Resilience of microbial communities in a simulated drinking water distribution system subjected to disturbances: role of conditionally rare taxa and potential implications for antibiotic-resistant bacteria†
Received
18th February 2016
, Accepted 9th May 2016
First published on 10th May 2016
Abstract
Many US water utilities using chloramine as their secondary disinfectant have experienced nitrification episodes that detrimentally impact water quality in their distribution systems. A semi-closed pipe-loop chloraminated drinking water distribution system (DWDS) simulator was used to evaluate the biological stability of the system and describe the response of microbial communities in the bulk water (BW) and biofilm (BF) phase to a disturbance caused by changes in the operational parameters. The DWDS simulator was operated through five successive operational schemes, including an episode of nitrification, followed by a ‘chlorine burn’ by switching the disinfectant from chloramine to free chlorine. Community comparisons showed significant differences in the structure based on disinfectant and phase (e.g., BW and BF). Both disturbances created changes in the relative abundances of the core microbiome and some members of the rare biosphere (i.e., conditionally rare taxa); however, the microbial community was resilient and returned to its stable state. Genes associated with multiple antibiotic resistance mechanisms were found to be a component of the core genomes of waterborne isolates. These results provide evidence of variations in the bulk water/biofilm microbial community structure during episodes of disturbance (e.g., disinfectant switching practices, nitrification) and its recovery after disturbance.
Water impact
This research provides evidence of the recovery (resilience) of the microbial community after episodes of disturbance (nitrification, disinfectant switching practices). Understanding changes in microbial communities in disturbed drinking water distribution systems is essential for monitoring biological stability and for proper risk management to ensure public and ecosystem health. Furthermore, it is essential to incorporate the resistome when monitoring these systems.
|
Introduction
Many US water treatment facilities use chloramination to limit disinfection by-product formation. However, chloramination has been shown to promote nitrifying bacteria, and more than half of these water utilities using chloramine as a secondary disinfectant experience nitrification episodes.1 Nitrification in drinking water distribution systems (DWDS) can impact water quality (e.g., taste) and is the consequence of changes in ammonia concentration, monochloramine concentration, temperature (above 15 °C), dissolved oxygen, pH, and organic carbon level.1 In addition, biofilms can harbor nitrifying bacteria (e.g., Ammonia-Oxidizing Bacteria [AOB] and Nitrite-Oxidizing Bacteria [NOB]) by reducing disinfectant efficiency, resulting in an increased likelihood of nitrification occurrence.2 Adequate chloramine residual levels appear to prevent nitrification in DWDS; however, once nitrification occurs, chloramine is rapidly degraded. The current approach to stop and control nitrification is to temporarily switch the disinfectant from chloramine to free chlorine (i.e., a ‘chlorine burn’).3
Nitrification and chlorine burns in a DWDS are considered disturbances to microbial communities, because they alter the immediate environment by changing the physico-chemical conditions of the system.4 In general, the outcome of a disturbance may be: (i) complete removal of the microbial community or (ii) changes in their relative abundances.5 Alternatively, only the functional potential (e.g., metabolic processes) but not the composition of the community changes after the disturbance.6 The community's response is mediated by stability, which consists of resistance and resilience.7 Resistance is the degree to which a community is sensitive to a disturbance, and resilience is the rate of recovery after disturbance.8 The consequences of disturbances in DWDS are the emergence of microbial agents associated with waterborne diseases,9 changes in ecosystem processes (i.e., microbial functional capabilities),6 and the occurrence and dissemination of antibiotic resistance genes (ARGs).10
Antibiotic resistance is a rising problem which threatens healthcare globally.11,12 Evidence suggests that ARGs are ubiquitous in natural environments,10 and their presence has been detected in a broad range of water systems, such as reclaimed water for irrigation,13 drinking water treatment plants and distribution systems,14,15 and wastewater treatment plants.16 The ARG source is likely to be the recurring discharge of antibiotics and resistance genes (e.g., from wastewater or run-off from livestock facilities and agriculture).11 The rapid evolution of bacterial resistance is clear and just recently non-clinical environmental isolates have been highlighted as an important factor in the dissemination of ARGs.11 As a result, effective monitoring of microbial contaminants (and their genomes) in DWDS is critical to reduce health risks, particularly for those populations with immuno-compromised systems such as children and the elderly.17
It is generally accepted that changes in environmental conditions (i.e., disturbance) can potentially impact the microbial composition and thereby affect ecosystem processes.8 Similarly, DWDS microbial communities are sensitive to changes in operational parameters (i.e., nitrification, disinfectant residual) and may respond to a disturbance by: maintaining the same composition (i.e., resistance), returning to their stable state after a shift in community composition (i.e., resilience), or alternatively, altering community performing process rates similar to those of the original community.7,8 The current research examined the bacterial populations in a chloraminated DWDS simulator. The research used next generation high-throughput technology and culture-dependent assays to (1) determine the extent to which the community structure varies temporally and spatially within the distribution system, (2) describe the response of microbial communities to a disturbance caused by changes in the operational parameters of the distribution system, and (3) characterize and compare waterborne isolate genomes carrying ARGs. Understanding the behavior of microbial communities in disturbed DWDS environments is essential for risk management18 and evaluation of the biological stability (i.e., maintaining microbial water quality) of the systems.19 It also provides a window into the bacterial genome structure and the occurrence of ARGs in waterborne isolates. Furthermore, this information could be incorporated into ecosystem process models and greatly enhance our ability to predict ecosystem responses to disturbances.8
Materials and methods
Drinking water distribution system (DWDS) simulator
A semi-closed pipe-loop DWDS simulator (Fig. S1†) was operated through five successive operational schemes (Table 1). Pipe-loop feed water was generated from a free chlorine municipal drinking water (DW) source in 5000 gallon batches approximately every two weeks (Fig. S1a†). Water in the pipe loop was re-circulated with a 20.4 (SD = 1.9) hour hydraulic residence time (HRT) and the temperature in the loop was controlled (Fig. S1b†). To generate and study attached communities, copper and PVC coupons were incubated in off-line CDC biofilm reactor devices (Biosurface Tech. Corp., Montana, USA) fed with water from the pipe loop and operated with a 20.8 (SD = 1.8) hour HRT (Fig. S1c†). Additional system properties and water quality characteristics are presented in Table S1.†
Table 1 Operational scheme summary
Operational scheme |
Operational time (days) |
Disinfectant |
Disinfectant concentrationa (mg Cl2 L−1) |
Pipe-loop temp.a (°C) |
Target operating level.
|
Pre-modified (SP + SS) |
345 |
Chloramines |
Ambient (1.6 to 2.3) |
18 |
Standard I (SI) |
112 |
Chloramines |
3.0 |
18 |
Failure (SF) |
155 |
Chloramines |
3.0 |
24 |
Restore (SR) |
77 |
Free chlorine |
3.0 |
24 |
Standard II (SII) |
220 |
Chloramines |
3.0 |
18 |
The initial operating scheme (Pre-Modified) established a baseline of operation based on only adding free ammonia to the free chlorine in the DW source, resulting in varied chloramine concentrations entering the pipe loop because of the variations in the concentration of free chlorine in the DW source. Also, Pre-Modified involved two periods corresponding to whether biological samples were taken (SP) or not (SS). In subsequent operating schemes, the DW source was amended as necessary with ammonium sulfate and sodium hypochlorite to generate a target monochloramine (Standard I [SI], Failure [SF], and Standard II [SII]) or free chlorine (Restore [SR]) residual concentration of 3 mg L−1. For monochloramine formation, a 4.5
:
1 chlorine-to-ammonia nitrogen mass ratio (Cl2
:
N) was used. The operational scheme sequence was designed to parallel a practical scenario where a chloraminated drinking water system progresses from normal operation in which a chloramine residual is maintained in the pipe loop (Standard I) to a failure period where no chloramine residual is maintained as a result of nitrification (Failure). Subsequently, the drinking water system operation is modified by switching disinfectants from chloramine to free chlorine to eliminate nitrification and maintain a disinfectant residual (Restore). After a period of time, the drinking water utility then switches back to chloramine and resumes normal operation (Standard II). To accelerate the development of nitrification and disinfectant residual loss, the temperature of the pipe-loop water was increased (from 18 °C to 24 °C) for the Failure operational scheme (Table 1).
