DOI:
10.1039/C5AY02898A
(Paper)
Anal. Methods, 2016,
8, 153-161
Quartz crystal microbalance based biosensors for detecting highly metastatic breast cancer cells via their transferrin receptors†
Received
3rd November 2015
, Accepted 10th November 2015
First published on 13th November 2015
Abstract
A quartz crystal microbalance (QCM) biosensor was developed to detect highly metastatic breast cancer cells by functionalizing the gold sensor surface with transferrin attachment. MDA-MB 231 breast cancer cells with high and MCF 7 cells with low metastatic potential and transferrin expression were used. Serum starved MDA-MB-231 cells were used as control cells. First, poly(2-hydroxyethyl methacrylate) (PHEMA) nanoparticles were prepared by mini-emulsion polymerization of hydroxyethyl methacrylate (HEMA) and ethylene glycol dimethacrylate (EGDMA). Nanoparticles were characterized with a zeta-sizer and then their suspension is dropped on the surface of the QCM and the dried QCM surface was modified further by activation with carbodiimide and transferrin attachment. The QCM biosensor was analyzed by using atomic force microscopy (AFM), ellipsometry, Fourier transform infrared spectrophotometry (FTIR) and contact angle measurements. The cells were applied to the derivatized QCM sensor to investigate the affinity and binding kinetics. The nanoparticles and transferrin were found to form a monolayer on the QCM surface. Binding kinetics were best fitted to the Langmuir–Freundlich adsorption model. The QCM signal was correlated well with the number of transferrin receptors on cells. It is concluded that the QCM biosensor functioning via transferrin receptor interactions may be used to detect breast cancer cells with high metastatic potential.
1. Introduction
Breast cancer is the most common cancer among women and the second most common cancer overall.1 Treatment of metastatic breast cancer is still a big challenge despite developing strategies.2 Early diagnosis and detection of metastasis saves many lives. Detection and characterization of tumor cells are crucial in cancer treatment. Therefore, it is of prime importance to detect these cells in tumors as well as in the circulation.3 Discrimination of breast cancer cells with high metastatic potential from those of low potential may shed light on treatment regimes. Current breast cancer diagnosis largely depends on highly developed radiological methods such as mammography. However, this does not provide information on the metastatic power and the degree of malignancy of cancer cells. A variety of laboratory tests help reveal these characteristics. However, these methods are usually expensive, and they require long and complicated processes and instruments. Therefore, the development of simple, rapid and sensitive methods to be used in these molecular analyses has gained great importance.4,5 Biosensors offer an efficient and rapid methodology in diagnosis. The simple sample preparation, low sample volume, compact structure, rapid detection, and high selectivity and sensitivity are some of their useful features.6–9 A biosensor has two basic components: biological (receptor, enzyme, tissue, microorganisms, antibodies and a large variety of molecules including DNA) and physical (transducer). According to the transducer mechanism biosensors are grouped into optical, electrochemical, piezoelectric and thermometric.10 A quartz crystal microbalance (QCM) is a piezoelectric transducer, which is known to be highly precise, stable oscillators that are capable of detecting nanogram mass changes.11,12 QCM biosensors have been designed and fabricated to detect a large selection of molecules.13,14 Transferrin receptors are expressed in all cells to internalize transferrin by receptor-mediated endocytosis in order to supply iron. Transferrin receptors are not only highly specific for transferrin but also has a great affinity towards this molecule. This high affinity is utilized in specific targeting of drugs.15,16 As most cancer cells are in need of large amounts of iron, they express a great number of transferrin receptors. Metastatic breast cancer cells also overexpress the number of transferrin receptors as compared to their less metastatic counterparts.17–19 Therefore, we have selected this ligand–receptor interaction with high affinity and selectivity to form a biosensor.20 Nowadays, nanoparticles play an important role in improving sensor performance due to their large specific surface areas.21 Combining the advantage of nanoparticle large surface with high receptor affinity may provide an efficient detection system. The other essential part of a biosensor is the signal transducer QCM that converts changes in mass into electrical signals. Under optimum conditions, it can measure a mass change of 0.1–1.0 ng cm−2.22,23 The high sensitivity of the QCM is combined with sensitivity and selectivity of receptor–ligand interactions in order to construct a biosensor which would discriminate breast cancer cells with high metastatic power from those of low or no metastatic potential as an attempt to develop a simple, fast and efficient system to be used in breast cancer diagnosis.
2. Materials and methods
2.1. Cells
MDA-MB 231 (ATTC-HTB-26) human breast cancer cells with high metastatic power were cultured in dMEM, high glucose supplemented with 10% FBS, 2 mM L-glutamine and 1% antibiotic–antimycotic solution in a carbon dioxide (5%) incubator at 37 °C. To test the binding efficiency, MCF 7 (ATTC-HTB-22) breast cancer cells with low metastatic power and a low number of transferrin receptors were grown under similar conditions. Additionally, MDA MB 231 cells preserved in 10 mM PBS, pH: 7.4 for 24 hours in order to inactivate transferrin receptors were also tested as control. Cells were washed in PBS and counted using a hemocytometer. Samples containing different numbers of cells were applied to the QCM surface in PBS.
