DOI:
10.1039/C6RA10533E
(Paper)
RSC Adv., 2016,
6, 64967-64976
Interaction of p-synephrine with p-chloranil: experimental design and multiple response optimization
Received
23rd April 2016
, Accepted 1st July 2016
First published on 4th July 2016
Abstract
In the current paper, and for the first time, spectrophotometry coupled with a set of factorial designs was executed as a new tool for investigating the interaction between p-synephrine (p-SY) and p-chloranil (p-CHL). Two types of complexes were observed as a result of this interaction. The first complex, an intermolecular charge transfer complex, was measured at 452 nm, while the second, an intramolecular charge transfer complex, was measured at 536 nm. A chemometric assisted screening and optimization of the absorbance at the two wavelengths was scrutinized. As a screening procedure, a 2-level full factorial design (2k-FFD) was employed. Method variables, such as reaction temperature, heating time, reagent volume, and solvent type were considered and the response was measured at the individual wavelengths. With the help of Pareto analysis, and ANOVA testing (following a response transformation employing Box–Cox treatment), variables ascertained to be significant (p < 0.05) were selected. Significant factors were carefully adjusted, exploiting a response surface methodology (RSM) with a face-centered central composite design (FCCCD). Afterwards, and with the help of the overlaid contour plots, and Derringer's desirability function, the multiple responses (Y1 and Y2) were simultaneously optimized and consolidated into a unified performance characteristic. The proposed model denoted the efficacy of experimental designs in adjusting the reaction variables and maximizing the output. Scott's plot, and other models were used to determine the formation constant of a 1
:
1 complex. Validation of the proposed procedure was performed using ANOVA. Linearity, detection and quantification limits as well as accuracy, within-day and intermediate precisions have been assessed following ICH guidelines for evaluation of analytical procedures.
1. Introduction
Synephrine (SY) is chemically recognized as 4-[1-hydroxy-2(methyl amino)ethyl]phenol (Scheme 1).1 Though being available via synthetic routes, SY was originally isolated from natural sources such as bitter orange. Acting by stimulating many receptors (α1, α2, β1, β2, β3 and 5-HT) to different degrees, SY has a wide variety of biological effects. Uses of SY as antihypotensive, and in treatment of conjunctival congestion have been reported.1 The controversial usage of SY as a thermogenic agent either alone or in combination with caffeine, has forced many regulations. According to Health Canada, weight loss products containing SY and caffeine are not allowed.2–6 Reviewing literature showed that most of the described procedures for SY determination are chromatographic approaches, which might not be suitable for routine applications.7–13 Having a look on the chemical structure of p-SY, Scheme 1, with its electron rich benzene ring, and electron donating hydroxyl and 2°-amino groups, one can observe its suitability for being an electron donor. To the best of our knowledge, no spectrophotometric procedure based on charge transfer complex (CTC) formation was reported for p-SY determination. With a variety of applications, donor (D)–acceptor (A) molecular interactions with subsequent formation of CTCs have become the focus of a variety of many recent investigations.14–17 Studying the electron transfer process between p-SY and quinones, which are known to be popular electron acceptor molecules,18,19 and which exist in many pharmaceutical products,20,21 would be of interest. p-Chloranil (p-CHL; tetrachloro-1,4-benzoquinone) was chosen for this study because of its promising property as an active electron accepting quinone. With four electron withdrawing chlorine atoms and a positive redox potential of 278 mV in acetonitrile (vs. NHE), p-CHL is a special electron pulling molecule for this study.22 In the current investigation, interaction of p-SY (as electron donor) with p-CHL (as electron acceptor) resulted in formation of a colored CTC. Two major peaks were detected and were attributed to the formation of intermolecular (inter, yellow colored, Y1, 452 nm) and intramolecular (intra, violet colored, Y2, 536 nm) CTCs. In reality, both complexes were of interest, keeping in mind the probability of their application as a novel class of chromogenic sensors.14
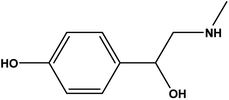 |
| Scheme 1 p-Synephrine. | |
A common feature among all literature reported techniques for determination of p-SY is that they were all based on examination of one factor at a time (OFAT). This approach and though still being used in analytical method development confronts many problems. One of the major restraints associated with this practice is the need for a large number of experiments. Yet, the resulting depiction of “optimal conditions” and hence the system performance cannot be treated with a high degree of inevitability. One reason for that is the lack of assessment of variable–variable interactions in the models designed using OFAT. With an ability to reflect the statistical impact of the discrete independent variables and likewise their interactions through a much fewer number of experiments, multivariate analysis would be the ‘ideal’ approach in this case.23–25
Two phases are usually recognized in building a factorial design: (1) screening and modelling: where all factors that might ‘shape’ the response are considered and only factors proved to have a vital effect on the anticipated response are selected; (2) tuning: where variable ‘best settings’ are modelled.23
Formation of inter- or intra-CTC, in our case, was affected by many variables. These variables and as described by “Minitab® 17” are either “numerical”, such as reaction temperature, reagent volume and reaction time, or “text” such as solvent type, and diluting solvent.26 Formation of inter- and/or intra-CTC was viewed as two dependent response variables. Since more than one response is involved, a discrete analysis of these responses might lead to incompatible findings. Thus, a compromised analysis that includes the overall response into the final model must be considered.27,28 One of the widely used approaches is the “desirability function” which is implemented in almost all software packages.29,30 This function is based on finding a ‘composite desirability’ or in other words, it combines the different responses into one mutual response.
With the purpose of maximizing and optimizing the multiple responses ‘absorbance’ of the CTCs resulting from interaction of p-SY and p-CHL; an experimental design (DOE) was utilized. A 2-level full factorial design (FFD) was used for the screening process, while a face-centred central composite design (CCD) was employed for optimization. A desirability function was used to optimize the multiple responses.
