the depletion is distributed over all of the other lipid classes, none rising to a 95% confidence level.
These interactions are driven by the “positive inside rule”:28 the receptor has 19 positively charged sidechains (Lys and Arg) located on its cytoplasmic half; it is these interactions that drive enrichment of PS headgroups around the receptor. Ten of the positive charges interact with a PS headgroup at least 80% of the time in both the asymmetric and symmetric membrane models (Fig. 3): R1113.59, R1204.41, R1995.60, R2055.66, R2065.67, K2336.35, R2917.56, R2937.58, R2967.61, R3007.65. Two additional residues interact with PS in the asymmetric simulation: R1073.55 and R3047.69. The fact that enrichment of PS is reproducible across two independent simulations with very different lipid compositions lends significance to the result.
 |
| Fig. 3 Positively charged side chains that interact with a PS headgroup at least 80% of the time in the asymmetric membrane simulation (red) or at least 50% of the time (yellow). The arginine of the D/ERY motif is marked on helix 3 with a black arrow. K2336.35 and R2917.56 mediate a PS interaction motif that allosterically activates the receptor; these are indicated by black arrows on helices 6 and 7. For the corresponding plot from the symmetric membrane simulation, see ESI Fig. S3.† | |
A pair of side chains (K2336.35 and R2917.56, black arrows in Fig. 3) are of particular interest, as we recently reported that they mediate an interaction with negatively charged headgroups, preorganizing the intracellular face for G-protein interaction.15 Both residues are interacting with PS headgroups nearly all of the time in both the asymmetric and symmetric simulations, suggesting that this interaction remains saturated even following loss of asymmetry.
Cholesterol interactions favor the exoplasmic leaflet
According to Fig. 2, the cholesterol composition of the first shell around the exoplasmic side of the receptor does not differ significantly from the bulk composition (defined again as a first shell composition that is outside the 95% CI of the bulk average). This is true of both the asymmetric model and its symmetrized counterpart. However, in the asymmetric model, because cholesterol is so abundant in the exoplasmic leaflet, the first shell of the protein in the exoplasmic leaflet contains many cholesterols—more than twelve in total, and more than 50% of all first shell lipids. This is apparent from the image in Fig. 1 (left panel) that shows the exoplasmic surface of the receptor decorated with individual sterols. In the cytoplasmic leaflet cholesterol is slightly depleted relative to its bulk concentration in both membrane models, with the PS enrichment described above displacing one to two cholesterols in the cytoplasmic leaflet. However, in neither case does the cholesterol depletion rise to a 95% confidence level.
Several long-lived cholesterol interactions are observed with specific locations on the receptor surface. In the asymmetric membrane model, three individual cholesterols remain bound to the receptor for the entire duration of the 10 μs simulation. The three locations are all in the exoplasmic leaflet on TM2, TM6, or TM7, and are indicated on the snake plot in Fig. 4 in purple. The binding disposition of all three cholesterols is shown also in Fig. 4 and a time series of the residues’ interaction with cholesterol is shown in Fig. S4.† The configurations shown in Fig. 4 are the final ones from the asymmetric membrane simulation. In the symmetrized membrane there is a single cholesterol bound for the entire duration of the simulation to the same location on TM6; a time series showing the interaction with this cholesterol is reported in Fig. S5.† This same site was also identified in our prior work, where we named it “h6o” for “helix 6, outer leaflet”. The prior observation was in a very different membrane environment (a ternary mixture of cholesterol, saturated, and di-unsaturated PC), and in both all atom and coarse-grained Martini 2.0 simulations.13 We do not observe long-lived interactions at the “cholesterol consensus motif”, which is located on the cytoplasmic leaflet in between TM2 and TM4. This motif has a lysine at position K1224.43; in these simulations negatively charged headgroups are abundant, and this residue is interacting primarily with PS headgroups (see lysine colored in yellow on H4 in Fig. 3). However, in the symmetrized membrane simulation we find that this lysine and several residues form the consensus motif on helix 4 interacting with cholesterol instead of PS (ESI Fig. S6†).