2.2. Preparation and characterization of nanoparticles
Poly(2-hydroxyethyl methacrylate) (PHEMA) nanoparticles were prepared by using a two phase mini-emulsion polymerization method.24 For polymerization, two different aqueous phases were prepared. The first one was prepared by dissolving 93.7 mg polyvinyl alcohol (PVA), 14.4 mg SDS and 11.7 mg sodium bicarbonate in 5 mL deionized water. The second phase was prepared by dissolving 50 mg PVA and 50 mg SDS in 100 mL deionized water. The monomer phase consisted of 0.45 mL HEMA and 1.05 mL EGDMA (ethylene glycol dimethacrylate). The monomer phase was added to the first phase to obtain a mini-emulsion; then the mixture was homogenized at 25
000 rpm. This mini-emulsion was slowly added to the second aqueous phase, which was being stirred in a sealed-cylindrical polymerization reactor. The reactor was stirred at 600 rpm. The polymerization mixture was heated to 40 °C. Then the initiators, 57.3 mg sodium bisulfide and 63 mg ammonium persulphate, were added to the mixture. The polymerization reaction was completed in 24 hours at 40 °C. Nanoparticles were washed with the water/ethyl alcohol mixture in order to remove unreacted monomers by centrifugation at 14
000 rpm. The size distribution of nanoparticles was determined by using a zeta-sizer (nanoS, Malvern Instruments, London, UK). Light scattering was done at incidence angles of 90 and 25 degrees. For data analysis, the density and refraction index of deionized water used were 0.88 mPa s and 1.33, respectively. The light scattering signal was calculated as nanoparticle number per second.
2.3. Preparation of QCM sensor chips
In order to attach nanoparticles to the QCM sensor, a 7 mL aliquot of 4.2% nanoparticle dispersion was dropped on the gold surface of the QCM sensor chips. The MAXTEK 5 MHZ Cr/Au polished quartz crystal chip was supplied from USA by INFICON. The AT-cut quartz is chosen for its superior mechanical and piezoelectric properties. Then, the sensor was dried in an oven at 37 °C for 6 h. Finally, the nanoparticle adsorbed QCM sensor surface was washed with deionized water and ethyl alcohol and dried in a vacuum incubator under 200 mmHg pressure at 40 °C. Transferrin was attached to the QCM biosensor surface through coated PHEMA nanoparticles by using carbodiimide. For attachment, 50 mg carbodiimide and 5 mg transferrin were dissolved in 10 mL of 0.1 M phosphate buffer (pH: 7.4). The QCM chip was dipped into this solution. Then the solution was stirred in an incubator at 100 rpm for 24 h. The chip was then washed with 0.5 M sodium chloride and phosphate buffer.
2.4. Characterization of QCM sensor chips
The nanoparticles and transferrin coated QCM surface were analyzed by FTIR-ATR, ellipsometry, contact angle measurements and atomic force microscopy.
For FTIR-ATR analysis, the QCM sensor was put into the sample holder of the FTIR-ATR spectrophotometer (Thermo Fisher Scientific, Nicolet iS10, Waltham, MA, USA) and total light reflection from the surface was measured in the wavenumber range of 400–4000 cm−1 at 2 cm−1 resolution.
The contact angle of the surface was determined with a KRUSS DSA100 (Hamburg, Germany) instrument. Measurements were made by the sessile drop method. Ten photos were obtained from different areas of QCM chips. The calculated values for the QCM surfaces were the average of 10 measurements.
Ellipsometry measurements were performed by using an auto-nulling imaging ellipsometer (Nanofil EP3, Germany). All the measurements have been carried out at a wavelength of 532 nm with an angle of incidence of 62°. In the layer thickness analysis, a four-zone auto-nulling procedure integrating over a sample area of approximately 50 μm × 50 μm followed by a fitting algorithm has been performed. The measurements were carried out at six different points of the QCM sensor surface and the results were given as the average value.
AFM observations were carried out using an AFM (Nanomagnetics Instruments, Oxford, UK) in tapping mode. The QCM chip was installed on a sample holder. Applied experimental parameters were oscillation frequency (341.30 Hz), vibration amplitude (1 VRMS) and free vibration amplitude (2 VRMS). A 5 μm × 5 μm sample area was observed with a 128 × 128 pixel resolution.
The surface area of the PHEMA nanoparticles was calculated by using the following equation (eqn (1)):
|  | (1) |
Here
N is the number of nanoparticles per milliliter;
S is the % of solids;
ρ is the density of the bulk polymer (g mL
−1); and
d is the nanoparticle diameter (nm). The number of nanoparticles in mL suspension was determined from the mass–volume graph of nanoparticles. The specific surface area of the PHEMA nanoparticles was calculated by multiplying
N and the surface area of 1 nanoparticle.