2. Experimental
2.1. Materials and reagents
All reagents were of analytical grade. p-SY was purchased from Sigma-Aldrich (USA) and it was used as received. An HPLC grade p-CHL was purchased from Merck (Darmstadt, Germany). The solvent ethanol was analytically pure. Both p-CHL and p-SY were prepared as 1 mM and 10 mM respectively in ethanol. Further dilutions with the same solvent were carried out to obtain different working solutions.
2.2. Apparatus
An Agilent diode array UV-Vis Spectrometer with 10 mm quartz cell was used for spectrophotometric measurements. A Jenway pH meter equipped with a glass combination electrode (UK) was used for adjusting pH of working solutions. A thermostatically controlled water bath (MLV, Salvis AG Emmenbruck, Luzern, Germany) was used throughout the work. A Minitab® 17 software (Minitab Inc., State College, Pennsylvania, USA) was used for screening and optimizing the factorial design.
2.3. Reaction procedure
Aliquots of standard p-SY solution (1–5 mM) were transferred into 10 mL test tubes, followed by 5 mL of p-CHL solution, and the volume was made up to the mark with ethanol. The mixture was heated up in a water bath (60 °C) for 25 minutes. The absorbance of the resulting colored solution was measured at (300–700 nm) against a reagent blank similarly prepared.
2.4. Experimental design
All the numerical variables that might affect the expected response are listed in Table 1. A 2-level FFD was used for the screening purpose. Upper and lower domains, in addition to one central point for each variable were defined. A 23-FFD (18 runs in the base design, 2 blocks, and 2 centre points in total added to the matrix of design) was executed and all factors were kept free from aliasing. In the second phase, tuning was done using a response surface design. A face-centred central composite design (FCCCD) was employed where the model was created from the full factorial design by adding 16 cube points, 12 axial points, and 12 central points in axial. Twenty experiments were performed in the base run, totalling 40 experiments after two replicates and within 2 blocks. The optimized response was obtained employing a polynomial fit and taking into account the quadratic terms. Multiple response optimization was done using overlaid contour plots and a composite desirability function (D). Three events were considered. In the first case, the goal was to maximize both responses; Y1 (absorbance of inter-CTC) and Y2 (absorbance of intra-CTC). Alternatively, Y1 was maximized while Y2 was minimized. In the last scenario, Y1 was minimized and Y2 was maximized. Proposed models were validated using the analysis of variance (ANOVA) at 95.0% confidence limits.
Table 1 Screened numerical factors and response domains for a two-level (23) full factorial design (FFD) premeditated for the charge transfer reaction
Screened factor “Numerical” |
Symbol |
Level |
Low (−) |
Center Pt (0) |
High (+) |
Temperature (°C) |
A |
25.00 |
42.5 |
60.00 |
Reagent volume (mL) |
B |
1.00 |
3.00 |
5.00 |
Reaction time (min) |
C |
0.00 |
20.0 |
40.00 |
Response |
Y1, Y2 |
Target |
3. Results and discussion
3.1. Charge transfer complex formation reaction
Investigations of the interaction between p-SY and p-CHL, as shown in Scheme 2 and Fig. 1 revealed that different types of complexes were formed in a timely controlled manner. Upon mixing a colourless solution of p-SY and a pale yellow solution of p-CHL in ethanolic medium, a lemon yellow colored inter-CTC was instantaneously formed which corresponds to the bands at 384 nm and 452 nm (Fig. 1: blue line). The band at 452 nm, which corresponds to the formation of p-CHL radical anion, increased for a period of 2 min (Fig. 1: black line). After 2 min, the radical anion band at 452 nm started to decrease and the band at 384 nm increased over a period of 30 min (Fig. 1: green line). After 30 min, the band at 384 nm decreased and a new band at 536 nm formed gradually (orange line), which corresponds to the intra-CTC. A clear isosbestic point can be observed at 426 nm.
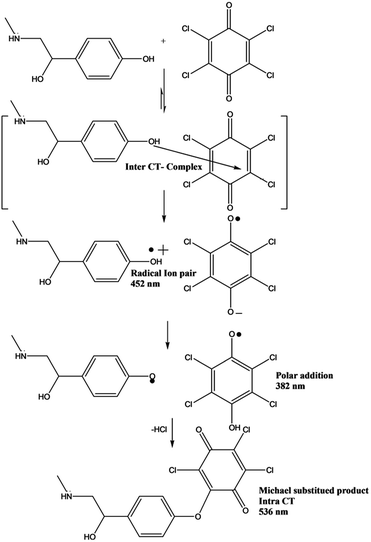 |
| Scheme 2 Suggested reaction mechanism for the formation of inter- and intra-CTCs due to interaction of p-SY with p-CHL. | |
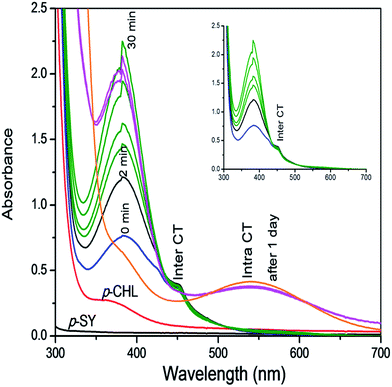 |
| Fig. 1 Electronic spectra for the interaction of p-SY with p-CHL in ethanol at 298 K. (Inset: absorption spectra for the interaction of p-SY with p-CHL up to 30 minutes, inter: intermolecular, intra: intramolecular, CT: charge transfer). [p-SY] was ten times more than [p-CHL]. | |
3.2. Assessment of substantial variables
As shown in Table 1; three factors were able to affect the interaction of p-SY with p-CHL. The executed screening design (23-FFD) allowed the determination of main as well as the interactions of these factors. Using Pareto chart of standardized effects, the magnitude of each factor on the anticipated responses was determined.
