DOI:
10.1039/C4SC03416C
(Edge Article)
Chem. Sci., 2015,
6, 2812-2821
Computational discovery and experimental verification of tyrosine kinase inhibitor pazopanib for the reversal of memory and cognitive deficits in rat model neurodegeneration†
Received
6th November 2014
, Accepted 12th January 2015
First published on 13th January 2015
Abstract
Cognition and memory impairment are hallmarks of the pathological cascade of various neurodegenerative disorders. Herein, we developed a novel computational strategy with two-dimensional virtual screening for not only affinity but also specificity. We integrated the two-dimensional virtual screening with ligand screening for 3D shape, electrostatic similarity and local binding site similarity to find existing drugs that may reduce the signs of cognitive deficits. For the first time, we found that pazopanib, a tyrosine kinase inhibitor marketed for cancer treatment, inhibits acetylcholinesterase (AchE) activities at sub-micromolar concentration. We evaluated and compared the effects of intragastrically-administered pazopanib with donepezil, a marketed AchE inhibitor, in cognitive and behavioral assays including the novel object recognition test, Y maze and Morris water maze test. Surprisingly, we found that pazopanib can restore memory loss and cognitive dysfunction to a similar extent as donepezil in a dosage of 15 mg kg−1, only one fifth of the equivalent clinical dosage for cancer treatment. Furthermore, we demonstrated that pazopanib dramatically enhances the hippocampal Ach levels and increases the expression of the synaptic marker SYP. These findings suggest that pazopanib may become a viable treatment option for memory and cognitive deficits with a good safety profile in humans.
Introduction
With the increase in the elderly population worldwide, neurodegenerative disorders such as Alzheimer’s disease (AD),1 Parkinson’s disease (PD)2 and Huntington’s disease (HD)3 remain to be devastating diseases for which effective treatments are urgently needed. Memory dysfunction and cognition impairment are the most common symptoms affecting patients with neurodegenerative diseases.4 To date, only four approved small-molecule therapeutics are on the market, of which three are acetylcholinesterase (AchE) inhibitors (donepezil, rivastigmine and galanthamine), for the treatment of mild to moderate AD symptoms.5 Unfortunately, there is no definitive evidence yet showing that the use of donepezil or other agents can alter the course of AD progression. Hence, the search is still on for molecules with tolerable side effects to benefit older patients with neurodegenerative disorders.
Traditionally, binding affinity has been used as the criterion in the virtual screening process for drug discovery. Yet, binding specificity is also crucial in the practice of drug design and discovery.6 We developed a novel way to quantify specificity7,8 based on our energy landscape theory of ligand binding.9,10 The native binding mode and the non-native conformations during the binding between a ligand and a receptor are statistically distributed in energy. Herein, specificity is quantified by the ISR (Intrinsic Specificity Ratio) value, calculated as
, where δE is the energy gap between the native binding mode and the average non-native binding states, ΔE is the energy variance or spread of the non-native states and S is the configurational entropy measuring the size of the configurational space, which scales with the size of the system (number of atoms). In other words, the entropy S represents the configurational entropy measuring the size of the configurational space that scales with the size of the system (number of atoms), which is the ligand–receptor binding complex in our study.
In the current study, the receptor targets are fixed as rigid, the ligands are flexible with certain flexible torsional bonds, the entropy S depends approximately linearly on the number of torsional bonds of each ligand molecule with a constant coefficient on the order of 1. S is only related to the size of the system, which is constant during the binding process for a specific ligand binding to the receptor. Consequently, a large ISR value leads to a high level of discrimination of the native binding mode from the non-native binding modes, which implies high intrinsic specificity.9,10 One can imagine a large receptor as composed of small receptors connected together through linkers. Then the explorations of the structures (binding modes) for the same (large) target and explorations of the sequences for different (small) receptors for the same ligand are approximately equivalent.7,8 Therefore, the intrinsic specificity is expected to be correlated with the conventional specificity of discriminating by affinities among different receptors for the same ligand7,8 for large receptors. In this way, one can quantify the intrinsic specificity with only the specific target and infer the conventional specificity without the need for searching through the entire receptor universe. The two-dimensional virtual screening strategy covering not only affinity but also specificity can be used to search for drug candidates such as inhibitors of COX-2,7,8 human serum albumin (HSA)11 and Ras protein12etc.
In this work, we first employed the two dimensional virtual screening strategy for 1385 FDA-approved small molecule drugs with AchE as the receptor structure. Interestingly, three tyrosine kinase inhibitors (TKIs), sorafenib, pazopanib and sunitinib stand out as three top hits. Meanwhile, we used ligand-based virtual screening with donepezil as the query molecule to search against the 1385 FDA-approved small molecule drugs retrieved from the DrugBank database.13 The ligand virtual screening was performed by the ROCS program for similar three-dimensional shapes14 and the EON program for electrostatics.15 Consistent with the results from the above two-dimensional virtual screenings, three TKIs (sorafenib, pazopanib and sunitinib) are also among the top 50 hits of the ligand virtual screening. In addition, we employed a local binding site similarity screening16,17 with AchE as the query structure to search against a set of 1105 crystallographic structures of 377 approved drug targets retrieved from the DrugBank. Intriguingly, the majority of the top-ranked targets belong to protein kinases. Remarkably, consistent with the computational prediction, we found that pazopanib inhibits AchE with sub-micromolar affinity. We explored pazopanib for its ability to rescue memory dysfunction and cognitive deficits in a rat model induced by quinolinic acid (QA).18 For the first time, we found that pazopanib is able to restore memory and cognitive deficits to a similar extent as donepezil in a dosage of just 15 mg kg−1.