Water quality
Temperature, pH, turbidity, and ORP measurements were taken from online sensors. Nitrate and phosphate analyses were performed with a discrete colorimetric SmartChem 200 autoanalyzer (Westco Scientific, Danbury, CT) using EPA Methods 353.2 (ref. 20) and 365.1.21 Chemical concentrations for free chlorine (HACH Method 8021), monochloramine and free ammonia (HACH Method 10200), total chlorine (HACH Method 8167), and nitrite (HACH Method 8507) were determined with a HACH DR/2400 portable spectrophotometer (HACH, Loveland, CO, USA). Bulk water concentrations were analysed from a composite of two samples taken from two different locations in the pipe loop (Ports A and B).
Sample collection, DNA extraction and sequencing
Bulk water (BW; n = 114) and biofilm (BF; n = 99) samples were collected from 26 sample times over 29 months (Fig. 1). Duplicate BW samples (3 L) were collected from a faucet receiving free chlorine-treated municipal drinking water (BW-C) source and two locations in the pipe-loop DWDS simulator (BW-L) using sterile polypropylene bottles (Nalgene, Rochester, NY). The BW samples were individually filtered on-site through polycarbonate membranes (47 mm diameter, 0.22 μm pore size). Three PVC (BF-P) and copper (BF-U) coupons were aseptically removed from the CDC reactors at each sample time point. Membranes and coupons were individually overlaid with 100 μmol L−1 Propidium Monoazide (PMA) and incubated in the dark for 20 min, followed by 15 min exposure to 460 nm light.22 PMA is a photo-reactive dye with a high affinity for DNA. The dye intercalates into dsDNA and forms a covalent linkage upon exposure to intense visible light, resulting in chemically modified DNA. PMA can inhibit PCR amplification of DNA from membrane-compromised cells and effectively discriminates between live and dead bacteria.23 The coupons were individually placed in a 15 mL tube containing 4 mL of ddH2O. The membranes and coupons were stored and transported on ice for DNA processing.
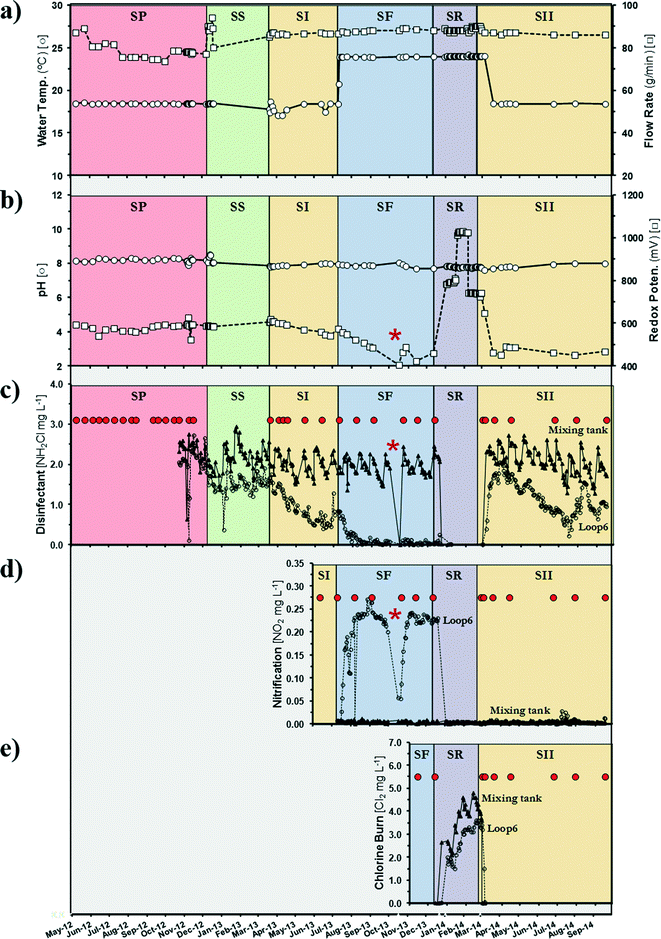 |
| Fig. 1 Operational details in a simulated distribution system: (a) water temperature and flow rate, (b) pH and redox potential, (c) chloramine [NH2Cl], (d) nitrite [NO2−], and (e) free chlorine [Cl2]. The study was organized into five distinct operational schemes: (1) PRE-MODIFIED (SP + SS), system stabilization; (2) STANDARD I (SI), stable chloramine residual; (3) FAILURE (SF), complete nitrification and minimal chloramine residual; (4) RESTORE (SR), chlorine burn; (5) STANDARD II (SII), stable chloramine residual. Red dots ( ) represent sampling dates. *US government shutdown (10/01/2013–10/16/2013). | |
To facilitate DNA extraction, the coupons were vortexed for 5 min at maximum speed, and the homogenate was removed and extracted using a MoBio PowerBiofilm® DNA Isolation Kit. Total DNA from BW was extracted using a MoBio PowerWater® DNA Isolation Kit. DNA extractions followed the manufacturer's instructions (MoBio Laboratories, Solana Beach, CA). DNA concentrations were measured using a Qubit® fluorometer (Life Technologies, Carlsbad, CA) and DNA samples stored at −80 °C.
PMA-treated total DNA served as a template for PCR amplification of the 16S rRNA genes. The V4 region of the 16S rRNA sequence was amplified using the bacterial primer set 515F and 806R.24 Paired-end 125 bp libraries were prepared using an Illumina MiSeq® Reagent v2 (500 cycles) kit on a MiSeq platform (Illumina Inc., San Diego, CA). Sequencing was performed at the CCHMC DNA Sequencing and Genotyping Core (Cincinnati, OH).
Determination of biomass
Biomass quantification is the most common and direct way to monitor bacterial growth; however, selecting the appropriate method is a contentious challenge.25 In this study, we utilized total ATP, DNA concentration, and protein content of bacterial cells as a surrogate for biomass present in both BW and BF samples. DNA concentration was measured as previously described (see Sample collection, DNA extraction and sequencing). The amount of ATP was determined by ATP-bioluminescence quantification using a Promega BacTiter-Glo™ Microbial Cell Viability Assay kit on a GloMax® 96 microplate luminometer (Promega, Madison, WI), following the protocol by Berney et al. (2008).26 Total protein was measured by using the bicinchoninic acid (BCA) method (Micro BCA™ Protein Assay Kit) with bovine serum albumin as a standard, according to the manufacturer's instructions (Thermo Fisher Scientific, Waltham, MA). All samples were analysed in triplicate.
16S rRNA sequence analysis
Reads processing was performed using the software MOTHUR v1.36.1 (ref. 27) (http://www.mothur.org) following the protocol of Kozich et al. (2013).28 Briefly, fastq files with forward and reverse reads were used to form contigs. Reads were screened and removed if they (i) had a length less than 255 bp, (ii) contained ambiguous bases (Ns), (iii) contained homopolymers greater than 7 bases, (iv) were identified as chimeras, or (v) were classified as unknown, chloroplasts, or mitochondria. Reads were aligned and grouped with 97% sequence identity as the cut-off point for each Operational Taxonomic Unit (OTU). Taxonomic classification was obtained using the Greengenes reference taxonomy database release gg_13_8_99. A total of 8
218
314 16S rRNA gene reads were analysed in this study. Prior to community analysis, samples were rarefied to the smallest dataset (1050 reads).