As shown in Fig. 2, the effect of the three factors as well as their second order interactions varied between Y1 and Y2. For both responses, the three main factors extend beyond the reference lines, indicating that all are substantial, with reagent volume (B, RV) being the most effective. The interactions (RV × RT) and (Temp × RV) strongly affected Y2, in comparison to a minor effect in case of Y1.
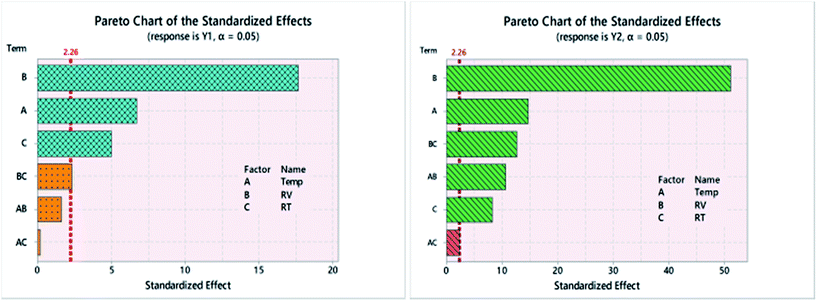 |
| Fig. 2 Pareto chart for 23 – full factorial design for the absorbance of the colored charge transfer complex. Y1 is the first response (absorbance of inter-CTC); Y2 is the second response (absorbance of intra-CTC). Graphs were obtained prior to Box–Cox transformation and include only main effects and second order interactions. | |
Similar implications were concluded employing half-normal and normal plots of standardized effects (figures are not shown).
On the other hand, detection of significant factors in the proposed multifactor model was also corroborated using ANOVA. In this test, all main effects as well as higher order interactions were considered. As shown in Table 2, the preliminary postulations were confirmed.
Table 2 Analysis of variance (ANOVA) at 95.0% confidence level for a 2-level full factorial design. The table is showing results for factorial regression: Y1 and Y2 versus A: Temp, B: RV, and C: RT
Response |
DFa |
Y1 |
Y2 |
Source |
Adj SSa |
Adj MSa |
F-Value |
P-Value |
Adj SSa |
Adj MSa |
F-Value |
P-Value |
DF is degrees of freedom, SS is sum of squares and MS is mean of squares. |
Model |
9 |
0.260132 |
0.028904 |
38.00 |
0.000 |
0.281896 |
0.031322 |
298.18 |
0.000 |
Linear |
3 |
0.257216 |
0.085739 |
112.71 |
0.000 |
0.260570 |
0.086857 |
826.37 |
0.000 |
A: Temp |
1 |
0.032194 |
0.032194 |
42.32 |
0.000 |
0.015686 |
0.015686 |
149.33 |
0.000 |
B: RV |
1 |
0.209618 |
0.209618 |
275.56 |
0.000 |
0.244033 |
0.244033 |
2323.19 |
0.000 |
C: RT |
1 |
0.015404 |
0.015404 |
20.25 |
0.002 |
0.000851 |
0.000851 |
8.10 |
0.022 |
2-Way interactions |
3 |
0.001908 |
0.000636 |
0.84 |
0.511 |
0.016426 |
0.005475 |
52.12 |
0.000 |
A: Temp × B: RV |
1 |
0.000092 |
0.000092 |
0.12 |
0.736 |
0.002322 |
0.002322 |
22.10 |
0.002 |
A: Temp × C: RT |
1 |
0.000069 |
0.000069 |
0.09 |
0.771 |
0.000953 |
0.000953 |
9.07 |
0.017 |
B: RV × C: RT |
1 |
0.001747 |
0.001747 |
2.30 |
0.168 |
0.013151 |
0.013151 |
125.20 |
0.000 |
3-Way interactions |
1 |
0.000554 |
0.000554 |
0.73 |
0.418 |
0.000006 |
0.000006 |
0.06 |
0.819 |
Curvature |
1 |
0.000111 |
0.000111 |
0.15 |
0.712 |
0.004866 |
0.000288 |
46.32 |
0.000 |
Error |
8 |
0.006086 |
0.000761 |
|
|
0.000840 |
0.000105 |
|
|
Total |
17 |
0.266218 |
|
|
|
0.282737 |
|
|
|
3.3. Response transformation and modelling
Residual plots (normplot, histograms, residuals versus fits, and residuals versus order) were used to examine goodness of fit in regression and ANOVA. Obtained normal probability plots and histograms showed an “s-shaped” distribution in case of Y1, compared to “bimodal” distributions in case of Y2, implying that the response variance (σY2) and though being related to the data mean (μY) in certain way, is not constant.31 Response transformations were necessary in this case, Fig. 3.32 As shown in Fig. 4, a better normal distribution was obtained after running Box–Cox transformation,33 where: |
(Transformed response) Y′ = (Yλ − 1)/λ (transformation factor)
| (1) |
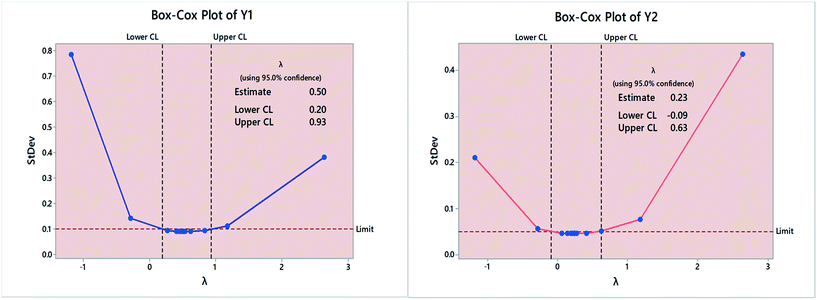 |
| Fig. 3 Box and Cox plots for Y1 and Y2 at 95.0% CI. | |
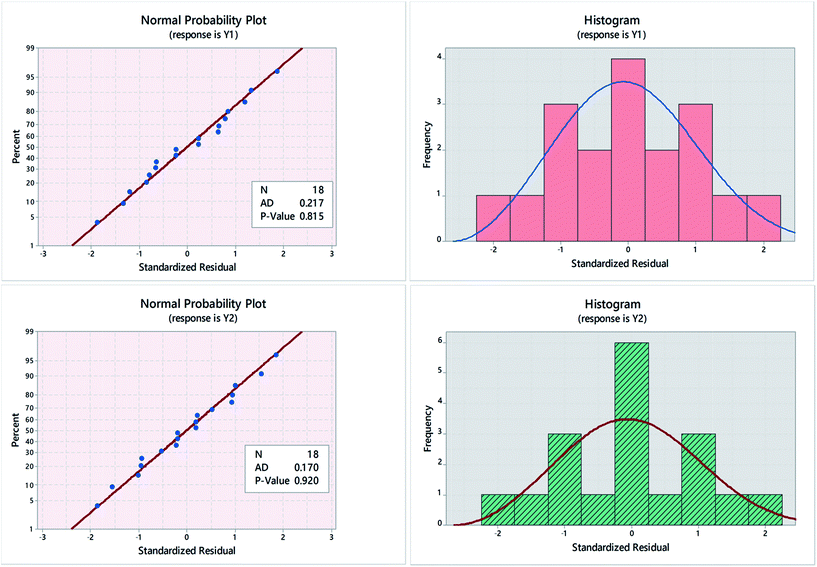 |
| Fig. 4 Normplots and histograms of residuals for Y1 and Y2. Plots were obtained after Box–Cox transformation and considering all terms up to the third order. Histograms were fitted to a 3-parameter Weibull distribution fit. | |