2.5. Cell binding studies
MDA MB 231 cell samples were prepared at seven different cell densities ranging between 500 and 125
000 cells per mL for determining the kinetics of binding. First, the QCM surface was washed with deionized water. Then, the transferrin functionalized QCM sensor was equilibrated with PBS and the resonance frequency (f0) was determined. The QCM sensor was then washed with PBS for 3 min. Five milliliters of each cell sample were applied to the sensor at a speed of 1.0 mL min−1. The shift in the resonance frequency was monitored and when equilibrium was reached in around 20 minutes; 1.0 M NaCl in PBS buffer was circulated for desorption. Before applying each sample, washing and equilibration were performed. For cell binding studies, a QCM system (RQCM, INFICON Acquires Maxtek Inc., New York, USA) was used. Data were evaluated with a RQCM (Maxtek) software system. In this system, the Sauerbrey equation25 is used to convert the frequency to mass by using geometrical and physical characteristics of the quartz crystal. The linear relationship between the changes in the resonance frequency of a quartz crystal and the mass of a thin rigid film added to its surface is shown in eqn (2). | 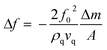 | (2) |
where f0 is the resonance frequency, A is the active electrode area, ρq is the density (2.65 g cm−3), Δm is the shear modulus and vq (3.34 × 105 cm s−1) is the shear wave velocity of the quartz crystal.
For the competitive binding analysis of high and low metastatic capacity and inactivated cells, they were applied to the QCM sensor with a density of 75
000 cells per mL in PBS. Furthermore, to test their affinity for transferrin, the same density of cells was applied to QCM sensors with surfaces coated with transferrin and with only PHEMA.
3. Results and discussion
3.1. Preparation and characterization of QCM sensors
The size distribution of the PHEMA nanoparticles, prepared by using EGDMA as a crosslinker, was measured with a zeta-sizer. As shown in Fig. 1, the average size of nanoparticles was detected to be 58 nm and the polydispersity was 0.229. The percentage of nanoparticles at the average size was 44%. This low nanometric scale is thought to be suitable for increasing the sensitivity of the QCM chip to be used as a sensor. Nanoparticles and nanorods which have special properties, such as specific area, fine particle size, and quantum confinement, are believed to be good candidates for biosensing or chemical sensing with high sensitivity.19,20 Nanoparticles can produce larger specific surface areas and therefore may result in high protein adsorption, which are used as ligands in cell adsorption studies. Therefore, it may be useful to synthesize nanoparticles with large surface areas and use them as suitable carriers for the adsorption of human breast cancer cells. The sensitivity of the signal response is proportional to the surface-area-to-volume ratio.26 58 nm-sized spheres were used because they have the largest surface-area-to-volume ratio while still having visible peak absorption. Poly(hydroxyethyl methacrylate) (PHEMA) nanoparticles with an average size of 58 nm in diameter were produced by the mini-emulsion polymerization method in which SDS was used as a surfactant. The specific surface area of the PHEMA nanoparticles was found to be 1899 m2 g−1. These particles were attached to the gold surface of the QCM chip and the surface was monitored by FTIR-ATR. The FTIR-ATR spectrum demonstrates the presence of nanoparticles on the chip surface. The band at 1730 cm−1 belongs to carbonyl of the ester group of HEMA while the wide band at 3000–3700 cm−1 belongs to the hydroxyl group of HEMA. Furthermore, the C
C double bonds belonging to the HEMA monomers which give a band at 1633 cm−1 were not detected in the PHEMA FTIR-ATR spectrum showing that polymerization was completed (Fig. S1A†).
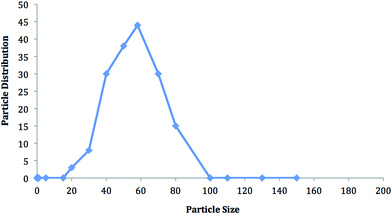 |
| Fig. 1 The size and size distribution of PHEMA nanoparticles. | |
Following the attachment of transferrin by carbodiimide the surface was analyzed by FTIR-ATR and the spectrum obtained is shown in Fig. S1B.† The band at 1107 cm−1 belongs to the C–O–C bond stretching, which is formed as a result of transferrin binding to PHEMA. The –C
O bond stretching band of HEMA at 1730 cm−1 has shifted to 1654 cm−1 as a result of transferrin binding. The intensity of the –OH band of HEMA at 3000–3700 cm−1 has increased in parallel to joining more –OH and –NH groups from transferrin. The amide I and II bands, which belong to the transferrin molecule, were found at 1640 cm−1 and 1577 cm−1, respectively. The width of these bands clearly demonstrates the presence of transferrin, which houses a great number of them. Fig. 2 shows the schematic illustration of transferrin modification of PHEMA nanoparticles by carbodiimide activation and QCM measurements.