Results
Two-dimensional drug screening of both affinity and specificity against AchE
The two-dimensional virtual screening of 1385 FDA-approved small molecule drugs (http://www.drugbank.ca/) against AchE was performed by the AutoDock 4.20 program (see Fig. 1). The binding affinity and ISR value for each molecule were recorded and used for the ranking. The 1385 approved drugs were used for making the contour map for the two-dimensional drug screening (Fig. 2b). Donepezil has high values in both affinity (−12.24 kcal mol−1) and intrinsic specificity (ISR = 3.31) among all the approved drugs. Three TKIs, sorafenib (affinity: −10.41 kcal mol−1; ISR: 3.00), pazopanib (affinity: −11.80 kcal mol−1; ISR: 2.61) and sunitinib (affinity: −9.74 kcal mol−1; ISR: 2.26) are the other three top hits considering both affinity and the ISR value.
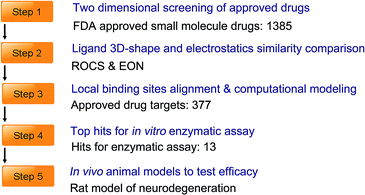 |
| Fig. 1 Discovery process of pazopanib for the rescue of neurodegeneration. | |
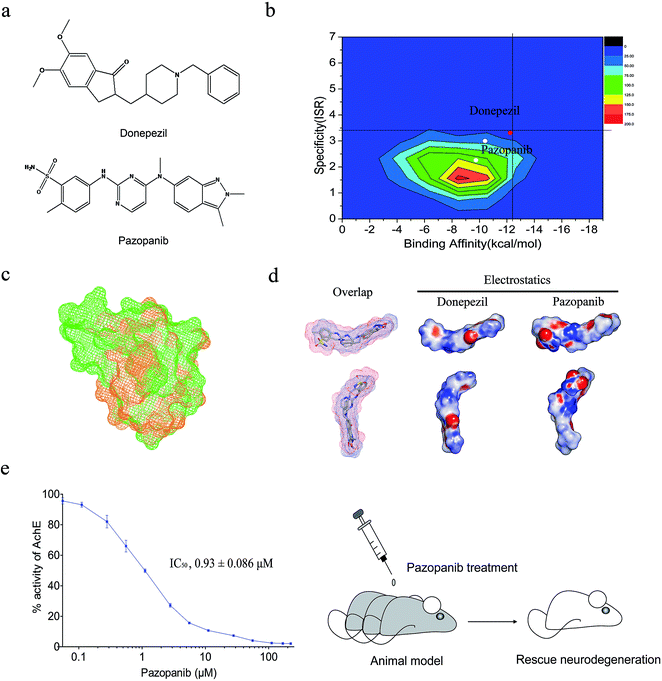 |
| Fig. 2 Identification of pazopanib as a potent ligand of AchE. (a) Two-dimensional chemical structures of marketed drugs donepezil and pazopanib; (b) the contour map of affinity and ISR value for 1385 approved drugs. Donepezil is depicted with a red dot and pazopanib is depicted as a yellow dot. Sorafenib and sunitinib are depicted as white dots. The vertical axis represents ISR value and the horizontal axis represents binding affinity; (c) local binding site alignment of VEGFR2 (vascular endothelial growth factor receptor 2, PDB ID: 3CJG, drug: pazopanib) versus AchE (PDB ID: 1EVE, drug: donepezil) by the TM-align program. VEGFR2 is depicted as the green mesh and AchE is depicted as the orange mesh. The alignment TM-score is 0.31; (d) overlay and three-dimensional chemical structures of donepezil and pazopanib with electrostatic surfaces coded by color (red for negative charges and blue for positive charges); (e) in vitro AchE enzymatic assay and in vivo animal model test. IC50 value of pazopanib: 0.93 ± 0.086 μM. The animal model shows that pazopanib can rescue neurodegeneration. | |
Ligand virtual screening with 3D shape and electrostatic comparison
The three-dimensional shape comparison between donepezil and 1385 FDA-approved drugs (http://www.drugbank.ca/) was performed by the ROCS program implemented within OpenEye (Rapid Overlay of Chemical Structures, version 3.1, OpenEye Scientific Software). The unique feature of ROCS is that it ranks compounds by their shape Tanimoto score, a quantitative assessment of three-dimensional overlap with a range from 0–1 (1 represents complete overlap). The molecule shape is computed by atom-centered overlapping Gaussian functions and the shape Tanimoto score is calculated by the maximal intersection of the volume of two molecules. The top 50 ‘hits’ had Tanimoto scores between 0.65 and 0.83. Next, we re-ranked the ROCS hits for electrostatic similarity with donepezil by the program EON (version, 3.1, OpenEye, Scientific Software), which computes the electrostatic Tanimoto score ranging from one (identical) to negative values judging by the overlap results of positive and negative charges. The electrostatic Tanimoto scores for the ROCS hits in the EON comparison ranged from −0.51 to 0.65. The final top 50 hits were re-ranked by the score of EON_ET_Combo, which is the sum of the 3D similarity and electrostatic similarity. The results were then manually inspected. Nearly one third of the top 50 hits belong to antipsychotic and antidepressant drugs (17 out of 50, see ESI† Table 3). For practical reasons, they were ruled out from further experimental testing. Interestingly, sorafenib, pazopanib and sunitinib, three tyrosine kinase inhibitors (TKIs), are among the top 50 hits (see ESI† Table 3). Pazopanib and donepezil were superimposed and displayed using the VIDA program (version 3.1, OpenEye Scientific Software) with a shape Tanimoto value of 0.612 and electrostatic Tanimoto score of 0.675 (Fig. 2d).