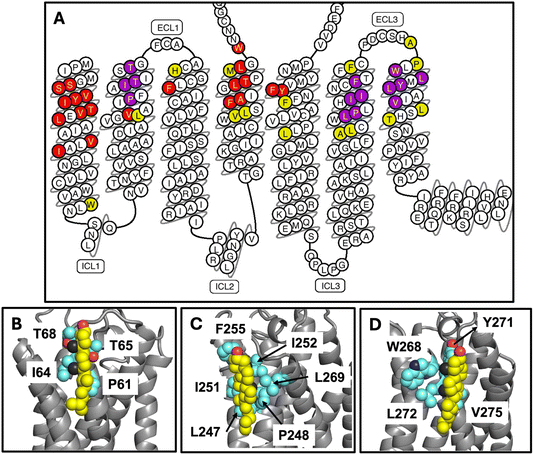 |
| Fig. 4 Cholesterol interactions with the receptor in the asymmetric membrane simulation. Interactions are colored by residue in panel (A), with purple indicating interaction with a single cholesterol for the entire duration of the simulation, red indicating interaction with any cholesterol for at least 80% of the simulation, and yellow any cholesterol for at least 50% of the simulation. The binding disposition of the cholesterol on helix 2 is shown in panel (B), on helix 6 in panel (C), and on helix 7 in panel (D). The heavy atoms of the sidechains indicated in purple in panel A are represented with cyan spheres and are labeled in panels (B–D). Carbon atoms in cholesterol are yellow spheres, the red sphere shows the hydroxyl oxygen, and the black spheres represent the methyls of the beta face. The same analysis is shown for the symmetrized membrane simulation in ESI Fig. S6.† | |
Discussion
There is an extensive literature documenting interactions between lipids and integral membrane proteins, and how such interactions modulate function across many classes of membrane proteins.51 Since the earliest work on rhodopsin,52 simulations of GPCRs have played an important role in establishing such interactions.25,53,54 Most simulation reports (with the notable exception of the early rhodopsin work) have focused on interactions with negatively charged headgroups like PS and inositides,18,55,56 or on cholesterol.13,26,57–60 There is experimental evidence that both negative charge and cholesterol modulate GPCR activity. However, most of the experimental reports of cholesterol modulation are obtained in cell-based assays, using methyl-β-cyclodextrin (MβCD) to deplete cholesterol from the plasma membrane. As shown recently by Doktorova et al., this reagent also triggers loss of membrane asymmetry,12 which results in a drastic change in the membrane environment of the receptor, including redistribution of half of the PS from the cytoplasmic to the exoplasmic leaflet. Thus in order to develop a fuller picture of lipid-dependent mechanisms it is necessary to compare lipid–protein interactions across symmetric and asymmetric lipid distributions. Since transient loss of asymmetry is a common cellular response, results from such comparisons have relevance beyond interpreting assays employing MβCD.
As far as we are aware, very few GPCR simulations have used an asymmetric membrane model,18 and all used the coarse-grained Martini model, with an asymmetric lipid distribution first reported by Ingólfsson et al.61 In this work we performed all-atom simulations of the A2A adenosine receptor in an asymmetric membrane model based on chemically detailed lipidomics measurements, including differences in lipid abundance between the leaflets as well as cholesterol. Cholesterol is significantly asymmetric in its distribution, with about two-thirds of the total membrane cholesterol in the exoplasmic leaflet, where it is about 50% of all lipids. We compared lipid interactions in the asymmetric membrane and its symmetrized counterpart.
The receptor follows the positive inside rule—there is an abundance of positively charged side chains on the cytoplasmic half of the receptor, which drives a substantial enrichment of PS headgroups in the first solvation shell of the receptor. Most of these are arginine, and most of them are interacting with PS headgroups (more than 80% of the simulation time) in both the asymmetric and symmetrized membrane models. This includes a recently reported motif of positive charge that mediates an interaction with negatively charged headgroups, which act as a positive allosteric modulator of the receptor.15 It interacts with PS in both membrane states, suggesting that the concentration of PS might still be sufficient to drive activation even following loss of asymmetry. One important exception to the interaction of positive sidechains with PS is the arginine of the D/ERY motif—due to its positioning, we do not observe it to interact with PS headgroups in either membrane state.
In the asymmetric simulation many locations on the protein interact with cholesterol on the exoplasmic half of the receptor—this is hardly surprising, given the abundance of cholesterol in the exoplasmic leaflet. Visually, the cholesterol solvation of the receptor looks strikingly similar to recent cryo-EM structures of membrane proteins obtained in native membrane environments (Fig. 1). Three locations (shown in purple in Fig. 4) each bind a single cholesterol during the entire duration of the 10 μs asymmetric simulation. None of these sites has the characteristics of a CRAC/CARC motif. One location on helix 6 also binds a single cholesterol for the entirety of the symmetrized membrane simulation. This same location was also observed to bind a single cholesterol in simulations using both an all-atom and a Martini representation of a ternary lipid mixture. The concurrence of observations in very different membrane environments suggests that this is a bona fide cholesterol binding site.