Box–Cox plots were used to determine the need for data transformation as well as the optimal λ value. As shown in Fig. 3, optimal λ value was determined as the curve minima at 95.0% confidence interval (95.0% CI). Estimated λ value was 0.5 and 0.23 in case of Y1 and Y2, respectively. A range of values within the 95.0% CI was tested in terms of p-value and Anderson–Darling (AD) statistic.34
Transformation to normality was indicated primarily by p-values > 0.05. Moreover, smaller AD values; compared with non-transformed data further confirms that data are coming from a normal population. The most appropriate fit was obtained using λ = 0.8 in case of Y1 and =0.6 in case of Y2. Fig. 4, shows the normal probability plots and histograms for Y1 and Y2, following response variable transformation to remove data skewness. The R2 for the fitted model was 97.71% and 99.70% while the adjusted R2 value was 95.14% and 99.37%, for Y1 and Y2 respectively. The regression equation for the proposed model in un-coded units is:
|
Y10.8 = 0.1460 − 0.00274Temp + 0.0582RV − 0.00159RT + 0.000099Temp × RV + 0.000019Temp × RT + 0.000096RV × RT − 0.000008Temp × RV × RT + 0.0079Ct Pt
| (2) |
|
Y20.6 = −0.0251 + 0.001250Temp + 0.03352RV − 0.000738RT + 0.000327Temp × RV − 0.000025Temp × RT + 0.000680RV × RT + 0.000001Temp × RV × RT + 0.05232Ct Pt
| (3) |
Fitting obtained data to either “normal” or 3-parameter Weibull distribution fit provided a good fit for obtained data, Fig. 4.
3.4. Response surface design
A trial for optimization of individual responses using optimization plots and employing individual desirability function (d) on screening data was performed. In general, the desirability function assesses how well a ‘blend’ of selected variables affects the target established for individual responses.29,30 According to the screening results, all main factors had a significant effect on the response (p < 0.05), Table 2. Thus the three factors were considered for the optimization stage. Domains for each factor were redefined and carefully re-adjusted. In case of Y1, reaction temperature was kept in the range of 25–40 °C, compared to 40–60 °C in case of Y2. Reaction time was in the range of 0–10 min for Y1 and 20–40 min for Y2. Reagent volume was kept at 2–5 mL in both cases. The following set of variables achieved the goal, maximum Y1; A = 35.0 °C, B = 5.00 mL, and C = 0 min. Implying that the CTC was formed on spot (upon mixing and at room temperature). For Y2, best conditions were A = 60.0 °C, B = 5.00 mL, and C = 40 min.
Both responses were initially described using a quadratic polynomial model. In case of Y1, normality of the assumption was not achieved. Exploiting Box–Cox normality plots showed that estimated λ value was 0.92 which can be rounded to 1, indicating that no need for transformation. However, a λ value of 0.6 showed the model best fit results. Subsequent ANOVA testing showed that formation of CTC at 452 nm would be best described using a reduced quadratic model, as follows:
|
Y10.6 = 0.35610 + 0.12791RV − 0.05624RT − 0.04661Temp × Temp + 0.04391RV × RT
| (4) |
R2: 95.78%, Adj
R2: 94.51%, standard deviation: 0.0258.
For Y2, the preliminary normality postulation was verified with no need for transformation. Formation of intra-CTC at 536 nm would be best described using the following quadratic model:
|
Y2 = 0.24891 + 0.01152Temp + 0.10368RV + 0.02323Temp × Temp − 0.01892RT × RT − 0.02145RV × RV + 0.01126Temp × RT + 0.02068Temp × RV + 0.01126RT × RV
| (5) |
R2: 96.58%, Adj
R2: 94.86%, standard deviation: 0.018.
According to eqn (4) and (5), the effect of Temp on Y1 was insignificant compared to its positive effect on Y2. On contrary, RT has a negative effect on Y1 compared to no effect on Y2. Quadratic factors had a negative effect in case of Y1. The two way interactions; however, had a positive impact on both Y1 and Y2.
In both cases, the high R2 values, in addition to the insignificant lack-of-fit, designated that the proposed models describes each individual response with high significance.