Local binding site similarity of AchE with approved drug targets
The searching of local binding site similarity among multiple targets is essential for studying polypharmacology.19 We compiled 377 FDA-approved drug targets from the DrugBank which led us to retrieve 1105 crystallographic structures from the PDB bank. Next, we searched and compared the pairwise ligand binding site similarity between AchE and the 1105 protein targets using TM-align,20 a structure alignment and comparison tool recommended by the PDB bank (http://www.pdb.org). Interestingly, 14 out of the top 20 targets ranked by local binding site similarity belong to the protein kinase family and the majority belong to the tyrosine kinase family including VEGFR2, EGFR, Src, JAK1, ERBB4, ERBB2, Lyn, JAK2, FGFR2, MAPK2 and HCK (see ESI† Tables 1 and 2). The remaining protein targets belong to the carboxylesterase, opioid receptor and phospholipase families, etc. The pairwise similarity TM-score between the pocket of VEGFR2 and AchE is about 0.31 (TM-score > 0.30 suggests significance, see Fig. 2c, ESI† Tables 1 and 2).
AchE enzymatic assay screening
The AchE enzymatic assay screening experiments determined that pazopanib is the most potent molecule among thirteen TKIs with an IC50 value of 0.93 μM (see Fig. 2e). The IC50 value of sunitinib is 5.87 μM. Sorafenib as well as other TKIs are weak binders for AchE (IC50 > 10 μM, see ESI† Table 4 for details).
Pazopanib binds with AchE similarly to donepezil
We performed molecular docking combined with molecular dynamics (MD) simulations to assess the possible binding mode of pazopanib with AchE. In short, the MD simulations (three independent 300 ns simulation and one independent 1000 ns simulation) revealed that pazopanib can fit well into the pocket of AchE (see Fig. 3). Similar to donepezil, pazopanib makes putative hydrophobic contacts with Tyr279, Tyr121, Trp84, and Phe330–331. Moreover, analogous to donepezil, it seems that pazopanib does not interact directly with the catalytic triad of AchE21 (Ser200, Glu327 and His440, see Fig. 3b). These results suggest that pazopanib binds with AchE similarly to donepezil.
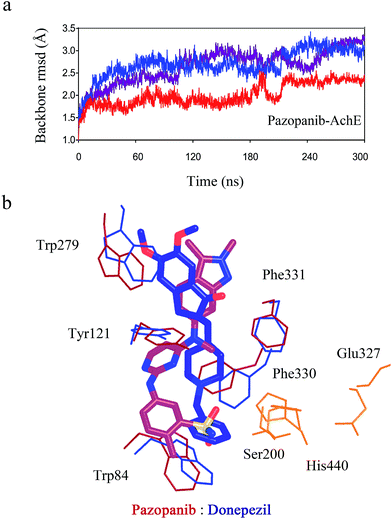 |
| Fig. 3 Computational modeling of pazopanib complexed with AchE. (a) Three independent long-timescale (300 ns) molecular dynamics simulations of pazopanib with AchE; (b) overlay of the complexed structure of pazopanib with AchE and the co-crystallized structure of donepezil with AchE (PDB ID: 1EVE). Pazopanib is depicted as the red stick model and donepezil is depicted as the blue stick model. The key residues in the catalytic pocket of pazopanib complexed with AchE are depicted as red lines and the key residues for the co-crystallized structure of donepezil with AchE are depicted as blue lines. The catalytic triad of AchE is depicted as orange lines. | |
Effects of pazopanib on cognition impairment of a rat model in the novel object recognition test
To assess whether pazopanib can restore cognition deficits, we used the novel object recognition test22 as a behavioral assay to evaluate recognition memory. Compared to the control group, the model group showed significant impairment in novel object exploration/recognition. On the other hand, both the group treated with donepezil and the group treated with pazopanib showed improved performance in the novel object recognition test (see Fig. 4a and b). The group treated with pazopanib in a high dose (15 mg kg−1) returned this phenotype to a similar extent as the treatment group with donepezil (0.95 mg kg−1).
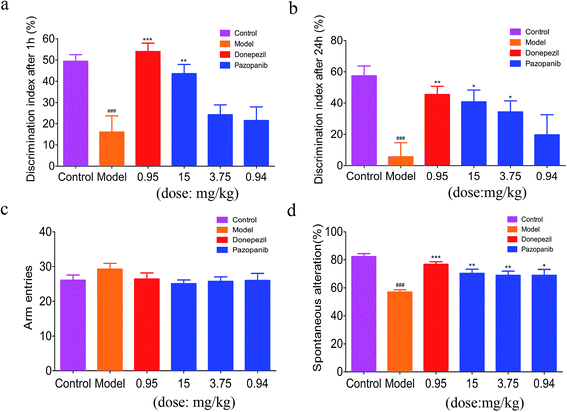 |
| Fig. 4 Effect of pazopanib on memory and cognition impairment in the novel object test and Y maze test. (a) The discrimination index for novel object recognition at 1 h into the test session; (b) the discrimination index for novel object recognition at 24 h into the test session. Results were expressed as the mean ± SEM (n = 10–12) and analyzed with one-way ANOVA, followed by Dunnett's post test for multiple comparisons. ###P < 0.001 vs. the control group; ***P < 0.001, **P < 0.01, *P < 0.05 vs. the model group; (c and d) effect of pazopanib on the impairment of spontaneous alteration of behavior in the Y maze test in rats. Results were expressed as the mean ± SEM (n = 10–12) and analyzed with one-way ANOVA, followed by Dunnett's post test for multiple comparisons. ###P < 0.001 vs. control group; ***P < 0.001, **P < 0.01, *P < 0.05 vs. model group. | |
Effects of pazopanib on memory dysfunction in the Y maze cognitive behavioral assay
The Y maze cognitive behavioral assay23 is conducted to measure the short-term working memory for model rats treated with pazopanib and donepezil. A significantly lower spontaneous alternation response rate was observed for the model group compared to the control group injected with PBS solution. In contrast, the group treated with pazopanib restored this phenotype to a similar extent as the group treated with donepezil (Fig. 4c and d). These data indicate that pazopanib can improve the short-term working memory of animals in a similar manner to donepezil.