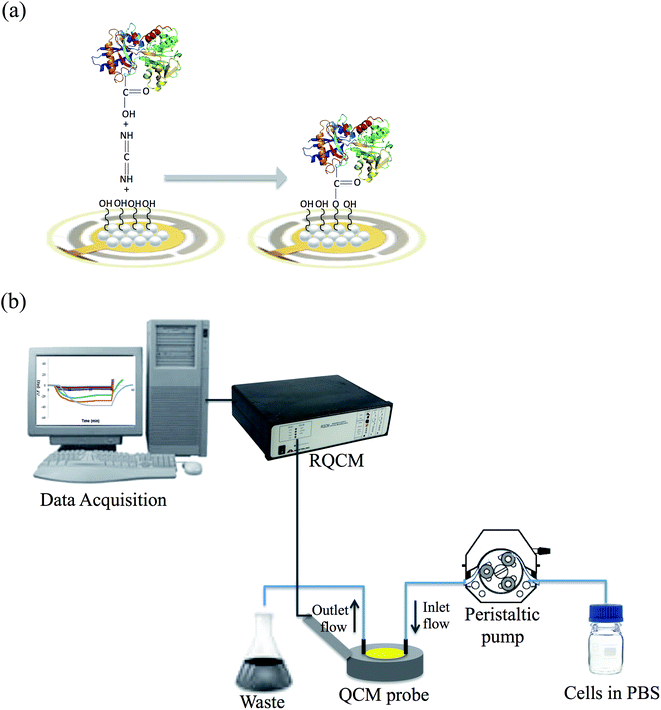 |
| Fig. 2 Schematic illustration of (a) transferrin modification of the PHEMA nanoparticle attached QCM sensor (b) QCM measurements. | |
The hydrophobicity of the surface was analyzed by contact angle measurements. As shown in Table 1, uncoated, nanoparticle coated, nanoparticle and transferrin coated surface contact angles were 84.0°, 78.3° and 73.6°, respectively. The decrease in contact angle shows that by coating with PHEMA nanoparticles and transferrin, the surface becomes more hydrophilic. This can be explained by the polar nature of PHEMA due to its –OH groups and this was increased by the addition of transferrin with charged amino acids.
Table 1 Contact angle values of QCM surfaces
Surface |
Contact angle (°) |
Uncoated surface |
84.0 ± 1.2 |
PHEMA nanoparticle coated surface |
78.3 ± 2.5 |
PHEMA and transferrin coated surface |
73.6 ± 3.2 |
The PHEMA nanoparticle attached QCM chip surface was further analyzed by using an ellipsometer to measure the mean surface layer depth (Fig. S2†). The QCM sensor surface with and without transferrin was analyzed. The depth of the surface with transferrin was 64.2 ± 3.1 nm and without transferrin it was 59.1 ± 2.04 nm. These measurements were in agreement with the data obtained by using a zeta-sizer. The QCM chip surfaces were also analyzed by AFM (Fig. 3). As shown in Table 2 the surface thickness was again in accordance with the previous data. These results show that the QCM surface was homogenously coated as a monolayer with PHEMA nanoparticles and transferrin.
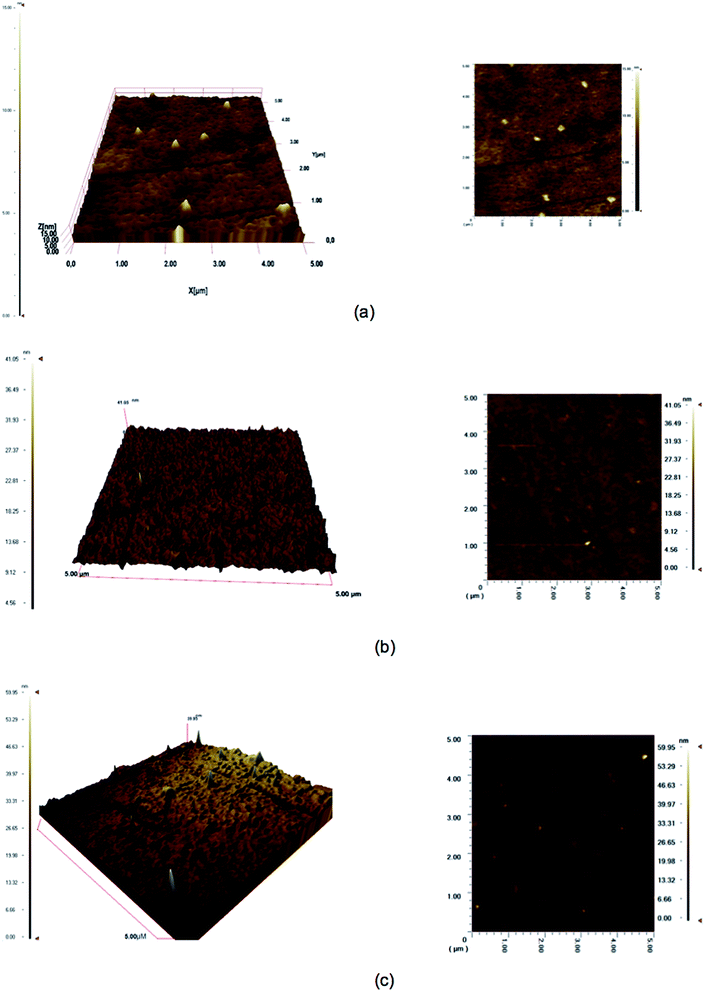 |
| Fig. 3 3D and surface AFM images of uncoated (a), PHEMA nanoparticle (b) transferrin coated (c) QCM surfaces. | |
Table 2 AFM characterization data of the QCM chip
Surface |
Thickness (nm) |
Uncoated surface |
10.0 |
PHEMA nanoparticle coated surface |
41.1 |
Transferrin coated surface |
59.9 |
3.2. Real time monitoring of the QCM biosensor response
In order to detect the highly metastatic MDA-MB-231 human breast cancer cells, they were applied to the QCM biosensor, which is functionalized with transferrin. To evaluate the sensitivity of the system, cell suspensions containing 500–125
000 cells per mL were used and the correlation between the QCM signal and the cell number was determined. The detection limit was found to be 500 cells per mL (S/N = 3). The sensorgram obtained by these measurements is given in Fig. 4a. As may be depicted by the figure, the real time change in the QCM response was parallel to the increase in cell number. In other words, Δf was correlated with the number of cells or rather their transferrin receptors. Fig. 4b shows the relationship between the mass shift and the number of cells. As demonstrated in the figure, the QCM sensor displays linearity for a wide range of concentrations from 500–125