Species richness, diversity and community structure
Normalized libraries were used to calculate diversity indices (e.g., OTU richness, ChaoI and SACE richness estimators, Shannon diversity and evenness) according to methods described by Hill et al. (2003).29 Diversity indices were calculated with MOTHUR v1.36.1.27 Non-metric multidimensional scaling (nMDS) and cluster analysis (CA) based on the square root Jensen–Shannon divergence coefficient were used to describe the relationships among microbial communities. Plots were generated with the software PAST v3.08.30
Statistical analysis of community assemblages
Analysis of similarities (ANOSIM) was used to determine if there were significant differences (p < 0.05) between operational schemes and surface material used (PVC and copper coupons) on community assemblages (see Table 1 for factor groups). R values (R test statistic) near 0 indicate a true null hypothesis of no difference between groups, whereas those greater than 0 (up to 1) indicate dissimilarities between groups.31 Similarity Percentage (SIMPER) analysis was used to determine which species were most responsible for the differences observed between water samples.31 ANOSIM and SIMPER analyses were performed with the software PAST v3.08.30
Phylogenetic community assembly was compared using the net relatedness index (NRI), which is a standardized measure based on the mean pairwise phylogenetic distance among OTUs in each sample.32 The NRI was calculated using the PHYLOCOM v4.2 software (http://www.phylodiversity.net/phylocom) with abundance data, null model #2, and 999 permutations.
Bacterial strains and DNA extraction
Fifteen strains collected from the chloraminated DWDS simulator were selected for whole-genome analysis of antibiotic resistance genes (ARGs). The strains are representatives of the Mycobacterium immunogenum (n = 6), Mycobacterium chelonae (n = 4), Variovorax paradoxus (n = 2), and Sphingopyxis spp. (n = 3) species. In addition, these strains represent the dominant members of the DWDS simulator community (100% 16S rRNA sequence homology). Isolates were collected from three distinct operational schemes (Fig. 1): (i) Standard I, stable chloramine residual; (ii) Failure, complete nitrification and minimal chloramine residual; (iii) Standard II, stable chloramine residual after a ‘chlorine burn’. The strains were isolated by plating 100 μL of a BW or BF homogenate on R2A plates for 7 days at 27 °C. Genomic DNA was extracted using an UltraClean DNA Microbial Isolation kit (MoBio Laboratories, Solana Beach, CA).
Genome sequencing, assembly and annotation
Whole-genome sequencing (WGS) was performed as previously described by Gomez-Alvarez et al. (2016).33 Briefly, genome sequences were obtained by rapid mode sequencing on a HiSeq 2500 platform (Illumina Inc., San Diego, CA) using a paired-end 125 bp Nextera XT DNA library. Prior to assembly, libraries were (i) cleaned from contaminants (adapters, phiX, artifacts, and human), (ii) error-corrected via Tadpole, (iii) normalized to ≤100×, (iv) removed of low (<6×) coverage reads, and (v) filtered to a minimum length read of 125 nt. Reads were processed using the software package BBMap v35.34 (http://sourceforge.net/projects/bbmap) and de novo assembly with SPAdes v3.5.0.34 Genome assemblies were annotated with Prokka v1.10 (ref. 35) available as an application in Illumina BaseSpace Labs.
Genome comparison and antibiotic resistance gene (ARG) databases
Whole-genome sequence alignments for similarity were performed using the software MAUVE v20150226 build 10,36 while PRALINE37 was used for multiple amino acid alignment of selected ARGs. The presence of ARGs and antibiotic efflux pumps was searched against the database Resfams v1.2 (ref. 38) using HMMER v3.1b2 (ref. 39) and with the Resistance Gene Identifier (RGI) v2 based upon the data available in the CARD (Comprehensive Antibiotic Resistance Database).40
Nucleotide sequence accession numbers
Illumina Miseq 16S rRNA sequence data were deposited in the NCBI sequence read archive (NCBI-SRA) database under the accession number SRP069876. The Whole Genome Shotgun project for M. immunogenum (accession numbers: LJFO00000000, LJFQ00000000, LJFT00000000, LJFU00000000, LJFX00000000, LJFY00000000), M. chelonae (LJYL00000000, LJYM00000000, LJYP00000000, LJYT00000000), V. paradoxus (LKTU00000000, LKTW00000000), and Sphingopyxis spp. (LNRS00000000, LNRU00000000, LNRW00000000) has been deposited in DDBJ/ENA/GenBank.
Results and discussion
Bacterial richness and diversity
A total of 213 16S rRNA libraries from 26 sample times were constructed from feed water from a free chlorine municipal drinking water source (BW-C) and loop bulk water (BW-L) and the respective biofilm (BF) samples from a drinking water distribution system (DWDS) simulator. Sequence analysis generated 16
336 bacterial Operational Taxonomic Units (OTUs) at a ≥97% sequence identity cutoff. The study detected 3431 (66% of the OTUs and 1.3% of reads), 6278 (81% and 0.4%) and 4555 (74% and 1.4%) OTUs exclusively in the BW-C, BW-L, and BF samples, respectively. A total of 2072 OTUs (13% of the total OTU diversity) were shared by the samples, representing 98.6% to 99.6% of the reads in their respective samples. Complex microbial communities are extremely diverse and typically exhibit a distribution pattern of dominant (represented by few taxa) and a majority of low-abundance bacterial species (i.e., rare biosphere).41 It should be noted that in this study we applied propidium monoazide (PMA, see Materials and methods) on the BF and BW samples, effectively discriminating between live and dead bacteria (Nocker et al., 2010). The removal of extracellular DNA or dead cells from the original samples is useful for the identification of the viable and possible “active” fraction of the community.42 Richness estimator values (SACE and ChaoI; Table 2) and rarefaction analysis (data not shown) suggested that more exhaustive sampling would be necessary to obtain complete coverage of the bacterial composition in the DWDS. However, there is evidence that deeper sequencing would only improve the detection of rare species and would not have significantly improved the accuracy of the analysis.43 Indeed, when singletons (i.e., rare members) were excluded from the analysis, the rarefaction curves did plateau (data not shown), indicating that the dominant representatives were likely identified. In general, community analysis shows the great diversity of bacterial groups contained in DWDS systems (Table 2).
Table 2 Community diversity estimates (±SD) of the domain Bacteria
Source (schemes)a |
Samplesb (n) |
Sample times |
Biofilm maturity (days) |
S
|
Richness estimators |
Diversity index |
ChaoI |
S
ACE
|
Shannon (H) |
Evenness (EH) |
Source: BW-L = pipe-loop DWDS simulator water; BF-P = biofilm/PVC surface; BF-U = biofilm/copper surface; BW-C = chlorine-treated municipal drinking water. Operational schemes: SP + SS = system stabilization; SI = stable chloramine residual; SF = complete nitrification and minimal chloramine residual; SR = chlorine burn; SII = stable chloramine residual.
For comparison, the species frequencies were normalized to the smallest library (n = 1050).
Richness is the total number of OTUs in the community (i.e., the total number of independent reads within the sample; defined as aligned sequences with >97% similarity).