Effect of pazopanib on spatial memory impairment in the Morris water maze
The Morris water maze (MWM) task demands incremental learning of a fixed platform location throughout the training period which results in the formation of spatial reference memory.24 Compared to the control-group rats (control group), we observed that the model group spent significantly more time and distance in finding the hidden platform. On the other hand, when donepezil (0.95 mg kg−1) was administered by intragastric infusion, the model rats showed a significant decrease in time and distance spent to find the hidden platform. Interestingly, when pazopanib was administered to the model rats, they showed a similar decrease in time and distance spent to find the hidden platform in a dose dependent manner (see Fig. 5a and b). Subsequently, we performed probe trial experiments in which the platform was removed. The model rats with spatial memory deficits were unable to develop a spatial preference for the area around the platform. By contrast, the control group as well as the model group administered with donepezil or pazopanib demonstrated a clear spatial preference in the correct quadrant of the platform (Fig. 5c and d). Moreover, bivariate histograms of the swimming patterns revealed that the group treated with pazopanib (15 mg kg−1) also developed a spatial preference in the correct quadrant with the platform removed (Fig. 5d). Together, the above results suggest that pazopanib can reverse the spatial memory deficits in a fashion similar to donepezil.
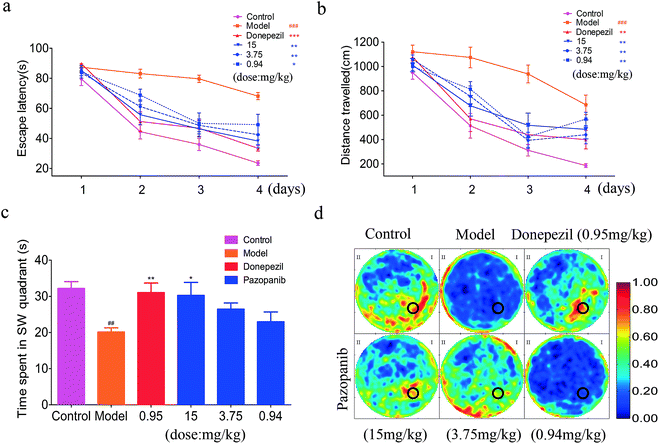 |
| Fig. 5 Effect of pazopanib on memory and cognition impairment in the Morris water maze test. (a) Effect of pazopanib on spatial memory impairment in the reference memory test in the water maze test in rats; (b) swimming distance spent to find the safety platform. Results were expressed as the mean ± SEM (n = 10–12) and analyzed with repeated measures ANOVA followed by Dunnett's post test for multiple comparisons. ###P < 0.001 vs. the control group; ***P < 0.001, **P < 0.01, *P < 0.05 vs. the model group; (c) effect of pazopanib on spatial memory impairment in the probe test of the water maze test in rats. The vertical axis represents the time spent in the fourth quadrant where the platform was located. Results were expressed as the mean ± SEM (n = 10–12). ##P < 0.01 vs. the control group; **P < 0.01, *P < 0.05 vs. the model group; (d) spatial preference in the probe test of the water maze test. The heat map is used to represent the frequently visited areas as orange and red. The frequency of crossing is calculated as (number of crossings at each point)/(maximum number of crossings at all points). Red represents the most frequently visited areas. The location of the platform is represented as a black circle. | |
Pazopanib enhances hippocampal Ach levels in the model rats
We used an ELISA assay to detect acetylcholine (ACh) levels in the hippocampus of the control and drug-treated rat groups. In agreement with prior findings,25 the QA-induced rats demonstrate a significant reduction in hippocampal Ach release compared to the control group. Remarkably, pazopanib administration was able to prevent the QA-induced decrease in ACh level in a dose dependent manner (see Fig. 6a). The beneficial effect of 15 mg kg−1 of pazopanib was similar in extent to that seen in the donepezil-treated group. This demonstrates that pazopanib prevents cholinergic degeneration and enhances Ach levels in model rats.
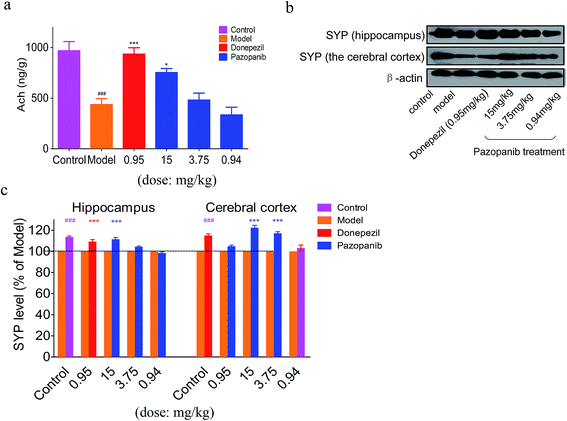 |
| Fig. 6 Effect of pazopanib on the hippocampal level of Ach and expression of synaptic markers. (a) The increase in Ach level in the hippocampus after treatment with donepezil or pazopanib in the rat model. Results were expressed as the mean ± SEM (n = 6) and analyzed with one-way ANOVA, followed by Dunnett's post test for multiple comparisons. ###P < 0.001 vs. the control group; ***P < 0.001, *P < 0.05 vs. the model group; (b and c) effect of pazopanib on the expression of synapse-related protein SYP in the hippocampus and the cerebral cortex of the rat model. The intensity of each protein band was quantified by densitometry using the Quantity One software (Bio-Rad, Hercules, CA, USA), and then corrected using the corresponding β-actin level. Results were expressed as the mean ± SEM (n = 6) and analyzed with one-way ANOVA, followed by Dunnett's post test for multiple comparisons. ###P < 0.001 vs. the control group; ***P < 0.001 vs. the model group. | |
Pazopanib increases the expression of synaptic markers in the model rats
Synaptophysin (SYP) is a synaptic vesicle protein and has been widely used as a presynaptic marker for presynaptic terminals.26 The expression level of SYP is significantly correlated with cognitive degeneration and the progression of neurodegenerative diseases.27,28 We observed a decrease in the SYP level in the CA1 region of the model group compared to the control group by western blotting, whereas SYP expression level was increased in donepezil- and pazopanib-treated model rats (Fig. 6b and c). In addition, PSD-95 is also a synaptic marker and plays an important role in synaptic maturation and pathogenesis of neurodegenerative disorders.29 The effects of donepezil and pazopanib on the PSD-95 level in the hippocampus and the cerebral cortex of treated animals are not statistically significant compared to the control group. Nevertheless, we observe a trend towards the decrease in the PSD-95 level in the model group and a trend towards the increase in the PSD-95 level in the donepezil- and pazopanib-treated groups (see ESI† Fig. 2). These data suggest that pazopanib might be able to protect the synapses and prevent the progression of neurodegenerative diseases.