Many cholesterols are observed to interact with A2A in several different structures, all solved by X-ray diffraction on protein crystallized from the cubic phase.18 Four of these are in the exoplasmic leaflet and five are in the cytoplasmic half of the receptor (counting only unique locations). The locations in the exoplasmic leaflet overlap partially with the residues that are observed to interact with cholesterol, but this observation needs to be balanced against the fact that much of the exoplasmic surface of the receptor interacts with cholesterol, which is to be expected based on its relative abundance in that leaflet. Many of these interactions are lost upon symmetrization.
The discrepancy between cholesterol interactions observed in X-ray structures and in our simulations raises an important question: to what extent are these interactions representative of the native membrane environment? We anticipate that the situation will become clearer as more native membrane structures are published, and as membrane protein simulations continue moving toward more native membrane models.
Data availability
The data supporting this article have been included as part of the supplementary information.†
Conflicts of interest
The authors declare no conflicts of interest.
Acknowledgements
EL and JJ were supported by the US National Institute of General Medical Sciences by award number R35-GM153273. Computational work utilized the Extreme Science and Engineering Discovery Environment supported by National Science Foundation Grant ACI-1548562. Anton2 computer time was provided by the Pittsburgh Supercomputing Center through grant R01GM116961 from the National Institutes of Health. The Anton2 machine was made available by D. E. Shaw Research.
References
- K. Mitsuoka, T. Hirai, K. Murata, A. Miyazawa, A. Kidera, Y. Kimura and Y. Fujiyoshi, J. Mol. Biol., 1999, 286, 861–882 CrossRef CAS PubMed.
- T. Gonen, Y. Cheng, P. Sliz, Y. Hiroaki, Y. Fujiyoshi, S. C. Harrison and T. Walz, Nature, 2005, 438, 633–638 CrossRef CAS PubMed.
- M. A. Hanson, V. Cherezov, M. T. Griffith, C. B. Roth, V.-P. Jaakola, E. Y. T. Chien, J. Velasquez, P. Kuhn and R. C. Stevens, Structure, 2008, 16, 897–905 CrossRef CAS PubMed.
- V. Cherezov, D. M. Rosenbaum, M. A. Hanson, S. G. F. Rasmussen, F. S. Thian, T. S. Kobilka, H.-J. Choi, P. Kuhn, W. I. Weis, B. K. Kobilka and R. C. Stevens, Science, 2007, 318, 1258–1265 CrossRef CAS PubMed.
- D. M. Rosenbaum, C. Zhang, J. A. Lyons, R. Holl, D. Aragao, D. H. Arlow, S. G. F. Rasmussen, H.-J. Choi, B. T. DeVree, R. K. Sunahara, P. S. Chae, S. H. Gellman, R. O. Dror, D. E. Shaw, W. I. Weis, M. Caffrey, P. Gmeiner and B. K. Kobilka, Nature, 2011, 469, 236–240 CrossRef CAS PubMed.
- T. Warne, R. Moukhametzianov, J. G. Baker, R. Nehmé, P. C. Edwards, A. G. W. Leslie, G. F. X. Schertler and C. G. Tate, Nature, 2011, 469, 241–244 CrossRef CAS PubMed.
- X. Tao, C. Zhao and R. MacKinnon, Proc. Natl. Acad. Sci. U. S. A., 2023, 120, e2302325120 CrossRef CAS PubMed.
- C. E. Coupland, R. Karimi, S. A. Bueler, Y. Liang, G. M. Courbon, J. M. Di Trani, C. J. Wong, R. Saghian, J.-Y. Youn, L.-Y. Wang and J. L. Rubinstein, Science, 2024, 385, 168–174 CrossRef CAS PubMed.
- J. H. Lorent, K. R. Levental, L. Ganesan, G. Rivera-Longsworth, E. Sezgin, M. Doktorova, E. Lyman and I. Levental, Nat. Chem. Biol., 2020, 16, 644–652 CrossRef CAS PubMed.