|
BW-L |
|
|
|
|
|
|
|
|
SP |
18 |
9 |
|
51 ± 7 |
83 ± 15 |
93 ± 21 |
2.23 ± 0.43 |
0.57 ± 0.10 |
SI |
20 |
5 |
|
53 ± 11 |
85 ± 18 |
97 ± 18 |
2.25 ± 0.34 |
0.57 ± 0.08 |
SF |
20 |
5 |
|
54 ± 6 |
96 ± 11 |
127 ± 22 |
2.34 ± 0.19 |
0.59 ± 0.04 |
SII |
20 |
5 |
|
62 ± 10 |
108 ± 17 |
138 ± 29 |
1.64 ± 0.46 |
0.40 ± 0.10 |
BF-P |
|
|
|
|
|
|
|
|
SI |
17 |
6 |
0–113 |
64 ± 12 |
85 ± 18 |
89 ± 20 |
2.83 ± 0.43 |
0.68 ± 0.09 |
SF |
12 |
5 |
114–268 |
82 ± 19 |
113 ± 28 |
121 ± 34 |
2.96 ± 0.36 |
0.68 ± 0.07 |
SII |
19 |
6 |
346–547 |
83 ± 44 |
113 ± 89 |
121 ± 119 |
3.16 ± 0.55 |
0.73 ± 0.08 |
BF-U |
|
|
|
|
|
|
|
|
SI |
17 |
6 |
0–113 |
55 ± 16 |
73 ± 17 |
80 ± 21 |
2.45 ± 0.84 |
0.61 ± 0.18 |
SF |
14 |
5 |
114–268 |
75 ± 18 |
102 ± 21 |
114 ± 29 |
2.68 ± 0.70 |
0.62 ± 0.14 |
SII |
20 |
6 |
346–547 |
80 ± 24 |
100 ± 29 |
100 ± 27 |
3.12 ± 0.58 |
0.72 ± 0.10 |
BW-C |
36 |
21 |
|
81 ± 49 |
159 ± 96 |
234 ± 137 |
2.22 ± 0.92 |
0.51 ± 0.16 |
Taxonomic classification revealed that the majority of the OTUs were associated with the class Alphaproteobacteria (44% of reads), Actinobacteria (19%), Gammaproteobacteria (12%), and Betaproteobacteria (9%) with additional representatives closely related to the class Nitrospira. In addition, Bacilli, Clostridia, Saprospirae, Cytophagia, and Planctomycetia were detected to a lesser extent (≤2%) (Fig. 2). The dominant OTUs (in the following order of decreasing abundance) were closely related to members of the species Mycobacterium, Reyranella, Magnetospirillum, Acinetobacter, Hyphomicrobium, Sphingomonas, Sphingopyxis, Methylobacterium, Acidovorax, Nitrospira, Rhizobium, Novosphingobium, Erythromicrobium, Bradyrhizobium and Caulobacter. These species are ubiquitous members of the DWDS25 with the majority associated with the Alphaproteobacteria class, with the exception of Mycobacterium (class Actinobacteria), Acidovorax (β-Proteobacteria), Nitrospira (Nitrospira), and Acinetobacter (γ-Proteobacteria).
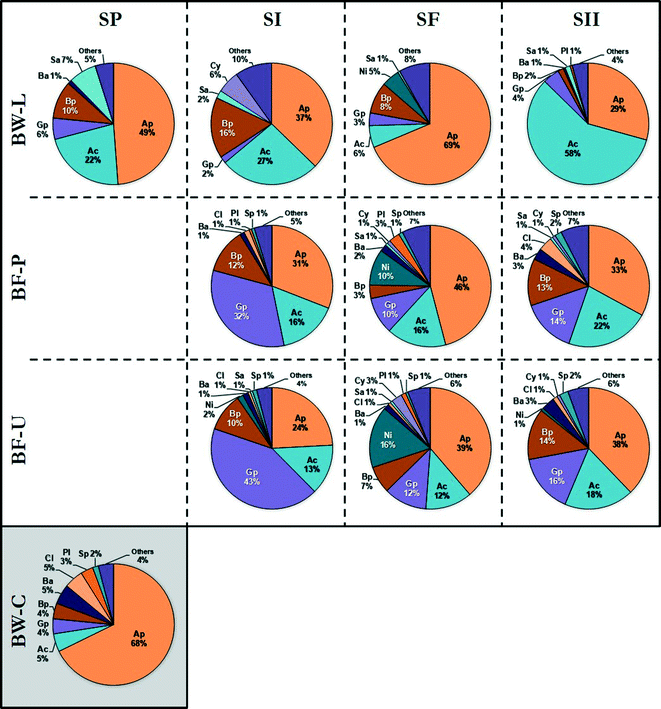 |
| Fig. 2 Distribution of bacterial classes in bulk water (BW) and biofilm (BF) samples as determined by partial 16S rRNA gene sequencing. Samples were identified by source: biofilm (PVC [BF-P]; copper [BF-U]) and bulk water (municipal water [BW-C]; DWDS simulator [BW-L]) and grouped in their corresponding operational schemes (see Table 1 and Fig. 1). Legend (class): Alphaproteobacteria [Ap], Actinobacteria [Ac], Gammaproteobacteria [Gp], Betaproteobacteria [Bp], Nitrospira [Ni], Bacilli [Ba], Clostridia [Cl], Saprospirae [Sa], Cytophagia [Cy], Planctomycetia [Pl], Sphingobacteriia [Sp], and others representing <1% [Others]. Figures represent the average relative abundance of classes. The number of samples (n) is listed in Table 2. | |
The occurrence and dominance of these groups and more specifically the classes Proteobacteria and Actinobacteria (Fig. 2) are apparent within other DWDS,44–49 albeit not at the same ratio. For example, a study of the microbial composition in a Michigan DWDS determined that these groups constituted approximately ≈75% of the total number of OTUs.50 However, the same study found that less than 1% of the sequences were identified as Mycobacterium-related species (Actinobacteria) compared with up to 77% recovered in this study. Differences in the proportion of members in the DWDS microbiome (i.e., community structure) may be attributed to three major factors:51 (i) water quality, including water source, disinfectant residual, treatment processes, and temperature; (ii) hydraulic-physical properties of the distribution system; (iii) infrastructure, such as pipe material and age, storage and physical integrity (e.g., leaks). Overall, the taxonomic composition and distribution of waterborne bacteria are consistent with previous studies of the microbial community in pipe-loop DWDS simulators using the same finished water source.52–55 These findings suggest that these bacterial groups may be considered part of the core microbiota of this DWDS.
Microbial community assemblages
The resulting nMDS ordination plot (n = 213; rarefied to 1050 reads per sample) highlighted a noticeable variability in the community structure between chlorine-treated and chloramine-treated systems and their corresponding biofilm samples (Fig. 3). The clustering of samples in the plot was further confirmed by one-way ANOSIM based on disinfectant (BW-C vs. BW-L; Global R = 0.851, p < 0.001) and source (BW vs. BF; Global R = 0.728, p < 0.001), indicating that each group consisted of a unique community (Table S2†). Overall, the bacterial community structure and diversity in DWDS differed depending on the disinfection strategy (BW-C vs. BW-L; Fig. 3a), source (BW-L vs. BF; Fig. 3b) and operational scheme (e.g., system failure; Fig. 3c). The microbial communities examined in this study are diverse (Table 2) and contain >66% of unique species (i.e., OTUs). However, the majority of these unique OTUs, representing 66% and 8% of the total OTU diversity, are singletons and doubletons (i.e., an OTU with only one and two sequences for all samples combined, respectively). These OTUs represent the rare biosphere and explained <0.99% (Similarity Percentage [SIMPER] analysis) of the dissimilarity within the DWDS simulator communities. Most of the dissimilarity (≈75%, SIMPER analysis) is explained by a few OTUs (46 out of 2923). These results suggest the minor contribution of this fraction of the rare biosphere to the dissimilarities between communities.44,52 Nevertheless, we cannot disregard the importance of this fraction and its possible implications for the contribution of microbial diversity to ecosystem functioning. Growing evidence suggests that members of this rare biosphere can become dormant by entering a reversible state of low metabolic activity and become members of a seed bank, which can have the potential to increase under favorable conditions.56 Our study provides evidence of several “viable” rare bacterial taxa (PMA-treated samples, see Materials and methods) that have the potential to respond to environmental disturbances (see discussion below).