Discussion
There are still unmet needs for disease-modifying therapies of neurodegenerative disorders with convenient dosing and excellent safety profiles. The identification of approved drugs for new uses becomes an attractive strategy as a complement to conventional approaches. The established safety profile for existing drugs might substantially reduce the time and cost to advance candidate compounds into clinical trials.30 Recently, minocycline, a tetracycline antibiotic used to treat bacterial infection has been found to reduce the level of pro-inflammatory mediators and microglial activation in the AD mouse model.31 However, notably, the beneficial dosage of minocycline (40–50 mg per kg per day) in AD animals is about 1.25–1.45 fold higher than the recommended maximum clinical dose for the treatment of bacterial infection. More recently, Cramer et al. have found that the drug bexarotene, which is FDA-approved for the treatment of cutaneous T cell lymphoma, can rapidly reverse memory deficits in the AD mouse model.32 However, the effective dosage of bexarotene used in AD animal models was 3-fold higher than that used in clinical cancer treatment. Therefore, it is of priority to find candidate compounds to treat AD and other neurodegenerative disease with acceptable dosages and tolerability for older patients.
In light of this, we designed a computational strategy of coupling two-dimensional virtual and ligand-based screening with analysis of 3D shape and electrostatic similarity, and comparison of local binding site similarity to search approved drugs for the treatment of AD and other neurological disorders (see Fig. 1). The intrinsic specificity ratio (ISR) for discrimination based on the energy landscape theory of ligand binding7 has been demonstrated to be well-correlated with the structural fit or structural specificity. Therefore, the concept of two-dimensional screening has the advantages of considering both affinity and specificity, the two requirements for efficient biomolecular recognition.33,34 The comparison of ligand shape and electrostatic similarity is based on the rationale that two ligands similar in volume and physical–chemical properties are likely to have similar target-binding activities. For instance, this approach has been found quite useful by Churchill et al.35 in the identification of the first small molecular inhibitor of NAADP (nicotinic acid adenine dinucleotide phosphate). On the other hand, the local binding site similarity comparison is based on the hypothesis that similar binding sites most likely bind the same molecules. This approach has led to a few successful reports36 such as the identification of HIV-protease inhibitor Nelfinavir as an anti-cancer agent37 and the identification of the PD drug Comtan for the treatment of tuberculosis.38 The ligand 3D shape and electrostatic similarity comparison of donepezil with 1385 approved drugs has indicated that three TKIs (sunitinib, pazopanib and sorafenib, see ESI† Table 3) are among the top 50 hits. We compared the binding pocket of AchE with the 1105 ligand binding sites of approved drug targets by a pairwise structure alignment and a similarity comparison tool. The local binding site similarity comparison study has concluded that the majority of the top-ranked hits (70%, 14 out of top 20, see ESI† Tables 1 and 2) belong to protein kinases among which 8 hits are for protein tyrosine kinases (PTK). We chose and purchased TKIs for further experimental testing for two practical reasons: (1) TKIs are among the top hits in our integrated computational pipelines; (2) TKIs are structurally divergent molecules with easy accessibility. We identified pazopanib as the most potent molecule with sub-micromolar affinity among the 13 purchased TKIs by enzymatic assay (see ESI† Table 4). Notably, this is the first report of integrating two-dimensional virtual screening, ligand-based screening and comparison of local binding site similarity to narrow down the list of candidate molecules for further experimental testing.
Our results suggest that pazopanib restored memory loss and cognition impairment in a rat model induced by quinolinic acid (QA). We found that pazopanib improves learning and memory deficits in a dose-dependent manner through in vivo experiments. In particular, pazopanib treatment at a dosage of 15 mg kg−1 prevented the QA-induced neurodegeneration to a similar extent as donepezil at a dosage of 0.95 mg kg−1 for rats, equivalent to the maximum dosage of 10 mg per day for human. This is significant because our findings demonstrated that pazopanib restored the cognition and memory deficits at only one fifth of the equivalent dosage used for the treatment of carcinoma (the recommended clinical dosage of pazopanib is 800 mg daily for cancer treatment39). Hence, the risk of side effects for pazopanib in the treatment of neurodegenerative diseases might be minimal, given its well-tolerated safety profile in cancer treatment.
In the present study, we demonstrated the efficacy of pazopanib using the QA-induced animal model because it has been well established that QA leads acutely to the loss of cholinergic neurons and therefore reproduces the neuroinflammatory events in diseases including AD, PD and HD.40,41 Moreover, the QA-induced animal model has been widely used as it resembles common pathological features of neurodegenerative disorders.42,43 However, given that the exact pathways or pathologies of neurodegenerative diseases are not definitely identified and continue to be a source of debate, one single animal model is insufficient to determine the effects of pazopanib for neurodegenerative disorders. Therefore, further studies in various animal models44 are warranted to assess pazopanib as an effective therapeutic for neurodegeneration.