- A. J. Verkleij, R. F. A. Zwaal, B. Roelofsen, P. Comfurius, D. Kastelijn and L. L. M. van Deenen, Biochim. Biophys. Acta, Biomembr., 1973, 323, 178–193 CrossRef CAS PubMed.
- A. Sandra and R. E. Pagano, Biochemistry, 1978, 17, 332–338 CrossRef CAS PubMed.
- M. Doktorova, J. L. Symons, X. Zhang, H.-Y. Wang, J. Schlegel, J. H. Lorent, F. A. Heberle, E. Sezgin, E. Lyman, K. R. Levental and I. Levental, Cell, 2025 DOI:10.1016/j.cell.2025.02.034.
- E. Rouviere, C. Arnarez, L. Yang and E. Lyman, Biophys. J., 2017, 113, 2415–2424 CrossRef CAS PubMed.
- L. Yang and E. Lyman, Biochemistry, 2019, 58, 4096–4105 CrossRef CAS PubMed.
- N. Thakur, A. P. Ray, L. Sharp, B. Jin, A. Duong, N. G. Pour, S. Obeng, A. V. Wijesekara, Z.-G. Gao, C. R. McCurdy, K. A. Jacobson, E. Lyman and M. T. Eddy, Nat. Commun., 2023, 14, 794 CrossRef CAS PubMed.
- A. N. Leonard and E. Lyman, Biophys. J., 2021, 120, 1777–1787 CrossRef CAS PubMed.
- C. McGraw, L. Yang, I. Levental, E. Lyman and A. S. Robinson, Biochim. Biophys. Acta, Biomembr., 2019, 1861, 760–767 CrossRef CAS PubMed.
- W. Song, H.-Y. Yen, C. V. Robinson and M. S. P. Sansom, Structure, 2019, 27, 392–403.e3 CrossRef CAS PubMed.
- W. Song, A. L. Duncan and M. S. P. Sansom, Structure, 2021, 29, 1312–1325.e3 CrossRef CAS PubMed.
- A. Bruzzese, J. A. R. Dalton and J. Giraldo, PLoS Comput. Biol., 2020, 16, e1007818 CrossRef CAS PubMed.
- H. W. Ng, C. A. Laughton and S. W. Doughty, J. Chem. Inf. Model., 2014, 54, 573–581 CrossRef CAS PubMed.
- R. A. Corey, O. N. Vickery, M. S. P. Sansom and P. J. Stansfeld, J. Chem. Theory Comput., 2019, 15, 5727–5736 CrossRef CAS PubMed.
- S. Genheden, J. W. Essex and A. G. Lee, Biochim. Biophys. Acta, Biomembr., 2017, 1859, 268–281 CrossRef CAS PubMed.
- D. Sengupta, X. Prasanna, M. Mohole and A. Chattopadhyay, J. Phys. Chem. B, 2018, 122, 5727–5737 CrossRef CAS PubMed.
- E. Lyman, C. Higgs, B. Kim, D. Lupyan, J. C. Shelley, R. Farid and G. A. Voth, Structure, 2009, 17, 1660–1668 CrossRef CAS PubMed.
- J. Y. Lee and E. Lyman, J. Am. Chem. Soc., 2012, 134, 16512–16515 CrossRef CAS PubMed.
- T. Sakuragi and S. Nagata, Nat. Rev. Mol. Cell Biol., 2023, 24, 576–596 CrossRef CAS PubMed.
- G. Von Heijne, EMBO J., 1986, 5, 3021–3027 CrossRef CAS PubMed.
- S. H. White, G. V. Heijne and D. M. Engelman, Cell Boundaries: How Membranes and Their Proteins Work, Garland Science, New York, 1st edn, 2021 Search PubMed.
- B. Carpenter, R. Nehmé, T. Warne, A. G. W. Leslie and C. G. Tate, Nature, 2016, 536, 104–107 CrossRef CAS PubMed.
- B. Webb and A. Sali, Curr. Protoc. Protein Sci., 2016, 86, 2.9.1–2.9.37 Search PubMed.
- J. Lee, D. S. Patel, J. Ståhle, S.-J. Park, N. R. Kern, S. Kim, J. Lee, X. Cheng, M. A. Valvano, O. Holst, Y. A. Knirel, Y. Qi, S. Jo, J. B. Klauda, G. Widmalm and W. Im, J. Chem. Theory Comput., 2019, 15, 775–786 CrossRef CAS PubMed.