Contour (2D) plots were used to investigate the probable relationship between the three variables. As shown in Fig. 5, the X and Y variables are plotted on the X and Y axes, respectively, and the response is denoted by the contour lines.
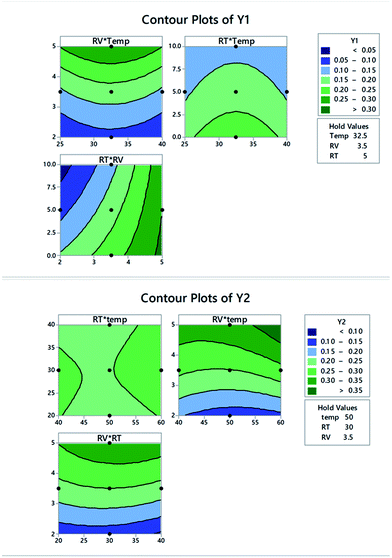 |
| Fig. 5 Two dimensional contour plots for FCC design showing Y1 and Y2 as a function of different variable interactions. | |
Results obtained from individual desirability plots, contour plots and polynomial eqn (4) and (5) are in good agreement.
3.5. Multiple response optimization
Analysing a single response was an easy exercise, where analysis of each model would simply pinpoint areas of desired results. On the other hand, simultaneous optimization of the two responses as a function of three variables was not that feasible. Two strategies were adopted for this purpose; overlaid contour plots and global desirability function. In both cases, and to account for both Y1 and Y2 at the same time, factor levels were returned to levels mentioned in Table 1.
3.5.1. Overlaid contour plots. This approach was implemented since only 2 responses are of interest. Simply, upper and lower limits for each response are defined. Contours for response bounds are then displayed vs. the two factors under study. An area that satisfies both responses is identified as the ‘feasible’ region.35,36 As shown in Fig. 6(a–c), overlaid contour plots of (a) Temp vs. RT, (b) RV vs. RT and (c) Temp vs. RV, were drawn at constant midpoint of the third variable. The white areas on Fig. 6 are the feasible regions where trade-off optimal values for both responses congregate. As shown in Fig. 6, reasonable Y1 and Y2 were obtained at any of the following combinations: (a) Temp 40–60 °C, RT 0–40 min and RV 3 mL; (b) RT 1–40 min, RV 2.5–4.6 mL and Temp of 42.5 °C; (c) RV 3–5 mL, Temp 30–60 °C and RT of 20 min. Since a single contour plot might not portrait a clear picture about the feasible area, overlaid contour plots for each 2 factors were considered at three hold values of the third variable (−1,0,+1). Moreover, these combinations were also considered for three different scenarios (maximum Y1 + minimum Y2; minimum Y1 + maximum Y2; and maximum Y1 + maximum Y2). This necessitates creation of at least 27 graphs in total, an issue which makes the process of visual observation tedious. Furthermore, the process of overlaying was not that feasible since the ideal regions for each response are a bit distant from each other according to the findings of individual response optimizations using RSM.
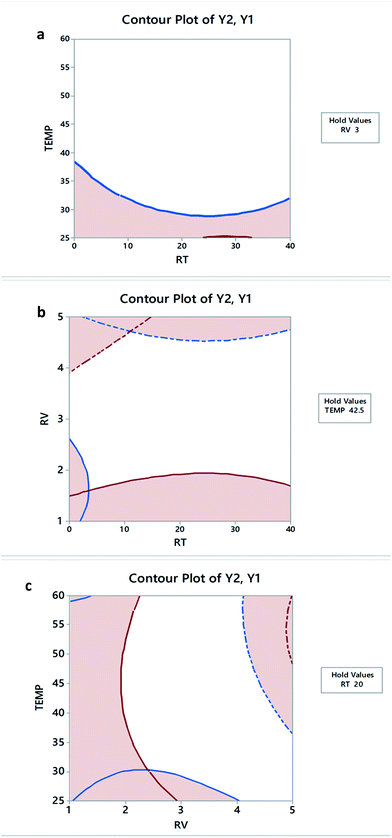 |
| Fig. 6 Overlaid contour plots for Y1 and Y2. Red and blue contour lines represent the boundaries for Y1 and Y2, respectively. The solid line (either red or blue) is the lower boundary of the response while the dashed line is the upper boundary of the same response. | |
3.5.2. Derringer function. As a multi-criteria optimization approach, Derringer function uses the individual desirability for each response to calculate the overall desirability employing the following function: |
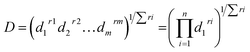 | (6) |
where D is the global desirability, d is the individual desirability, r is the importance of each response compared to the other, and m is the number of responses to be optimized.29,30 In general, the closer the value of D to 1.0000, the higher the desirability of this variable combination on the proposed response.As shown on Fig. 7, where the target was to maximize the absorbance at the two wavelengths, the composite desirability was 0.9956. This value is close to 1.0000, indicating that the selected optimal conditions are in favour of both responses. Table 3 shows the other scenarios proposed for optimizing the responses and the corresponding values of global desirability.