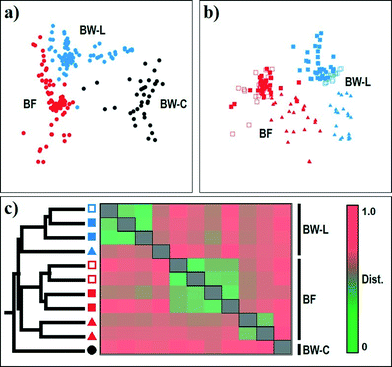 |
| Fig. 3 Non-metric multidimensional scaling (nMDS) ordination plots of the bacterial community for (a) all sampling sites and (b) all DWDS simulator sampling sites combined. (c) Cluster analysis and dissimilarity coefficient heat map of bacterial community profiles for all sampling sites. Hierarchical clustering indicates five clusters, one containing all BW-C samples, two for SF samples from BW-L and BF, and the other two representing SP, SI, and SII samples from BW-L and BF. Analyses were based on the Jensen–Shannon dissimilarity of 16S rRNA OTU-level bacterial profiles (cutoff = 0.03). Samples were identified by source: biofilm (BF) and bulk water (municipal water [BW-C]; DWDS simulator [BW-L]) and grouped in their corresponding operational schemes (see Table 1 and Fig. 1). Legend: BW-C [●]; BW-L (SP, SI) [ ]; BW-L (SF) [ ]; BW-L (SII) [ ]; BF (SI) [ ]; BF (SF) [ ]; BF (SII) [ ]. | |
The major dissimilarities between microbial assemblages were attributed to the variation of two major components of the community: (i) the core microbiome and (ii) conditionally rare taxa (CRT). The OTUs identified within all sample sites in the DWDS are considered part of the core microbiota.44,57 The core microbiome is composed of 194 OTUs (7% of the total OTU diversity), representing 86% of the reads with a high proportion of members of the phyla Actinobacteria and Proteobacteria. The majority of the core representatives were identified as the numerically dominant bacterial species in their respective samples but at a different abundance. For example, one OTU (Mycobacterium spp.) was the dominant representative in the chloramine-treated (BW-L) samples with a relative distribution of 27% but only 3% in the chlorine-treated (BW-C) samples (i.e., disinfectant; Fig. 3a). Furthermore, the combined relative distribution of two OTUs (Rhizobiales [order] and Magnetospirillum spp.) was 19% in the BW-C samples but <0.06% in the BW-L samples (Fig. 3a). CRT are rare microbial taxa that occasionally become very abundant, and in many ecosystems play an important role in structuring microbial communities.58 For instance, the rare member Nitrospira spp. was detected in low abundance or below the limit of detection (<0.14%) under normal operational parameters (i.e., SI and SII) in the BW-L samples, but their relative abundance increased to noticeable levels during the failure of the system (i.e., SF). Similar patterns were observed with several OTUs in the BW-L and BF samples during marked disturbance events (see discussion below). CRT can be more useful than current indicators (e.g., monitoring of chemical parameters) of environmental changes that are difficult to detect,58 for example, providing early-warning signals for critical assessment of nitrification events in chloraminated DWDS. This is evidenced by changes in the relative distribution of some members of the rare biosphere and their contribution to the temporal shifts in the community structure during nitrification (Fig. 3b).
Effect of nitrification and chlorine burn on total bacteria
In this study, the effects of disturbance on BW and BF communities were demonstrated by comparison of bacterial biomass (measured as total ATP, protein content, and DNA concentration) and community diversity indices. An increase in temperature (Fig. 1) accelerated chloramine decay and promoted nitrification (i.e., SF), causing an increase in microbial biomass (Fig. S2a†). As expected, a chlorine burn (i.e., SR; based on measurements taken in SII) significantly affected the microbial biomass (Fig. S2a†). Following the chlorine burn, a continuous increase in biomass was observed even after the DWDS simulator was switched back to chloramine and normal operation (data not shown).
As opposed to the bulk water phase, biofilms provide physical stability and protection against the direct action of disinfectants.59 For instance, biomass and species richness were higher in BF compared to BW communities (Fig. S2†). Furthermore, the response to disturbance by the microbial population at each phase is different; for example, richness increased in BF during failure of the system, while slightly decreasing in BW-L samples (Fig. S2b†). An opposite pattern was observed for NRI values (Fig. S2c†). Higher NRI values indicate a clustered phylogeny where coexisting taxa are more related to each other, while lower NRI values indicate an overdispersed phylogeny where coexisting taxa are less related to each other than would be expected by chance.32 A system failure, or disturbance, may play an important role in shaping the community structure by creating an opportunity for distantly related species to coexist with local assemblages (e.g., low NRI values) in biofilms (Fig. S2c†). For example, nitrifiers were undetected or detected in very low numbers through stable operational schemes (SI and SII), however their relative abundance increased significantly during SF (i.e., minimal disinfectant residual). Again, this demonstrates the major role of CRT members (e.g., nitrifiers) in structuring microbial communities.58
It is important to understand the ecology of DWDS biofilms to better assess potential public health risks and ultimately to develop control options for opportunistic environmental pathogens that utilize biofilms as transient or even long-term habitats. For instance, BF assemblages sampled immediately after a chlorine burn were composed of low diverse communities of closely related taxa, while BW-L were represented with highly diverse populations of phylogenetically overdispersed communities. These results suggest a slower rate of recovery by BF, compared to BW-L communities. In general, phylogenetic clustering in stable operational schemes (SI and SII) may indicate potential positive interactions or habitat filtering, where groups with suitable colonization abilities survive.32 Conversely, nitrification (i.e., failure) was characterized by phylogenetic overdispersion, suggesting competition for establishment in the available substrate.60
Disinfectants and waterborne opportunistic pathogens
Disinfection in drinking water provides the final barrier to transmission of a wide variety of potentially waterborne infectious agents; however, waterborne disease outbreaks continue to occur, and in many cases, efforts to reduce potential health risks from disinfection by-products (DBPs) likely play an important role in increasing this risk.61 Chlorine-based disinfectants are the most commonly used disinfectants but produce DBPs, a potential human health risk. As alternatives to chlorine-based treatments, disinfectants such as chloramine are favored as secondary water disinfectants. A weaker oxidant than chlorine, chloramines are more persistent in the DWDS allowing a residual disinfectant to last much longer, although the microbial community may produce nitrate via nitrification causing a system failure.1 Maintaining the required levels of microbial inactivation and water quality while minimizing potential health risks (e.g., DBPs) requires the application of proper risk and disinfection management protocols.61
DWDS are complex engineered ecosystems that, under certain disinfection management protocols, promote the selective growth and release of water-based opportunistic pathogens, such as Legionella pneumophila and non-tuberculous mycobacteria (NTM).53 Indeed, evidence from this study corroborates previous studies indicating that chloramine (e.g., BW-L) may create environmental conditions favourable for NTM, while chlorine (e.g., BW-C) may produce environmental conditions disadvantageous to environmental Mycobacterium-related species (relative abundance: 27% vs. 3%, respectively).9,53 The use of chloramine for residual disinfection compared with chlorine-treated water was associated with a lower prevalence of free Legionella pneumophila (relative abundance: <0.01% vs. 0.11%, respectively).9,53 DWDS are harsh environments characterized by relatively low organic and inorganic nutrients and the presence of microbial disinfectants. The ability of some microorganisms to tolerate stressors characteristic of DWDS environments and their resistance mechanisms have been well-documented.9,62 For example, Mycobacterium-related species can tolerate stressors through protection within biofilms59 and their unique cell wall composition,63,64 while L. pneumophila is resistant to host cells.62 Further analysis detected additional species (e.g., Reyranella, Methylobacterium, Bradyrhizobium) that are able to resist digestion by amoebae.65 In addition to the aforementioned resistance mechanisms, the DWDS metagenome may contain intrinsic mechanisms or acquired genetic elements to tolerate stressors (e.g., low nutrients and disinfectant).53,66
Antibiotic resistance genes (ARGs)
Genomic analysis revealed that a total of 165 antibiotic resistance determinants (i.e., sequence clusters) were identified in all fifteen genomes mainly grouped in 4 classes (16 types), with the largest proportion of homology to ARGs found to be antibiotic efflux and β-lactamase (Table 3). Our analyses revealed the presence of at least 19 ABC (e.g., macAB, isaE, msbA), 33 RND (mexB, mexE-oprN, mexHI, mexW, mexX, mdtBC, mdtN, mdtP, smeS) and 48 MFS (tcmA, tetA, tetV) antibiotic efflux pump systems with thirty-five β-lactamases (10 class-A and 25 class-B) (Table 3). These mechanisms and their genes potentially provide resistance against erythromycin (e.g., macAB), lincosamide (isaE), amphipathic drugs (msbA), ciprofloxacin (mexE-oprN), novobiocin (mdtBC), tetracenomycin C (tcmA) and tetracycline (tetV).40 Enzymatic modification is a common type of antibiotic resistance; for example, an existing cellular enzyme is modified to react with the antibiotic or alternatively the binding site is altered. Genes encoding for aminoglycoside, chloramphenicol, and rifampin modifying enzymes and two types of mechanisms for the alteration of the binding site (methylation of 23S rRNA [cfrA] and modification of lipopolysaccharides [pmrE]) were detected in the genomes (Table 3). Four clusters carrying resistance mutations (soxR, embAB, katG and parE) were detected, potentially providing resistance against ethambutol, isoniazid, and fluoroquinolones (Table 3). The genome of V. paradoxus contained ≈32 ARGs (CARD; Fig. S3†), followed by M. immunogenum with ≈14 (Fig. S4†), and M. chelonae and Sphingopyxis spp. with 11 (Fig. 4a) and 10 (Fig. S5†), respectively. The genome of V. paradoxus contains genomic features seen in autotrophic and heterotrophic lifestyles that provide remarkable adaptability to variation in environmental conditions.67 However, many of these antibiotic resistance phenotypes are likely intrinsic in these isolates, and resistance transfer to human-related bacteria can be considered highly unlikely.68
Table 3 Antimicrobial resistance gene mechanisms in bacterial genomes
Mechanism [gene] (antibiotic) |
Bacterial genusb |
Mi. |
Mc. |
Vp. |
Sp. |
Databases: RESFAMS = AR family profile HMMs;38 CARD = Comprehensive Antibiotic Resistance Database.40
Isolates: Mi. = Mycobacterium immunogenum (n = 6); Mc. = Mycobacterium chelonae (n = 4); Vp. = Variovorax paradoxus (n = 2); Sp. = Sphingopyxis spp. strain H (n = 3).