- W. L. Jorgensen, J. Chandrasekhar, J. D. Madura, R. W. Impey and M. L. Klein, J. Chem. Phys., 1983, 79, 926–935 CrossRef CAS.
- J. B. Klauda, R. M. Venable, J. A. Freites, J. W. O’Connor, D. J. Tobias, C. Mondragon-Ramirez, I. Vorobyov, A. D. MacKerell and R. W. Pastor, J. Phys. Chem. B, 2010, 114, 7830–7843 CrossRef CAS PubMed.
- J. Huang, S. Rauscher, G. Nawrocki, T. Ran, M. Feig, B. L. De Groot, H. Grubmüller and A. D. MacKerell, Nat. Methods, 2017, 14, 71–73 CrossRef CAS PubMed.
- K. Vanommeslaeghe, E. Hatcher, C. Acharya, S. Kundu, S. Zhong, J. Shim, E. Darian, O. Guvench, P. Lopes, I. Vorobyov and A. D. Mackerell, J. Comput. Chem., 2010, 31, 671–690 CrossRef CAS PubMed.
- J. C. Phillips, R. Braun, W. Wang, J. Gumbart, E. Tajkhorshid, E. Villa, C. Chipot, R. D. Skeel, L. Kalé and K. Schulten, J. Comput. Chem., 2005, 26, 1781–1802 CrossRef CAS PubMed.
- S. E. Feller, Y. Zhang, R. W. Pastor and B. R. Brooks, J. Chem. Phys., 1995, 103, 4613–4621 CrossRef CAS.
- J.-P. Ryckaert, G. Ciccotti and H. J. C. Berendsen, J. Comput. Phys., 1977, 23, 327–341 CrossRef CAS.
- T. Darden, D. York and L. Pedersen, J. Chem. Phys., 1993, 98, 10089–10092 CrossRef CAS.
- R. A. Lippert, C. Predescu, D. J. Ierardi, K. M. Mackenzie, M. P. Eastwood, R. O. Dror and D. E. Shaw, J. Chem. Phys., 2013, 139, 164106 CrossRef PubMed.
- S. Nosé, J. Chem. Phys., 1984, 81, 511–519 CrossRef.
- G. J. Martyna, D. J. Tobias and M. L. Klein, J. Chem. Phys., 1994, 101, 4177–4189 CrossRef CAS.
- C. Predescu, A. K. Lerer, R. A. Lippert, B. Towles, J. P. Grossman, R. M. Dirks and D. E. Shaw, J. Chem. Phys., 2020, 152, 084113 CrossRef CAS PubMed.
- V. Kräutler, W. F. Van Gunsteren and P. H. Hünenberger, J. Comput. Chem., 2001, 22, 501–508 CrossRef.
- W. Humphrey, A. Dalke and K. Schulten, J. Mol. Graphics, 1996, 14, 33–38 CrossRef CAS PubMed.
- C. R. Harris, K. J. Millman, S. J. Van Der Walt, R. Gommers, P. Virtanen, D. Cournapeau, E. Wieser, J. Taylor, S. Berg, N. J. Smith, R. Kern, M. Picus, S. Hoyer, M. H. Van Kerkwijk, M. Brett, A. Haldane, J. F. Del Río, M. Wiebe, P. Peterson, P. Gérard-Marchant, K. Sheppard, T. Reddy, W. Weckesser, H. Abbasi, C. Gohlke and T. E. Oliphant, Nature, 2020, 585, 357–362 CrossRef CAS PubMed.
- N. Michaud-Agrawal, E. J. Denning, T. B. Woolf and O. Beckstein, J. Comput. Chem., 2011, 32, 2319–2327 CrossRef CAS PubMed.
- J. D. Hunter, Comput. Sci. Eng., 2007, 9, 90–95 Search PubMed.