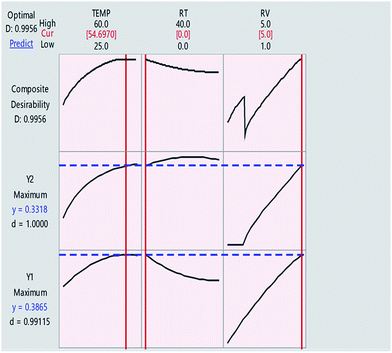 |
| Fig. 7 Desirability function plots for the FCC design. The horizontal dashed blue lines represent current response values. The vertical solid red lines show the optimal value for each variable. | |
Table 3 Variable settings and optimal composite desirability values for three different response scenarios
Response criteria |
Variable settings |
Composite desirabilitya |
Temp (°C) |
RT (min) |
RV (mL) |
Composite desirability calculated employing eqn (6). |
Case 1: maximum Y1 + maximum Y2 |
54.69 |
0 |
5.0 |
0.9956 |
Case 2: maximum Y1 + minimum Y2 |
25.00 |
0 |
4.84 |
0.9952 |
Case 3: minimum Y1 + maximum Y2 |
60.00 |
25.45 |
5.0 |
0.9355 |
3.6. Spectral and physical characteristics of the CT complexes
The formation constant (KCT) and the molar extinction coefficient (ε) of the formed CTCs were determined using Scott's linear equation.37 This equation can be written as: |
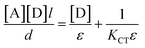 | (7) |
where [A] and [D] are the initial molar concentrations of the electron acceptor and the electron donor, respectively, l is the optical path length of the cell and d is the absorbance of the complex. This equation is applicable when [D] ≫ [A] and the complex absorbs at a wavelength where both electron acceptors and donors are completely transparent. KCT and ε were calculated from the slope and the intercept of the curve obtained from the linear plots of [A] [D] l/d against [D]. Considering the inter-CTC measured at 452 nm, and formed employing ‘Case 2’ conditions mentioned in Table 3, Scott's plot (figure not shown) was linear (r2 = 0.9984). The linearity of the Scott's plot indicated the formation of 1
:
1 D
:
A complex. Values of KCT and ε are listed in Table 4.
Table 4 Spectral and physical properties of inter- and intra-CTCs
System |
Temp (K) |
λCT (nm) |
K (L mol−1) |
ε (L mol−1 cm−1) |
ECT (eV) |
ID (eV) |
ΔG° (J mol−1) |
Complex was obtained employing ‘Case 2’ conditions. Scott's plot [eqn (7)] was used to calculate the rest of parameters. Complex was obtained employing ‘Case 3’ conditions. Inversed Benesi–Hildebrand plot [eqn (8)] was used to calculate the rest of parameters. |
Inter-CTCa |
298 |
452 |
875 |
5.55 × 102 |
2.75 |
9.14 |
−1.68 × 104 |
Intra-CTCb |
333 |
536 |
167 |
1.42 × 102 |
2.32 |
8.61 |
−1.42 × 104 |
The large value of KCT implies that the formed complex is strong.38 With some restrictions on concentrations ([D] ≫ [A]), Scott's equation was not applicable (distorted linearity) when [D] ≈ [A]. In this case, several models have been tested.39–42 The best linearity was obtained employing the following equation:42
|
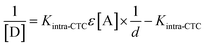 | (8) |
Plotting 1/[D] against 1/d at 536 nm ‘Case 3, intra-CTC’ resulted in a linear plot (r2 = 0.9511, figure is not shown, Table 4).
The energy of the CTC can be calculated using the following formula:43
|
ECTC = (hνCTC) = 1243.667/λCTC(nm)
| (9) |
where
λCTC is the wavelength at which CT bands absorb,
Table 4.
Using the formation constant (KCT), the standard free energy changes of CTC formation (ΔG°) can be calculated using the following equation:44
|
ΔG° = −2.303RT log KCT
| (10) |
where
R is the gas constant (8.314 J mol
−1 K
−1);
T is the temperature in Kelvin, and
KCTC is the association constant of inter-, and intra-CTC at 25, and 60 °C respectively. Calculated Δ
G° values were negative (
Table 4), indicating that the reaction is spontaneous in the favour of CT complex formation.
The ionization potential (ID) of p-Sy as a donor; defined as the energy needed to remove one electron from the highest occupied molecular orbital (HOMO), can be calculated using the following formula:45a–c
|
ID(eV) = 5.76 + 1.53 × 10−4νCTC
| (11) |
where
νCTC is the wavenumber in cm
−1 corresponding to inter- or intra-CTC band formed by the interaction of donor and acceptor,
Table 4.
3.7. Validation of the proposed method
Statistical validation of the suggested method was performed following the regulations of International Conference on Harmonization (ICH) on analytical method validation.46,47
3.7.1. Linearity and range. Under the optimized experimental conditions mentioned in Table 3, linear relationships between the [p-SY] and absorbance of the colored reaction product at both wavelengths exist. Table 5 summarizes the optical and regression data for the intra-CTC at 536 nm. The standard deviation of residuals, Sy/x, calculated as the √(SS/df) is a measure for the uncertainty in regression. The smaller the values of Sy/x, the closer the residuals are to the straight line. In other words, the lower is the uncertainty in where the regression line actually lies.
Table 5 Optical and regression characteristics for the determination of p-SY using the proposed procedure. Case 3 conditions mentioned in Table 3, were applied
Parameter |
Value |
Parameter |
Value |
Regression equation: A = bC + a, where A is the absorbance, C is concentration in mM, a is intercept, b is slope, Sb = SD of slope, ±tSb = confidence limit for slope, Sa = SD of intercept, ±tSa = confidence limit for intercept, Sy/x = SD of the regression, SS is sum of squares. LOD = limit of detection, LOQ = limit of quantification, r2 = coefficient of determination. |
Wavelength, λmax (nm) |
536 |
Slope (b) |
0.2111 |
Linear rangea, (mM) |
2.50–5.00 |
Intercept (a) |
−0.3860 |
Sb |
0.00538 |
r2 |
0.9974 |
±tSb |
0.00431 |
LODb (mM) |
0.1599 |
Sa |
0.02069 |
LOQb (mM) |
0.5330 |
±tSa |
0.01656 |
Residual SS |
0.0005 |
Sy/x |
0.01125 |
Regression SS |
0.1950 |
Another measure of goodness-of-fit is the high value for coefficient of determination, r2 = 0.9974. Mean recoveries for application of the optimized procedure with the corresponding SD, and RSD are shown in Table 6.