Individual types in ARG mechanisms.
|
RESFAMS databasea |
|
|
|
|
Efflux pumps |
|
|
|
|
ABC transporter |
2c |
1 |
9 |
2 |
MFS transporter |
|
|
3 |
2 |
RND transporter |
|
|
11 |
5 |
β-Lactamase |
|
|
|
|
Class A |
1 |
1 |
2 |
1 |
Class B |
5 |
4 |
9 |
2 |
Mutations |
|
|
|
|
Redox [soxR] |
|
|
1 |
|
Modifications |
|
|
|
|
Nucleotidyltransferases [ANT] (aminoglycosides) |
|
|
2 |
|
O-Acetyltransferases [CAT] (chloramphenicol) |
1 |
|
|
|
O-Phosphotransferases [APH] (aminoglycosides) |
3 |
2 |
2 |
|
CARD database |
|
|
|
|
Efflux pumps |
|
|
|
|
ABC transporter |
|
|
4 |
|
MFS transporter |
10 |
8 |
14 |
3 |
RND transporter |
|
|
12 |
5 |
β-Lactamase |
|
|
|
|
Class A |
1 |
1 |
1 |
|
Class B |
|
|
1 |
|
Mutations |
|
|
|
|
Arabinosyltransferases [embAB] (ethambutol) |
2 |
|
|
|
Catalase-peroxidase [katG] (isoniazid) |
1 |
|
|
|
Topoisomerase IV [parE] (fluoroquinolones) |
1 |
1 |
|
|
Modifications |
|
|
|
|
Adenyltransferases [ANT(3′′)-Ia] (aminoglycosides) |
|
|
1 |
|
Lipopolysaccharide and lipid A [pmrE] (polymyxin) |
|
|
|
2 |
Methylation of 23S rRNA [cfrA] (florfenicol) |
1 |
1 |
1 |
|
O-Acetyltransferases [CAT] (chloramphenicol) |
|
|
|
1 |
Ribosyltransferase [arr-1] (rifampin) |
1 |
|
|
|
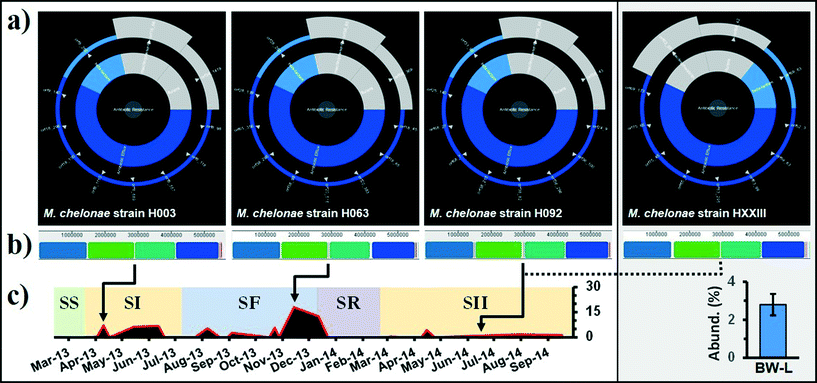 |
| Fig. 4 (a) Analysis of the whole genome of Mycobacterium chelonae strains by the Resistance Gene Identifier (RGI) with overall resistance in the centre, resistance classes in the middle, and individual resistance genes on the outer (open reading frames). “Resistance wheel” for strains H003 (SI; BF-P), H063 (SF; BF-P), H092 (SII; BF-P) and HXXIII (SII; BW-L), predicting resistance to a broad range of antibiotic classes. (b) Alignment of M. chelonae genomes using progressive Mauve. Similar colored blocks indicate regions in each genome that are homologous. M. chelonae ATCC 35752 was used as the reference genome for reordering of contigs. (c) Relative distribution (%) of Otu000001 Mycobacterium spp. in BF-P and BW-C. Samples were identified by source: biofilm (PVC [BF-P]) and bulk water (DWDS simulator [BW-L]) and grouped in their corresponding operational schemes (see Table 1 and Fig. 1). | |
There is a common notion that biofilms play an important role in DWDS ecosystem processes as reservoirs of antibiotic-resistant bacteria (ARB);68 however, their contribution to the acquisition and spread of antibiotic resistance has not been fully explored in this ecosystem.69 For example, there is little information about the genome structure of bacterial cells in biofilms under selection pressures (e.g., disturbances). In this particular study, the genome structure is defined as the entire DNA sequence and their associated gene functions (i.e., ARGs). Three biofilm M. chelonae strains corresponding to the SI, SF, and SII operational schemes were randomly selected from a collection of isolates to study the genome structure after episodes of disturbance. Additionally, one strain (HXXIII) representing the bulk water population was used to compare against the biofilm strains. These strains represent the dominant member of the DWDS microbial community (i.e., 100% 16 rRNA similarity with the dominant OTU representative; see the Microbial community assemblages section). ARG annotation of the four M. chelonae genomes shows similar ARGs with a high sequence homology among strains (Fig. 4a). For example, sequence alignments show that M. chelonae strains contained no point mutation in genes encoding for a MFS transporter (TetV; Fig. S6†) and a β-lactamase (class A; Fig. S7†). Multiple M. chelonae genome alignment (i.e., DNA sequence) identified and aligned 11 Locally Collinear Blocks (LCBs; conserved sequence segments internally free of genomic rearrangements) with an average of 97.6% of each genome contained within 4 LCBs, and the remaining lineage-specific regions reside in breakpoint regions (Fig. 4b). Although episodes of disturbance (e.g., system failure and disinfectant) may have induced a selection pressure on the M. chelonae sub-population (Fig. 4c), our genomic comparisons demonstrated that at least some isolates (from similar strains) maintained a comparable genome structure with a core assemblage of ARGs. This is relevant because non-clinical environmental isolates have been highlighted as an important factor in the dissemination of antibiotic resistance genes (ARGs). To ensure public health and improve risk assessments, it is essential to incorporate the resistome when monitoring the biological stability of these systems.