000 cells per mL. The results show that the developed system may be used in detecting the MDA-MB-231 cells quantitatively. One of the critical parameters of biosensors is the limit of detection (LOD). Only a few references have reported the detection of cancer cells via the QCM. The designed biosensors have different limits of detection values such as in Ma's report in which a QCM biosensor for detection of human lung carcinoma cells was designed (LOD = 100 cells per mL).27 Zhang has designed a chitosan-based QCM biosensor for detection of breast cancer cells (LOD = 430 cells per mL),28 Shan et al. have developed an aptamer based QCM biosensor for the detection of leukemia cells (LOD = 1160 cells per mL).29 A label-free and highly sensitive sensing technology for tumor cell recognition and detection was developed based on a novel 2 × 3 model of leaky surface acoustic wave (LSAW) aptasensor array by Chang et al.30 The detection limit as low as 32 cells per mL was achieved for MCF-7 cells. The LSAW aptasensor also exhibited excellent specificity and stability. The system included a mercapto modified aptamer sequence and random sequence. The mercapto modified aptamer was firstly immobilized on the detection sensor, and the random sequence was immobilized on the reference sensor. So manipulation procedures for preparing aptamer-based sensors are relatively time-consuming. Synthetic polymers designed for the cell studies must comply with many requirements, the most important of them being a very good biocompatibility with recipient's tissue, mechanical stability, resistance to degradation and hydrolysis.31 PHEMA nanoparticles with excellent biocompatibility properties are suitable for clinical applications and were chosen as a matrix for this study. Our biosensor showed a wide linear range and an acceptably low limit of detection (500 cells per mL). Compared with the other methods based on the QCM, the proposed QCM cytosensor has a relatively wider linear range and lower detection limit.32,33
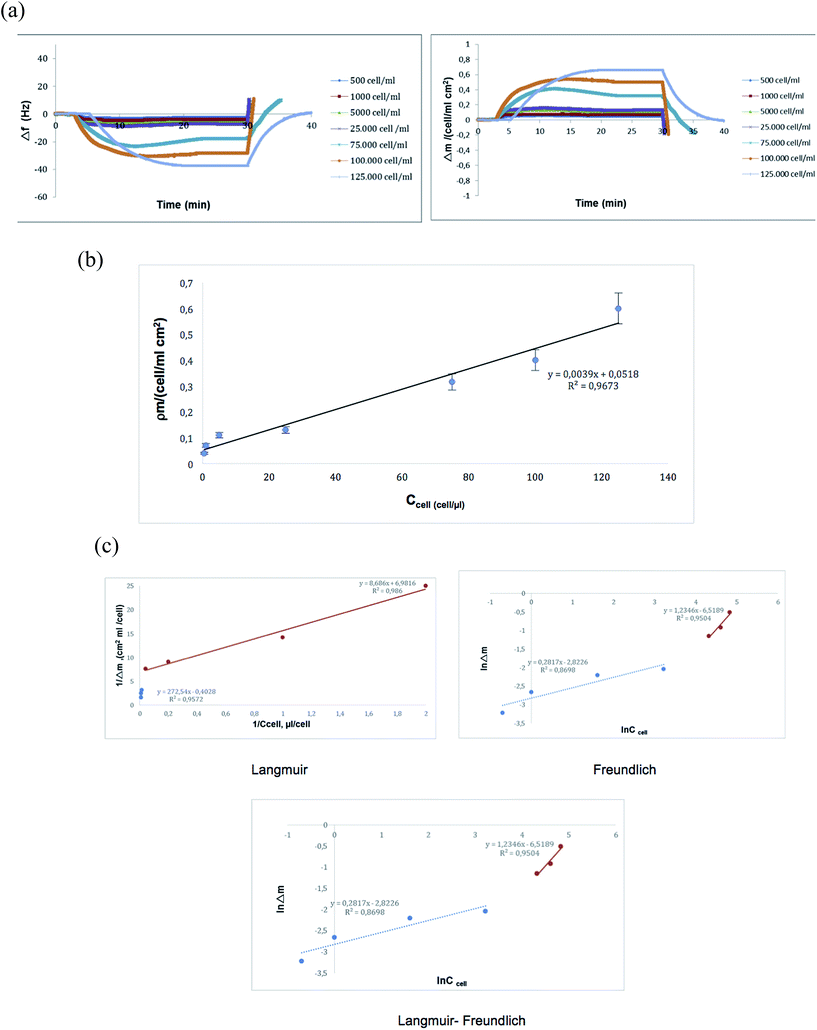 |
| Fig. 4 Kinetic (a and b) and isotherm (c) studies with the QCM sensor. | |
To determine the kinetic and equilibrium isotherm parameters of the QCM biosensor, the binding data were analyzed using a pseudo-first-order kinetic analysis and four different equilibrium isotherm models (Fig. 4c).34
| Equilibrium kinetic analysis dΔm/dt = kaCΔmmax − (kaC + kd)Δm | (3) |
| Scatchard Δmex/C = KAΔmmax − KAΔmeq | (4) |
| Langmuir Δm = {Δmmax[C]/KD + [C]} | (5) |
| Freundlich Δm = Δmmax[C]1/n | (6) |
| Langmuir–Freundlich Δm = {Δmmax[C]1/n/KD + [C]1/n} | (7) |
where Δ
m defines the amount of increased mass on the unit area of a QCM biosensor (number of cells per cm
2);
C is the cell concentration (number of cells per μL); 1/
n is the Freundlich exponent;
ka (number of cells per μL) and
kd (s
−1) are forward and reverse kinetic rate constants;
KA (number of cells per μL) and