Table 6 Application of the proposed procedure for determination of p-SY in bulk powder. Measurement was done at 536 nm applying ‘Case 3’ conditions listed in Table 3
Amount taken (mM) |
Amount found (mM) |
% recoverya |
Average of 3 determinations. |
2.50 |
2.49 |
98.80 |
3.00 |
2.95 |
100.67 |
3.50 |
3.53 |
101.50 |
4.00 |
4.06 |
100.85 |
4.50 |
4.53 |
98.33 |
5.00 |
4.94 |
99.60 |
Mean ± SD |
|
99.96 ± 1.249 |
RSD |
|
1.249 |
3.7.2. Limits of detection and quantification. As shown in Table 5, LOD and LOQ for determination of p-SY using the proposed procedure are small enough to indicate the sensitivity of the proposed procedure.
3.7.3. Accuracy and precision. The accuracy and precision of the optimized procedure was determined at 95.0% CI. Assessment of accuracy and the within-day precision was performed by measuring the absorbance of the reaction product at three concentration levels of p-SY in 3–5 replicates on the same day (intra-day, Table 7). On the other hand, accuracy and intermediate precision were measured by assessing the same concentrations on three subsequent days (inter-day, Table 7). Results obtained indicate good accuracy and high precision of the proposed procedure as indicated by low values of Er, and RSD percentages respectively.
Table 7 Inter-day and intra-day accuracy and precision for the determination of p-SY using the proposed conditions of ‘Case 3’ at 536 nm
Concentration (mM) |
Mean % recoverya ± SD |
RSD (%) |
Er (%) |
Mean ± SD of 3 determinations. The inter-day (n = 3), average of three concentrations of p-SY repeated three times in three successive days. The intra-day (n = 3), average of three concentrations of p-SY repeated three times within the same day. |
Inter-day precision and accuracyb |
2.50 |
98.40 ± 0.400 |
0.406 |
1.601 |
3.50 |
100.76 ± 1.003 |
0.996 |
−0.762 |
5.00 |
99.47 ± 0.833 |
0.837 |
0.533 |
![[thin space (1/6-em)]](https://www.rsc.org/images/entities/char_2009.gif) |
Intra-day precision and accuracyc |
2.50 |
99.20 ± 1.058 |
1.066 |
0.800 |
3.50 |
99.14 ± 2.157 |
2.175 |
0.857 |
5.00 |
100.20 ± 0.800 |
0.798 |
−0.200 |
3.7.4. Robustness. System robustness was measured by employing minor variations in experimental conditions and noticing their effect on the anticipated response. The tested factors were those proved to be significant by the optimized factorial design, in addition to the wavelength. Variations were done as follows: wavelength ± 5 nm, temperature ± 5 °C, reaction time ± 5 min, and reagent volume ± 0.3 mL. The impact of these deliberate changes on both responses was minimal with RSD% value that doesn't exceed 0.659% in case of Y1 and 0.702% in case of Y2.It is noteworthy to mention that the impact of factorial change on the target response can be also tested by moving the red lines showing the optimal value for each variable within the suggested range and noticing the effect on composite desirability, Fig. 7.
4. Conclusion
Charge transfer interaction between p-SY as an electron donor and p-CHL as an acceptor was studied employing a full factorial design (FFD) followed by a face-cantered central composite design (FCCCD). The two steps were statistically validated using ANOVA. Two types of responses were measured spectrophotometrically in the current investigation, inter- and intra-molecular CT complexes at two wavelengths. Formation constant was determined at both wavelengths. Multi-response optimization was performed using two approaches; graphical utilizing the overlaid contour plots, and mathematical using the Derringer function. Results obtained from both approaches indicate that the proposed paradigm is appropriate for detecting the response without running the experiment. In addition, employing the multivariate technique, and in comparison to the traditional one-variable-at-time approach, is significantly reflected on the method performance in terms of time, and resources consumption. It is noteworthy to mention that the majority of procedures reported for SY determination are based on HPLC, and GC, which are sophisticated and tedious in comparison to the current approach, especially after usage of DOE. Validation of the current method was executed using the ICH strategies, and results obtained reinforce the legitimacy of the optimized procedure.
References
- S. C. Sweetman, Martindale: The Extra Pharmacopeia-The Complete Drug Reference, Pharmaceutical Press, 2007 Search PubMed.
- H. Wagner and G. Ulrich-Merzenich, Phytomedicine, 2009, 16, 97–110 CrossRef CAS PubMed.
- R. C. Baselt, Disposition of Toxic Drugs and Chemicals in Man, Biomedical Publications, Foster City, California, 8th edn, 2008, pp. 1471–1472, ISBN 978-0-9626523-7-0 Search PubMed.
- B. A. Arias and L. Ramón-Laca, J. Ethnopharmacol., 2005, 97, 89–95 CrossRef PubMed.
- A. Fugh-Berman and A. Myers, Exp. Biol. Med., 2004, 229, 698–704 CAS.
- S. Haaz, K. R. Fontaine, G. Cutter, N. Limdi, S. Perumean-Chaney and D. B. Allison, Obes. Rev., 2006, 7, 79–88 CrossRef CAS PubMed.
- Y. Liu, F. Xu, Z. Zhang, C. Yang, R. Song and Y. Tian, J. Chromatogr. Sci., 2009, 47, 925–930 CAS.
- B. T. Schaneberg and I. A. Khan, Pharmazie, 2004, 59, 819–8223 CAS.
- F. Tang, L. Tao, X. Luo, L. Ding, M. Guo, L. Nie and S. Yao, J. Chromatogr. A, 2006, 1125, 182–188 CrossRef CAS PubMed.
- B. Avula, S. K. Upparapalli and A. Navarrete, J. AOAC Int., 2005, 88, 1593–1606 CAS.
- L. G. Rossato, P. G. de Pinho, R. Silva, H. Carmo, F. Carvalho, Md. L. Bastos, V. M. Costa and F. Remião, J. Pharm. Biomed. Anal., 2010, 52, 721–726 CrossRef PubMed.