Conclusions
This study demonstrated that DWDS microbial communities are sensitive to changes in operational parameters (i.e., nitrification, disinfectant residual) and respond to a disturbance by returning to their stable state after a shift in community composition (i.e., resilience). There is a common notion that episodes of disturbance induce a selection pressure on DWDS populations; however, we examined genomes of cultured isolates that maintained the same genomic structure (including a core assemblage of ARGs) among representatives of the same bacterial taxa. It is important to note that our study characterized the genome structure of a limited number of isolates of the dominant bacterial taxa from different operational schemes to address the question of comparable genome structures. Consequently, there is the possibility that the response (e.g., genome structure) to disturbance under these scenarios by other waterborne representatives may reveal a different outcome. Nevertheless, we found that at least some isolates (from similar strains) maintained a comparable genome structure with a core assembly of ARGs. Overall, several members of the dominant population are antibiotic-resistant bacteria (ARB), and the clearest consequence of disturbance is a shift in the relative abundance of ARB and conditionally rare taxa (CRT) in the ecosystem (e.g., increased during system failure). The prevalence of ARB in DWDS is exacerbated by additional variables, such as the anthropogenic release of antibiotics and the presence of biofilms in DWDS. Biofilms provide physical stability and protection against the direct action of environmental stressors, also favoring metabolic interactions and genetic interchange between microbial populations. Understanding the behavior of microbial communities in disturbed DWDS environments is essential for risk management and evaluation of the biological stability of these systems. Such information could be incorporated into ecosystem process models and greatly enhance our ability to predict ecosystem responses to disturbances. The information generated in this study improves our understanding of the drinking water exposome, particularly the potential sources of adverse exposure (unintended consequences of disinfectant switching practices), and how these sources may be monitored to ensure public and ecosystem health.
Acknowledgements
The authors thank Dawn King, Tim Kling, Dave Elstun, Sharon Kidney and Gary Lubbers for assistance in this project. The US EPA through the Office of Research and Development funded and managed this research. The opinions expressed are those of the authors, and do not necessarily reflect the official positions and policies of the US EPA. Any mention of product or trade names does not constitute recommendation for use by the US EPA.
References
- Y. Zhang, N. Love and M. Edwards, Crit. Rev. Environ. Sci. Technol., 2009, 39, 153–208 CrossRef CAS.
- K. D. Pintar and R. M. Slawson, Water Res., 2003, 37, 1805–1817 CrossRef CAS PubMed.
- B. A. Carrico, F. A. DiGiano, N. G. Love, P. Vikesland, K. Chandran, M. Fiss and A. Zaklikowski, J. - Am. Water Works Assoc., 2008, 100, 104–115 CAS.
- E. J. Rykiel, Aust. J. Ecol., 1985, 10, 361–365 CrossRef.
- W. P. Sousa, Annu. Rev. Ecol. Syst., 1984, 15, 353–391 CrossRef.
- M. Berga, A. J. Székely and S. Langenheder, PLoS One, 2012, 7, e36959 CAS.
- A. Shade, H. Peter, S. D. Allison, D. L. Baho, M. Berga, H. Bürgmann, D. H. Huber, S. Langenheder, J. T. Lennon, J. B. H. Martiny, K. L. Matulich, T. M. Schmidt and J. Handelsman, Front. Microbiol., 2012, 3, 417 CrossRef PubMed.
- S. D. Allison and J. B. H. Martiny, Proc. Natl. Acad. Sci. U. S. A., 2008, 105, 11512–11519 CrossRef CAS PubMed.
- N. J. Ashbolt, Curr. Environ. Health Rep., 2015, 2, 95–106 CrossRef PubMed.
- B. Li, Y. Yang, L. Ma, F. Ju, F. Guo, J. M. Tiedje and T. Zhang, ISME J., 2015, 9, 2490–2502 CrossRef CAS PubMed.
- B. Berglund, Infect. Ecol. Epidemiol., 2015, 5, 28564 Search PubMed.
- R. Laxminarayan, A. Duse, C. Wattal, A. K. M. Zaidi, H. F. L. Wertheim, N. Sumpradit, E. Vlieghe, G. Levy Hara, I. M. Gould, H. Goossens, C. Greko, A. D. So, M. Bigdeli, G. Tomson, W. Woodhouse, E. Ombaka, A. Q. Peralta, F. N. Qamar, F. Mir, S. Kariuki, Z. A. Bhutta, A. Coates, R. Bergstrom, G. D. Wright, E. D. Brown and O. Cars, Lancet Infect. Dis., 2013, 13, 1057–1098 CrossRef PubMed.
- N. Fahrenfeld, Y. Ma, M. O'Brien and A. Pruden, Front. Microbiol., 2013, 4, 130 Search PubMed.
- P. Shi, S. Jia, X. X. Zhang, T. Zhang, S. Cheng and A. Li, Water Res., 2013, 47, 111–120 CrossRef CAS PubMed.
- C. Xi, Y. Zhang, C. F. Marrs, W. Ye, C. Simon, B. Foxman and J. Nriagu, Appl. Environ. Microbiol., 2009, 75, 5714–5718 CrossRef CAS PubMed.
- Q. B. Yuan, M. T. Guo and J. Yang, PLoS One, 2015, 10, e0119403 Search PubMed.
- M. J. Figueras and J. J. Borrego, Int. J. Environ. Res. Public Health, 2010, 7, 4179–4202 CrossRef PubMed.
- G. Dunn, L. Harris, C. Cook and N. Prystajecky, Sci. Total Environ., 2014, 468–469, 544–552 CrossRef CAS PubMed.
- D. van der Kooij, Water, Air, Soil Pollut., 2000, 123, 25–34 CrossRef CAS.
-
USEPA (United States Environmental Protection Agency), Method 353.2, Revision 2.0: Determination of Nitrate-Nitrite by Automated Colorimetry, ed. J. W. O'Dell, Office of Research and Development, Cincinnati, OH, 1993 Search PubMed.
-
USEPA (United States Environmental Protection Agency), Method 365.1: Determination of Phosphorus by Semi-Automated Colorimetry, ed. J. W. O'Dell, Office of Research and Development, Cincinnati, OH, 1993 Search PubMed.
- K. N. Hellein, E. M. Kennedy, V. J. Harwood, K. V. Gordon, S. Y. Wang and J. E. Lepo, J. Microbiol. Methods, 2012, 89, 76–78 CrossRef CAS PubMed.
- A. Nocker, T. Richter-Heitmann, R. Montijn, F. Schuren and R. Kort, Int. Microbiol., 2010, 13, 59–65 CAS.
- J. G. Caporaso, C. L. Lauber, W. A. Walters, D. Berg-Lyons, J. Huntley, N. Fierer, S. M. Owens, J. Betley, L. Fraser, M. Bauer, N. Gormley, J. A. Gilbert, G. Smith and R. Knight, ISME J., 2012, 6, 1621–1624 CrossRef CAS PubMed.
- G. Liu, J. Q. Verberk and J. C. Van Dijk, Appl. Microbiol. Biotechnol., 2013, 97, 9265–9276 CrossRef CAS PubMed.
- M. Berney, M. Vital, I. Hülshoff, H. U. Weilenmann, T. Egli and F. Hammes, Water Res., 2008, 42, 4010–4018 CrossRef CAS PubMed.
- P. D. Schloss, S. L. Westcott, T. Ryabin, J. R. Hall, M. Hartmann, E. B. Hollister, R. A. Lesniewski, B. B. Oakley, D. H. Parks, C. J. Robinson, J. W. Sahl, B. Stres, G. G. Thallinger, D. J. Van Horn and C. F. Weber, Appl. Environ. Microbiol., 2009, 75, 7537–7541 CrossRef CAS PubMed.
- J. J. Kozich, S. L. Westcott, N. T. Baxter, S. K. Highlander and P. D. Schloss, Appl. Environ. Microbiol., 2013, 79, 5112–5120 CrossRef CAS PubMed.
- T. C. J. Hill, K. A. Walsh, J. A. Harris and B. F. Moffett, FEMS Microbiol. Ecol., 2003, 43, 1–11 CrossRef CAS PubMed.
- Ø. Hammer, D. A. T. Harper and P. D. Ryan, Palaeontol. Electron., 2001, 4, 1–9 Search PubMed.