In summary, by integrating methods of two-dimensional screening, ligand-based virtual screening, computational modeling and in vitro and in vivo experimental testing, we predicted and found that pazopanib is a sub-micromolar affinity ligand of AchE and is capable of ameliorating memory and cognitive dysfunctions that characterize neurodegenerative disorders. Notably, pazopanib showed a similar extent of activities as donepezil at a much lower dosage than that used to treat cancer. Another significance of the present work is that we provide a useful paradigm for evaluating new uses for approved drugs. In particular, the concept of virtual screening based on both affinity and specificity could be generally applied in the drug discovery pipeline. Neurodegeneration is a form of multifactorial disease and a variety of mechanisms may contribute to its pathogenesis. Further studies are warranted to clarify the detailed mechanism and the impact of pazopanib for the treatment of neurodegeneration. To the best of our knowledge, this is the first report on the effect of pazopanib in abrogating memory and cognitive deficits under in vivo conditions.
Materials and methods
Two dimensional screening of approved drugs
The Autodock 4.20 program was used to perform the two-dimensional screening of approved small molecule drugs against AchE. For each molecule, we calculated the binding affinity, the energy gap between the lowest binding energy state and average binding energy and the variance in the free energies of different binding modes. The configurational entropy, which measures the size of the configurational space that scales with the size of the system (number of atoms), is also calculated. The ISR value is calculated as
.
Ligand virtual screening by shape and electrostatics
We used OMEGA 2.4.6 to generate 100 conformers for each molecule of 1385 approved drugs. The input donepezil structure was entered as a query molecule for the initial screening. We used ROCS (3.1.2) for 3-dimensional shape comparisons and the top 500 hits were output in the order of shape Tanimoto values. For comparisons of electrostatic properties (EON 2.10), the lowest-energy conformer of donepezil was used for all comparisons to re-rank the top 500 ROCS hits in the order of electrostatic Tanimoto scores. Lastly, the top 50 hits were ranked by EON_combo, the sum of 3D shape Tanimoto score and electrostatic Tanimoto score (ESI† Table 3).
Local binding site similarity search
The crystallographic structure of AchE complexed with donepezil has been solved (PDB ID: 1EVE). 1105 protein target structures co-crystallized with ligands from the PDB bank (http://www.pdb.org/) were compiled for use. Herein, the ligand binding pocket was defined as the residues within 8 Å of the complexed ligand and the ligand binding pockets of the 1200 protein targets were extracted using the Pymol program (Educational version 1.3). Amino acid residues within 8 Å of the co-crystallized ligand were defined as the ligand binding pocket. The TM-align program was then used to compute the pairwise ligand binding site similarity between human AchE and 1105 protein targets. The alignment results were ranked in descending order (see ESI† Tables 1 and 2).
Animals used in the experiments
The experiments were performed strictly following the ethics regulation and institutional guidelines of Shenyang Pharmaceutical University. The Sprague-Dawley (SD) rats were maintained at 21 °C in standard ventilated cages holding 3 rats per cage and water ad libitum.
Rodent model of neurodegeneration induced by quinolinic acid (QA)
An injection of 2 μl PBS solution (pH 7.4) containing 120 nmol quinolinic acid (QA; Sigma, Shanghai) was applied to both left and right nucleus basalis of Meynert (NBM) of the animals following the standard protocol. The control group animals received an injection of 2 μl of the vehicle PBS solution.
Drug treatment of the experimental animals
For treating experimental rats, donepezil and pazopanib (as salts) were dissolved in physiological saline containing 0.5% Tween-80. Experimental rats received a daily dose of drugs or vehicle by intragastric administration until the end of the experiments. For the behavioral assay, drug treatments were conducted after the last trial of every day during the testing period.
Novel object recognition task
The object recognition task was performed in an open circle arena (80 cm × 80 cm). After habituation, two identical objects (A1, A2) were placed in the arena with an equal distance to the edge. Subsequently, the rats were exposed to the familiar open arena and allowed to explore for 5 min. The time that rats spent exploring each object (tA1, tA2) was recorded. After one hour, one object was replaced with a new object (B) and the time spent exploring each object (tA1, tB) was recorded. Similarly, after 24 hours, object B was replaced with another new object C and the time spent exploring each object (tA1, tC) was recorded. The object preferential index and object discrimination index were used to evaluate the performance in object recognition and calculated as: (1) preferential index (1 h) = tB/(tA1 + tB); (2) preferential index (24 h) = tC/(tA1 + tC); (3) discrimination index (1 h) = (tB − tA1)/(tA1 + tB); (4) discrimination index (24 h) = (tC − tA1)/(tC + tB).
Y maze cognitive behavioral assay
During the experiments, each rat was first placed at one end of the arm and the total number (N) and the order of the arm entries were recorded by video camera for every 8 minutes. Successful spontaneous alternations are defined as consecutive triple entries of different arms choices. The spontaneous alternation response rate is calculated as: spontaneous alternation rate (%) = number of successful alternation/(N − 2) × 100.
Morris water maze task and probe trial test
In brief, the water maze consisted of a dark gray pool filled with opaque water. The testing platform was hidden below the opaque water surface but accessible for the rats. The animals were subjected to two trials per day for 4 days with a video system monitoring and recording the percentage of time spent in the various quadrants. On the next day, the platform was removed and each rat was allowed to explore in the water pool for 90 s. The swimming time and the swimming distance in the quadrant where the platform was located were calculated.
Tissue handling and western blotting
Upon anesthesia, the animals were perfused transcardially with ice-cold saline solution and then paraformaldehyde solution (pH 7.4, 3.5% in phosphate buffer). The brain was immediately isolated and postfixed in 4% paraformaldehyde–2% glutaraldehyde for 48 hours at 4 °C before processing for immunohistochemical analysis. Animals for western blotting analysis were dissected rapidly. Proteins (SYP and PSD-95) were detected using horseradish peroxidase-conjugated secondary antibodies (Santa Cruz Biotechnology and Abcam, USA).