- P. Virtanen, R. Gommers, T. E. Oliphant, M. Haberland, T. Reddy, D. Cournapeau, E. Burovski, P. Peterson, W. Weckesser, J. Bright, S. J. Van Der Walt, M. Brett, J. Wilson, K. J. Millman, N. Mayorov, A. R. J. Nelson, E. Jones, R. Kern, E. Larson, C. J. Carey, İ. Polat, Y. Feng, E. W. Moore, J. VanderPlas, D. Laxalde, J. Perktold, R. Cimrman, I. Henriksen, E. A. Quintero, C. R. Harris, A. M. Archibald, A. H. Ribeiro, F. Pedregosa, P. Van Mulbregt, SciPy 1.0 Contributors, A. Vijaykumar, A. P. Bardelli, A. Rothberg, A. Hilboll, A. Kloeckner, A. Scopatz, A. Lee, A. Rokem, C. N. Woods, C. Fulton, C. Masson, C. Häggström, C. Fitzgerald, D. A. Nicholson, D. R. Hagen, D. V. Pasechnik, E. Olivetti, E. Martin, E. Wieser, F. Silva, F. Lenders, F. Wilhelm, G. Young, G. A. Price, G.-L. Ingold, G. E. Allen, G. R. Lee, H. Audren, I. Probst, J. P. Dietrich, J. Silterra, J. T. Webber, J. Slavič, J. Nothman, J. Buchner, J. Kulick, J. L. Schönberger, J. V. De Miranda Cardoso, J. Reimer, J. Harrington, J. L. C. Rodríguez, J. Nunez-Iglesias, J. Kuczynski, K. Tritz, M. Thoma, M. Newville, M. Kümmerer, M. Bolingbroke, M. Tartre, M. Pak, N. J. Smith, N. Nowaczyk, N. Shebanov, O. Pavlyk, P. A. Brodtkorb, P. Lee, R. T. McGibbon, R. Feldbauer, S. Lewis, S. Tygier, S. Sievert, S. Vigna, S. Peterson, S. More, T. Pudlik, T. Oshima, T. J. Pingel, T. P. Robitaille, T. Spura, T. R. Jones, T. Cera, T. Leslie, T. Zito, T. Krauss, U. Upadhyay, Y. O. Halchenko and Y. Vázquez-Baeza, Nat. Methods, 2020, 17, 261–272 CrossRef CAS PubMed.
- I. Levental and E. Lyman, Nat. Rev. Mol. Cell Biol., 2023, 24, 107–122 CrossRef CAS PubMed.
- A. Grossfield, S. E. Feller and M. C. Pitman, Proc. Natl. Acad. Sci. U. S. A., 2006, 103, 4888–4893 CrossRef CAS PubMed.
- R. A. Corey, P. J. Stansfeld and M. S. P. Sansom, Biochem. Soc. Trans., 2020, 48, 25–37 CrossRef CAS PubMed.
- B. I. Sejdiu and D. P. Tieleman, Biophys. J., 2020, 118, 1887–1900 CrossRef CAS PubMed.
- H.-Y. Yen, K. K. Hoi, I. Liko, G. Hedger, M. R. Horrell, W. Song, D. Wu, P. Heine, T. Warne, Y. Lee, B. Carpenter, A. Plückthun, C. G. Tate, M. S. P. Sansom and C. V. Robinson, Nature, 2018, 559, 423–427 CrossRef CAS PubMed.
- M. Damian, M. Louet, A. A. S. Gomes, C. M’Kadmi, S. Denoyelle, S. Cantel, S. Mary, P. M. Bisch, J.-A. Fehrentz, L. J. Catoire, N. Floquet and J.-L. Banères, Nat. Commun., 2021, 12, 3938 CrossRef CAS PubMed.
- R. Guixà-González, J. L. Albasanz, I. Rodriguez-Espigares, M. Pastor, F. Sanz, M. Martí-Solano, M. Manna, H. Martinez-Seara, P. W. Hildebrand, M. Martín and J. Selent, Nat. Commun., 2017, 8, 14505 CrossRef PubMed.
- S. Genheden, J. W. Essex and A. G. Lee, Biochim. Biophys. Acta, Biomembr., 2017, 1859, 268–281 CrossRef CAS PubMed.
- A. G. Lee, Biophys. J., 2019, 116, 1586–1597 CrossRef CAS PubMed.
- D. Sengupta and A. Chattopadhyay, Biochim. Biophys. Acta, Biomembr., 2015, 1848, 1775–1782 CrossRef CAS PubMed.
- H. I. Ingólfsson, M. N. Melo, F. J. van Eerden, C. Arnarez, C. A. Lopez, T. A. Wassenaar, X. Periole, A. H. de Vries, D. P. Tieleman and S. J. Marrink, J. Am. Chem. Soc., 2014, 136, 14554–14559 CrossRef PubMed.
|
This journal is © The Royal Society of Chemistry 2025 |
Click here to see how this site uses Cookies. View our privacy policy here.