KD (μL per number of cell) are forward and reverse equilibrium constants; and subscripts ex, max, and eq indicate experimental, maximum and equilibrium, respectively. When applying the equilibrium kinetic analysis, we have calculated slopes of curves and plotted concentration
vs. slope curve to determine
ka and
kd, respectively.
35
The adsorption models can be used to define recognition ability, interaction selectivity and surface homogeneity of the biosensors. For testing the experimental data Langmuir, Freundlich and Langmuir–Freundlich isotherm models were evaluated (Table 3). Langmuir–Freundlich was the best-fitted isotherm model among the applied models with a high regression coefficient (R2: 0.99). The Langmuir model is generally used to explain monolayered binding isotherms on a homogeneous surface and the binding sites have the same adsorption affinity, which is energetically equal without any interaction between the adsorbed molecules.36 The Freundlich model is used to show multilayer binding of analyte molecules. The Langmuir–Freundlich model can be applied to a system that is not fitted to both systems, and provides heterogeneity information of adsorption behavior over wide concentration regions. These results show that the binding of cells onto the QCM sensor is heterogeneous due to the complexity of interactions between the cell–cell and nanoparticles–cell.
Table 3 Calculated values of the isotherm models
Langmuir |
Freundlich |
Langmuir–Freundlich |
Δmmax |
0.14 |
Δmmax |
1.4 |
Δmmax |
0.12 |
K
A, cells per μL |
0.8 |
1/n |
1.23 |
1/n |
0.1272 |
K
D, μL per cell |
1.21 |
R
2
|
0.95 |
K
A, cells per μL |
1.4 |
R
2
|
0.986 |
|
|
K
D, 1 μL per cell |
0.7 |
|
|
|
|
R
2
|
0.99 |
3.3. Selectivity of the QCM biosensor
In order to show the selectivity of the transferrin functionalized PHEMA nanoparticle attached QCM biosensor, competitive adsorption of MDA-MB-231, MCF-7 and starved MDA-MB-231 human breast cancer cell lines was investigated (75
000 cells per mL). As seen in Fig. 5, the transferrin functionalized QCM biosensor was more selective for MDA-MB 231 cells as compared to MCF-7 cells and starved MDA-MD-231 cells. Selectivity coefficient (k) and relative selectivity coefficients (k′) values are given in Table 4. The transferrin functionalized QCM biosensor was 1.85 and 6.32 times more selective for highly metastatic MDA-MB-231 than MCF-7 and starved MDA-MB-231 cells, respectively. These results indicate that the transferrin functionalized QCM biosensor has higher adsorption affinity for highly metastatic MDA-MB-231, due to higher transferrin receptor expression. To show the specificity of the biosensor, the PHEMA nanoparticle attached QCM biosensor (without transferrin) was used and the responses of this non-functionalized QCM system to MDA-MB-231, MCF-7 and starved MDA-MB-231 cell lines were determined to be 0.030, 0.033 and 0.013, respectively. These results demonstrated that the transferrin had successfully bound to the chip surface and the interaction of cells occurs through the transferrin molecules.