Determination of Ach levels
The remaining half of the 10% (w/v) homogenate (without sodium dodecyl sulfate (SDS)) from the immunohistochemical analysis was centrifuged for 30 min at 4 °C without adding any detergent. Supernatants containing Ach were collected for assays. Brain extracts (soluble protein from PBS and insoluble protein from formic acid) from the animals were used for two-site ELISAs that specifically detect the Ach levels as suggested by the manufacturer (Shanghai HoraBio).
Molecular dynamics simulation
The starting structure of the MD simulation was obtained from the best binding mode of docking. One 1000 ns and three 300 ns production molecular dynamics simulations were performed using PMEMD.CUDA enabled NVIDIA graphics processing units (GPUs) implemented with Amber 10.
Statistical analysis
Student's t-test and one-way and two-way analysis of variance (ANOVA) with Dunnett's post-test were performed using GraphPad Prism version 5.00 for Windows in statistical analysis. P < 0.05 was considered significant.
Conflict of interest
The authors have declared that no competing financial interests exist.
Acknowledgements
The Y. Yang laboratory thanks the Research Funds for Key Laboratory of Liaoning Educational Council (Grant: L2013025) and National Science Foundation in China, Medical Board Oncology Department for support. X.Z. thanks National Natural Science Foundation of China (Grant no. 21190040, 11174105, 91227114, 91430217) for financial support. X.Z. is grateful to Computing Center of Jilin Province for essential support. J.W. thanks NSF for support. G. Li thanks 973 project: (grant: 2012CB721000), 863 project (grant: 2012AA01A305) and NSFC project (grant: 31070641) for support. Y. Yang wishes to thank Prof. S. James Adelstein from Harvard Medical School for his support all along and his critical reading and comments on the manuscript.
References
- K. Blennow, M. J. de Leon and H. Zetterberg, Alzheimer's disease, Lancet, 2006, 368(9533), 387–403 CrossRef CAS.
- A. J. Lees and E. Smith, Cognitive deficits in the early stages of Parkinson's disease, Brain, 1983, 106(Pt 2), 257–270 CrossRef PubMed.
- F. O. Walker, Huntington's Disease, Semin. Neurol., 2007, 27(2), 143–150 CrossRef PubMed.
- C. Ballard,
et al. Alzheimer's disease, Lancet, 2011, 377(9770), 1019–1031 CrossRef.
- A. Corbett,
et al. Drug repositioning for Alzheimer's disease, Nat. Rev. Drug Discovery, 2012, 11(11), 833–846 CrossRef CAS PubMed.
- A. Wlodawer and J. W. Erickson, Structure-based inhibitors of HIV-1 protease, Annu. Rev. Biochem., 1993, 62, 543–585 CrossRef CAS PubMed.
- J. Wang,
et al. Quantifying intrinsic specificity: a potential complement to affinity in drug screening, Phys. Rev. Lett., 2007, 99(19), 198101 CrossRef.
- Z. Yan, X. Zheng, E. Wang and J. Wang, Thermodynamic and kinetic specificities of ligand binding, Chem. Sci., 2013, 4, 2387–2395 RSC.
- J. Wang and G. M. Verkhivker, Energy landscape theory, funnels, specificity, and optimal criterion of biomolecular binding, Phys. Rev. Lett., 2003, 90(18), 188101 CrossRef.
- J. N. Onuchic, Z. Luthey-Schulten and P. G. Wolynes, Theory of protein folding: the energy landscape perspective, Annu. Rev. Phys. Chem., 1997, 48, 545–600 CrossRef CAS PubMed.
- Z. Liu, X. Zheng, X. Yang, E. Wang and J. Wang, Affinity and specificity of levamlodipine-human serum albumin interactions: insights into its carrier function, Biophys. J., 2009, 96(10), 3917–3925 CrossRef CAS PubMed.
- X. Zheng, Z. Liu, D. Li, E. Wang and J. Wang, Rational drug design: the search for Ras protein hydrolysis intermediate conformation inhibitors with both affinity and specificity, Curr. Pharm. Des., 2013, 19(12), 2246–2258 CrossRef CAS.
- V. Law,
et al. DrugBank 4.0: shedding new light on drug metabolism, Nucleic Acids Res., 2014, 42(Database issue), D1091–D1097 CrossRef CAS PubMed.
- S. W. Muchmore, A. J. Souers and I. Akritopoulou-Zanze, The use of three-dimensional shape and electrostatic similarity searching in the identification of a melanin-concentrating hormone receptor 1 antagonist, Chem. Biol. Drug Des., 2006, 67(2), 174–176 CAS.
- J. Bostrom, J. A. Grant, O. Fjellstrom, A. Thelin and D. Gustafsson, Potent fibrinolysis inhibitor discovered by shape and electrostatic complementarity to the drug tranexamic acid, J. Med. Chem., 2013, 56(8), 3273–3280 CrossRef PubMed.
- D. L. Ma, D. S. Chan and C. H. Leung, Drug repositioning by structure-based virtual screening, Chem. Soc. Rev., 2013, 42(5), 2130–2141 RSC.
- J. T. Dudley, T. Deshpande and A. J. Butte, Exploiting drug-disease relationships for computational drug repositioning, Briefings Bioinf., 2011, 12(4), 303–311 CrossRef CAS PubMed.
- L. Aubry,
et al. Striatal progenitors derived from human ES cells mature into DARPP32 neurons in vitro and in quinolinic acid-lesioned rats, Proc. Natl. Acad. Sci. U. S. A., 2008, 105(43), 16707–16712 CrossRef CAS PubMed.
- F. Milletti and A. Vulpetti, Predicting polypharmacology by binding site similarity: from kinases to the protein universe, J. Chem. Inf. Model., 2010, 50(8), 1418–1431 CrossRef CAS PubMed.
- Y. Zhang and J. Skolnick, TM-align: a protein structure alignment algorithm based on the TM-score, Nucleic Acids Res., 2005, 33(7), 2302–2309 CrossRef CAS PubMed.