- L. G. Rossato, V. M. Costa, P. G. De Pinho, R. Silva, F. Carvalho, H. Carmo, M. D. L. Bastos and F. Remião, Toxicol. Lett., 2010, 196, S293 CrossRef.
- A. S. Andrade, G. C. Schmitt, L. G. Rossato, D. Russowsky and R. P. Limberger, Chromatographia, 2009, 69, S225–S229 CAS.
- C. Balraj, A. Satheshkumar, K. Ganesh and K. P. Elango, Spectrochim. Acta, Part A, 2013, 114, 256–266 CrossRef CAS PubMed.
- Š. Budzák, P. Mach, G. Juhász, M. Medved' and O. Kysel', Comput. Theor. Chem., 2015, 1051, 129–136 CrossRef.
- O. R. Shehab and A. M. Mansour, J. Mol. Struct., 2015, 1093, 186–194 CrossRef CAS.
- M. Schäferling, T. Lang and A. Schnettelker, J. Lumin., 2014, 154, 458–464 CrossRef.
- J.-M. Lü, S. V. Rosokha, I. S. Neretin and J. K. Kochi, J. Am. Chem. Soc., 2006, 128, 16708–16719 CrossRef PubMed.
- A. Saha and A. K. Mukherjee, Spectrochim. Acta, Part A, 2004, 60, 1731–1737 CrossRef PubMed.
- G. Powls, Free Radical Biol. Med., 1989, 6, 63–101 CrossRef.
- H. Nohl, W. Jordan and R. J. Youngman, Adv. Free Radical Biol. Med., 1986, 2, 211–279 CrossRef CAS.
- X.-Q. Zhu and C.-H. Wang, J. Org. Chem., 2010, 75, 5037–5047 CrossRef CAS PubMed.
- R. Leardi, Anal. Chim. Acta, 2009, 652, 161–172 CrossRef CAS PubMed.
- M. M. De Zan, C. M. Teglia, J. C. Robles and H. C. Goicoechea, Talanta, 2011, 85, 142–150 CrossRef CAS PubMed.
- A. A. Eiroa, P. Diévart and P. Dagaut, Talanta, 2010, 81, 265–274 CrossRef PubMed.
- Minitab 17 Statistical Software (2010). [Computer software], State College, PA: Minitab, Inc., www.minitab.com Search PubMed.
- M. A. Islam, M. R. Alam and M. O. Hannan, Composites, Part B, 2012, 43, 861–868 CrossRef CAS.
- S. Pal and S. K. Gauri, Qual. Eng., 2010, 22, 336–350 CrossRef.
- R. H. Myers and D. C. Montgomery, Response Surface Methodology: Process and Product Optimization Using Designed Experiments (Wiley Series in Probability and Statistics), Wiley, New York, 2009 Search PubMed.
- G. Derringer and R. Suich, J. Qual. Tech., 1980, 12, 214–219 Search PubMed.
- R. E. Bruns, I. S. Scarminio and B. B. Neto, Data Handling in Science and Technology, Statistical Design—Chemometrics, Elsevier, Amsterdam, 2006, vol. 25 Search PubMed.
- L. V. Candioti, M. M. De Zan, M. S. Cámara and H. C. Goicoechea, Talanta, 2014, 124, 123–138 CrossRef PubMed.
- G. E. P. Box and D. R. Cox, J. Roy. Stat. Soc. B, 1964, 26(2), 211–252 Search PubMed.
- T. W. Anderson and D. A. Darling, J. Am. Stat. Assoc., 1954, 49(268), 765–769 CrossRef.
- E. Sivertsen, F. Bjerke, T. Almøy, V. Segtnan and T. Næs, Chemom. Intell. Lab. Syst., 2007, 85, 110–118 CrossRef CAS.
- M. R. Hasniyati, H. Zuhailawati, R. Sivakumar and B. K. Dhindaw, Surf. Coat. Technol., 2015, 280, 250–255 CrossRef CAS.
- R. L. Scott, Recl. Trav. Chim. Pays-Bas, 1956, 75, 787 CrossRef CAS.
- M. Pandeeswaran and K. P. Elango, Spectrochim. Acta, Part A, 2009, 72, 789–795 CrossRef CAS PubMed.
- M. T. El-Haty, Spectrochim. Acta, Part A, 1991, 47, 1017–1021 CrossRef.
- M. M. Ayad, Spectrochim. Acta, Part A, 1994, 50, 671–676 CrossRef.
- N. J. Rose and R. S. Drago, J. Am. Chem. Soc., 1959, 81, 6138–6141 CrossRef CAS.
- G. B. Raju, Ph. D. thesis, Pondicheny University, Pondicheny, 2000.
- R. Rathore, S. V. Lindeman and J. K. Kochi, J. Am. Chem. Soc., 1997, 119, 9393–9404 CrossRef CAS.
- A. N. Martin, J. Swarbrick and A. Cammarata, Physical Pharmacy, Lee and Febiger, Philadelphia, PA, 3rd edn, 1969, p. 344 Search PubMed.
-
(a) G. G. Aloisi and S. Pignataro, J. Chem. Soc., Faraday Trans., 1972, 69, 534 RSC;
(b) G. Briegleb and J. Czekalla, Angew. Chem., 1960, 72, 401 CrossRef CAS;
(c) G. Briegleb, Angew. Chem., 1964, 76, 326 CrossRef CAS.
- ICH, Q2A (R1), Validation of Analytical Procedures: Text and Methodology, International Conference on Harmonisation, November 2005 Search PubMed.
- J. N. Miller and J. C. Miller, Statistics and Chemometrics for Analytical Chemistry, Prentice Hall/Pearson, 2010 Search PubMed.
|
This journal is © The Royal Society of Chemistry 2016 |
Click here to see how this site uses Cookies. View our privacy policy here.