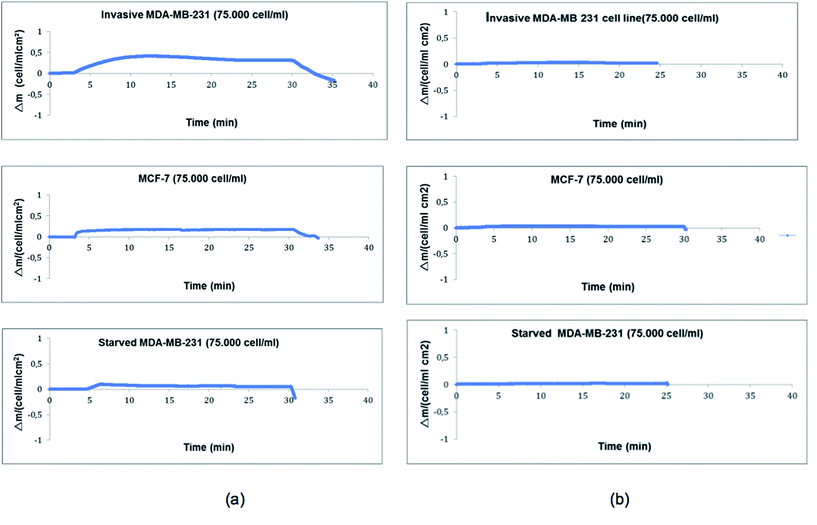 |
| Fig. 5 Selectivity (a) and specificity (b) of the QCM sensor. | |
Table 4 Selectivity coefficient (k) and relative selectivity coefficient (k′) values
Cell sample |
PHEMA with transferrin |
PHEMA without transferrin |
k′ |
Δm |
k
|
Δm |
k
|
MDA-MB-231 |
0.316 |
— |
0.03 |
— |
— |
MCF-7 |
0.17 |
1.85 |
0.033 |
0.92 |
2.01 |
Starved MDA-MB-231 |
0.05 |
6.32 |
0.013 |
2.3 |
2.74 |
4. Conclusion
A QCM sensor was developed to detect human breast cancer cells with high metastatic power. PHEMA nanoparticles were attached to the sensor surface to increase the surface area followed by transferrin attachment to functionalize the QCM surface. The analysis of nanoparticles and the functionalized QCM sensor has revealed that an efficient sensing platform is formed. This QCM sensor has shown good sensitivity and selectivity for the real time detection of metastatic breast cancer cells via their over expressed transferrin receptors. The results revealed that the QCM sensor had an excellent capability in discriminating MCF-7 breast cancer cells from other two kinds of cells. Binding data were best fit to the Langmuir–Freundlich adsorption model. The system offers label-free detection, low volume sample application, real-time monitoring, quantitative measurements and kinetic evaluation. Manipulation procedures for preparing QCM sensors are relatively not time-consuming and require labeled molecules. This transferrin receptor targeting QCM biosensor may also enable the fabrication of a low cost and rapid detection system, which can be used for the screening of metastatic breast cancer cells in serum or biopsy samples.
References
- R. Siegel, J. Ma, Z. Zou and A. Jemal, Cancer statistics, Ca-Cancer J. Clin., 2014, 64, 9–29 CrossRef PubMed.
- E. Senkus, F. Cardosob and O. Pagani, Cancer Treat. Rev., 2014, 40(2), 220–228 CrossRef PubMed.
- B. Hong and Y. Zu, Theranostics, 2013, 3(6), 377–394 CrossRef CAS PubMed.
- T. J. Ladd, Q. M. Yu, S. F. Chen, J. Homola and S. Y. Jiang, Biosens. Bioelectron., 2006, 22, 752–758 CrossRef PubMed.
- A. A. Yanik, M. Huang, O. Kamohara, A. Artar, T. W. Geisbert, J. H. Connor and H. Altug, Nano Lett., 2010, 10, 4962–4969 CrossRef CAS PubMed.
- J. Parellada, A. Narvaez, M. A. Lopez, E. Domingue, J. J. Fernandez and V. Pavlov, Anal. Chim. Acta, 1998, 362, 47–57 CrossRef CAS.
- A. D. Dias, D. M. Kingsley and D. T. Corr, Biosensors, 2014, 4, 111–136 CrossRef CAS PubMed.
- M. Rai, A. Gade, S. Gaikwad, P. D. Marcato, N. Duran and J. Braz, J. Chem. Soc., 2012, 23, 14–24 CAS.
- Y. E. Choi, J. W. Kwak and J. W. Park, Sensors, 2010, 10, 28–45 Search PubMed.
- J. Kirsch, C. Siltanen, Q. Zhou, A. r Revzin and A. Simonian, Chem. Soc. Rev., 2013, 42, 8733–8876 RSC.
- W. Shan, Y. Pan, H. Fang, M. Guo, Z. Nie, Y. Huang and S. Yao, Talanta, 2014, 126, 130–135 CrossRef CAS PubMed.