- K. R. Clarke, Aust. J. Ecol., 1993, 18, 117–143 CrossRef.
- C. O. Webb, D. D. Ackerly, M. A. McPeek and M. J. Donoghue, Annu. Rev. Ecol. Syst., 2002, 33, 475–505 CrossRef.
- V. Gomez-Alvarez and R. P. Revetta, Genome Announc., 2016, 4, e01538 Search PubMed.
- A. Bankevich, S. Nurk, D. Antipov, A. A. Gurevich, M. Dvorkin, A. S. Kulikov, V. M. Lesin, S. I. Nikolenko, S. Pham, A. D. Prjibelski, A. V. Pyshkin, A. V. Sirotkin, N. Vyahhi, G. Tesler, M. A. Alekseyev and P. A. Pevzner, J. Comput. Biol., 2012, 19, 455–477 CrossRef CAS PubMed.
- T. Seemann, Bioinformatics, 2014, 30, 2068–2069 CrossRef CAS PubMed.
- A. C. E. Darling, B. Mau, F. R. Blattner and N. T. Perna, Genome Res., 2004, 14, 1394–1403 CrossRef CAS PubMed.
- V. A. Simossis and J. Heringa, Nucleic Acids Res., 2005, 33, W289–W294 CrossRef CAS PubMed.
- M. K. Gibson, K. J. Forsberg and G. Dantas, ISME J., 2015, 9, 207–216 CrossRef CAS PubMed.
- S. R. Eddy, Genome Inform., 2009, 23, 205–211 Search PubMed.
- A. G. McArthur, N. Waglechner, F. Nizam, A. Yan, M. A. Azad, A. J. Baylay, K. Bhullar, M. J. Canova, G. De Pascale, L. Ejim, L. Kalan, A. M. King, K. Koteva, M. Morar, M. R. Mulvey, J. S. O'Brien, A. C. Pawlowski, L. J. Piddock, P. Spanogiannopoulos, A. D. Sutherland, I. Tang, P. L. Taylor, M. Thaker, W. Wang, M. Yan, T. Yu and G. D. Wright, Antimicrob. Agents Chemother., 2013, 57, 3348–3357 CrossRef CAS PubMed.
- D. R. Nemergut, S. K. Schmidt, T. Fukami, S. P. O'Neill, T. M. Bilinski, L. F. Stanish, J. E. Knelman, J. L. Darcy, R. C. Lynch., P. Wickey and S. Ferrenberg, Microbiol. Mol. Biol. Rev., 2013, 77, 342–356 CrossRef PubMed.
- R. P. Revetta, R. S. Matlib and J. W. Santo Domingo, Curr. Microbiol., 2011, 63, 50–59 CrossRef CAS PubMed.
- A. J. Pinto and L. Raskin, PLoS One, 2012, 7, e43093 CAS.
- V. Gomez-Alvarez, B. W. Humrighouse, R. P. Revetta and J. W. Santo Domingo, J. Water Health, 2015, 13, 140–151 CrossRef PubMed.
- J. El-Chakhtoura, E. Prest, P. Saikaly, M. van Loosdrecht, F. Hammes and H. Vrouwenvelder, Water Res., 2015, 74, 180–190 CrossRef CAS PubMed.
- J. J. Kelly, N. Minalt, A. Culotti, M. Pryor and A. Packman, PLoS One, 2014, 9, e98542 Search PubMed.
- K. Henne, L. Kahlisch, I. Brettar and M. G. Höfle, Appl. Environ. Microbiol., 2012, 78, 3530–3538 CrossRef CAS PubMed.
- P. Y. Hong, C. Hwang, F. Ling, G. L. Andersen, M. W. LeChevallier and W. T. Liu, Appl. Environ. Microbiol., 2010, 76, 5631–5635 CrossRef CAS PubMed.
- S. Eichler, R. Christen, C. Holtje, P. Westphal, J. Botel, I. Brettar, A. Mehling and M. G. Höfle, Appl. Environ. Microbiol., 2006, 72, 1858–1872 CrossRef CAS PubMed.
- A. J. Pinto, C. Xi and L. Raskin, Environ. Sci. Technol., 2012, 46, 8851–8859 CrossRef CAS PubMed.
-
M. Ingerson-Mahar and A. Reid, Microbes in Pipes: The Microbiology of the Water Distribution System, American Society for Microbiology, Washington DC, 2013 Search PubMed.
- R. P. Revetta, V. Gomez-Alvarez, T. L. Gerke, C. Curioso, J. W. Santo Domingo and N. J. Ashbolt, FEMS Microbiol. Ecol., 2013, 86, 404–414 CrossRef CAS PubMed.
- V. Gomez-Alvarez, R. P. Revetta and J. W. Santo Domingo, Appl. Environ. Microbiol., 2012, 78, 6095–6102 CrossRef CAS PubMed.
- M. M. Williams, J. W. Santo Domingo, M. C. Meckes, C. A. Kelty and H. S. Rochon, J. Appl. Microbiol., 2004, 96, 954–964 CrossRef CAS PubMed.
- M. M. Williams, J. W. Santo Domingo and M. C. Meckes, Biofouling, 2005, 21, 279–288 CrossRef PubMed.
- S. E. Jones and J. T. Lennon, Proc. Natl. Acad. Sci. U. S. A., 2010, 107, 5881–5886 CrossRef CAS PubMed.
- F. Ling, C. Hwang, M. W. LeChevallier, G. L. Andersen and W. T. Liu, ISME J., 2016, 10, 582–595 CrossRef PubMed.
- A. Shade, S. E. Jones, J. G. Caporaso, J. Handelsman, R. Knight, N. Fierer and J. A. Gilbert, mBio, 2014, 5, e01371 CrossRef PubMed.
- A. Bridier, R. Briandet, V. Thomas and F. Dubois-Brissonnet, Biofouling, 2011, 27, 1017–1032 CrossRef CAS PubMed.
- N. Fierer, D. Nemergut, R. Knight and J. M. Craine, Res. Microbiol., 2010, 161, 635–642 CrossRef PubMed.
- N. Ngwenya, E. J. Ncube and J. Parsons, Rev. Environ. Contam. Toxicol., 2013, 222, 111–170 CAS.
- D. Berry, C. Xi and L. Raskin, Curr. Opin. Biotechnol., 2006, 17, 297–302 CrossRef CAS PubMed.
- S. M. September, V. S. Brözel and S. N. Venter, Appl. Environ. Microbiol., 2004, 70, 7571–7573 CrossRef CAS PubMed.
- M. J. Vaerewijck, G. Huys, J. C. Palomino, J. Swings and F. Portaels, FEMS Microbiol. Rev., 2005, 29, 911–934 CrossRef CAS PubMed.
- G. Greub and D. Raoult, Clin. Microbiol. Rev., 2004, 17, 413–433 CrossRef PubMed.
- Y. Chao, L. Ma, Y. Yang, F. Ju, X. X. Zhang, W. M. Wu and T. Zhang, Sci. Rep., 2013, 3, 3550 Search PubMed.
- J. I. Han, H. K. Choi, S. W. Lee, P. M. Orwin, J. Kim, S. L. Laroe, T. G. Kim, J. O'Neil, J. R. Leadbetter, S. Y. Lee, C. G. Hur, J. C. Spain, G. Ovchinnikova, L. Goodwin and C. Han, J. Bacteriol., 2011, 193, 1183–1190 CrossRef CAS PubMed.
- I. Vaz-Moreira, O. C. Nunes and C. M. Manaia, FEMS Microbiol. Rev., 2014, 38, 761–778 CrossRef CAS PubMed.
- J. L. Balcázar, J. Subirats and C. M. Borrego, Front. Microbiol., 2015, 6, 1216 Search PubMed.
Footnote |
† Electronic supplementary information (ESI) available. See DOI: 10.1039/c6ew00053c |
|
This journal is © The Royal Society of Chemistry 2016 |
Click here to see how this site uses Cookies. View our privacy policy here.