- G. Kryger, I. Silman and J. L. Sussman, Structure of acetylcholinesterase complexed with E2020 (Aricept): implications for the design of new anti-Alzheimer drugs, Structure, 1999, 7(3), 297–307 CrossRef CAS.
- J. Karasawa, K. Hashimoto and S. Chaki, d-Serine and a glycine transporter inhibitor improve MK-801-induced cognitive deficits in a novel object recognition test in rats, Behav. Brain Res., 2008, 186(1), 78–83 CrossRef CAS PubMed.
- C. D. Conrad, L. A. Galea, Y. Kuroda and B. S. McEwen, Chronic stress impairs rat spatial memory on the Y maze, and this effect is blocked by tianeptine pretreatment, Behav. Neurosci., 1996, 110(6), 1321–1334 CrossRef CAS.
- R. Morris, Developments of a water-maze procedure for studying spatial learning in the rat, J. Neurosci. Methods, 1984, 11(1), 47–60 CrossRef CAS.
- E. Arenas, E. Perez-Navarro, J. Alberch and J. Marsal, Selective resistance of tachykinin-responsive cholinergic neurons in the quinolinic acid lesioned neostriatum, Brain Res., 1993, 603(2), 317–320 CrossRef CAS.
- M. Hilbe, F. Guscetti, S. Wunderlin and F. Ehrensperger, Synaptophysin: an immunohistochemical marker for animal dysautonomias, J. Comp. Pathol, 2005, 132(2–3), 223–227 CrossRef CAS PubMed.
- C. I. Sze,
et al. Loss of the presynaptic vesicle protein synaptophysin in hippocampus correlates with cognitive decline in Alzheimer disease, J. Neuropathol. Exp. Neurol., 1997, 56(8), 933–944 CrossRef CAS PubMed.
- D. L. King and G. W. Arendash, Maintained synaptophysin immunoreactivity in Tg2576 transgenic mice during aging: correlations with cognitive impairment, Brain Res., 2002, 926(1–2), 58–68 CrossRef CAS.
- X. Chen,
et al. PSD-95 is required to sustain the molecular organization of the postsynaptic density, J. Neurosci., 2011, 31(17), 6329–6338 CrossRef CAS PubMed.
- J. T. Dudley,
et al. Computational repositioning of the anticonvulsant topiramate for inflammatory bowel disease, Sci. Transl. Med., 2011, 3(96), 96ra76 CAS.
- R. Fan,
et al. Minocycline reduces microglial activation and improves behavioral deficits in a transgenic model of cerebral microvascular amyloid, J. Neurosci., 2007, 27(12), 3057–3063 CrossRef CAS PubMed.
- P. E. Cramer,
et al. ApoE-directed therapeutics rapidly clear beta-amyloid and reverse deficits in AD mouse models, Science, 2012, 335(6075), 1503–1506 CrossRef CAS PubMed.
- Z. Yan and J. Wang, Specificity quantification of biomolecular recognition and its implication for drug discovery, Sci. Rep., 2012, 2, 309 Search PubMed.
- Z. Yan and J. Wang, Optimizing scoring function of protein–nucleic acid interactions with both affinity and specificity, PLoS One, 2013, 8(9), e74443 CAS.
- E. Naylor,
et al. Identification of a chemical probe for NAADP by virtual screening, Nat. Chem. Biol., 2009, 5(4), 220–226 CrossRef CAS PubMed.
- L. Xie and P. E. Bourne, Structure-based systems biology for analyzing off-target binding, Curr. Opin. Struct. Biol., 2011, 21(2), 189–199 CrossRef CAS PubMed.
- L. Xie, T. Evangelidis and P. E. Bourne, Drug discovery using chemical systems biology: weak inhibition of multiple kinases may contribute to the anti-cancer effect of nelfinavir, PLoS Comput. Biol., 2011, 7(4), e1002037 CAS.
- S. L. Kinnings,
et al. Drug discovery using chemical systems biology: repositioning the safe medicine Comtan to treat multi-drug and extensively drug resistant tuberculosis, PLoS Comput. Biol., 2009, 5(7), e1000423 Search PubMed.
- A. Zivi, L. Cerbone, F. Recine and C. N. Sternberg, Safety and tolerability of pazopanib in the treatment of renal cell carcinoma, Expert Opin. Drug Saf., 2012, 11(5), 851–859 CrossRef CAS PubMed.
- C. Scali,
et al. The selective cyclooxygenase-2 inhibitor rofecoxib suppresses brain inflammation and protects cholinergic neurons from excitotoxic degeneration in vivo, Neuroscience, 2003, 117(4), 909–919 CrossRef CAS.
- C. Portera-Cailliau, J. C. Hedreen, D. L. Price and V. E. Koliatsos, Evidence for apoptotic cell death in Huntington disease and excitotoxic animal models, J. Neurosci., 1995, 15(5 Pt 2), 3775–3787 CAS.
- K. R. Leaver,
et al. Effects of translocator protein (18 kDa) ligands on microglial activation and neuronal death in the quinolinic-acid-injected rat striatum, ACS Chem. Neurosci., 2012, 3(2), 114–119 CrossRef CAS PubMed.
- M. F. Beal, R. J. Ferrante, K. J. Swartz and N. W. Kowall, Chronic quinolinic acid lesions in rats closely resemble Huntington's disease, J. Neurosci., 1991, 11(6), 1649–1659 CAS.
- C. Li, A. Ebrahimi and H. Schluesener, Drug pipeline in neurodegeneration based on transgenic mice models of Alzheimer's disease, Ageing Res. Rev., 2013, 12(1), 116–140 CrossRef PubMed.
Footnote |
† Electronic supplementary information (ESI) available. See DOI: 10.1039/c4sc03416c |
|
This journal is © The Royal Society of Chemistry 2015 |