- R. Hao, D. Wang, X. E. Zhang, G. Zuo, H. Wei, R. Yang, Z. Zhang, Z. Cheng, Y. Guo and Z. Cui, Biosens. Bioelectron., 2009, 24, 1330–1335 CrossRef CAS PubMed.
- M. Minunni, S. Tombelli, A. Gullotto, E. Luzi and M. Mascini, Biosens. Bioelectron., 2004, 20, 1149–1156 CrossRef CAS PubMed.
- X. Wang, H. Y. D. Lu, J. Zhang and W. Deng, Sens. Actuators, B, 2014, 195, 630–634 CrossRef CAS.
- A. Thomas, H. Hamilton, G. Wade and H. H. Sussman, Proc. Natl. Acad. Sci. U. S. A., 2013, 3(6), 377–394 Search PubMed.
- N. Bien-Ly, Y. J. Yu, D. Bumbaca, J. Elstrott, C. A. Boswell, Y. Zhang, W. Luk, Y. Lu, M. S. Dennis, R. M. Weimer, I. Chung and R. J. Watts, J. Exp. Med., 2014, 211(2), 233–244 CrossRef CAS PubMed.
- J. Wang and K. Pantopoulos, Biochem. J., 2011, 434, 365–381 CrossRef CAS PubMed.
- D. Trinder, O. Zak and P. Aisen, Hepatology, 1996, 23, 1512–1520 CrossRef CAS PubMed.
- O. Marques, B. Martins da Silva, G. Porto and C. Lopes, Cancer Lett., 2014, 347, 1–14 CrossRef CAS PubMed.
- J. Zhang, Y. Sun, B. Xu, H. Zhang, Y. Gao, H. Zhang and D. Song, Biosens. Bioelectron., 2013, 45, 230–236 CrossRef CAS PubMed.
- A. K. M. Kafi, A. Ahmedalinezdad, J. P. Wang, D. F. Thomas and A. C. Chen, Biosens. Bioelectron., 2010, 25, 2458 CrossRef CAS PubMed.
- R. L. Bunde, E. J. Jarvi and J. J. Rosentreter, Talanta, 1998, 46, 1223–1236 CrossRef CAS PubMed.
-
Piezoelectric Sensors, Series on Chemical Sensors and Biosensors, ed. C. Steinem and A. Janshoff, Springer, 2007 Search PubMed.
- C. J. Tan, S. Wangrangsimakul, R. Bai and Y. W. Tong, Chem. Mater., 2008, 20, 118–127 CrossRef CAS.
- G. Sauerbrey, Z. Phys., 1959, 155(2), 206–222, DOI:10.1007/BF01337937.
- T. Morris, K. Kloepper, S. Wilson and G. Szulczewski, J. Colloid Interface Sci., 2002, 254, 49–55 CrossRef CAS PubMed.
- Z. Ma, J. Wu, T. Zhou, Z. Chen, Y. Dong, J. Tang and S.-F. Sui, New J. Chem., 2002, 26, 1795–1798 RSC.
- S. Zhang, H. Bai, J. Luo, P. Yang and J. Cai, Analyst, 2014, 139, 6259–6265 RSC.
- W. Shan, Y. Pan, H. Fang, M. Guo, Z. Nie, Y. Huang and S. Yao, Talanta, 2014, 126, 130–135 CrossRef CAS PubMed.
- K. Chang, Y. Pi, W. Lu, F. Wang, F. Pan, F. Li, S. Jia, J. Shi, S. Deng and M. Chen, Biosens. Bioelectron., 2014, 60, 318–324 CrossRef CAS PubMed.
- J. Mokry, J. Karbanova, J. Lukas, V. Paleckova and B. Dvorankova, Biotechnol. Prog., 2000, 16, 897–904 CrossRef CAS PubMed.
- A. Wisitsoraat, P. Sritongkham, C. Karuwan, D. Phokharatkul, T. Maturos and A. Tuantranont, Biosens. Bioelectron., 2010, 26, 1514–1520 CrossRef CAS PubMed.
- A. Liu, Biosens. Bioelectron., 2008, 24, 167–177 CrossRef CAS PubMed.
- L. P. Lin, L. S. Huang, C. W. Lin, C. K. Lee, J. L. Chen, S. M. Hsu and S. Lin, Curr. Drug Targets, 2005, 5, 61–72 CAS.
- G. Sener, E. Ozgür, E. Yilmaz, L. Uzun, R. Say and A. Denizli, Biosens. Bioelectron., 2010, 26, 815–821 CrossRef CAS PubMed.
- S. K. Papageorgiou, F. K. Katsaros, E. P. Kouvelos and N. K. Kanellopoulos, J. Hazard. Mater., 2009, 162, 1347–1354 CrossRef CAS PubMed.
Footnote |
† Electronic supplementary information (ESI) available. See DOI: 10.1039/c5ay02898a |
|
This journal is © The Royal Society of Chemistry 2016 |
Click here to see how this site uses Cookies. View our privacy policy here.